DOI:
10.1039/D0RA07368G
(Paper)
RSC Adv., 2021,
11, 397-407
Lipidomics investigations into the tissue phospholipidomic landscape of invasive ductal carcinoma of the breast
Received
27th August 2020
, Accepted 27th November 2020
First published on 22nd December 2020
Abstract
The need of identifying alternative therapeutic targets for invasive ductal carcinoma (IDC) of the breast with high specificity and sensitivity for effective therapeutic intervention is crucial for lowering the risk of fatality. Lipidomics has emerged as a key area for the discovery of potential candidates owing to its several shared pathways between cancer cell proliferation and survival. In the current study, we performed comparative phospholipidomic analysis of IDC, benign and control tissue samples of the breast to identify the significant lipid alterations associated with malignant transformation. A total of 33 each age-matched tissue samples from malignant, benign and control were analyzed to identify the altered phospholipids by using liquid chromatography-multiple reaction monitoring mass spectrometry (LC-MRM/MS). A combination of univariate and multivariate statistical approaches was used to select the phospholipid species with the highest contribution in group segregation. Furthermore, these altered phospholipids were structurally confirmed by tandem mass spectrometry. A total of 244 phospholipids were detected consistently at quantifiable levels, out of which 32 were significantly altered in IDC of the breast. Moreover, in pairwise comparison of IDC against benign and control samples, 11 phospholipids were found to be significantly differentially expressed. Particularly, LPI 20:3, PE (22:1/22:2), LPE 20:0 and PC (20:4/22:4) were observed to be most significantly associated with IDC tissue samples. Apart from that, we also identified that long-chain unsaturated fatty acids were enriched in the IDC tissue samples as compared to benign and control samples, indicating its possible association with the invasive phenotype.
1. Introduction
According to Globocan 2018 data, breast cancer is the most commonly encountered malignant disease among women which accounts for nearly 2.1 million fresh cases every year and causes the highest number of cancer-related mortality worldwide.1 In 2018 alone, 627000 women lost their lives to breast cancer which is nearly 15% of total cancer-associated deaths.2 Invasive ductal carcinoma (IDC) is considered as the most important subtype as it accounts for more than 80% of total incidence of breast cancer.3 As the name suggests, IDC originates in milk ducts of the breast and gradually invades the surrounding fat and/or fibrous tissues. Though, recent advances in diagnostics and effective therapeutic regimen have resulted in the enhanced disease-free survival rate but, it has not eliminated the risk of cancer-associated fatalities. Therefore, the efforts to understand the molecular basis of cancer in a more detailed manner to identify effective therapeutic targets never ceased. The initiation and progression of malignant disease like IDC of breast involves alterations at many levels such as genomics, transcriptomics, proteomics and metabolomics.4–11 Such a multitude of changes in the cellular machinery, brought upon by malignant transformation, aid cancerous cells to survive, adapt, thrive and even evade host defence mechanisms.
Interestingly, altered lipid metabolism is recently established as one of the hallmarks of cancer.12 Lipids are basic building blocks of organelles and cell membrane and could serve as an energy source when nutrients are scarce.13–16 Moreover, lipid moieties could also function as secondary messengers in various signal transduction pathways.12,17 Lipid biosynthesis is substantially elevated in various malignant diseases to compensate for the increasing demand for membrane synthesis in proliferating cancer cells.18,19 Furthermore, higher uptake and storage of lipids are also observed in various cancers.20–23 Sterol Regulatory Element-Binding Proteins (SREBPs) are crucial transcription factors that control the expression of lipid metabolizing genes and are highly up-regulated during pathological conditions such as cancer.24–27
It's now known that several key pathways of lipid metabolism such as synthesis, storage, transport and oxidation overlap with cancer cell proliferation and survival.28 Therefore, it's not surprising that several anti-obesity treatments and anti-lipid peroxidation drugs have been employed and shown promising results in anticancer therapy.29–34 Therefore, it's imperative to further elaborate on molecular changes associated with lipid metabolism upon cancer initiation and progression for the development of novel therapeutic targets.35
The conventional lipidomics methods such as Thin Layer Chromatography (TLC) and High Performance Liquid Chromatography (HPLC) are low throughput approaches and requires large sample quantity to obtain the substantial coverage of all the biologically active lipid molecules present in scarce amount. GC based approaches are time consuming, laborious and often requires derivatization of sample which ultimately results in low metabolic coverage. Moreover, contemporary NMR based method allow us to perform relative and absolute quantitation, it is less sensitive as compared to mass spectrometry based methods due to overlapping signals in either 1H or 31P NMR. With the advent of advanced high throughput mass spectrometry and efficient separation technologies, it's now possible to precisely assess the qualitative and quantitative lipidomics changes associated with malignant disease pathophysiology. Therefore, MS based approaches turned out to be the most popular methods for the lipidomics analysis. The targeted LC-MRM/MS method offers high specificity, sensitivity and robust reproducibility in lipidomics analysis. We have already shown in our earlier study that specific serum phospholipidomic alterations exist for breast cancer patients and can be exploited for diagnostic purposes.36
In this study, we have carried out five classes of phospholipid profiling viz. phosphatidylcholine (PC), phosphatidylethanolamine (PE), phosphatidylserine (PS), phosphatidylinositol (PI) and sphingomyelin (SM) in a cohort of 99 tissue specimen comprising of IDC (n = 33), benign (n = 33) and control (non-malignant) samples (n = 33). Particularly, this study is important to understand the role of altered lipid metabolism in invasive malignant pathology. To the best of our knowledge, this is the first attempt of tissue phospholipid profiling in Indian clinical cohort to identify the lipid alterations associated with invasive ductal carcinoma of the breast.
2. Materials and methods
2.1 Recruitment of study cohort, sample collection and storage
All the tissue samples were collected from the Poona Medical Research Foundation's Ruby Hall Clinic Cancer Centre, Pune. Prior informed written consent was obtained from all the participants of the study. Ethical approval to conduct the study was obtained from the Poona Medical Research Foundation and National Centre for Cell Science, Pune. Total 99 tissue samples (IDC = 33, benign = 33, control = 33) were collected for the study (Table 1). Only freshly diagnosed study subjects without any neoadjuvant chemotherapeutic intervention were included in the study. The study subjects were segregated into malignant and benign groups based upon histopathological analysis of excised tumour tissue. Non-malignant tissue samples were obtained from the same patient by excising normal peripheral breast tissue 5–10 cm away from the malignant tumour. Samples were transported back to the laboratory on ice within 1 h of collection. Samples were then transferred to cryovials, labelled and snap-frozen in liquid nitrogen and stored at −80 °C until further use.
Table 1 Clinicopathological information of the study cohort
Description |
Tissue |
Control (normal) samples |
No. cases |
33 |
Age (average ± standard deviation) |
48 ± 8 |
![[thin space (1/6-em)]](https://www.rsc.org/images/entities/char_2009.gif) |
Benign samples |
No. cases |
33 |
Age (average ± standard deviation) |
45 ± 13 |
Subtype |
|
Fibroadenoma |
17 |
Chronic inflammation |
6 |
Granuloma |
5 |
Other |
5 |
![[thin space (1/6-em)]](https://www.rsc.org/images/entities/char_2009.gif) |
Breast cancer samples |
No. cases |
33 |
Age (average ± standard deviation) |
53 ± 12 |
Type |
|
Invasive ductal carcinoma |
33 |
Tumour grade |
|
Grade 1 |
10 |
Grade 2 |
23 |
Tumour stage |
|
Stage II (T2N1M0, T3N0M0) |
24 |
Stage III (T0N2M0, T1N2M0, T2N2M0, T3N1M0, T3N2M0) |
9 |
Subtype |
|
Luminal A |
16 |
Luminal B |
8 |
HER2 enriched |
6 |
Triple-negative |
3 |
2.2 Lipid extraction and sample preparation
Methyl tert-butyl ether (MTBE) extraction protocol was adopted for the lipid extraction as described elsewhere.37 Briefly, 50 mg of tissue sample was thawed on ice, transferred to homogeniser vial containing zirconium beads and suspended in 400 μL of ice-cold methanol. The tissue sample was homogenised (Precellys Homogeniser, Bertin Corp, USA) at 6000 rpm for 20 s and 6500 rpm for 30 s with 2 cycles each with intermittent cooling on ice. The homogenate was transferred to a glass vial containing 750 μL of methanol and vigorously vortexed for 10 s with subsequent incorporation of 2.5 mL of MTBE. The homogenate was again vortexed for 2 min and incubated for 1 h at room temperature. The homogenate mixture was further treated for phase separation by adding 625 μL of ultrapure water and centrifuged at 1000g for 10 min at 15 °C. The upper organic MTBE containing phase was carefully transferred to separate vial by pasture pipette and evaporated until dryness under vacuum and treated as lipid extract.
2.3 Quantitative phospholipid profiling by LC-MRM/MS
The dried lipid extract was reconstituted in 80 μL of deionised ultrapure water/isopropanol (Sigma-Aldrich, USA) in a ratio of 1/19 containing internal standard for every lipid class viz. PS25:0, PI25:0, PE25:0, SM25:0 and PC25:0 (Avanti Polar Lipids, USA). In tissue samples, phospholipid abundance varies greatly, hence four different injection volumes for each lipid species (PC/SM and PE 10 μL, PS and PI 20 μL) was optimised for better coverage. The data was acquired on 4000 QTRAP® system (SCIEX, USA) coupled with Shimadzu HPLC (Shimadzu Corporation, Japan) equipped with ProSphere™ (150 × 3.2 mm, 5 μm, 300 Å) C4 column (Grace, Albani, USA). The chromatographic parameters, MRM library generation and MS settings were adopted from earlier reported studies.36,38,39
2.4 Data pre-processing
The data obtained by LC-MRM/MS was further processed by the Analyst® 1.5 software (SCIEX, Foster city, USA) for manual inspection of chromatograms and compound identification. The order of analysis was randomised and peak integration was performed in a blinded manner to avoid any bias in the data processing. Lipidview™ software was employed for manual scrutiny and graphical output of the phospholipid differences. Phospholipid species qualifying the minimum 15% of baseline peak intensity were considered for the quantitation purpose. Integrated peak areas were then computed and exported in excel spreadsheet in a data matrix format. The missing values in the data matrix were imputed by half of the minimum positive value in the data set. The data thus obtained was further normalised using MetaboAnalyst webserver to obtain Gaussian distribution. Metabolomics standard initiative (MSI) guidelines were carefully followed during the lipidomics data analysis.40
2.5 Statistical analysis
The normalised data were subjected to multivariate statistical analysis using SIMCA 13.0.2 (Umetrics, Sweeden) software.41 Orthogonal Projections to Latent Structures Discriminant Analysis (OPLS-DA), a supervised multivariate statistical tool was employed for the visualization of group segregation of study cohort based upon differential lipid expression.42 Variable Importance in Projection (VIP) score >1.2 was used to select the phospholipids significantly contributing to the group separation in OPLS-DA score plot. Since, supervised multivariate statistical model such as OPLS-DA has a tendency of overfitting the data, the model was cross-validated by generating 200 permutated models and comparing their R2 (goodness of fit) and Q2 (predictive accuracy) values with the original model.43 Furthermore, Hierarchical Cluster Analysis (HCA) was also performed on data sets to identify the clustering pattern associated with the altered phospholipid profiles of the study cohort.
Univariate statistical parameters such as one way ANOVA with Tukey's HSD post-test and Student's t-test (FDR < 0.05) was carried out to highlight the altered phospholipids with statistical significance in three groups as well as two group comparisons respectively. Finally, the combination of multivariate (VIP > 1.2) and univariate (FDR < 0.05) statistical approaches were used to identify the altered phospholipids signature associated with the malignant group of the study cohort. The ability of particular phospholipid species to discriminate between the malignant, benign and control samples with high specificity and sensitivity was judged by the Receiver Operator Characteristic (ROC) curve analysis.44 SPSS 17.0 software was used for the ROC curve analysis and plotting of box and whisker plots.
2.6 Confirmation of statistically significant phospholipid species by enhanced product ion spectra
Fatty acid composition of the selected phospholipid species was established by the MS/MS fragmentation in enhanced product ion (EPI) mode to produce negatively charged anionic fatty acid fragments.45 Similar conditions of MRM experiments were applied for generation of product ion spectra for selected phospholipid species and corresponding retention time was scrutinised for specific fragmentation pattern. The phospholipid classes PE, PI, PS and PC were acquired in negative mode while SM class was analysed in positive mode. The chromatographic parameters and buffer compositions were adopted from our earlier report.36 The fatty acid position was designated as per the notion that animal phospholipid is saturated at sn1 position and unsaturated at sn2 position.46
3. Results
3.1 Breast IDC tissue phospholipid profiling using LC-MRM/MS
A total of 244 phospholipid species were detected at a quantifiable threshold in LC-MRM/MS analysis which includes 52 PC, 74 PE, 59 PI, 50 PS and 9 SM phospholipid species. The Gaussian distribution of data was obtained by the combination of median normalization, cube root transformation and pareto scaling. The OPLS-DA score plot depicted in Fig. 1a indicates robust separation between control, malignant and benign groups. The separation achieved in the score plot specifies the inherent phospholipid concentration differences present among the study groups. To avoid overfitting of the data, we validated our model with permutation test with 200 permutation models. The goodness of fit (R2) and predictive ability (Q2) of the original model was 0.87 and 0.72 respectively, which is significantly higher than the permutated models (Fig. 1b). Thus, the permutation test showed that there was no overfitting of data in the OPLS-DA model. Clear segregation was observed in the HCA analysis indicative of differential phospholipid expression unique to each group (Fig. 1c).
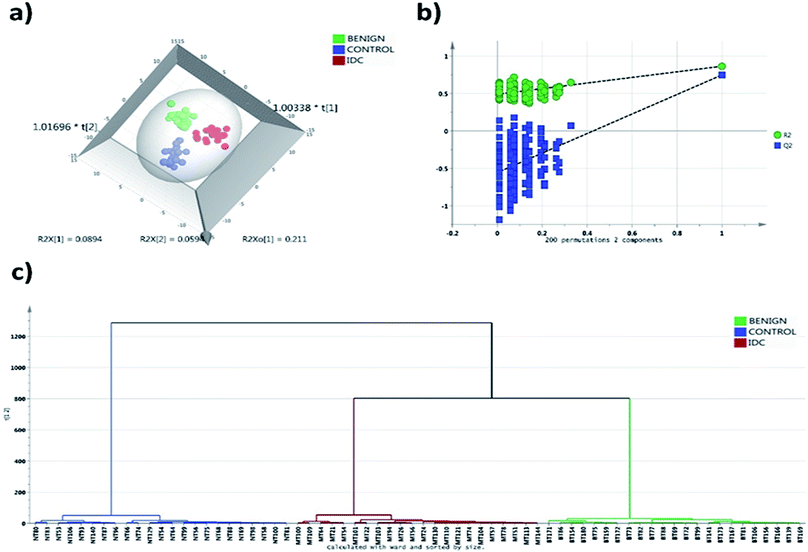 |
| Fig. 1 Multivariate statistical analysis of phospholipids of IDC, benign and control tissue samples. (a) OPLS-DA score plot depicting segregation of IDC (n = 33), benign (n = 33) and control (n = 33) samples. (b) Plot depicting random permutation test (n = 200) on OPLS-DA model. The R2 = 0.87, Q2 = 0.72. (c) Hierarchical cluster analysis of study cohort depicting clear segregation of IDC, benign and control samples. | |
3.2 Confirmation of statistically significant phospholipid species by tandem LC-MS/MS
Enhanced Product Ion (EPI) scan for each phospholipid species identified as significant was carried out to generate information regarding their fatty acid composition. The EPI scans were performed in positive (SM) as well as negative (PE, PC, PS and PI) ionization mode. PE class of phospholipids generated major [M − H]− ions in negative mode ionization. A typical spectrum of PE 40:6 phospholipid (m/z 790.5) eluted at 23.1 min acquired in negative mode is depicted in Fig. 2a. MS/MS fragmentation of PE 40:6 in negative ionization mode generated carboxylate anion fragment at 283.1 m/z and 327.2 m/z corresponding to [M − H]− ion of 18:0 and 22.6 FA respectively. As reported elsewhere, phospholipid from animal sources contains saturated fatty acid at the sn-1 position and unsaturated fatty acid at the sn-2 position.47 Therefore, PE 40:6 was reconfirmed as PE (18:0/22:6). Likewise, remaining PE species along with other PI and PS classes were also analysed in EPI scan mode and their FA composition were established.
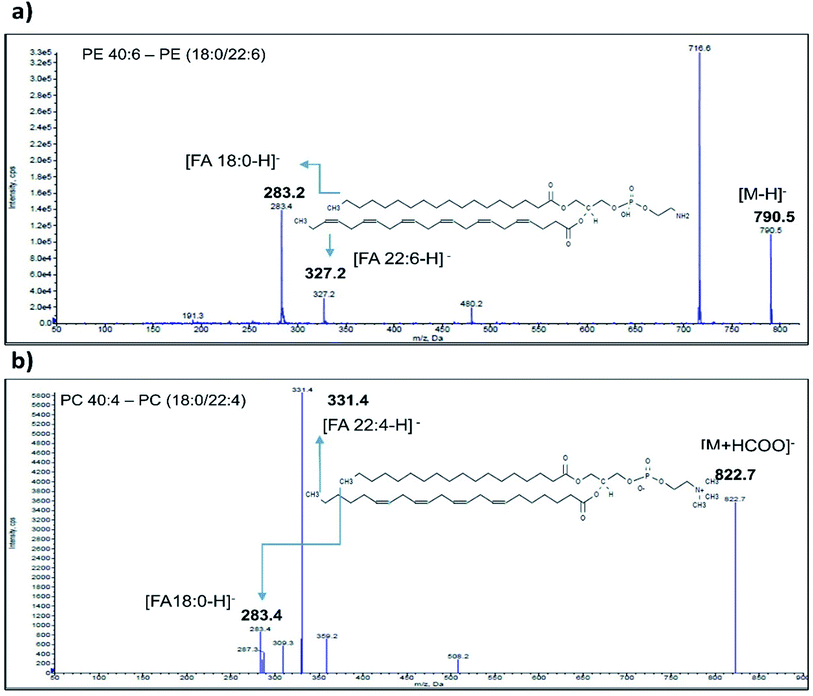 |
| Fig. 2 Confirmation of significant phospholipid species by Enhanced Product Ion (EPI) spectra. (a) Representative EPI spectrum of PE 40:6 showing prominent carboxylate ion fragment at m/z 283.2 corresponding to [FA 18:0-H]− and another fragment ion at m/z 327.2 corresponding to [FA 22:6-H]−. Hence PE 40:2 is confirmed as PE (18:0/22:6). (b) Representative EPI spectrum of PC 40:4 at m/z 822.7 [M + HCOOH]− showing fragment at m/z 283.2 [FA 18:0-H]− and 331.4 [FA 22:4-H]− confirms PC 40:4 as PC (18:0/22:4). | |
Furthermore, the PC class of phospholipids generated formate adduct [M + 45]− ions in greater abundance as compared to the [M − H]− ions and hence subjected to further MS/MS analysis. EPI scanning of formate adduct [M + 45]− resulted in the generation of prominent fatty acid fragments corresponding to PC lipids. Typical EPI scan spectrum of PC 40:4 generated 822.7 m/z formate adduct eluted at 22.1 min is depicted in Fig. 2b. Similarly, MS/MS fragment ions observed at 283.4 m/z and 331.4 m/z corresponds to PC (18:0/22:4) (Fig. 2b). Likewise, the remaining PC class of fatty acids were investigated by using [M + 45]− formate adduct ion. SM phospholipid species contains phosphorylcholine ester bound to the ceramide and generates a specific fragment of 184 m/z after head group loss. The fragments generated in EPI scan carried out in a positive mode contains a long-chain base (LCB) and smaller FA ions.
3.3 Identification of altered tissue phospholipid signature associated with IDC of breast
Univariate and multivariate statistical approaches were combined (VIP > 1.2, p-value < 0.05) to identify the phospholipid species that were significantly altered in IDC of breast and characterised by tandem mass spectrometry are summarised in Table 2. Moreover, Tukey's HSD posthoc analysis was also carried out to enhance confidence in group separation.
Table 2 Statistically significant phospholipids identified through a combination of univariate (ANOVA p-value < 0.05) and multivariate (OPLS-DA VIP > 1.2) analysis
Sr. no. |
Lipid name |
Confirmed name |
VIP score |
p-value |
FDR |
Tukey's HSD |
1 |
LPC 22:4 |
LPC 22:4 |
1.56 |
2.14 × 10−11 |
5.23 × 10−9 |
Control-benign; IDC-Benign |
2 |
PI 20:3 |
LPI 20:3 |
1.53 |
2.32 × 10−7 |
2.38 × 10−5 |
IDC-benign; IDC-control |
3 |
PC 34:4 |
PC (14:0/20:4) |
1.5 |
5.18 × 10−6 |
1.15 × 10−4 |
IDC-benign; IDC-control |
4 |
PE 40:6 |
PE (18:0/22:6) |
1.45 |
4.77 × 10−6 |
1.15 × 10−4 |
Control-benign; IDC-control |
5 |
PE 18:0 |
LPE 18:0 |
1.44 |
7.99 × 10−6 |
1.62 × 10−4 |
IDC-benign; IDC-control |
6 |
PI 22:1 |
LPI 22:1 |
1.42 |
3.89 × 10−7 |
2.38 × 10−5 |
Control-benign; IDC-control |
7 |
PE 42:5 |
PE (20:0/22:5) |
1.39 |
5.97 × 10−5 |
7.29 × 10−4 |
Control-benign; IDC-control |
8 |
PC 32:1 |
PC (16:0/16:1) |
1.39 |
2.51 × 10−4 |
2.27 × 10−3 |
IDC-benign; IDC-control |
9 |
PI 14:0 |
LPI 14:0 |
1.38 |
1.09 × 10−6 |
4.43 × 10−5 |
Control-benign; IDC-control |
10 |
PE 20:0 |
LPE 20:0 |
1.38 |
1.78 × 10−5 |
3.10 × 10−4 |
IDC-benign; IDC-control |
11 |
PS 34:2 |
PS (16:0/18:2) |
1.35 |
1.91 × 10−5 |
3.10 × 10−4 |
Control-benign; IDC-control |
12 |
PI 16:0 |
LPI 16:0 |
1.32 |
7.98 × 10−4 |
4.99 × 10−3 |
IDC-benign; IDC-control |
13 |
PI 42:8 |
PI (20:4/22:4) |
1.31 |
4.57 × 10−6 |
1.15 × 10−4 |
IDC-benign; IDC-control |
14 |
PC 34:3 |
PC (16:0/18:3) |
1.31 |
7.89 × 10−3 |
2.27 × 10−2 |
IDC-benign |
15 |
PI 18:2 |
LPI 18:2 |
1.3 |
1.30 × 10−3 |
6.81 × 10−3 |
IDC-benign; IDC-control |
16 |
PE 44:3 |
PE (22:1/22:2) |
1.28 |
9.31 × 10−7 |
4.43 × 10−5 |
Control-benign; IDC-benign; IDC-control |
17 |
PC 34:1 |
PC (16:0/18:1) |
1.28 |
3.33 × 10−3 |
1.29 × 10−2 |
IDC-benign |
18 |
PC 40:8 |
PC (20:4/20:4) |
1.27 |
6.21 × 10−3 |
2.02 × 10−2 |
IDC-benign |
19 |
PE 44:7 |
PE (22:2/22:5) |
1.26 |
5.45 × 10−5 |
7.20 × 10−4 |
Control-benign; IDC-control |
20 |
PE 18:1 |
LPE 18:1 |
1.25 |
3.27 × 10−4 |
2.75 × 10−3 |
IDC-benign; IDC-control |
21 |
PI 38:2 |
PI (18:0/20:2) |
1.25 |
5.61 × 10−5 |
7.20 × 10−4 |
Control-benign; IDC-control |
22 |
PI 44:8 |
PI (22:4/22:4) |
1.24 |
1.98 × 10−6 |
6.91 × 10−5 |
Control-benign; IDC-benign; IDC-control |
23 |
PI 38:4 |
PI (18:0/22:4) |
1.24 |
9.27 × 10−3 |
2.58 × 10−2 |
IDC-benign; IDC-control |
24 |
PC 40:3 |
PC (18:1/22:2) |
1.24 |
4.46 × 10−3 |
1.58 × 10−2 |
IDC-benign; IDC-control |
25 |
PC 40:7 |
PC (18:2/22:5) |
1.24 |
4.46 × 10−3 |
1.58 × 10−2 |
IDC-benign; IDC-control |
26 |
PE 42:2 |
PE (20:0/22:2) |
1.24 |
1.97 × 10−4 |
2.09 × 10−3 |
Control-benign; IDC-control |
27 |
PC 32:2 |
PC (14:0/18:2) |
1.23 |
6.64 × 10−3 |
2.08 × 10−2 |
IDC-benign |
28 |
PI 36:0 |
PI (18:0/18:0) |
1.23 |
1.66 × 10−4 |
1.84 × 10−3 |
IDC-control |
29 |
PE 42:4 |
PE (20:0/22:4) |
1.23 |
4.70 × 10−4 |
3.49 × 10−3 |
Control-benign; IDC-control |
30 |
PI 32:1 |
PI (14:0/18:1) |
1.22 |
2.42 × 10−3 |
1.03 × 10−2 |
IDC-control |
31 |
PC 42:8 |
PC (20:4/22:4) |
1.22 |
5.06 × 10−4 |
3.53 × 10−3 |
IDC-benign; IDC-control |
32 |
PC 40:4 |
PC (20:0/20:4) |
1.2 |
1.55 × 10−3 |
7.43 × 10−3 |
IDC-control |
3.3.1 Comparative analysis of IDC against controls. A total of 24 phospholipid species were identified as differentially expressed in IDC as compared to the control group, out of which, 20 were up-regulated and 4 were down-regulated (Table 3). The altered phospholipids comprise of 10 PI species, 8 PE species, 5 PC species and 1 PS species. Out of ten differentially regulated PI species, 8 were up-regulated and 2 were down-regulated. The up-regulated PI species constitutes LPI (20:3), LPI (22:1), PI (18:0/20:2), PI (18:0/18:0), PI (14:0/18:1), (PI 18:0/22:4), PI (22:4/22:4) and LPI 18:2. The down-regulated PI species comprises PI (20:4/22:4) and LPI 14:0. For PE species, 7 were up-regulated and 1 was down-regulated. The up-regulated PE species were PE (22:1/22:2), LPE 20:0, PE (18:0/22:6), PE (20:0/22:2), PE (20:0/22:5), LPE 18:0 and PE (20:0/22:4). Only one PE species i.e. PE (22:2/22:5) was down-regulated. All the five PC species were up-regulated which constitutes PC (20:4/22:4), PC (20:0/20:4), PC (14:0/20:4), PC (16:0/16:1) and PC (18:1/22:2). The lone PS species PS (16:0/18:2) was detected as down-regulated in IDC as compared to the control. Among all these altered phospholipid species, LPI 20:3, PE (22:1/22:2), LPE 20:0 and LPI 22:1 were most significantly altered in IDC (AUC > 0.87) as compared to rest of the phospholipids.
Table 3 Significantly altered phospholipids identified in IDC by pairwise comparison with control tissue samples
Sr. no. |
Lipid name |
Confirmed name |
VIP score |
p-value |
FDR |
FC |
AUC |
1 |
PI 20:3 |
LPI 20:3 |
1.53 |
2.32 × 10−7 |
2.38 × 10−5 |
18.49 |
0.89 |
2 |
PE 44:3 |
PE (22:1/22:2) |
1.28 |
9.31 × 10−7 |
4.43 × 10−5 |
7.2 |
0.89 |
3 |
PE 20:0 |
LPE 20:0 |
1.38 |
1.78 × 10−5 |
3.10 × 10−4 |
8.18 |
0.87 |
4 |
PI 22:1 |
LPI 22:1 |
1.42 |
3.89 × 10−7 |
2.38 × 10−5 |
7.16 |
0.87 |
5 |
PI 42:8 |
PI (20:4/22:4) |
1.31 |
4.57 × 10−6 |
1.15 × 10−4 |
0.36 |
0.86 |
6 |
PI 14:0 |
LPI 14:0 |
1.38 |
1.09 × 10−6 |
4.43 × 10−5 |
0.5 |
0.85 |
7 |
PI 38:2 |
PI (18:0/20:2) |
1.25 |
5.61 × 10−5 |
7.20 × 10−4 |
9.48 |
0.85 |
8 |
PI 36:0 |
PI (18:0/18:0) |
1.23 |
1.66 × 10−4 |
1.84 × 10−3 |
7.78 |
0.83 |
9 |
PS 34:2 |
PS (16:0/18:2) |
1.35 |
1.91 × 10−5 |
3.10 × 10−4 |
0.53 |
0.83 |
10 |
PE 40:6 |
PE (18:0/22:6) |
1.45 |
4.77 × 10−6 |
1.15 × 10−4 |
5.19 |
0.82 |
11 |
PE 18:0 |
LPE 18:0 |
1.44 |
7.99 × 10−6 |
1.62 × 10−4 |
7.27 |
0.82 |
12 |
PE 42:2 |
PE (20:0/22:2) |
1.24 |
1.97 × 10−4 |
2.09 × 10−3 |
4.69 |
0.81 |
13 |
PE 42:5 |
PE (20:0/22:5) |
1.39 |
5.97 × 10−5 |
7.29 × 10−4 |
3.97 |
0.8 |
14 |
PC 42:8 |
PC (20:4/22:4) |
1.22 |
5.06 × 10−4 |
3.53 × 10−3 |
7.75 |
0.8 |
15 |
PI 32:1 |
PI (14:0/18:1) |
1.22 |
2.42 × 10−3 |
1.03 × 10−2 |
28.48 |
0.79 |
16 |
PC 40:4 |
PC (20:0/20:4) |
1.2 |
1.55 × 10−3 |
7.43 × 10−3 |
6.88 |
0.79 |
17 |
PC 34:4 |
PC (14:0/20:4) |
1.5 |
5.18 × 10−6 |
1.15 × 10−4 |
9.05 |
0.79 |
18 |
PE 42:4 |
PE (20:0/22:4) |
1.23 |
4.70 × 10−4 |
3.49 × 10−3 |
4.61 |
0.78 |
19 |
PI 38:4 |
PI (18:0/22:4) |
1.24 |
9.27 × 10−3 |
2.58 × 10−2 |
1.82 |
0.77 |
20 |
PE 44:7 |
PE (22:2/22:5) |
1.26 |
5.45 × 10−5 |
7.20 × 10−4 |
0.59 |
0.76 |
21 |
PI 44:8 |
PI (22:4/22:4) |
1.24 |
1.98 × 10−6 |
6.91 × 10−5 |
3.9 |
0.75 |
22 |
PC 32:1 |
PC (16:0/16:1) |
1.39 |
2.51 × 10−4 |
2.27 × 10−3 |
6.73 |
0.75 |
23 |
PI 18:2 |
LPI 18:2 |
1.3 |
1.30 × 10−3 |
6.81 × 10−3 |
5.71 |
0.74 |
24 |
PC 40:3 |
PC (18:1/22:2) |
1.24 |
4.46 × 10−3 |
1.58 × 10−2 |
6.04 |
0.7 |
3.3.2 Comparative analysis of IDC against benign. Furthermore, comparative analysis between IDC and benign samples were also performed to identify the distinctive phospholipids. A total of 17 phospholipid species were significantly altered in IDC as compared to benign which includes 14 up-regulated and 3 down-regulated lipids (Table 4). Total 5 PI species were dysregulated out of which 3 were up-regulated and 2 were down-regulated. The up-regulated PI species comprises PI (22:4/22:4), LPI 20:3 and LPI 18:2. The down-regulated PI species consist of PI (20:4/22:4) and LPI 16:0. Total 3 PE species i.e. LPE 18:0, LPE 18:1 and LPE 20:0 found to be significantly up-regulated in IDC as compared to benign samples. Furthermore, a total of 9 PC species were detected at significantly altered levels in IDC out of which 8 were up-regulated and 1 was down-regulated. The up-regulated PC species constitutes PC (14:0/20:4), PC (16:0/16:1), PC (16:0/18:1), PC (16:0/18:3), PC (20:4/20:4), PC(18:2/22:5), PC (14:0/18:2) and PC (20:4/22:4). The only one down-regulated PC species detected was LPC 22:4. Among all of these altered phospholipids, PI (22:4/22:4), LPC 22:4, PI (20:4/22:4) and LPI 20:3 are the most significantly altered lipids with highest segregation ability (AUC > 0.84) in ROC curve plot.
Table 4 Significantly altered phospholipids identified in IDC by pairwise comparison with benign tissue samples
Sr. no. |
Lipid name |
Confirmed name |
VIP score |
p-value |
FDR |
FC |
AUC |
1 |
PI 44:8 |
PI (22:4/22:4) |
1.24 |
1.98 × 10−6 |
6.91 × 10−5 |
5.34 |
0.92 |
2 |
LPC 22:4 |
LPC 22:4 |
1.56 |
2.14 × 10−11 |
5.23 × 10−9 |
0.79 |
0.87 |
3 |
PI 42:8 |
PI (20:4/22:4) |
1.31 |
4.57 × 10−6 |
1.15 × 10−4 |
0.37 |
0.85 |
4 |
PI 20:3 |
LPI 20:3 |
1.53 |
2.32 × 10−7 |
2.38 × 10−5 |
5.73 |
0.84 |
5 |
PC 34:4 |
PC (14:0/20:4) |
1.5 |
5.18 × 10−6 |
1.15 × 10−4 |
4.84 |
0.82 |
6 |
PE 18:0 |
LPE 18:0 |
1.44 |
7.99 × 10−6 |
1.62 × 10−4 |
4.43 |
0.81 |
7 |
PI 16:0 |
LPI 16:0 |
1.32 |
7.98 × 10−4 |
4.99 × 10−3 |
0.4 |
0.8 |
8 |
PC 32:1 |
PC (16:0/16:1) |
1.39 |
2.51 × 10−4 |
2.27 × 10−3 |
3.95 |
0.78 |
9 |
PI 18:2 |
LPI 18:2 |
1.3 |
1.30 × 10−3 |
6.81 × 10−3 |
3.21 |
0.78 |
10 |
PE 18:1 |
LPE 18:1 |
1.25 |
3.27 × 10−4 |
2.75 × 10−3 |
7.6 |
0.77 |
11 |
PC 34:1 |
PC (16:0/18:1) |
1.28 |
3.33 × 10−3 |
1.29 × 10−2 |
2.87 |
0.77 |
12 |
PE 20:0 |
LPE 20:0 |
1.38 |
1.78 × 10−5 |
3.10 × 10−4 |
3.15 |
0.75 |
13 |
PC 34:3 |
PC (16:0/18:3) |
1.31 |
7.89 × 10−3 |
2.27 × 10−2 |
2.65 |
0.75 |
14 |
PC 40:8 |
PC (20:4/20:4) |
1.27 |
6.21 × 10−3 |
2.02 × 10−2 |
2.76 |
0.74 |
15 |
PC 40:7 |
PC (18:2/22:5) |
1.24 |
4.46 × 10−3 |
1.58 × 10−2 |
3.04 |
0.74 |
16 |
PC 32:2 |
PC (14:0/18:2) |
1.23 |
6.64 × 10−3 |
2.08 × 10−2 |
3.29 |
0.72 |
17 |
PC 42:8 |
PC (20:4/22:4) |
1.22 |
5.06 × 10−4 |
3.53 × 10−3 |
3.02 |
0.71 |
3.3.3 Comparative analysis of IDC against both controls and benign. Finally, a panel of 11 phospholipids that was most significantly associated with IDC subjects as compared to benign as well as control samples was identified (Table 5). The phospholipid species with the highest discriminatory potential for IDC group as compared to controls were selected. The same panel was also probed for their ability to distinguish between IDC samples from the benign group. The phospholipids were selected by Tukey's HSD test and compared AUC values between IDC vs. Control and IDC vs. benign samples obtained from ROC curve analysis. It is observed that phospholipid species LPI 20:3, PE (22:1/22:2), LPE 20:0 and PC (20:4/22:4) are having the highest discriminatory potential for IDC samples. Moreover, these phospholipids were also able to differentiate benign samples from controls but with reduced discriminatory ability. The differential expressions of these phospholipids are indicated using box-and-whisker plots (Fig. 3a). The ROC curve analysis of the selected phospholipids showed higher AUC values (0.85) for the IDC samples than that of benign samples (0.78) when compared to controls (Fig. 3b). This indicates that the phospholipids selected have higher specificity towards IDC discrimination as compared to benign from control samples.
Table 5 Significantly altered phospholipids associated with IDC as compared to benign and control tissue samples (p-value < 0.05 for all the altered phospholipids mentioned below)
Sr. no. |
Lipid name |
Confirmed name |
FC IDC Vs C |
AUC IDC Vs C |
FC IDC Vs B |
AUC IDC Vs B |
1 |
PI 20:3 |
LPI 20:3 |
18.49 |
0.894 |
5.73 |
0.837 |
2 |
PE 44:3 |
PE (22:1/22:2) |
7.2 |
0.892 |
2.96 |
0.697 |
3 |
PE 20:0 |
LPE 20:0 |
8.18 |
0.871 |
3.15 |
0.746 |
4 |
PC 42:8 |
PC (20:4/22:4) |
7.75 |
0.8 |
3.02 |
0.708 |
5 |
PC 34:4 |
PC (14:0/20:4) |
9.05 |
0.788 |
4.84 |
0.824 |
6 |
PI 44:8 |
PI (22:4/22:4) |
3.9 |
0.75 |
5.34 |
0.917 |
7 |
PC 32:1 |
PC (16:0/16:1) |
6.73 |
0.749 |
3.95 |
0.783 |
8 |
PI 18:2 |
LPI 18:2 |
5.71 |
0.741 |
3.21 |
0.776 |
9 |
PC 40:7 |
PC (18:2/22:5) |
6.04 |
0.703 |
3.04 |
0.736 |
10 |
PE 18:1 |
LPE 18:1 |
8.13 |
0.679 |
7.6 |
0.774 |
11 |
LPC 22:4 |
LPC 22:4 |
1.74 |
0.652 |
0.79 |
0.871 |
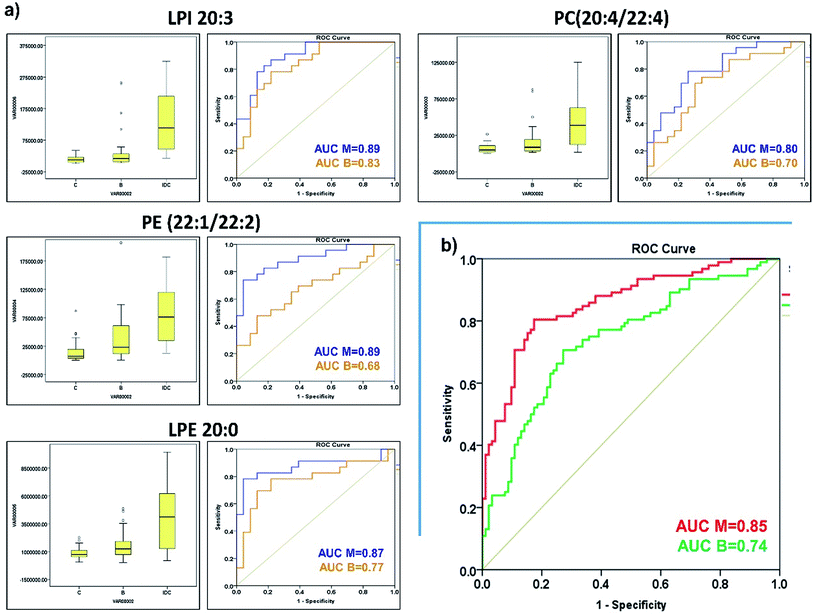 |
| Fig. 3 (a) Box and whisker plots illustrating abundance differences along with ROC curve analysis of selected phospholipids predicting IDC (blue) and benign (orange) from control samples. (b) Combined predictive ability of selected phospholipids to discriminate IDC (red) and benign (green) samples from control samples. | |
4. Discussion
Several clinical and preclinical studies highlighted the crucial role of altered lipid metabolism in cancer initiation, progression and metastasis.48–53 The lipidomics is the most preferred and widely used approach to identify the differentially expressed lipid moieties in various malignant pathophysiologies. The qualitative and quantitative analysis of lipids require robust and reliable methodology with high sensitivity and specificity. With the advent of high throughput hyphenated liquid chromatography-mass spectrometry instruments, it is now possible to determine the precise abundance levels of individual lipid species in complex biological matrices. In this study, we performed targeted LC/MRM-MS of tissue samples to investigate the altered phospholipid profiles associated with IDC of the breast. Total 24 phospholipid species belonging to various classes were selected as most significantly altered in IDC of the breast as compared to controls with the help of univariate and multivariate statistical approaches.
PC class lipids constitute a major component of a mammalian membrane lipid bilayer and play an important part in various signalling cascades. In our analysis, we observed elevated levels of several PC lipid species viz. PC (16:0/16:1), PC (14:0/20:4), PC (20:0/20:4), PC (18:1/22:2) and PC (20:4/22:4) in IDC tissue samples as compared to normal tissues. Similarly, all the statistically significant PC lipids found to be up-regulated in IDC tissues as compared to the benign samples. Our findings are in good agreement with earlier reports where authors have shown that PC lipids are up-regulated in breast cancer.54 Moreover, we have observed increased levels of LPC 22:4 (lysophosphocholines) in IDC tissue samples as compared to the benign tissues. Interestingly, LPC, a partial hydrolysis by-product of PC lipids act as potent signalling modulator and can activate several signalling pathways of importance such as cell growth, proliferation, migration, metastasis and apoptosis mediated through G protein-coupled receptors.55–57 Since we have observed up regulation of LPC in IDC as compared to benign samples, its role in malignant transformation needs to be probed further.
PE is the second most abundant phospholipids of cell membrane and regarded as crucial for the membrane fluidity. Moreover, PE lipids also regulate several signalling pathways by controlling Ca+ transport. In our study, we observed elevated levels of several PE lipids viz. PE (18:0/22:4), PE (20:0/22:2), PE (20:0/22:4), PE (20:0/22:5) and PE (22:1/22:2) in IDC tissue samples as compared to normal tissues. Furthermore, we have detected increased levels of lysophosphatidylethanolamine (LPE) 18:0 and LPE 20:0 in IDC tissue samples as compared to normal tissues. Besides, LPE 18:0, LPE 18:1 and LPE 20:0 were also detected at higher levels in IDC tissues as compared to benign samples. LPE a lyso-type metabolite of PE lipids has been known to increase intracellular Ca++ concentration via lysophosphatidic acid LPA1 and CD97 receptors and contributes to the proliferation and migration of breast cancer cells.58,59 Therefore, it is not surprising that LPE abundance levels are enhanced in the IDC tissues as compared to benign as well as normal tissues.
PI's are situated on the cytoplasmic side of the plasma membrane and serve as a precursor of crucial signalling molecule phosphatidylinositol-3,4,5-triphosphate (PIP3).60 It's a well-established fact that PIP3 signalling regulates cancer progression by controlling critical cellular processes such as cell growth, survival and migration.60 Furthermore, in our study we have detected enhanced abundance of several PI species in IDC tissue samples as compared to normal tissues. It is interesting to observe the proportional increase in the PI abundance levels in IDC samples as compared to normal samples but their correlation needs to be further probed. Statistically significant PI lipids viz. PI (22:4/22:4) and (20:4/22:4) have shown mixed response in IDC vs. benign comparison but LPI lipids (LPI 20:3, 18:2 and 16:0) has shown overall up regulation in IDC tissues as compared to benign samples. Recently, it has been shown that metastatic breast cancer patients exhibit elevated plasma LPI lipid levels as compared to the healthy individuals.61 In the same study, it has also shown that external feeding of LPI results in increased cell migratory abilities of breast cancer cells in a dose-dependent manner. Interestingly, LPI acts through G protein-coupled receptor GPR55 implicated in the regulation of breast cancer cell migration.
PS is an anionic lipid species which is asymmetrically distributed in the intracellular side of the membrane and flipped to the extracellular surface upon malignant transformation.62,63 We have noticed overall decreased abundance levels of PS (16:0/18:2) in IDC tissue samples as compared to normal tissues. Though, it's known that the PS lipids have increased exposure on the extracellular surface of tumour cells but unfortunately, their total abundance levels and their correlation with malignant disease pathophysiology is not probed in detail.64,65
Moreover, it should be noted that we have detected higher levels of several long-chain unsaturated fatty acid-containing phospholipids in IDC tissues as compared to normal tissue. It is a well known fact that higher content of unsaturated fatty acid-containing phospholipids are directly proportional to membrane fluidity.66 The cancer cells with high membrane fluidity tend to be more flexible and distort easily which enhances their invasive ability.67 Furthermore, increased levels of unsaturated fatty acids lead to the higher vascular permeability in the tumour which affects several crucial processes such as proliferation, differentiation, migration and metastasis.68 Thus, in our study cohort, unsaturated fatty acid-containing phospholipids are abundant in IDC tissue samples as compared to the normal tissues indicating their predisposition toward invasive malignant disease.
Furthermore, it's also worth noting that from our earlier serum lipidomics study of the same study cohort, we have identified statistically significant few common phospholipids.36 Interestingly, these phospholipids viz., PC (18:1/22:2), PE (20:0/22:2) and PE (20:0/22:4) exhibit inverse abundance correlations with each other. Their abundance was increased in IDC tissue and decreased in IDC serum as compared to their respective control samples. The possible explanation for this could be that these phospholipids contain very long-chain unsaturated fatty acids and are essential to modulate the membrane fluidity. Hence, these phospholipids could be sourced from the serum to fulfil the membrane synthesis demand. Therefore, the high abundance of these phospholipids in tissue samples and lower abundance in serum implies an invasive tendency of malignant disease. Indeed, similar results were reported in case of key metabolites altered in tissue and serum samples of same patients from IDC.11
5. Conclusion
Uncontrolled cell proliferation is one of the most defining parameters of malignant transformation. These proliferating cancer cells accumulate biomass by upregulating lipid and cholesterol biosynthesis pathways to support rapid cell growth and division. Phospholipids are a key constituent of lipid bilayer membrane and play a crucial role in several signalling cascades involved in tumorigenesis. Therefore, it's imperative to profile phospholipid alterations specific to the IDC of the breast to gain useful insights into disease pathophysiology. In the current study, we have identified a panel of phospholipids viz., LPI 20:3, PE (22:1/22:2), LPE 20:0 and PC (20:4/22:4) that can distinguish IDC from benign and control samples. We observed that phospholipid alterations taking place in IDC to control are different from IDC to benign samples. However, we also detected some phospholipid changes that are common to benign and malignant samples. The altered landscape of phospholipid is indicative of excessively active endogenous lipid biosynthesis pathway, higher activity of phospholipases and increased demand of unsaturated very long-chain fatty acids in IDC tumours as compared to normal tissues. Moreover, we also identified PC (18:1/22:2), PE (20:0/22:2) and PE (20:0/22:4) as common phospholipids altered in tissue and serum but with inverse correlation. Overall, the observed alterations in lipid metabolism offer us valuable inputs regarding the IDC pathophysiology and panel of phospholipids identified in this study reflects altered lipid metabolism upon invasive malignant transformation. But we also understand that the results obtained in this study needs to be validated in the large independent clinical cohort before being employed in clinical settings with high confidence.
Conflicts of interest
The authors declare no conflict of interest
Acknowledgements
This research was supported by NCCS intramural funding and Department of Biotechnology, Govt. of India, India (grant no. BT/PR10855/BRB/10/1330/2014). The authors are grateful to all the volunteers who participated in this study. RT and KT acknowledges CSIR, New Delhi for research associateship.
References
- F. Bray, J. Ferlay, I. Soerjomataram, R. L. Siegel, L. A. Torre and A. Jemal, Ca-Cancer J. Clin., 2018, 68, 394–424 CrossRef
. - World Heal. Organ., The Global Cancer Observatory, 2019, 876, pp. 2018–2019 Search PubMed
. - J. Makki, Clin. Med. Insights: Pathol., 2015, 8, 23–31 Search PubMed
. - M. Behring, S. Shrestha, U. Manne, X. Cui, A. Gonzalez-Reymundez, A. Grueneberg and A. I. Vazquez, Oncotarget, 2018, 9, 36836–36848 CrossRef
. - L. Aswad, S. P. Yenamandra, G. S. Ow, O. Grinchuk, A. V. Ivshina and V. A. Kuznetsov, Oncotarget, 2015, 6, 36652–36674 CrossRef
. - Y. J. Heng, S. C. Lester, G. M. K. Tse, R. E. Factor, K. H. Allison, L. C. Collins, Y. Y. Chen, K. C. Jensen, N. B. Johnson, J. C. Jeong, R. Punjabi, S. J. Shin, K. Singh, G. Krings, D. A. Eberhard, P. H. Tan, K. Korski, F. M. Waldman, D. A. Gutman, M. Sanders, J. S. Reis-Filho, S. R. Flanagan, D. M. A. Gendoo, G. M. Chen, B. Haibe-Kains, G. Ciriello, K. A. Hoadley, C. M. Perou and A. H. Beck, J. Pathol., 2017, 241, 375–391 CrossRef CAS
. - J. Eswaran, D. Cyanam, P. Mudvari, S. D. N. Reddy, S. B. Pakala, S. S. Nair, L. Florea, S. A. W. Fuqua, S. Godbole and R. Kumar, Sci. Rep., 2012, 2, 264 CrossRef
. - N. C. S. Oliveira, T. H. B. Gomig, H. H. Milioli, F. Cordeiro, G. G. Costa, C. A. Urban, R. S. Lima, I. J. Cavalli and E. M. S. F. Ribeiro, GMR, Genet. Mol. Res., 2016, 2, gmr.15027701 Search PubMed
. - J. Yang, J. Zhu, K. He, L. Y. Zhao, L. Y. Liu, T. S. Song and C. Huang, J. Clin. Lab. Anal., 2015, 29, 321–327 CrossRef CAS
. - G. G. Da Costa, T. H. B. Gomig, R. Kaviski, K. S. Sousa, C. Kukolj, R. S. De Lima, C. D. A. Urban, I. J. Cavalli and E. M. S. F. Ribeiro, Cancer Genomics Proteomics, 2015, 12, 251–262 Search PubMed
. - T. H. More, S. RoyChoudhury, J. Christie, K. Taunk, A. Mane, M. K. Santra, K. Chaudhury and S. Rapole, Oncotarget, 2018, 9, 2678–2696 CrossRef
. - C. Cheng, F. Geng, X. Cheng and D. Guo, Cancer Commun., 2018, 38, 27 CrossRef
. - G. Van Meer, D. R. Voelker and G. W. Feigenson, Nat. Rev. Mol. Cell Biol., 2008, 9, 112–124 CrossRef CAS
. - J. C. M. Holthuis and A. K. Menon, Nature, 2014, 510, 48–57 CrossRef CAS
. - A. Efeyan, W. C. Comb and D. M. Sabatini, Nature, 2015, 517, 302–310 CrossRef CAS
. - J. A. Menendez and R. Lupu, Nat. Rev. Cancer, 2007, 7, 763–777 CrossRef CAS
. - D. Guo, E. H. lavi. Bell and A. Chakravarti, CNS Oncol., 2013, 2, 289–299 CrossRef CAS
. - S. Yoon, M. Y. Lee, S. W. Park, J. S. Moon, Y. K. Koh, Y. H. Ahn, B. W. Park and K. S. Kim, J. Biol. Chem., 2007, 282, 26122–26131 CrossRef CAS
. - F. Röhrig and A. Schulze, Nat. Rev. Cancer, 2016, 16, 732–749 CrossRef
. - S. Yue, J. Li, S. Y. Lee, H. J. Lee, T. Shao, B. Song, L. Cheng, T. A. Masterson, X. Liu, T. L. Ratliff and J. X. Cheng, Cell Metab., 2014, 19, 393–406 CrossRef CAS
. - S. Koizume and Y. Miyagi, Int. J. Mol. Sci., 2016, 9, 1430 CrossRef
. - A. Nath, I. Li, L. R. Roberts and C. Chan, Sci. Rep., 2015, 5, 14752 CrossRef CAS
. - J. Zhao, Z. Zhi, C. Wang, H. Xing, G. Song, X. Yu, Y. Zhu, X. Wang, X. Zhang and Y. Di, Oncol. Rep., 2017, 38, 2105–2115 CrossRef CAS
. - T. Il Jeon and T. F. Osborne, Trends Endocrinol. Metab., 2012, 23, 65–72 CrossRef
. - W. Shao and P. J. Espenshade, Cell Metab., 2012, 16, 414–419 CrossRef CAS
. - Y. A. Yang, P. J. Morin, W. F. Han, T. Chen, D. M. Bornman, E. W. Gabrielson and E. S. Pizer, Exp. Cell Res., 2003, 282, 132–137 CrossRef CAS
. - J. Bao, L. Zhu, Q. Zhu, J. Su, M. Liu and W. Huang, Oncol. Lett., 2016, 12, 2409–2416 CrossRef CAS
. - J. Long, C.-J. Zhang, N. Zhu, K. Du, Y.-F. Yin, X. Tan, D.-F. Liao and L. Qin, Am. J. Cancer Res., 2018, 8, 778–791 CAS
. - M. J. Roberts, J. W. Yaxley, G. D. Coughlin, T. R. J. Gianduzzo, R. C. Esler, N. T. Dunglison, S. K. Chambers, R. J. Medcraft, C. W. K. Chow, H. J. Schirra, R. S. Richards, N. Kienzle, M. Lu, I. Brereton, H. Samaratunga, J. Perry-Keene, D. Payton, C. Oyama, S. A. Doi, M. F. Lavin and R. A. Gardiner, Contemp. Clin. Trials, 2016, 50, 16–20 CrossRef
. - S. N. Apostolova, R. A. Toshkova, A. B. Momchilova and R. D. Tzoneva, Adv. Anticancer Agents Med. Chem., 2016, 16, 1512–1522 CrossRef CAS
. - B. Smith and H. Land, Cell Rep., 2012, 2, 580–590 CrossRef CAS
. - R. U. Svensson, S. J. Parker, L. J. Eichner, M. J. Kolar, M. Wallace, S. N. Brun, P. S. Lombardo, J. L. Van Nostrand, A. Hutchins, L. Vera, L. Gerken, J. Greenwood, S. Bhat, G. Harriman, W. F. Westlin, H. J. Harwood, A. Saghatelian, R. Kapeller, C. M. Metallo and R. J. Shaw, Nat. Med., 2016, 22, 1108–1119 CrossRef CAS
. - E. S. Pizer, F. J. Chrest, J. A. DiGiuseppe and W. F. Han, Cancer Res., 1998, 58, 4611–4615 CAS
. - H. Xu, M. bo Hu, P. de Bai, W. hui Zhu, Q. Ding and H. wen Jiang, Int. Urol. Nephrol., 2014, 46, 2327–2334 CrossRef CAS
. - M. Hilvo, C. Denkert, L. Lehtinen, B. Müller, S. Brockmöller, T. Seppänen-Laakso, J. Budczies, E. Bucher, L. Yetukuri, S. Castillo, E. Berg, H. Nygren, M. Sysi-Aho, J. L. Griffin, O. Fiehn, S. Loibl, C. Richter-Ehrenstein, C. Radke, T. Hyötyläinen, O. Kallioniemi, K. Iljin and M. Orešič, Cancer Res., 2011, 71, 3236–3245 CrossRef CAS
. - T. H. More, M. Bagadi, S. RoyChoudhury, M. Dutta, A. Uppal, A. Mane, M. K. Santra, K. Chaudhury and S. Rapole, Metabolomics, 2017, 13, 3 CrossRef
. - V. Matyash, G. Liebisch, T. V. Kurzchalia, A. Shevchenko and D. Schwudke, J. Lipid Res., 2008, 49, 1137–1146 CrossRef CAS
. - E. Marien, M. Meister, T. Muley, S. Fieuws, S. Bordel, R. Derua, J. Spraggins, R. Van De Plas, J. Dehairs, J. Wouters, M. Bagadi, H. Dienemann, M. Thomas, P. A. Schnabel, R. M. Caprioli, E. Waelkens and J. V. Swinnen, Int. J. Cancer, 2015, 137, 1539–1548 CrossRef CAS
. - E. P. Rhee, S. Cheng, M. G. Larson, G. A. Walford, G. D. Lewis, E. McCabe, E. Yang, L. Farrell, C. S. Fox, C. J. O'Donnell, S. A. Carr, R. S. Vasan, J. C. Florez, C. B. Clish, T. J. Wang and R. E. Gerszten, J. Clin. Invest., 2011, 121, 1402–1411 CrossRef CAS
. - J. L. Griffin, A. W. Nicholls, C. A. Daykin, S. Heald, H. C. Keun, I. Schuppe-Koistinen, J. R. Griffiths, L. L. Cheng, P. Rocca-Serra, D. V. Rubtsov and D. Robertson, Metabolomics, 2007, 3, 179–188 CrossRef CAS
. - G. Blekherman, R. Laubenbacher, D. F. Cortes, P. Mendes, F. M. Torti, S. Akman, S. V. Torti and V. Shulaev, Metabolomics, 2011, 7, 329–343 CrossRef CAS
. - M. Bylesjö, D. Eriksson, A. Sjödin, S. Jansson, T. Moritz and J. Trygg, BMC Bioinf., 2007, 8, 207 CrossRef
. - M. N. Triba, L. Le Moyec, R. Amathieu, C. Goossens, N. Bouchemal, P. Nahon, D. N. Rutledge and P. Savarin, Mol. BioSyst., 2015, 11, 13–19 RSC
. - J. A. Hanley and B. J. McNeil, Radiology, 1982, 143, 29–36 CrossRef CAS
. - X. Han and R. W. Gross, J. Am. Soc. Mass Spectrom., 1995, 6, 1202–1210 CrossRef CAS
. - E. Hvattum, G. Hagelin and Å. Larsen, Rapid Commun. Mass Spectrom., 1998, 12, 1405–1409 CrossRef CAS
. - T. Karupaiah and K. Sundram, Nutr. Metab., 2007, 4, 16 CrossRef
. - Y. Zhu, M. D. Aupperlee, Y. Zhao, Y. S. Tan, E. L. Kirk, X. Sun, M. A. Troester, R. C. Schwartz and S. Z. Haslam, Oncotarget, 2016, 7, 83409–83423 CrossRef
. - G. Yan, L. Li, B. Zhu and Y. Li, Oncotarget, 2016, 7, 33429–33439 CrossRef
. - E. Thysell, I. Surowiec, E. Hörnberg, S. Crnalic, A. Widmark, A. I. Johansson, P. Stattin, A. Bergh, T. Moritz, H. Antti and P. Wikström, PLoS One, 2010, 5, e14175 CrossRef CAS
. - C. H. Byon, R. W. Hardy, C. Ren, S. Ponnazhagan, D. R. Welch, J. M. McDonald and Y. Chen, Lab. Invest., 2009, 89, 1221–1228 CrossRef CAS
. - C. W. Lu, Y. H. Lo, C. H. Chen, C. Y. Lin, C. H. Tsai, P. J. Chen, Y. F. Yang, C. H. Wang, C. H. Tan, M. F. Hou and S. S. F. Yuan, Cancer Lett., 2017, 388, 130–138 CrossRef CAS
. - D. de Gonzalo-Calvo, L. López-Vilaró, L. Nasarre, M. Perez-Olabarria, T. Vázquez, D. Escuin, L. Badimon, A. Barnadas, E. Lerma and V. Llorente-Cortés, BMC Cancer, 2015, 15, 460 CrossRef
. - M. L. Dória, C. Z. Cotrim, C. Simões, B. Macedo, P. Domingues, M. R. Domingues and L. A. Helguero, J. Cell. Physiol., 2013, 228, 457–468 CrossRef
. - G. Schneider, Z. P. Sellers, A. Abdel-Latif, A. J. Morris and M. Z. Ratajczak, Mol. Cancer Res., 2014, 12, 1560–1573 CrossRef CAS
. - J. W. Choi and J. Chun, Biochim. Biophys. Acta, Mol. Cell Biol. Lipids, 2013, 1831, 20–32 CrossRef CAS
. - Y. Xu, Biochim. Biophys. Acta, Mol. Cell Biol. Lipids, 2002, 1582, 81–88 CrossRef CAS
. - K. S. Park, H. Y. Lee, S. Y. Lee, M. K. Kim, S. D. Kim, J. M. Kim, J. Yun, D. S. Im and Y. S. Bae, FEBS Lett., 2007, 581, 4411–4416 CrossRef CAS
. - S. J. Park, K. P. Lee, S. Kang, H. Y. Chung, Y. S. Bae, F. Okajima and D. S. Im, Cell. Signalling, 2013, 25, 2147–2154 CrossRef CAS
. - M. P. Wymann and R. Schneiter, Nat. Rev. Mol. Cell Biol., 2008, 9, 162–176 CrossRef CAS
. - X. L. Zhou, X. Guo, Y. P. Song, C. Y. Zhu and W. Zou, Acta Pharmacol. Sin., 2018, 39, 459–471 CrossRef CAS
. - J. H. Stafford and P. E. Thorpe, Neoplasia, 2011, 13, 299–308 CrossRef CAS
. - D. Zhao, J. H. Stafford, H. Zhou and P. E. Thorpe, Transl Oncol., 2011, 4, 355–364 CrossRef
. - R. F. A. Zwaal and A. J. Schroit, Blood, 1997, 89, 1121–1132 CrossRef CAS
. - S. Ran and P. E. Thorpe, Int. J. Radiat. Oncol., Biol., Phys., 2002, 54, 1479–1484 CrossRef CAS
. - W. K. Subczynski and A. Wisniewska, Acta Biochim. Pol., 2000, 47, 613–625 CrossRef CAS
. - S. Beloribi-Djefaflia, S. Vasseur and F. Guillaumond, Oncogenesis, 2016, 5, e189 CrossRef CAS
. - A. Hendrich and K. Michalak, Curr. Drug Targets, 2005, 4, 23–30 CrossRef
.
Footnote |
† Both authors contributed equally to the preparation of this manuscript. |
|
This journal is © The Royal Society of Chemistry 2021 |
Click here to see how this site uses Cookies. View our privacy policy here.