Structural variation of protein–ligand complexes of the first bromodomain of BRD4†
Received
5th April 2021
, Accepted 3rd June 2021
First published on 4th June 2021
Abstract
The bromodomain-containing protein 4 (BRD4), a member of the bromodomain and extra-terminal domain (BET) family, plays a key role in several diseases, especially cancers. With increased interest in BRD4 as a therapeutic target, many X-ray crystal structures of the protein in complex with small molecule inhibitors are publicly available over the recent decade. In this study, we use this structural information to investigate the conformations of the first bromodomain (BD1) of BRD4. Structural alignment of 297 BRD4-BD1 complexes shows a high level of similarity between the structures of BRD4-BD1, regardless of the bound ligand. We employ WONKA, a tool for detailed analyses of protein binding sites, to compare the active site of over 100 of these crystal structures. The positions of key binding site residues show a high level of conformational similarity, with the exception of Trp81. A focused analysis on the highly conserved water network in the binding site of BRD4-BD1 is performed to identify the positions of these water molecules across the crystal structures. The importance of the water network is illustrated using molecular docking and absolute free energy perturbation simulations. 82% of the ligand poses were better predicted when including water molecules as part of the receptor. Our analysis provides guidance for the design of new BRD4-BD1 inhibitors and the selection of the best structure of BRD4-BD1 to use in structure-based drug design, an important approach for faster and more cost-efficient lead discovery.
1 Introduction
The bromodomains and extra-terminal domain (BET) family of proteins recognize acetylated N-terminal tails of histones through interactions of their bromodomains (BDs) and acetylated lysine residues.1,2 BET proteins play a crucial role in regulating gene expression and are an important target to develop inhibitors to regulate protein expression by inhibiting these epigenetic interactions. The most extensively studied member of the BET family is the bromodomain-containing protein 4 (BRD4), because of its promise as a therapeutic target for diseases such as cancer, neurodegenerative disorders, inflammation and obesity.3–7 Increased interest in BRD4 and its potential inhibitors has resulted in a wealth of X-ray crystal structures, providing atomistic insight into its binding site interactions. However, this also means that it is increasingly difficult to choose which crystal structure is preferred as the starting point of any in silico study. Within this work, we use the structural information, which is publicly available, to help understand the flexibility of BRD4 and examine the effects of small molecule inhibitors, with a focus on the BD1 binding pocket. This analysis provides guidance in selecting crystal structures and other features, such as crystallographic water molecules.
Like its family members, BRD2, BRD3 and BRDT, BRD4 consists of two N-terminal BDs (BD1 and BD2) and an extra C-terminal domain (ET).8 Each BD is composed of four helices (αZ, αA, αB and αC), which are connected by the ZA loop and BC loop, creating the acetyl-lysine binding site (Fig. 1a). BRD4-BD1 recognises histone H4, which is anchored by a hydrogen bonding interaction between the carbonyl oxygen atom on the acetylated lysine and an asparagine residue Asn140 on the BC loop of the receptor (Fig. 1b).9 A second interaction is formed through a water mediated hydrogen bond between the acetyl lysine and tyrosine residue Tyr97 on the ZA loop. Additional binding site residues create a deep hydrophobic cavity, with Trp81 and Met149 also considered key residues in H4 and small molecule inhibitor binding.10 Over the last decade, there have been many small molecule inhibitors published, with some of them reaching human clinical trials.11–16 Contained within these inhibitors is a wide chemical diversity with core motifs encompassing azepines, 3,5-dimethyl isoxazoles, pyridones, triazolopyridines, tetrahydroquinolines, 4-acyl pyrroles and 2-thiazolidinones.17,18 Each of these structural classes contains a unique warhead, which competes with H4 to replicate the interactions with Asn140 and Tyr97. An additional common feature of small drug-like compounds bound to BRD4-BD1 is a lipophilic group, which can extend into the binding pocket and interact with the hydrophobic WPF shelf (Trp81-Pro82-Phe83).
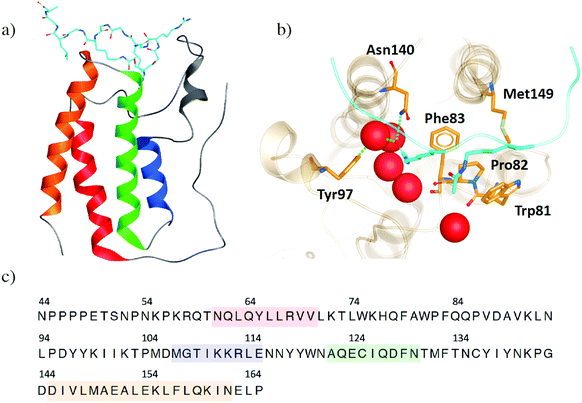 |
| Fig. 1 (a) Structure of BRD4-B1 (PDB 3UVW) with histone H4 (light blue) bound. (b) Binding site of BRD4-BD1. Key binding site residues are highlighted as sticks. Histone H4 is shown in light blue, with acetylated lysine residues shown as sticks. Water molecules at the base of the binding site are shown as red spheres. (c) The common sequence of BRD4-BD1 across the X-ray crystal structures in our data set. Secondary structures of α-helices αZ, αA, αB and αC are coloured in red, blue, green and orange, respectively. | |
The consideration of water molecules is important for ligand binding, as they can stabilize a complex by acting as a bridge for hydrogen bonds and their displacement by a ligand can result in a decrease in binding affinity of that ligand.19 However, it is also possible for displacement to be associated with a favorable gain in entropy with the release of a well-ordered molecule into the bulk solvent, resulting in an increase in ligand binding affinity. It is therefore crucial in drug design to understand which water molecules mediate protein–ligand interactions and which can be targeted for displacement. At the base of the BRD4-BD1 binding pocket, there is a network of highly conserved water molecules, which has been found to be important in ligand binding and stabilising the protein structure.10,20,21 Zhong et al.22 investigated the structural and thermodynamic properties of the crystallographic water molecules. They found that it is energetically unfavourable to displace a water molecule with a small drug-like compound, as this would require a large amount of energy to compensate breaking the hydrogen bonding network. Furthermore, molecular dynamics simulations demonstrated a high occupancy for several of the water sites. Using these findings, Zhong et al. developed a docking based virtual screening protocol to identify novel inhibitors towards BRD4. This study highlighted the importance of the water network in structure-based drug design.
The pool of BRD4 inhibitors continues to grow, with most identified through fragment or structure-based drug design based on properties of known BRD4 inhibitors.23–27 Additionally, a recent review28 identified three novel strategies in targeting BRD4, including bivalent BRD4 inhibitors, proteolytic targeting chimeric molecules and re-purposing of kinase inhibitors. The selectivity of inhibitors is also being targeted.29–31 Initially many compounds were developed as BRD4 inhibitors. However, due to the structural similarity across the BDs of BET proteins, many of these were pan-inhibitors which could cause adverse side effects such as dizziness and nausea.32,33 Gilan et al.29 used structure-based design to discover compounds that interact specifically with either the BD1 or BD2 of BET proteins, providing new insights into improving therapeutic strategies with fewer toxic side effect. Speck-Planche et al.31 have also developed the first multi-target quantitative structure activity relationship (mt-QSAR) model, which can predict BET inhibitor potency against BRD2, BRD3 and BRD4.
As in many drug discovery campaigns, computational methods remain an important tool in finding BRD4 inhibitors.23,25,34,35 Structure based virtual screening methods, such as docking and 3D-QSAR, can facilitate high-throughput approximations of binding affinities. Molecular dynamics simulations expand on static representations of protein–ligand complexes by providing a more dynamic view and develop our understanding of structural patterns and interactions, which lead to high potency and selectivity.36,37 As a crucial goal of drug development is designing a compound that binds competitively and strongly, alchemical free energy calculations are becoming increasingly important as, when done well, they can provide accurate estimations of binding free energies and present a way to minimize the number of compounds that are made in the laboratory. Pan et al.23 discovered a BRD4-histone deactylase (HDAC) inhibitor, a promising therapeutic strategy for colorectal carcinoma, through high-throughput rigid molecular docking of fragments of known BRD4 inhibitors, embedded into the fragment-like library of ZINC.38 A flexible docking method was then implemented on the top 200 scoring fragments. Following this docking, 24 compounds were synthesised and tested based on the 10 top scoring fragments, resulting in a promising lead compound. The extensive research already conducted on BRD4 and its inhibitors also makes it an excellent test case for the development of novel computational workflows. For example, Fusani et al.39 merged active learning with the comparative binding energy (COMBINE) method and demonstrated its performance using a BRD4 dataset. Active learning was used to introduce an uncertainty estimation component to the COMBINE method, which is a powerful tool in studying the structural information of protein–ligand complexes and deriving QSAR for structurally similar series of compounds.
A fundamental component of structure based in silico methods is the use of X-ray crystal structures. It is therefore important to be able to rely on the starting conformations of proteins in order to make accurate predictions. For example, different active site conformations may lead to different binding poses, which can severely impact predicted binding affinities.40,41 While molecular dynamics simulations, combined with enhanced sampling methods, can be used to find the most stable binding pose, these methods come with a computational expense and it is still sensible to choose the starting structure with care. In a study on the T4 lysozyme L99A, Lim et al.42 demonstrated that predicted relative free energy values are sensitive to initial protein conformation, even when using enhanced sampling. In our study, we examine the X-ray crystal structures of BRD4-BD1 complexes in the PDB and perform structural clustering, to identify the variation of conformations and the best static representative structures of the receptor. To compare the binding site of multiple complexes and identify ligands which cause structural variation, we use WONKA.43,44 WONKA is a tool for ligand-based, residue-based and water-based analyses of protein–ligand structural ensembles. The advantage of using WONKA over other visualisation and analysis tools, such as PyMOL,45 is its ability to identify trends within a data set. It can identify patterns between structure and individual ligand complexes, and these observations are displayed on a web based graphical user interface. WONKA also analyses water displacements and relates which ligands displace conserved water molecules. Therefore, we are able to explore the extent of the conservation of crystallographic water molecules in the BRD4-BD1 binding pocket and highlight functional groups present in the ligands, which displace the usually highly conserved water network. Molecular docking is also used to assess the accuracy of predicted binding poses, with and without the water network present.
2 Materials and methods
Structural data
A survey of the PDB reveals, at the time of this study, 323 X-ray crystal structures of BRD4 in complex with a variety of ligands. To identify the common sequence, multiple sequence alignment was performed using the Clustal Omega46 alignment tool in Chimera.47 Sequence alignment shows that 26 of the ligands are co-crystallised with BD2 and are therefore discounted. In total, 297 BRD4-BD1 complexes are taken forward for further analysis.
Structural clustering
To prepare the receptor structures for clustering, the ligands were first removed, multiple sequence alignment was performed and the common sequence across all structures (Fig. 1c) was retained, with the remaining tails removed. Protein structural clustering was performed using ClusCo,48 a software tool for the clustering and comparison of protein models. This utilizes an open source K-means49 code for Hierarchical Agglomerative Clustering. To identify a sensible number of groups to cluster the structures into, all-vs-all pairwise root-mean-square deviation (RMSD) values were calculated. RMSD was based on Cα atoms. The centroid of the whole ensemble was established by clustering with the cluster number set to one.
Although there is no specified upper limit to the number of crystal structures that WONKA can analyse, we found that a cutoff of 100 structures is preferable for the software to perform smoothly. To ensure that effects of a wide range of ligand activity and structural diversity were captured, the crystal structures studied using WONKA were chosen based on structural clustering of the co-crystallised compounds. Out of the 297 crystal structures of BRD4-BD1, there are 266 unique ligands in complex with the receptor, which have accessible experimental data. Compounds that show little or no activity (pIC50 < 5) were discounted, leaving 175 compounds to be clustered. Ligand structural clustering was performed in DataWarrior,50 using Fragp descriptors and Tanimoto similarity (T). Two structures were considered to be similar if T > 0.8. Representative compounds from each cluster, and their respective crystal structures, were chosen for analysis using WONKA.
Molecular docking
The representative compounds from ligand-based clustering were selected for molecular docking. Ligand coordinates were extracted from their original crystal structures and docked against the centroid crystal structure of BRD4-BD1 (PDB 4BJX), with and without the water network included as part of the receptor. All other crystallographic water molecules were removed in both cases. To prepare the crystal structure for docking, the receptor generation software as part of the OpenEye docking toolkit51,52 was used. The co-crystallised compound with ID 73B was assigned as the ligand and therefore did not interact with docked molecules. A box centred around the original ligand with sides of length 15.7 Å × 20.7 Å × 19.0 Å was situated to cover the BRD4-BD1 binding cavity, giving a total receptor volume of 6151 Å3. Constraints were applied to ensure a heavy atom contact with Asn140. Compounds to be docked were protonated according to physiological pH and prepared using OpenEye OMEGA.52 Conformers were generated using a truncated form of the MMFF94s force field53 with a maximum energy difference of 20 kcal mol−1 set from the lowest energy conformer. A maximum of 1000 conformers was allowed and those within 0.5 Å of any others were considered as duplicates and removed. Docking was performed using OpenEye FRED.51 Compounds were docked using the high resolution setting with rotational and translational step sizes of 1 Å. To measure the accuracy of the docking with and without the water network present, heavy atom RMSD was calculated between the docked poses and the crystallographic poses of each of the ligands.
Absolute free energy calculations
Absolute free energy perturbation (FEP) simulations were performed to calculate binding free energies. To illustrate the impact of starting structure, two calculations were performed, each starting from a different binding pose of the same ligand. Initial coordinates were obtained from the molecular docking study, where the ligand (PDB 83T) was docked to the centroid structure of BRD4-BD1 (PDB 4BJX), with and without active site crystallographic water molecules. This binding site water network was retained for the FEP calculation in the case where it had been involved in the docking. System preparation and the simulations were performed using GROMACS 2020.3
54 and the CHARMM force field.55 Ligand parameters were generated using CGenFF.56 The complexes were solvated in a dodecahedral box with an edge distance of 3 nm, to construct an explicitly modelled solvent consisting of around 32
000 TIP3P water molecules57 and two Cl− ions to give a net neutral charge. In absolute FEP simulations, ligand binding affinity is estimated by calculating the difference between the free energy change of decoupling the ligand from solution and decoupling from the receptor. Therefore, a system was also prepared with the ligand free in solution. Upon setup, systems were minimized for 200 ps using a steepest descent algorithm. Equilibration was performed for 200 ps in the NVT ensemble with harmonic position restraints applied to the heavy atoms with a force constant of 1000 kJ mol−1 nm−2. Temperature coupling was achieved by using velocity rescaling with a stochastic term and a reference temperature of 298 K. A further equilibration was performed for 4 ns in the NPT ensemble with a Berendsen pressure and temperature coupling scheme. The decoupling of the ligands from the receptor was split into 30 lambda windows, where ligand restraints were applied and van der Waals and Coulomb interactions were gradually turned off. The relative positions of the ligands with respect to the receptor were restrained by one bond, two angles and three dihedral harmonic potentials. To account for these restraints, a correction is applied to the free energy of binding. For the free ligand in solvent, van der Waals and Coulomb interactions were decoupled over 20 lambda windows. Each lambda window consisted of 1 ns of equilibration in the NPT ensemble, followed by 2 ns of data collection. Free energy changes were evaluated with the Bennett Acceptance Ratio (BAR) method58 as implemented in GROMACS.
3. Results and discussion
Receptor based structural clustering
Fig. 2 shows the distribution of the resolution of the crystal structures identified in the PDB; they have an average resolution of 1.60 Å. Overall, we can consider the structures to be high-resolution and have confidence in the quality of the crystal structure data.
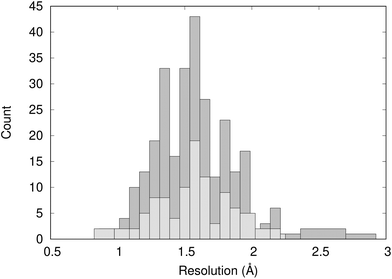 |
| Fig. 2 Distribution of the resolution of 297 X-ray crystal structures of BRD4-BD1 complexes (dark grey) and the 101 crystal structures analysed using WONKA (light grey). | |
Structural differences within the ensemble of crystal structures were measured using pairwise RMSD. The median and mean pairwise RMSDs are 0.56 Å and 0.58 ± 0.22 Å respectively. These RMSD values are small and suggest high similarity between most protein structures within the ensemble. The structural similarity between the superimposed structures can be observed in Fig. 3a. The maximum pairwise RMSD is 1.70 Å between PDB entries 5KU3 and 6V1L. The overlay of these structures (Fig. 3b) suggests the largest structural variance occurs in the tail leading to the N-terminus. There is a small amount of deviation in the ZA loop. Given the narrow distribution of RMSD values, it is sensible to group the structures into five clusters. Fig. 4 shows an overlay of the representation crystal structures of the five structural clusters. The position of the binding site residues further demonstrates the similarity between different structures of the receptor. The centroid of the whole ensemble is the crystal structure with PDB code 4BJX, which has a resolution of 1.59 Å. With such a high number of crystal structures available for BRD4, these results can aid the selection of the most representative structures to use for the computational study of BRD4-BD1.
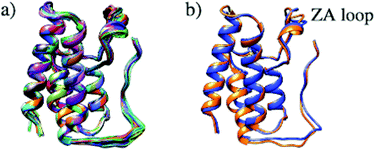 |
| Fig. 3 (a) Superimposed structures of 297 BRD4-BD1 X-ray crystal structures available in the PDB. (b) Comparison of structures PDB 5KU3 (blue) and 6V1L (orange), which have the highest pairwise RMSD. | |
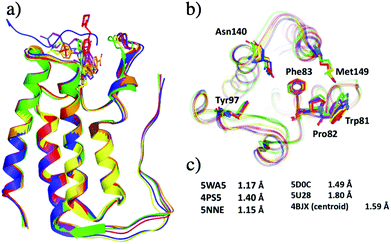 |
| Fig. 4 Representative X-ray crystal structures after grouping 297 crystal structures of BRD4-BD1 into five clusters. The PDB codes for the structures are 5WA5 (purple), 4PS5 (red), 5NNE (blue), 5D0C (yellow), 5U28 (lime) and 4BJX (orange). (a) Secondary structures of the five representative structures. (b) Active site of the five structures, key binding residues are highlighted as sticks. (c) The resolutions of each X-ray crystal structure. | |
Ligand based structural clustering
Clustering the co-crystallised ligands, which have experimental pIC50 values of >5, based on structural similarity resulted in 101 groups. The distribution of ligand activity for the whole data set and the 101 representative compounds from each cluster is shown in Fig. 5. The representative compounds have a pIC50 range of 5.0 to 8.8 and cover a relatively wide span of activity. Therefore, no further filtering of the compounds was performed and the complexes containing these ligands were taken forward for analysis using WONKA.
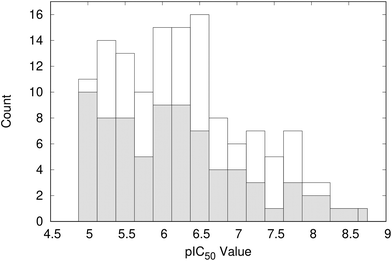 |
| Fig. 5 Distribution of pIC50 values for the representative 101 compounds (shaded) compared to the total data set (open). | |
Structural diversity of the binding site
WONKA enables the identification of trends in the position of active site residues for multiple crystal structures of the same protein. Fig. 6 shows the superimposition of the key binding site residues in BRD4-BD1 for each of the co-crystal structures, identified by ligand based structural clustering. WONKA clusters a particular residue's conformations into different groups based on an all-vs-all heavy atom RMSD of 2.5 Å between like-residues in a structural ensemble. Asn140, Tyr97, Met149, Trp81, Pro82 and Phe83 show 5, 3, 6, 9, 4 and 2 clusters respectively.
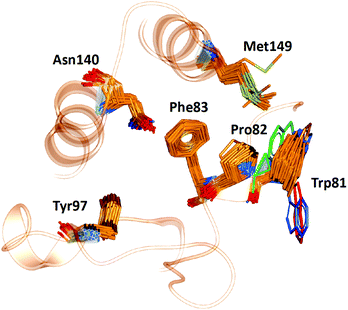 |
| Fig. 6 The position of key binding site residues in BRD4-BD1 over 101 X-ray crystal structures. Conformations of Trp81, which show the largest deviation, are shown in blue, red and green. | |
Visual inspection reveals that all key binding site residues, with the exception of Trp81, have a high level of conformational similarity across all of the crystal structures, regardless of the structure or activity of the bound ligand. There are three structures of Trp81 that show dissimilar conformations, highlighted in blue, red and green in Fig. 6. There are multiple factors that could play a role in the observed disorder of Trp81. It is important to recognise that these crystal structures provide information on only one static conformation. A protein is flexible in solution, and it is possible that the positions of these Trp81 residues are more ordered when sampling a different conformation. Interactions with other amino acids within the protein and with the bound ligand can also influence the position of binding site residues. The bound ligands that correlate with these deviations in Trp81 position are shown in Fig. 7. The compounds have experimental pIC50 values of 5.26, 5.89 and 5.20, which are towards the lower end of the range. As these values were measured using different biological assays, we should be cautious about directly comparing them with each other and the remaining dataset.59 However, for a potent compound, we would expect a pIC50 value upwards of 7.5, regardless of the assay conditions. A possible reason for these Trp81 positions could be that any hydrophobic interactions formed by the ligand with Trp81 do not outweigh the stability gained by polar ligand atoms forming solvent interactions. No additional binding site residue interactions are observed, in place of an interaction with Trp81. Therefore, compounds that result in this disorder of Trp81 are not desirable and do not correlate with higher binding affinity. Furthermore, it would be sensible to use structures with a ‘regular’ Trp81 position for the basis of computational studies.
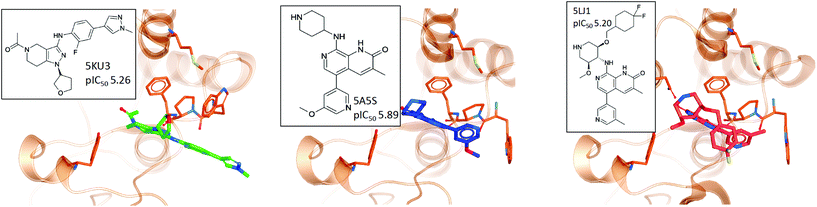 |
| Fig. 7 The three crystal structures and corresponding ligands, which contain Trp81 conformations most dissimilar from the whole PDB ensemble. | |
Active site water molecules
It is well documented that a network of conserved water molecules plays an important role in BRD4-BD1 ligand binding.20–22,29,60,61 Including crystallographic water molecules is an important consideration for computational studies, and there has been many tools developed to locate water molecules in protein binding sites.62–65 Crystallographic water molecules can drastically change the binding mode in protein–ligand docking22 and also provide stability to the system in more advanced methods such as binding free energy calculations.66 As part of our exploration of X-ray crystal structures, we used WONKA to analyse the occupancy of the water network, which lines the BRD4-BD1 binding pocket, as shown in Fig. 8. From the positions of the water molecules, we can identify that the water molecule at site 2 mediates a hydrogen bond between Tyr97 and bound ligands. The size of the red spheres, which represent crystallographic water molecules, reflect how conserved they are across the ensemble of crystal structures. For example, the sphere at site 1 is the largest as there is only one crystal structure, which does not include a water molecule at this position. All water clusters within 8 Å of the ligand are displayed and there is a maximum distance of 1.5 Å between a point in a cluster and the cluster centre.
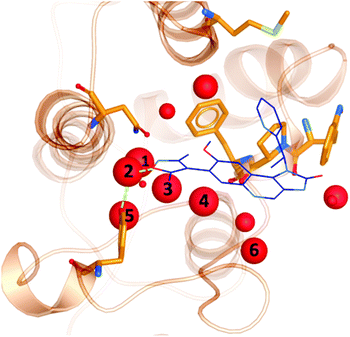 |
| Fig. 8 Crystallographic water molecules (red) in the binding pocket of BRD4-BD1 (orange). The size of the spheres indicate the extent of their conservation across 101 crystal structures. For perspective, a small molecule binder (PDB 3ZYU) is shown in blue. | |
Using WONKA, we can easily identify the ligands which displace the water molecules in the sites where they are not present. The only crystal structure with no water molecules at site 1 or 2 is PDB 6MH1 (Fig. 9). Water molecules at sites 3 and 4 are also displaced. Divakaran et al.67 acknowledge the reorganisation of the usually conserved water network and attribute it to the fluorophenyl group of the ligand. A moderate pIC50 value of 5.77 was measured for this compound. However, increased selectivity over other BET receptors was observed and it was hypothesised that this was in part due to the displacement of the water molecules. In our ensemble of crystal structures, which were analysed using WONKA, there are no other fluorine containing groups which occupy the same region of the binding site, which perhaps explains why sites 1, 2, 3 and 4 are occupied by water molecules for the majority of remaining complexes.
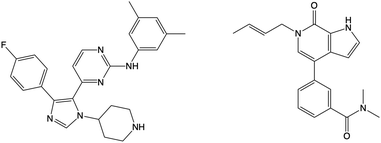 |
| Fig. 9 The structure of the compound that displaces crystallographic water molecules at sites 1–4 is shown on the left. The structure on the right displaces water molecules at sites 3 and 4. | |
Beside the structure previously discussed, there is one other crystal structure, PDB 5I88, which does not show water molecules at sites 3 and 4. The butenyl group on the ligand displaces the water network and induces a rearrangement. The addition of a butenyl group to the compound corresponds with a reduction in activity as the butenyl containing compound has a pIC50 of 6.43, while its equivalent without the butenyl group has a pIC50 of 7.04. Crawford et al.21 suggest that, while there may be multiple parameters that contribute to the decreased potency, the position of the active site water molecules may play a role.
Site 5 is occupied by a water molecule in all crystal structures, with the exception of PDB 4GPJ and 5DLZ. The displacement of this water molecule does not correlate with high activity ligands. Furthermore, there are ten crystal structures in our data set, which do not contain a water molecule at site 6. The corresponding ligands for these crystal structures have a pIC50 range of 5.30 to 7.30. The remaining water molecules depicted in Fig. 8 are present in ≤80% of the crystal structures (see ESI† for a full list of PDB codes and the presence of active site crystallographic water molecules in each). While we have not found a correlation between the displacement of specific water molecules in the network and the activity of the co-crystallised compounds, we have demonstrated the extent of their conservation. We expect this analysis to be a useful tool in selecting the best crystal structure and number of crystallographic water molecules to retain in computational studies of BRD4-BD1.
Molecular docking
To demonstrate the importance of the conserved binding site water network in modelling an experimentally accurate system, molecular docking was performed with and without the presence of the water network. Ligands from 101 crystal structures of BRD4-BD1 complexes were docked against structure PDB 4BJX. To compare the two setups, the RMSD values between the docked poses and the crystallographic poses were calculated. On average, the improvement in RMSD when including the water molecules was 1.52 Å (distributions of each data set can be found in the ESI†). Furthermore, 82% of the ligand poses were better predicted when including water molecules as part of the receptor. For example, Fig. 10 shows a large difference in bound conformation of one of the compounds. The docked pose has a RMSD of 0.21 Å when water molecules are included and 1.54 Å when docked without water molecules. Different functional groups occupying different regions of the active site, such as in this example, can lead to large differences in predicted activity when using more involved, but more accurate, methods such as free energy calculations. The accuracy of the binding poses when docking with the conserved water molecules indicates that these water molecules should be retained in computational studies of BRD4-BD1. Furthermore, this aids the design of new inhibitors. Compounds should be designed with these solvent interactions in mind, while all other crystallographic water molecules are likely to be able to be displaced.
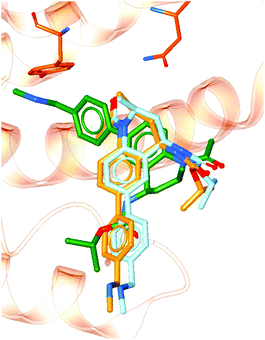 |
| Fig. 10 Docked poses with (light blue) and without (green) including crystallographic water molecules as part of the receptor. The crystallographic pose (orange) is also shown for comparison. | |
The receptor used in this study was the centroid of the ensemble of 297 PDB crystal structures of BRD4-BD1. Regardless of the inclusion of crystallographic water molecules as part of the receptor, all docked compounds show a good similarity to their original crystallographic conformations. This indicates that crystal structure 4BJX is a suitable starting conformation for the in silico study of BRD4-BD1.
Binding free energies
To further illustrate the impact of binding site water molecules, the free energy of binding was calculated for the two docked poses shown in Fig. 10. The orange structure is the co-crystallised binding conformation of the compound. The structure in light blue is the docked pose when including the active site water network and the green pose is the docked pose obtained without including the water molecules. The experimental binding free energy for the compound is −8.9 kcal mol−1.68 Absolute FEP resulted in a predicted binding free energy of −11.2 ± 0.6 kcal mol−1 for the light blue binding pose and a value of −1.5 ± 0.5 kcal mol−1 for the green pose. There are a number of factors that can affect the accuracy of FEP calculations, such as the quality of the small molecule force field parameters and the number of lambda windows used. However, the only difference in procedure between our two calculations was the inclusion of the active site water molecules in the generation and setup of the simulation starting conformations. The large difference between our predicted free energy values demonstrates the importance of starting structure in these types of calculations. Furthermore, the inclusion of water molecules resulted in a free energy of binding which is closer to experiment. This supports our conclusion that these water molecules are crucial for accurately modelling a BRD4 system.
4 Conclusions
There has been increasing interest in BRD4 as a therapeutic target, resulting in a large number of X-ray crystal structures of the receptor in complex with small molecule ligands. In this work, we review an ensemble of structures of BRD4-BD1 complexes in order to compare different conformations of the protein, without the need, for example, to carry out molecular dynamics simulations. By superimposing 297 crystal structures of BRD4-BD1 and calculating pairwise RMSD values, we have found a high level of similarity between the conformations, regardless of the bound ligand. Clustering algorithms identify PDB 4BJX as the centroid of the ensemble and clustering into five groups gave structures 5WA5, 4PS5, 5NNE, 5D0C and 5U28 as the representative structures of each cluster.
To achieve a more detailed view of the binding site, we used WONKA to compare the conformations of individual residues that are important for histone and small molecule binding. In this analysis the positions of Asn140, Tyr97, Met149, Trp81, Pro82 and Phe83 in 101 X-ray crystal structures were compared. With the exception of a handful of Trp81 conformations, the positions of these residues were extremely similar. This shows the size and shape of the BD1 cavity remains unchanged with different ligands bound, highlighting the importance of the chemical features needed in a potential inhibitor. A polar group at the head of the ligand is necessary to form both the interaction with Asn140 and a water mediated interaction with Tyr97. Simultaneously, a lipophilic group is needed to extend into the hydrophobic cavity of BD1 and strengthen ligand binding.
Water molecules also play an important role in BRD4 ligand binding. Therefore, we examined the conservation of crystallographic water molecules in the binding site. Analysis in WONKA showed that the majority of crystal structures contain the four or five water molecules generally considered important for ligand binding. In total, there are up to 11 water molecules within 8 Å of the bound ligands, which are largely conserved across the ensemble. While there have been previous studies on this highly conserved water network,21,22 ours is the first to consider such a high number of experimental structures. Our work demonstrates the extent of the conservation and, through molecular docking and absolute FEP calculations, highlights the importance of retaining binding site water molecules in computational studies. Through this examination of BRD4-BD1 crystal structures, we have provided a quantitative basis to facilitate the selection of structures in future computational studies.
Conflicts of interest
There are no conflicts to declare.
Acknowledgements
This work was supported by the Engineering and Physical Sciences Research Council (grant numbers EP/S035990/1, EP/R512059/1). We acknowledge PRACE for awarding us access (grant #2020235572) to the Marconi100 system of the CINECA supercomputing centre in Bologna, Italy. J. H. is supported by the Royal Academy of Engineering under the Chairs in Emerging Technologies scheme. We thank OpenEye Scientific Software, Inc. for providing an academic license for their software.
Notes and references
- C.-M. Chiang, F1000Prime Rep., 2009, 1, 1–7 Search PubMed.
- A. Dey, F. Chitsaz, A. Abbasi, T. Misteli and K. Ozato, Proc. Natl. Acad. Sci. U. S. A., 2003, 100, 8758–8763 CrossRef CAS.
- B. Padmanabhan, S. Mathur, R. Manjula and S. Tripathi, J. Biosci., 2016, 41, 295–311 CrossRef CAS.
- E. Korb, M. Herre, I. Zucker-Scharff, R. B. Darnell and C. D. Allis, Nat. Neurosci., 2015, 18, 1464–1473 CrossRef CAS.
- A. C. Belkina and G. V. Denis, Nat. Rev. Cancer, 2012, 12, 465–477 CrossRef CAS PubMed.
- P. Filippakopoulos, J. Qi, S. Picaud, Y. Shen, W. B. Smith, O. Fedorov, E. M. Morse, T. Keates, T. T. Hickman, I. Felletar, M. Philpott, S. Munro, M. R. McKeown, Y. Wang, A. L. Christie, N. West, M. J. Cameron, B. Schwartz, T. D. Heightman, N. La Thangue, C. A. French, O. Wiest, A. L. Kung, S. Knapp and J. E. Bradner, Nature, 2010, 468, 1067–1073 CrossRef CAS PubMed.
- L. F. Chen, Y. Mu and W. C. Greene, EMBO J., 2002, 21, 6539–6548 CrossRef CAS PubMed.
- S. Mujtaba, L. Zeng and M. M. Zhou, Oncogene, 2007, 26, 5521–5527 CrossRef CAS PubMed.
- P. Filippakopoulos, S. Picaud, M. Mangos, T. Keates, J. P. Lambert, D. Barsyte-Lovejoy, I. Felletar, R. Volkmer, S. Müller, T. Pawson, A. C. Gingras, C. H. Arrowsmith and S. Knapp, Cell, 2012, 149, 214–231 CrossRef CAS PubMed.
- M. Jung, M. Philpott, S. Müller, J. Schulze, V. Badock, U. Eberspächer, D. Moosmayer, B. Bader, N. Schmees, A. Fernández-Montalván and B. Haendler, J. Biol. Chem., 2014, 289, 9304–9319 CrossRef CAS PubMed.
- S. Picaud, C. Wells, I. Felletar, D. Brotherton, S. Martin, P. Savitsky, B. Diez-Dacal, M. Philpott, C. Bountra, H. Lingard, O. Fedorov, S. Müller, P. E. Brennan, S. Knapp and P. Filippakopoulos, Proc. Natl. Acad. Sci. U. S. A., 2013, 110, 19754–19759 CrossRef CAS PubMed.
- O. Mirguet, R. Gosmini, J. Toum, C. A. Clément, M. Barnathan, J. M. Brusq, J. E. Mordaunt, R. M. Grimes, M. Crowe, O. Pineau, M. Ajakane, A. Daugan, P. Jeffrey, L. Cutler, A. C. Haynes, N. N. Smithers, C. W. Chung, P. Bamborough, I. J. Uings, A. Lewis, J. Witherington, N. Parr, R. K. Prinjha and E. Nicodème, J. Med. Chem., 2013, 56, 7501–7515 CrossRef CAS PubMed.
- M. M. Coudé, T. Braun, J. Berrou, M. Dupont, S. Bertrand, A. Masse, E. Raffoux, R. Itzykson, M. Delord, M. E. Riveiro, P. Herait, A. Baruchel, H. Dombret and C. Gardin, Oncotarget, 2015, 6, 17698–17712 CrossRef PubMed.
- K. T. Siu, H. Eda, L. Santo, J. Ramachandran, M. Koulnis, J. Mertz, R. J. Sims, M. Cooper and N. S. Raje, Blood, 2015, 126, 4255 CrossRef.
- G. Shapiro, A. Dowlati, P. LoRusso, J. Eder, A. Anderson, K.Đ. M. Kagey, C. Sirard, J. Bradner and S. Landau, Mol. Cancer Ther., 2015, 14, A49 Search PubMed.
- A. Sarthy, L. Li, D. H. Albert, X. Lin, W. Scott, E. Faivre, M. H. Bui, X. Huang, D. M. Wilcox, T. Magoc, F. G. Buchanan, P. Tapang, G. S. Sheppard, L. Wang, S. D. Fidanze, J. Pratt, D. Liu, L. Hasvold, P. Hessler, T. Uziel, L. Lam, G. Rajaraman, G. Fang, S. W. Elmore, S. H. Rosenberg, K. McDaniel, W. Kati and Y. Shen, Cancer Res., 2016, 76, 4718 Search PubMed.
- T. Lu, W. Lu and C. Luo, Expert Opin. Ther. Pat., 2020, 30, 57–81 CrossRef CAS.
- Z. Liu, P. Wang, H. Chen, E. A. Wold, B. Tian, A. R. Brasier and J. Zhou, J. Med. Chem., 2017, 60, 4533–4558 CrossRef CAS PubMed.
- C. Barillari, J. Taylor, R. Viner and J. W. Essex, J. Am. Chem. Soc., 2007, 129, 2577–2587 CrossRef CAS PubMed.
- P. Filippakopoulos and S. Knapp, Nat. Rev. Drug Discovery, 2014, 13, 337–356 CrossRef CAS PubMed.
- T. D. Crawford, V. Tsui, E. M. Flynn, S. Wang, A. M. Taylor, A. Côté, J. E. Audia, M. H. Beresini, D. J. Burdick, R. Cummings, L. A. Dakin, M. Duplessis, A. C. Good, M. C. Hewitt, H. R. Huang, H. Jayaram, J. R. Kiefer, Y. Jiang, J. Murray, C. G. Nasveschuk, E. Pardo, F. Poy, F. A. Romero, Y. Tang, J. Wang, Z. Xu, L. E. Zawadzke, X. Zhu, B. K. Albrecht, S. R. Magnuson, S. Bellon and A. G. Cochran, J. Med. Chem., 2016, 59, 5391–5402 CrossRef CAS PubMed.
- H. Zhong, Z. Wang, X. Wang, H. Liu, D. Li, H. Liu, X. Yao and T. Hou, Phys. Chem. Chem. Phys., 2019, 21, 25276–25289 RSC.
- Z. Pan, X. Li, Y. Wang, Q. Jiang, L. Jiang, M. Zhang, N. Zhang, F. Wu, B. Liu and G. He, J. Med. Chem., 2020, 63, 3678–3700 CrossRef CAS PubMed.
- J. P. Hilton-Proctor, O. Ilyichova, Z. Zheng, I. G. Jennings, R. W. Johnstone, J. Shortt, S. J. Mountford, M. J. Scanlon and P. E. Thompson, Eur. J. Med. Chem., 2020, 191, 112120 CrossRef CAS PubMed.
- Z. Li, S. Xiao, Y. Yang, C. Chen, T. Lu, Z. Chen, H. Jiang, S. Chen, C. Luo and B. Zhou, J. Med. Chem., 2020, 63, 3956–3975 CrossRef CAS PubMed.
- F. Jiang, Q. Hu, Z. Zhang, H. Li, H. Li, D. Zhang, H. Li, Y. Ma, J. Xu, H. Chen, Y. Cui, Y. Zhi, Y. Zhang, J. Xu, J. Zhu, T. Lu and Y. Chen, J. Med. Chem., 2019, 62, 11080–11107 CrossRef CAS PubMed.
- C. R. Wellaway, D. Amans, P. Bamborough, H. Barnett, R. A. Bit, J. A. Brown, N. R. Carlson, C. W. Chung, A. W. Cooper, P. D. Craggs, R. P. Davis, T. W. Dean, J. P. Evans, L. Gordon, I. L. Harada, D. J. Hirst, P. G. Humphreys, K. L. Jones, A. J. Lewis, M. J. Lindon, D. Lugo, M. Mahmood, S. McCleary, P. Medeiros, D. J. Mitchell, M. O'Sullivan, A. Le Gall, V. K. Patel, C. Patten, D. L. Poole, R. R. Shah, J. E. Smith, K. A. Stafford, P. J. Thomas, M. Vimal, I. D. Wall, R. J. Watson, N. Wellaway, G. Yao and R. K. Prinjha, J. Med. Chem., 2020, 63, 714–746 CrossRef CAS PubMed.
- D. Liang, Y. Yu and Z. Ma, Eur. J. Med. Chem., 2020, 200, 112426 CrossRef CAS PubMed.
- O. Gilan, I. Rioja, K. Knezevic, M. J. Bell, M. M. Yeung, N. R. Harker, E. Y. Lam, C. Chung, P. Bamborough, M. Petretich, M. Urh, S. J. Atkinson, A. K. Bassil, E. J. Roberts, D. Vassiliadis, M. L. Burr, A. G. Preston, C. Wellaway, T. Werner, J. R. Gray, A. M. Michon, T. Gobbetti, V. Kumar, P. E. Soden, A. Haynes, J. Vappiani, D. F. Tough, S. Taylor, S. J. Dawson, M. Bantscheff, M. Lindon, G. Drewes, E. H. Demont, D. L. Daniels, P. Grandi, R. K. Prinjha and M. A. Dawson, Science, 2020, 368, 387–394 CrossRef CAS PubMed.
- R. J. Watson, P. Bamborough, H. Barnett, C. W. Chung, R. Davis, L. Gordon, P. Grandi, M. Petretich, A. Phillipou, R. K. Prinjha, I. Rioja, P. Soden, T. Werner and E. H. Demont, J. Med. Chem., 2020, 63, 9045–9069 CrossRef CAS PubMed.
- A. Speck-Planche and M. T. Scotti, Mol. Diversity, 2019, 23, 555–572 CrossRef CAS PubMed.
- S. Postel-Vinay, K. Herbschleb, C. Massard, V. Woodcock, J.-C. Soria, A. O. Walter, F. Ewerton, M. Poelman, N. Benson, M. Ocker, G. Wilkinson and M. Middleton, Eur. J. Cancer, 2019, 109, 103–110 CrossRef CAS PubMed.
- M. Pervaiz, P. Mishra and S. Günther, Chem. Rec., 2018, 18, 1808–1817 CrossRef CAS PubMed.
- B. K. Allen, S. Mehta, S. W. Ember, J. Y. Zhu, E. Schönbrunn, N. G. Ayad and S. C. Schürer, ACS Omega, 2017, 2, 4760–4771 CrossRef CAS PubMed.
- A. Tahir, R. D. Alharthy, S. Naseem, N. Mahmood, M. Ahmed, K. Shahzad, M. N. Akhtar, A. Hameed, I. Sadiq, H. Nawaz and M. Muddassar, Molecules, 2018, 23, 1–16 CrossRef.
- J. Su, X. Liu, S. Zhang, F. Yan, Q. Zhang and J. Chen, Chem. Biol. Drug Des., 2018, 91, 828–840 CrossRef CAS PubMed.
- J. Su, X. Liu, S. Zhang, F. Yan, Q. Zhang and J. Chen, Chem. Biol. Drug Des., 2019, 93, 163–176 CrossRef CAS PubMed.
- J. J. Irwin and B. K. Shoichet, J. Chem. Inf. Model., 2005, 45, 177–182 CrossRef CAS PubMed.
- L. Fusani and A. C. Cabrera, J. Comput.-Aided Mol. Des., 2019, 33, 287–294 CrossRef CAS PubMed.
-
S. E. Nichols, R. Baron and J. A. McCammon, in On the Use of Molecular Dynamics Receptor Conformations for Virtual Screening, ed. R. Baron, Springer New York, New York, NY, 2012, pp. 93–103 Search PubMed.
- M. Suruzhon, M. S. Bodnarchuk, A. Ciancetta, R. Viner, I. D. Wall and J. W. Essex, J. Chem. Theory Comput., 2021, 17, 1806–1821 CrossRef CAS PubMed.
- N. M. Lim, L. Wang, R. Abel and D. L. Mobley, J. Chem. Theory Comput., 2016, 12, 4620–4631 CrossRef CAS PubMed.
- A. R. Bradley, I. D. Wall, F. Von Delft, D. V. Green, C. M. Deane and B. D. Marsden, J. Comput.-Aided Mol. Des., 2015, 29, 963–973 CrossRef CAS PubMed.
- C. M. Deane, I. D. Wall, D. V. Green, B. D. Marsden and A. R. Bradley, Acta Crystallogr., Sect. D: Struct. Biol., 2017, 73, 279–285 CrossRef CAS PubMed.
- Schrödinger, LLC.
- F. Sievers, A. Wilm, D. Dineen, T. J. Gibson, K. Karplus, W. Li, R. Lopez, H. McWilliam, M. Remmert, J. Söding, J. D. Thompson and D. G. Higgins, Mol. Syst. Biol., 2011, 7, 1–6 CrossRef.
- E. F. Pettersen, T. D. Goddard, C. C. Huang, G. S. Couch, D. M. Greenblatt, E. C. Meng and T. E. Ferrin, J. Comput. Chem., 2004, 25, 1605–1612 CrossRef CAS.
- M. Jamroz and A. Kolinski, BMC Bioinf., 2013, 14, 62 CrossRef CAS PubMed.
-
J. MacQueen, Proc. Fifth Berkeley Symp. on Math. Statist. and Prob., 1967, 1, 281–297.
- T. Sander, J. Freyss, M. Von Korff and C. Rufener, J. Chem. Inf. Model., 2015, 55, 460–473 CrossRef CAS PubMed.
- M. McGann, J. Chem. Inf. Model., 2011, 51, 578–596 CrossRef CAS PubMed.
- P. C. Hawkins, A. G. Skillman, G. L. Warren, B. A. Ellingson and M. T. Stahl, J. Chem. Inf. Model., 2010, 50, 572–584 CrossRef CAS PubMed.
- T. A. Halgren, J. Comput. Chem., 1999, 20, 720–729 CrossRef CAS.
- H. J. C. Berendsen, D. van der Spoel and R. van Drunen, Comput. Phys. Commun., 1995, 91, 43–56 CrossRef CAS.
- J. Huang, S. Rauscher, G. Nawrocki, T. Ran, M. Feig, B. L. De Groot, H. Grubmüller and A. D. MacKerell, Nat. Methods, 2016, 14, 71–73 CrossRef PubMed.
- K. Vanommeslaeghe, E. Hatcher, C. Acharya, S. Kundu, S. Zhong, J. Shim, E. Darian, O. Guvench, P. Lopes, I. Vorobyov and A. D. MacKerell, J. Comput. Chem., 2010, 31, 671–690 CAS.
- W. L. Jorgensen, J. Chandrasekhar, J. D. Madura, R. W. Impey and M. L. Klein, J. Chem. Phys., 1983, 79, 926–935 CrossRef CAS.
- C. H. Bennett, J. Comput. Phys., 1976, 22, 245–268 CrossRef.
- T. Kalliokoski, C. Kramer, A. Vulpetti and P. Gedeck, PLoS One, 2013, 8, 1–16 Search PubMed.
- J. P. Hilton-Proctor, O. Ilyichova, Z. Zheng, I. G. Jennings, R. W. Johnstone, J. Shortt, S. J. Mountford, M. J. Scanlon and P. E. Thompson, Bioorg. Med. Chem., 2019, 27, 115157 CrossRef CAS PubMed.
- E. Watts, D. Heidenreich, E. Tucker, M. Raab, K. Strebhardt, L. Chesler, S. Knapp, B. Bellenie and S. Hoelder, J. Med. Chem., 2019, 62, 2618–2637 CrossRef CAS PubMed.
- L. Fusani, I. Wall, D. Palmer and A. Cortes, Bioinformatics, 2018, 34, 1947–1948 CrossRef CAS PubMed.
- R. Abel, T. Young, R. Farid, B. J. Berne and R. A. Friesner, J. Am. Chem. Soc., 2008, 130, 2817–2831 CrossRef CAS PubMed.
- C. N. Nguyen, T. Kurtzman Young and M. K. Gilson, J. Chem. Phys., 2012, 137, 973–980 Search PubMed.
- D. Beglov and B. Roux, J. Phys. Chem. B, 1997, 101, 7821–7826 CrossRef CAS.
- Z. Cournia, B. Allen and W. Sherman, J. Chem. Inf. Model., 2017, 57, 2911–2937 CrossRef CAS.
- A. Divakaran, S. K. Talluri, A. M. Ayoub, N. K. Mishra, H. Cui, J. C. Widen, N. Berndt, J. Y. Zhu, A. S. Carlson, J. J. Topczewski, E. K. Schonbrunn, D. A. Harki and W. C. Pomerantz, J. Med. Chem., 2018, 61, 9316–9334 CrossRef CAS PubMed.
- S. J. Atkinson, P. E. Soden, D. C. Angell, M. Bantscheff, C. W. Chung, K. A. Giblin, N. Smithers, R. C. Furze, L. Gordon, G. Drewes, I. Rioja, J. Witherington, N. J. Parr and R. K. Prinjha, MedChemComm, 2014, 5, 342–351 RSC.
Footnote |
† Electronic supplementary information (ESI) available: Full list of PDB codes of X-ray crystal structures of BRD4-BD1; details of active site crystallographic water molecules in each PDB used in this study; input scripts for the molecular docking experiments and absolute free energy calculations; RMSD distributions from the docking analysis. See DOI: 10.1039/d1ob00658d |
|
This journal is © The Royal Society of Chemistry 2021 |
Click here to see how this site uses Cookies. View our privacy policy here.