DOI:
10.1039/D1SM00251A
(Paper)
Soft Matter, 2021,
17, 5747-5755
Assembly of cellulose nanocrystals and clay nanoplatelets studied by time-resolved X-ray scattering†
Received
17th February 2021
, Accepted 18th May 2021
First published on 18th May 2021
Abstract
Time-resolved small-angle X-ray scattering (SAXS) was used to probe the assembly of cellulose nanocrystals (CNC) and montmorillonite (MNT) over a wide concentration range in aqueous levitating droplets. Analysis of the SAXS curves of the one-component and mixed dispersions shows that co-assembly of rod-like CNC and MNT nanoplatelets is dominated by the interactions between the dispersed CNC particles and that MNT promotes gelation and assembly of CNC, which occurred at lower total volume fractions in the CNC:MNT than in the CNC-only dispersions. The CNC dispersions displayed a d ∝ ϕ−1/2 scaling and a low-q power-law exponent of 2.0–2.2 for volume fractions up to 35%, which indicates that liquid crystal assembly co-exists and competes with gelation.
Introduction
Nanostructured materials based on nanoscale building blocks extracted from renewable, non-food sources1,2 have the potential to mitigate growing concerns about pollution and the depletion of fossil-based resources3,4 within numerous industrially important sectors.5
Cellulose nanomaterials (CNM) can be isolated from naturally abundant and renewable sources, e.g. wood, cotton, bacteria.6,7 The high strength, low density and chemical versatility make CNM excellent candidates for functional nanomaterials and nanocomposites.8–10 Cellulose nanocrystals (CNC) obtained by sulfuric acid hydrolysis are rigid rod-like particles that can form chiral nematic liquid-crystalline phases in aqueous dispersions11 and yield materials with high specific mechanical strength and toughness12 and useful optical13–15 properties, making CNC particularly interesting for a range of high-performance applications.16
Nature provides many examples of multiscale organic–inorganic hybrid and composite materials with intricate structures and impressive properties;17e.g. mechanically strong and tough vertebrate teeth,18 diatoms with photonic crystal behaviour19 or magnetosomes with directional magnetic properties.20
CNM-based composite and hybrid coatings, films and aerogels containing various inorganic components can have favourable mechanical properties.21–25 Furthermore, materials consisting of CNM with nanoclays, especially montmorillonite (MNT), have displayed combinations of high strength with heat tolerance,26 fire retardancy,27 dye adsorption,28 and low oxygen29 and water–vapour30 permeability. While self-assembly has been studied in single-component CNC and MNT dispersions,11,31–33 including, in the case of CNC, in confined geometries such as sessile,34 emulsion35 and levitating36 droplets, studies of multicomponent CNC:MNT systems are sparse.28,37 Exploring and probing how CNC and inorganic nanoparticles such as clays assemble in aqueous dispersions is important for the development of processing routes and new classes of sustainable materials.
In the present work, aqueous dispersions of CNC and MNT in acoustically levitating and slowly evaporating droplets were investigated using time-resolved small-angle X-ray scattering (SAXS). Analysis of the SAXS patterns of the CNC:MNT composite mixtures have been compared to the single-component dispersions to elucidate the co-assembly of CNC and MNT.
Materials and methods
Preparation of cellulose nanocrystals
Cellulose nanocrystals (CNC) were obtained by sulfuric acid hydrolysis of sulfite softwood cellulose dry sheets (Aditya Birla Domsjö, Sweden) by dispersing 100 g of wood pulp under mechanical stirring in 1 L of 64% sulfuric acid at 45 °C. The hydrolysis reaction was quenched by adding approximately 10 L of deionized water after 45 minutes. After approximately three hours, the dispersion was centrifuged twice at 6000 G for 10 min, and the sediment was collected and dialyzed against deionized water for seven days, using dialysis membranes with a molecular weight cut-off of 14
000 Da (Sigma-Aldrich). After the dialysis, the dispersion was sonicated for 30 min with an ultrasonic probe at 60% energy output (QSonica Q500, with a 13 mm wide titanium probe), and filtered through a glass filter. The resulting CNC dispersion (with a concentration around 2 wt%) was further purified with an additional centrifugation protocol: the dispersion was diluted to 0.2 wt%, centrifuged at 450 G for ten minutes and 2500 G for 10 minutes, then up-concentrated again via rotary evaporation.
Preparation of clay dispersions
Aqueous dispersions of sodium montmorillonite (MNT, Cloisite Na+, BYK Additives) with a concentration of 10 g L−1 were prepared by stirring using an Ultra-Turrax and dialyzed against MilliQ water for seven days using dialysis membranes with a molecular weight cut-off of 14
000 Da (Sigma-Aldrich).
Particle and dispersion characterization
The CNC thicknesses and lengths were determined via Atomic Force Microscopy (AFM, Dimension 3100, Bruker, USA). Tapping mode images of CNC deposited onto freshly cleaved mica were analysed by the software Nanoscope Analysis to determine the thicknesses and lengths of 100 particles.
Transmission electron microscopy (TEM) (JEM-2100F) with an accelerating voltage of 200 kV was used to image MNT deposited onto a carbon-coated copper TEM grid. The images were processed using the Gatan Inc. software.
The charge density of the CNCs was determined by automatic conductometric titration using the 888 titrando module of Metrohm, combined with an 856 conductivity module and 800 dosino dosing device. Based on a method described elsewhere,38 3 mL of CNC dispersion were diluted with 120 g of 1 mM NaCl solution before titration. The titrant was a 1.23 mM solution of NaOH. The sulfur content was estimated to be 0.82 ± 0.12%S.
Zeta potential measurements were performed using a Zetasizer Nano ZS (Malvern Instruments Ltd, UK), equipped with a 633 nm laser and backscatter detector (at an angle of 173°). The measurements were performed on dispersions diluted with deionized water, at a total concentration of 0.05 wt%, and sonicated for ten minutes (USC500TH, VWR International). For each sample, the final results were averaged from the data obtained from three measurements of 60 to 100 runs each after 60 seconds of equilibration time. The obtained zeta potential values were −60 ± 2 mV for the CNC and −35 ± 1 mV for the MNT.
Thermogravimetric analysis (TGA, TA Instruments Discovery) was used to determine the weight fraction of CNC and MNT in the mother stock dispersions used for the preparation of the dispersions used for droplet levitation. Alternatively, simple mass comparison, after water evaporation in an oven at 105 °C for 3 h, was used to estimate weight fractions.
Drop levitation
Droplets of aqueous CNC and/or clay dispersions were placed into the acoustic field of a levitator (TEC5, model 13K11, f0 = 100 kHz). The droplets, with initial volumes of 2 to 3 μL, were stabilized by tuning the amplitude of the acoustic stationary field and the distance between the sonotrode and reflector. The droplet environment was surrounded by an acrylic glass chamber with four openings to allow the X-ray beam to pass through, and to allow the video recording of the droplets using a USB camera (1.3 Mpixels, 200× magnification). The influence of internal flow and droplet rotation was assumed to be negligible, as a previous study39 showed identical assembled structures formed in levitating drops and by drop casting. The processing of the resulting videos was performed using ImageJ software.40
Small-angle X-ray scattering (SAXS)
In situ small-angle X-ray scattering (SAXS) experiments were carried out at the ID02 beamline at the European Synchrotron Radiation Facility (ESRF), Grenoble, France. The droplets were irradiated by a rectangular beam with a spot size of 60 × 250 μm2 and a wavelength of 1 Å. The data was recorded by a CCD detector Rayonix MX-170HS covering the following scattering vector (q) range: 0.0035 < q < 0.374 Å−1. Each frame was acquired with an exposure time of 30 ms, leading to time-resolved data with a resolution of 2 s. The collected data was reduced by a data reduction routine from the SPEC acquisition program. The reduced data is presented as angular averages of the scattering patterns (Fig. S1, ESI†). A few patterns showed anisotropic features, which is further discussed in the results section. The SAXS data was background corrected using the scattering of a water droplet of equivalent size.
SAXS peak and pattern analysis
To determine the centre of the structural peaks observed in the CNC SAXS curves, the second derivative of the signal was smoothed using a second order Savitzky–Golay filter.41 The sharper negative peaks in the second derivative, compared to the corresponding main signal peaks (Fig. S2, ESI†), were fitted with Gaussian functions where the baseline was fixed at a value of 0, except for the highest volume fractions where it had to be set to slightly higher values due to the peak data points having positive values. The data range chosen for the fittings included as many points as possible below the set deadline, avoiding non-converging fitting algorithms and adjusted R2 factors lower than 0.95. The information extracted from the fittings consists of both the qmax values (peak centres) and the variance, from which the vertical error bars are estimated.
The SAXS patterns were fitted using the Sasview software (version 5.0.2). SAXS signals from both CNC and MNT systems with low volume fractions were fitted using a parallelepiped model.42 The scattering length densities of the CNC and MNT were 1.446 × 10−5 and 1.937 × 10−5 Å−2, respectively. The dimensions of the CNC and MNT particles could be estimated using the SAXS data of dilute dispersions. The CNC length was near or exceeding the available q range and was fixed to 160 nm using the estimates provided by AFM measurements. The polydispersity was not accounted for in the fitting process. The SAXS curves for the dilute CNC
:
MNT 5
:
1 and CNC
:
MNT 2
:
1 mixed dispersions were fitted using a sum of two parallelepiped models with dimensional parameters obtained from the analysis of the dilute CNC and MNT dispersions.
The chi-square (χ2), which is a measure of the quality of the fit, is calculated as defined in eqn (1):43
| 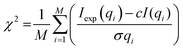 | (1) |
where
Iexp(
qi) and
I(
qi) are the scattering intensity from experimental measurement and theoretical calculation, respectively,
M is the number of data points,
c corresponds to the scale factor and
σ is the error of the experimental data.
Results and discussion
The assembly of CNC, MNT and CNC:MNT were probed by SAXS in shrinking aqueous droplets as the water slowly evaporated over twenty to forty minutes (the measurement setup is illustrated in Fig. 1a). The evaporation-driven increase in the nanoparticle volume fraction (ϕ) allowed for a wide range of volume fractions to be studied by time-resolved SAXS measurements of a single shrinking droplet (see ESI† for more information).
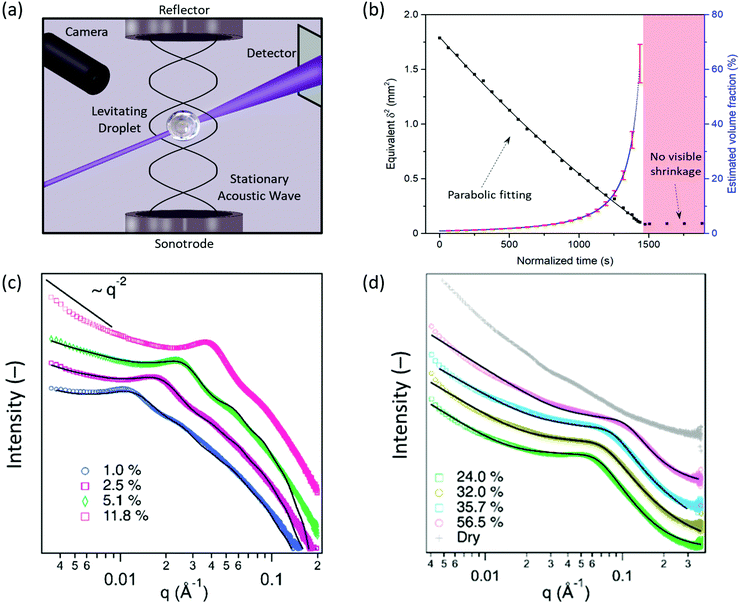 |
| Fig. 1 Time-resolved volume fraction and scattering patterns of aqueous CNC levitating droplet dispersions. (a) Schematic illustration of the time-resolved SAXS measurements of acoustically levitating droplets. (b) Temporal evolution of the equivalent square diameter (δ2, the black squares correspond to measurements, the black line corresponds to a parabolic fit), and estimated volume fraction of the droplets (blue line, error bars calculated from single measurements in red). Log–log visualization of time-resolved SAXS data of CNC aqueous dispersions at; (c) low/intermediate volume fractions (1.0–11.8%) and; (d) high volume fractions (24.0–56.5%), and in a “dry” state. The black lines correspond to fits using; (c) a parallelepiped form factor and a hard-sphere structure factor and; (d) the “broad-peak” function (see eqn (1)), respectively. | |
Assembly of CNC
The assembly of negatively charged CNC with an average thickness of 43 ± 13 Å and an average length of 1540 ± 440 Å (Fig. S3, ESI†), dispersed in aqueous media at pH = 3, was studied in levitating droplets over a wide volume fraction range, from 1% up to about 60% (Fig. 1b). The volume fraction values above 60% may correspond to the semi-dry regime and were thus not analysed further.
SAXS patterns (Fig. 1c) of CNC dispersions with lower volume fractions (1.0, 2.5 and 5.1%) were fitted using a parallelepiped form factor and a hard sphere structure factor. The best fitted results corresponded to CNC particles with a cross-section of (34 ± 4) × (181 ± 15) Å2. The thickness of the CNC particles derived from SAXS (34 Å) is slightly lower than that obtained from the AFM images (43 Å) (Fig. S3, ESI†). The absence of a Guinier region (a plateau in the low-q range) in the SAXS patterns indicates that the characteristic dimension of the CNC particles (here, the length of the particle) is at least partially outside of the investigated q range. Furthermore, the low-q slope (between 0.0035 and 0.008 Å−1) gradually diverged from a q−1 dependence, which is characteristic of rod-like objects, to higher negative exponents as the volume fraction increased; this trend is attributed to the transition from essentially non-interacting to strongly interacting CNC particles with increasing volume fraction in the shrinking levitating droplet.44
The 2D SAXS patterns (Fig. S1, ESI†) for volume fractions above 3% were anisotropic, which is commonly observed in systems with localized nematic arrangements.45
At volume fractions above 24.0%, the reduced SAXS data was fitted to a “broad-peak” function (Fig. 1d), see eqn (2):46,47
|  | (2) |
The first term corresponds to low-
q correlations extending to distances larger than hundreds of Å,
48 with a low-
q power-law exponent (also called Porod exponent),
n, and a low-
q scale factor,
A. The second term is a Lorentzian-type function that describes the high-
q broad peak corresponding to length scales from 10 to 100 Å, with a Lorentzian exponent,
m, and a Lorentzian scale factor,
B, where
qmax is the peak centre and,
ξ, called the Lorentzian screening length, can be interpreted as the mesh size in case of a gel-like network.
49
The fit yielded a low-q power-law exponent of ca. 2.2 (Table 1) that is characteristic of gel-like systems.50,51 The Lorentzian screening length, or mesh size, decreased with increasing volume fraction, from 29 to 20 Å for volume fractions between 24.0 and 56.5%. The decrease in mesh size is probably related to a compression of the assembled network. The scattering pattern collected for the CNC droplet (Fig. 1d) in the semi-dry or “dry” stage (when the droplet volume became constant as shown in Fig. 1b) displayed two weak but separated bumps at around 0.020 and 0.040 Å−1, which could be indicative of a short-range ordering. The SAXS pattern for the “dry” beads also displayed a power-law dependence of ∼q−4 in the Guinier (low-q) region, which suggests that the assembled structures are larger than what the investigated q range can probe, and a high-q region dependence of ∼q−2.3, which is indicative of mass fractal structures. These features differ from the CNC dispersions reported by Rodrigues et al., where the observed power-law dependence of ∼q−3.7 over the investigated q-range was attributed to a disordered structure.52
Table 1 Fitting parameters obtained with the broad-peak model for the aqueous CNC droplet
Volume fraction (%) |
Low-q power-law exponent, n |
Lorentzian exponent, m |
Mesh size, ξ (Å) |
24.0 ± 1.9 |
2.2 ± 0.1 |
3.4 ± 0.1 |
29 ± 2 |
32.0 ± 2.8 |
2.1 ± 0.1 |
3.2 ± 0.1 |
26 ± 2 |
35.7 ± 3.2 |
2.0 ± 0.1 |
3.5 ± 0.1 |
23 ± 1 |
56.5 ± 6.2 |
2.2 ± 0.1 |
3.2 ± 0.1 |
20 ± 1 |
The position of the main SAXS intensity peak shifted towards higher q values with increasing volume fraction (Fig. 1c and d), which implies that the centre-to-centre separation distances between the CNC particles decreased. These distances, also commonly called d-spacings (d), were calculated using the qmax values obtained from the intensity peak analysis viaeqn (3):
|  | (3) |
The peaks of the structure factors, which could be obtained by dividing the intensity of the experimental SAXS patterns by the fitted form factor (black line in Fig. S4a, ESI
†), were used as a comparison (Fig. S4b, ESI
†). The
d-spacings decreased from 570 Å at
ϕ = 1% to 63 Å at
ϕ ≈ 60%, (
Fig. 2a). A power-law scaling of
d ∝
ϕ−1/2 was observed over almost the entire volume fraction range, up to around
ϕ = 35%. The scaling exponent increased at very high volume fractions, approaching
d ∝
ϕ−1. Power-law scaling with a gradually changing exponent from
d ∝
ϕ−1/3 (characteristic for isotropic contraction of randomly oriented particles) to
d ∝
ϕ−1 (attributed to unidimensional consolidation) was previously observed by Liu
et al.,
36 but over a more narrow volume fraction range. Here, the extended region with a
d ∝
ϕ−1/2 scaling, which is characteristic of rod-like colloids in nematic arrangement,
53 indicates that there is a competition between the assembly of liquid crystalline (anisotropic) phases and gel formation over a wide volume fraction range. The peak analysis from the structure factor curves (Fig. S4b, ESI
†) corroborated the
d ∝
ϕ−1/2 scaling up to around 20% (Fig. S4c, ESI
†). The scaling exponent then increased at volume fractions above 20%, which is in line with the volume fraction at which close-packed networks were first observed (24%). At higher volume fractions, it was not possible to determine a single structure peak centre.
Fig. 2b shows that the CNC
d-spacings varied linearly with inverse volume fraction, which has been suggested to represent an estimate of the average thickness of anisotropic nanoparticles, such as rods and platelets.
32,54,55 The estimated thickness obtained from the slope, 31 Å, is in good agreement with the average CNC thickness obtained by fitting the low-volume fraction SAXS patterns (34 Å).
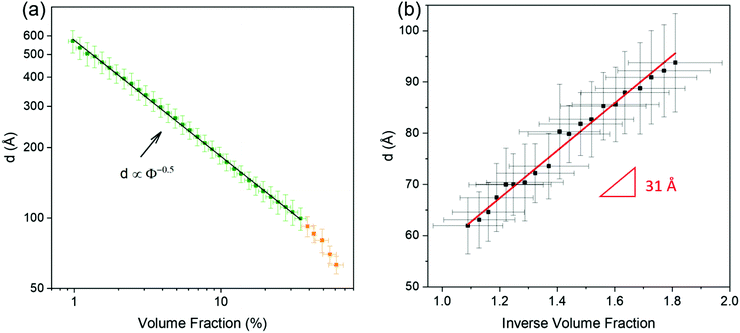 |
| Fig. 2 Assembly of CNC in aqueous dispersions. (a) Centre-to-centre separation distances between CNC particles, d, versus volume fraction, highlighting the logarithmic d ∝ ϕ−1/2 scaling. (b) Centre-to-centre separation distances between CNC particles, d, versus inverse volume fraction, with the slope of the linear fit indicated on the graph. | |
Assembly of MNT platelets
The average diameter of the MNT platelets was estimated to be 1220 ± 650 Å by TEM with average x–y dimensions of 1510 × 930 Å2 (Fig. S5, ESI†). The MNT platelets are very thin and their thickness is usually close to 10 Å55,56 which corresponds to one Al octahedral layer surrounded by two Si tetrahedral layers (Fig. S6, ESI†). Fitting the SAXS pattern of a MNT aqueous solution droplet at a low volume fraction of 0.4% (Fig. S7, ESI†) to a parallelepiped model42 yielded a thickness of 9.6 Å and an average plate size of (1319 ± 130) × (985 ± 120) Å2, which is in good agreement with the TEM measurements.
The SAXS curves at low volume fractions (ϕ = 0.4 and 1.8%) and high volume fractions (ϕ = 35.0%) of the MNT dispersions displayed a power-law behaviour with exponents of −2.2, −2.1, and −2.7, respectively (Fig. 3a). The close to q−2 behaviour at low volume fractions can be related to the bidimensional (plate-like) morphology of the scattering objects,57 and confirms that the MNT particles were well dispersed within the droplet at the start of the measurement. At intermediate volume fractions (ϕ = 4.3%) the scattering curve deviated from power-law behaviour in the medium-q range.
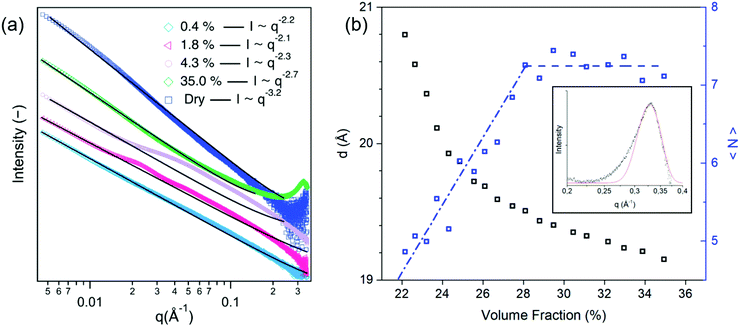 |
| Fig. 3 Aqueous dispersions of MNT platelets. (a) SAXS curves and power-law fitting (black lines) of MNT dispersions at volume fractions between 0.4 and 35.0%. (b) Repeat distances between MNT platelets (d, black circles) and average number of platelets per tactoid (〈n〉, red squares) as a function of MNT volume fraction. Inset: Correlation peak (black) and corresponding Gaussian fit (red) for a volume fraction of 34.9%. | |
Kratky plots (q2 × intensity against q) (Fig. S8, ESI†) highlight that a diffuse peak, which is indicative of structures with short range order,32 dominated the SAXS patterns at intermediate volume fractions (approximately between 3 and 10%), but disappeared at higher volume fractions. Similarly, the 2D scattering patterns displayed anisotropy at these intermediate volume fractions, but not at higher volume fractions. The increasing intensity of the high-q correlation peak (q = 0.33 Å−1) with increasing volume fraction suggests that the MNT formed a stacked structure, probably driven by attractive van der Waals interactions.58
The correlation peak that corresponds to repeat distances decreased to approximately 19 Å at the highest measurable volume fractions, as shown in Fig. 3b. Similar values have previously been reported and attributed to 10 Å thick aqueous layers between the MNT particles in the stacked structure.57,59 The average number of MNT platelets per tactoid, 〈N〉, can be estimated by the Scherrer relation (eqn (4)):55
|  | (4) |
where FWHM is the full-width at half-maximum of the Gaussian fitted correlation peak and
qmax is obtained from SAXS intensity curves.
Fig. 3b shows that 〈
N〉 increased with increasing volume fraction up to a value slightly higher than 7 at
ϕ = 30%. The obtained 〈
N〉 values are in agreement with reported values for analogous plate-like clay systems.
60,61
The SAXS pattern of the “dry” bead (Fig. 3a) displayed no correlation peak and had a power-law exponent (∼q−3.2) that persisted over a very wide q range. Such a power law behaviour cannot be related to any of the well-defined mesoscale structures,62 but is possibly related to the formation of solvent-rich and -poor regions within the levitating droplet, which has been observed in both dispersions63 and solutions.58,64
Co-Assembly of mixed CNC:MNT dispersions
The co-assembly of CNC
:
MNT mixed dispersions with mixing ratios of 5
:
1 and 2
:
1 (the MNT content being identical in both dispersions) was studied by SAXS in levitating droplets with initial volume fractions of 1.8 and 1.6% for the 5
:
1 and 2
:
1 mixtures, respectively. The SAXS patterns for the dilute dispersions could be fitted using a model for two different parallelepipeds with a scale factor (Fig. 4a and b). The SAXS curves displayed broad peaks at all the investigated volume fractions, which suggests that the dispersed particles were interacting even at the lowest measured volume fractions. The obtained scale factors corresponded to a ratio of 5.46 for the 5
:
1 mixture and 1.86 for the 2
:
1 mixture, which correlates relatively well with the mixing ratios of CNC and MNT.
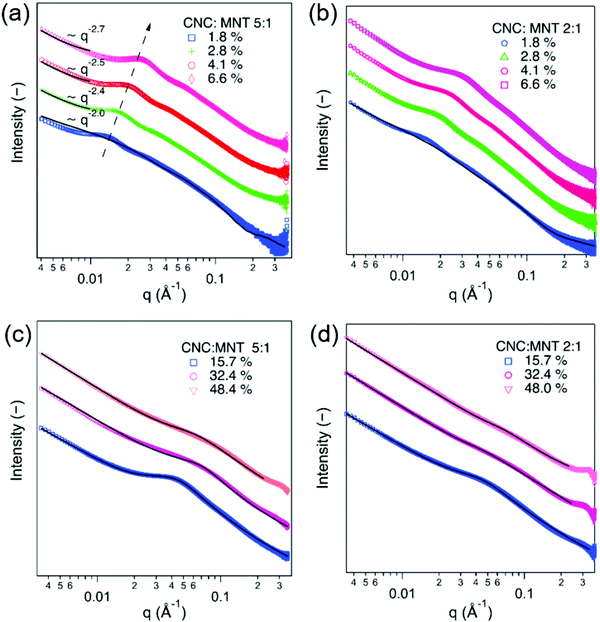 |
| Fig. 4 SAXS curves with combined parallelepiped and broad peak fitting for CNC:MNT mixtures. SAXS curves and corresponding combined parallelepiped fits (black lines) for lower volume fractions of CNC:MNT; (a) 5 : 1 mixtures and; (b) 2 : 1 mixtures. SAXS curves and corresponding broad peak fits (black lines) for higher volume fractions of CNC:MNT; (c) 5 : 1 mixtures and; (d) 2 : 1 mixtures. | |
The SAXS curves for both of the investigated mixed CNC:MNT dispersions displayed a structural peak that gradually increased in intensity and moved to higher q values when the total volume fraction increased (Fig. 4a and b). The intensity of the scattering peak for the 5
:
1 mixture was significantly stronger than the 2
:
1 mixture, which suggests that the structural peak is dominated by the interactions between the dispersed CNC particles. In fact, the characteristics of the concentration-dependent structural peak for the CNC:MNT mixtures resemble the scattering behaviour of the pure CNC dispersions (Fig. 1c). Unlike for the pure CNC dispersions, the 2D scattering patterns corresponding to the mixed dispersions were isotropic at all volume fractions. The isotropic scattering patterns can be related to the higher scattering length density of MNT compared to CNC, which could obscure nematic CNC arrangements.
The SAXS patterns of the CNC:MMT dispersions of a total volume fraction of 15.7% could be fitted with the broad-peak model (Fig. 4c and d), with a mesh size of 47 Å for the 5
:
1 mixture and 39 Å for the 2
:
1 mixture (Table 2). The mesh sizes of 35 Å for the 2
:
1 mixture and 29 Å for the 5
:
1 mixture at 32.4% (Table 2) were slightly larger than the 26 Å obtained for the pure CNC dispersion (Table 1), which suggests that an increasing MNT content inhibits the formation of tightly packed CNC domains. At very high total volume fractions, e.g. at 48.4%, the peak became very broad, which indicates a disordered structure.
Table 2 Fitting parameters obtained with the broad-peak model for CNC:MNT mixtures at high volume fractions
Dispersion mixture |
Volume fraction (%) |
Low-q power-law exponent, n |
Lorentzian exponent, m |
Mesh size, ξ (Å) |
CNC : MNT = 5 : 1 |
15.7 ± 0.3 |
2.1 ± 0.1 |
2.4 ± 0.1 |
47 ± 3 |
32.4 ± 0.9 |
2.2 ± 0.1 |
2.8 ± 0.1 |
29 ± 2 |
48.4 ± 1.4 |
2.3 ± 0.1 |
2.3 ± 0.1 |
25 ± 2 |
CNC : MNT = 2 : 1 |
15.7 ± 0.8 |
2.5 ± 0.1 |
2.2 ± 0.1 |
39 ± 2 |
32.4 ± 2.2 |
1.8 ± 0.1 |
2.3 ± 0.1 |
35 ± 2 |
48.0 ± 3.4 |
2.1 ± 0.1 |
2.3 ± 0.1 |
30 ± 4 |
Conclusions
The time-resolved SAXS study of evaporating levitating droplets provided information over a very large volume fraction range on the structural evolution of aqueous dispersions of CNC rod-like particles with an average thickness of 43 ± 13 Å and an average length of 1540 ± 440 Å, and plate-like MNT particles with a thickness of 10 Å.
The CNC dispersions displayed a d ∝ ϕ−1/2 scaling up to ϕ = 20%, indicating the presence of nematic arrangements competing with gelation, and the d ∝ ϕ−1 scaling that is characteristic of unidimensional helicoidal consolidation only occurred at high volume fractions. The low-q power-law exponent of 2.0–2.2 and a Lorentzian screening length, ξ, between 29 and 23 Å supported the notion of gel-like structures at high volume fractions. The relatively weak and diffuse peak at intermediate volume fractions of the MNT dispersions shows that the MNT platelets were only weakly coordinated but the appearance of a sharper high-q correlation peak at higher volume fractions indicates that the MNT formed stacks. Scherrer analysis suggested that the stacked domains, i.e. the tactoids, contained an average of 7 platelets.
The scattering behaviour of the CNC:MNT dispersions indicates that the co-assembly is dominated by the interactions between the dispersed CNC particles. The applicability of the broad-peak model occurred at lower total volume fractions in the CNC:MNT than in the CNC-only dispersion, which indicates that MNT promotes gelation and the assembly of CNC. Probing the small-angle scattering behaviour of composite mixtures in shrinking levitating droplets over a very broad volume fraction range can elucidate structural transitions and be useful for establishing structure–property relations in assembled nanocomposite materials.
Author contributions
P. M. designed the experiments, prepared the samples, conducted the experiments and participated in the data analysis and the manuscript writing. A. D. performed the curve fittings, participated in the rest of the data analysis and the manuscript writing. S. E. H. assisted in the data analysis and performed TEM imaging. M. K. was the leading coordinator of the synchrotron session, assembled the acoustic levitation setup and assisted in conducting the experiments. M. S. participated in designing and conducting the synchrotron experiments and in the data analysis. L. B. designed the study and participated in the data interpretation and manuscript writing. All authors revised the manuscript.
Conflicts of interest
There are no conflicts to declare.
Acknowledgements
The authors thank the Wallenberg Wood Science Centre (WWSC) and the Swedish Energy Agency (Energimyndigheten, Grant No. 48566-1) for funding. T. S. Plivelic is thanked for providing the acoustic levitator. The experiments were performed on beamline ID02 at the European Synchrotron Radiation Facility (ESRF), Grenoble, France. The authors are grateful to L. Sharpnack, M. Sztucki, and T. Narayanan for technical support at beamline ID02, to M. Agthe, Y. Liu and Z. Lyu for assisting during the experiments, and to E. Chatzigeorgiou for the visualization of the MNT crystal structure. This work benefited from the use of the SasView application, originally developed under NSF award DMR-0520547. SasView contains code developed with funding from the European Union's Horizon 2020 research and innovation programme under the SINE2020 project, grant agreement no. 654000.
References
- F. J. Martin-Martinez, K. Jin, D. López Barreiro and M. J. Buehler, ACS Nano, 2018, 12, 7425–7433 CrossRef CAS PubMed.
-
K. G. Satyanarayana, A. Rangan, V. S. Prasad and W. L. E. Magalhães, in Handbook of Composites from Renewable Materials, ed. V. K. Thakur, M. K. Thakur and M. R. Kessler, Wiley, 2017, vol. 7, pp. 1–66 Search PubMed.
- R. J. Hamers, Acc. Chem. Res., 2017, 50, 633–637 CrossRef CAS PubMed.
-
A. Livingston, B. L. Trout, I. T. Horvath, M. D. Johnson, L. Vaccaro, J. Coronas, C. W. Babbitt, X. Zhang, T. Pradeep, E. Drioli, J. D. Hayler, K. C. Tam, C. O. Kappe, A. G. Fane and G. Szekely, in Sustainable Nanoscale Engineering, ed. G. Szekely and A. Livingston, Elsevier, 2020, pp. 1–18 Search PubMed.
- J. Jeevanandam, A. Barhoum, Y. S. Chan, A. Dufresne and M. K. Danquah, Beilstein J. Nanotechnol., 2018, 9, 1050–1074 CrossRef CAS PubMed.
- H. Kargarzadeh, M. Mariano, D. Gopakumar, I. Ahmad, S. Thomas, A. Dufresne, J. Huang and N. Lin, Cellulose, 2018, 25, 2151–2189 CrossRef CAS.
- R. J. Moon, A. Martini, J. Nairn, J. Simonsen and J. Youngblood, Chem. Soc. Rev., 2011, 40, 3941–3994 RSC.
-
L. Zhong and X. Peng, in Handbook of Composites from Renewable Materials, ed. V. K. Thakur, M. K. Thakur and M. R. Kessler, Wiley, 2017, vol. 7, pp. 155–226 Search PubMed.
- K. De France, Z. Zeng, T. Wu and G. Nyström, Adv. Mater., 2020, 2000657 CrossRef PubMed.
- C. M. Clarkson, S. El Awad Azrak, E. Forti, G. T. Schueneman, R. J. Moon and J. P. Youngblood, Adv. Mater., 2020, 2000718 CrossRef PubMed.
- Y. Liu, C. Schütz, G. Salazar-Alvarez and L. Bergström, Langmuir, 2019, 35, 3600–3606 CrossRef CAS PubMed.
- A. Tripathi, B. L. Tardy, S. A. Khan, F. Liebner and O. J. Rojas, J. Mater. Chem. A, 2019, 7, 15309–15319 RSC.
-
T. Abitbol and E. D. Cranston, Handbook of Green Materials, Vol. 3: Self- and Direct-Assembling of Bionanomaterials, World Scientific, 2014, pp. 37–56 Search PubMed.
- M. Giese, L. K. Blusch, M. K. Khan and M. J. MacLachlan, Angew. Chem., Int. Ed., 2015, 54, 2888–2910 CrossRef CAS.
- A. Tran, C. E. Boott and M. J. MacLachlan, Adv. Mater., 2020, 1905876 CrossRef CAS PubMed.
-
W. Y. Hamad, Cellulose Nanocrystals: Properties, Production and Applications, John Wiley & Sons, 2017 Search PubMed.
- M. Faustini, L. Nicole, E. Ruiz-Hitzky and C. Sanchez, Adv. Funct. Mater., 2018, 28, 1704158 CrossRef.
- Y. Politi and J. C. Weaver, Science, 2015, 347, 712–713 CrossRef CAS PubMed.
- S. Cassaignon, R. de Maleprade, N. Nassif and J. Livage, Mater. Today Proc., 2014, 1, 209–215 CrossRef.
- D. Faivre and T. U. Godec, Angew. Chem., Int. Ed., 2015, 54, 4728–4747 CrossRef CAS.
- S. Islam, L. Chen, J. Sisler and K. C. Tam, J. Mater. Chem. B, 2018, 6, 864–883 RSC.
- B. Wicklein and G. Salazar-Alvarez, J. Mater. Chem.
A, 2013, 1, 5469–5478 RSC.
- E. Kontturi, P. Laaksonen, M. B. Linder, Nonappa, A. H. Gröschel, O. J. Rojas and O. Ikkala, Adv. Mater., 2018, 30, 1703779 CrossRef PubMed.
- V. Singh, P. Srivastava, A. Singh, D. Singh and T. Malviya, Polym. Rev., 2016, 56, 113–136 CrossRef CAS.
- A. A. Oun, S. Shankar and J.-W. Rhim, Crit. Rev. Food Sci. Nutr., 2019, 60, 435–460 CrossRef PubMed.
- A. E. Donius, A. Liu, L. A. Berglund and U. G. K. Wegst, J. Mech. Behav. Biomed. Mater., 2014, 37, 88–99 CrossRef CAS PubMed.
- Q. Fu, L. Medina, Y. Li, F. Carosio, A. Hajian and L. A. Berglund, ACS Appl. Mater. Interfaces, 2017, 9, 36154–36163 CrossRef CAS PubMed.
- S. P. Santoso, L. Laysandra, J. N. Putro, J. Lie, F. E. Soetaredjo, S. Ismadji, A. Ayucitra and Y. H. Ju, J. Mol. Liq., 2017, 233, 29–37 CrossRef CAS.
- C. N. Wu, T. Saito, S. Fujisawa, H. Fukuzumi and A. Isogai, Biomacromolecules, 2012, 13, 1927–1932 CrossRef CAS PubMed.
- U. M. Garusinghe, S. Varanasi, V. S. Raghuwanshi, G. Garnier and W. Batchelor, Colloids Surf., A, 2018, 540, 233–241 CrossRef CAS.
- R. M. Parker, G. Guidetti, C. A. Williams, T. Zhao, A. Narkevicius, S. Vignolini and B. Frka-Petesic, Adv. Mater., 2018, 30, 1704477 CrossRef PubMed.
- L. J. Michot, I. Bihannic, S. Maddi, S. Funari, C. Baravian, P. Levitz and P. Davidson, Proc. Natl. Acad. Sci. U. S. A., 2006, 103, 16101–16104 CrossRef CAS PubMed.
- A. S. Sonin, N. A. Churochkina, A. V. Kaznacheev and A. V. Golovanov, Colloid J., 2018, 80, 593–614 CrossRef CAS.
- Y. Liu, D. Stoeckel, K. Gordeyeva, M. Agthe, C. Schütz, A. B. Fall and L. Bergström, ACS Macro Lett., 2018, 172–177 CrossRef.
- R. M. Parker, B. Frka-Petesic, G. Guidetti, G. Kamita, G. Consani, C. Abell and S. Vignolini, ACS Nano, 2016, 10, 8443–8449 CrossRef CAS PubMed.
- Y. Liu, M. Agthe, M. Salajková, K. Gordeyeva, V. Guccini, A. Fall, G. Salazar-Alvarez, C. Schütz and L. Bergström, Nanoscale, 2018, 10, 18113–18118 RSC.
- H. Liu, H. He and B. Huang, J. Appl. Polym. Sci., 2020, 137, 1–13 Search PubMed.
- T. Abitbol, E. Kloser and D. G. Gray, Cellulose, 2013, 20, 785–794 CrossRef CAS.
- M. Kapuscinski, M. Agthe, Z. P. Lv, Y. Liu, M. Segad and L. Bergström, ACS Nano, 2020, 14, 5337–5347 CrossRef CAS PubMed.
- M. D. Abramoff, P. J. Magalhaes and S. J. Ram, Biophotonics Int., 2004, 11, 36–42 Search PubMed.
-
T. O’Haver, A Pragmatic Introduction to Signal Processing with Applications in Scientific Measurement, Independently Published, 2018 Search PubMed.
- R. Nayuk and K. Huber, Z. Phys. Chem., 2012, 226, 837–854 CrossRef CAS.
- D. Schneidman-Duhovny, M. Hammel and A. Sali, Nucleic Acids Res., 2010, 38, 540–544 CrossRef PubMed.
- J. Schmitt, V. Calabrese, M. A. Da Silva, S. Lindhoud, V. Alfredsson, J. L. Scott and K. J. Edler, Phys. Chem. Chem. Phys., 2018, 20, 16012–16020 RSC.
- P. Bertsch, A. Sánchez-Ferrer, M. Bagnani, S. Isabettini, J. Kohlbrecher, R. Mezzenga and P. Fischer, Langmuir, 2019, 35, 4117–4124 CrossRef CAS PubMed.
- D. A. Ballard, P. Qiao, B. Cattoz, P. J. Dowding, S. Prevost, M. Alshamsi, T. Charpentier, K. J. Roberts, Z. Xu and D. Harbottle, Energy and Fuels, 2020, 34, 6894–6903 CrossRef CAS.
- A. Doekhie, R. Dattani, Y. C. Chen, Y. Yang, A. Smith, A. P. Silve, F. Koumanov, S. A. Wells, K. J. Edler, K. J. Marchbank, J. M. H. van den Elsen and A. Sartbaeva, Sci. Rep., 2020, 10, 9243 CrossRef CAS.
-
L. A. Feigin and D. I. Svergun, Structure Analysis by Small-Angle X-Ray and Neutron Scattering, 1987 Search PubMed.
- B. Tian, Z. Wang, L. de Campo, E. P. Gilbert, R. M. Dalgliesh, E. Velichko, A. J. van der Goot and W. G. Bouwman, Food Hydrocolloids, 2020, 106, 105912 CrossRef CAS.
- G. Paladini, V. Venuti, L. Almásy, L. Melone, V. Crupi, D. Majolino, N. Pastori, A. Fiorati and C. Punta, Cellulose, 2019, 26, 9005–9019 CrossRef CAS.
- T. Matsunaga, T. Sakai, Y. Akagi, U. Il Chung and M. Shibayama, Macromolecules, 2009, 42, 6245–6252 CrossRef CAS.
- A. P. H. Rodrigues, I. M. Pereira, S. D. de Souza, C. S. B. Gil, G. Machado, S. M. Carvalho, F. V. Pereira, P. R. P. Paiva, L. C. A. de Oliveira and P. S. Patrícia, Cellulose, 2017, 24, 1731–1744 CrossRef CAS.
- C. Baravian, L. J. Michot, E. Paineau, I. Bihannic, P. Davidson, M. Impéror-Clerc, E. Belamie and P. Levitz, EPL, 2010, 90, 36005 CrossRef.
- J. C. P. Gabriel, F. Camerel, B. J. Lemaire, H. Desvaux, P. Davidson and P. Batail, Nature, 2001, 413, 504–508 CrossRef CAS PubMed.
- M. Segad, S. Hanski, U. Olsson, J. Ruokolainen, T. Åkesson and B. Jönsson, J. Phys. Chem. C, 2012, 116, 7596–7601 CrossRef CAS.
-
R. A. Schoonheydt and C. T. Johnston, in Developments in Clay Science, ed. B. Faïza and G. Lagaly, Elsevier, 2013, vol. 5, pp. 139–172 Search PubMed.
- L. J. Michot, I. Bihannic, F. Thomas, B. S. Lartiges, Y. Waldvogel, C. Caillet, J. Thieme, S. S. Funari and P. Levitz, Langmuir, 2013, 29, 3500–3510 CrossRef CAS PubMed.
- C. C. Tester, S. Aloni, B. Gilbert and J. F. Banfield, Langmuir, 2016, 32, 12039–12046 CrossRef CAS PubMed.
-
G. Lagaly and I. Dékány, in Developments in Clay Science, ed. B. Faïza and G. Lagaly, Elsevier, 2013, vol. 5, pp. 243–345 Search PubMed.
- M. Segad, J. Appl. Crystallogr., 2013, 46, 1316–1322 CrossRef.
- M. Segad, B. Cabane and B. Jönsson, Nanoscale, 2015, 7, 16290–16297 RSC.
- H. E. King, S. T. Milner, M. Y. Lin, J. P. Singh and T. G. Mason, Phys. Rev. E: Stat. Nonlinear, Soft Matter Phys., 2007, 75, 1–20 CrossRef PubMed.
- M. Morvan, D. Espinat, J. Lambard and T. Zemb, Colloids Surf., A, 1994, 82, 193–203 CrossRef CAS.
- M. Yoonessi, H. Toghiani, T. L. Daulton, J. S. Lin and C. U. Pittman, Macromolecules, 2005, 38, 818–831 CrossRef CAS.
Footnotes |
† Electronic supplementary information (ESI) available. See DOI: 10.1039/d1sm00251a |
‡ These authors have equally contributed to the work. |
|
This journal is © The Royal Society of Chemistry 2021 |