DOI:
10.1039/D1RA06487H
(Paper)
RSC Adv., 2021,
11, 36511-36517
Deciphering the potential anti-COVID-19 active ingredients in Andrographis paniculata (Burm. F.) Nees by combination of network pharmacology, molecular docking, and molecular dynamics†
Received
28th August 2021
, Accepted 3rd November 2021
First published on 11th November 2021
Abstract
Currently, coronavirus disease 2019 (COVID-19) caused by Severe Acute Respiratory Syndrome Coronavirus 2 has posed an enormous threat to public health worldwide. An andrographolide sulfonates preparation, named Xiyanping injection in Chinese, which was prepared from the aqueous extract of Andrographis paniculata (Burm. F.) Nees, showed favorable therapeutic effectiveness on COVID-19, suggesting A. paniculata could contain powerful therapeutic ingredients against COVID-19. In this study, to search for the potential drug candidates for COVID-19 in the herb, 68 potential target proteins and 24 active ingredients from A. paniculata were screened out using TCMSP, STP, Genecards and TTD databases firstly. A. paniculata-Compound-Target network constructed by cytoscape software showed that the protein targets PTGS2, EGFR, MAPK14, etc. had a high network relevance value. GO and KEGG enrichment analysis indicated that the 24 compounds in A. paniculata might exert their therapeutic effects by the biological processes, cellular response to biotic stimulus, response to lipopolysaccharide, response to molecule of bacterial origin, etc. And AGE-RAGE signaling pathway in diabetic complications (hsa04933), Kaposi sarcoma-associated herpesvirus infection (hsa05167), Human cytomegalovirus infection (hsa05163), etc. were predicted as the most significant effect pathways. Andrographidine C (MOL008223) and andrographolide (MOL008232) were found with strong binding affinity to the target active sites of the potential targets by molecular docking. Ultimately, the application of molecular dynamics simulations demonstrated that andrographidine C could bind well to the ACE2 and PIK3CG proteins. This research identified novel molecules against COVID-19 for developing natural medicines from A. paniculate and also provides a possible explanation for the molecular mechanisms of Xiyanping Injection against COVID-19.
Introduction
Since December 2019, the pneumonia with fever, dry cough and fatigue as the main manifestations caused by a newly identified β-coronavirus has posed a major threat to people all over the world.1 The World Health Organization named the β-coronavirus COVID-19. This virus might have been transferred to human beings via their intermediate hosts by binding to angiotensin-converting enzyme-2 (ACE-2) receptor.2 Up to now, although governments and scientists have made progress in prevention and control of this deadly pandemic, the global epidemic is still proceeding.
Herba Andrographitis, the dry aerial part of Andrographis paniculata (Burm. f.) Nees, is a medicinal plant from the family Acanthaceae for clearing away heat and toxic materials that contains more than 55 diterpene lactones, 30 flavonoids, 8 quininates and 5 rare noriridoid compounds.3,4 Modern pharmacological studies have disclosed that A. paniculata possessed anti-microbial, cytotoxicity, antiprotozoan, anti-inflammatory, and antioxidant activities.5,6 Andrographis paniculata injection, Xiyanping injection, Andrographolide drop pills and Compound Andrographis paniculata tablets were commercially made by the herb extracts exhibited remarkable antiviral effect and used widely for upper respiratory tract infections and viral pneumonia in China. Among these preparations, the andrographolide sulfonates preparation, also called Xiyanping injection in Chinese, whose active pharmaceutical ingredients are sulfonated andrographolides, has effects of antipyretic, anti-inflammatory, and antiviral.7 Especially, in the Diagnosis and Treatment Protocol for Novel Coronavirus Pneumonia (Trial Version 7),8 in China, Xiyanping injection was recommended for the treatment of severe or critically ill patients with the syndrome of flaring heat in qifen and yingfen in COVID-19, due to its clinical-confirmed efficacy on the disease.9,10 The favourable treatment effect suggests that A. paniculata could contain powerful therapeutic ingredients, which deserved to be mined for developing new drug entity against COVID-19.
In this paper, an integration strategy (Fig. 1) was carried out to dig the potential anit-COVID-19 drug candidates in A. paniculata. Firstly, the main ingredients in A. paniculata and their potential corresponding targets possibly acting on COVID-19 were screened based on target fishing and network pharmacology. After that, the combination of the ingredients-key targets binding was predicted by molecular docking. Finally, molecular dynamics (MD) simulation was utilized to further verify the docking results and speculate on the mechanism of ingredients against COVID-19. This work could gain a scientific basis for mining natural anti-COVID-19 medicines and provide insights into the effect mechanism of Xiyanping injection in the treatment of COVID-19.
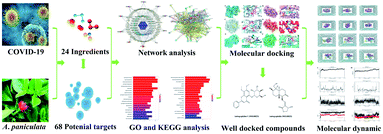 |
| Fig. 1 The whole framework based on an integration strategy in this research. | |
Experimental
Collecting the main active ingredients of A. paniculata
Oral bioavailability (OB ≥ 30%)11 and drug-likeness (DL ≥ 0.18)12 were used as screening thresholds in the Traditional Chinese Medicine Systems Pharmacology (TCMSP) database (http://tcmspw.com/) to screen out the potential active ingredients of the A. paniculate.13
Establishing database of targets
The potential active ingredients in A. paniculata and related compound targets information were preserved on TCMSP database and Swiss Target Prediction (http://www.swisstargetprediction.ch/) database.14 Known COVID-19 related targets were acquired from GeneCards (https://omim.org/) database15 and Therapeutic Targets database16 (http://bidd.nus.edu.sg/BIDD-Databases/TTD/TTD.asp). The targets of the active ingredients in A. paniculata were intersected with the COVID-19 disease targets to obtain the target genes of A. paniculata ingredients against COVID-19.
Network construction and analysis
A. paniculata-Compound-Targets network involving compositive compounds and the known therapeutic targets related COVID-19 was constructed and visualized by Cytoscape (http://cytoscape.org/ver.3.7.1)17 in order to understand the association between the A. paniculata and COVID-19 disease. Relevant degree as critical parameters to evaluate the significance of the nodes and edges on the network.
Protein–protein interaction (PPI) network also established by online STRING (https://string-db.org/) database18 (PPI score > 0.4 was considered relevant) to predict the effect mechanisms of A. paniculata multiple ingredients on COVID-19.
Pathway enrichment analysis
Gene ontology (GO) and Kyoto Encyclopedia of Genes and Genomes (KEGG) pathway enrichment analysis were performed by using the clusterProfiler package19 org.Hs.eg.db,20 and in R (version 3.5.1) to further pry into the pharmacological effects and mechanisms underlying A. paniculata acting against COVID-19 (p < 0.05).
Molecular docking
To investigate the interaction relationship and binding efficiency of putative targets to the potential active ingredients in A. paniculata, molecular docking was performed by both softwares, Sybyl-X 1.3 and Autodock vina,21 which provided the precise binding geometry of a ligand–protein complex. Both technologies were known for a high prediction performance with fast speed.
The crystal structures of the targets were downloaded from the RCSB protein data bank (www.rcsb.org/), and all crystallographic water, ions and ligands were removed before docking. Total Score and binding affinity (kcal mol−1) were calculated as measures for the assessment of binding efficiency between targets and the corresponding chemical composition, the higher Total Score or more negative affinity energy indicated the stronger binding of ligand–target complex.
Molecular dynamics
MD simulations was performed using GROMACS 2016.3 (ref. 22–24) program on the best docking conformation to evaluate the stability of the complexes. Proteins were modeled with the Amber99sb-ildn force field,25 and all small molecular compounds parameterised using acpype26 to generate the general amber force field (GAFF).27 The size of the simulated box was set so that the distance between each atom of the protein and the box was greater than 1.0 nm. The binding stability of complex in the systems was analyzed in terms of the root mean square deviation (RMSD), resultant root means square fluctuations (RMSF), the number of hydrogen bonds formed and the interaction energy.
Cell viability assay
Cell viability was assessed by MTT colorimetric assay. BEAS-2B and NIH-3T3 cells (both from the Shanghai Cell Bank of Chinese Academy of Sciences) were seeded in 96-well plates at a density of 1 × 104 cells per well. Andrographidine C (CAS: 113963-39-6, HPLC >95%, obtained from Shanghai yuanye Bio-Technology Co., Ltd) was used at final concentrations of 0–100 μM to treat cells at 37 °C for 24 h, respectively. Next, with 100 μL of 0.5 mg mL−1 MTT added into each well. This was followed by incubation with cells for 4 h at 37 °C and the addition of 150 μL DMSO. The absorbance was measured at a wavelength of 490 nm by Thermo Scientific Multiskan GO (Thermo Fisher Scientific, type: 1510, USA). The survival rate of cells was calculated as: Cell viability (%) = (OD490test − OD490blank)/(OD490control − OD490blank) × 100%.
Test of ACE2 activity
The ACE2 activities of BEAS-2B cells treated with different concentrations of andrographidine C for 24 h were tested by Human Angiotensin Converting Enzyme 2 (ACE2) ELISA Kit (Catalog #SMK3569B, Jiangsu Sumeike Biological Technology Co., Ltd) according to the manufacturer's written instructions. All data points were tested with three duplications and were expressed by mean values. Error bars represented the SD of three parallel determinations. GraphPad Prism 8.0.2 (GraphPad Software, Inc., La Jolla, CA, USA) was utilized for statistical analysis and graphing.
Results and discussion
Network pharmacology analysis
In recent years, a growing number of researches on Traditional Chinese Medicine are based on network pharmacology that has been considered as a more novel and scientific strategy for disecting the pharmacological properties of multiple components and multiple targets of drugs.28,29 A. paniculata, as a type of antipyretic-detoxicate drugs, has notable effects of anti-viral and anti-inflammatory.30,31 Besides, previous research implied that Xiyanping injection might have huge potentials to relieve some drug-induced liver damage in the therapy of COVID-19,32 but its underlying pharmacological mechanisms have not been fully elucidated due to a lack of appropriate approach. Hence, a network pharmacology approach was implemented.
A total of 49 compounds in A. paniculata were recorded in the TCMSP database, of which 24 active chemical compounds were screened as candidate compounds under the condition of OB ≥ 30% and DL ≥ 0.18. The basic information of the obtained compounds was shown in Table 1 of ESI.† 68 potential therapeutic targets associated with A. paniculata ingredients and COVID-19 were screened.
Based on the prediction of potential targets of A. paniculata ingredients in the treatment of COVID-19, a A. paniculata-Compound-Targets network was constructed using Cytoscape software. As shown in Fig. 2A, the network embodied 93 nodes and 552 edges, in which hexagon represents A. paniculata, diamond represents its main active ingredients, and ellipse represents targets. The layout of nodes is according to degree value, which goes better in large nodes. In the network, the target genes with node degree ≥8 were screened, among which the top ten target genes were PTGS2, EGFR, MAPK14, NOS2, PIK3CG, PPARG, PTGS1, PARP1, DPP4 and F10 in order. These genes were regarded as the hit targets of the 24 ingredients from A. paniculata. These targets were considered as hit targets including ACE2 that has been recommended as an available target for screening drugs. Given that proteins usually interact to achieve specific functions, thus 68 potential therapeutic targets were introduced into the STRING online website in order to investigate its interaction relationship. The PPI network showed in Fig. 2B consisted of 87 interaction nodes and 1558 interaction edges in total.
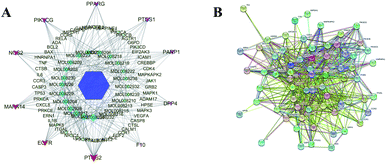 |
| Fig. 2 Network analysis. (A) A. paniculata-Compound-Targets network and (B) PPI network. | |
Enrichment of GO and KEGG pathways
GO analysis and pathway enrichment analysis were adopted to investigate the molecular mechanisms of A. paniculata ingredients acting on COVID-19. As shown in Fig. 3A, GO biological process (BP) terms were highly related to the cellular response to biotic stimulus and response to lipopolysaccharide. The top 10 pathways were listed in Fig. 3B. The KEGG pathways centered on AGE-RAGE signaling pathway in diabetic complications (hsa04933), Kaposi sarcoma-associated herpesvirus infection (hsa05167), Human cytomegalovirus infection (hsa05163), Apoptosis (hsa04210), Hepatitis B (hsa05161), Chagas disease (American trypanosomiasis) (hsa05142), C-type lectin receptor signaling pathway (hsa04625), HIF-1 signaling pathway (hsa04066), EGFR tyrosine kinase inhibitor resistance (hsa01521), etc.
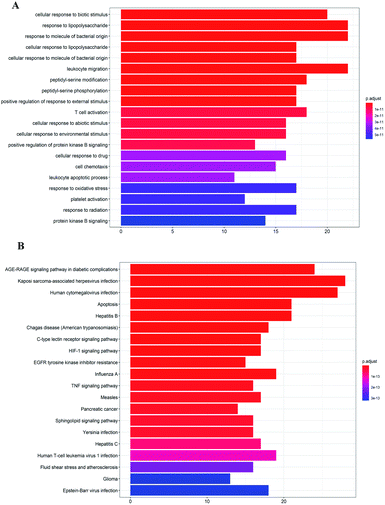 |
| Fig. 3 Enrichment analysis of GO function (A) and KEGG pathway (B). | |
Molecular docking analysis of key targets and potential active ingredients
The application of Sybyl 1.3 and AutoDock Vina elucidated the interactions between the potential active ingredients and the key targets including angiotensin-converting enzyme 2 (ACE2). Total Score and binding energy were used as measures to determine whether a ligand ingredient had a stable complex interaction with its corresponding receptors. It indicated that most of the ingredients combined well with key targets (total score ≥ 6; binding energy ≤ −7 kcal mol−1). Fig. 4 suggested that the ingredients andrographidine C (MOL008223) and andrographolide (MOL008232) could interact well with the active site pocket of its receptors with high docking Total Score and low binding energy. The structures for the two small molecular were shown in Fig. 5. Andrographidine C (MOL008223) had good affinity to PIK3CG (total score = 7.1445; binding energy = −8.3 kcal mol−1), PPARG (total score = 7.432; binding energy = −8 kcal mol−1) and ACE2 (total score = 7.411; binding energy = −8.9 kcal mol−1). Andrographolide (MOL008232) also conjugated well with some key proteins, such as PPARG (total score = 7.6012; binding energy = −7.7 kcal mol−1), MAPK14 (total score = 6.3581; binding energy = −8.7 kcal mol−1) and ACE2 (total score = 6.4494; binding energy = −8.4 kcal mol−1). Additionally, andrographolide has low binding energy to F10, NOS2, DPP4, PTGS1, and EGFR proteins with the binding energy of −8.6, −8, −7.5, −7.3, and −7 kcal mol−1 respectively, suggesting that the formed protein-ligand complex could be stable in realitics. The ingredients with favorable docking results for their potential targets by using Sybyl 1.3 and AutoDock Vina were listed in Table 2 of ESI.† In summary, it can be found that combination between andrographoside C and its potential target is much closer than andrographolide by comparing the total score and binding energy.
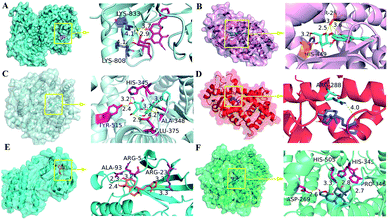 |
| Fig. 4 Analysis of the binding mode of the best docking conformation. (A) PIK3CG/MOL008223; (B) PPARG/MOL008223; (C) ACE2/MOL008223; (D) PPARG/MOL008232; (E) MAPK14/MOL008232; (F) ACE2/MOL008232. | |
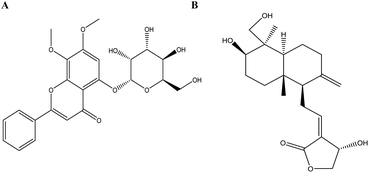 |
| Fig. 5 The Structure of potential leading compounds. (A) Andrographidine C (MOL008223); (B) andrographolide (MOL008232). | |
Analysis of molecular dynamic
Molecular docking exhibited that andrographidine C could interact very well with its related targets than andrographolide. To further predict the possibility and stability of the structure of compounds ACE2/MOL008223 and PIK3CG/MOL008223, MD simulations were conducted. The structural changes during 100 ns of MD simulations process were shown in Fig. 6. The green part represents ligands, red sphere represents sodium ions, purple represents the α-helix structure of the protein, cotton represents water molecules, yellow represents the β-fold structure of the protein, cyan and blue represent the irregular curl structure and Π spiral structure on the protein, respectively. The simulation images every 20 ns revealed that MOL008223 in both ACE2/MOL008223 and PIK3CG/MOL008223 systems could stably bound to the ACE2 and PIK3CG proteins.
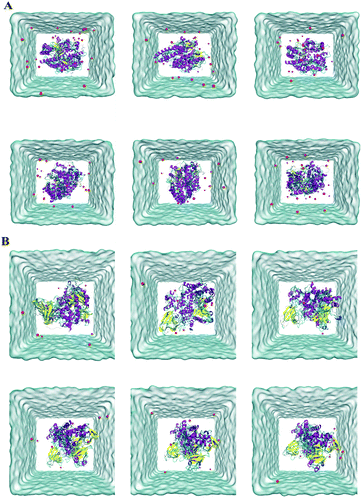 |
| Fig. 6 Schematic diagram of structural changes during 100 ns MD simulations. (A) ACE2/MOL008223 system; (B) PIK3CG/MOL008223 system. | |
RMSD values are critical indexes to reflect the local flexibility of a protein. When it fluctuates steadily, it is demonstrating that the systems reached its equilibration state. As shown in Fig. 7, the RMSD deviations of ACE2/MOL008223 and PIK3CG/MOL008223 systems were basically within the range of 0.1 nm, which provided a suitable basis for further analysis. RMSF values are also used to measure the flexibility of a certain region of the protein. The 80–90, 130–140, 330–360, 425–430, 525–570 amino acids of the ACE2 protein and 200–280, 80–420, 900–910, 1040–1060 amino acids of PIK3CG protein have larger RMSF. It indicated the instability of these fragments. In both systems, the number of hydrogen bonds formed by the ligands and the proteins was at most 7. The number of hydrogen bonds formed by ACE2 and MOL008223 was about 2–3, and the number of hydrogen bonds formed by PIK3CG and MOL008223 was about 3–4. The energy of protein-ligand interaction was mainly reflected in the electrostatic interaction energy (Coul-SR) and van der Waals force interaction energy (LJ-SR). The average electrostatic interaction energy and van der Waals interaction energy in ACE2/MOL008223 system were about −50 kcal mol−1 and −170 kcal mol−1, respectively; those in PIK3CG/MOL008223 system were about −150 kcal mol−1 and −190 kcal mol−1, respectively. The stable combination of ligand and protein in these two systems indicated that MOL008223 was very likely to produce the therapeutic effect against COVID-19 through targets proteins ACE2 and PIK3CG. Andrographidine C could well bind to most of key proteins screened by network pharmacology, especially for ACE2 and PIK3CG. The results of MD simulation showed that the binding conformation of andrographidine C with ACE2 and PIK3CG protein was stable, indicating that they had high affinity. It is concluded that andrographidine C in A. paniculata is likely to directly affect the infection and replication of COVID-19 by ACE2 and PIK3CG proteins, thereby improving lung symptoms and producing certain antiviral effects.
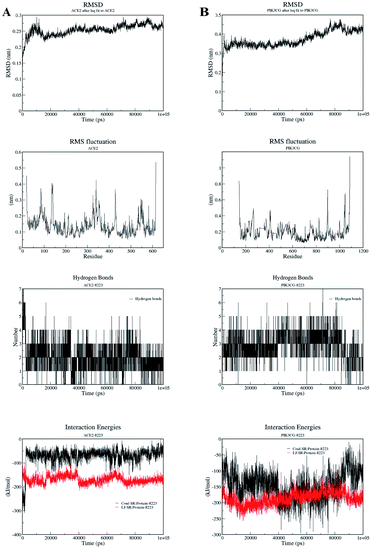 |
| Fig. 7 RMSD value, RMSF value, hydrogen bonding and interaction energy of ligand–protein complex. (A) ACE2/MOL008223 system; (B) PIK3CG/MOL008223 system. | |
Effect of andrographidine C on ACE2 activity
To further validate the in vitro activity of andrographidine C, the cytotoxicity of andrographidine C was first analysed. The effect of andrographidine C on the cell viability of BEAS-2B and NIH-3T3 cells was examined by MTT. BEAS-2B and NIH-3T3 cells were incubated with andrographidine C at different doses for 24 h. As shown in Fig. 8A, after pretreatment for 24 h, the effect on the viability of BEAS-2B and NIH-3T3 cells in the range of 0–80 μM was not significant, but at a concentration of 100 μM, cell viability would be slightly reduced. Given that the viability of cell lines showed a minimal influence after incubation with 0–20 μM, the intervention of andrographidine C at the concentration of 1, 5, 10 and 20 μM was conducted. The effect of andrographiside C on ACE2 activity was assayed using the ACE2 Activity Assay Kit. As shown in Fig. 8 B, 1, 5, 10 and 20 μM of andrographidine C all resulted in a significant decrease in ACE2 protein activity in BEAS-2B (***p < 0.01). Results of virtual screening predictions combined with ACE2 activity analysis showed that andrographidine C could bind to ACE2, occupying four active sites, HIS-345, HIS-505, PRP-346 and ASP-269, and generating four hydrogen bonds with surrounding amino acid residues, affecting its binding to substrates and showing a decrease in enzyme activity. Andrographidine C plays a potential role in the inhibition of SARS-CoV-2 by reducing ACE2 activity and affecting the binding of ACE2 to spike protein of SARS-CoV-2, which displays a great potential against COVID-19.
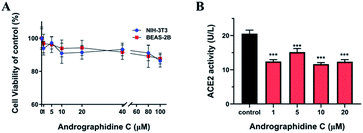 |
| Fig. 8 Effect of andrographidine C on ACE2 activity. (A) Changes in cell viability after pretreatment of BEAS-2B and NIH-3T3 cells with various concentrations of andrographidine C. (B) andrographidine C inhibits AEC2 activity in BEAS-2B cells. Each set Each set of test values was compared with the control column and subjected to a one-way ANOVA. ***p < 0.01 as indicated. | |
Conclusion
In conclusion, a comprehensive in silico approach was developed to decipher the active ingredients of A. paniculata and their possible effect mechanism against COVID-19 from the molecular level. 11 hit targets and 24 potential ingredients were excavated based on network pharmacology. Furthermore, molecular docking and molecular dynamic techniques were employed to evaluate binding properties between candidate molecules and their related proteins. The ingredient andrographidine C formed a stable complex with the key target ACE2 could be considered as a drug candidate against COVID-19. However, further experimental verification is required for the results from this research based on computational analysis.
Author contributions
Conceptualization, H. Y., P. S. and J. H.; methodology, R. X.; software, H. Y.; validation, R. X., Z. L. and C. Z.; formal analysis, L. H.; investigation, R. X.; resources, H. Y.; data curation, P. S.; writing—original draft preparation, R. X.; writing—review and editing, H. Y., P. S. and Y. W.; visualization, Z. L. and S. L.; supervision, B. C.; project administration, H. Y.; funding acquisition, H. Y. and J. H. All authors have read and agreed to the published version of the manuscript.
Conflicts of interest
There are no conflicts to declare.
Acknowledgements
This work was supported by National Natural Science Foundation of China (81973558), key project supported by the Natural Science Foundation of Fujian province (2021J02033) and the Joint Funds for the Innovation of Science and Technology, Fujian province (2019Y9068), China.
References
- W. J. Guan, Z. Y. Ni, Y. Hu, W. H. Liang, C. Q. Ou, J. X. He, L. Liu, H. Shan, C. L. Lei, D. S. C. Hui, B. Du, L. J. Li, G. Zeng, K. Y. Yuen, R. C. Chen, C. L. Tang, T. Wang, P. Y. Chen, J. Xiang, S. Y. Li, J. L. Wang, Z. J. Liang, Y. X. Peng, L. Wei, Y. Liu, Y. H. Hu, P. Peng, J. M. Wang, J. Y. Liu, Z. Chen, G. Li, Z. J. Zheng, S. Q. Qiu, J. Luo, C. J. Ye, S. Y. Zhu, N. S. Zhong and C. China Medical Treatment, N. Engl. J. Med., 2020, 382, 1708–1720 CrossRef CAS PubMed.
- P. Zhou, X. L. Yang, X. G. Wang, B. Hu, L. Zhang, W. Zhang, H. R. Si, Y. Zhu, B. Li, C. L. Huang, H. D. Chen, J. Chen, Y. Luo, H. Guo, R. D. Jiang, M. Q. Liu, Y. Chen, X. R. Shen, X. Wang, X. S. Zheng, K. Zhao, Q. J. Chen, F. Deng, L. L. Liu, B. Yan, F. X. Zhan, Y. Y. Wang, G. F. Xiao and Z. L. Shi, Nature, 2020, 579, 270–273 CrossRef CAS PubMed.
- W. W. Chao and B. F. Lin, China's Med., 2010, 5, 17 CrossRef PubMed.
- M. S. Hossain, Z. Urbi, A. Sule and K. M. Hafizur Rahman, Sci. World J., 2014, 2014, 274905 Search PubMed.
- C. V. Chandrasekaran, A. Gupta and A. Agarwal, J. Ethnopharmacol., 2010, 129, 203–207 CrossRef CAS PubMed.
- P. K. Singha, S. Roy and S. Dey, Fitoterapia, 2003, 74, 692–694 CrossRef PubMed.
- H. Shi, W. Guo, H. Zhu, M. Li, C. O. L. Ung, H. Hu and S. Han, J. Evidence-Based Complementary Altern. Med., 2019, 2019, 4510591 Search PubMed.
- National Health Commission & National Administration of Traditional Chinese Medicine, China Med. J., 2020, 133, pp. 1087–1095 Search PubMed.
- G. Zhang, Y. Hou, Y. Li, L. He, L. Tang, T. Yang, X. Zou, Q. Zhu, S. Yan, B. Huang, J. Zhao and J. Huang, J. Tradit. Chin. Med., 2017, 37, 397–403 CrossRef PubMed.
- S.-g. Sun, Y.-f. Shi, H. Yan, Y. Li, R. Wang, S.-h. Wang and X.-d. Sun, Chin. Herb. Med., 2015, 7, 173–178 CrossRef.
- X. Xu, W. Zhang, C. Huang, Y. Li, H. Yu, Y. Wang, J. Duan and Y. Ling, Int. J. Mol. Sci., 2012, 13, 6964–6982 CrossRef CAS PubMed.
- W. P. Walters and M. A. Murcko, Adv. Drug Delivery Rev., 2002, 54, 255–271 CrossRef CAS PubMed.
- J. Ru, P. Li, J. Wang, W. Zhou, B. Li, C. Huang, P. Li, Z. Guo, W. Tao, Y. Yang, X. Xu, Y. Li, Y. Wang and L. Yang, J. Cheminf., 2014, 6, 13 Search PubMed.
- D. Gfeller, A. Grosdidier, M. Wirth, A. Daina, O. Michielin and V. Zoete, Nucleic Acids Res., 2014, 42, W32–W38 CrossRef CAS PubMed.
- G. Stelzer, N. Rosen, I. Plaschkes, S. Zimmerman, M. Twik, S. Fishilevich, T. I. Stein, R. Nudel, I. Lieder, Y. Mazor, S. Kaplan, D. Dahary, D. Warshawsky, Y. Guan-Golan, A. Kohn, N. Rappaport, M. Safran and D. Lancet, Curr. Protoc. Bioinf., 2016, 54, 1.30.1–1.30.33 Search PubMed.
- Y. H. Li, C. Y. Yu, X. X. Li, P. Zhang, J. Tang, Q. Yang, T. Fu, X. Zhang, X. Cui, G. Tu, Y. Zhang, S. Li, F. Yang, Q. Sun, C. Qin, X. Zeng, Z. Chen, Y. Z. Chen and F. Zhu, Nucleic Acids Res., 2018, 46, D1121–D1127 CrossRef CAS PubMed.
- P. Shannon, A. Markiel, O. Ozier, N. S. Baliga, J. T. Wang, D. Ramage, N. Amin, B. Schwikowski and T. Ideker, Genome Res., 2003, 13, 2498–2504 CrossRef CAS PubMed.
- D. Szklarczyk, A. L. Gable, D. Lyon, A. Junge, S. Wyder, J. Huerta-Cepas, M. Simonovic, N. T. Doncheva, J. H. Morris, P. Bork, L. J. Jensen and C. V. Mering, Nucleic Acids Res., 2019, 47, D607–D613 CrossRef CAS PubMed.
- G. C. Yu, L. G. Wang, Y. Y. Han and Q. Y. He, Omics, 2012, 16, 284–287 CrossRef CAS PubMed.
- M. C. org and H. eg.db, Genome wide annotation for Human. 2015 Search PubMed.
- O. Trott and A. J. Olson, J. Comput. Chem., 2010, 31, 455–461 CAS.
- H. J. C. Berendsen, D. van der Spoel and R. van Drunen, Comput. Phys. Commun., 1995, 91, 43–56 CrossRef CAS.
- D. Van Der Spoel, E. Lindahl, B. Hess, G. Groenhof, A. E. Mark and H. J. Berendsen, J. Comput. Chem., 2005, 26, 1701–1718 CrossRef CAS PubMed.
- M. J. Abraham, T. Murtola, R. Schulz, S. Páll, J. C. Smith, B. Hess and E. Lindahl, SoftwareX, 2015, 1–2, 19–25 CrossRef.
- K. Lindorff-Larsen, S. Piana, K. Palmo, P. Maragakis, J. L. Klepeis, R. O. Dror and D. E. Shaw, Proteins, 2010, 78, 1950–1958 CrossRef CAS PubMed.
- A. W. Sousa da Silva and W. F. Vranken, BMC Res. Notes, 2012, 5, 367 CrossRef PubMed.
- K. L. Meagher, L. T. Redman and H. A. Carlson, J. Comput. Chem., 2003, 24, 1016–1025 CrossRef CAS PubMed.
- X. Li, Y. Hou, X. Wang, Y. Zhang, X. Meng, Y. Hu and Y. Zhang, Biol. Pharm. Bull., 2020, 43, 296–305 CrossRef CAS PubMed.
- F. Xia, C. Liu and J. B. Wan, Phytomedicine, 2020, 74, 153071 CrossRef CAS PubMed.
- C. Wiart, K. Kumar, M. Y. Yusof, H. Hamimah, Z. M. Fauzi and M. Sulaiman, Phytother. Res., 2005, 19, 1069–1070 CrossRef CAS PubMed.
- L. Liu, Y. Yan, L. Zheng, H. Jia and G. Han, Nat. Prod. Res., 2020, 34, 782–789 CrossRef CAS PubMed.
- M. Rehan, F. Ahmed, S. M. Howladar, M. Y. Refai, H. M. Baeissa, T. A. Zughaibi, K. M. Kedwa and M. S. Jamal, Front. Immunol., 2021, 12, 648250 CrossRef CAS PubMed.
Footnotes |
† Electronic supplementary information (ESI) available. See DOI: 10.1039/d1ra06487h |
‡ These authors contributed equally to this work. |
|
This journal is © The Royal Society of Chemistry 2021 |