DOI:
10.1039/D1SC02237G
(Edge Article)
Chem. Sci., 2021,
12, 10321-10333
An integrated mass spectrometry imaging and digital pathology workflow for objective detection of colorectal tumours by unique atomic signatures†
Received
22nd April 2021
, Accepted 29th June 2021
First published on 29th June 2021
Abstract
Tumours are abnormal growths of cells that reproduce by redirecting essential nutrients and resources from surrounding tissue. Changes to cell metabolism that trigger the growth of tumours are reflected in subtle differences between the chemical composition of healthy and malignant cells. We used LA-ICP-MS imaging to investigate whether these chemical differences can be used to spatially identify tumours and support detection of primary colorectal tumours in anatomical pathology. First, we generated quantitative LA-ICP-MS images of three colorectal surgical resections with case-matched normal intestinal wall tissue and used this data in a Monte Carlo optimisation experiment to develop an algorithm that can classify pixels as tumour positive or negative. Blinded testing and interrogation of LA-ICP-MS images with micrographs of haematoxylin and eosin stained and Ki67 immunolabelled sections revealed Monte Carlo optimisation accurately identified primary tumour cells, as well as returning false positive pixels in areas of high cell proliferation. We analysed an additional 11 surgical resections of primary colorectal tumours and re-developed our image processing method to include a random forest regression machine learning model to correctly identify heterogenous tumours and exclude false positive pixels in images of non-malignant tissue. Our final model used over 1.6 billion calculations to correctly discern healthy cells from various types and stages of invasive colorectal tumours. The imaging mass spectrometry and data analysis methods described, developed in partnership with clinical cancer researchers, have the potential to further support cancer detection as part of a comprehensive digital pathology approach to cancer care through validation of a new chemical biomarker of tumour cells.
Introduction
Metabolic reprogramming during the earliest stages of cancer growth initiates a radical change in cell chemistry.1 Evidence of oncogenesis can be found in subtle changes to the composition of tumours as they progress from abnormal cells to aggressive metastatic cancers.2 The cytopathological features used to visually diagnose and characterise tumours have remained largely unchanged for over half a century,3 and are a direct result of changes in the underlying chemical composition of pre-malignant cells. Therefore, more accurate and precise measurements of subtle variations in cell composition may allow proto-tumour cells to be identified before visibly identifiable characteristics appear.
Carbon, hydrogen, nitrogen, and oxygen account for >95% of all chemical elements in the human body. The remaining fraction is a dynamic mixture of minor and trace elements at micro-to-nanomolar concentrations. Initially termed the metallome when first introduced in the early 2000s,4–6 this exchangeable pool of elements has now grown to include abundant non-metals and metalloids that encompasses the chemical base units of cell physiology. In total, 28 elements have been identified as essential for life, with bromine added to the list in 2014.7 Non-essential environmental elements are also common in human tissue and fluids, such as inert alkali metals absorbed from drinking water to highly toxic anthropogenic metals. Importantly, this complement of elements is in a constant state of flux between cellular compartments that comprise the genome, transcriptome, proteome, and metabolome; and capturing a snapshot of this atomic signature gives one of the most comprehensive pictures possible of a biophysical state.
Concentrations and arrangement of chemical elements in the cellular milieu is the ultimate determinant of tissue morphology, changing as cells divide, develop and reach maturity. Similarly, there is a quantitative and spatial rearrangement of elements that commences when a cell shifts from a healthy cell to becoming a proto-tumour environment.8 These fundamental biochemical processes occur prior to any of the morphological changes or immunoactivity that are currently used to detect the appearance of malignancy. Common across all forms of cancer, this “Warburg effect”9 of increased ATP production, biosynthesis of the four major macromolecule classes (carbohydrates, proteins, lipids, and nucleic acids), and tightened redox control serves as a prelude to tumour growth and differentiation.10 Subsequent cell division, angiogenesis, autoimmune responses, and eventual metastasis all stem from this shift in fundamental cell chemistry.
Early-stage alterations in small molecule metabolites produced through the pentose phosphate pathway,11 Krebs cycle,12 Ser–Gly-one carbon metabolism,13 glutathione synthesis,14 and choline metabolism15 have all been identified as diagnostic and biomarker discovery targets for colorectal cancer (CRC),16 as well as other tumour types.17 When considering that redox-active metal species, such as iron-containing haem, copper, and manganese redox substrates, are all metabolic cofactors within mitochondrial one-carbon metabolism,18 there is an innate link between metals and tumorigenic disruptions to crucial metabolic processes. Iron, copper, zinc, and calcium ions are all intrinsically linked to chemical processes that promote metastasis;19 from the generation of reactive oxygen species (ROS) by labile iron20 to copper-mediated acceleration of angiogenesis.21
Laser ablation-inductively coupled plasma-mass spectrometry (LA-ICP-MS) was first introduced in 1985 for discrete micro-sampling of geological samples.22 Imaging applications were first described in 1994,23 and the technology has matured into a key component of the broader mass spectrometry imaging field.24,25 Applications for LA-ICP-MS imaging in cancer research have grown steadily over the last decade, ranging from observational studies of in26 and ex vivo27 tumour chemical microenvironments to the development and commercialisation of time-of-flight LA-ICP-MS instruments for high-dimensional multiplexed immunoimaging28 and precision oncology.29
Here, in this proof of concept study, we present a completely new approach to supporting cancer detection using LA-ICP-MS that is inspired by recent innovations in chemical imaging that fuse automated feature detection and molecular mass spectrometry imaging.30 We describe each step in the development and preliminary validation of an atomic signature biomarker of colorectal cancer and discuss where next generation analytical technology and digital pathology can be used for future validation.
Results and discussion
Elemental imaging of surgical colon resections
For the initial algorithm development, we began with formalin-fixed, paraffin-embedded (FFPE) tissue blocks taken from human subjects during surgical colorectal resectioning. Cohort characteristics that were relevant for this study are presented in Table 1. Our training cohort consisted of FFPE sections of matched normal intestinal wall (NIW) and tumour-containing tissue from three CRC patients, as well as one additional tumour-containing known–unknown section for supervised algorithm testing and a matched case for unsupervised testing.31
Table 1 Deidentified case numbers and pathological examination of H&E-stained surgical resections for algorithm development. CRC = colorectal cancer; NIW = normal intestinal wall; TNM = tumour, node, metastasis; x = could not be assessed
Case ID |
Case notes |
TNM classification |
NIW2 |
Normal intestinal wall, single lymphoid follicle in bottom left submucosa |
n/a |
NIW3 |
Normal intestinal wall, three lymphoid follicles in mucosa |
n/a |
NIW1 |
Normal intestinal wall, no lymphoid follicles present |
n/a |
CRC12 |
Colon cancer. Moderately differentiated adenocarcinoma. Small clusters of tumour cells infiltrating muscle layer |
T4N1M0 |
CRC13 |
Colon cancer with adenomatous polyp on left edge. Moderately differentiated adenocarcinoma |
T3N0Mx |
CRC10 |
Colon cancer. No normal mucosa, muscle or polyp. High levels of mucin at leading edge of tumour. Poorly differentiated mucinous carcinoma |
T3N0M0 |
CRC11 |
Colon cancer. Moderately differentiated adenocarcinoma. Small clusters of tumour cells infiltrating muscle layer |
T3N0M0 |
NIW4 |
Normal intestinal wall, no lymphoid follicles present |
n/a |
CRC18 |
Colon cancer. Moderately differentiated adenocarcinoma |
T3N0M0 |
Dewaxed sections were analysed by LA-ICP-MS at 40 × 40 μm spatial resolution (Fig. 1A; total pixel area = 1600 μm2; see Methods and materials for complete experimental parameters). Periodic analysis of in-house prepared matrix-matched tissue standards32 was used to correct for signal instability (or instrument ‘drift’) over the 24 hours needed to collect image data for one sample. Image pre-processing was performed using the biolite33 add-on for iolite 3 (University of Melbourne, Parkville, Victoria, Australia);34 and post-processing in Igor Pro 6.37 (WaveMetrics, Portland, Oregon, USA). After background subtraction, the measured mass in each pixel was normalised to the corresponding high intensity (>8%) phosphorus signal. This step was applied to remove uncertainty between imaging experiments arising from variable element response when using LA-ICP-MS. We reason that applying a high abundance reference element that meets most of the criteria set by Austin et al.35 during this stage of algorithm development was necessary to correct for possible inter-sample variability in ablation performance for a sample set collected over a six-month period on a high-use LA-ICP-MS system. Carbon normalisation was not applied, as 13C signal showed a poorer signal-to-background ratio in dewaxed FFPE tissue and the total 13C signal was <8% of the total ion count.35 Tissue standards were also used to generate multi-point external calibrations, which were then applied to each image pixel, producing fully quantitative images for each measured m/z. The experimental parameters used were optimised for polyatomic interference-free36 imaging using a triple quadrupole ICP-MS,37 and we refer to these maps as elemental images hereafter. The raw data used to produce the images shown in Fig. 1A and subsequent figures can be accessed via the ESI.†
 |
| Fig. 1 Workflow for data generation, image analysis, and feature detection. (A) Surgical tissue resections were analysed by LA-ICP-MS to produce quantitative maps of each measured element. (B) Haematoxylin and eosin (H&E) stained sections were annotated according to tissue type by a pathologist, which were then overlaid on LA-ICP-MS images and used to extract spatial element concentrations in four distinct anatomical features: primary tumour, adenomatous polyp, mucosa layers, and smooth muscle tissue. | |
Extraction of tissue-specific elemental profiles
A pathologist provided a roughly annotated photomicrograph of an adjacent section stained with haematoxylin and eosin (H&E), which was then coarsely overlaid on corresponding LA-ICP-MS images and used to extract elemental data (Fig. 1B). Tissue was classified according to four major anatomical categories: smooth muscle, mucosa, adenomatous polyp, and primary tumour (where present). On average, a 40 × 40 μm laser spot produces images of colorectal resections with approximately 200
000 pixels per measured m/z, with sufficient signal-to-noise ratios for up to eight mid-mass range elements with a scan cycle time of 0.25 s. We experimented with 6–8 element panels with m/z ranging from 13 to 66, and a conservative laser scan speed of 160 μm s−1, and acknowledge that future clinical translation work will require the use of new hardware advances38 that eliminate laser and ICP-MS syncing issues and aliasing effects for statistically robust high throughput imaging.39 Pixels falling within these set x–y coordinates were extracted in Igor Pro (see Methods) using the annotated H&E for later statistical analyses and comparisons.
Training a detection algorithm for atomic signatures of colorectal tumours
The training cohort of matched NIW and primary tumour resections were imaged using LA-ICP-MS and the quantitative phosphorus-normalised calcium, copper, iron, magnesium, sulfur, zinc data; as well as 13C signal standardised to matrix-matched tissue standard carbon content for each tissue type was extracted (Fig. 2A). The mean and two standard deviations (SD) per tissue type was pooled to produce a universal coarse value (expanded mean ± 2SD) for the sample training set. The areas identified by a pathologist in the annotated H&E image were used to create a binary ideal image, where each tumour pixel was assigned a value of 1 and all others assigned a value of 0 (Fig. 2B). For matched NIW sections that did not contain identified tumour cells, the ideal image had a value of 0 for all pixels. Ideal images and elemental maps were merged into a single hyperspectral dataset for optimization of tumour threshold signatures for each analyte. We used a Monte Carlo optimisation approach (Fig. 2C), similar to that previously used to detect serum biomarkers of ovarian cancer using bead-based immunoassays40 and spatially profile microscale tooth mineralisation patterns by synchrotron X-ray tomography.41 In our application, each pixel was treated as an independent observation, resulting in approximately 1.4 million (7 analytes with c.a. 200
000 pixels each) measurements that were used in the predictive model.
 |
| Fig. 2 Determination of atomic thresholds for colorectal tumours. (A) LA-ICP-MS images were made for a total of six matched tissue samples from three colorectal cancer patients, three containing the primary tumour and three of normal intestinal wall. Each phosphorus-normalised image was used to extract the mean ± 2SD according to tissue type. From these values, a pooled mean ± 2SD for tumours was generated. (B) A binary ‘ideal’ image of a tumour-containing biopsy was then generated using an annotated H&E image as a reference, where tumour-containing pixels were assigned a value of 1 and all others assigned 0. (C) A Monte Carlo simulation for each atomic threshold was performed, where each pixel with a value below the optimised cut-off were considered positive signatures of pixels representing areas of high cell proliferation. Phosphorus-normalised Ca was a strong discriminator between tumour and non-tumour pixels: here, (i) a total difference of approximately 220 000 pixels is the default case where no pixels pass the threshold process, and the Monte Carlo process determined Ca/P < 0.140 as the optimized threshold. (ii) Below this value, the Ca:P threshold becomes over-specific, and the match metric shows this as trending back up towards the no match value of 220 000. (iii) For the optimized threshold value of 0.14, there is still some variation in the total difference due to the random trial of other atomic threshold values in the Monte Carlo process. | |
Testing the automated tumour detection algorithm
The supervised Monte Carlo optimisation experiment was tested using a tumour-positive case (6451T). The final ideal threshold-set was determined by applying a brute-force search within the pooled mean ± 2SD of tumours and summing the total difference between each ideal image and the resulting tumour map for each sample in the training set. Specifically, a random P-normalised atomic threshold within the defined mean ± 2SD range was tested for each element; a process repeated 1000 times. In each iteration, the pixels for each elemental image were compared to the threshold and those greater than the threshold were eliminated from the map of pixels identified as tumour. (Fig. 3A) Only pixels that passed all stages of the thresholding process and a 3 × 3 Gaussian filter applied to remove pixels representing intrinsic MS noise42 remained in the final predictive map (Fig. 3B). Overall, Ca content showed the highest discriminating power and was generally lower in tumours relative to non-malignant tissue, though the subsequent optimisation of copper and zinc thresholds removed approximately 10% of remaining false-positive pixels.
 |
| Fig. 3 Application of a supervised learning model for detecting atomic signatures in tumours. (A) The P-normalised quantitative element thresholds were optimised in a stepwise fitting of the Monte Carlo simulation, (B) leaving an image where only pixels fitting the model criteria were displayed (i) that can be contrasted side-by-side with a H&E-stained slide (ii; primary tumour outlined in red). Scale bars = 2 mm. All colour LA-ICP-MS images are overlaid on greyscale images of P distribution to show anatomical boundaries. | |
Assessing algorithm specificity
We tested the Monte Carlo optimisation using an unsupervised learning test case with a moderately differentiated adenocarcinoma tumour in situ and matched NIW. The algorithm and learned atomic signatures from the individual quantitative element maps were applied to the dataset to produce two binary thresholded images, which were then compared against H&E and Ki67 immunohistochemical stains. Ki67 is widely used in clinical cancer research as an endpoint biomarker43 and debated prognostic indicator for colorectal tumours.44,45 The non-histone nuclear protein is expressed throughout the active phase of cell cycle, and increased expression in tumours above a 25% cut-off is associated with higher levels of cell proliferation in colorectal cancer.46
The NIW image (Fig. 4A) showed sparse clusters of positive pixels, and when overlaid on the adjacent H&E section it was confirmed these areas did not correspond to any visible invasion of the submucosa required to meet the standard histopathological classification of a T1 primary tumour.47 False-positive pixels did, however, correspond to high Ki67 expression in lymphoid follicles; consistent with increased expression in germinal centres and distal lymph nodes of colorectal cancer patients.48 Conversely, the moderately advanced T3 primary adenocarcinoma was clearly demarcated from the adjacent non-neoplastic tissue and showed infiltration into the submucosa and smooth muscle tissue layers (Fig. 4B).
 |
| Fig. 4 Testing the predictive Monte Carlo simulation model. (A) NIW tissue (sample NIW4) returned matching pixels (i) in lymphoid follicles (via H&E; ii) but no apparent primary tumour mass (CRC11, via Ki67 expression in; iii). (B) In tissue taken from the primary tumour site, adenocarcinoma infiltration of submucosal layers is apparent in tumour signature maps (i), and H&E (ii; tumour outlined in red) and Ki67 (iii) images. Scale bars = 2 mm. | |
A visual inspection of corresponding atomic signature images was used to assess specificity of the Monte Carlo optimisation approach in identifying tumour cells, with and without assistance from cell morphology (H&E) and Ki67 expression micrographs (Fig. 5).
 |
| Fig. 5 Atomic signature images of high cell proliferation in T3N0M0 colorectal adenocarcinoma (case CRC18). (A) LA-ICP-MS imaging and application of the Monte Carlo optimisation algorithm returned positive pixel results in areas corresponding to the primary tumour margins (i and ii), distal lymph lodes (iii), and mucosal crypt cells, as confirmed by (B) H&E staining and (C) Ki67 immunoreactivity. Scale bars = 1 mm (whole sections) and 2 mm (i–v). | |
Monte Carlo optimisation detects an atomic signature of cell proliferation
The Monte Carlo approach used to this point appeared to accurately detect and discriminate tumour tissue from adjacent non-neoplastic cells, though two of the three NIW cases consistently returned false positive results that were confirmed to correspond with lymphoid follicles (shown in Fig. 6A). H&E staining confirmed that they were too small and displayed no invasion of the submucosa to meet the standard classification of a primary tumor.47 Additionally, NIW3 showed 63 positive areas (maximum width > 0.2 mm) in the lower crypts of normal mucosal epithelium, where Ki67 expression is typical of gastrointestinal cell proliferation49 and may represent an early marker of adenocarcinoma formation.50 While we cannot determine if these signatures are specific to tumours without unmatched samples, the possibility these signatures represent a chemical state of pre-malignancy and hyper-proliferation cannot be excluded.
 |
| Fig. 6 Atomic signatures of cell proliferation and malignancy. (A) NIW samples (left to right NIW2, NIW3, NIW1) had scant areas with positive LA-ICP-MS image pixels that corresponded with lymphoid follicles (i) and basal crypt cells (ii and iii) in two cases. (B) Two adenocarcinoma (CRC12, left; and CRC13, middle columns) and one mucinous carcinoma (CRC10, right column) were accurately detected with positive atomic signature values corresponding to confirmed primary tumours in H&E stain (outlined in red) and Ki67 expression micrographs. Black arrow indicates an adenomatous polyp. Scale bar = 2 mm. | |
Colorectal tumours have a characteristic atomic signature
In addition to identifying lymphoid follicles and proliferating mucosal cells in NIW tissue, the Monte Carlo method demarcated tumours from visually non-neoplastic tissue in tumour resections (Fig. 6B). Interestingly, the algorithm correctly identified tumour cells in one case where the primary malignancy was a mucinous carcinoma. Detection of mucinous carcinoma, which account for 10–15% of colorectal tumours, is prone to errors arising from subjective assessment, and diagnosis is generally associated with poorer prognosis and treatment response.51 Our algorithm delineated tumour from high levels of mucin, and lesion dimensions accordingly reflected a poorly differentiated tumour. We were also able to correctly identify a contiguous adenomatous pre-malignant polyp in one adenocarcinoma case.
The detection algorithm correctly identified NIW and adenocarcinoma cells in the unsupervised learning stage T3N0M0 moderately differentiated adenocarcinoma test case shown in Fig. 4, again observing areas of high cell proliferation below the dimensional threshold for primary tumours in the NIW sample. Significant tumour area and clear infiltration of the adjacent muscularis propria confirmed staging as a T3 primary tumour.47,52
Improving the predictive model using random decision forests
Our initial attempt at determining a unique chemical signature for tumours used a very basic decision tree. This decision tree only used each element once, and the output of this decision tree is a simple 1 where the pixel is considered to be part of a tumour, and 0 elsewhere. The optimum value for each element's threshold was determined by this Monte Carlo approach of setting each threshold and passing each pixel value through the decision tree, and then calculating the total error with each set of thresholds. Here total error refers to the sum of the difference between the ‘ideal’ image, and output of the decision tree.
Machine learning performs best with larger datasets and confidence in the training data. We investigated if more sophisticated machine learning methods were able to improve model specificity and eliminate false positive pixels from the final predictive images. Random forests are a deep ensemble learning method for natural non-linear predictive modelling with a greater tolerance for noise and outliers and less likelihood of data overfitting.53,54 We used an expanded set of samples from the Australasian Colorectal Cancer Family Registry (ACCFR; see Table 2)55,56 to develop an extended algorithm using random forests instead of Monte Carlo optimisation, and demonstrate how deep learning reveals a new biomarker for colorectal tumour growth in elemental composition.
Table 2 Unique case identifiers with AJCC stage (where available) and TNM classification for random forest algorithm development and testing. Cases CRC10-14 and NIW1-3 from the Monte Carlo algorithm development were also included in the training set, and CRC18 and NIW4 were used in blinded testing (see Table 1). AJCC = American Joint Commission on Cancer52
Case ID |
Case notes |
AJCC stage |
TNM classification |
Training set
|
CRC1 |
Moderately differentiated adenocarcinoma in caecum |
I |
T2N0Mx |
CRC2 |
Moderately differentiated adenocarcinoma in ascending colon |
IIA |
T3N0Mx |
CRC3 |
Well differentiated adenocarcinoma in ascending colon |
IIIB |
T3N1Mx |
CRC4 |
Moderately differentiated adenocarcinoma in ascending colon |
I |
T1NxMx |
CRC5 |
Moderately differentiated adenocarcinoma in caecum |
X |
T3N0Mx |
CRC6 |
Poorly differentiated adenocarcinoma in descending colon |
X |
T4N1Mx |
CRC7 |
Poorly differentiated adenocarcinoma in rectum |
X |
T3N0Mx |
CRC8 |
Poorly differentiated mucinous carcinoma in transverse colon |
X |
T1N0Mx |
CRC9 |
Poorly differentiated mucinous carcinoma in caecum |
X |
T3N0Mx |
CRC10 |
Moderately differentiated adenocarcinoma in ascending colon |
X |
T3bN0M0 |
CRC12 |
Moderately differentiated adenocarcinoma in sigmoid colon |
X |
T4N1M0 |
![[thin space (1/6-em)]](https://www.rsc.org/images/entities/char_2009.gif) |
Blinded testing set
|
CRC14 |
Well differentiated adenocarcinoma in ascending colon |
IIA |
T3N0Mx |
CRC15 |
Moderately differentiated adenocarcinoma in descending colon |
IIA |
T3N0Mx |
CRC16 |
Moderately differentiated adenocarcinoma site unknown |
U |
T2N0Mx |
CRC17 |
Moderately differentiated adenocarcinoma in sigmoid colon |
IIIB |
T3N1Mx |
CRC19 |
Poorly differentiated adenocarcinoma in sigmoid colon |
X |
T3N1Mx |
Using random forests, the “yes/no” output describing whether data aligns to the ideal tumour is exponentially improved. Given that our initial results justified the confidence in our background subtraction and between-run standardisation,33 that data was collected with a varying panel of analytes resulting in P signal not consistently being >8% of total signal intensity, and the possibility that false positives reflect a discriminating variable in hyperactive phosphate metabolism in neoplastic tissue2 we did not normalise signal to P when using random forests. We included all tumour and NIW data from the Monte Carlo algorithm development, which were used in algorithm training and blinded testing.
Introducing a quantitative and spatial metric of algorithm performance
The model design is presented in Fig. 7. The pooled data were divided into training, testing, and validation groups, and then split into 100 different decision trees to build the final model (Fig. 7A). Each tree can be visually depicted as a series of challenges, where each element concentration determines the next decision leaf in the growing tree (ESI Fig. 1†). A total of 168
930 samples were used per tree, which amounts to 1.6893 × 106 total decisions, or “leaves” used in the final model. A pixel that successfully meets all criteria and remains after 100 iterations of the random decision forest processing has a perfect score of 1, which was assigned to a simple RGB colour scale for all scores above an arbitrary cut-off that we set at 50 positive hits for that data pass. This can be interpreted as a yellow pixel having a value that met the predefined conditions (as determined by manual input of tumour-positive training data from annotated H&E images) in ∼90% of the 100 decision trees.
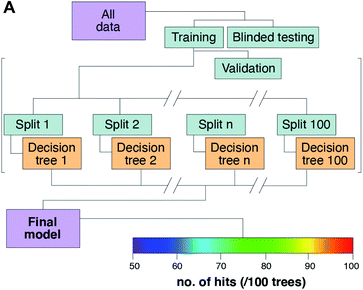 |
| Fig. 7 Random decision forest model design. (A) Input data were allocated to training, testing, and blinded validation sets for later comparisons against Monte Carlo simulation images; and used to grow 100 decision trees that present a final result as a number, or score, of hits against the training ideal dataset. | |
Random forests outperform Monte Carlo simulations
Eleven confirmed primary tumour resections were used to train the random forests model. The data from these cases was then used to validate model accuracy against the same ‘ideal’ tumour image. The trained model correctly identified and visually demarcated primary tumour cells from surrounding non-neoplastic tissue, confirmed by pathological examination of H&E-stained tissue (Fig. 8; ESI Fig. 2†). A further six cases were then used for operator blinded testing, all of which were correctly identified as either tumour containing (n = 5; Fig. 9; ESI Fig. 3†) or NIW (n = 1). Previously false-positive pixels in NIW4 (shown in Fig. 4A), which we attributed to lymphoid follicles and areas of rapid cell proliferation were no longer detected when reprocessed using the random forests (ESI Fig. 4†).
 |
| Fig. 8 H&E stain micrographs and corresponding atomic signature images for random forest regression model training. Primary tumours (outlined in red) included T classifications of: (A) T2 and (B and C) T3. Scale bar = 2 mm. | |
 |
| Fig. 9 Blind testing of random forest model for automated detection of colorectal tumours by atomic signature. Primary tumours (outlined in red) were classified according to TNM staging as: (A) T3, (B) T3, and (C) T2. Scale bars = 2 mm. | |
Interpretation of algorithm outcomes
The individual features for each element are broadly consistent with established knowledge in oncogenetics. For instance, we observed lower calcium levels in tumours relative to unaffected mucosa and smooth muscle layers, which is consistent with depleted intracellular Ca2+ and broader Ca metabolic remodelling that occurs in colorectal cancer cells.57 While this has preclinical research relevance-non-steroidal anti-inflammatories like aspirin may have protective effects against colorectal cancer58,59 by regulating intracellular Ca2+ levels-it is also important to recognise risks of overinterpreting LA-ICP-MS imaging. While it is certainly possible that elemental distribution changes are reflective of an underlying oncogenic pathology, each mechanism must be further interrogated using native-state conditions to preserve chemical state as much as possible.60
Fixation, dehydration and paraffin embedding undoubtedly and irrevocably alters tissue biochemistry in a way that limits direct interpretation of changes in metal species, and each analyte is affected to differing degrees. However, LA-ICP-MS allows for thorough interrogation of tissue composition in the same way histochemical staining allow for morphological assessment. Our results show that even if prior chemical treatment has influenced the chemical composition of tissue, it has done so in a reproducible way that has not impaired accurate detection of primary colorectal tumours.
Cautions against overinterpretation
It is important to highlight here that tissue heterogeneity is a major consideration for the accuracy of any future algorithm that uses chemical imaging, much as it is for any other approach to visualising tumour microenvironment.61,62 While we used a rough-cut segmentation for ease of transfer between imaging software and algorithm code, more detailed input from a pathologist at the cellular level is an achievable mid-term goal. Other mass spectrometry imaging approaches to examining tumour heterogeneity, such as CyTOF imaging mass cytometry63 (which incidentally uses a modified LA-ICP-TOF-MS platform28) could be easily run in sequence in a multi-omic imaging workflow using common metal tags for cell segmentation as fiducial markers. Future integration with automated feature detection from morphological classifiers like those used by Yu et al.64 to objectively predict non-small lung cell cancer prognosis is a key research focus for integrating mass spectrometry imaging with digital pathology.
Future analytical and experimental considerations
The most pressing limitations that must be overcome should it have future implications in cancer research is the time taken for analysis, and we were admittedly restricted with respect to technical capabilities. As per our previous work on the fundamentals of LA-ICP-MS imaging using low repetition (<20 Hz) lasers and sequential quadrupole-based mass analysers,65 and acknowledging the importance of avoiding image artefact when increasing laser speed,38,66 we were limited with respect to sample throughput. Future studies would be well suited to newer LA-ICP-MS systems specifically engineered for high-throughput bioimaging.67
Elemental composition is not typically considered to be a discriminating feature of cancer, and while variations in inorganic content relative to control have been reported in observational studies of small CRC cohorts68–70 they lack the specificity necessary for future translation as clinical biomarkers. In practice, like any biochemical marker, diagnostic and prognostic value will ultimately be found in an orthogonal approach that encompasses multiple imaging modalities capable of spatially resolving visual and chemical components of intact tissue specimens.71 Alignment of LA-ICP-MS images with data from spatial profiling of proteins,72 lipids,30 established tumour markers,73 and other emerging applications of MS imaging using an experimental paradigm where optical microscopy and direct input from pathologists extracts information not apparent in individual images.71
Finally, we would like to further acknowledge and comment on aspects of our experimental design that could be improved in retrospect and highlight the importance of direct engagement with clinicians. This study was performed over approximately three years on a high use LA-ICP-MS system using relatively low clinical value surgical resections. As a method development project, we were focused on creation of our image processing algorithm rather than possible clinical translation. Smaller punch biopsies or tissue microarrays that have previously been analysed by LA-ICP-MS imaging74 could be measured in less time and greater numbers with dedicated infrastructure support. It is also important to note that no automated detection method can ever be devoid of human error, and we advocate for this and all other LA-ICPMS methods to be developed with and alongside pathologists to support diagnostic accuracy. Further integration of digital pathology with mass spectrometry imaging, where direct input from pathologists is determining data extraction parameters, is essential if future clinical use is the goal.
Conclusions
Our results using random forest regression highlights the highly heterogeneous nature of in situ primary tumours over the simpler “all or nothing” Monte Carlo simulation model. LA-ICP-MS imaging was able to independently identify primary tumours, confirmed by direct comparisons with histopathological stains by a trained clinician. As with any machine learning approach to analytical biochemistry, the quality of training information and data is key to accurate detection, and our proof-of-concept study justifies continued integration between clinicians and mass spectrometry data analysts. Future work using LA-ICP-MS imaging to chemically analyse tumours should work in conjunction with innovations in digital pathology to investigate how robust the atomic signature we describe here is, which combined with multi modal imaging may prove to be a useful diagnostic and prognostic tool in for cancer research and management.
Methods and materials
Human ethics approval
Tissue samples were obtained from the Jeremy Jass Memorial Tissue Bank from participants recruited to the Australasian Colorectal Cancer Family Registry (ACCFR). Details of ACCFR recruitment, tumour collection, pathological assessment, and mutation testing have been described in detail previously55,75 and are available on the international Colon Cancer Family Registry website.76 Written informed consent was obtained from all participants to collect tumour pathology materials. The study protocols were approved by Human Research Ethics Committees at the University of Melbourne (ID: 1339757).
Sample preparation
FFPE tissue was obtained from surgical colectomy specimens collected and routinely processed from various pathology laboratories throughout Australia. 4 × 4 μm serial sections were obtained from each block. Slides were air-dried and stored in a dust-free environment prior to analysis. The sections were floated and mounted on to uncoated Superfrost Plus® adhesive glass slides (ProSciTech, Kirwan, Queensland, Australia). Sections were taken from the same paraffin ribbon cut from each tissue block but were not immediately adjacent. All sections underwent the same dewaxing procedure of three changes xylene followed by two changes of 95% ethanol (EtOH; v/v) in H2O, and a H2O wash. Sections were then either stained using H&E, used for immunohistochemistry, or analysed by LA-ICP-MS according to the methods described.
Histochemical and immunohistochemical staining
Sections were stained with H&E per standard protocols.77 Slides with dewaxed sections were immersed in a 0.5% hematoxylin (w/v) solution for four minutes, rinsed in distilled H2O, differentiated using 0.3% HCl (v/v) in 70% EtOH (v/v), rinsed in distilled H2O again, and counterstained in a 0.1% acidified eosin (v/v) solution in 95% EtOH (v/v). Stained sections were mounted in Depex® and cover slipped. Photomicrographs were recorded using a Mirax digital slide scanner (Zeiss). The Ki67 staining was be performed on the Ventana® Ultra platform using the OptiView® detection system using established antibodies and protocols carried out as part of the ACRF cohort study.
Laser ablation-inductively coupled plasma-mass spectrometry
All mass spectrometry imaging experiments were performed using an Elemental Scientific Lasers NWR213 (Kenelec Scientific, Mitcham, Victoria, Australia) laser ablation system and an Agilent Technologies 8800x Series (Mulgrave, Victoria, Australia) triple-quadrupole inductively coupled plasma-mass spectrometer (ICP-QQQ-MS), using methods previously described.37 The NWR213 was fitted with a standard 15 × 15 cm two-volume cell. Argon was used as the carrier gas at 1.05 mL min−1. A constant laser fluence of 0.3 mJ cm−2 was used, which was sufficient to ablate tissue but below the minimum threshold of 2.4 J cm−2 required to ablate silicate glass.78 A 40 μm square 213 nm laser pulse with a frequency of 20 Hz was used, scanning the sample at 160 μm s−1. The total integration time for each scan cycle was 0.25 s, producing pixels with 1600 μm2 total area.65 The ICP-MS was fitted with a tapered quartz torch and Pt sampler and skimmer cones. The ICP-MS was operated in MS/MS mode with time resolved analysis. Hydrogen was used as a reaction gas (3 mL min−1) to eliminate 40Ar16O+ polyatomic interference on 56Fe arising from O2 impurities in the Ar carrier gas.79 Each sample required approximately 24 hours to complete data acquisition.
Image pre-processing
Data were exported from MassHunter (Agilent Technologies) as comma separated value (.csv) files, which were then collated into a single file using a Python script. Images were constructed using Igor Pro 6.37 (WaveMetrics, Portland, Oregon, USA). Data were then imported using the Biolite33 module for iolite 3.34 See Hare et al.80 for a step-by-step visual tutorial.
Image post-processing
Elemental concentration data from tissue sections were extracted by manually drawing regions of interest (ROIs) onto the P image using the pathologist-annotated H&E-stained images for reference and using these ROIs with Igor Pro's ImageStats function. The ‘ideal’ image (see Fig. 2) was constructed by using the tumour ROI, and setting all values within this ROI to 1, and all outside to 0 (ideal images for NCW samples were set to 0 for all pixels).
Thresholding, and optimization of the threshold values, was conducted using a custom script written in Igor Pro. The optimisation process selected a random threshold for each elemental image from a defined range (initially informed by the tumour average and 2SD results from the previous step). A tumour map was created for each sample where 1 a pixel identified as tumour, and 0 everywhere else. Initially all pixels in the tumour map were set to 1. Each image was then examined in turn, and any pixels within the current image that are above the set threshold were set to 0. At the end of the thresholding process, the only pixels that were still set to 1 were those that have passed each step of the thresholding process. The pixels in the tumour map were then compared to the ‘ideal’ image for the sample, and the absolute difference between the two was recorded as the result for that set of threshold values. The set of threshold values with the lowest total result (that is, the lowest total difference between the ideal image and tumour map for each sample) was considered the optimised threshold result. Following application to each case, a 3 × 3 Gaussian filter was applied to remove pixels representing intrinsic noise.
Data availability
Data for this paper, including mass spectrometry data and processed LA-ICP-MS images are available online at https://doi.org/10.26188/14912964.
Author contributions
Conceptualisation: BP, KK, PJC, DJH. Experimental data collection: KK, JBH, DDB, CR, DJH. Data analysis: BP, MWMJ, DJH. Resources: RWH, AIB, JMH, JDW, RW, PAD, MMH, PJC, DJH. Data visualisation: BP, CR, DJH. Writing (drafting): BP, DJH. Writing (editing): BP, KK, JBH, MWMJ, RWH, DDB, JDW, JMH, DPB, PJC, DJH.
Conflicts of interest
There are no conflicts to declare.
Acknowledgements
We wish to thank Associate Professor Fred Hollande and Dr Corina Behrenbruch of the University of Melbourne, Dr Tristan Rawling of the University of Technology Sydney, and Dr Nerida Cole of Swinburne University for helpful advice in preparing this manuscript. This study utilised the Australian Phenomics Network Histopathology and Organ Pathology Service, University of Melbourne. Surgical sections used in this study were obtained from the Jeremy Jass Memorial Pathology Bank. The content of this manuscript does not necessarily reflect the views or policies of the National Cancer Institute or any of the collaborating centres in the CCFR, nor does mention of trade names, commercial products, or organisations imply endorsement by the US Government or the CCFR. This work was funded in part by a Linkage Project grant from the Australian Research Council (ARC; LP120200081 to P. A. D. and D. J. H. in partnership with Agilent Technologies and ESI Ltd). K. K. held a Research Fellowship from the Sigrid Juselius Foundation. C. R. is a Jass Pathology Fellow. M. A. J. is a National Health and Medical Research Council (NHMRC) Senior Research Fellow (GNT1117611). D. D. B. is a University of Melbourne Research at Melbourne Accelerator Program (R@MAP) Senior Research Fellow and NHMRC R. D. Wright Career Development Fellow (GNT1125268). D. J. H. was a NHMRC Industry Career Development Fellow (GNT1122981; in partnership with Agilent Technologies) and was a UTS Chancellor's Postdoctoral Research Fellow. P. J. C. was a NHMRC R. D. Wright Career Development Fellow (GNT1084927). This work was supported by grant UM1 CA167551 from the National Cancer Institute to the Colon Cancer Family Registry (CCFR) and through cooperative agreement with the Australasian CCFR (U01/U24 CA097735).
Notes and references
- G. J. Yoshida, J. Exp. Clin. Cancer Res., 2015, 34, 111 CrossRef PubMed
.
- A. Schulze and A. L. Harris, Nature, 2012, 491, 364–373 CrossRef CAS
.
- M. M. Boddington and A. I. Spriggs, Br. Med. J., 1965, 1, 1523 CrossRef CAS PubMed
.
- H. Haraguchi, J. Anal. At. Spectrom., 2003, 19, 5–14 RSC
.
- H. Haraguchi, Metallomics, 2017, 9, 1001–1013 CrossRef PubMed
.
- J. Szpunar, Anal. Bioanal. Chem., 2004, 378, 54–56 CrossRef CAS PubMed
.
- S. A. McCall, C. F. Cummings, G. Bhave, R. Vanacore, A. Page-McCaw and B. G. Hudson, Cell, 2014, 157, 1380–1392 CrossRef
.
-
H. Chial, Proto-oncogenes to Oncogenes to Cancer, Nat. Educ., 2008, vol. 1, p. 33 Search PubMed
.
- O. Warburg, Science, 1956, 123, 309–314 CrossRef CAS PubMed
.
- R. A. Cairns, I. S. Harris and T. W. Mak, Nat. Rev. Cancer, 2011, 11, 85–95 CrossRef CAS
.
- W. Gao, Y. Xu, T. Chen, Z. Du, X. Liu, Z. Hu, D. Wei, C. Gao, W. Zhang and Q. Li, PLoS Biol., 2019, 17, e3000425 CrossRef CAS
.
- K. Sajnani, F. Islam, R. A. Smith, V. Gopalan and A. K.-Y. Lam, Biochimie, 2017, 135, 164–172 CrossRef CAS PubMed
.
- J. W. Locasale, Nat. Rev. Cancer, 2013, 13, 572–583 CrossRef CAS PubMed
.
- S. C. Cotton, L. Sharp, J. Little and N. Brockton, Am. J. Epidemiol., 2000, 151, 7–32 CrossRef CAS PubMed
.
- K. Glunde, Z. M. Bhujwalla and S. M. Ronen, Nat. Rev. Cancer, 2011, 11, 835–848 CrossRef CAS
.
- A. N. Lane, R. M. Higashi and T. W. M. Fan, Trends Anal. Chem., 2019, 120, 115322 CrossRef
.
- J. R. Mayers, Science, 2017, 358, 1265 CrossRef
.
- C. J. Chang, Nat. Chem. Biol., 2015, 11, 744–747 CrossRef CAS PubMed
.
- D. S. Kalinowski, C. Stefani, S. Toyokuni, T. Ganz, G. J. Anderson, N. V. Subramaniam, D. Trinder, J. K. Olynyk, A. Chua, P. J. Jansson, S. Sahni, D. Lane, A. M. Merlot, Z. Kovacevic, M. Huang, S. C. Lee and D. R. Richardson, Biochim. Biophys. Acta, Mol. Cell Res., 2016, 1863, 727–748 CrossRef CAS
.
- S. V. Torti and F. M. Torti, Nat. Rev. Cancer, 2013, 13, 342–355 CrossRef CAS PubMed
.
- K. Garber, Science, 2015, 349, 129 CrossRef PubMed
.
- A. L. Gray, Analyst, 1985, 110, 551–556 RSC
.
- S. Wang, R. Brown and D. J. Gray, Appl. Spectrosc., 1994, 48, 1321–1325 CrossRef CAS
.
- P. A. Doble, R. Gonzalez de Vega, D. P. Bishop, D. J. Hare and D. Clases, Chem. Rev., 2021 DOI:10.1021/acs.chemrev.0c01219
.
- D. J. Hare, O. Shimoni and D. P. Bishop, Trends Chem., 2020, 2, 403–406 CrossRef CAS
.
- D. Hare, F. Burger, C. Austin, F. Fryer, R. Grimm, B. Reedy, R. A. Scolyer, J. F. Thompson and P. Doble, Analyst, 2009, 134, 450–453 RSC
.
- S. Theiner, E. Schreiber-Brynzak, M. A. Jakupec, M. Galanski, G. Koellensperger and B. K. Keppler, Metallomics, 2016, 8, 398–402 CrossRef CAS PubMed
.
- C. Giesen, H. A. O. Wang, D. Schapiro, N. Zivanovic, A. Jacobs, B. Hattendorf, P. J. Schüffler, D. Grolimund, J. M. Buhmann, S. Brandt, Z. Varga, P. J. Wild, D. Günther and B. Bodenmiller, Nat. Methods, 2014, 11, 417–422 CrossRef CAS
.
- H. W. Jackson, J. R. Fischer, V. R. T. Zanotelli, H. R. Ali, R. Mechera, S. D. Soysal, H. Moch, S. Muenst, Z. Varga, W. P. Weber and B. Bodenmiller, Nature, 2020, 578, 615–620 CrossRef CAS PubMed
.
- K. A. Veselkov, R. Mirnezami, N. Strittmatter, R. D. Goldin, J. Kinross, A. V. M. Speller, T. Abramov, E. A. Jones, A. Darzi, E. Holmes, J. K. Nicholson and Z. Takats, Proc. Natl. Acad. Sci. U. S. A., 2014, 111, 1216–1221 CrossRef CAS PubMed
.
- J. W. Prescott, J. Digit. Imag., 2013, 26, 97–108 CrossRef PubMed
.
- D. J. Hare, J. Lear, D. Bishop, A. Beavis and P. A. Doble, Anal. Methods, 2013, 5, 1915 RSC
.
- B. Paul, D. J. Hare, D. P. Bishop, C. Paton, V. T. Nguyen, N. Cole, M. M. Niedwiecki, E. Andreozzi, A. Vais, J. L. Billings, L. Bray, A. I. Bush, G. McColl, B. R. Roberts, P. A. Adlard, D. I. Finkelstein, J. Hellstrom, J. M. Hergt, J. D. Woodhead and P. A. Doble, Chem. Sci., 2015, 6, 5383–5393 RSC
.
- C. Paton, J. Hellstrom, B. Paul, J. Woodhead and J. Hergt, J. Anal. At. Spectrom., 2011, 26, 2508 RSC
.
- C. Austin, F. Fryer, J. Lear, D. Bishop, D. Hare, T. Rawling, L. Kirkup, A. McDonagh and P. Doble, J. Anal. At. Spectrom., 2011, 26, 1494–1501 RSC
.
- D. J. Hare, F. Fryer, B. Paul, D. P. Bishop and P. A. Doble, Anal. Methods, 2016, 8, 7552–7556 RSC
.
- D. P. Bishop, D. Clases, F. Fryer, E. Williams, S. Wilkins, D. J. Hare, N. Cole, U. Karst and P. A. Doble, J. Anal. At. Spectrom., 2016, 31, 197–202 RSC
.
- C. A. Norris, L. Danyushevsky, P. Olin and N. R. West, J. Anal. At. Spectrom., 2021, 36, 733–739 RSC
.
- J. T. v. Elteren, D. Metarapi, M. Šala, V. S. Šelih and C. C. Stremtan, J. Anal. At. Spectrom., 2020, 35, 2494–2497 RSC
.
- Z. Yurkovetsky, S. Skates, A. Lomakin, B. Nolen, T. Pulsipher, F. Modugno, J. Marks, A. Godwin, E. Gorelik, I. Jacobs, U. Menon, K. Lu, D. Badgwell, R. C. Bast Jr and A. E. Lokshin, J. Clin. Oncol., 2010, 28, 2159–2166 CrossRef PubMed
.
- D. R. Green, G. M. Green, A. S. Colman, F. B. Bidlack, P. Tafforeau and T. M. Smith, PLoS One, 2017, 12, e0186391 CrossRef
.
- T. Alexandrov, BMC Bioinf., 2012, 13, S11 CrossRef CAS PubMed
.
- F. Klauschen, S. Wienert, W. D. Schmitt, S. Loibl, B. Gerber, J.-U. Blohmer, J. Huober, T. Rüdiger, E. Erbstößer, K. Mehta, B. Lederer, M. Dietel, C. Denkert and G. v. Minckwitz, Clin. Cancer Res., 2015, 21, 3651–3657 CrossRef CAS PubMed
.
- Z.-W. Luo, M.-G. Zhu, Z.-Q. Zhang, F.-J. Ye, W.-H. Huang and X.-Z. Luo, BMC Cancer, 2019, 19, 123 CrossRef PubMed
.
- N. Melling, C. M. Kowitz, R. Simon, C. Bokemeyer, L. Terracciano, G. Sauter, J. R. Izbicki and A. H. Marx, J. Clin. Pathol., 2016, 69, 209 CrossRef CAS PubMed
.
- G. Tong, G. Zhang, J. Liu, Z. Zheng, Y. Chen, P. Niu and X. Xu, Oncol. Rep., 2020, 43, 1187–1198 CAS
.
- C. C. Compton, Mod. Pathol., 2003, 16, 376–388 CrossRef PubMed
.
- J. P. Väyrynen, S. A. Sajanti, K. Klintrup, J. Mäkelä, K. H. Herzig, T. J. Karttunen, A. Tuomisto and M. J. Mäkinen, Int. J. Cancer, 2014, 134, 2126–2135 CrossRef PubMed
.
- W. M. Wong and N. A. Wright, J. Clin. Pathol., 1999, 52, 321–333 CrossRef CAS PubMed
.
- R. G. J. Vries, M. Huch and H. Clevers, Mol. Oncol., 2010, 4, 373–384 CrossRef PubMed
.
- N. Hugen, G. Brown, R. Glynne-Jones, J. H. W. de Wilt and I. D. Nagtegaal, Nat. Rev. Clin. Oncol., 2015, 13, 361–369 CrossRef
.
-
M. B. Amin, S. Edge, F. Greene, D. R. Byrd, R. K. Brookland, M. K. Washington, J. E. Gershenwald, C. C. Compton, K. R. Hess, D. C. Sullivan, J. M. Jessup, J. D. Brierley, L. E. Gaspar, R. L. Schilsky, C. M. Balch, D. P. Winchester, E. A. Asare, M. Madera, D. M. Gress and L. R. Meyer, AJCC Cancer Staging Manual, Springer International Publishing: American Joint Commission on Cancer, 8th edn, 2017 Search PubMed
.
- V. Rodriguez-Galiano, M. P. Mendes, M. J. Garcia-Soldado, M. Chica-Olmo and L. Ribeiro, Sci. Total Environ., 2014, 476, 189–206 CrossRef PubMed
.
- M. Wu, Q. Feng, X. Wen, R. C. Deo, Z. Yin, L. Yang and D. Sheng, Hydrol. Res., 2020, 51, 648–665 CrossRef
.
- P. A. Newcomb, J. Baron, M. Cotterchio, S. Gallinger, J. Grove, R. Haile, D. Hall, J. L. Hopper, J. Jass, L. L. Marchand, P. Limburg, N. Lindor, J. D. Potter, A. S. Templeton, S. Thibodeau, D. Seminara and C. C. F. Registry, Cancer Epidemiol., Biomarkers Prev., 2007, 16, 2331–2343 CrossRef
.
- I. Winship and A. K. Win, Med. J. Aust., 2012, 197, 480–481 CrossRef PubMed
.
- C. Villalobos, D. Sobradillo, M. Hernández-Morales and L. Núñez, Biochim. Biophys. Acta, Mol. Cell Res., 2017, 1864, 843–849 CrossRef CAS PubMed
.
- J. A. Baron, B. F. Cole, R. S. Sandler, R. W. Haile, D. Ahnen, R. Bresalier, G. McKeown-Eyssen, R. W. Summers, R. Rothstein, C. A. Burke, D. C. Snover, T. R. Church, J. I. Allen, M. Beach, G. J. Beck, J. H. Bond, T. Byers, E. R. Greenberg, J. S. Mandel, N. Marcon, L. A. Mott, L. Pearson, F. Saibil and R. U. v. Stolk, N. Engl. J. Med., 2003, 348, 891–899 CrossRef CAS PubMed
.
- R. S. Sandler, S. Halabi, J. A. Baron, S. Budinger, E. Paskett, R. Keresztes, N. Petrelli, J. M. Pipas, D. D. Karp, C. L. Loprinzi, G. Steinbach and R. Schilsky, N. Engl. J. Med., 2003, 348, 883–890 CrossRef CAS PubMed
.
- S. Genoud, M. W. M. Jones, B. G. Trist, J. Deng, S. Chen, D. J. Hare and K. L. Double, Chem. Sci., 2020, 11, 8919–8927 RSC
.
- F. Davnall, C. S. P. Yip, G. Ljungqvist, M. Selmi, F. Ng, B. Sanghera, B. Ganeshan, K. A. Miles, G. J. Cook and V. Goh, Insights into Imaging, 2012, 3, 573–589 CrossRef PubMed
.
- G. Lin, K. R. Keshari and J. M. Park, Contrast Media Mol. Imaging, 2017, 2017, 1–18 CrossRef PubMed
.
- B. Bodenmiller, Cell Syst., 2016, 2, 225–238 CrossRef CAS PubMed
.
- K.-H. Yu, C. Zhang, G. J. Berry, R. B. Altman, C. Ré, D. L. Rubin and M. Snyder, Nat. Commun., 2016, 7, 12474 CrossRef CAS PubMed
.
- J. Lear, D. Hare, P. Adlard, D. Finkelstein and P. Doble, J. Anal. At. Spectrom., 2012, 27, 159–164 RSC
.
- S. J. M. V. Malderen, T. V. Acker and F. Vanhaecke, Anal. Chem., 2020, 92, 5756–5764 CrossRef PubMed
.
- A. J. Managh and P. Reid, J. Anal. At. Spectrom., 2019, 34, 1369–1373 RSC
.
- A. M. Nawi, S. F. Chin, L. Mazlan and R. Jamal, Sci. Rep., 2020, 10, 18670 CrossRef CAS
.
- M. Sohrabi, A. Gholami, M. Azar, M. Yaghoobi, M. Shahi, S. Shirmardi, M. Nikkhah, Z. Kohi, D. Salehpour, M. Khoonsari, G. Hemmasi, F. Zamani, M. Sohrabi and H. Ajdarkosh, Biol. Trace Elem. Res., 2018, 183, 1–8 CrossRef CAS
.
- H. Wang, H. Liu, M. Zhou, H. Shi and M. Shen, Biol. Trace Elem. Res., 2020, 198, 58–67 CrossRef CAS PubMed
.
- R. V. d. Plas, J. Yang, J. Spraggins and R. M. Caprioli, Nat. Methods, 2015, 12, 366–372 CrossRef PubMed
.
- K. Schwamborn and R. M. Caprioli, Nat. Rev. Cancer, 2010, 10, 639–646 CrossRef CAS PubMed
.
- H. R. Ali, H. W. Jackson, V. R. T. Zanotelli, E. Danenberg, J. R. Fischer, H. Bardwell, E. Provenzano, H. R. Ali, M. A. Sa'd, S. Alon, S. Aparicio, G. Battistoni, S. Balasubramanian, R. Becker, B. Bodenmiller, E. S. Boyden, D. Bressan, A. Bruna, B. Marcel, C. Caldas, M. Callari, I. G. Cannell, H. Casbolt, N. Chornay, Y. Cui, A. Dariush, K. Dinh, A. Emenari, Y. Eyal-Lubling, J. Fan, E. Fisher, E. A. González-Solares, C. González-Fernández, D. Goodwin, W. Greenwood, F. Grimaldi, G. J. Hannon, O. Harris, S. Harris, C. Jauset, J. A. Joyce, E. D. Karagiannis, T. Kovačević, L. Kuett, R. Kunes, A. K. Yoldaş, D. Lai, E. Laks, H. Lee, M. Lee, G. Lerda, Y. Li, A. McPherson, N. Millar, C. M. Mulvey, F. Nugent, C. H. O'Flanagan, M. Paez-Ribes, I. Pearsall, F. Qosaj, A. J. Roth, O. M. Rueda, T. Ruiz, K. Sawicka, L. A. Sepúlveda, S. P. Shah, A. Shea, A. Sinha, A. Smith, S. Tavaré, S. Tietscher, I. Vázquez-García, S. L. Vogl, N. A. Walton, A. T. Wassie, S. S. Watson, S. A. Wild, E. Williams, J. Windhager, C. Xia, P. Zheng, X. Zhuang, O. M. Rueda, S.-F. Chin, S. Aparicio, C. Caldas and B. Bodenmiller, Nat. Cancer, 2020, 1, 163–175 CrossRef
.
- P. A. Doble and G. L. Miklos, Metallomics, 2018, 10, 1191–1210 CrossRef CAS PubMed
.
- M. A. Jenkins, A. K. Win, A. S. Templeton, M. S. Angelakos, D. D. Buchanan, M. Cotterchio, J. C. Figueiredo, S. N. Thibodeau, J. A. Baron, J. D. Potter, J. L. Hopper, G. Casey, S. Gallinger, L. L. Marchand, N. M. Lindor, P. A. Newcomb, R. W. Haile, D. Ahnen, K. Anton, J. Arnold, M. Aronson, K. Aujard, B. Bapat, J. Baron, M. Barker, A. Bickerstaffe, T. Burnett, I. Cheng, J. Church, T. Church, M. Clendenning, D. Daftary, M. DeRycke, E. Dicks, A. Diep, D. Duggan, M. J. Esplen, D. Fisher, S. Fox, A. French, G. Giles, K. Glanz, J. Goldblatt, R. Goldberg, E. Goode, W. Grady, C. Greenberg, J. Green, R. Green, J. Grove, R. Gryfe, P. Harmon, E. Holowaty, S. Holter, J. Hopper, L. Keogh, H. Kim, J. Kirk, P. Lance, M. Laurino, B. Leggett, A. J. Levine, P. Limburg, J. Lowery, L. Lydum, F. Macrae, L. Madlensky, K. Makar, R. Malen, J. Maskiell, P. McAllister, E. McGannon, G. McKeown-Eyssen, J. McLaughlin, H. Miller-Pakvasa, G. Moslein, N. Nguyen, S. Nigon, P. Parafrey, S. Parry, S. Peterson, A. Phipps, A. Pollett, M. Redston, S. Rogers, R. Sandler, S. Schully, T. Selander, D. Seminara, S. Shiovitz, K. Siegmund, T. Smyrk, D. Snazel, M. Southey, J. Stubbs, G. Suthers, D. Thomas, K. Tucker, D. West, M. Woods, B. Younghusband and J. Young, Int. J. Epidemiol., 2018, 47, 387–388i CrossRef PubMed
.
-
Colon Cancer Family Registry Infomatics Center, 2021, http://coloncfr.org/, accessed 5 March 2021.
- A. T. Feldman and D. Wolfe, Methods Mol. Biol., 2014, 1180, 31–43 CrossRef CAS
.
- M. Grehn, T. Seuthe, M. Höfner, N. Griga, C. Theiss, A. Mermillod-Blondin, M. Eberstein, H. Eichler and J. Bonse, Opt. Mater. Express, 2014, 4, 689–700 CrossRef CAS
.
- J. Lear, D. J. Hare, F. Fryer, P. A. Adlard, D. I. Finkelstein and P. A. Doble, Anal. Chem., 2012, 84, 6707–6714 CrossRef CAS PubMed
.
- D. J. Hare, K. Kysenius, B. Paul, B. Knauer, R. W. Hutchinson, C. O'Connor, F. Fryer, T. P. Hennessey, A. I. Bush, P. J. Crouch and P. A. Doble, J. Visualized Exp., 2017, 55042 Search PubMed
.
Footnotes |
† Electronic supplementary information (ESI) available. See DOI: 10.1039/d1sc02237g |
‡ These authors contributed equally to this work. |
|
This journal is © The Royal Society of Chemistry 2021 |
Click here to see how this site uses Cookies. View our privacy policy here.