DOI:
10.1039/D1RA05327B
(Paper)
RSC Adv., 2021,
11, 38868-38879
Whole genome sequencing for revealing the point mutations of SARS-CoV-2 genome in Bangladeshi isolates and their structural effects on viral proteins†
Received
11th July 2021
, Accepted 30th October 2021
First published on 3rd December 2021
Abstract
Coronavirus disease-19 (COVID-19) caused by SARS-CoV-2 has already killed more than one million people worldwide. Since novel coronavirus is a new virus, mining its genome sequence is of crucial importance for drug/vaccine(s) development. Whole genome sequencing is a helpful tool in identifying genetic changes that occur in a virus when it spreads through the population. In this study, we performed complete genome sequencing of SARS-CoV-2 to unveil the genomic variation and indel, if present. We discovered thirteen (13) mutations in Orf1ab, S and N gene where seven (7) of them turned out to be novel mutations from our sequenced isolate. Besides, we found one (1) insertion and seven (7) deletions from the indel analysis among the 323 Bangladeshi isolates. However, the indel did not show any effect on proteins. Our energy minimization analysis showed both stabilizing and destabilizing impact on viral proteins depending on the mutation. Interestingly, all the variants were located in the binding site of the proteins. Furthermore, drug binding analysis revealed marked difference in interacting residues in mutants when compared to the wild type. Our analysis also suggested that eleven (11) mutations could exert damaging effects on their corresponding protein structures.
Introduction
COVID-19 can be currently considered a menace to humankind brought about by the novel Severe Acute Respiratory Syndrome Coronavirus-2 (SARS-CoV-2) which began its journey from the Wuhan province of the People's Republic of China.1–8 The infection basically targets the respiratory framework of its host causing influenza-like sickness with symptoms such as cough, fever, and in progressively serious cases, troubled breathing.9–15 According to the data available, mortality is higher in individuals of advanced ages (>60 years) and the ones with comorbidities.16–21 Apart from intense respiratory problems, COVID-19 has been shown to cause systemic irritation prompting sepsis,22–25 intense cardiovascular injury,26–31 cardiovascular breakdown26,32–35 and multiorgan failure in critical patients.36 COVID-19 has been rightly announced as a global pandemic by the World Health Organization (WHO) as it has spanned over 200 countries and territories around the world.37–42
Coronaviruses (CoVs) are enveloped, single-stranded, (+) RNA viruses that are pathogenic to their hosts.43–47 SARS-CoV-2 is the causative agent behind COVID-19 and is more pathogenic in contrast with previously observed SARS-CoV (2002) and Middle East respiratory syndrome coronavirus (MERS-CoV, 2013).48–57 There is a dire need to examine the virus more comprehensively to analyze the pathogenesis, its destructiveness and development of powerful therapeutic measures.58 CoVs belongs to the Coronaviridae family under Nidovirales order. They have been grouped into four genera that belong to α-, β-, γ-, and δ-coronaviruses.59 Among them, α-and β-COVs infect vertebrates, γ-coronaviruses avians, while the δ-coronaviruses infect both. SARS-CoV, mouse hepatitis coronavirus (MHV), MERS-CoV, Bovine coronavirus (BCoV), bat coronavirus HKU4, and human coronavirus OC43, including SARS-CoV-2, are β-coronaviruses.60 Zoonotic transmission is the medium of transmission for each of the three CoVs, SARS-, MERS-, and SARS-CoV-2, and they spread through close contact. The essential multiplication number (R0) of the individual-to-individual spread of SARS-CoV-2 is around 2.2–2.7, which implies that the confirmed cases develop at a striking exponential rate.61 CoVs being 26 to 32 kb long have the biggest RNA viral genome.62 The SARS-CoV-2 genome share approximately 90% identity with essential enzymes and structural proteins of SARS-CoV. Fundamentally, SARS-CoV-2 contains four basic proteins known as-spike (S), envelope (E), membrane (M), and nucleocapsid (N) proteins. These proteins share high sequence similarity with the sequence of the corresponding proteins in SARS-CoV, and MERS-CoV. Hence, it is vital to scrutinize the SARS-CoV-2 genome to determine why this infection is progressively inclined to be more infectious and lethal than its predecessors.
Utilizing Sanger sequencing and cutting-edge whole genome sequencing of SARS-CoV-2 isolates from oropharyngeal samples, we depicted the genomic portraits of two genomes alongside other Bangladeshi strains.63
In this study, we have analyzed the genomic arrangements of SARS-CoV-2 to identify the mutations found within the genomes and anticipate their effect on the protein structure from a structural biology perspective in order to shed light on the suitable therapeutics against this deadly virus.
Methods
Virus isolation
The oropharyngeal samples from two COVID-19 patients were collected. Two viral genome SARS-CoV-2/human/BGD/NIB_01/2020 and SARS-CoV-2/human/BGD/NIB-BCSIR_02/2020 were obtained using the UTM™ kit containing 1 mL of viral transport media (Copan Diagnostics Inc., Murrieta, CA, USA) on day 7 of the patient's illness with symptoms of cough, mild fever, and throat congestion. The specimens were tested positive for SARS-CoV-2 by real-time reverse transcriptase PCR (rRT-PCR). Then, the viral RNA was extracted directly from the patient's swab using PureLink Viral RNA/DNA Mini kit (Invitrogen). The viral RNA was then converted into cDNA using SuperScript™ VILO™ cDNA synthesis kit (Invitrogen) according to the manufacturer's instructions.
DNA sequencing
Sanger dideoxy based sequencing. The forty eight (48) pair primers were designed to cover the whole genome of the virus by following two conditions: (1) their sequence is conserved among all the available SARS-CoV-2 isolates and (2) the terminal of the amplicons will overlap with neighboring amplicons. The polymerase chain reaction (PCR) was performed and the 48 primers then generated 47 amplicons which were visualized in 1.5% agarose gel electrophoresis. The amplicons were further purified using Purelink PCR purification kit (ThermoFisher Scientific, USA). These purified amplicons were sequenced using Sanger dideoxy method by “ABI 3500” with BigDye Terminator version 3.1 cycle sequencing kit (Applied Biosystems, USA). The raw reads were assembled by DNA Baser (https://www.dnabaser.com) and verified by SeqMan Pro®. Version 14.1. DNASTAR.64 Madison, WI. These overlapping regions were visualized by CLC Genomics Workbench 20.0.4 (https://digitalinsights.qiagen.com) and merged with EMBOSS: merger (https://www.bioinformatics.nl/cgi-bin/emboss/merger).
Next generation sequencing
Illumina Nextseq 550 next-generation sequencing technology was implemented to sequence the complete genome of the SARS-CoV-2/human/BGD/NIB-BCSIR_02/2020 virus to where Nextera DNA Flex was utilized as library preparation kit for the synthesis of the nucleotides.65 To cover the 300 cycle, the NextSeq High Output kit was utilized as the reagent cartridge. To generate the FASTQ data workflow the run mode was set as local run manager in every NextSeq 4-channel chemistry. Analysis and quality check was performed using a customized version of the DRAGEN RNA pipeline, which was also available on local DRAGEN server hardware. The Illumina® DRAGEN RNA Pathogen Detection App uses a combined human and virus reference to analyze pathogen data. The raw reads were cleaned by trimming low-quality bases with Trimmomatic 0.36 (-phred33, LEADING:20, TRAILING:20, SLIDCitation). The assembly was performed by the utilization of SPAdes using default parameters as well as used to cross-validate with the reference-based method as an internal control. The assembly statistics were executed by QUAST.66
Variant identification
Basic Local Alignment Search Tools (BLAST) was employed to identify possible mutations in Sanger Based sequenced nucleotide sequences. Nucleotide program of blast was selected for this identification. The mapped polymorphisms were investigated for their frequency worldwide and checked for their profile at China National Center for Bioinformation (CNCB) (https://www.cncb.ac.cn/) resource. Chimera was utilized to visualize the mapped polymorphisms. Besides, all the available Bangladeshi strains (n = 323) of SARS-CoV-2 were retrieved from GISAID67 and further explored to find out the most common mutations.68
Protein modeling and mutational effect analysis
To observe the mutational effect of the polymorphisms in 3 dimensional (3D) structure, homology modeling were executed using deep learning based RoseTTAfold algorithm in the ROBETTA server.69 Later, the difference of the energy was calculated by Gromos96 in both wild type and mutant 3D structures to estimate the structural abnormality and change in stability.70 Binding site of both wild and mutant structures were analyzed to check whether the amino acid residues are into the binding site region or not.
Virtual screening and molecular docking
Remdisivir and Ivermectin were selected since these drugs were suggested by DrugBank Protein Basic Local Alignment Tool (BLASTp) (https://go.drugbank.com/structures/search/bonds/sequence). We retrieved the structures of all the interacting drugs (.pdb files) by virtual screening of the Drugbank database. We have performed molecular docking simulation using Autodock vina71 for the analysis of interacting residues to the druggable targets. At first, we generated the .pdbqt files of the targets (both mutant and wild types) for docking experiments. After that blind docking was performed for the identification of the most effective binding site of these drugs. The grid box parameter covered the whole protein for all docking runs. Finally, Autodock vina predicted the drug receptor interactions. This interactions were visualized via UCSF Chimera, Pymol and Discovery Studio Visualizer.
Molecular dynamic simulation
In order to evaluate the evaluate the impact of novel mutations on the stability of the SARS-CoV-2 proteins under physiological conditions, 50 ns molecular dynamics simulation was carried out using GROningen MAchine for Chemical Simulations aka GROMACS (version 5.1.1). The GROMOS96 43a1 force-field, 300 K temperature, pH 7.4, and 0.9% NaCl was used for building the system. It was then solvated in a triclinical box of the simple point charge water model with its edges at 0.5 nm distance from the protein surface. The overall charge of the system was neutralized using necessary ions using the genion module. Energy minimization of the neutralized system was carried out using the steepest descent minimization algorithm with maximum number of minimization steps to perform was set at 50
000. The ligand was restrained before carrying out the isothermal-isochoric (NVT) equilibration of the system for 100 ps with short-range electrostatic cutoff value of 1.2 nm. Isobaric (NPT) equilibration of the system was carried out for 100 ps following the NVT with short-range van der Waals cutoff fixed at 1.2 nm. Finally, a 50 ns molecular dynamic simulation was run using periodic boundary conditions and time integration step of 2 fs. The energy of the system was saved every 100 ps. For calculating the long-range electrostatic potential, the Particle Mesh Ewald (PME) method was applied. Short-range van der Waals cutoff was kept at 1.2 nm. Modified Berendsen thermostat was used to control simulation temperature while the pressure was kept constant using the Parrinello-Rahman algorithm. The simulation time step was selected as 2.0 fs. The snapshot interval was set to 100 ps for analyzing the trajectory data. Finally, all of the trajectories were concatenated to calculate and plot root mean square deviation (RMSD), root mean square fluctuation (RMSF), radius of gyration (Rg) and solvent accessible surface area (SASA) data. MD simulations were performed on the “bioinfo-server” running on Ubuntu 18.4.5 operating system located at the Bioinformatics Division, National Institute of Biotechnology.
Root Mean Square Deviation (RMSD) calculation was performed in order to evaluate when a system attains equilibrium. The “rms” module built into the GROMACS software was utilized to extract RMSD information throughout the course of the simulation. The result can be plotted graphically using the Xmgrace package.
Room Mean Square Fluctuation (RMSF) is used to determine the flexibility of a certain region of the protein. The higher the RMSF value the higher is the flexibility of an amino acid. RMSF calculations were carried out using the “rmsf” module and the figures were generated using Xmgrace.
The radius of gyration of our proteins was measured to determine its degree of compactness. A relatively steady value of radius of gyration means stable folding of a protein. Fluctuation of radius of gyration implies the unfolding of the protein. The “gyrate” module was used to generate the radius of gyration graphs for our proteins.
Hydrophobic interactions composed of non-polar amino acids are crucial for maintaining the stability of the hydrophobic core of proteins. They do so by covering the non-polar amino acids within the hydrophobic cores and keeping them at a distance from the solvent. Solvent Accessible Surface Area (SASA) is used in molecular dynamic simulations to predict the hydrophobic core stability of proteins. In this study, SASA was calculated using the “sasa” module and the resulting graph was visualized using Xmgrace.
Results
Revealing the SARS-CoV-2 genome
The workflow of this manuscript has been shown in Fig. 1.
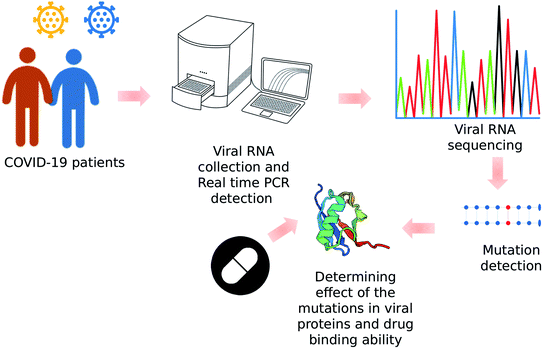 |
| Fig. 1 Workflow of the study. | |
In case of NIB-01 virus, forty eight (48) contigs with ninety four (94) overlapping regions were obtained. The sequence had 2X coverage (both forward and reverse reads). It was then assembled by SeqMan Pro and EMBOSS merger.72 The assembled viral genome consisted of a single stranded positive (+) RNA that is 29
724 nucleotides long with: 8882 adenosines (29.88%), 5455 cytosine (18.35%), 5836 guanine (19.63%), and 9551 thymine (32.13%). The GC content of the whole genome was 38%. A total of 17
822
898 reads were produced in the reference-based alignment after trimming 99% of them were mapped to the SARS-CoV-2 reference genome.
The complete nucleotide sequence of SARS-CoV-2 isolate SARS-CoV-2/human/BGD/NIB_01/2020 from the Sanger sequencing has been deposited in GenBank under the accession number MT509958 (https://www.ncbi.nlm.nih.gov/nuccore/MT509958). The complete nucleotide sequence of SARS-CoV-2/human/BGD/NIB-BCSIR_02/2020 isolate from the NGS has been also deposited under the accession number MT568643 (https://www.ncbi.nlm.nih.gov/nuccore/MT568643.1?report=genbank). The contig length for NIB_01/202 and NIB-BCSIR_02/2020 were 29
724 and 29
737 bases respectively. Detail statistics are given ESI File 1.†
Exploration of variant in the sequenced isolates
We have found thirteen (13) mutations at the 93rd; C → T, 479th; T → A, 481st; C → A; 1015th; A → T, 2889th; C → T, 5098th; G → T; 5237th; C → T, 5642nd; G → T, 8023rd; G → A, 23
255th; A → G 28
733rd; G → A, 28
734th; G → A and 28
735th; G → C from the MT509958 whole genome (Table 1). However, the MT568643 whole genome showed no mutation against the reference sequence. From them, six (6) mutations namely 93rd; C → T, 2889th; C → T, 23
255th; A→G, 28
733rd; G → A, 28
734th; G → A and 28
735th; G → C were found in CNCB resource where the available mutations of SARS-CoV-2 were enlisted (Table 1). These mutations have already been found in different countries where the SARS-CoV-2 has been sequenced. These mutations were mostly found in the United States of America (USA) and the United Kingdom (UK) (Table 1). The position of 93rd; C → T mutation is located in 5′UTR upstream region. And the position 2889th; C → T mutation has shown no change to its protein sequence. The other 23
255th; A→G, 28
733rd; G → A, 28
734th; G → A and 28
735th; G → C mutations can alter the amino acid sequence and can have the missense effect on the protein (Table 1). Apart from these 6 mutations, seven (7) mutations have shown as unique variants against the reference sequence (Table 1 and Fig. 2). These mutations were not previously reported. Besides, we analyzed our assemble genome to look for any insertion/deletions but these two genomes contain no deletions/insertions. However, we have identified one (1) insertion and seven (7) deletions of the eight (8) Bangladeshi strain EPI_ISL_466692, EPI_ISL_450343, EPI_ISL_450344, EPI_ISL_468074, EPI_ISL_514614, EPI_ISL_445213, EPI_ISL_445217 and EPI_ISL_450842 (Table 2 and Fig. 3). We additionally scrutinized these deleted regions but we didn't find any domain or motif on this region. Apart from our reported complete genomes, we have also identified the most common mutations occurred in Bangladesh from the complete genomes reported in GSAID database from Bangladesh. These genomes showed three mutations in the positions 14
408, 23
403 and 28
878 compared to reference genome (Table 1).
Table 1 NIB_01 polymorphisms against reference sequence and their mutational effect
S. no. |
Query (reference) position |
Position in subject (NIB-01) sequence |
Amino acid |
Query → subject |
Gene |
Mutants observed |
Mutants observed in no. in virus |
Impact on proteins |
Protein ID of mutants |
First date of detection in GISAID |
1 |
241st |
93 |
— |
C → T |
5′UTR |
US, UK |
15 042 |
Upstream variant |
QHD43415.1 |
Not available |
2 |
627th |
479 |
Val121 |
T → A |
Orf1ab |
— |
— |
— |
— |
Not available |
3 |
629th |
481 |
Leu122 |
C → A |
Orf1ab |
— |
— |
— |
— |
Not available |
4 |
1163rd |
1015 |
Ile120 |
A → T |
Orf1ab |
— |
— |
Coding variant |
— |
March, 2020 in hCoV-19/France/IDF_HB_112003047702/2020 |
5 |
5246th |
5098 |
Val843 |
G → T |
Orf1ab |
— |
— |
Coding variant |
QHD43415.1 |
May 2020 in hCoV-19/Bangladesh/NIB-01/2020 |
6 |
5385th |
5237 |
Ala889 |
C → T |
Orf1ab |
— |
— |
Coding variant |
QHD43415.1 |
May 2020 in hCoV-19/Bangladesh/NIB-01/2020 |
7 |
7790th |
5642 |
Gly1691 |
G → T |
Orf1ab |
— |
— |
Coding variant |
QHD43415.1 |
First found in May 2020 from hCoV-19/Bangladesh/NIB-01/2020 |
From Later Update/submission: in April 2020, from hCoV-19/Canada/AB-50997/2020 |
8 |
8171st |
8023 |
Ala1818 |
G → A |
Orf1ab |
— |
— |
Coding variant |
QHD43415.1 |
March 2020 in hCoV-19/England/GSTT-265DF65/2020. |
9 |
23 403rd |
23 255 |
Asp614 |
A → G |
“S” |
US, UK |
15 118 |
Coding variant |
QHD43416.1 |
January 2020 in hCoV-19/Japan/20200409-129/2020 |
10 |
28 882nd |
28 734 |
Arg203 |
G → A |
“N” |
UK |
4542 |
Coding variant |
QHD43423.2 |
January 2020 in hCoV-19/Japan/20200409-129/2020 |
11 |
28 883rd |
28 735 |
Gly204 |
G → C |
“N” |
UK |
4537 |
Coding variant |
QHD43423.2 |
January 2020 in hCoV-19/Japan/20200409-129/2020. |
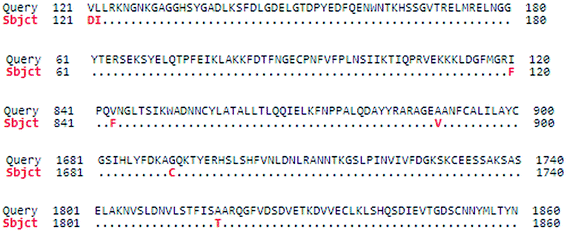 |
| Fig. 2 Novel mutations in the protein sequence in NIB_01 whole genome. In total, seven novel mutations was identified. | |
Table 2 Indel profile of Bangladeshi whole genome
Serial no. |
Sequence identifier |
Indel type |
Region |
1 |
hCoV-19/Bangladesh/BCSIR-NILMRC-071/2020|EPI_ISL_466692|2020-05-26 |
Insertion |
ORF8 (27 910–27 985) |
2 |
hCoV-19/Bangladesh/BARJ-CVASU-CTG-511/2020|EPI_ISL_450343|2020-05-09 |
Deletion |
ORF8 (27 913–28 254) |
3 |
hCoV-19/Bangladesh/BARJ-CVASU-CTG- 517/2020|EPI_ISL_450344|2020-05-03 |
Deletion |
ORF8 (27 913–28 254) |
4 |
hCoV-19/Bangladesh/CHRF-0006/2020|EPI_ISL_468074|2020-05-17 |
Deletion |
ORF8 (27 913–28 254) |
5 |
hCoV-19/Bangladesh/NGRI-NSTU-31/2020|EPI_ISL_514614|2020-07-21 |
Deletion |
ORF8 (27 913–28 254) |
6 |
hCoV-19/Bangladesh/DNAS-CPH-467/2020|EPI_ISL_445213|2020-04-28 |
Deletion |
ORF7 (27 476–27 668) |
7 |
hCoV-19/Bangladesh/DNAS-CPH-436/2020|EPI_ISL_445217|2020-04-28 |
Deletion |
ORF7 (27 476–27 668) |
8 |
hCoV-19/Bangladesh/DU-50761/2020|EPI_ISL_450842|2020-05-06 |
Deletion |
ORF7 (27 476–27 668) |
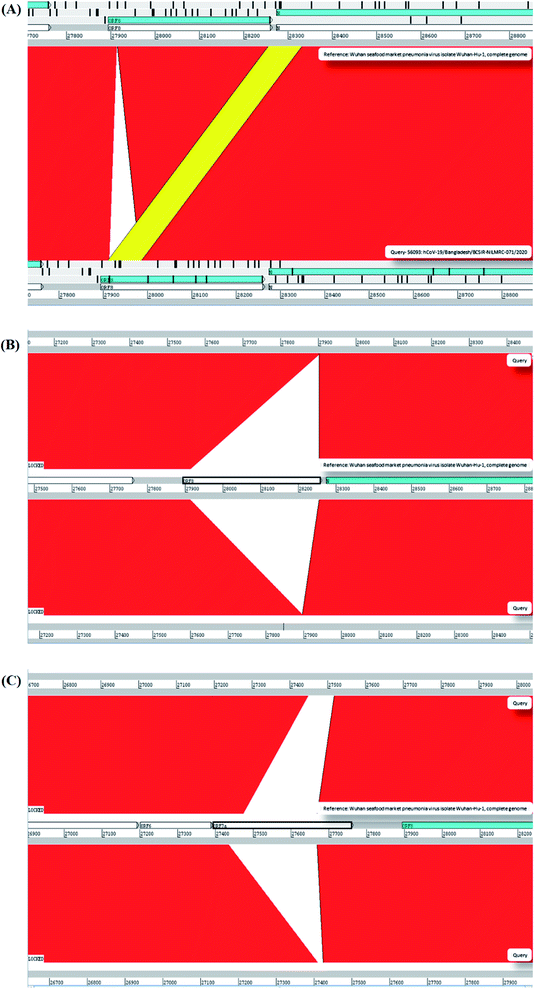 |
| Fig. 3 Deletion of sequences in complete genome of the Bangladesh strain. Indel experimentation result from comparative genome browsing against reference genome in Artemis window. (a) Mobile element insertion from n to ORF8 in EPI_ISL_466692. Yellow bar representing homologous sequence moiety between these two regions. (b) EPI_ISL_450343, EPI_ISL_450344, EPI_ISL_468074, EPI_ISL_514614 are sharing the same deletion pattern in ORF8. Majority portion of ORF8, lost in the deletion event. (c) EPI_ISL_445213, EPI_ISL_445217 and EPI_ISL_450842 are also sharing the same deletion pattern in Orf7A. Majority portion of Orf7A, lost in the deletion event. | |
Point mutations alter respective protein structures
We have analyzed the mutational effect of all the mutations. Therefore, the 3D structure was built to explore the mutational effect on the protein structure (Fig. 4). In this case, two types of 3D structure was built (i) the structure with wild type residue and (ii) the structure with mutant residue. We have performed the energy minimization of both the wild type and mutants. We have found significant differences in the stability of the structure upon mutation (Table 3). Mutants 479th; T → A and 1015th; A → T showed higher energy minimization which predicted these proteins to be more stable than the wild type. All the other protein models based on mutation showed less energy minimization than the wild type protein model. Therefore, these protein structures could be more unstable upon mutation in the protein sequence. The highest difference was observed in the mutation in the 5642nd position; G → T mutation (from −23
276.78 kJ mol−1 to −22
377.976 kJ mol−1) (Table 3). Afterwards, the binding site was analyzed to determine whether the wild type and the mutant residues fell within the ligand binding site or not. The binding site residues confirmed that all the mutations from the complete genome belonged to the binding site region (Fig. 5). Later, we have performed the drug binding analysis followed by virtual drug screening in DrugBank server. Ivermectin and Remdisivir drugs topped the list of potential drug candidates. We then prepared the protein structures and converted them to .pdbqt format for molecular docking experiment. We identified the binding site region for each protein and set the grid box to allow the drugs only to bind to that specific region. The binding affinity analysis showed that compared to the wild type, the drug Ivermectin bound with higher score to the proteins which has mutation at positions 479 (T → A), 5642 (G → T), and 8023 (G → A) whereas they bound with less score to proteins with mutations at positions 481 (C→A), 1015 (A → T), 5098 (G → T), and 5237 (C → T) (ESI Fig. 1†). Remdisivir binds with more score to proteins with mutations at 28
733 (G → A), 28
734 (G → A), and 28
735 (G → C) with less score to 23
255 (A → G) compared to the wild type (Table 4). It is to be noted that the interaction of residues of wild type protein were found to be different than that of mutant model. For example, 479th; T → A mutant model which acquired the V → D amino acid interacted with GLU37, GLU41, LEU177, GLY180, LEU104, VAL108, HIS110, GLU87, LEU88, LYS141, TYR154 residues whereas wild type interacted with LEU18, VAL28, GLU37, GLU41, HIS 45, LEU53, VAL54, ILE71, ARG73, VAL86, VAL121, LEU122, ASP139 (Fig. 4).
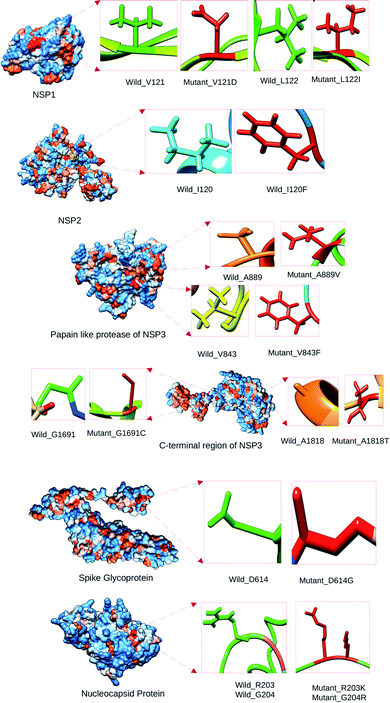 |
| Fig. 4 Location of the wild type and the mutant residues on the tertiary structure of SARS-CoV-2 proteins. | |
Table 3 Energy minimization of the wild type and mutant model
Serial number |
Variant in NIB_01 genome position |
Energy minimization score |
Stability of protein |
1 |
V121 (wild type) |
−4714.146 kJ mol−1 |
Increase |
2 |
V121D (mutant type) |
−4718.002 kJ mol−1 |
3 |
L122 (wild type) |
−4710.914 kJ mol−1 |
Decrease |
4 |
L122I (mutant type) |
−4382.711 kJ mol−1 |
5 |
I120 (wild type) |
−14 247.945 kJ mol−1 |
Increase |
6 |
I120F (mutant type) |
−14 678.767 kJ mol−1 |
7 |
V843 (wild type) |
−10 989.878 kJ mol−1 |
Decrease |
8 |
V843F (mutant) |
−10 847.174 kJ mol−1 |
9 |
A889 (wild type) |
−10 989.688 kJ mol−1 |
Decrease |
10 |
A889V (mutant type) |
−10 731.945 kJ mol−1 |
11 |
G1691 (wild type) |
−23 276.789 kJ mol−1 |
Decrease |
12 |
G1691C (mutant type) |
−22 377.976 kJ mol−1 |
13 |
A1818 (wild type) |
−23 276.789 kJ mol−1 |
Decrease |
14 |
A1818T (mutant type) |
−23 051.338 kJ mol−1 |
15 |
D614 (wild type) |
−9876.789 kJ mol−1 |
Decrease |
16 |
D614G (mutant type) |
−9308.898 kJ mol−1 |
19 |
R203 (wild type) |
−8789.976 kJ mol−1 |
Decrease |
20 |
R203K (mutant type) |
−8356.435 kJ mol−1 |
21 |
G204 (wild type) |
−8789.976 kJ mol−1 |
Decrease |
22 |
G204R (mutant type) |
−8089.172 kJ mol−1 |
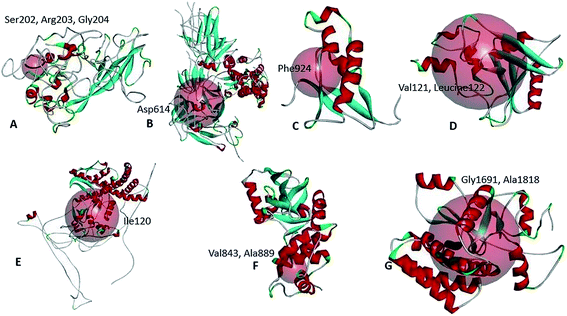 |
| Fig. 5 Mutation in the ligand binding site of the SARS-CoV-2 proteins. | |
Table 4 Drug binding analysis of variant. Red labeled residues denotes the mutated residues
Variant position in subject (NIB-01) sequence and protein coding region |
Drug name |
Binding energy |
479 (wild type) V121 NSP1 |
Ivermectin |
−7.9 kcal mol−1 |
479 (mutant type) V121D NSP1 |
Ivermectin |
−8.2 kcal mol−1 |
481(wild type) L122 NSP1 |
Ivermectin |
−8.7 kcal mol−1 |
481 (mutant type) L122I NSP1 |
Ivermectin |
−7.7 kcal mol−1 |
1015 (wild type) I120 NSP2 |
Ivermectin |
−7.9 kcal mol−1 |
1015 (mutant type) I120F NSP2 |
Ivermectin |
−6.9 kcal mol−1 |
5098 (wild type) V843 NSP3 |
Ivermectin |
−8.8 kcal mol−1 |
5098 (mutant) V843F NSP3 |
Ivermectin |
−8.0 kcal mol−1 |
5237 (wild type) A889 NSP3 |
Ivermectin |
−7.6 kcal mol−1 |
5237 (mutant type) A889V NSP3 |
Ivermectin |
−7.1 kcal mol−1 |
8023 (wild type) A1818 NSP3 |
Ivermectin |
−6.6 kcal mol−1 |
8023 (mutant type) A1818T NSP3 |
Ivermectin |
−7.5 kcal mol−1 |
23 255 (wild type) D614 spike |
Remdisivir |
−8.7 kcal mol−1 |
23 255 (mutant type) D614G spike |
Remdisivir |
−8.3 kcal mol−1 |
28 734 (wild type) R203 N |
Remdisivir |
−6.8 kcal mol−1 |
28 734 (mutant type) R203K N |
Remdisivir |
−7.8 kcal mol−1 |
28 735 (wild type) G204 N |
Remdisivir |
−7.1 kcal mol−1 |
28 735 (mutant type) G204R N |
Remdisivir |
−8.4 kcal mol−1 |
Molecular dynamic simulation
Results of molecular dynamic simulation analysis is presented in ESI Fig. 2.† NSP3 V843F had higher RMSD compared to the Wild type NSP3 while in case of NSP3 A889V it was lower. In case of NSP1, the mutant V121D exhibited higher RMSD than its wild type. Radius of gyration analysis revealed that both the mutants of NSP3 namely V843F and A889V were more compact than their wild counterpart. However, the NSP1 mutant V121D was more flexible than the wild type. Similar trend was observed from SASA calculation as well. These results imply that the mutations V843 and A889V in the NSP3 protein of SARS-CoV-2 made it more stable while the mutant V121D of NSP1 made the protein less stable.
Discussion
COVID-19 is highly contagious and the variation in its genome could be a leading reason for this feature. Besides, to understand the origin of the strains, the exploration of the whole-genome sequencing (WGS) data of SARS-CoV-2 strains is highly necessary.73 Insights into the mutations of SARS-CoV-2 is an important factor in developing therapeutics against the virus.74 In this study, we investigated the variation, insertion, and deletion of the Bangladeshi SARS-CoV-2 strains. We collected the samples SARS-CoV-2/human/BGD/NIB_01/2020 and SARS-CoV-2/human/BGD/NIB-BCSIR_02/2020 from the patients who were tested as COVID-19 positive. We extracted the viral RNA from the samples and converted them to cDNA. We performed the Sanger sequencing of SARS-CoV-2/human/BGD/NIB_01/2020 and next generation sequencing of SARS-CoV-2/human/BGD/NIB-BCSIR_02/2020. The total length of the genomes were 29
724 and 29
737 nucleotides respectively. These two genomes were submitted in both Global Initiative on Sharing All Influenza Data (GISAID) and National Center for Biotechnology Information (NCBI) databases. These two databases accepted the genomes and provided the accession number EPI_ISL_458133 from GISAID and MT509958 and MT568643 from NCBI. We investigated the possible variations of these two genome and found thirteen (13) mutations in SARS-CoV-2/human/BGD/NIB_01/2020 against the reference genome of SARS-CoV-2 (Table 1 and Fig. 2). The mutations belonged different regions of the genome, but mostly found in the Orf11 ab gene. Eight (8) mutations were found in the Orf1ab region. These mutations were 479th; T → A, 481st; C→A; 1015th; A → T, 2889th; C → T, 5098th; G → T; 5237th; C → T, 5642nd; G → T, 8023rd; G → A. The mutation 93rd; C → T was located in the 5′ upstream region of the gene. The mutations 28
733rd; G → A, 28
734th; G → A and 28
735th; G → C were located in the N gene of the genome. 23
255th; A → G mutation was located in the S gene of the genome (Table 1). Among the thirteen (13) mutations, six (6) mutations, namely 93rd; C → T, 2889th; C → T, 23
255th; A → G, 28
733rd; G → A, 28
734th; G → A and 28
735th; G → C were reported in different countries according to CNCB database (Table 1). All the variations showed a missense effect upon structure except 2889th; C → T variant (Table 1). The other seven (7) mutations, 479th; T → A, 481st; C→A; 1015th; A → T, 5098th; G → T; 5237th; C → T, 5642nd; G → T and 8023rd; G → A presented themselves as unique mutations in the MT509958 genome (Table 1 and Fig. 2). Surprisingly, we did not find any mutation in SARS-CoV-2/human/BGD/NIB-BCSIR_02/2020. We also looked for indel profile of our assembled genomes. However, we did not find any insertion/deletion occurred into the genome. We looked for it in the rest of the genomes of SARS-CoV-2 in Bangladesh. Seven (7) deletions were found in Bangladeshi strains EPI_ISL_450343, EPI_ISL_450344, EPI_ISL_468074, EPI_ISL_514614, EPI_ISL_445213, EPI_ISL_445217 and EPI_ISL_450842. Among them, EPI_ISL_450343, EPI_ISL_450344, EPI_ISL_468074 and EPI_ISL_514614 shared common deletions. These deletions belonged to the ORF8 gene and the length ranged from 27
913 to 28
254 (Table 2). We have found another deletion in the Orf7a gene whose position in the genome ranged from 27
476 to 27
668. This was found in an isolate from Dhaka region of Bangladesh (EPI_ISL_450842).
Three (3) dimensional structures were built for both wild type and the mutants in order to observe the mutational impact on their corresponding proteins (Fig. 4). We have predicted the stability of the protein structure of the corresponding variant based on energy minimization analysis. The variants 479th; T → A (V121D Orf1ab) and 1015th; A → T (I120F Orf1ab) were the locally more stable compare to the other variants in the proteins. These variant's structures consumed more energy than the wild type structure. The other variants exhibited a decrease in stability. The decrease in stability corresponds to protective effect against SARS-CoV-2 and vice versa (Table 3). The binding site of the protein structures was analyzed to look for the location of the relevant amino acid variant. We have found that all the variants were located within the ligand binding site (Fig. 5). Therefore, these residues could be considered very important in terms of ligand/drug binding. Ivermectin and Remdisivir were selected for the drug binding analysis. We performed molecular docking for each of the wild type and mutant structures with these two drugs and analyzed the interactions. We observed that the interacting wild type residues were replaced with different residues in after molecular docking with the drugs (Table 4). Here, the binding affinity was also found to be different from the wild type structure. It is to be clearly understood that only a single amino acid change from the wild type structure was responsible for these changes. From these analysis, it can be concluded that if any therapeutics are to be applied on these variants, the therapeutics might not work effectively due to the alteration of the residues in the mutant proteins. From the 50 ns molecular dynamics simulation carried out in GROMACS, it was observed that the mutations had an impact on the stability of SARS-CoV-2 proteins such as NSP1 and NSP3.
To reiterate the core of our study, we have performed whole genome sequencing of SARS-CoV-2 to identify genetic variations and then analyzed their impact on the structures of their corresponding proteins. We have also identified the insertions/deletions among all the sequenced Bangladeshi SARS-CoV-2 strains. The energy minimization and the drug binding analysis suggested that the identified mutations might have significant impact on structure and function of their target proteins. Therefore, the present study might be of great interest to the researchers/companies working to develop therapeutics against SARS-CoV-2 as well as gaining fundamental insights into pathogenesis of the virus.
Ethical approval
Appropriate international, national, and/or institutional guidelines were followed during the sample collections from the patients. The ethical approval numbers are National Institute of Biotechnology record no. NIBREC2020-01 and NIBREC2020-02.
Funding
This study was funded internally by the National Institute of Biotechnology, Ganakbari, Ashulia, Savar, Dhaka-1349, Bangladesh from its regular annual budget. No external or special funding was received for this project.
Data availability statement
The complete nucleotide sequence of SARS-CoV-2 isolate SARS-CoV-2/human/BGD/NIB_01/2020 from the Sanger sequencing has been deposited in GenBank under the accession number MT509958 (https://www.ncbi.nlm.nih.gov/nuccore/MT509958). The complete nucleotide sequence of SARS-CoV-2/human/BGD/NIB-BCSIR_02/2020 isolate from the NGS has been also deposited under the accession number MT568643 (https://www.ncbi.nlm.nih.gov/nuccore/MT568643.1?report=genbank). The rest of the data generated or analyzed during this study are included within this article and its ESI files.†
Author contributions
MUH, IA, MTHE. AB, and ZMC carried out mutational impact analysis and wrote and edited the manuscript. CAK and MS also edited the manuscript. KCD led the whole genome sequencing. MS supervised the whole project. All authors read and approved the manuscript.
Conflicts of interest
All authors declare no conflict of interest.
References
- C. Huang, et al., Clinical features of patients infected with 2019 novel coronavirus in Wuhan, China, Lancet, 2020, 395(10223), 497–506, DOI:10.1016/S0140-6736(20)30183-5.
- Q. Li, et al., Early Transmission Dynamics in Wuhan, China, of Novel Coronavirus-Infected Pneumonia, N. Engl. J. Med., 2020, 382(13), 1199–1207, DOI:10.1056/NEJMOA2001316.
- X. Tang, et al., On the origin and continuing evolution of SARS-CoV-2, Natl. Sci. Rev., 2020, 7(6), 1012–1023, DOI:10.1093/NSR/NWAA036.
- S.-M. Chaw, et al., The origin and underlying driving forces of the SARS-CoV-2 outbreak, J. Biomed. Sci., 2020, 27(1), 1–12, DOI:10.1186/S12929-020-00665-8.
- J. S. Mackenzie and D. W. Smith, COVID-19: a novel zoonotic disease caused by a coronavirus from China: what we know and what we don't, Microbiol. Aust., 2020, 41(1), 45–50, DOI:10.1071/MA20013.
- N. S. AlTakarli, China's Response to the COVID-19 Outbreak: A Model for Epidemic Preparedness and Management, Dubai Med. J., 2020, 3(2), 44–49, DOI:10.1159/000508448.
- W. Wang, et al., Detection of SARS-CoV-2 in Different Types of Clinical Specimens, JAMA, J. Am. Med. Assoc., 2020, 323(18), 1843–1844, DOI:10.1001/JAMA.2020.3786.
- T. Burki, The origin of SARS-CoV-2, Lancet Infect. Dis., 2020, 20(9), 1018, DOI:10.1016/S1473-3099(20)30641-1.
- L. Zou, et al., SARS-CoV-2 Viral Load in Upper Respiratory Specimens of Infected Patients, N. Engl. J. Med., 2020, 382(12), 1177–1179, DOI:10.1056/NEJMC2001737.
- J. R. Larsen, M. R. Martin, J. D. Martin, P. Kuhn and J. B. Hicks, Modeling the Onset of Symptoms of COVID-19, Public Health Front., 2020, 8(2296–2565), 478, DOI:10.3389/fpubh.2020.00473.
- W. J. Guan, et al., Clinical Characteristics of Coronavirus Disease 2019 in China, N. Engl. J. Med., 2020, 382(18), 1708–1720, DOI:10.1056/NEJMOA2002032.
- A. Lovato and C. de Filippis, Clinical Presentation of COVID-19: A Systematic Review Focusing on Upper Airway Symptoms, Eye, Ear, Nose Throat Mon., 2020, 99(9), 569–576, DOI:10.1177/0145561320920762.
- C. Menni, C. H. Sudre, C. J. Steves, S. Ourselin and T. D. Spector, Quantifying additional COVID-19 symptoms will save lives, Lancet, 2020, 395(10241), e107, DOI:10.1016/S0140-6736(20)31281-2.
- N. Chen, et al., Epidemiological and clinical characteristics of 99 cases of 2019 novel coronavirus pneumonia in Wuhan, China: a descriptive study, Lancet, 2020, 395(10223), 507–513, DOI:10.1016/S0140-6736(20)30211-7.
- M. Cascella, M. Rajnik, A. Aleem, S. C. Dulebohn, and R. Di Napoli, Features, Evaluation, and Treatment of Coronavirus (COVID-19), StatPearls, Apr. 2021, Accessed: Jul. 10, 2021. [Online]. Available: https://www.ncbi.nlm.nih.gov/books/NBK554776/ Search PubMed.
- L. Mizrahi, H. A. Shekhidem and S. Stern, Age separation dramatically reduces COVID-19 mortality rate in a computational model of a large population, Open Biol., 2020, 10(11), 200213, DOI:10.1098/RSOB.200213.
- J. R. Goldstein and R. D. Lee, Demographic perspectives on the mortality of COVID-19 and other epidemics, Proc. Natl. Acad. Sci. U. S. A., 2020, 117(36), 22035–22041, DOI:10.1073/PNAS.2006392117.
- M. H. B. Siam, M. M. Hasan, M. E. Raheem, H. R. Khan, M. H. Siddiqee and M. S. Hossain, Insights into the first wave of the COVID-19 pandemic in Bangladesh: Lessons learned from a high-risk country, medRxiv, 2020 DOI:10.1101/2020.08.05.20168674.
- C. Bonanad, et al., The Effect of Age on Mortality in Patients With COVID-19: A Meta-Analysis With 611,583 Subjects, J. Am. Med. Dir. Assoc., 2020, 21(7), 915–918, DOI:10.1016/J.JAMDA.2020.05.045.
- M. Brandén, et al., Residential context and COVID-19 mortality among adults aged 70 years and older in Stockholm: a population-based, observational study using individual-level data, Lancet Healthy Longev., 2020, 1(2), e80, DOI:10.1016/S2666-7568(20)30016-7.
- N. D. Yanez, N. S. Weiss, J.-A. Romand and M. M. Treggiari, COVID-19 mortality risk for older men and women, BMC Public Health, 2020, 20(1), 1–7, DOI:10.1186/S12889-020-09826-8.
- D. Liu, et al., Viral sepsis is a complication in patients with Novel Corona Virus Disease (COVID-19), Med. Drug Discovery, 2020, 8, 100057, DOI:10.1016/J.MEDIDD.2020.100057.
- H. C. Prescott and T. D. Girard, Recovery From Severe COVID-19: Leveraging the Lessons of Survival From Sepsis, JAMA, J. Am. Med. Assoc., 2020, 324(8), 739–740, DOI:10.1001/JAMA.2020.14103.
- A. O. C. Yataco and S. Q. Simpson, Coronavirus Disease 2019 Sepsis: A Nudge Toward Antibiotic Stewardship, Chest, 2020, 158(5), 1833–1834, DOI:10.1016/J.CHEST.2020.07.023.
- J. Beltrán-García, et al., Sepsis and Coronavirus Disease 2019: Common Features and Anti-Inflammatory Therapeutic Approaches, Crit. Care Med., 2020, 48(12), 1841–1844, DOI:10.1097/CCM.0000000000004625.
- C. Basso, et al., Pathological features of COVID-19-associated myocardial injury: a multicentre cardiovascular pathology study, Eur. Heart J., 2020, 41(39), 3827–3835, DOI:10.1093/EURHEARTJ/EHAA664.
- K. B. Shaha, D. N. Manandhar, J. R. Cho, A. Adhikari and M. Bahadur KC, COVID-19 and the heart: what we have learnt so far, Postgrad. Med. J., 2020, 97, 655–666, DOI:10.1136/POSTGRADMEDJ-2020-138284.
- R. D. Mitrani, N. Dabas and J. J. Goldberger, COVID-19 cardiac injury: Implications for long-term surveillance and outcomes in survivors, Heart Rhythm, 2020, 17(11), 1984, DOI:10.1016/J.HRTHM.2020.06.026.
- C. Chen, H. Li, W. Hang and D. W. Wang, Cardiac injuries in coronavirus disease 2019 (COVID-19), J. Mol. Cell. Cardiol., 2020, 145, 25–29, DOI:10.1016/J.YJMCC.2020.06.002.
- A. Tajbakhsh, et al., COVID-19 and cardiac injury: clinical manifestations, biomarkers, mechanisms, diagnosis, treatment, and follow up, Expert Rev. Anti Infect. Ther., 2021, 19(3), 345–357, DOI:10.1080/14787210.2020.1822737.
- S. Shi, M. Quin and B. Yang, Coronavirus Disease 2019 (COVID-19) and Cardiac Injury-Reply, JAMA Cardiol., 2020, 5(10), 1199–1200, DOI:10.1001/JAMACARDIO.2020.2456.
- S. D. Unudurthi, P. Luthra, R. J. C. Bose, J. R. McCarthy and M. I. Kontaridis, Cardiac inflammation in COVID-19: Lessons from heart failure, Life Sci., 2020, 260, 118482, DOI:10.1016/J.LFS.2020.118482.
- S. Dan, M. Pant and S. K. Upadhyay, The Case Fatality Rate in COVID-19 Patients With Cardiovascular Disease: Global Health Challenge and Paradigm in the Current Pandemic, Curr. Pharmacol. Rep., 2020, 6(6), 315–324, DOI:10.1007/S40495-020-00239-0.
- W. Jacobs, et al., Fatal lymphocytic cardiac damage in coronavirus disease 2019 (COVID-19): autopsy reveals a ferroptosis signature, ESC Heart Fail., 2020, 7(6), 3772–3781, DOI:10.1002/ehf2.12958.
- J. P. Lang, X. Wang, F. A. Moura, H. K. Siddiqi, D. A. Morrow and E. A. Bohula, A current review of COVID-19 for the cardiovascular specialist, Am. Heart J., 2020, 226, 29, DOI:10.1016/J.AHJ.2020.04.025.
- D. Wang, et al., Clinical Characteristics of 138 Hospitalized Patients With 2019 Novel Coronavirus-Infected Pneumonia in Wuhan, China, JAMA, J. Am. Med. Assoc., 2020, 323(11), 1061–1069, DOI:10.1001/JAMA.2020.1585.
- M. L. Holshue, et al., First Case of 2019 Novel Coronavirus in the United States, N. Engl. J. Med., 2020, 382(10), 929–936, DOI:10.1056/NEJMOA2001191.
- K. Prem, et al., The effect of control strategies to reduce social mixing on outcomes of the COVID-19 epidemic in Wuhan, China: a modelling study, Lancet Public Health, 2020, 5(5), e261–e270, DOI:10.1016/S2468-2667(20)30073-6.
- T.-L. Xu, et al., China's practice to prevent and control COVID-19 in the context of large population movement, Infect. Dis. Poverty, 2020, 9(1), 1–14, DOI:10.1186/S40249-020-00716-0.
- M. Fugazza, Impact of the COVID-19 Pandemic on Commodities Exports to China: UNCTAD Research Paper No. 44, Jun. 2020, doi: DOI:10.18356/93EBF4D1-EN.
- M. Vinceti, et al., Lockdown timing and efficacy in controlling COVID-19 using mobile phone tracking, EClinicalMedicine, 2020, 25 DOI:10.1016/J.ECLINM.2020.100457.
- J. D. Hamadani, et al., Immediate impact of stay-at-home orders to control COVID-19 transmission on socioeconomic conditions, food insecurity, mental health, and intimate partner violence in Bangladeshi women and their families: an interrupted time series, Lancet Glob. Health, 2020, 8(11), e1380–e1389, DOI:10.1016/S2214-109X(20)30366-1.
- Z. Xu, et al., Pathological findings of COVID-19 associated with acute respiratory distress syndrome, Lancet Respir. Med., 2020, 8(4), 420–422, DOI:10.1016/S2213-2600(20)30076-X.
- L. M. Casanova, S. Jeon, W. A. Rutala, D. J. Weber and M. D. Sobsey, Effects of air temperature and relative humidity on coronavirus survival on surfaces, Appl. Environ. Microbiol., 2010, 76(9), 2712–2717, DOI:10.1128/AEM.02291-09.
- S. Kumar, R. Nyodu, V. K. Maurya and S. K. Saxena, Morphology, Genome Organization, Replication, and Pathogenesis of Severe Acute Respiratory Syndrome Coronavirus 2 (SARS-CoV-2), Coronavirus Dis., 2020, 2019, 23, DOI:10.1007/978-981-15-4814-7_3.
- M. Bianchi, D. Benvenuto, M. Giovanetti, S. Angeletti, M. Ciccozzi and S. Pascarella, Sars-CoV-2 Envelope and Membrane Proteins: Structural Differences Linked to Virus Characteristics?, Biomed Res. Int., 2020, 2020, 1–6, DOI:10.1155/2020/4389089.
- D. Schoeman and B. C. Fielding, Coronavirus envelope protein: current knowledge, J. Virol., 2019, 16(1), 1–22, DOI:10.1186/S12985-019-1182-0.
- F. A. Rabi, M. S. A. Zoubi, G. A. Kasasbeh, D. M. Salameh and A. D. Al-Nasser, SARS-CoV-2 and Coronavirus Disease 2019: What We Know So Far, Pathogens, 2020, 9(3), 231, DOI:10.3390/PATHOGENS9030231.
- H. Abboud, F. Z. Abboud, H. Kharbouch, Y. Arkha, N. E. Abbadi and A. E. Ouahabi, COVID-19 and SARS-Cov-2 Infection: Pathophysiology and Clinical Effects on the Nervous System, World Neurosurg., 2020, 140, 49–53, DOI:10.1016/J.WNEU.2020.05.193.
- S. Khan, et al., Coronaviruses disease 2019 (COVID-19): Causative agent, mental health concerns, and potential management options, J. Infect. Public Health, 2020, 13(12), 1840–1844, DOI:10.1016/j.jiph.2020.07.010.
- K. A. Adedokun, A. O. Olarinmoye, J. O. Mustapha and R. T. Kamorudeen, A close look at the biology of SARS-CoV-2, and the potential influence of weather conditions and seasons on COVID-19 case spread, Infect. Dis. Poverty, 2020, 9(1), 1–5, DOI:10.1186/S40249-020-00688-1.
- S. Ludwig and A. Zarbock, Coronaviruses and SARS-CoV-2: A Brief Overview, Anesth. Analg., 2020, 131(1), 93–96, DOI:10.1213/ANE.0000000000004845.
- M. Cevik, M. Tate, O. Lloyd, A. E. Maraolo, J. Schafers and A. Ho, SARS-CoV-2, SARS-CoV, and MERS-CoV viral load dynamics, duration of viral shedding, and infectiousness: a systematic review and meta-analysis, Lancet Microbe, 2021, 2(1), e13–e22, DOI:10.1016/S2666-5247(20)30172-5.
- D. Wu, T. Wu, Q. Liu and Z. Yang, The SARS-CoV-2 outbreak: What we know, Int. J. Infect. Dis., 2020, 94, 44–48, DOI:10.1016/J.IJID.2020.03.004.
- Z. Abdelrahman, M. Li and X. Wang, Comparative Review of SARS-CoV-2, SARS-CoV, MERS-CoV, and Influenza A Respiratory Viruses, Front. Immunol., 2020, 11, 2309, DOI:10.3389/FIMMU.2020.552909.
- M. Fani, A. Teimoori and S. Ghafari, Comparison of the COVID-2019 (SARS-CoV-2) pathogenesis with SARS-CoV and MERS-CoV infections, Future Virol., 2020, 15(5), 317–323, DOI:10.2217/FVL-2020-0050.
- Z. Zhu, X. Lian, X. Su, W. Wu, G. A. Marraro and Y. Zeng, From SARS and MERS to COVID-19: a brief summary and comparison of severe acute respiratory infections caused by three highly pathogenic human coronaviruses, Respir. Res., 2020, 21(1), 1–14, DOI:10.1186/S12931-020-01479-W.
- Y. Chen, Q. Liu and D. Guo, Emerging coronaviruses: Genome structure, replication, and pathogenesis, J. Med. Virol., 2020, 92(4), 418–423, DOI:10.1002/JMV.25681.
- A. Wu, et al., Genome Composition and Divergence of the Novel Coronavirus (2019-nCoV) Originating in China, Cell Host Microbe, 2020, 27(3), 325–328, DOI:10.1016/J.CHOM.2020.02.001.
- A. R. Fehr and S. Perlman, Coronaviruses: an overview of their replication and pathogenesis, Methods Mol. Biol., 2015, 1282, 1–23, DOI:10.1007/978-1-4939-2438-7_1.
- J. Hellewell, et al., Feasibility of controlling COVID-19 outbreaks by isolation of cases and contacts, Lancet Glob. Health, 2020, 8(4), e488–e496, DOI:10.1016/S2214-109X(20)30074-7.
- R. Lu, et al., Genomic characterisation and epidemiology of 2019 novel coronavirus: implications for virus origins and receptor binding, Lancet, 2020, 395(10224), 565–574, DOI:10.1016/S0140-6736(20)30251-8.
- M. Moniruzzaman, et al., Coding-Complete Genome Sequence of SARS-CoV-2 Isolate from Bangladesh by Sanger Sequencing, Microbiol. Resour. Announc., 2020, 9(28), e00626–20, DOI:10.1128/MRA.00626-20.
- T. G. Burland, DNASTAR’s Lasergene sequence analysis software, Methods Mol. Biol., 2000, 132, 71–91, DOI:10.1385/1-59259-192-2:71.
- J. L. A. Paijmans et al., Sequencing single-stranded libraries on the Illumina NextSeq 500 platform, Nov. 2017, Accessed: Jul. 10, 2021. [Online]. Available: https://arxiv.org/abs/1711.11004v1 Search PubMed.
- A. Gurevich, V. Saveliev, N. Vyahhi and G. Tesler, QUAST: quality assessment tool for genome assemblies, Bioinformatics, 2013, 29(8), 1072–1075, DOI:10.1093/bioinformatics/btt086.
- Y. Shu and J. McCauley, GISAID: Global initiative on sharing all influenza data – from vision to reality, Eurosurveillance, 2017, 22(13), 1, DOI:10.2807/1560-7917.ES.2017.22.13.30494.
- E. F. Pettersen, T. D. Goddard, C. C. Huang, G. S. Couch, D. M. Greenblatt, E. C. Meng and T. E. Ferrin, UCSF Chimera--a visualization system for exploratory research and analysis, J. Comput. Chem., 2004, 25(13), 1605–1612, DOI:10.1002/jcc.20084.
- D. E. Kim, D. Chivian and D. Baker, Protein structure prediction and analysis using the Robetta server, Nucleic Acids Res., 2004, 32, W526–W531, DOI:10.1093/nar/gkh468.
- E. Lindahl, B. Hess and D. van der Spoel, GROMACS 3.0: a package for molecular simulation and trajectory analysis, J. Mol. Model., 2001, 7(8), 306–317, DOI:10.1007/S008940100045.
- O. Trott and A. J. Olson, AutoDock Vina: improving the speed and accuracy of docking with a new scoring function, efficient optimization and multithreading, J. Comput. Chem., 2010, 31(2), 455, DOI:10.1002/JCC.21334.
- T. G. Bell and A. Kramvis, Fragment Merger: An Online Tool to Merge Overlapping Long Sequence Fragments, Viruses, 2013, 5(3), 824–833, DOI:10.3390/V5030824.
- I. Ahammad et al., Comparative genomic study for revealing the complete scenario of COVID-19 pandemic in Bangladesh, medRxiv, p. 2020, Jun. 2021, DOI:10.1101/2020.11.27.20240002.
- B. Dearlove, et al., A SARS-CoV-2 vaccine candidate would likely match all currently circulating variants, Proc. Natl. Acad. Sci. U. S. A., 2020, 117(38), 23652–23662, DOI:10.1073/PNAS.2008281117.
Footnote |
† Electronic supplementary information (ESI) available. See DOI: 10.1039/d1ra05327b |
|
This journal is © The Royal Society of Chemistry 2021 |
Click here to see how this site uses Cookies. View our privacy policy here.