DOI:
10.1039/D1RA04254H
(Paper)
RSC Adv., 2021,
11, 28565-28572
Non-invasive Raman spectroscopy for time-resolved in-line lipidomics†
Received
1st June 2021
, Accepted 5th August 2021
First published on 24th August 2021
Abstract
Oil-producing yeast cells are a valuable alternative source for palm oil production and, hence, may be one important piece of the puzzle for a more sustainable future. To achieve a high-quality product, the lipid composition inside oil-producing yeast cells is a crucial parameter for effective process control. Typically, the lipid composition is determined by off-line gas chromatography. A faster, less cumbersome approach is proposed here, by using non-invasive in-line Raman spectroscopy. A fed-batch fermentation of C. oleaginosus – a well-known oleaginous yeast – is used as model experiment to highlight the potential of Raman spectroscopy for in-line lipidomics. The temporal progression of biomass formation, lipid production and glucose consumption are determined based on PLS-regression models allowing process-relevant information on time to be accessed. Additionally, Gaussian curve fitting was applied to extract increasing and decreasing trends of saturated and unsaturated fatty acids produced by C. oleaginosus throughout the fermentation process.
1. Introduction
The world is looking to slow down the effect of climate change and preserve biodiversity by offering sustainable solutions to implement the cyclic-bioeconomy concept. In that respect, the conversion of tropical rainforest and peat bog to mono-culturing plantations, such as oil palm trees, is a major challenge that frustrates all efforts to meet the world's sustainability goals.1,2 In addition to threatening sensitive wildlife, deforestation emits massive amounts of greenhouse gas (GHG), estimated to be in the range of 70–117 t CO2 eq per ha per year.3 Therefore, the life-cycle of crude palm oil (CPO) is assessed at 30.24 tCO2 eq tCPO−1 on the newly cleared forest.4 Nevertheless, global palm oil demand is constantly rising due to its outstanding physical and chemical properties and areal productivities. To that end, meeting the market and prosperity demand while preserving environmental standards requires the decoupling of the edible oil sourcing from the mono-culturing methodology.
The similarity in fatty acid composition and characteristic texture remarkably drives the attention to yeast oil as a sustainable alternative to palm and cocoa oils in the last years.5–7 Cutaneotrichosporon oleaginosus, one of the widely used oily yeast, shows unique abilities in the growth to a high cell density with lipid content exceeding 80%.8 C. oleaginosus efficiently catabolites complex carbon matrix from a wide variety of waste streams and biomass.9,10 It shows a high toxicity tolerance10,11 and simultaneous up-taking of co-feeding such as xylose and glucose or acetic acid with the glucose.11,12
The oily yeast fermentation industry needs to implement an in situ method that offers an instantaneous pursuing for the culture's vitality and productivity. Such a method allows continuous quality control on time to adapt and optimize process control parameters if needed and, hence, reduce the overall costs for yeast oil. From an industrial point of view, the usage of yeast oil in a particular application is strongly dependent on the fatty acids (FAs) profile of the oil. A highly saturated fatty acid is needed in some applications, whilst other usages require liquid oil with more unsaturated fatty acid. As FAs composition is changed over the fermentation time, it is essential to find an immediate monitoring method to indicate the best harvesting time. In that regard, several rapid in-process monitoring tools have been reported. For example, a high-throughput lipid qualification methodology based on a Nile red spectrofluorimetric assay or flow cytometry has been described recently as rapid biomass, growth, and lipid accumulation monitoring method.13,14 However, these approaches are considered off-line analysis where samples from the bioreactor are drawn at defined points in time (e.g. every 2 hours). Here, the information gained by analysis is limited regarding its significance for the ongoing process as it only mirrors e.g. the lipid content at one specific point in time. Sudden changes cannot be captured until the next sample is drawn. This means that valuable time to set certain actions in process control – if necessary – is lost. A more accurate picture of the process can be gained by in-line monitoring, where a sensor is inserted into the bioreactor to gain information of the process with high frequency (minutes instead of hours). This approach, however, creates high demands for the sensor material since it needs to be autoclavable, inert and stable over time and, thus, pushes the costs upwards.
Raman spectroscopy is a valuable tool in a process analytical chemist's toolbox.15 It has been proven beneficial for in-line monitoring of various organisms such as bacteria,16,17 fungi,18 yeast,19–22 or mammalian cells.23 Raman spectroscopy is a non-invasive, optical method that mirrors the chemical composition of the probed focal volume. Upon interaction of the monochromatic laser light with the sample, the inelastically (back-)scattered light is detected corresponding to vibrational transitions in the molecule, hence, providing characteristic, molecule-specific information. Raman spectroscopy can be used in a confocal arrangement allowing reaction monitoring without the probe being in direct contact with the fermentation broth. Nonetheless, a transparent window is required for the Raman probe to enable continuous monitoring. This non-invasive monitoring approach offers several advantages such as lower material requirements for the in-line sensor (e.g. aluminum instead of stainless steel) which in turn reduces costs.
The successful study of lipids by means of Raman spectroscopy has been shown extensively in literature.24–27 For example, in 2011, Wu et al. described an approach based on single-cell Raman spectroscopy to determine lipid composition in oil-producing microalgae.26 They demonstrated that there is a linear correlation between the ratio of bands at 1650 cm−1 (C
C str. lipids) and 1440 cm−1 (CH2 def. lipids), and the number of C
C bonds. In 2018, Kochan et al. used the same band ratio to determine the degree of unsaturation of lipid bodies in genetically modified yeast cells.24 They also mention that the comparison of intensities at 3012 cm−1 (
CH str. lipids) and 2855 cm−1 (CH3 str. lipids) are less sensitive to changes in the degree of unsaturation. Grace et al. demonstrated the applicability of both, infrared and Raman spectroscopy to determine the ratio of unsaturated vs. saturated fatty acids.27 In their study, they used freeze-dried samples while others drop cast a small amount of sample on a substrate and let it dry prior to off-line Raman or infrared analysis. Here, we investigate the ability of Raman spectroscopy in combination with different data analysis strategies to investigate the lipid composition in yeast cells in an in-line monitoring approach. Besides classical PLS (partial least squares) regression providing comprehensive information on biomass growth, feedstock consumption, and lipid accumulation on time, Gaussian curve fitting is employed to explore the possibility of extracting information on the production of unsaturated fatty acids (UFAs), and saturated fatty acids (SAFs). Especially the latter ones are essential quality control parameters for oil-producing microorganisms typically accessed by tedious off-line GC analysis.
2. Experimental
2.1 Strain and medium components
The well-known oleaginous yeast C. oleaginosus (ATCC 20509) was cultivated in Yeast extract–Peptone–Dextrose broth medium (YPD) containing 10 g L−1 yeast extract, 20 g L−1 peptone, and 20 g L−1 glucose. 10 mg L−1 ampicillin, 10 mg L−1 kanamycin, and 12 mg L−1 tetracycline were added to avoid any cross-contamination. The inoculum was incubated in a rotary shaker (2 days, 100 rpm, and 28 °C).
2.2 Bioreactor and process parameters
Bioengineering fermentation system (Bioengineering AG, Switzerland) with a working volume of 50 L was used for the cultivation of C. oleaginosus. The applied parameters were as follows: temperature: 28 °C, pH 6.5 ± 0.02, and 120 h. The dissolved oxygen was automatically controlled (pO2 > 50%) by regulating stirrer speed (350–800 rpm), aeration (0.8–1.5 vvm), and pressure (1.0–1.25 bar). The pH of the bioreactor was adjusted with 3 M NaOH and 1 M HCl. Antifoam agent (Antifoam 204; Merck) was used to avoid foaming. The starting medium composition of the main nutrition was as follows: 30.0 g L−1 glucose and 0.5 g L−1 yeast extract; 0.2 g L−1 (NH4)2SO4, 2.4 g L−1 KH2PO4, 0.9 g L−1 Na2HPO4·12H2O, 1.5 g L−1 MgSO4·7H2O, 0.025 g L−1 FeCl3·6H2O, 0.001 g L−1 ZnSO4·7H2O, 0.2 g L−1 CaCl2·2H2O, 0.024 g L−1 MnSO4·5H2O, 0.025 g L−1 CuSO4·5H2O, and 0.003 g L−1 Co(NO3)2·6H2O. A glucose feed (50% w/w) was started after the initial batch phase of 18 h with a constant feeding rate of 3 g L−1 h−1 between 24 and 72 h. Between 72 and 120 h, the glucose feed rate was reduced to 1.5 g L−1 h−1.
2.3 In-line Raman spectroscopy
A tec5 MultiSpec® process Raman spectrometer (tec5 AG, Steinbach, Germany) equipped with a temperature-stabilized 785 nm diode laser (500 mW power output), a Peltier cooled 16 bit CCD diode array spectral sensor, and a Raman probe (Process Raman Probe 785 mini/MUB25 mm for Ingold socket) with a focal length of 0.5 mm was employed for sensitive in-line analysis covering the spectral range between 300 and 3100 cm−1 with a spectral resolution of 7 cm−1. Balancing signal-to-noise ratio (SNR) and measurement intervals, an integration time of 4 × 30 s was chosen, resulting in a measurement interval of 2 minutes. To account for inside light fluctuations due to day/night cycles and medium turbidity, a dark current correction was performed every 3 hours with identical parameter settings.
The Raman probe was only inserted into the SCHOTT ViewPort™ (SCHOTT AG, Landshut, Germany) immediately before the start of the fermentation whereas the ViewPort™ was autoclaved with the vessel. The optical properties of the Raman probe were chosen to allow focusing through the sapphire window of the ViewPort™ into the medium. Due to the combination of ViewPort™ and Raman probe, there is no need for the probe to be autoclaved, as there is no contact between probe and medium at any time. Hence, material requirements for the probe are lower allowing a more cost-efficient probe design. It also enables a more flexible probe usage, as the probe can easily be removed during the fermentation process e.g. for re-calibration purposes or to use a different port also equipped with a ViewPort™ losing neither sterile conditions nor longer-term control of the process parameters derived from the respective measuring system.
2.3.1 Data processing. Data processing steps and subsequent data analysis were performed in MATLAB (R2019b, The MathWorks Inc., USA), Eigenvector MIA toolbox (Eigenvector Research Inc., WA, USA), and Python (numpy, scipy and matplotlib libraries for version 3.6). First, the spectral fingerprint between 400 cm−1 and 2000 cm−1 was selected (Fig. 1B) to reduce the hyperspectral data set and speed up analysis time. Second, spectral artifacts depicted as sharp negative bands in the spectra (Fig. 1A) were removed by excluding these data points from the data matrix followed by an interpolation step with a cubic function to close the spectral gaps of 4–7 wavenumbers. The spectral artifacts originate from the automatic dark current correction performed every 3 hours and the fluctuating effect of inside lighting. To increase the SNR and remove any effects from the interpolation step, Savitzky–Golay smoothing (1st order polynomial, 11 pt window size) was applied. Finally, baseline correction was performed using the Automatic Whittaker Filter algorithm with λ = 10 and p = 10−5 (Fig. 1C). The spectral region between 400 cm−1 and 1800 cm−1 featuring bands evoked by glucose, lipids and yeast/biomass was used for data analysis (Fig. 1D).
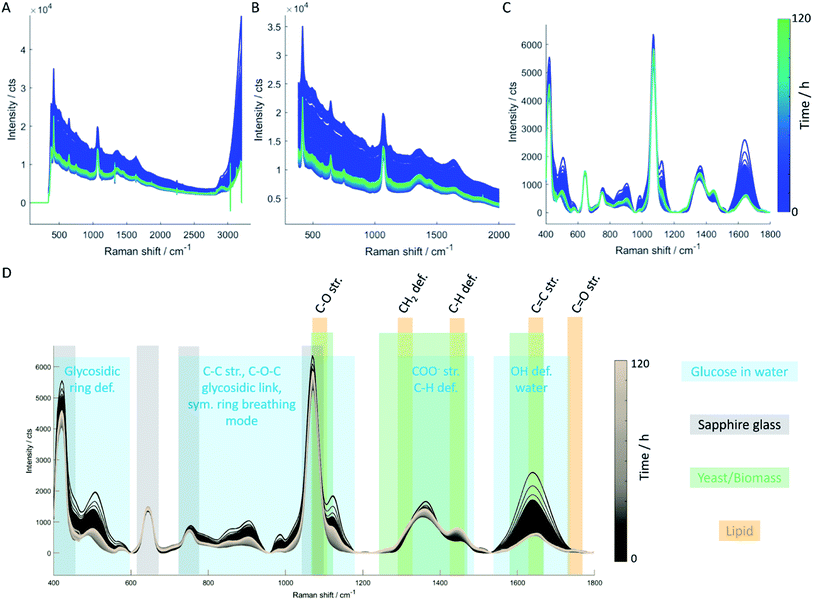 |
| Fig. 1 Data processing steps of in-line Raman spectra. The spectral fingerprint between 400 cm−1 and 2000 cm−1 of unprocessed data (A) was selected and sharp negative bands were removed through interpolation (B). Next, Savitzky–Golay smoothing, and baseline correction was applied (C). For data evaluation, the spectral region between 400 cm−1 and 1800 cm−1 was employed which contains Raman bands of carbohydrates, protein, and lipid (D). | |
2.3.2 PLS regression. For each component of interest (glucose, biomass, total lipid content), a PLS regression model was obtained using the Eigenvector MIA toolbox (Eigenvector Research Inc., WA, USA). Each model was built based on the concentration values determined by off-line analysis (biomass dry weight, glucose concentration, total lipid content in dry weight biomass) of the samples drawn during the 120 h-fermentation. For the glucose model, the complete spectral fingerprint region (400–1800 cm−1) was used after calculating the 2nd derivative (with Savitzky–Golay smoothing: 2nd order polynomial; 15 pt window size) and mean centering. The model for the total lipid content was established based on the spectral region between 1200 cm−1 and 1800 cm−1 exhibiting the most important spectral features attributed to lipids (Fig. 1D). The spectra were processed by calculating the 2nd derivative (2nd order polynomial, 11 pt window size) and mean centering. The same spectral region (1200–1800 cm−1) was employed for the biomass regression model applying 1st derivative (2nd order polynomial, 15 pt window size) and mean centering. The calibration dataset comprises spectra that were recorded by the in-line Raman probe at the same time point the sample was drawn. The model was then applied to the remaining 2805 spectra serving as a test dataset.
2.4 Off-line analysis
Biomass growth was estimated by measuring the optical density at 600 nm, whereas gravimetry was applied to quantify dry biomass. The sugar concentration in the medium was analyzed by high-performance liquid chromatography (HPLC; Agilent 1100 series). The total lipid content was determined by the Bligh–Dyer method. GC-FID was used to measure the fatty acid profiles. Detailed procedures are discussed elsewhere.8,28
2.4.1 Raman analysis. 1 mL-aliquots of the samples drawn during the fermentation process were used for off-line Raman analysis. To analyze either the medium composition or the lipid content in single yeast cells over time, the cells were separated from the medium by centrifugation. This step, however, was hampered by increasing lipid content in the yeast cells. Nevertheless, medium spectra could be obtained without yeast cell interference. For single-cell analysis, samples with higher cell densities were diluted with deionized water 1
:
20, 1
:
30, 1
:
50, or 1
:
100, depending on the respective cell density. Samples were filled into small channels (20 × 0.5 × 0.2 mm3) formed by a double-sided adhesive tape on top of a microscope glass slide, covered with a coverslip, and sealed with wax after filling.A WITec alpha300R spectrometer (WITec GmbH, Ulm, Germany) equipped with a Cobolt DPL 532 nm solid-state laser (Cobolt AB, Sona, Sweden), a 600 L mm−1 grating, true-power module for stable laser power output set to 50 mW, and a 50× LWD objective (N.A. 0.55, Carl Zeiss AG, Germany) was used for single-point spectra acquisition and imaging purposes. The Stokes-shifted Raman photons were collected with a Newton CCD camera (Andor Technology Ltd, Belfast, UK). Single spectra were acquired using the same integration time as for in-line Raman spectroscopy (4 × 30 s) whereas an integration time of 1 × 0.5 s was set for Raman images of single yeast cells with a step size of ≈300–400 nm in x- and y-direction.
Single spectra were processed with ProjectFIVE (WITec GmbH, Ulm Germany) software using cosmic ray removal (filter size: 4, dynamic factor: 3) and baseline correction (shape size: 500, noise factor: 2). Raman images were processed similar to the single spectra (cosmic ray removal, baseline correction) in Project FIVE (WITec GmbH, Ulm Germany). Background pixels were excluded by PCA of the standardized data (
= 0, σ = ±1). Subsequently, the ratio of
C–H at 3012 cm−1 and the CH3 stretch vibration of lipids (2855 cm−1) was calculated. To visualize the degree of unsaturation within single yeast cells drawn at different points in time, HCA of the mean-centered data was calculated using Ward's method and Mahalanobis distance.
3. Results and discussion
Fed-batch fermentation of C. oleaginosus has been designed as a model experiment for the in-line assessment of biomass production, glucose consumption, or lipid production. Glucose was applied as the sole carbon source. Biomass growth, lipid accumulation, and glucose consumption were measured over the fermentation time with well-known classical off-line procedures (Fig. 2). In parallel, the in-line Raman spectroscopy sensor was set up for non-invasive in-line monitoring. A sapphire window separates the sensor from the medium, still allowing continuous measurements of the reaction inside the bioreactor. This approach was chosen as it allows flexible, and cost-efficient reaction monitoring with a multitude of parameters that can be extracted from a single Raman spectrum (e.g. glucose consumption, lipid production, etc.). Finally, the selection of standard off-line analysis methods (see Fig. 2) was completed by off-line Raman spectroscopy of both, the medium and single yeast cells. Off-line analysis data serve as a foundation for regression model development and are used for “counter-check”-purposes with in-line data analysis results.
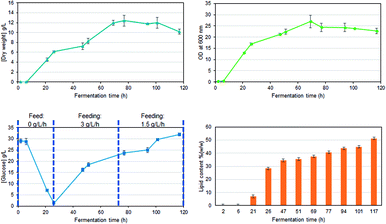 |
| Fig. 2 Growth rates, substrate consumption, and lipid accumulation, for a glucose fed-batch fermentation of C. oleaginosus at 28 °C, pH 6.5, for 120 h. | |
3.1 Off-line analysis of the fermentation medium
Off-line Raman spectra of the fermentation medium are dominated by bands such as the C–C–C and C–C–O deformation vibrations between 420 cm−1 and 530 cm−1, the C–C and C–H stretch vibrations between 850 cm−1 and 920 cm−1, the C–O stretch vibration at 1070 cm−1, the C–O–H deformation vibration at 1127 cm−1 and the CH2 wagging vibration at 1370 cm−1 which can all be assigned to glucose.29 Integration of the spectral region attributed to C–O stretch and C–O–H deformation vibration (1000–1180 cm−1; highlighted in gray in Fig. 3A) for each sampling time allows establishing a fragmented temporal progression of glucose content in the medium during the fermentation process (Fig. 3B) which is in good agreement with HPLC reference data (Fig. 3C). First, glucose is consumed by the yeast cells. After 24 hours, a 3 g L−1 h−1 glucose feed was started to ensure a constant carbon source supply. The glucose concentration increases again indicating that the yeast cells need significantly less glucose as is fed to them. After 72 hours, the glucose feed was reduced to 1.5 g L−1 h−1 resulting in a final glucose concentration similar to the initial amount set at the beginning of the fermentation. Comparison of the integrated spectral range of the Raman spectrum which is directly proportional to the glucose content in the medium with the glucose concentration determined by HPLC measurements confirms a linear correlation (Fig. 3C).
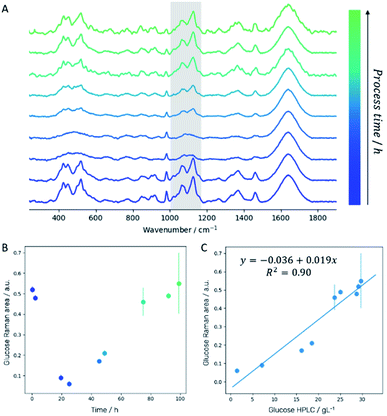 |
| Fig. 3 (A) Raman spectra of fermentation medium indicating decrease and increase of glucose during fermentation. (B) Temporal progression of glucose (integral of the spectral region between 1000 cm−1 and 1180 cm−1 highlighted in gray in panel (A)) concentration. (C) Linear correlation between glucose Raman signal and the glucose concentration determined by HPLC. | |
3.2 Quantitative analysis based on in-line Raman spectroscopy
The temporal progression of glucose, lipid, and biomass (Fig. 4A) concentrations was determined based on PLS regression (Fig. 4B). Since a Raman spectrum has been collected every 2 minutes, the in-line Raman monitoring provides prompt feedback on changes that might occur during the fermentation process such as contaminations or problems with cell reproduction which would ultimately result in a lower product yield. All these conditions are reflected in the Raman data e.g. by a less steep increase of biomass, the C-source that despite the calculations of a process specialist is not fully consumed (increase in glucose concentration) or the lipid concentration that is lower than expected. Furthermore, in our case study, the product (lipids) can be consumed by the yeast cells after production if conditions are not right, thus resulting in a decrease in product yield. All these different states can effectively be mirrored by in-line Raman spectroscopy supported by mathematical models that help predict the temporal progression of selected components on time. Specifically, we see a decrease in glucose concentration during the first 24 hours while the biomass exponentially increases. Also, lipid production is started during this time frame, after roughly 10 hours. In the subsequent time frame (24–72 h), the glucose feed set to 3 g L−1 h−1 aims to keep the concentration of the C-source at a constant level such that the lipid production by yeast cells would not be hampered by a glucose shortage. Biomass and lipid concentration further increase; however, also the glucose concentration in the medium increases, indicating an imbalance between C-source supply and demand. Towards the end of this time frame, the lipid concentration seems to have reached a plateau while the biomass is still increasing and only plateaus in the last time frame (72–120 h). Here, the glucose feed is reduced to 1.5 g L−1 h−1; however, the glucose concentration in the medium is still increasing, indicating an excess of glucose. During the last 48 hours of the fermentation, no significant increase is detected neither for lipid nor for biomass concentration. Hence, to operate at an optimal workload of the bioreactors and, therefore, to minimize costs, the fermentation could have been stopped after 72 hours which would have reduced the overall fermentation time by 40%, saved costs for raw material, and allowed an earlier start of cleaning and refilling for the next production cycle.
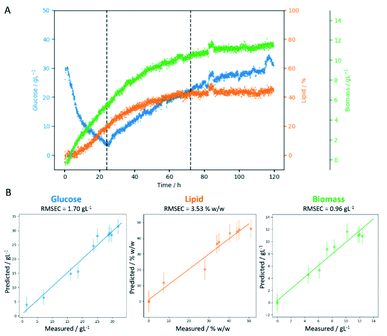 |
| Fig. 4 Concentration profiles of glucose, lipid, and biomass during the 120 h fermentation (A) based on PLS regression models (B) established for each of the components using offline measurement data. | |
3.3 Off-line single-cell lipidomics
The change in the degree of unsaturation of the lipids produced by the yeast cells was first investigated through an off-line Raman imaging approach. Single yeast cells drawn at four different points in time (after 0.5, 2.23, 19.5, and 45.25 h of fermentation time, respectively) were analyzed collecting Raman images with a pixel size of 300–400 nm. An increase or decrease of double bonds in the fatty acid chains is detected by the ratio of
C–H at 3012 cm−1 and CH3 stretching of lipids at 2855 cm−1 (Fig. 5). For better comparison, the ratio was normalized to the CH3 str. band intensity (2855 cm−1). Subsequently, cluster analysis was used to determine regions within single yeast cells containing different lipids. In addition, the mean value and single standard deviation of all pixels representing one yeast cell allow a first assessment of the general trend of the lipid composition produced and stored in the yeast cell. Within the first few hours of fermentation, the overall amount of double bonds in the produced lipids first increases before a significant decrease is observed. Additionally, the distribution of fatty acids within single cells seems to be arranged in a way that the highest amount of C
C double bonds is observed at the edge of the yeast cells (cell wall), while the ratio of unsaturated vs. saturated fatty acids is lower in the cytoplasm region (Fig. 5). Interestingly, unsaturated fatty acids are essential components of the yeast cell's plasma membrane30 which is also confirmed by our findings. Additionally, the Raman image of the yeast cell after 2.5 h of fermentation indicates ongoing cell fission with two yeast cells being depicted, each surrounded by an area expressing higher C
C content compared to the inner part of the cells (cytoplasm). This example demonstrates that Raman imaging allows valuable insights into cell metabolism. However, it is not applicable for in-line monitoring as the cells need to be fixed locally while the Raman image is collected (here: 10–15 min per cell).
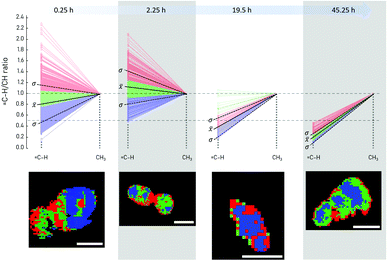 |
| Fig. 5 Ratio of C–H (3012 cm−1) and CH3 stretching vibration of lipids (around 2855 cm−1) within single yeast cells after 0.25, 2.25, 19.5, and 49.25 h of fermentation time. Regions of different C–H/CH3 ratios are highlighted in different colors based on the three main clusters determined by cluster analysis. The first row depicts the ratio values at each pixel including mean and single standard deviation. The second row shows the lateral distribution thereof within single yeast cells following the color code used in the first row. Scale bar is 5 μm. | |
3.4 In-line lipidomics
As demonstrated in the previous paragraphs, PLS regression can be used for direct monitoring of lipid accumulation, glucose consumption, and biomass production. Another important parameter that requires monitoring during the fermentation process is the production of unsaturated fatty acids (UFAs) and saturated fatty acids (SFAs). Following from off-line Raman imaging analysis, either the spectral fingerprint region (C
C str. lipids at 1650 cm−1 vs. CH2 def. lipids at 1440 cm−1) or the CH stretch region (
CH str. lipids at 3012 cm−1 vs. CH3 str. lipids at 2855 cm−1) can be employed to assess the degree of saturation of the produced lipids. Using in-line data, typically a lower SNR compared to off-line measurements is achieved. Moreover, spectral interferences of medium components and water need to be considered. These aspects can be circumvented in off-line analyses by introducing a washing step (to remove medium components) and adequate sample preparation (e.g. a thin flow-cell that allows setting the focus of the Raman laser directly onto the yeast cell, thereby minimizing the interference of water or measuring dried cells). Here, the
CH stretch region around 3000 cm−1 was very much affected by the intense and broad OH stretch vibration of water (around 3400 cm−1). Therefore, the fingerprint region was selected for further data analysis steps. Nevertheless, the OH def. vibration of water around 1640 cm−1 has a strong impact on the C
C str. vibration around 1656 cm−1 which only appears as a small shoulder in the in-line Raman spectra (Fig. 6B). Hence, calculating the C
C/CH2 ratio using the intensities at the respective wavenumbers is hampered in a way as it results in a trend that is superimposed by water. Consequently, a Gaussian curve fitting approach was employed in the spectral region between 1230 cm−1 and 1790 cm−1 to achieve a better separation of these two bands. The list of Raman bands located in the selected spectral region and used for curve fitting are listed in the table included in Fig. 6. As can be seen, all components of the yeast fermentation process (medium, C-source, yeast, lipids) are represented. The fitting result of one spectrum is shown exemplarily in Fig. 6A indicating good agreement between the original spectrum and the calculated fit and highlighted by the residuals plotted as gray line. The temporal evolution of the most important bands (1364 cm−1 glucose, 1440 cm−1 CH2 def., 1656 cm−1 C
C str.) is depicted in Fig. 6B showing good agreement with the trends observed by glucose off-line analysis (Fig. 2c). Both, the C
C as well as the CH2 bands increase within the batch phase (first 24 hours). During the subsequent fed-batch phase with two different glucose feed rates, the C
C content levels off and remains constant until the end of the fermentation. On the contrary, the temporal evolution of the CH2 deformation vibration shows a constant increase until a plateau level is reached after 72 hours. At the same time, the glucose feed rate is reduced. The ratio of C
C vs. CH2 increases during the batch phase, and subsequently keeps a slight upward trend until a similarly slight decrease is observed. Hence, the in-line data reflects the off-line, but to a much lesser extent. The decrease in the amount of unsaturated fatty acids is more dramatic in the single-cell off-line analysis than can be observed for the in-line batch analysis. This may have several reasons: first, there was no interfering water band in the off-line spectra, hence the calculation of the ratio is straightforward. Admittedly, there still is the amide I band around 1660 cm−1 but compared to the intensity of the C
C band at 1656 cm−1, the first one is negligible. Second, the SNR in the off-line data is better compared to the in-line data. The off-line imaging approach focuses on one single cell at a time using a collection optic with a numerical aperture of 0.55 which is about twice the value of the in-line probe. This means, that more backscattered photons are collected by the collection optics during off-line analysis, resulting in a more intense Raman signal. Third, the imaging approach only focuses on single cells which is statistically hard to hold up against a more general batch analysis as performed with the in-line probe. Therefore, the in-line data might be more representative than the off-line Raman images. In addition, the C
C/CH2 trend extracted by curve fitting is in good agreement with off-line GC data (Fig. 6C) which, once again, highlights the potential of in-line Raman spectroscopy.
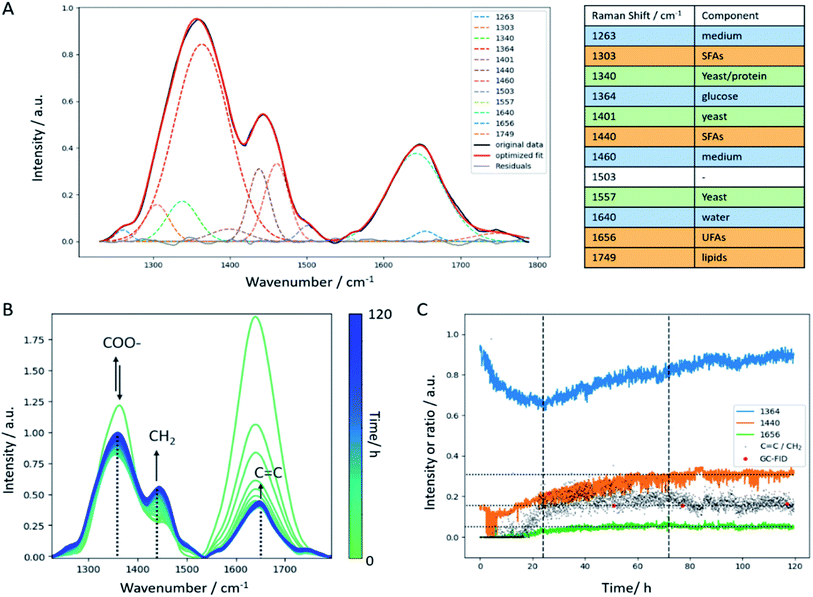 |
| Fig. 6 (A) Curve fit result exemplarily shown for the spectrum recorded after 85 h of fermentation. Original data (black line) and the sum of 12 Gaussian fits (red line) show good agreement. Residuals are depicted by the gray line. The list of bands used for the fit including band assignment to the respective component in the fermentation broth is shown on the right. (B) Temporal progression of the in-line Raman spectra with the selected marker bands for glucose (COO−), SFA (CH2), and UFA (C C). Every 50th Raman spectrum is shown. (C) Temporal progression as a result of the Gaussian curve fitting (amplitude values) shown for the selected bands marked in (B). The black dots depict C C/CH2 ratio which is in good agreement with GC reference measurements (red). | |
4. Conclusions
This work presents a comprehensive study on the production of lipids by the oleaginous yeast C. oleaginosus. In-line Raman spectroscopy as an in situ tool combined with different mathematical strategies allows monitoring of biomass growth, feedstock consumption, lipid accumulation, as well as the dynamic profile of the lipid composition on time. The extracted parameters allow an instantaneous understanding of the culture vitality, diauxic shifting, and nutrition shortage/excess. Having access to this knowledge almost in real-time is key to optimal process control which leads to optimized product yields and quality, and ultimately to a more sustainable crude palm oil life-cycle.
Author contributions
Conceptualization – KW, MM, TB, CH; data curation – KW, MM, JP; formal analysis – KW, MM; funding acquisition – TB, CH; investigation – KW, MM, JP; methodology – KW, MM, TB, CH; project administration – TB, CH; supervision – TB, CH; visualization – KW, MM; writing – original draft, KW, MM; writing – review & editing – JP, TB, CH.
Conflicts of interest
There are no conflicts to declare.
Acknowledgements
This work was financially supported by Schott AG (Landshut Germany). The authors thank Christian Ott (Schott AG) for triggering this work and providing the SCHOTT ViewPort™ that was used for in-line Raman monitoring. Christian Lux (tec5 AG) and Sebastian Dedera (tec5 AG) are gratefully acknowledged for support with the in-line Raman set-up during the yeast fermentation. KW also acknowledges financial support through the COMET Centre CHASE, funded within the COMET-Competence Centers for Excellent Technologies programme by the BMK, the BMDW and the Federal Provinces of Upper Austria and Vienna. The COMET programme is managed by the Austrian Research Promotion Agency (FFG).
Notes and references
- E. B. Fitzherbert, M. J. Struebig, A. Morel, F. Danielsen, C. A. Brühl, P. F. Donald and B. Phalan, Trends Ecol. Evol., 2008, 23, 538–545 CrossRef.
- B. Wicke, R. Sikkema, V. Dornburg and A. Faaij, Land Use Pol., 2011, 28, 193–206 CrossRef.
- H. V. Cooper, S. Evers, P. Aplin, N. Crout, M. P. B. Dahalan and S. Sjogersten, Nat. Commun., 2020, 11, 1–8 Search PubMed.
- R. A. Butler, Emissions per metric ton of crude palm oil, https://rainforests.mongabay.com/deforestation/charts/commodities/palm-oil-emissions_cpo.html Search PubMed.
- A. T. McCurdy, A. J. Higham, M. R. Morgan, J. C. Quinn and L. C. Seefeldt, Fuel, 2014, 137, 269–276 CrossRef CAS.
- S. Pinzi, D. Leiva, I. López-García, M. D. Redel-Macías and M. P. Dorado, Biofuels, Bioprod. Biorefin., 2014, 8, 126–143 CrossRef CAS.
- D. J. Kyle and C. Ratledge, Industrial applications of single cell oils, AOCS Publishing, 1992 Search PubMed.
- M. A. Masri, D. Garbe, N. Mehlmer and T. B. Brück, Energy Environ. Sci., 2019 10.1039/c9ee00210c.
- P. J. Slininger, B. S. Dien, C. P. Kurtzman, B. R. Moser, E. L. Bakota, S. R. Thompson, P. J. O'Bryan, M. A. Cotta, V. Balan and M. Jin, Biotechnol. Bioeng., 2016, 113, 1676–1690 CrossRef CAS PubMed.
- G. M. Rodriguez, M. S. Hussain, L. Gambill, D. Gao, A. Yaguchi and M. Blenner, Biotechnol. Biofuels, 2016, 9, 149 CrossRef.
- A. Yaguchi, A. Robinson, E. Mihealsick and M. Blenner, Microb. Cell Fact., 2017, 16, 206 CrossRef PubMed.
- M. Spagnuolo, M. S. Hussain, L. Gambill and M. Blenner, Front. Microbiol., 2018, 9 DOI:10.3389/fmicb.2018.01077.
- D. Awad, S. Younes, M. Glemser, F. M. Wagner, G. Schenk, N. Mehlmer and T. Brueck, Sustainable Energy Fuels, 2020, 4, 5958–5969 RSC.
- M. A. Masri, W. Jurkowski, P. Shaigani, M. Haack, N. Mehlmer and T. Brück, Appl. Energy, 2018, 224, 1–12 CrossRef CAS.
- K. A. Esmonde-White, M. Cuellar, C. Uerpmann, B. Lenain and I. R. Lewis, Anal. Bioanal. Chem., 2017, 409, 637–649 CrossRef CAS.
- H. L. T. Lee, P. Boccazzi, N. Gorret, R. J. Ram and A. J. Sinskey, Vib. Spectrosc., 2004, 35, 131–137 CrossRef CAS.
- G. D. Metcalfe, T. W. Smith and M. Hippler, Anal. Bioanal. Chem., 2020, 412, 7307–7319 CrossRef CAS.
- A. Golabgir and C. Herwig, Chem. Ing. Tech., 2016, 88, 764–776 CrossRef CAS.
- J. A. Iversen, R. W. Berg and B. K. Ahring, Anal. Bioanal. Chem., 2014, 406, 4911–4919 CrossRef CAS PubMed.
- A. Nemcova, D. Gonova, O. Samek, M. Sipiczki, E. Breierova and I. Marova, Microorganisms, 2021, 9 DOI:10.3390/microorganisms9020277.
- R. Schalk, F. Braun, R. Frank, M. Radle, N. Gretz, F. J. Methner and T. Beuermann, Bioprocess Biosyst. Eng., 2017, 40, 1519–1527 CrossRef CAS.
- E. Hirsch, H. Pataki, J. Domjan, A. Farkas, P. Vass, C. Feher, Z. Barta, Z. K. Nagy, G. J. Marosi and I. Csontos, Biotechnol. Prog., 2019, 35, e2848 CrossRef CAS PubMed.
- B. Berry, J. Moretto, T. Matthews, J. Smelko and K. Wiltberger, Biotechnol. Prog., 2015, 31, 566–577 CrossRef CAS.
- K. Kochan, H. Peng, B. R. Wood and V. S. Haritos, Biotechnol. Biofuels, 2018, 11, 106 CrossRef PubMed.
- M. Uematsu, Y. Kita, T. Shimizu and H. Shindou, FASEB J., 2020, 34, 10357–10372 CrossRef CAS PubMed.
- H. Wu, J. V. Volponi, A. E. Oliver, A. N. Parikh, B. A. Simmons and S. Singh, Proc. Natl. Acad. Sci. U. S. A., 2011, 108, 3809–3814 CrossRef CAS PubMed.
- C. E. E. Grace, P. K. Lakshmi, S. Meenakshi, S. Vaidyanathan, S. Srisudha and M. B. Mary, Spectrochim. Acta, Part A, 2020, 224, 117382 CrossRef CAS PubMed.
- M. Mahmoud, Y. Samer, H. Martina, Q. Farah, M. Norbert and B. Thomas, Energy Technol., 2018, 6, 1026–1038 CrossRef.
- D. V. L. Mohamed Mathlouthi, Carbohydr. Res., 1980, 81 DOI:10.1016/S0008-6215(00)85652-9.
- J. E. Stukey, V. M. McDonough and C. E. Martin, J. Biol. Chem., 1990, 265, 20144–20149 CrossRef CAS.
Footnote |
† Electronic supplementary information (ESI) available: Representative Raman spectra of single yeast cell imaging. See DOI: 10.1039/d1ra04254h |
|
This journal is © The Royal Society of Chemistry 2021 |