DOI:
10.1039/D0RA09585K
(Paper)
RSC Adv., 2021,
11, 1450-1460
Experimentally designed chromatographic method for the simultaneous analysis of dimenhydrinate, cinnarizine and their toxic impurities†
Received
11th November 2020
, Accepted 17th December 2020
First published on 5th January 2021
Abstract
Recently, experimental design has beaten the traditional optimization approach (one variable at a time) by providing better quality for chromatographic separation using minimal effort and resources. Benzophenone (BZP) and [1-(diphenylmethyl)piperazine] (DPP) were reported to be the most toxic impurities for dimenhydrinate (DMH) and cinnarizine (CIN), respectively. Additionally, there is no reported HPLC method for the simultaneous determination of DMH, CIN and their toxic impurities. A custom experimental design was adopted to estimate the optimum conditions that achieved the most acceptable resolution with adequate peak symmetry within the shortest run time. Desirability function was used to define the optimum chromatographic conditions and the optimum separation was achieved using XBridge® HPLC RP-C18 (4.6 × 250 mm, 5 μm), acetonitrile: 0.1% sodium lauryl sulphate (SLS) in water (90
:
10, v/v) as a mobile phase at flow rate 2 mL min−1 and UV detection at 215 nm. Method validation was carried out according to ICH guidelines and linearity was achieved in the ranges of 2–25, 1–25, 1–12.5, and 1–12.5 μg mL−1 for DMH, CIN, BZP and DPP, respectively. By application of the proposed method to the market dosage form, no interference from excipients was observed. Moreover, the greenness of the method was evaluated using the National Environmental Method Index (NEMI), Analytical Eco-Scale and Green Analytical Procedure Index (GAPI) metrics and the results revealed the green environmental impact of the developed method.
1. Introduction
Dimenhydrinate (DMH), is chemically defined as 1-((2-(benzhydryloxy)ethyl)(methyl)amino)-8-chloro-3-methyl-3,7-dihydro-1H-purine-2,6-dione.1 It consists of a 1
:
1 mixture salt of diphenhydramine (DIP) and 8-chlorotheophylline (CHP) as reported by the United States Pharmacopeia (USP),2 British Pharmacopeia (BP)3 and European Pharmacopeia (EP).4 Cinnarizine (CIN), is a piperazine derivative that is chemically named 1-(diphenylmethyl)-4-(3-phenyl-2-propenyl)piperazine.1 It has an antihistaminic and a calcium channel blocking activity. Dimenhydrinate and cinnarizine are either prescribed separately or in combination for the treatment of nausea and vomiting, motion sickness and vertigo from vestibular or non-vestibular sources.5–7
Dimenhydrinate has several official impurities.3 In the last published work of our team, we reported that the precursor of DMH, benzophenone (BZP) is the most toxic DMH impurity.8 p-Hydroxybenzophenone is the carcinogenic, estrogenic and hepatocellular necrotic metabolite of BZP.9–13 The in silico toxicity studies of CIN official impurities revealed that 1-(diphenylmethyl)piperazine (DPP) had higher toxicity than the other CIN impurities.8
The USP,2 BP3 and EP,4 and reports potentiometric, argentometric, and HPLC methods for the assay of DMH. Additionally, the BP3 and EP4 developed a potentiometric titration for the assay of CIN. On the other hand, the DMH and CIN combination is not officially included in any pharmacopeia. The literature review revealed a spectrophotometric,14 electrochemical15 and chromatographic16–18 methods for determination of DMH and its impurities. CIN was assayed spectrophotometrically,19,20 electrochemically21,22 and by chromatographic methods23–28 either alone or in combination with other drugs and/or impurities. Moreover, CIN and DMH were simultaneously determined by TLC-densitometry,29,30 HPLC30,31 and UV-spectrophotometry.32,33 The binary mixture of DMH and CIN was analyzes in presence of DPP a spectrophotometric,34 TLC-densitometric35 and an HPLC35 methods. A single TLC-densitometric method was developed in our laboratory for the simultaneous assay of DMH, CIN and their toxic impurities; BZP and DPP.8
HPLC separation includes a wide range of variables that control the separation process; mobile phase pH, buffer concentration, flow rate, column temperature, detecting wavelength, etc. For several decades, HPLC method development strategies were based on trial and error supported by the experience and knowledge of the analyst. This conventional one factor at a time (OFAT) strategy consumes time, effort and resources to produce incompletely justified outcomes.36 On the contrary, the design of experiment approach (DOE) uses the minimum number of experiments to evaluate the effect of the variables and their interactions on the measured responses to achieve the desired output.37 Custom design is a flexible experimental design tailored to meet the available budget and desired output. It allows analysts to define factor constraints and assess the main effects and interaction through the minimum number of experiments.38,39
On the other way, environmental awareness has grown over the last few decades to introduce the greenness concepts to analytical chemistry. Analysts aim to reduce or avoid the consumption and emission of hazardous substances throughout the analytical procedure.40,41 Different green chemistry metrics have been developed for the evaluation of greenness standards in such procedures. The National Environmental Method Index (NEMI) is among the oldest techniques built to provide a clear representation of the greenness of the analytical method.42 Another green chemistry metric is the Analytical Eco-Scale,43 which is a semi-quantitative tool involving additional environmental impact investigating parameters.44 The Green Analytical Procedure Index (GAPI) is a recent approach that utilizes additional safety and health criteria to provide a more comprehensive evaluation for each step during the analytical procedures.45
The literature failed to reveal any HPLC method for simultaneous determination of DMH, CIN and their toxic impurities; BZP and DPP. The current work aims to implement a custom experimental design to evaluate the critical main effects and interactions controlling the separation process. The developed method was optimized to quantify the toxic impurities along with their active drugs with high sensitivity within short analysis time. It was validated according to the ICH validation guidelines.46 Analyst's considered the greenness concepts throughout the method development process to minimize the environmental burdens. The ability of the NEMI, Analytical Eco-Scale and GAPI greenness assessment metrics were compared to evaluate the overall method greenness. The method was validated as per ICH guidelines.
2. Experimental
2.1. Materials and reagents
Dimenhydrinate sample was kindly supplied by Kahira Pharmaceuticals and Chemical Industries Company (Shoubra, Cairo, Egypt) with a purity of 99.10% according to the reported method.35 Cinnarizine pure sample was obtained as a gift from Amoun Pharmaceuticals (El Obour City, Cairo, Egypt) with a purity of 100.26% according to the reported method.35 Benzophenone and DPP were bought from Sigma Aldrich, Chemie GmbH (Darmstadt, Germany) with a purity of 97% and 99%, respectively according to the manufacturing certificates of analysis. Methanol and acetonitrile were of HPLC grade (Fisher, Loughborough, UK) and were purchased from Sigma Aldrich Chemie GmbH (Darmstadt, Germany). Orthophosphoric acid, sodium lauryl sulphate (SLS) and potassium dihydrogen phosphate were purchased from EL-Nasr Pharmaceutical, Chemicals Co., Abu Zabaal, Cairo, Egypt and were of analytical grade. Amocerebral® tablets (batch no. 6221025030658) were manufactured by Amoun Pharmaceutical Company (Cairo, Egypt), each tablet was labeled to contain 20 mg of DMH and 10 mg of CIN.
2.2. Instruments
Thermo Scientific Dionex Ultimate S 3000 HPLC, equipped with Ultimate quaternary solvent delivery pump, ACC-3000 autosampler and DAD-3000 diode array detector (Germany) was used. The stationary phases tested were Hypersil Gold® C18 (2.1 × 50 mm, 1.9 μm), Hypersil Gold® C18 (4.6 × 150 mm, 3 μm), XBridge® HPLC RP-C18 (4.6 × 250 mm, 5 μm), Xterra® CN (4.6 × 150 mm, 3 μm) and Xterra® RP-C8 (2.1 × 50 mm, 1.9 μm). The output signal was monitored and processed using Chromeleon chromatography data system software.
Digital balance (Sartorius, Germany) and Sonix TVSS-series ultrasonicator (CA, USA) were used.
2.3. Software programs
Custom experimental design and data analysis calculations were performed using the JMP® software version 15.2.0 Copyright© 2019, SAS Institute Inc., Cary, North Carolina, USA software.47
2.4. Solutions
(a) Stock standard solutions of DMH, BZP, CIN, and DPP were prepared by accurate weighing of 0.1 g of each and dissolving in acetonitrile to obtain a 100 mL stock solution of 1 mg mL−1 each.
(b) Working standard solutions (100 μg mL−1) of the studied components were prepared by transferring 1 mL of each component from its respective stock standard solution (1 mg mL−1) into four separate 10 mL volumetric flasks, then the volume was completed to the mark with acetonitrile.
2.5. Experimental design
The JMP® software version 15.2.0 (466311) Copyright© 2019, SAS Institute Inc., Cary, North Carolina, USA software47 was used to build two levels-three factors custom experimental with one center point. An initial screening study was carried out to select the critical factors affecting the chromatographic separation. The flow rate, SLS concentration and acetonitrile percentage in the mobile phase were selected as the independent variables. The run time, tailing factors of CIN and DPP, and resolution of BZP, CIN, and DIP were recorded for each run and used as the dependent variables (responses) (Table 1). Sixteen experiments were conducted in random order. The second-order polynomial equations characterizing the quadratic models and indicated the interaction between the factors were computed using the standard least square method. The results were statistically analyzed and the final optimization was achieved using the desirability function. The run time and tailing factors were set to minimize while the resolution was set to maximize.
Table 1 Levels, factors and responses in the custom experimental design
Factors |
Responses |
Experiment no. |
Flow rate |
SLS conc. |
% acetonitrile |
Run time |
TFCIN |
TFDPP |
RSBZP |
RSCIN |
RSDIP |
1 |
1.5 |
0.05 |
95 |
25 |
1.1 |
1.5 |
6.61 |
6.29 |
6.1 |
2 |
1.5 |
0.1 |
85 |
13.5 |
1.25 |
1.2 |
4.29 |
5.09 |
2.37 |
3 |
2 |
0.05 |
90 |
13.38 |
0.98 |
1.38 |
3.68 |
4.64 |
3.95 |
4 |
1.5 |
0.05 |
85 |
14.5 |
1.29 |
1.3 |
4.12 |
4.98 |
2.17 |
5 |
2 |
0.1 |
95 |
25 |
0.99 |
1.24 |
7.23 |
7.3 |
4.94 |
6 |
2 |
0.05 |
85 |
14 |
1.22 |
1.25 |
3.75 |
4.39 |
2.07 |
7 |
1.75 |
0.075 |
90 |
13.06 |
0.98 |
1.42 |
3.92 |
4.16 |
3.66 |
8 |
1.75 |
0.05 |
95 |
25 |
0.98 |
1.31 |
6.7 |
6.59 |
6.21 |
9 |
1.5 |
0.075 |
90 |
14.3 |
1.14 |
1.3 |
4.21 |
4.5 |
3.99 |
10 |
1.5 |
0.05 |
85 |
14.5 |
1.34 |
1.3 |
4.12 |
4.98 |
2.17 |
11 |
2 |
0.1 |
85 |
12.5 |
1.17 |
1.19 |
2.85 |
4.7 |
2.55 |
12 |
1.75 |
0.1 |
90 |
14.52 |
1.02 |
1.3 |
3.95 |
4.74 |
2.19 |
13 |
2 |
0.075 |
95 |
25 |
0.99 |
1.4 |
7.21 |
7.29 |
4.9 |
14 |
1.75 |
0.075 |
85 |
13.45 |
1.22 |
1.25 |
3.85 |
4.64 |
2.09 |
15 |
1.5 |
0.1 |
95 |
25 |
1.1 |
1.32 |
6.8 |
6.7 |
4.6 |
16 |
2 |
0.05 |
95 |
25 |
0.99 |
1.25 |
7.12 |
7.25 |
6.8 |
2.6. Chromatographic conditions
A Thermo Scientific Dionex Ultimate S 3000 HPLC (Germany) instrument and XBridge® HPLC RP-C18 (4.6 × 250 mm, 5 μm) columns were used. The injection volume was 30 μL and the separation was carried out using isocratic elution with a mobile phase mixture of acetonitrile: 0.1% SLS (90
:
10, v/v) flowed at a rate of 2 mL min−1. The overall run time was 15 min and the UV detection was carried out at 215 nm while the temperature was adjusted to 25 °C.
2.7. Construction of calibration curves
Sample solutions with different concentrations 2–25, 1–12.5, 1–25, and 1–12.5 μg mL−1 for CHP, BZP, CIN, and DPP, respectively were prepared by dilution of their respective working solutions with the mobile phase in 5 mL volumetric flasks. A volume of 30 μL of each solution was injected in triplicates and the above chromatographic instructions were then performed.
Calibration plots relating the area under the peak against the corresponding analyte concentration were plotted and the regression equations were computed.
2.8. Application to the pharmaceutical formulation
Ten Amocerebral® tablets were ground to a uniform mixture, and an amount equivalent to 50 mg DMH and 25 mg CIN was accurately weighed and transferred to a 25 mL volumetric flask, ultrasonicated with 10 mL acetonitrile for 30 min, cooled to room temperature and the volume was completed with acetonitrile and finally filtered using a 0.22 μm nylon membrane filter.
The previously prepared solution was diluted using the mobile phase to reach the concentration of 2 and 1 mg mL−1 for DMH and CIN, respectively, and a volume of 30 μL was injected in triplicates and chromatographed under the optimum chromatographic conditions.
3. Results and discussion
Optimization of an HPLC method is a complex process since several variables must be adjusted simultaneously to achieve the desired separation. Multiple advantages can be acquired by using an experimental design during the optimization process, among these advantages are enhancing the quality of separation, reducing the number of experiments, and minimizing the demand of laboratory resources and trained labor.36,48
The custom design is of choice when the targeted design has specific parameters like time, budget and resources. Its flexibility allows the building of smart validated designs with minimum effort, time and resources.38
3.1. Preliminary separation and screening of the critical chromatographic variables
Different stationary phases with different packing materials, lengths, and particle sizes were tested, including Hypersil Gold® C18 (2.1 × 50 mm, 1.9 μm), C18 (4.6 × 150 mm, 3 μm), XBridge® HPLC RP-C18 (4.6 × 250 mm, 5 μm), C8 (2.1 × 50 mm, 1.9 μm) and CN (4.6 × 150 mm, 3 μm) columns. Preliminary results showed that the XBridge® HPLC RP-C18 (4.6 × 250 mm, 5 μm) column produced acceptable retention and separation for the studied compounds.
Different mobile phase mixtures of different compositions were tested as trails to achieve the most efficient separation and the least peak tailing within suitable analysis time. Mobile phases containing different composition of water, 0.05 M potassium dihydrogen phosphate buffer, SLS solution, acetonitrile or methanol were tried. All mobile phases containing methanol resulted in asymmetric peaks as well as strong retention of CIN on the stationary phase while acetonitrile containing mobile phases improved the peak shape and resulted in a better resolution except for DPP which was best eluted using of SLS solution and acetonitrile mobile phase mixture.
The four analytes demonstrated UV absorption at the wavelength range of 200–400 nm. However, the limited conjugation in the chromophore of DMH and DPP forced the UV detection at shorter wavelengths. The four analytes demonstrated adequate sensitivity at 215 nm (Fig. 1S†).
3.2. Experimental design
The preliminary separation study results showed that the tailing factor for CHP and BZP as well as resolution between DPP and DIP were not critical. Besides, DMH was determined as CHP due to its higher response in comparison to DIP, hence, DIP peak shape was not targeted during the optimization process. The optimization study assigned the mobile phase flow rate, SLS concentration, and acetonitrile% as the critical factors affecting the separation; levels were chosen in the range (2.0 and 1.5 mL min−1), (0.1 and 0.05%), and (95 and 85%), respectively. The run time, tailing factors of CIN and DPP, and resolution of BZP, CIN and DIP were measured to evaluate the quality of the separation process.
The JMP® software47 version 15.2.0 was used to build a custom design of sixteen experiments. The design included four experiments at the center point (zero levels of the three factors) to evaluate the curvature. The experiments were performed, and the assigned responses were measured to feed the model (Table 1). The prediction models were constructed for each critical response. The models compute a coefficient for each main effect, interaction, and quadratic term. The latter defined the non-linear (polynomial) relationships, whereas, the coefficients defined the direction and strength of each effect on the respective response. ANOVA statistical analyses were performed, and P-values were calculated to indicate the significance of each term at significance level α = 0.05. Significant terms are highlighted in Table 2.
Table 2 The calculated coefficients of the prediction models and P-values obtained from the ANOVA test resultsa
Term |
Run time |
TFCIN |
TFDPP |
RsBZP |
RsCIN |
RsDIP |
Coef. |
P-Value |
Coef. |
P-Value |
Coef. |
P-Value |
Coef. |
P-Value |
Coef. |
P-Value |
Coef. |
P-Value |
Highlighted values (bold) indicate significant effect on the corresponding response. Coef. = coefficient (slope), I = intercept, A = flow rate, B = SLS conc., C = % acetonitrile, TF = tailing factor and Rs = resolution. |
I |
13.5939 |
<0.0001 |
1.0153 |
<0.0001 |
1.2510 |
<0.0001 |
3.9337 |
<0.0001 |
4.3554 |
<0.0001 |
3.2752 |
0.0002 |
A |
−0.2147 |
0.2119 |
−0.0554 |
0.0023 |
−0.0412 |
0.0006 |
−0.1322 |
0.0148 |
0.0871 |
0.0751 |
0.0239 |
0.8849 |
B |
−0.1679 |
0.3099 |
−0.0076 |
0.4643 |
−0.0561 |
0.0001 |
−0.0083 |
0.8261 |
0.1267 |
0.0216 |
−0.4136 |
0.0451 |
C |
5.7151 |
<0.0001 |
−0.0993 |
0.0002 |
−0.0426 |
0.0005 |
1.5824 |
<0.0001 |
1.0442 |
<0.0001 |
1.5920 |
0.0002 |
AB |
−0.0176 |
0.9206 |
0.0039 |
0.7349 |
0.0197 |
0.0216 |
−0.1261 |
0.0262 |
−0.0250 |
0.5883 |
−0.0125 |
0.9459 |
AC |
0.2296 |
0.2247 |
−0.0069 |
0.5484 |
0.0042 |
0.5145 |
0.3418 |
0.0004 |
0.3288 |
0.0006 |
0.0221 |
0.9036 |
BC |
0.3313 |
0.1048 |
0.0148 |
0.2319 |
0.0028 |
0.6619 |
0.1406 |
0.0177 |
0.0090 |
0.8435 |
−0.5300 |
0.0294 |
ABC |
0.0610 |
0.7376 |
−0.0016 |
0.8946 |
0.0074 |
0.2846 |
0.1240 |
0.0307 |
−0.0699 |
0.1776 |
−0.0724 |
0.7049 |
A2 |
−0.2483 |
0.4826 |
0.0499 |
0.0654 |
0.0294 |
0.0537 |
−0.0154 |
0.8537 |
0.1417 |
0.1556 |
0.3461 |
0.3597 |
B2 |
0.4116 |
0.2582 |
−0.0150 |
0.5061 |
0.0207 |
0.1326 |
−0.0972 |
0.2674 |
0.1178 |
0.2176 |
−0.0808 |
0.8207 |
C2 |
5.5814 |
<0.0001 |
0.0917 |
0.0077 |
−0.0113 |
0.3797 |
1.5101 |
<0.0001 |
1.2410 |
<0.0001 |
0.3404 |
0.3684 |
It was observed that the SLS concentration and acetonitrile percentage significantly affected the resolution of DIP peak. Besides, the run-time prediction model indicated a significant, non-linear, and directly proportional effect of acetonitrile percentage and its quadratic term on the run time (Table 2) (Fig. 1 and 2S†). On the other hand, the tailing factor of CIN was significantly affected by the flow rate, acetonitrile percent and its quadratic term. It decreased at high flow rates and higher acetonitrile percentages. The three factors had a significant inversely proportional effect on the tailing factor of DPP. Lower tailing factors could be achieved at high levels of each factor. However, the though lower yet significant directly proportional effect of the interaction between the flow rate and SLS concentration necessitates a prudent selection of the working levels from each factor (Table 2) (Fig. 1 and 2S†). The resolution of BZP and CIN was affected by certain main effects, interactions, and the quadratic term of acetonitrile percentage. The relatively high coefficient for acetonitrile and its quadratic term indicated its pronounced effect on the resolution of the two compounds (Table 2) and (Fig. 1 and 2S†).
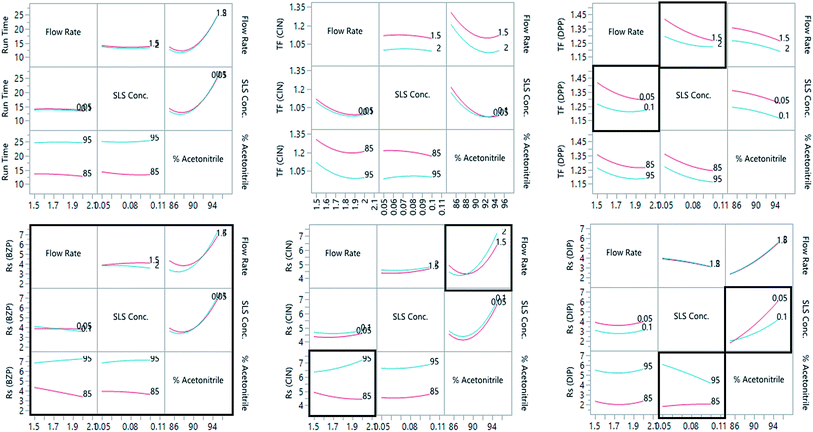 |
| Fig. 1 Interaction plots of the studied factors and their effects on the measured response. | |
The constructed model we used to predict the responses. The latter were plotted against the experimentally found responses. The calculated R2 for the six responses approached unity indicating the perfect fit of the predicted and experimental responses. Additionally, the small gap between R2 and R2 adjusted confirmed good fitting of the model. The root mean square error of predictions (RMSEP) was negligible revealing the accuracy of the prediction model respectively. ANOVA tests were carried out for each of the six prediction models. The calculated low P-values (<0.05) proved the significance of the six models for the prediction of their respective responses (Table 3). On the other hand, the calculated residuals presented in Fig. 3S† appeared to be approximately normally distributed (with a mean of zero), and be independent of one another over time. Consequently, residual analysis confirmed that the models appropriately represent the data.
Table 3 Fitting results of the predicted against the found responses of the developed model
Term |
Run time |
TFCIN |
TFDPP |
RsBZP |
RsCIN |
RsDIP |
P-Value define the model significance for prediction of the corresponding response. |
R2 |
0.9973 |
0.9775 |
0.9841 |
0.9980 |
0.9958 |
0.9658 |
R-Adjusted |
0.9920 |
0.9324 |
0.9609 |
0.9941 |
0.9874 |
0.8975 |
Predicted root mean square error |
0.5034 |
0.0326 |
0.0180 |
0.1215 |
0.1303 |
0.5277 |
P-Valuea |
<0.0001 |
0.0017 |
0.007 |
<0.0001 |
<0.0001 |
0.0046 |
In the presence of multiple responses, the desirability function approach provides a simple, easy, and accurate tool for their optimization. Optimum settings for separation were determined using the response optimizer tool (Fig. 2). Also, the “maximize desirability” option was selected for achieving maximum desirability. Acetonitrile: 0.1% SLS in water (90
:
10, v/v) flowed at 2 mL min−1 was the optimum chromatographic conditions since appropriate resolution, symmetric peaks and suitable run time were obtained, Fig. 3.
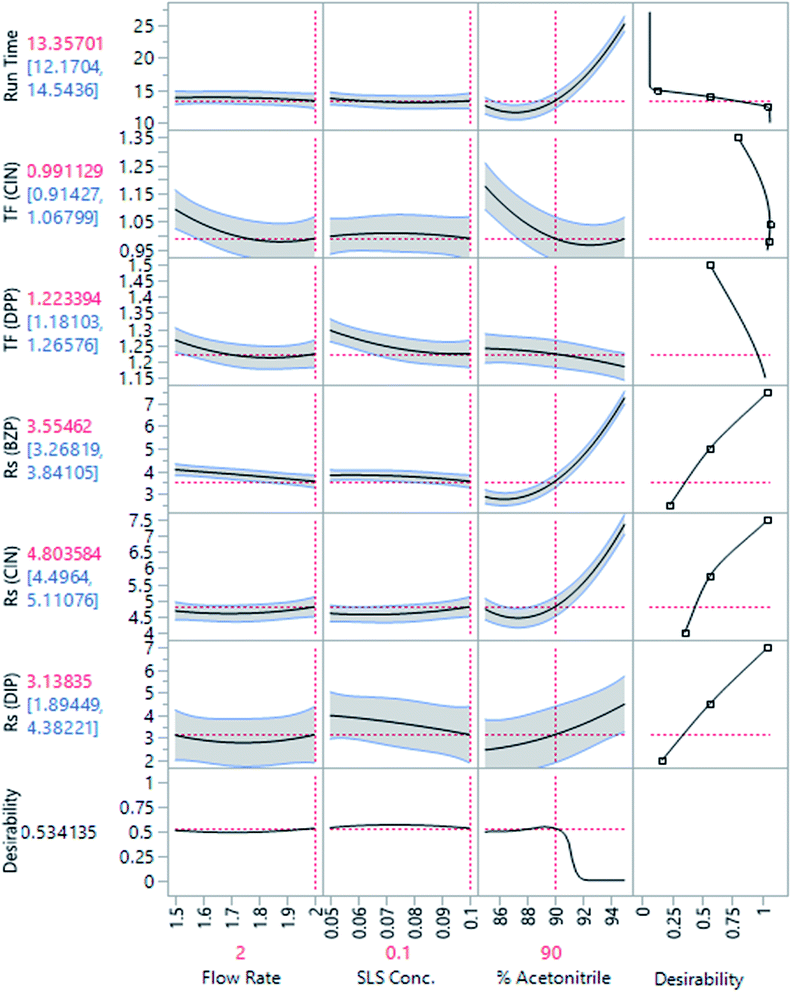 |
| Fig. 2 Prediction profiler of the experimental design. | |
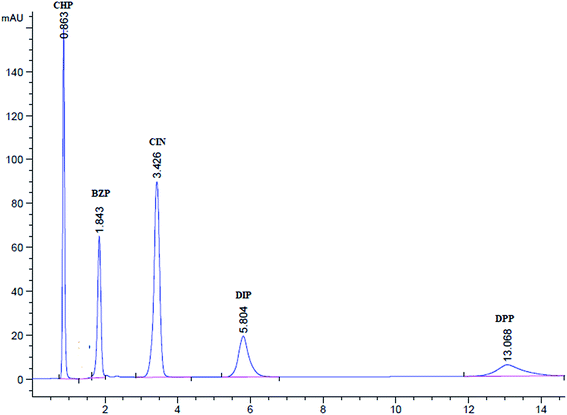 |
| Fig. 3 HPLC chromatogram of the studied compounds using XBridge® HPLC RP-C18 (4.6 × 250 mm, 5 μm), and mobile phase of acetonitrile: 0.1% sodium lauryl sulphate in water (90 : 10, v/v) at flow rate 2 mL min−1 and UV detected at 215 nm. *Both 8-chlorotheophylline and DIP represent DMH. | |
3.3. Methods validation
Method validation was carried out according to guidelines for method validation from the International Conference on Harmonization (2005).46
3.3.1. Linearity of the calibration curves. Linearity was evaluated by analyzing seven concentrations of each drug and six concentrations for each impurity in triplicates. Calibration curves were linear over the concentration ranges of 2–25, 1–12.5, 1–25 and 1–12.5 μg mL−1 for CHP, BZP, CIN and DPP, respectively with correlation coefficients (r) of 0.9999, 0.9999, 1.0000 and 0.9992, respectively. The calculated regression parameters including the slopes and intercepts are presented in Table 4.
Table 4 Regression and analytical parameters of the proposed HPLC method
Parameters |
CHP |
BZP |
CIN |
DPP |
The intra-day precision (n = 9), average SD of three different concentrations repeated three times within one day. The inter-day precision (n = 9), average SD of three different concentrations repeated three times on three successive days. Limit of detection. Limit of quantification. |
Calibration range (μg mL−1) |
2–25 |
1–12.5 |
1–25 |
1–12.5 |
Slope |
38.6891 |
52.4460 |
65.2321 |
39.9060 |
Intercept |
0.9905 |
26.4510 |
1.4030 |
−23.9060 |
Correlation coefficient |
0.9999 |
0.9999 |
1.0000 |
0.9992 |
Accuracy |
100.88 |
99.66 |
99.74 |
100.33 |
Repeatability (% RSD)a |
0.39 |
0.73 |
2.00 |
1.81 |
Intermediate precision (% RSD)b |
0.62 |
2.19 |
2.18 |
2.60 |
LODc |
0.89 |
0.13 |
0.05 |
0.15 |
LOQd |
1.24 |
0.38 |
0.15 |
0.46 |
3.3.2. Accuracy and precision. Accuracy of the developed method was assessed by analyzing different concentrations of the analytes in their linearity ranges following the instructions of the established method and calculated as percentage recovery. Good recoveries have been obtained and are given in Table 4. Additionally, the standard addition technique was applied to Amocerebral® tablets to evaluate the accuracy of the proposed method, the good results obtained confirmed that no interference was found from additives (Table 5).
Table 5 Statistical analysis of proposed HPLC and the reported method for the determination of dimenhydrinate and cinnarizine in its dosage form and results of standard addition technique
Parameter |
Proposed method |
Reported HPLC method35 |
DMH |
CIN |
DMH |
CIN |
The values between parentheses correspond to the theoretical values of t and F (α = 0.05). Standard addition was performed on three different levels and each was repeated three time. |
Mean |
100.61 |
99.70 |
100.21 |
100.26 |
SD |
1.52 |
0.87 |
2.29 |
0.88 |
t-Test (2.228)a |
0.07 |
1.12 |
— |
— |
F-Value (5.050)a |
2.27 |
1.02 |
— |
— |
Standard addition (mean ± SD)b |
99.00 ± 1.34 |
99.35 ± 1.81 |
|
|
The precision was assessed by calculating the intra and interday variations. Intraday variation (repeatability) was calculated by separately analyzing three concentration of CIN and CHP (5, 10, and 15 μg μL−1), DPP and BZP (7.5, 10, and 12.5 μg μL−1). Samples were analyzed three times on the same day following the instructions of the method and results were expressed as RSD (%). Interday variations (intermediate precision) were assessed by analyzing the same concentrations on three consecutive days. Acceptable results and low RSD were obtained (Table 4).
3.3.3. Limits of detection and quantitation. Calculation of the limits of detection (LOD) and quantitation (LOQ) has proven the sensitivity of the established system. The following equations were used to calculate them: LOD = 3.3 × SD/slope and LOQ = 10 × SD/slope. The standard deviation (SD) was measured from the lower part of the calibration curve, while the slope for each analyte was referred to be the calibration curve slope. The developed method showed high sensitivity as demonstrated by LOD and LOQ values given in (Table 4).
3.3.4. Selectivity. The selectivity of the proposed method was confirmed by the complete separation between the four analytes shown in Fig. 3. Additionally, values of resolution and selectivity factors were within the acceptable limits ensuring complete separation among the five components (Table 1S†).
3.3.5. Robustness. Robustness is defined as the ability of the method to withstand slight changes in chromatographic conditions such as the alteration of SLS concentration (±0.01%) and (±1%) percent of acetonitrile. The impact of these changes was measured as the SD on the tR value. Changes in the studied parameters were found to have no significant effect on the tR values of the separated peaks (Table 2S†).
3.3.6. System suitability testing parameters. System suitability was evaluated by calculating the tailing factor (TF), column efficiency (number of theoretical plates), resolution (Rs), selectivity (α), capacity factor (K′) and height equivalent of a theoretical plate (HETP). All measured parameters (Table 1S†) were within acceptable ranges,2 indicating the reproducibility, selectivity and resolution were adequate for the developed method.
3.3.7. Application of the method. The developed method was successfully applied to the available dosage form; Amocerebral® tablet. The results presented in Table 5 showed that the method developed was suitable for the simultaneous determination of DMH and CIN in their available dosage form without interfering from tablets excipients. Furthermore, the application of standard addition technique confirmed the accuracy of the method.
3.4. Greenness assessment
Green analytical chemistry is proposed to eliminate or minimize the amount of hazardous solvents that are globally produced and consumed every day.49,50 The NEMI tool offers a clear visualization and simple comparison of the results.42 However, the NEMI pictogram preparation is a merely qualitative method that consumes a lot of time and effort in excessive searches for every reagent and chemical in multiple lists and does not present any information about the quantity of hazards produced.
The developed HPLC and the reported TLC-densitometric8 methods were used non-PBT noncorrosive solvents and produced waste less than 50 g. However, they failed to satisfy the NEMI requirements by using of the hazardous solvents, acetonitrile and hexane, respectively (Fig. 4).
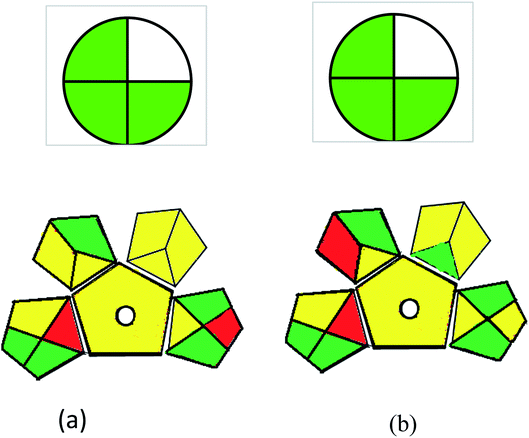 |
| Fig. 4 Assessment of the greenness profile of (a) the developed HPLC and (b) reported methods using NEMI and GAPI tools. | |
Analytical Eco-Scale provides a semi-quantitative tool that is superior to other qualitative approaches (NEMI, Efactor) for evaluating the greenness of an analytical process, taking into account the environmental impacts and energy use over the life cycle of the analytical method. Analytical Eco-Scale was used to assess and easily compare the proposed and reported8 methods greenness, the calculated Analytical Eco-Scale scores of both were >75 meaning excellent green analysis.43 (Table 3S†).
Green Analytical Procedure Index (GAPI) is considered as a well-organized and efficient alternative to NEMI as it employs additional criteria to assess each stage of the analytical procedure. In GAPI, to classify the green character of each stage, a particular pictogram utilizes ranked from green to yellow to red for the low, medium and high environmental impacts, respectively.45 Interpretation of the GAPI pentagram for the proposed and the reported method8 (Table 4S†) (Fig. 4) revealed a comparable green impact of the proposed method by using of greener solvents.
Using the three-assessment metrics: NEMI, Analytical Eco-Scale and GAPI, a clear image of the greenness profile of the proposed and reported8 methods and was given. There was a parallel consensus between the three metrics that there is an environmental impact of the proposed approach equal to or greener than the developed one.
3.5. Statistical analysis
The results obtained by the proposed HPLC method for Amocerebral® tablets were statistically compared to the reported method.35 using Student's t-test and variance ratio F-test at a 95% confidence level and the values of the calculated t and F were less than the tabulated ones (Table 5), revealing no significant difference concentration accuracy and precision between the proposed method and the reported one.
3.6. Comparison to the reported HPLC methods
The developed method showed higher selectivity due to its ability to simultaneously determine DMH, CIN, BZP and DPP. In addition to its lower detection limit and wider linearity range relative to the reported ones.30,31,35 Moreover, the developed method is greener when compared with the reported method.30 On the other hand, using of the experimental design during the optimization of the developed method saved time, effort and money.
4. Conclusion
In the present work, a worthwhile custom experimental design was adopted for the optimization of an RP-HPLC method for the determination of the combined therapy DMH and CIN simultaneously with their toxic impurities; BZP and DPP. Interpretation of the regression and ANOVA test results allowed the estimation of main, quadratic and interaction effects of the studied factors on each response. Besides, the created response surface plots were statistically validated and used for response prediction. Optimum separation with adequate peak shape and suitable run time was acquired with a minimum number of experiments. The developed method was successfully applied for the determination of the studied compounds in their combined dosage form. Evaluation of the greenness profile of the proposed method proved that it is an excellent green analytical procedure. The developed method has advantages over the published chromatographic method, of being time and cost effective. Additionally, method greenness assessment was firstly performed during the analysis of DMH and CIN combined therapy.
Conflicts of interest
There are no conflicts to declare.
References
- G. Bennett, The merck index: an encyclopedia of chemicals, drugs and biologicals, J. Hazard. Mater., 1992, 30, 373, DOI:10.1016/0304-3894(92)87022-8.
- United States Pharmacopeia, 32th Revision, NF 27, The United States Pharmacopoeial Convention Inc., Rockville, MD, 2015 Search PubMed.
- British Pharmacopoeia, Her Majesty’s, the Stationery Office, London, 2014 Search PubMed.
- European Pharmacopoeia, 6.0, European Directorate for the Quality of Medicines, 2014 Search PubMed.
- A.-W. Scholtz, R. Steindl, N. Burchardi, I. Bognar-Steinberg and W. Baumann, Comparison of the therapeutic efficacy of a fixed low-dose combination of cinnarizine and dimenhydrinate with betahistine in vestibular neuritis, Clin. Drug Invest., 2012, 32, 387–399 CrossRef CAS.
- P. Kranke, A. M. Morin, N. Roewer and L. H. J. Eberhart, Dimenhydrinate for prophylaxis of postoperative nausea and vomiting: a meta-analysis of randomized controlled trials, Acta Anaesthesiol. Scand., 2002, 46, 238–244, DOI:10.1034/j.1399-6576.2002.t01-1-460303.x.
- R. Teggi, B. Colombo, O. Gatti, G. Comi and M. Bussi, Fixed combination of cinnarizine and dimenhydrinate in the prophylactic therapy of vestibular migraine: an observational study, Neurol. Sci., 2015, 36, 1869–1873 CrossRef CAS.
- N. S. Abdelwahab, F. H. Edrees, M. T. Alsaadi, N. H. Amin and A. S. Saad, Simultaneous estimation of dimenhydrinate, cinnarizine and their toxic impurities benzophenone and diphenylmethylpiperazine; in silico toxicity profiling of impurities, RSC Adv., 2020, 10, 37439–37448, 10.1039/D0RA06147F.
- H. K. Jeon, Comparative toxicity related to metabolisms of benzophenone-type UV filters, potentially harmful to the environment and humans, Mol. Cell. Toxicol., 2017, 13, 337–343, DOI:10.1007/s13273-017-0037-2.
- Y. Nakagawa and K. Tayama, Estrogenic potency of benzophenone and its metabolites in juvenile female rats, Arch. Toxicol., 2001, 75, 74–79, DOI:10.1007/s002040100225.
- M. C. Rhodes, J. R. Bucher, J. C. Peckham, G. E. Kissling, M. R. Hejtmancik and R. S. Chhabra, Carcinogenesis studies of benzophenone in rats and mice, Food Chem. Toxicol., 2007, 45, 843–851, DOI:10.1016/j.fct.2006.11.003.
- G. A. Burdock, D. H. Pence and R. A. Ford, Safety evaluation of benzophenone, Food Chem. Toxicol., 1991, 29, 741–750, DOI:10.1016/0278-6915(91)90182-7.
- Y. Nakagawa, T. Suzuki and S. Tayama, Metabolism and toxicity of benzophenone in isolated rat hepatocytes and estrogenic activity of its metabolites in MCF-7 cells, Toxicology, 2000, 156, 27–36, DOI:10.1016/S0300-483X(00)00329-2.
- A. Kar and G. I. Aniuha, Spectrophotometric determination of dimenhydrinate with reinecke salt, J. Pharm. Sci., 1981, 70, 690–691, DOI:10.1002/jps.2600700632.
- J. Machado Freitas, T. Da Costa Oliveira, P. L. Silva, D. Tofanello Gimenes, R. A. Abarza Munoz and E. M. Richter, Development of a simple and fast electrochemical method for screening and stoichiometric determination of dimenhydrinate, Electroanalysis, 2014, 26, 1905–1911, DOI:10.1002/elan.201400300.
- U. Döge and K. Eger, A simple HPLC-UV method for the determination of dimenhydrinate and related substances - identification of an unknown impurity, Pharmazie, 2007, 62, 174–178, DOI:10.1691/ph.2007.3.6156.
- B. V. Girish, S. Praveen and N. Kathyayini, Analytical quality by design for development of a sensitive, rapid and stability indicating method for estimation of impurities of dimenhydrinate in its pharmaceutical formulation, Res. J. Pharm., Biol. Chem. Sci., 2016, 7, 1309–1322 CAS.
- T. S. Belal, K. M. Abdel-Hay and C. R. Clark, Selective determination of dimenhydrinate in presence of six of its related substances and potential impurities using a direct GC/MS method, J. Adv. Res., 2016, 7, 53–58, DOI:10.1016/j.jare.2015.01.010.
- H. Abdine, F. Belal and N. Zoman, Simple spectrophotometric determination of cinnarizine in its dosage forms, Farmaco, 2002, 57, 267–271, DOI:10.1016/S0014-827X(02)01204-1.
- S. M. Tawakkol, M. B. El-Zeiny and A. Hemdan, Full spectrum and selected spectrum based chemometric methods for the simultaneous
determination of cinnarizine and dimenhydrinate in laboratory prepared mixtures and pharmaceutical dosage form, Spectrochim. Acta, Part A, 2017, 173, 892–896, DOI:10.1016/j.saa.2016.10.055.
- S. S. M. Hassan, R. M. Abdel-Aziz and A. B. Abbas, Potentiometric membrane sensors for the selective determination of cinnarizine in pharmaceutical preparations, Anal. Chim. Acta, 1996, 321, 47–52, DOI:10.1016/0003-2670(95)00515-3.
- G. O. El-Sayed, S. A. Yasin and A. A. El Badawy, Voltammetric behavior and determination of cinnarizine in pharmaceutical formulations and serum, Anal. Lett., 2008, 41, 3021–3033, DOI:10.1080/00032710802463915.
- S. S. M. Hassan, M. A. F. Elmosallamy and A. B. Abbas, LC and TLC determination of cinnarizine in pharmaceutical preparations and serum, J. Pharm. Biomed. Anal., 2002, 28, 711–719, DOI:10.1016/S0731-7085(01)00662-8.
- A. A. Heda, A. R. Sonawane, G. H. Naranje and P. K. Puranik, A rapid determination of cinnarizine in bulk and pharmaceutical dosage form by LC, E-Journal of Chemistry, 2010, 7, 1080–1084, DOI:10.1155/2010/294096.
- M. T. Rosseel and R. A. Lefebvre, Sensitive determination of cinnarizine in human plasma by high performance liquid chromatography and fluorescence detection, Chromatographia, 1993, 36, 356–358, DOI:10.1007/BF02263891.
- A. P. Argekar and S. J. Shah, Simultaneous determination of cinnarizine and domepiridone maleate from tablet dosage form by reverse phase ion pair high performance liquid chromatography, J. Pharm. Biomed. Anal., 1999, 19, 813–817, DOI:10.1016/S0731-7085(98)00103-4.
- A. El-Gindy, S. Emara and A. Mostafa, Spectrophotometric and LC determination of two binary mixtures containing antihistamines, Il Farmaco, 2004, 59, 713–722, DOI:10.1016/j.farmac.2004.04.010.
- A. Rao, T. Prasanthi, C. Meenakshi, J. Banu, J. Mrunalini, M. C. S. Teja and V. Abhishek, Analytical method development and validation for the estimation of cinnarizine by RP-HPLC in bulk and pharmaceutical dosage forms, Asian J. Pharm. Health Sci., 2019, 9, 2053–2058 CAS.
- D. S. El-Kafrawy and T. S. Belal, Validated HPTLC method for the simultaneous determination of cinnarizine and dimenhydrinate in their combined dosage form, J. Assoc. Arab Univ. Basic Appl. Sci., 2016, 19, 15–22, DOI:10.1016/j.jaubas.2014.06.004.
- N. T. Lamie and H. H. Monir, Simultaneous determination of cinnarizine and dimenhydrinate in binary mixture using chromatographic methods, J. Chromatogr. Sci., 2016, 54, 36–42, DOI:10.1093/chromsci/bmv103.
- A. J. Nathan and A. Scobell, Development and validation of analytical method for simultaneous estimation of cinnarizine and dimenhydrinate in tablet dosage form, Int. J. Pharma Sci. Res., 2014, 5, 4815–4819, DOI:10.1017/CBO9781107415324.004.
- N. T. Lamie and A. M. Yehia, Development of normalized spectra manipulating spectrophotometric methods for simultaneous determination of dimenhydrinate and cinnarizine binary mixture, Spectrochim. Acta, Part A, 2015, 150, 142–150, DOI:10.1016/j.saa.2015.05.048.
- P. B. Shah and P. U. Patel, Q-absorbance ratio spectrophotometric method for the simultaneous estimation of cinnarizine and dimenhydrinate in their combined dosage form, Journal of Pharmaceutical Science and Bioscientific Research, 2012, 2, 83–87 Search PubMed.
- N. S. Abdelwahab, M. M. Abdelrahman, F. M. Salama and A. B. Ahmed, Determination of dimenhydrinate and cinnarizine in combined dosage form in presence of cinnarizine impurity, Eur. J. Chem., 2015, 6, 475–481, DOI:10.5155/eurjchem.6.4.475-481.1324.
- A. B. Ahmed, N. S. Abdelwahab, M. M. Abdelrahman and F. M. Salama, Simultaneous determination of Dimenhydrinate, Cinnarizine and Cinnarizine impurity by TLC and HPLC chromatographic methods, Bull. Fac. Pharmacy, Cairo Univ., 2017, 55, 163–169, DOI:10.1016/j.bfopcu.2017.01.003.
- P. K. Sahu, N. R. Ramisetti, T. Cecchi, S. Swain, C. S. Patro and J. Panda, An overview of experimental designs in HPLC method development and validation, J. Pharm. Biomed. Anal., 2018, 147, 590–611, DOI:10.1016/j.jpba.2017.05.006.
- E. J. Klein and S. L. Rivera, A review of criteria functions and response surface methodology for the optimization of analytical scale HPLC separations, J. Liq. Chromatogr. Relat. Technol., 2000, 23, 2097–2121, DOI:10.1081/JLC-100100475.
- https://www.jmp.com/en_us/applications/design-of-experiments.html#Custom-Designs.
- A. S. Saad, F. H. Edrees, M. T. Elsaady, N. H. Amin and N. S. Abdelwahab, Experimentally Designed Sensor for Direct Determination of the Environmentally Hazardous Compound and Occupational Exposure Biomarker (p-aminophenol) in Different Sampling Matrices, J. Electrochem. Soc., 2020, 167, 147504 CrossRef CAS.
- N. S. Abdelwahab, F. H. Edrees, M. T. Alsaadi, N. H. Amin and A. S. Saad, Therapeutic drug monitoring of two co-administered drugs through development of two ecological chromatographic methods: invivo application, Microchem. J., 2020, 156, 104935, DOI:10.1016/j.microc.2020.104935.
- A. R. Bagheri, M. Arabi, M. Ghaedi, A. Ostovan, X. Wang, J. Li and L. Chen, Dummy molecularly imprinted polymers based on a green synthesis strategy for magnetic solid-phase extraction of acrylamide in food samples, Talanta, 2019, 195, 390–400 CrossRef CAS.
- M. Tobiszewski, M. Marć, A. Gałuszka and J. Namie
nik, Green chemistry metrics with special reference to green analytical chemistry, Molecules, 2015, 20, 10928–10946, DOI:10.3390/molecules200610928. - A. Gałuszka, Z. M. Migaszewski, P. Konieczka and J. Namieśnik, Analytical Eco-Scale for assessing the greenness of analytical procedures, TrAC, Trends Anal. Chem., 2012, 37, 61–72, DOI:10.1016/j.trac.2012.03.013.
- K. Van Aken, L. Strekowski and L. Patiny, EcoScale, a semi-quantitative tool to select an organic preparation based on economical and ecological parameters, Beilstein J. Org. Chem., 2006, 2, 3 Search PubMed.
- J. Płotka-Wasylka, A new tool for the evaluation of the analytical procedure: Green Analytical Procedure Index, Talanta, 2018, 181, 204–209, DOI:10.1016/j.talanta.2018.01.013.
- ICH, Q2 (R1) Validation of Analytical Procedures: Text and Methodology, ICH Harmonized Tripartite Guideline, 2005 Search PubMed.
- https://www.jmp.com/en_us/software/data-analysis-software.html.
- Y. Vander Heyden, A. Nijhuis, J. Smeyers-Verbeke, B. G. M. Vandeginste and D. L. Massart, Guidance for robustness/ruggedness tests in method validation, J. Pharm. Biomed. Anal., 2001, 24, 723–753, DOI:10.1016/S0731-7085(00)00529-X.
- M. Arabi, A. Ostovan, A. R. Bagheri, X. Guo, J. Li, J. Ma and L. Chen, Hydrophilic molecularly imprinted nanospheres for the extraction of rhodamine B followed by HPLC analysis: a green approach and hazardous waste elimination, Talanta, 2020, 120933 CrossRef CAS.
- A. Ostovan, M. Ghaedi, M. Arabi, Q. Yang, J. Li and L. Chen, Hydrophilic multitemplate molecularly imprinted biopolymers based on a green synthesis strategy for determination of B-family vitamins, ACS Appl. Mater. Interfaces, 2018, 10, 4140–4150 CrossRef CAS.
Footnote |
† Electronic supplementary information (ESI) available. See DOI: 10.1039/d0ra09585k |
|
This journal is © The Royal Society of Chemistry 2021 |