DOI:
10.1039/D1RA05276D
(Paper)
RSC Adv., 2021,
11, 31923-31932
Recombinant protein linker production as a basis for non-invasive determination of single-cell yeast age in heterogeneous yeast populations†
Received
8th July 2021
, Accepted 16th September 2021
First published on 28th September 2021
Abstract
The physiological and metabolic diversity of a yeast culture is the sum of individual cell phenotypes. As well as environmental conditions, genetics, and numbers of cell divisions, a major factor influencing cell characteristics is cell age. A postcytokinesis bud scar on the mother cell, a benchmark in the replicative life span, is a quantifiable indicator of cell age, characterized by significant amounts of chitin. We developed a binding process for visualizing the bud scars of Saccharomyces pastorianus var. carlsbergensis using a protein linker containing a polyhistidine tag, a superfolder green fluorescent protein (sfGFP), and a chitin-binding domain (His6-SUMO-sfGFP-ChBD). The binding did not affect yeast viability; thus, our method provides the basis for non-invasive cell age determination using flow cytometry. The His6-SUMO-sfGFP-ChBD protein was synthesized in Escherichia coli, purified using two-stage chromatography, and checked for monodispersity and purity. Linker-cell binding and the characteristics of the bound complex were determined using flow cytometry and confocal laser scanning microscopy (CLSM). Flow cytometry showed that protein binding increased to 60
455 ± 2706 fluorescence units per cell. The specific coupling of the linker to yeast cells was additionally verified by CLSM and adsorption isotherms using yeast cells, E. coli cells, and chitin resin. We found a relationship between the median bud scar number, the median of the fluorescence units, and the chitin content of yeast cells. A fast measurement of yeast population dynamics by flow cytometry is possible, using this protein binding technique. Rapid qualitative determination of yeast cell age distribution can therefore be performed.
1 Introduction
Aging is defined as “a persistent decline in the age-specific fitness components of an organism due to internal physiological deterioration”.1 It is a complex physiological process that involves the decreased ability to adapt to environmental changes, leading to increased morbidity and mortality.2 Aged cells are less resistant to endogenous and exogenous stressors.3 For example carbonyl stress results in a decline in reproductive capacity as stated by Semchyshyn et al.4 Dietary conditions with reduced glucose concentrations of 0.5% results in an increase of aging potential.5 In yeast, physiological function is related to cell viability and vitality. Viability is the percentage of living cells in a yeast population, while vitality is a measure of physiological cell capability, and is an important indicator for expressing the physiological state of yeast cells.6
In the analysis of cell aging, it is important to distinguish between chronological and replicative life spans. The chronological life span is the survival time of nondividing cells in a population,7 while the replicative life span is the number of generative events which occur during the life cycle.8,9 The following characteristic morphological and physiological changes occur during aging: decreased metabolic activity, an increased number of bud scars, a decrease in the number of single budding events, and increased cell size.10,11
In the absence of exogenous stressors, the life span of a yeast cell is governed by replicative events and the accumulation of extrachromosomal ribosomal DNA circles, mitochondrial defects, protein oxidation, and decreases in the length of telomeres, which protect chromosomes from degradation.12,13 Mitochondrial defects result in an accumulation of reactive oxygen and nitrogen species (RONS), which are produced by endogenous metabolic processes. An imbalance between RONS production and metabolization leads to DNA damage.14 During the aging of yeast cultures, some proteins, such as alcohol dehydrogenase 1, are oxidatively modified, reducing their in vivo activity and therefore reducing the replicative lifespan.15 A detailed summary of the impact of exogenous and endogenous stress conditions on aging is given by Eigenfeld et al.16
Yeast cells are usually exposed to multiple exogenous stressors that reduce cell viability and vitality. These exogenous stressors include osmolality, which causes cells to contend with high concentration gradients of, for example, glucose, a potent inhibitor of cellular respiration (the Crabtree effect).17 Low pH,18,19 the presence of denaturing substances such as ethanol or acetaldehyde,20 and deviations from optimal growth temperatures have also been reported.21
Micromanipulation is often used for determining the replicative yeast age in a research environment. The technique involves the separation of mother and daughter cells for budding events, and analysis of the aging characteristics of the mother cell using single-cell observation under a microscope.8,22 This approach is time consuming and therefore not suited to high-throughput analysis, which is why published studies using micromanipulation were limited to a maximum of 19 budding cells analysed22 or an analysis time of five and a half hours for 120 cells.23 Another approach is the use of a centrifugal elutriator, which separates mother and daughter cells using centrifugal forces.24,25 Due to the focus on senescent cells, in the study of Woldringh,24 no information about age distributions is given. A more recent method is the use of microfluidic devices.26,27 These devices retain mother cells and flush away daughter cells, allowing a single-cell aging analysis of a maximum of 8000 mother cells over their whole lifespan, whereas no information about daughter cells can be obtained. Due to these disadvantages, none of these techniques is suitable for a high-throughput analysis of yeast cell age. Methods such as micromanipulation do not provide real-time information, which makes them unsuitable for industrial applications. Methods of rapidly processing large numbers of cells, which are less disruptive than the centrifugal elutriator, are therefore preferable. Flow cytometric methods have recently been developed as an alternative. These methods do not directly assess yeast age but offer the possibility of high-throughput screening, including real-time analysis. To date, there is one flow cytometric approach which has been used for determining the mean population age, but none for directly determining the age of single yeast cells. Powell et al. reported a staining method for chitin based on fluorescein isothiocyanate (FITC)-labeled wheat germ agglutinin (WGA), evaluated using microscopy.10 Kurec et al. used fluorescein isothiocyanate labelled wheat germ agglutinin (WGA-FITC) for the first time to analyze the mean yeast cell age in populations by flow cytometry.28 The technique presented by Kurec et al., which takes into account the interconnection between mean fluorescence intensities and mean bud scar number and the transfer toward fermentation processes is valuable, although there are still some limitations. For example, this approach does not consider non-Gaussian age distributions or yeast cell autofluorescence. Moreover, fewer yeast cells are analyzed for the calibration curve. WGA-FITC cannot be used for further applications such as yeast separation or manipulation, due to the lack of necessary binding tags.
Novel targets and methods of determining the age of yeast cells are required to overcome these difficulties. One such target is the number of bud scars, which can act as a characteristic marker of replicative aging. Limited budding events occur before the onset of replicative senescence. During each cytokinesis event, a bud scar, largely composed of chitin is formed on the cell surface. Molecules, especially proteins such as serpentine, vermiform, and resilin, with chitin-binding domains (ChBDs) interact with chitin molecules.29,30 ChBDs are widely used to study chitin distributions in Drosophila melanogaster.31 Various potential reporter molecules that selectively bind to chitin can be used in fluorescence labeling for flow cytometry.32 Other applications of ChBDs include their use as tags for purifying proteins from different species,33 or as targets for primary antibodies in different immunostaining/immobilizing techniques.34 The first chitin staining experiments were performed using Calcofluor white,35,36 which stains bud scars as well as cell walls.37 However, none of these established methods have yet been successfully adapted for high-throughput yeast age determination of individual cells.
Yeast cultures play a critical role in many industrial processes, including the production of refined fermented products such as beer and ale,38 wine,39 bioethanol40 and the synthesis of recombinant proteins.41–43 The physiological state of each cell is of vital importance to the performance of these processes. Especially in cases of continuous fermentation, or yeast cultures being reused batch-wise, as in industrial beer fermentations, the percentage of non-vital cells in outflows44 or foam,45 increases significantly. The impact of the singular age on fermentation performance is still unknown.
In this study a non-invasive method as basis for determining single-cell yeast age, using fluorescence-coupled flow cytometry, was developed. A limitation of the study is the focus on single cells such as Saccharomyces pastorianus (S. pastorianus) yeasts or other bottom-fermenting yeast strains; its effectiveness for budding cells or cell aggregates remains to be analyzed. The advantages of our method include high-throughput, its non-invasiveness, and the ability to perform real-time investigation of whole-yeast cultures used in industrial processes.
2 Experimental
2.1 Strain and strain maintenance
Escherichia coli Top10 (catalog #C404010; Invitrogen, Carlsbad, CA, USA) was used for cloning experiments. E. coli BL21 (DE3) (New England Biolabs [NEB] catalog #C2527I; Ipswich, MA, USA) was used for protein overexpression; the strain was stored in glycerol at −80 °C. E. coli DH5 α (New England Biolabs [NEB] catalog #C2987I; Ipswich, MA, USA) was used for adsorption experiments.
In addition, we used the bottom-fermenting yeast strain Saccharomyces pastorianus var. carlsbergensis TUM 34/70 in this study. The strain was grown on yeast extract peptone dextrose (YPD) agar plates (10 g L−1 of Bacto yeast extract, 20 g L−1 of Bacto peptone, and 20 g L−1 of glucose, and 15 g L−1 agar) for 48 h.
For the analysis of different aged yeast cell samples, 10 mL of YPD liquid medium was inoculated with S. pastorianus to a cell concentration of 1 Mio cells per mL yeast, measured by optical density, each day for nine days.
2.2 Cloning experiments
The pET28b(+) small ubiquitin-like modifier (SUMO) plasmid, which codes for a His-tagged SUMO gene, was obtained from Martin Haslbeck (Technical University of Munich, Germany) and used as a ligation backbone, in addition to a gene coding for superfolder green fluorescent protein (sfGFP). The pTXB3 plasmid, which codes for the ChBD gene, was a kind gift from the Institute of Biological Chemistry (University of Vienna, Austria).46 Using PTXB3 as a template, ChBD was cloned into pET28b(+) and transformed into E. coli BL21(DE3). The method is described in the ESI Section 1.1.†
2.3 His6-SUMO-GFP-ChBD synthesis and purification
The His6-SUMO-GFP-ChBD gene was overexpressed in E. coli BL21 (DE3) regulated by a T7 promotor in a 15 L Biostat C stirring tank reactor (Sartorius AG, Göttingen, Germany). Bacteria were cultivated in batches in Terrific Broth medium with a stirrer speed of 600 rpm and oxygen enrichment of 40% at 37 °C. His6-SUMO-GFP-ChBD expression was induced at an optical density of 0.6 at wavelength 600 nm (OD600) with 1 mM isopropyl-β-D-1-thiogalactopyranoside. After induction, the temperature was decreased to 25 °C to prevent the development of inclusion bodies47 and enhance solubility.48 Next, cells were separated from the residual fermentation broth by centrifugation at 12
000 rpm for 5 min. The cell pellet was resuspended in lysis buffer, followed by ultrasound cell lysis (2 × 4 min; cycles 5 × 10%; power 50%). Protein with a purity of >95% was obtained via two-step purification. First, purification of the soluble intracellular protein was carried out using immobilized metal affinity chromatography (IMAC) (ESI Fig. S1†). A single elution peak was collected with a retention volume of 35.8 mL. Subsequently, a second purification step was performed using anion-exchange chromatography (AEC) according to a modified protocol of Malho et al.49
Affinity chromatography with 6 mL nickel–nitrilo triacetic acid (Ni2+–NTA) loaded onto a HiScale chromatography column was used in an ÄKTA purifier system (GE Healthcare, Uppsala, SE, USA). To bind free nickel ions and inhibit protein aggregation, 2 mM EDTA was added to the target sample after protein elution with 50% elution buffer. Subsequently, protein solutions were purified using an anion-exchange column (Q-FF). Fractions containing His6-SUMO-GFP-ChBD were dialyzed against 20 mM Tris–HCl buffer (pH 8).
For non-purified samples, we measured protein content according to Bradford.50
For purified samples, protein content was measured photometrically at 280 nm. We therefore compared the absorption of a defined protein concentration with the absorption of the denatured protein.51 The extinction coefficient of the native protein was then calculated using the Lambert–Beer law. The theoretical extinction coefficient of 44
475 mol−1 cm−1 was calculated according to the amino acid sequence.
2.4 Tricine SDS-PAGE
We analyzed chromatography samples using tricine sodium dodecyl sulfate-polyacrylamide gel electrophoresis (tricine SDS-PAGE), as described previously.52 Samples of the fast protein liquid chromatography (FPLC) fractions load, flow, wash, and elution were loaded onto tricine SDS-PAGE for visualization of the purification process and size determination. Briefly, proteins were denatured at 70 °C for 10 min in a two-fold sample buffer and then loaded on 5% acrylamide stacking gels. Gel running was performed on 10% separating gels under reducing conditions, and a pre-stained protein marker (broad range, 10–250 kDa) was used as a reference, with 10 and 20 μL of reference and samples, respectively.
Two tricine SDS-PAGE cycles were performed by running gels at 40 mA and 180 V for 4 h. Protein staining of one gel was performed using Coomassie Brilliant Blue G-250, while the other gel was electroblotted using a tank blot system.
2.5 Western blotting
To verify successful protein synthesis, western blotting was performed. Proteins obtained from the 10% tricine SDS-PAGE gels were transferred to a nitrocellulose membrane using a tank blot system for 2 h at 100 mA using Towbin transfer buffer53 and then blocked with skim milk powder (5% skim milk in 1× Tris-buffered saline) overnight. The blocked nitrocellulose membranes were successively incubated with anti-his primary antibody (1
:
10
000 dilution (mouse, Roche diagnostics, Mannheim)) and anti-mouse immunoglobulin G (IgG) secondary antibodies (1
:
7500 dilution (goat, Roche diagnostics, Mannheim)), coupled with alkaline phosphatase (ALP) for analysis, and detected using colorimetric visualization with nitro blue tetrazolium and 5-bromo-4-chloro-3-indolyl-phosphate (BCIP) solutions. The gels were blotted and probed with a His-tag-specific antibody. Quantitation of the purity of the AEC elution fraction >95% was determined using high-performance liquid chromatography analysis.54 The correct protein size was checked using electrospray ionization-tandem mass spectrometry (ESI-MS/MS).
2.6 Bud scar staining
Yeast cell suspensions were centrifuged at 1000×g for 1 min. The supernatant was discarded, and the cells were resuspended in 20 mM Tris–HCl buffer (pH 8). Next, 2 mL of the cell suspension was washed twice with 20 mM Tris–HCl buffer (pH 8) and diluted to an OD600 of 1.0 using 20 mM Tris–HCl buffer. Then, 50 μL of yeast suspension and 100 μL of 4000 nM protein solution was mixed to achieve the final concentration of 4.76 × 10−7 nmol per yeast cell. The cells were gently stirred in the dark for 30 min at room temperature, harvested by centrifugation at 850×g for 1 min, and washed five times with 20 mM Tris–HCl. Finally, the stained cell culture was resuspended in 1 mL Tris–HCl. WGA-FITC was used for comparison.10,28 The yeast cells were washed in sterile phosphate-buffered saline (PBS) twice, adjusted to an OD600 of 0.8, centrifuged, resuspended in 500 μL of 1 mg mL−1 WGA-FITC solution, incubated for 15 min in darkness, washed in PBS three times, and centrifuged at 2000×g. The specific binding of the protein was examined using flow cytometry and confocal laser scanning microscopy (CLSM).
The width and length of the cells, of different yeast samples were analyzed using CLSM image analysis with a Python script. The number of the visible bud scars in front (n) and on the edge (k) was counted, and the cell volume (V) of each cell was calculated. According to the relationship between cell surface area and bud scar number described by Powell et al.10 the probability of the total bud scar number (m) can be calculated using Bayes theorem:
is proportional to the value of the density function of the normal distribution, hence:
μn = 132.34 + ln(n) × 59.41 |
σ: standard deviation of
V;
μn: mean of continuous
V for a given class
n.
At a sample cell number of 9.11 × 105, the sample corresponds to infinity while a cell number of 300 is considered to be representative for the cell population. By use of a confidence interval of 90% (Z-value: 1.65), a margin of error of 0.05 and a standard deviation of 0.5 (corresponding to the worst-case scenario) a minimum sample size of 272 cells is necessary.55,56
The non-invasive nature of the process was verified using methylene blue (MB) staining for viability check over 24 h. One part yeast suspension was mixed with one part of the buffered MB solution and incubated for 3 min before counting at least 200 cells. The experimental controls were prepared by replacing the protein solution with 200 μL of 20 mM Tris–HCl. Each experiment was performed independently in triplicate.
2.7 Microscopic visualization
We performed a cell viability check by mixing control samples without an immobilized protein linker, and the same amount of yeast cells with an immobilized protein linker with 0.1 mg mL−1 of MB dissolved in a 2% dehydrate sodium citrate solution. After incubation for 5 min at room temperature, we evaluated the cell viability using a microscope and a Thoma cell-counting chamber (0.1 mm depth).
Next, CLSM was performed using an Olympus FV1000 system (Olympus, Tokyo, Japan) from the Centre for Advanced Light Microscopy, equipped with 10×, 20×, 40×, and 60× objectives (numerical apertures = 0.30, 0.50, 0.90, and 1.20). The system included a blue diode laser with wavelength of 488 nm and an external transmitted light photomultiplier detector, which enabled both laser scanning and transmitted light observation. We prepared yeast samples for flow cytometry measurements. The samples were loaded on a microscope slide and measured under 4% laser power (high voltage = 650 V; gain = 1.25; offset = 10%) with simultaneous transmission light (high voltage = 140 V; gain = 1.00; offset = 0%).
2.8 Statistical analysis
Q–Q plots were used to evaluate whether a normal distribution was present under the flow cytometric FSC-A and 525-40-A parameters. Due to the non-Gaussian particle distribution of the flow cytometry data, indicated by the Q–Q plots, the median of fluorescence intensities was used. Because of the non-Gaussian distribution and the sample number of 20.000 per flow cytometric measurement, the differences between the samples were determined using the nonparametric Kruskal–Wallis test and post hoc Dunn's test using R.57 Kruskal–Wallis is the alternative test to ANOVA, suited for non-normally distributed data. Instead, Kruskal–Wallis test is reliable for the number of observations given by each measurement. Post hoc Dunn's tests were used, because the Kruskal–Wallis test only identifies, that a difference between the variables exists, while the Dunn's test provides information about, which data groups differ.
Outlier analysis of flow cytometric data was performed using R and the package mvoutlier58 for the removal of values originating from different sources, such as dust, budding cells or similar.
2.9 Experimental information
All details about the monodispersity check of the protein solution and its affinity can be found in the ESI 1.3 and 1.4† as well as the flow cytometric analysis of the protein binding (1.5†) and the determination of the chitin contents of the yeast cells (1.6†). Briefly, the field flow fractionation method used was based on the method published by Wyatt Technology Corporation.59 Adsorption isotherms were made by mixing a constant amount of adsorption material with an increasing amount of protein, as described by Bathen and Breitbach.60 Chitin determination was done according to methods published by Dallies et al.61 and Katano et al.62
3 Results and discussion
3.1 Cloning and expression of His6-SUMO-sfGFP-ChBD genes
A His6-SUMO-sfGFP-ChBD vector was constructed. It included a polyhistidine tag (His6) for purification using IMAC, a SUMO for protein solubility and prevention of inclusion bodies, a superfolder green fluorescent protein (sfGFP) for fluorescence detection, and a ChBD to recognize the bud scars of the yeast cells. Amplification products with the expected MW (921 bp), corresponding to sfGFP and ChBD, were detected in five of the six clones. Subsequently, DNA sequencing confirmed that the plasmid sequence and size were correct, and this plasmid was transformed into chemocompetent E. coli BL21(DE3) cells using heat shock. One strain was fermented and induced using isopropyl β-D-1-thiogalactopyranoside.
The His6-SUMO-sfGFP-ChBD protein was isolated, and purified using a two-step procedure. The soluble intracellular protein (340.2 mg) was first purified with IMAC (Fig. S1†) (26.28 mg), then with AEC (Fig. S2†), resulting in a flow fraction peak area of 662.2 mAu mL−1, corresponding to 86.22% of the entire peak area, and a protein content of 22.5 mg. The total protein content of the fermentation was calculated as 75.3 mg L−1 in E. coli BL21 (DE3) culture broth, an amount which was consistent with previously reported yields.63,64 According to the fluorescence and peak area of the HPLC analysis, the protein purity was determined >95%.
3.2 Protein characterization
The identity of the protein was verified using SDS-PAGE and western blot analysis. Fig. S3† shows a visualization of the protein purification and size determination. PAGE indicated a predominant band in the IMAC load, with a mass of 54 kDa. The eluted AEC-fraction solely showed this predominant band, devoid of any other band. The gel was blotted and probed with His-tag specific antibodies.
Reaction signals of the western blot were present in all fractions at a protein size of 54 kDa. The protein size calculated using tricine SDS-PAGE was slightly above the theoretically calculated molar mass of 47.23 kDa, corresponding to a deviation of 14%. Superfolder GFP is mainly comprised of β-sheets. The deviation in protein size may be due to incomplete denaturation of the protein, which mainly consists of this structure.65 Remaining buffer salt concentrations also affect the stability of the native GFP in the tested temperature range of 70–95 °C resulting in a decrease in the D-value. The D-value describes the protein stability over the time period to reduce the initial protein concentration by one decimal logarithm.66,67 The protein size calculated by ESI-MS/MS was 47.35 kDa, consistent with the theoretically calculated molar mass of 47.23 kDa. The extinction coefficient, used for photometric protein concentration determination was calculated to be 47
234.79 mol−1 cm−1.
To prevent false-positive results due to the binding of dimers or trimers, polydispersity was evaluated using asymmetric field flow fractionation. Fig. S4† shows the chromatogram of this fractionation. In minutes 3–5, the crossflow increased, increasing the DRI signal and checking the system pressure. The sample was injected and concentrated in minutes 5–11 in focus mode. Sample elution occurred at minutes 14–19. Due to the simultaneous behavior (alignment) of all three detectors, field flow fractionation can reveal a monodisperse sample. The polydispersity index, Đ, which describes the width of the molecular weight distribution, is calculated by mass average divided by number average (MW/Mn), producing a value is 1 for molecularly uniform solutions.68 For the protein solution, the polydispersity index Đ was 1.01 ± 0.056, a value consistent with a homogenous, monodisperse solution. A slight tailing observed in the signals might have arisen because of salt deposits from the purification buffer, adsorption effects on the membrane, the protein concentration, or the large protein size itself.69–71
3.3 Evaluation of specific binding parameters
The specific binding of His6-SUMO-sfGFP-ChBD was determined using binding experiments. The binding affinities of His6-SUMO-sfGFP-ChBD to S. pastorianus var. carlsbergensis yeast cells, chitin resin (positive control) and E. coli DH5 α (lacking chitin; negative control) were tested (Fig. 1). The dissociation constants, KD were calculated to be 228.21 ± 60.86 nM for chitin resin, and 149.72 ± 30.44 nM for the yeast cells. Therefore, qmax values of 1.58 × 109 ± 0.95 × 108 molecules per μm2 and 2.45 × 106 ± 0.11 × 106 molecules per μm2 could be reached for chitin resin and yeast cells, respectively. The maximal load of yeast cells indicated a maximum protein mass of 2.01 × 10−7 nmol per yeast cell in the bud scar staining experiments. The load of chitin resin was 645.5 times higher than the maximum load of yeast, indicating that the yeast cells had a surface layer consisting of approximately 0.15% free chitin, resulting from budding. In comparison E. coli cells had no significant amount of protein bound. Lastly, WGA had a qmax value of 7.65 × 106 ± 3.47 × 106 molecules per μm2 and a KD value of 26.66 ± 12.079 μM, indicating a 176-fold lower affinity of WGA toward yeast cells compared to the protein linker. The KD values for ChBD are described in literature as being in the range 0.6–2.5 μM,72–74 and those for WGA as 57 μM.75 The binding affinity of His6-SUMO-sfGFP-ChBD measured in this study was higher than previously reported values. The differences may be due to a lack of knowledge about the reversibility of the binding procedure.76,77 Instead, the used fit model of Langmuir was used for the calculation of the parameters of binding because the adsorption follows a Langmuir behaviour.76,78
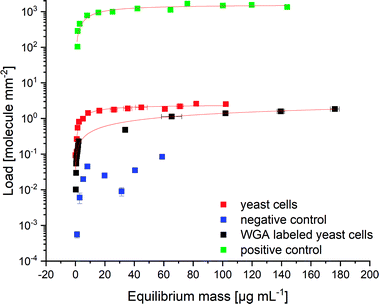 |
| Fig. 1 Adsorption isotherms indicating the specific binding of the protein linker to chitin. 2.1 × 106 yeast cells; positive control: 1200 chitin resin particles; negative control: 8.5 × 107 E. coli DH5 α cells; calibration curves of the fluorescence intensities plotted against the protein concentration can be found in ESI S1;† N = 3. | |
3.4 Fluorescence-coupled flow cytometry detection of yeast cells with an immobilized protein linker
The flow cytometric analysis of the labeled and unlabeled yeast cells was performed by the selective binding of the protein linker or WGA-FITC followed by three washes and fluorescence detection. The yeast cells were stained with His6-SUMO-sfGFP-ChBD at 4.76 × 10−7 nmol per yeast cell to achieve an equilibrium mass of 9.48 μg, washed three times with 20 mM Tris buffer, and detected by flow cytometry. In comparison, the yeast cells were stained with WGA-FITC according to Powell et al.10
The Q–Q plots (Fig. S5 and S6†) indicated the data for the FSC-A and 525-A data were non-normally distributed, so Kruskal–Wallis tests were used to determine the significance of between-group differences. Q–Q plots are the most widely used graphic method for the investigation of the normality of data sets.79 The measured values are z-standardized, the theoretical quantiles are plotted on the x-axis and the actual quantiles on the y-axis. By including the normality line, which represents the ideal normal distribution, it can be determined whether the data is normally distributed. In the case of a normal distribution, the data would lie on the line or at least within the confidence interval.80 First, the FSC data (Fig. 2, left and Fig. S8†) of the yeast cells coupled with His6-SUMO-sfGFP-ChBD (test sample) were analyzed and compared to the reference (without linker) as well as to the WGA-FITC-labeled yeast cells. Kruskal–Wallis multiple comparison tests indicated significant differences between the FSC-A values of the three samples (p-value ≤ 0.0001), indicating a significant difference in the particle size and granularity. A post hoc Dunn Kruskal–Wallis multiple comparison test with p-values adjusted using the Holm method showed a more significant difference between the WGA-labeled yeast cells and the test sample (p-value ≤ 0.0001) than that between the test and reference samples (p-value ≤ 0.0001) (Table 1). The significant difference in particle size and granularity is due to the high number of particles. A graphical comparison showed that yeast cells with and without the protein linker exhibited similar morphology (Fig. S7†).
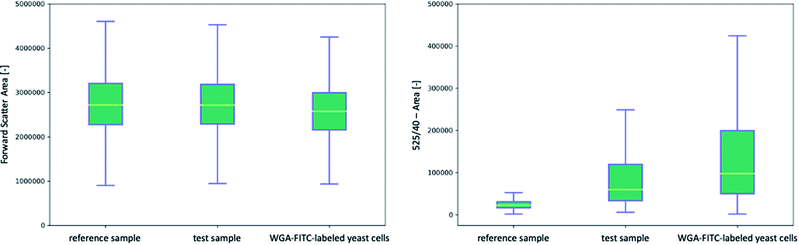 |
| Fig. 2 Comparison of the front scatter signals (left) and fluorescence signals (right) of three samples of unstained yeast cells (autofluorescence), yeast cells coupled with sfGFP containing protein linker (His6-SUMO-sfGFP-ChBD) and yeast cells, coupled with WGA-FITC; SUMO, small ubiquitin-like modifier; sfGFP, superfolder green fluorescent protein; ChBD, chitin-binding domain; yellow line: median of the dataset; box: first and third quartile; whisker: minimum and maximum excluding outliers detected by the interquartile range. | |
Table 1 Statistical analysis using the post hoc Dunn test
Comparison |
Adjusted p-value (−) |
FSC-A |
525-A |
Test sample vs. reference sample |
1.73 × 10−7 |
<0.0001 |
Test sample vs. WGA-labeled yeast |
1.06 × 10−81 |
<0.0001 |
Reference sample vs. WGA-labeled yeast |
4.26 × 10−131 |
<0.0001 |
Significant differences were also found in the fluorescence data at the 525-A channel (Fig. 2, right and Fig. S9†). A comparison of all three samples, the reference sample, a test sample, and WGA-FITC-labeled yeast showed an increase in median fluorescence signal from 21
996 ± 691 fluorescence units per cell to 60
455 ± 2706 in the test sample. WGA-FITC labeled yeast cells produced a fluorescence signal of 95
482 ± 2515. The post hoc Dunn test indicated a p-value of ≤0.0001 between the WGA-labeled yeast cells and the test sample. The p-value of the test and reference samples was also calculated to be ≤0.0001. It also can be seen that the autofluorescence signal of yeast cells overlaps with the signal, measured by both bud scar staining methods, limiting the possibility to determine yeast cells with fewer bud scars.
Both bud scar staining methods revealed a significant increase in fluorescence intensity caused by the protein linker. However, the 525-40-A detector signals of the yeast cells coupled with WGA-FITC showed a wide variation in fluorescence signal compared to the cells coupled with His6-SUMO-sfGFP-ChBD. A comparison of the histograms also verified that the signals varied between 1947 and 630
090 fluorescence units per cell in the WGA-FITC-labeled cells. The His6-SUMO-sfGFP-ChBD coupled yeast cells varied between 5935 and 367
566 fluorescence units. This variation may be addressed with additional washing steps. Nevertheless, our data demonstrated that both methods of staining yeast cells resulted in a significant increase in fluorescence units. Direct comparison of both methods indicated a fluorescence difference of 35
037 fluorescence units per cell of the test sample and the WGA-FITC labeled yeast cells, but also a two-fold higher variation in fluorescence signals.
In contrast to the existing method of Kurec et al., we compared the fluorescence signal of unstained yeast cells and stained yeast cells determined using flow cytometry. We showed a significant increase in the fluorescence signal, caused by the binding of the protein linker or WGA-FITC. A limitation of both approaches is that to date, the focus has been on bottom-fermenting yeast cells. Due to the required single-cell stage, no analysis of cell aggregates or cell groups, such as top fermenting cells81 is possible because these could lead to clogging of the capillaries. It is therefore unknown, whether there is the same correlation between the fluorescence signal of chitin compounds in bud scars and replicative cell age. Further research into this problem is necessary.
3.5 Microscopic verification of the protein linker binding to yeast cells
A non-invasive examination using MB staining showed a cell viability of 97.5% ± 0.9% in the control sample and 96.8% ± 1.6% for yeast cells with an immobilized protein linker. This result indicates that there was no significant impact of the protein binding on yeast viability.
Besides the determination of the binding parameters, the specific coupling of the protein linker to yeast cells was verified using CLSM. Levels of green fluorescence consistent with successful protein linker binding was observed (Fig. 3 and S10†). The areas of bud scars were associated with fluorescence due to protein linker binding, whereas other areas of the cell surface showed no detectable fluorescence, indicating that the protein linker bound specifically to chitin in surface scars on yeast cells. In contrast to Calcofluor white staining,37 which dyes the cell wall and bud scars, our method stains the bud scars specifically, as indicated by CLSM. Also, the flow cytometric applications of Calcofluor white require an excitation wavelength of 380 nm; thus, special equipment is needed for Calcofluor white staining. In contrast, our method is suitable for use with standard flow cytometry lasers, which use a wavelength of 488 nm, most frequently for GFP or fluorescein molecules. WGA-FITC images (Fig. S11 and S12†) also showed specific labelling of the bud scars, but also higher background level of signal. The visualized bud scars seemed to be less sharp than scars stained using the protein linker. Especially for budding yeast cells, the fluorescence signal was weak due to bud scar formation. Therefore, budding cells were not included in the analysis. This signal confirms the higher fluorescence intensity range, as reported in Section 3.4 and may be avoided by the use of more washing steps.
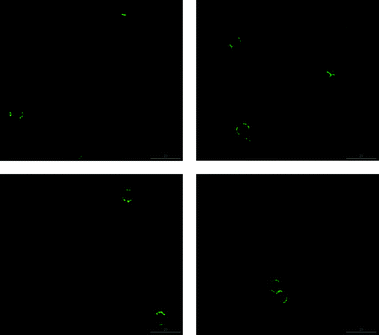 |
| Fig. 3 CLSM images of yeast cells that are successfully coupled with the His6-SUMO-sfGFP-ChBD protein linker from different cultures. CLSM, confocal laser scanning microscopy; scale bar indicates 20 μm. | |
The cell surface area can be calculated using the length and width of the non-budding cells measured from CLSM images. The cell surface areas were in the range of 131–493 μm2. Due to the lack of stacked imaging on an unmovable object table, the probability of bud scars occurring on the non-visible cell sides was calculated using Bayes theorem. Therefore, we estimated the absolute number of bud scares on the yeast cell from a given CLSM image via the calculated yeast cell surface according to the given interconnection of Powell et al.10 Since every cell has a minimum of one scar, the birth scar, the minimum absolute bud scar number was set to one. Bayesian statistics have previously been used in microscopy by Fuchigmi et al.82,83 Outliers were detected using the mvoutlier package of R, using a multivariate outlier analysis according to Filzmoser and Gschwandtner.84 The correlation between the mean fluorescence intensity and the mean bud scar number was calculated as y = 5700.20x + 66
841.35, with an R2 of 0.45 (Fig. 4), indicating an increase of fluorescence intensity with an increasing number of bud scars. The correlation between the median fluorescence intensity and the median bud scar number was calculated to be y = 4524.46x + 35
976.56 with an R2 of 0.82.
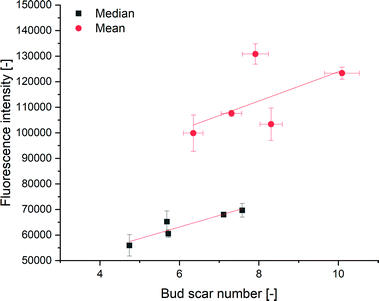 |
| Fig. 4 Correlation between the median bud scar number (minimum 300 cells per sample) and median fluorescence intensity (minimum 19.000 cells) of different aged yeast cell populations. Mean bud scar number and mean fluorescence intensity: R2 of 0.45. Median bud scar number and median fluorescence intensity: R2 of 0.82. | |
Unlike Kurec et al.,28 we used the median because the fluorescence intensity had a non-Gaussian distribution, as determined using Q–Q plots. Therefore, a better correlation produced using the median fluorescence was expected. Higher values of the mean, compared to the median indicated a right shift of the distribution. We also examined a higher number of cells per sample, thereby examining a representative part of the yeast population in our experiments. We could not compare the calibration curve of Kurec et al. with ours due to the dependency of the fluorescence signals on the instrument settings. Compared to existing methods, the technique's main advantage is the possibility of yeast separation or age-dependent flow manipulation using the polyhistidine tag included in the protein.
3.6 Chitin determination
To test for an increase of chitin with age, we detected the amount of chitin in yeast cells of different ages. The chitin content was determined by the acid hydrolysis of chitin to glucosamine, which reduces sodium molybdate to form a molybdosilicate anion. The anion can be detected by adsorption measurements at 750 nm.62
A calibration curve fit to eight points was determined to be y = 0.02932x (Fig. S10†). According to DIN32645
85 (Deutsches Institut für Normung), the detection limit was calculated to be 1.70 mg chitin (α = 95). By measuring yeast samples of different ages, a positive correlation between the mean bud scar number and the chitin content of the yeast cells was shown via linear regression to be y = 0.83695x + 1.322 with an R2 of 0.95 (Fig. 5). A positive correlation between the median number of bud scars and the chitin content could be shown, with an R2 of 0.85. Due to the presence of chitin components within the cell walls, the regression curve did not run through the origin. Accordingly, the assay showed that the yeast cells contained an average of 1.322% chitin. Hence, we could show for the first time that every bud scar appears to results in a significant increase in the chitin content of a yeast cell.
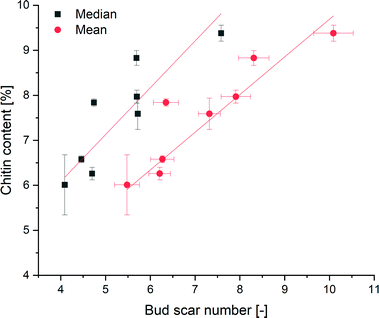 |
| Fig. 5 Correlation between the mean/median bud scar number and chitin content of yeast cells. | |
4 Conclusions
ChBD binding is reversible.86 Immobilization of ChBD to bud scars of yeast cells is non-invasive, and does not affect cell viability. Non-invasiveness is important to avoid changes in stress related autofluorescence. Furthermore, viability is important for the reuse of analyzed cells after cell sorting. One additional advantage is the high affinity of ChBD to chitin, and the coupling of the protein with sfGFP for subsequent detection by fluorescence microscopy and flow cytometry. In comparison to established protocols in the literature, the included polyhistidine-tag can be used for either purification or further applications such as immobilization of coupled yeast cells to solid surfaces for age-sorting. As demonstrated, a combination of this binding process with fluorescence-coupled flow cytometry has many advantages. We showed that the binding of His6-SUMO-sfGFP-ChBD to yeast cells resulted in a correlation between the median fluorescence intensity and median bud scar number. We also demonstrated a correlation between the chitin content and the number of bud scars on the yeast cells. Therefore, every bud scar probably results in a significant increase in the chitin content of a yeast cell. Our method is the basis for providing detailed insights into the age distribution in heterogeneous yeast cell populations, enables the determination of the impact of stress on yeast age distribution in industrial processes,87 and provides data about the replicative cell age-vitality interconnection.88 The method on hands will be used in industrial processes of brewing and wine-fermentations to analyze the interconnection between the fermentation performance or fermentation duration and the yeast cell age distribution. In industrial processes, the determination of yeast cell age distributions will provide a deeper understanding of yeast dynamics, and ways in which to refine quality.89 Further research tests the reliability to other yeast strains, enabling applications in other processes e.g. bakeries. Furthermore a deconvolution of the fluorescence signal and the autofluorescence signal of yeast cells is necessary before directly assess single cell replicative age. Our method provides a basis for determining the replicative age of yeast cells and offers a wide range of further possibilities for basic research, such as the interconnection between cell age and the fermentation power of aroma formation. Additionally, our approach is the basis for analyzing the singular cell age and the importance to process performance.
Author contributions
M. E., R. K. and T. B. conceptualized research; M. E. performed the experiments and the data analysis. M. E. wrote the manuscript. R. K. coordinated the research. R. K. and T. B. revised and discussed the manuscript.
Conflicts of interest
There are no conflicts to declare.
Acknowledgements
This work was funded by the Deutsche Forschungsgemeinschaft (DFG, German Research Foundation) – 441672360. We thank Dr Martin Haslbeck (Technical University of Munich), Univ.-Prof. Dr Christian Becker (Department of Biological Chemistry, Technical University of Vienna) and Gerhard Niederacher (Department of Biological Chemistry, Technical University of Vienna) for support of the plasmid construction process. Furthermore, we would like to thank Rolando Cesar Moreno Ravelo for supporting AFFF measurements, Prof. Dr Ramon Torres Ruiz (Technical University of Munich) for microscopic instructions and acknowledge the support of the Centre for Advanced Light Microscopy at the TUM School of Life Sciences.
Notes and references
- M. R. Rose, Evolutionary biology of aging, Oxford University Press, New York, 1991 Search PubMed
. - T. Flatt, Front. Genet., 2012, 3, 148 CrossRef PubMed
. - F. Mochaba, E. S. C. O'Connor-Cox and B. C. Axcell, J. Am. Soc. Brew. Chem., 1998, 56, 1–6 CrossRef CAS
. - H. M. Semchyshyn, L. M. Lozinska, J. Miedzobrodzki and V. I. Lushchak, Carbohydr. Res., 2011, 346, 933–938 CrossRef CAS PubMed
. - A. Leonov, R. Feldman, A. Piano, A. Arlia-Ciommo, V. Lutchman, M. Ahmadi, S. Elsaser, H. Fakim, M. Heshmati-Moghaddam, A. Hussain, S. Orfali, H. Rajen, N. Roofigari-Esfahani, L. Rosanelli and V. I. Titorenko, Oncotarget, 2017, 8, 69328–69350 CrossRef PubMed
. - M. Kwolek-Mirek and R. Zadrag-Tecza, FEMS Yeast Res., 2014, 14, 1068–1079 CAS
. - M. M. Y. Kwong, J. W. Lee, M. R. Samian, N. Watanabe, H. Osada and E. B. B. Ong, J. Microbiol. Methods, 2019, 167, 105743 CrossRef CAS PubMed
. - R. K. Mortimer and J. R. Johnston, Nature, 1959, 183, 1751–1752 CrossRef CAS PubMed
. - V. D. Longo, G. S. Shadel, M. Kaeberlein and B. K. Kennedy, Cell Metab., 2012, 16, 18–31 CrossRef CAS PubMed
. - C. D. Powell, D. E. Quain and K. A. Smart, Microbiology, 2003, 149, 3129–3137 CrossRef CAS PubMed
. - Y. Kamei, Y. Tamada, Y. Nakayama, E. Fukusaki and Y. Mukai, J. Biol. Chem., 2014, 289, 32081–32093 CrossRef CAS PubMed
. - C. B. Harley, A. B. Futcher and C. W. Greider, Nature, 1990, 345, 458–460 CrossRef CAS PubMed
. - K. A. Steinkraus, M. Kaeberlein and B. K. Kennedy, Annu. Rev. Cell Dev. Biol., 2008, 24, 29–54 CrossRef CAS PubMed
. - C. Guo, L. Sun, X. Chen and D. Zhang, Neural Regener. Res., 2013, 8, 2003–2014 CAS
. - G. Reverter-Branchat, E. Cabiscol, J. Tamarit and J. Ros, J. Biol. Chem., 2004, 279, 31983–31989 CrossRef CAS PubMed
. - M. Eigenfeld, R. Kerpes and T. Becker, Frontiers in Fungal Biology, 2021, 2, 665490 CrossRef
. - G. Perez-Samper, B. Cerulus, A. Jariani, L. Vermeersch, N. Barrajón Simancas, M. M. M. Bisschops, J. van den Brink, D. Solis-Escalante, B. Gallone, D. De Maeyer, E. van Bael, T. Wenseleers, J. Michiels, K. Marchal, P. Daran-Lapujade and K. J. Verstrepen, mBio, 2018, 9, e01331-18 CrossRef PubMed
. - C. M. Rogers, D. Veatch, A. Covey, C. Staton and M. L. Bochman, Food Microbiol., 2016, 57, 151–158 CrossRef CAS PubMed
. - X. Hakkaart, Y. Liu, M. Hulst, A. el Masoudi, E. Peuscher, J. Pronk, W. van Gulik and P. Daran-Lapujade, Biotechnol. Bioeng., 2020, 117, 721–735 CrossRef CAS PubMed
. - E. Navarro-Tapia, R. K. Nana, A. Querol and R. Pérez-Torrado, Food Microbiol., 2016, 7, 189 Search PubMed
. - J. Verghese, J. Abrams, Y. Wang and K. A. Morano, Microbiol.
Mol. Biol. Rev., 2012, 76, 115–158 CrossRef CAS PubMed
. - N. K. Egilmez and S. M. Jazwinski, J. Bacteriol., 1989, 171, 37–42 CrossRef CAS PubMed
. - H. Mashmoushy, Z. Zhang and C. R. Thomas, Biotechnol. Tech., 1998, 12, 925–929 CrossRef CAS
. - C. L. Woldringh, K. Fluiter and P. G. Huls, Yeast, 1995, 11, 361–369 CrossRef CAS PubMed
. - A. Svenkrtova, L. Belicova, A. Volejnikova, K. Sigler, S. M. Jazwinski and A. Pichova, Biogerontology, 2016, 17, 395–408 CrossRef CAS PubMed
. - Y. Zhang, C. Luo, K. Zou, Z. Xie, O. Brandman, Q. Ouyang and H. Li, PLoS One, 2012, 7, e48275 CrossRef PubMed
. - S. S. Lee, I. A. Vizcarra, D. H. E. W. Huberts, L. P. Lee and M. Heinemann, Proc. Natl. Acad. Sci. U. S. A., 2012, 109, 4916–4920 CrossRef CAS PubMed
. - M. Kuřec, M. Baszczyňski, R. Lehnert, A. Mota, J. A. Teixeira and T. Brányik, J. Inst. Brew., 2009, 115, 253–258 CrossRef
. - S. Luschnig, T. Bätz, K. Armbruster and M. A. Krasnow, Curr. Biol., 2006, 16, 186–194 CrossRef CAS PubMed
. - G. Qin, S. Lapidot, K. Numata, X. Hu, S. Meirovitch, M. Dekel, I. Podoler, O. Shoseyov and D. L. Kaplan, Biomacromolecules, 2009, 10, 3227–3234 CrossRef CAS PubMed
. - L. F. Sobala, Y. Wang and P. N. Adler, Development, 2015, 142, 3974–3981 CAS
. - H. Okada and Y. Ohya, Cold Spring Harbor Protocols, 2016, 2016, pdb.prot085241 CrossRef PubMed
. - J. J. Lichty, J. L. Malecki, H. D. Agnew, D. J. Michelson-Horowitz and S. Tan, Protein Expression Purif., 2005, 41, 98–105 CrossRef CAS PubMed
. - K. Fushimi, S. Uchida, R. Matsushita and T. Tsukahara, Sci. Technol. Adv. Mater., 2005, 6, 475–483 CrossRef CAS
. - B. Harrington and G. Hageage, Lab. Med., 2003, 34, 361–367 CrossRef
. - J. R. Pringle, in Methods Enzymol, Academic Press, 1991, vol. 194, pp. 732–735 Search PubMed
. - I. H. Hidalgo, T. Fleming, V. Eckstein, S. Herzig, P. P. Nawroth and J. Tyedmers, BioTechniques, 2016, 61, 137–148 CrossRef CAS PubMed
. - E. J. Lodolo, J. L. F. Kock, B. C. Axcell and M. Brooks, FEMS Yeast Res., 2008, 8, 1018–1036 CrossRef CAS PubMed
. - E. Matallana and A. Aranda, Lett. Appl. Microbiol., 2017, 64, 103–110 CrossRef CAS PubMed
. - W. H. van Zyl, L. R. Lynd, R. den Haan and J. E. McBride, in Biofuels, ed. L. Olsson, Springer Berlin Heidelberg, Berlin, Heidelberg, 2007, pp. 205–235, DOI:10.1007/10_2007_061
. - T. Vogl and A. Glieder, New Biotechnol., 2013, 30, 385–404 CrossRef CAS PubMed
. - D. Zavec, B. Gasser and D. Mattanovich, Biotechnol. Bioeng., 2020, 117, 1394–1405 CrossRef CAS PubMed
. - P. Zhou, W. Xie, Z. Yao, Y. Zhu, L. Ye and H. Yu, Biotechnol. Bioeng., 2018, 115, 1321–1330 CrossRef CAS PubMed
. - I. Virkajärvi and J. Kronlöf, J. Am. Soc. Brew. Chem., 1998, 56, 70–75 CrossRef
. - E. J. Pires, J. A. Teixeira, T. Brányik, M. Côrte-Real and A. A. Vicente, J. Inst. Brew., 2014, 120, 52–59 CrossRef CAS
. - C. C. Lechner and C. F. W. Becker, Biomater. Sci., 2015, 3, 288–297 RSC
. - C. H. Schein and M. H. M. Noteborn, Bio/Technology, 1988, 6, 291–294 CAS
. - A. Vera, N. González-Montalbán, A. Arís and A. Villaverde, Biotechnol. Bioeng., 2007, 96, 1101–1106 CrossRef CAS PubMed
. - J.-M. Malho, H. Heinonen, I. Kontro, N. E. Mushi, R. Serimaa, H.-P. Hentze, M. B. Linder and G. R. Szilvay, Chem. Commun., 2014, 50, 7348–7351 RSC
. - M. M. Bradford, Anal. Biochem., 1976, 72, 248–254 CrossRef CAS PubMed
. - S. C. Gill and P. H. von Hippel, Anal. Biochem., 1989, 182, 319–326 CrossRef CAS PubMed
. - S. R. Haider, H. J. Reid and B. L. Sharp, in Protein Electrophoresis: Methods and Protocols, Humana Press, Totowa, NJ, 2012, pp. 81–91, DOI:10.1007/978-1-61779-821-4_8
. - H. Towbin, T. Staehelin and J. Gordon, Proc. Natl. Acad. Sci. U. S. A., 1979, 76, 4350–4354 CrossRef CAS PubMed
. - S. P. Schwaminger, P. Fraga-García, S. A. Blank-Shim, T. Straub, M. Haslbeck, F. Muraca, K. A. Dawson and S. Berensmeier, ACS Omega, 2019, 4, 3790–3799 CrossRef CAS PubMed
. - C. C. Serdar, M. Cihan, D. Yücel and M. A. Serdar, Biochem. Med., 2021, 31, 010502 Search PubMed
. - W. G. Cochran, Stichprobenverfahren, de Gruyter, Berlin, 1972 Search PubMed
. - R Core Team, R: A language and environment for statistical computing, R Foundation for Statistical Computing, Vienna, Austria, 2021, https://www.R-project.org/ Search PubMed
. - P. Filzmoser, R. G. Garrett and C. Reimann, Comput. Geosci., 2005, 31, 579–587 CrossRef CAS
. - W. T. Corporation, 2016, 21, https://wyatttechnology.zendesk.com/hc/en-us/articles/360037324853-Technical-Notes.
- D. Bathen and M. Breitbach, in Adsorptionstechnik, Springer Berlin Heidelberg, Berlin, Heidelberg, 2001, pp. 49–101, DOI:10.1007/978-3-642-18235-8_3
. - N. Dallies, J. François and V. Paquet, Yeast, 1998, 14, 1297–1306 CrossRef CAS PubMed
. - H. Katano, M. Takakuwa, H. Hayakawa and H. Kimoto, Anal. Sci., 2016, 32, 701–703 CrossRef CAS PubMed
. - H. Waegeman, S. De Lausnay, J. Beauprez, J. Maertens, M. De Mey and W. Soetaert, New Biotechnol., 2013, 30, 255–261 CrossRef CAS PubMed
. - C. Lopes, N. V. dos Santos, J. Dupont, D. B. Pedrolli, S. R. Valentini, V. de Carvalho Santos-Ebinuma and J. F. B. Pereira, Biotechnol. Appl. Biochem., 2019, 66, 527–536 CrossRef CAS PubMed
. - A. Nagy, A. Malnasi and B. Somogyi, Thermochim. Acta, 2004, 410, 161–163 CrossRef CAS
. - L. C. de Lencastre Novaes, P. G. Mazzola, A. Pessoa Jr and T. C. V. Penna, Biotechnol. Prog., 2011, 27, 269–272 CrossRef PubMed
. - M. Ishii, J. S. Kunimura, H. T. Jeng, T. C. V. Penna and O. Cholewa, Appl. Biochem. Biotechnol., 2007, 137, 555–571 CrossRef PubMed
. - M. Danaei, M. Dehghankhold, S. Ataei, F. Hasanzadeh Davarani, R. Javanmard, A. Dokhani, S. Khorasani and M. R. Mozafari, Pharmaceutics, 2018, 10, 57 CrossRef PubMed
. - M. Marioli and W. T. Kok, Anal. Bioanal. Chem., 2019, 411, 2327–2338 CrossRef CAS PubMed
. - C. R. M. Bria, F. Afshinnia, P. W. Skelly, T. M. Rajendiran, P. Kayampilly, T. P. Thomas, V. P. Andreev, S. Pennathur and S. Kim Ratanathanawongs Williams, Anal. Bioanal. Chem., 2019, 411, 777–786 CrossRef CAS PubMed
. - K. Eskelin, M. M. Poranen and H. M. Oksanen, Microorganisms, 2019, 7, 555 CrossRef CAS PubMed
. - S. Vogt, M. Kelkenberg, T. Nöll, B. Steinhoff, H. Schönherr, H. Merzendorfer and G. Nöll, Analyst, 2018, 143, 5255–5263 RSC
. - M. Hardt and R. A. Laine, Arch. Biochem. Biophys., 2004, 426, 286–297 CrossRef CAS PubMed
. - G. Vaaje-Kolstad, D. R. Houston, A. H. K. Riemen, V. G. H. Eijsink and D. M. F. van Aalten, J. Biol. Chem., 2005, 280, 11313–11319 CrossRef CAS PubMed
. - Y. Itakura, S. Nakamura-Tsuruta, J. Kominami, H. Tateno and J. Hirabayashi, Int. J. Mol. Sci., 2017, 18, 1160 CrossRef PubMed
. - R. A. Latour, J. Biomed. Mater. Res., Part A, 2015, 103, 949–958 CrossRef PubMed
. - A. Frutiger, A. Tanno, S. Hwu, R. F. Tiefenauer, J. Vörös and N. Nakatsuka, Chem. Rev., 2021, 121, 8095–8160 CrossRef CAS PubMed
. - S. P. Schwaminger, S. A. Blank-Shim, I. Scheifele, P. Fraga-García and S. Berensmeier, Faraday Discuss., 2017, 204, 233–250 RSC
. - N. Mohd Razali and B. Yap, Journal of Statistical Modeling and Analytics, 2011, 2, 21–33 Search PubMed
. - P. P. Eckstein, in Angewandte Statistik mit SPSS: Praktische Einführung für Wirtschaftswissenschaftler, Springer Fachmedien Wiesbaden, Wiesbaden, 2016, pp. 55–106, DOI: DOI:10.1007/978-3-658-10918-9_3
. - M. Hutzler, J. Koob, R. Riedl, H. Schneiderbanger, K. Mueller-Auffermann and F. Jacob, in Brewing Microbiology, ed. A. E. Hill, Woodhead Publishing, Oxford, 2015, pp. 65–104, DOI: DOI:10.1016/B978-1-78242-331-7.00005-8
. - S. Fuchigami, T. Niina and S. Takada, Front. Mol. Biosci., 2021, 8, 636940 CrossRef PubMed
. - P. Cossio and G. Hummer, J. Struct. Biol., 2013, 184, 427–437 CrossRef CAS PubMed
. - P. Filzmoser and M. Geschwandtner, 2018, https://cran.r-project.org/web/packages/mvoutlier/index.html.
- Deutsches Institut für Normung e.V., DIN 32645:2008-11, Beuth-Verlag, Berlin, 2008 Search PubMed
. - J.-T. Chern and Y.-P. Chao, J. Biotechnol., 2005, 117, 267–275 CrossRef CAS PubMed
. - I. W. Dawes and G. G. Perrone, FEMS Yeast Res., 2019, 20, foz085 CrossRef PubMed
. - S. N. Mouton, D. J. Thaller, M. M. Crane, I. L. Rempel, A. Steen, M. Kaeberlein, C. P. Lusk, A. J. Boersma and L. M. Veenhoff, bioRxiv, 2019, 858720, DOI:10.1101/858720
. - A. Rudolph, A. J. MacIntosh, R. A. Speers and C. St. Mary, J. Am. Soc. Brew. Chem., 2020, 78, 63–73 CrossRef CAS
.
Footnote |
† Electronic supplementary information (ESI) available. See DOI: 10.1039/d1ra05276d |
|
This journal is © The Royal Society of Chemistry 2021 |
Click here to see how this site uses Cookies. View our privacy policy here.