DOI:
10.1039/D0NP00029A
(Viewpoint)
Nat. Prod. Rep., 2021,
38, 292-300
The need to innovate sample collection and library generation in microbial drug discovery: a focus on academia
Received
30th May 2020
First published on 24th July 2020
Abstract
The question of whether culturable microorganisms will continue to be a viable source of new drug leads is inherently married to the strategies used to collect samples from the environment, the methods used to cultivate microorganisms from these samples, and the processes used to create microbial libraries. An academic microbial natural products (NP) drug discovery program with the latest innovative chromatographic and spectroscopic technology, high-throughput capacity, and bioassays will remain at the mercy of the quality of its microorganism source library. This viewpoint will discuss limitations of sample collection and microbial strain library generation practices. Additionally, it will offer suggestions to innovate these areas, particularly through the targeted cultivation of several understudied bacterial phyla and the untargeted use of mass spectrometry and bioinformatics to generate diverse microbial libraries. Such innovations have potential to impact downstream therapeutic discovery, and make its front end more informed, efficient, and less reliant on serendipity. This viewpoint is not intended to be a comprehensive review of contributing literature and was written with a focus on bacteria. Strategies to discover NPs from microbial libraries, including a variety of genomics and “OSMAC” style approaches, are considered downstream of sample collection and library creation, and thus are out of the scope of this viewpoint.
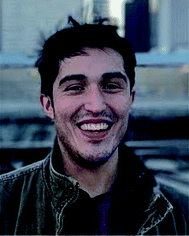 Antonio Hernandez | Antonio Hernandez received his Bachelor of Science in Biological Sciences in 2017 from the University of Illinois at Chicago (UIC). During his undergraduate time at UIC he was an undergraduate researcher in the Murphy Lab where he received formal training in microbial isolation and cultivation. Antonio was awarded the summer undergraduate research fellowship (SURF) at UIC and this quickly led to a heavy interest in academia and graduate school. Antonio is now an NIH fellow and PhD student in the Department of Pharmaceutical Sciences at UIC. His research revolves around antibiotic discovery from freshwater sponge microbiomes. |
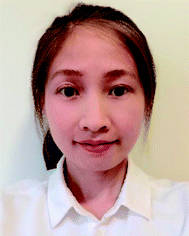 Linh T. Nguyen | Linh Thuy Nguyen received her PhD in Medicinal Chemistry from the Faculty of Pharmacy at Paris-Sud University in 2017. She is currently an NIH postdoctoral fellow and trainee of an NIH D43 Global Infectious Disease Research Training Program between the Institute of Marine Biochemistry at the Vietnam Academy of Science and Technology in Vietnam and the University of Illinois at Chicago in the United States. Her research interests include the discovery of bioactive natural products from plants, microorganisms and the synthesis/semi-synthesis of their derivatives. |
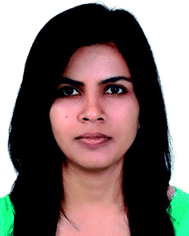 Radhika Dhakal | Radhika Dhakal obtained her BS and MS degrees in plant biology from Tribhuvan University, Nepal. She was awarded her Ph.D. at Yeungnam University, South Korea, where she studied the roles of genes in the process of tomato ripening in response to different wavelengths of light. Later, she carried out her post-doctoral research at the University of Illinois at Chicago and focused her research on generating diverse microbial libraries from different environmental samples for drug discovery using MALDI-TOF MS and IDBac analysis. She also earned entrepreneurship training from the NSF I-corps program. |
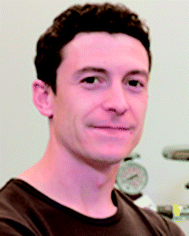 Brian T. Murphy | Brian T. Murphy is an Associate Professor in Pharmaceutical Sciences at the University of Illinois at Chicago. He earned BS and MS degrees in chemistry from the University of Massachusetts Dartmouth, a PhD in chemistry from Virginia Tech, and did postdoctoral training at the University of California San Diego. Brian runs a microbial antibiotic discovery program that seeks to both discover new therapies and develop innovative methods that make the discovery process more efficient. His lab is committed to weekly science partnership with local Chicago community centers, and combating systemic inequities that women and underrepresented communities encounter in science. |
1 The front end of microbial natural product (NP) drug discovery requires innovation
Libraries of culturable environmental microorganisms have been a major source of drugs this past century. To date, this cultivated population has provided nearly all of the clinical therapeutics that are derived from microorganisms.1,2 Most of these discoveries came from industry, government, and academic research programs that employed some form of the process depicted in Fig. 1.3,4 In the time since our Golden Age of antibiotic discovery, many stages of this process have undergone a renaissance in method innovation: chromatographic separation and compound detection, dereplication and structure characterization, biosynthetic gene cluster detection and expression, bioinformatic-driven NP discovery, and biological activity screening. Despite these advances, the philosophy we use to create microbial strain libraries from the environment (the front end, arguably the most important steps) has undergone relatively few widely applied conceptual changes since the 1940s.
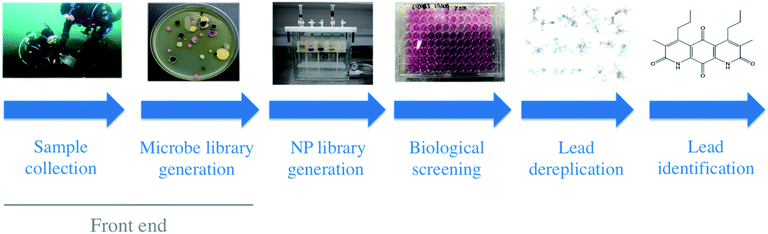 |
| Fig. 1 Earth's culturable microbiome has been the largest source of antibiotics used in the clinic. Above is a natural product discovery pipeline that has been commonly employed when sourcing culturable microorganisms for drug-leads. Herein the “front end” is defined as the collection of samples from the environment, and the creation of a library of cultured microorganisms from those samples (SCUBA photo credit: Erlendur Bogason). | |
2 Sample collection: an exercise in serendipity
After studies on Pyocyanase, and discoveries of tyrothricin and penicillin, the potential of microorganism-based antibiotic discovery was realized, and an unprecedented global effort was undertaken to amass large collections of microorganisms from the environment.5–7 Though it's reductive to assign a single unifying philosophy to such a global effort, generally speaking, collection expeditions aimed at isolating microorganisms have been guided by the following mantra: environments in diverse geographic locations contain different evolutionary pressures, which result in minimally-overlapping populations of endemic microorganisms, and thus the presence of divergent NP biosynthetic pathways (i.e., increased potential to isolate new microbial NPs). This is a logical approach, however in contrast to other steps in the Fig. 1 pipeline that have evolved considerably this past century, current collection expeditions have seldom expanded on this philosophy, which is reliant on a high degree of serendipity. For example, when performing untargeted field expeditions, the number of samples needed to collect the optimum microbial and NP diversity for discovery efforts is unclear. Further, few attempts have been made to define the available NP chemical space in the environment.8 Researchers have been limited to, generously speaking, educated guesses as to the extent to which geographic locations should be sampled. Looking forward, it is necessary to define the available microbial and NP diversity in collection regions from as large as several kilometers, to areas as small as one cm3 of sediment, and understand dynamics of NP populations over time. This is among the most understudied topics in our field.
The aforementioned are all important considerations for discovery programs with limited resources. It is becoming increasingly difficult for academic researchers to fund untargeted collection expeditions using federal dollars, which can cost upwards to $10
000 USD and require 1–2 years of meticulous planning. One strategy is to crowdsource sample collection through citizen science campaigns.9 Another successful but underutilized collection strategy has been to undertake a targeted, ecology-based approach to microbial NP discovery. The merging of microbial and chemical ecology to discover niche-specific NPs, which has been advocated for previously, has been a successful driver of NP discovery.10–13 Compared to what is known, researchers have just begun to map the cues that govern the vast array of inter-and intra-species relationships in the environment, and recent studies that focus on the human microbiome highlight just one area of particular promise.14,15
In regard to untargeted sample collections, three fundamental questions that can inform collection strategies are: (1) How are NP biosynthetic genes distributed in the environment?; (2) How are NP biosynthetic genes distributed across taxa?; (3) Are there patterns to this distribution that researchers can use to inform collection expeditions? These are grand challenges that face our field. Relatively few bodies of work have attempted to address these, despite their potential to make front end discovery more efficient. For example, a series of studies laid a foundation toward documenting the occurrence of NP biosynthetic gene clusters across large geographic regions.16–23 However, analysis of NP distribution patterns across environmental scales that are representative of single collection expeditions could facilitate more accurate predictions of the extent to which particular environments should be sampled in order to efficiently access microbial taxonomic and NP diversity (e.g., across sediment of individual lakes; across distances in sediment as small as a few cm; inter- and intra-NP distribution across insect, sponge, plant taxa; etc.). Similarly, it is necessary to better understand microbial genus/species patterns of NP occurrence in order to estimate the depth to which we should attempt to collect, target, and explore individual taxa. Advances have been made toward these ends, particularly in the last decade; we cite a few examples here.24–27 Generally speaking, understanding NP biosynthetic gene distribution is still woefully understudied and remains a major roadblock toward overcoming the degree of guesswork on which researchers rely when collecting samples from the environment.
3 Cultivation of microorganisms: many understudied phyla harbor isolates with large genome sizes and are culturable
The use of agar as a solid support for nutrient media was one of the most important developments in microbiology.28 In nearly 150 years of studies since, researchers have exploited several strategies to access culturable bacterial taxa from the environment including: use of diverse carbon and nitrogen sources; manipulation of photo-/chemotrophic, anaerobic, oligotrophic, halophilic, psychrophilic, and other conditions; addition of growth factors and antibiotics to nutrient media; among many other strategies.29,30 Despite these efforts, the majority of bacteria that have been cultivated to date can be classified into just 4 of greater than 100 known phyla (Actinobacteria, Proteobacteria, Firmicutes, and Bacteroidetes).29,31 NP discovery efforts have focused almost exclusively on genera within these and one additional phylum, Cyanobacteria.32,33 To emphasize this point, of the 9703 bacterial metabolites compiled in NPAtlas, a database of microbially produced NP structures, these 5 phyla were the source of nearly the entire group (Actinobacteria, 63.68%; Proteobacteria, 15.58%; Firmicutes, 5.31%; Bacteroidetes, 1.35%; Cyanobacteria, 11.23%; other, 2.85%).34 A similar trend was observed within the 1248 bacterial NP biosynthetic gene clusters (BGCs) deposited in the repository MIBiG to date (Actinobacteria, 67.95%; Proteobacteria, 17.23%; Firmicutes, 12.58%; Bacteroidetes, 0.88%; Cyanobacteria, 0.72%; other, 0.64%).35 This leaves a large expanse of taxonomic space that has seldom been explored for its NP-producing potential. Although not all of the greater than 100 additional phyla may be prolific sources of new NPs, what strategies can our field apply to best navigate toward the promising ones?
A few recent studies established the genome taxonomy database (GTDB), a collection of Bacteria and Archaea genomes sourced from both cultivation-dependent and -independent data.31,36 The database is comprised of 143
512 bacterial genomes spanning 112 phyla. Interestingly, although the 5 phyla previously discussed make up 90.64% of the total entries in the database, an additional 8 phyla (6 established and 2 proposed) harbor representatives in the database with a genome size >8 Mbp (Table 1). Among these, researchers have been able to obtain culturable isolates from 6 of the 8 phyla, though the extent of cultivability within each phylum remains to be determined and is worthy of study. Further, we found that as genome size increases from 8–9 Mbp, 9–10 Mbp, and >10 Mbp, 5 of the 8 understudied phyla contain a representative genome listed in at least two of the three size categories. Myxococcota aside (it was recently proposed to re-classify this group that has traditionally been categorized as Myxobacteria, which are relatively well-studied for their NP-producing potential),37–39 only a small number of NPs or associated BGCs have been identified from these phyla; a few are listed here.40–46 If the assumption that both large genome sizes and understudied taxa represent increased potential to produce new NP scaffolds, this indicates that these 8 phyla (nearly double the number of phyla that have been at the focus of our field since the 1940s) may contain genera that are prolific NP producers, and this potential remains largely unrealized and ripe for targeted discovery efforts. Assessment of the frequency of occurrence of large genome representatives within each of the phyla would help to further prioritize the list.
Table 1 Entries of understudied phyla in GTDB that show promising NP potential31
Genome size |
Phylum |
No. of GTDB entries |
Cultured representatives?a |
At least one cultured representative exists within the phylum.
Formerly Proteobacteria (class: Deltaproteobacteria) within a relatively well-studied group known as Myxobacteria, suggested as new phylum.36
Denotes candidate phylum.
|
>10.0 Mbp |
Total
|
304
|
— |
Actinobacteria |
208 |
Yes |
Proteobacteria |
39 |
Yes |
Firmicutes |
1 |
Yes |
Bacteroidetes |
4 |
Yes |
Cyanobacteria |
11 |
Yes |
Acidobacteria |
1 |
Yes |
Chloroflexi |
1 |
Yes |
Myxococcotab |
33 |
Yes |
Planctomycetes |
4 |
Yes |
Verrucomicrobia |
2 |
Yes |
9.00–9.99 Mbp |
Total
|
447
|
— |
Actinobacteria |
296 |
Yes |
Proteobacteria |
89 |
Yes |
Firmicutes |
3 |
Yes |
Bacteroidetes |
6 |
Yes |
Cyanobacteria |
26 |
Yes |
Acidobacteria |
3 |
Yes |
Chloroflexi |
1 |
Yes |
Myxococcotab |
15 |
Yes |
Planctomycetes |
7 |
Yes |
Tectomicrobia |
1 |
Not to date |
8.00–8.99 Mbp |
Total
|
1101
|
— |
Actinobacteria |
500 |
Yes |
Proteobacteria |
474 |
Yes |
Firmicutes |
21 |
Yes |
Bacteroidetes |
33 |
Yes |
Cyanobacteria |
32 |
Yes |
Acidobacteria |
15 |
Yes |
Chloroflexi |
2 |
Yes |
Myxococcotab |
8 |
Yes |
Planctomycetes |
9 |
Yes |
Verrucomicrobia |
4 |
Yes |
Armatimonadetes |
2 |
Yes |
Moduliflexotac |
1 |
Not to date |
Though relatively few NP discovery efforts have focused on cultivating understudied taxa, it has been well established that this approach shows much promise to produce new NPs47–49 (studies often refer to these as “rare” taxa, though we advise the field to adopt the more precise nomenclature “understudied”). Successful strategies have included targeting understudied bacterial types45,50–52 and employing novel cultivation technologies.53–57 But in the absence of innovative cultivation methods, more traditional techniques may still show promise as a route to new NP scaffolds. A common sentiment is that standard cultivation methods regularly afford bacteria that produce known NPs, but there is a marked absence of studies that document the potential of the full culturable microbiome to afford new structural scaffolds. Work found in Elfeki et al. showed that readily culturable environmental bacteria from lake sediment (grown on five common laboratory nutrient media) have strong potential to produce new NPs.58 This is contradictory to current practice that consistently exhibits a high scaffold re-discovery rate, so it was opined that bias colony picking practices, and as a consequence lack of microbial diversity, have selected for a redundancy of taxa in some libraries sourced for discovery over several decades. Though this remains speculative, it was supported by Costa et al. upon the isolation of 16 understudied actinomycete genera (among them a total of 11 publications that report a NP) that were readily observed and isolated from nutrient agar by hand, using common cultivation conditions and untargeted colony picking practices.59 Importantly, all of these colonies exhibited morphology that was atypical of what is commonly described for actinomycete bacteria, and likely have been overlooked by traditional, phenotypic colony profiling practices. Therefore, we strongly recommend that the field adopt untargeted colony picking coupled to high-throughput methods for discriminating those colonies (described in next section) to ensure that maximum NP chemical space with minimum overlap is incorporated into a library from a given environmental sample.
Of course, focusing on the isolation of representatives of understudied taxa does not preclude the continued study for new NP scaffolds from common culturable genera such as Streptomyces or Bacillus, however innovative techniques should be more widely employed to explore the full biosynthetic capacity of these microorganisms. These practices typically occur after the creation of a microbial library and as a result are beyond the scope of this viewpoint, though examples of these include activating silent NP pathways using elicitors and transcriptional regulators,60–62 using heterologous expression systems,63 CRISPR-Cas9 genome engineering,64,65 resistance gene-guided discovery approaches,66–68 and single cell analyses.69
4 Microbial library generation: large libraries are not necessarily useful. Diverse libraries are needed
Perspectives from researchers at Wyeth, Novartis, Pfizer, Merck, Cetek, and Fundacion Medina have provided insight to front end NP discovery strategies employed by the pharmaceutical industry, in particular the generation and use of microbial libraries.3,70–74 In the context of antibiotic discovery, massive libraries of microorganisms and associated NP fractions were screened in high-throughput bioassays, and after many successes over several decades, this pipeline was largely abandoned by pharma due to diminishing returns on investment, a shift toward combinatorial chemistry approaches, limitations of target-based assays, and the rediscovery of known compounds.3,73,75–78 In regard to the latter, a major cause of this was strain and NP redundancy in these libraries, which resulted from a few practices: (1) strain libraries were often created based on common morphological phenotypes or 16S rRNA gene sequencing, which do not fully account for NP production;72,74,78,79 (2) bacteria isolation efforts focused almost exclusively on the spore-forming taxa Actinobacteria and Firmicutes,4,70,80 and as a consequence these taxa have yielded the highest number of biologically active bacterial NPs discovered to date. To overcome these obstacles, companies Naicons and Medina are two notable examples of large-scale industrial efforts to diversify the microbial taxa present in a library.81 Unfortunately, academic researchers often lack resources to build large, diverse libraries.
Contrary to the dogma that large libraries are needed to discover new therapeutic leads, smaller, more diverse strain libraries have the potential make NP discovery more accessible to those with fewer resources, such as academic researchers at smaller institutions. Until just recently, costs associated with personnel, infrastructure, and supplies can quickly reach hundreds of thousands of dollars in order to create and maintain a microbial and NP fraction library of appreciable size. Further, heavy demands on young academic researchers (Assistant Professor, postdoctoral researcher, graduate student) to publish preclude most from daring to invest the resources and time to create a strain and NP fraction library as was done in previous decades, which requires years of investment with no obvious payback. Conversely, a streamlined library results in fewer isolates that have to be grown, extracted, screened, dereplicated, and selected for larger scale compound isolation studies. This effectively lessens the burden on downstream steps in the discovery process, and allows a program to operate under a smaller budget.81
To significantly reduce these costs and mitigate the risks to which young researchers are rightfully averse, recent efforts have been made to maximize the amount of information that can be obtained from a single bacterial colony in the context of library creation. Clark et al. designed a matrix-assisted laser desorption/ionization time-of-flight mass spectrometry (MALDI-TOF MS) workflow to extract protein and NP MS data from unidentified bacterial colonies derived from environmental samples in high-throughput.82 Using the open-access software IDBac, hierarchical clustering was used to organize isolates by pseudo-taxonomic groupings based on protein MS profiles, then these subgroups were further distinguished based on their degree of overlap within corresponding NP spectra.59,82,83 Although the workflow shares limitations inherent in most MS methodologies (as discussed in the original publication), it is fast and accessible for non-MS experts in academia. Sample preparation and data analysis of 384 colonies can occur in fewer than 4 h and provides a more cost-effective way to generate libraries with diverse taxa and minimal NP redundancy. Information obtained will not be comprehensive, but the workflow represents a significant improvement to current practice.
Using methods reported in Costa et al.,59 IDBac was used to assess the degree of NP overlap in our bacterial strain collection amassed over ten years of field expeditions. This collection was created based on manual selection of colonies from multiple types of nutrient agar, considering only phenotypic traits. This work has historically been done by industrial microbiology experts, trained to identify specific taxa on a plate. We hypothesized that biased colony picking practices based solely on morphology or target taxa resulted in a significant degree of redundancy in this library. Cellular material from each isolate was analyzed using the MALDI/IDBac pipeline. Fig. 2 shows the metabolite association network (MAN) analysis of one subgroup in the library – a group of Micromonospora strains that are all classified as the same species. Visualization of these data allowed for the estimation that a similar chemical space can be covered using just 2 of the 8 entries in the library. In total, the size of the library was reduced from 697 to 381 strains, after removing those that exhibited significant NP overlap. Importantly, the streamlined library is able to cover similar chemical space and was reduced in size by 45.3%. We view this to be a large reduction in redundancy, particularly given that the library spans 14 distinct geographic regions. We previously reported reductions of up to 72% (833 to 233 isolates).59 Of course, this analysis would achieve greater accuracy as isolates are profiled on additional media types, since the chemical space pertaining to differentially regulated NP biosynthetic genes would be included. This can be accomplished through use of multiwell plates that contain diverse nutrient media, which is quite amenable to MALDI-TOF MS sample prep and analysis.
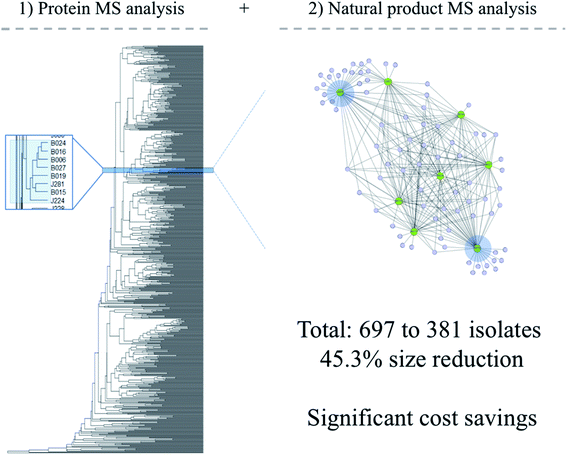 |
| Fig. 2 (Left) Dendrogram depicting 697 bacterial isolates that made up our in-house library, grouped by similarity of protein spectra; (right) large nodes represent cellular material scraped from a bacterial colony, and smaller nodes represent m/z values from the resulting mass spectrum. The two blue highlights indicate isolates chosen to be retained in the new, streamlined library. | |
In an academic setting, reduction by half or more is a significant achievement, particularly if a program aims to create extract or NP fraction libraries. Although in some cases researchers are not limited by the number of samples entering into a downstream biological screen, they do face a barrier to effectively dereplicate and/or pursue the number of bioassay hits that contain overlapping NP space. Reducing the number of required NMR, HPLC-, or UPLC-MS/MS runs and subsequent data analyses by half would save appreciable time and money. After employment of innovative NP dereplication tools such as Global Natural Products Social molecular networking (GNPS),84,85 downstream bioassay-guided fractionation (BGF) will remain the most effective way to discover a bioactive NP.
The high-throughput nature of MALDI-TOF MS/IDBac analysis and ease by which libraries can be grown in multiwell agar plates makes this pipeline a viable option for both academia (where smaller libraries can be created by trained undergraduate researchers) and industry (where robotic colony pickers can be employed to create larger libraries). Since the process requires single colonies of bacteria, stacks of multiwell agar plates, and few reagents, the associated costs are minimal compared to the effort required and the information received as a result. Once a microbial library has been created, extensive metabolomic surveys26 and comparative genomics/genome mining are effective tools to discover new NPs; these topics have been covered extensively, and a few studies are mentioned here.45,49,86–89 Finally, several steps surrounding colony-based discovery are amenable to automation including plating environmental samples on nutrient agar, colony picking and transfer, application of colonies onto MALDI target plates,90 liquid handling for cryostorage, and biological screening of colonies. Given the current availability of online tools and funding mechanisms to purchase instrumentation, an automated pipeline that escorts hundreds to thousands of colonies from environmental isolation plates to bioassay screening is not as out of reach to academic researchers as it once used to be. This ‘drug-lead discovery from a bacterial colony’ approach stands as an achievable goal for microbial NP discovery programs in academia that wish to employ a competitive, untargeted discovery strategy.
5 Conclusion
The era of drug lead discovery from culturable bacteria is not nearing its end, however its success relies on researchers' ability to innovate strategies used to collect samples from the environment, methods used to cultivate bacteria, and processes used to create microbial libraries. Particularly in the past decade, innovative methods have been developed to prioritize microbial isolates in existing libraries (list not comprehensive).91–96 Coupling these efforts with strong front-end dereplication techniques that take microbial taxa and NP production into account, will achieve low-redundancy libraries. As a result this will ensure that diverse, minimally-overlapping NP populations are input into downstream library mining efforts. The resulting cost and time savings make untargeted microbial NP discovery in academia much more accessible, particularly to new research programs. Further, a NP discovery program that does not have industry-scale resources at its disposal may also be successful by targeting specific understudied taxa either by selective cultivation techniques or by targeted MS-based interrogation of isolates from environmental diversity plates. Either of these efforts are poised to have an impact on downstream drug-lead discovery and development efforts, and may level the playing field for smaller academic labs to discover novel biologically active leads.
6 Conflicts of interest
There are no conflicts to declare.
7 Acknowledgements
Research work of the authors was supported by the National Institute of General Medical Sciences of the National Institutes of Health under Award Number R01GM125943 and R01GM125943-02S1, and the National Institute of Allergy and Infectious Diseases/Fogarty International Center under Award Number D43TW010530. The content is solely the responsibility of the authors and does not necessarily represent the official views of the National Institutes of Health. The authors would also like to acknowledge Professor William Fenical of the Scripps Institution of Oceanography, University of California San Diego for helpful discussions.
8 References
- W. Wohlleben, Y. Mast, E. Stegmann and N. Ziemert, Microbial Biotechnology, 2016, 9, 541–548 CrossRef
.
- G. Pishchany, Curr. Opin. Microbiol., 2020, 57, 7–12 CrossRef CAS
.
- F. E. Koehn and G. T. Carter, Nat. Rev. Drug Discovery, 2005, 4, 206–220 CrossRef CAS
.
- L. Katz and R. H. Baltz, J. Ind. Microbiol. Biotechnol., 2016, 43, 155–176 CrossRef CAS
.
- A. T. Bull, M. Goodfellow and J. H. Slater, Annu. Rev. Microbiol., 1992, 46, 219–252 CrossRef CAS
.
- A. T. Bull, J. E. Stach, A. C. Ward and M. Goodfellow, Antonie van Leeuwenhoek, 2005, 87, 65–79 CrossRef
.
- R. I. Aminov, Front. Microbiol., 2010, 1, 134 Search PubMed
.
- C. R. Pye, M. J. Bertin, R. S. Lokey, W. H. Gerwick and R. G. Linington, Proc. Natl. Acad. Sci. U. S. A., 2017, 114, 5601–5606 CrossRef CAS
.
- L. Du, A. J. Robles, J. B. King, D. R. Powell, A. N. Miller, S. L. Mooberry and R. H. Cichewicz, Angew. Chem., Int. Ed., 2014, 53, 804–809 CrossRef CAS
.
- R. Schmidt, D. Ulanova, L. Y. Wick, H. B. Bode and P. Garbeva, ISME J., 2019, 13, 2656–2663 CrossRef
.
- N. J. Tobias, H. Wolff, B. Djahanschiri, F. Grundmann, M. Kronenwerth, Y. M. Shi, S. Simonyi, P. Grun, D. Shapiro-Ilan, S. J. Pidot, T. P. Stinear, I. Ebersberger and H. B. Bode, Nat. Microbiol., 2017, 2, 1676–1685 CrossRef CAS
.
- D. C. Oh, M. Poulsen, C. R. Currie and J. Clardy, Nat. Chem. Biol., 2009, 5, 391–393 CrossRef CAS
.
- D. Heine, N. A. Holmes, S. F. Worsley, A. C. A. Santos, T. M. Innocent, K. Scherlach, E. H. Patrick, D. W. Yu, C. Murrell, P. C. Vieria, J. J. Boomsma, C. Hertweck, M. I. Hutchings and B. Wilkinson, Nat. Commun., 2018, 9, 2208 CrossRef
.
- Y. Sugimoto, F. R. Camacho, S. Wang, P. Chankhamjon, A. Odabas, A. Biswas, P. D. Jeffrey and M. S. Donia, Science, 2019, 366, eaax9176 CrossRef CAS
.
- C. J. Guo, B. M. Allen, K. J. Hiam, D. Dodd, W. Van Treuren, S. Higginbottom, K. Nagashima, C. R. Fischer, J. L. Sonnenburg, M. H. Spitzer and M. A. Fischbach, Science, 2019, 366, 1331 CrossRef
.
- C. Lemetre, J. Maniko, Z. Charlop-Powers, B. Sparrow, A. J. Lowe and S. F. Brady, Proc. Natl. Acad. Sci. U. S. A., 2017, 114, 11615–11620 CrossRef CAS
.
- Z. Charlop-Powers and S. F. Brady, Bioinformatics, 2015, 31, 2909–2911 CrossRef CAS
.
- Z. Charlop-Powers, J. G. Owen, B. V. Reddy, M. A. Ternei, D. O. Guimaraes, U. A. de Frias, M. T. Pupo, P. Seepe, Z. Feng and S. F. Brady, eLife, 2015, 4, e05048 CrossRef
.
- Z. Charlop-Powers, J. G. Owen, B. V. Reddy, M. A. Ternei and S. F. Brady, Proc. Natl. Acad. Sci. U. S. A., 2014, 111, 3757–3762 CrossRef CAS
.
- B. V. Reddy, D. Kallifidas, J. H. Kim, Z. Charlop-Powers, Z. Feng and S. F. Brady, Appl. Environ. Microbiol., 2012, 78, 3744–3752 CrossRef CAS
.
- A. Edlund, S. Loesgen, W. Fenical and P. R. Jensen, Appl. Environ. Microbiol., 2011, 77, 5916–5925 CrossRef CAS
.
- K. C. Freel, A. Edlund and P. R. Jensen, Environ. Microbiol., 2012, 14, 480–493 CrossRef CAS
.
- C. Borsetto, G. C. A. Amos, U. N. da Rocha, A. L. Mitchell, R. D. Finn, R. F. Laidi, C. Vallin, D. A. Pearce, K. K. Newsham and E. M. H. Wellington, Microbiome, 2019, 7, 78 CrossRef
.
- N. V. Patin, K. R. Duncan, P. C. Dorrestein and P. R. Jensen, ISME J., 2016, 10, 478–490 CrossRef CAS
.
- N. Ziemert, A. Lechner, M. Wietz, N. Millan-Aguinaga, K. L. Chavarria and P. R. Jensen, Proc. Natl. Acad. Sci. U. S. A., 2014, 111, E1130–E1139 CrossRef CAS
.
- T. Hoffmann, D. Krug, N. Bozkurt, S. Duddela, R. Jansen, R. Garcia, K. Gerth, H. Steinmetz and R. Muller, Nat. Commun., 2018, 9, 803 CrossRef
.
- K. S. Ju, J. Gao, J. R. Doroghazi, K. K. Wang, C. J. Thibodeaux, S. Li, E. Metzger, J. Fudala, J. Su, J. K. Zhang, J. Lee, J. P. Cioni, B. S. Evans, R. Hirota, D. P. Labeda, W. A. van der Donk and W. W. Metcalf, Proc. Natl. Acad. Sci. U. S. A., 2015, 112, 12175–12180 CrossRef CAS
.
- R. Koch, Berl. Klin. Wochenschr., 1882, 19, 221–230 Search PubMed
.
- J. Overmann, B. Abt and J. Sikorski, Annu. Rev. Microbiol., 2017, 71, 711–730 CrossRef CAS
.
- S. R. Vartoukian, R. M. Palmer and W. G. Wade, FEMS Microbiol. Lett., 2010, 309, 1–7 CAS
.
- D. H. Parks, M. Chuvochina, P. A. Chaumeil, C. Rinke, A. J. Mussig and P. Hugenholtz, Nat. Biotechnol., 2020 DOI:10.1038/s41587-020-0501-8
.
-
H. Choi, A. R. Pereira and W. H. Gerwick, in Handbook of Marine Natural Products, ed. W. H. G. Ernesto Fattorusso and O. Taglialatela-Scafati, Springer, 2012, ch. 2, vol. 1, pp. 55–152 Search PubMed
.
- G. E. Chlipala, S. Mo and J. Orjala, Curr. Drug Targets, 2011, 12, 1654–1673 CrossRef CAS
.
- J. A. van Santen, G. Jacob, A. L. Singh, V. Aniebok, M. J. Balunas, D. Bunsko, F. C. Neto, L. Castano-Espriu, C. Chang, T. N. Clark, J. L. Cleary Little, D. A. Delgadillo, P. C. Dorrestein, K. R. Duncan, J. M. Egan, M. M. Galey, F. P. J. Haeckl, A. Hua, A. H. Hughes, D. Iskakova, A. Khadilkar, J. H. Lee, S. Lee, N. LeGrow, D. Y. Liu, J. M. Macho, C. S. McCaughey, M. H. Medema, R. P. Neupane, T. J. O'Donnell, J. S. Paula, L. M. Sanchez, A. F. Shaikh, S. Soldatou, B. R. Terlouw, T. A. Tran, M. Valentine, J. J. J. van der Hooft, D. A. Vo, M. Wang, D. Wilson, K. E. Zink and R. G. Linington, ACS Cent. Sci., 2019, 5, 1824–1833 CrossRef CAS
.
- S. A. Kautsar, K. Blin, S. Shaw, J. C. Navarro-Munoz, B. R. Terlouw, J. J. J. van der Hooft, J. A. van Santen, V. Tracanna, H. G. Suarez Duran, V. Pascal Andreu, N. Selem-Mojica, M. Alanjary, S. L. Robinson, G. Lund, S. C. Epstein, A. C. Sisto, L. K. Charkoudian, J. Collemare, R. G. Linington, T. Weber and M. H. Medema, Nucleic Acids Res., 2020, 48, D454–D458 Search PubMed
.
- D. H. Parks, M. Chuvochina, D. W. Waite, C. Rinke, A. Skarshewski, P. A. Chaumeil and P. Hugenholtz, Nat. Biotechnol., 2018, 36, 996–1004 CrossRef CAS
.
- K. Gemperlein, N. Zaburannyi, R. Garcia, J. J. La Clair and R. Muller, Mar. Drugs, 2018, 16, 314 CrossRef
.
- J. Herrmann, A. A. Fayad and R. Muller, Nat. Prod. Rep., 2017, 34, 135–160 RSC
.
- T. F. Schaberle, F. Lohr, A. Schmitz and G. M. Konig, Nat. Prod. Rep., 2014, 31, 953–972 RSC
.
- M. Nett, O. Erol, S. Kehraus, M. Kock, A. Krick, E. Eguereva, E. Neu and G. M. Konig, Angew. Chem., Int. Ed., 2006, 45, 3863–3867 CrossRef CAS
.
- Y. Igarashi, K. Yamamoto, C. Ueno, N. Yamada, K. Saito, K. Takahashi, M. Enomoto, S. Kuwahara, T. Oikawa, E. Tashiro, M. Imoto, Y. Xiaohanyao, T. Zhou, E. Harunari and N. Oku, J. Antibiot., 2019, 72, 653–660 CrossRef CAS
.
- F. Panter, R. Garcia, A. Thewes, N. Zaburannyi, B. Bunk, J. Overmann, M. V. Gutierrez, D. Krug and R. Muller, ACS Chem. Biol., 2019, 14, 2713–2719 CrossRef CAS
.
- O. Jeske, F. Surup, M. Ketteniss, P. Rast, B. Forster, M. Jogler, J. Wink and C. Jogler, Front. Microbiol., 2016, 7, 1242 Search PubMed
.
- K. Shindo, E. Asagi, A. Sano, E. Hotta, N. Minemura, K. Mikami, E. Tamesada, N. Misawa and T. Maoka, J. Antibiot., 2008, 61, 185–191 CrossRef CAS
.
- A. Crits-Christoph, S. Diamond, C. N. Butterfield, B. C. Thomas and J. F. Banfield, Nature, 2018, 558, 440–444 CrossRef CAS
.
- J. Lopera, I. J. Miller, K. L. McPhail and J. C. Kwan, mSystems, 2017, 2, e00096 CrossRef CAS
.
- A. T. Bull and M. Goodfellow, Microbiology, 2019, 165, 1252–1264 CrossRef CAS
.
- R. Subramani and D. Sipkema, Mar. Drugs, 2019, 17, 249 CrossRef CAS
.
- M. A. Schorn, M. M. Alanjary, K. Aguinaldo, A. Korobeynikov, S. Podell, N. Patin, T. Lincecum, P. R. Jensen, N. Ziemert and B. S. Moore, Microbiology, 2016, 162, 2075–2086 CrossRef CAS
.
- J. S. Li, C. C. Barber and W. Zhang, J. Ind. Microbiol. Biotechnol., 2019, 46, 375–383 CrossRef CAS
.
- Y. Imai, K. J. Meyer, A. Iinishi, Q. Favre-Godal, R. Green, S. Manuse, M. Caboni, M. Mori, S. Niles, M. Ghiglieri, C. Honrao, X. Ma, J. J. Guo, A. Makriyannis, L. Linares-Otoya, N. Bohringer, Z. G. Wuisan, H. Kaur, R. Wu, A. Mateus, A. Typas, M. M. Savitski, J. L. Espinoza, A. O'Rourke, K. E. Nelson, S. Hiller, N. Noinaj, T. F. Schaberle, A. D'Onofrio and K. Lewis, Nature, 2019, 576, 459–464 CrossRef CAS
.
- M. C. Wilson, T. Mori, C. Ruckert, A. R. Uria, M. J. Helf, K. Takada, C. Gernert, U. A. Steffens, N. Heycke, S. Schmitt, C. Rinke, E. J. Helfrich, A. O. Brachmann, C. Gurgui, T. Wakimoto, M. Kracht, M. Crusemann, U. Hentschel, I. Abe, S. Matsunaga, J. Kalinowski, H. Takeyama and J. Piel, Nature, 2014, 506, 58–62 CrossRef CAS
.
- L. L. Ling, T. Schneider, A. J. Peoples, A. L. Spoering, I. Engels, B. P. Conlon, A. Mueller, T. F. Schaberle, D. E. Hughes, S. Epstein, M. Jones, L. Lazarides, V. A. Steadman, D. R. Cohen, C. R. Felix, K. A. Fetterman, W. P. Millett, A. G. Nitti, A. M. Zullo, C. Chen and K. Lewis, Nature, 2015, 517, 455–459 CrossRef CAS
.
- L. Mahler, K. Wink, R. J. Beulig, K. Scherlach, M. Tovar, E. Zang, K. Martin, C. Hertweck, D. Belder and M. Roth, Sci. Rep., 2018, 8, 13087 CrossRef
.
- E. Zang, S. Brandes, M. Tovar, K. Martin, F. Mech, P. Horbert, T. Henkel, M. T. Figge and M. Roth, Lab Chip, 2013, 13, 3707–3713 RSC
.
- A. D'Onofrio, J. M. Crawford, E. J. Stewart, K. Witt, E. Gavrish, S. Epstein, J. Clardy and K. Lewis, Chem. Biol., 2010, 17, 254–264 CrossRef
.
- B. Berdy, A. L. Spoering, L. L. Ling and S. S. Epstein, Nat. Protoc., 2017, 12, 2232–2242 CrossRef
.
- M. Elfeki, M. Alanjary, S. J. Green, N. Ziemert and B. T. Murphy, ACS Chem. Biol., 2018, 13, 2074–2081 CrossRef CAS
.
- M. S. Costa, C. M. Clark, S. Omarsdottir, L. M. Sanchez and B. T. Murphy, J. Nat. Prod., 2019, 82, 2167–2173 CrossRef CAS
.
- F. Xu, Y. Wu, C. Zhang, K. M. Davis, K. Moon, L. B. Bushin and M. R. Seyedsayamdost, Nat. Chem. Biol., 2019, 15, 161–168 CrossRef CAS
.
- H. N. Lyu, H. W. Liu, N. P. Keller and W. B. Yin, Nat. Prod. Rep., 2020, 37, 6–16 RSC
.
- E. Bode, A. K. Heinrich, M. Hirschmann, D. Abebew, Y. N. Shi, T. D. Vo, F. Wesche, Y. M. Shi, P. Grun, S. Simonyi, N. Keller, Y. Engel, S. Wenski, R. Bennet, S. Beyer, I. Bischoff, A. Buaya, S. Brandt, I. Cakmak, H. Cimen, S. Eckstein, D. Frank, R. Furst, M. Gand, G. Geisslinger, S. Hazir, M. Henke, R. Heermann, V. Lecaudey, W. Schafer, S. Schiffmann, A. Schuffler, R. Schwenk, M. Skaljac, E. Thines, M. Thines, T. Ulshofer, A. Vilcinskas, T. A. Wichelhaus and H. B. Bode, Angew. Chem., Int. Ed., 2019, 58, 18957–18963 CrossRef CAS
.
- S. Kunakom and A. S. Eustaquio, mSystems, 2019, 4, e00113 CrossRef CAS
.
- E. J. Culp, G. Yim, N. Waglechner, W. Wang, A. C. Pawlowski and G. D. Wright, Nat. Biotechnol., 2019, 37, 1149–1154 CrossRef CAS
.
- Y. Tong, T. Weber and S. Y. Lee, Nat. Prod. Rep., 2019, 36, 1262–1280 RSC
.
- M. N. Thaker, N. Waglechner and G. D. Wright, Nat. Protoc., 2014, 9, 1469–1479 CrossRef CAS
.
- X. Tang, J. Li, N. Millan-Aguinaga, J. J. Zhang, E. C. O'Neill, J. A. Ugalde, P. R. Jensen, S. M. Mantovani and B. S. Moore, ACS Chem. Biol., 2015, 10, 2841–2849 CrossRef CAS
.
- Y. Yan, N. Liu and Y. Tang, Nat. Prod. Rep., 2020 10.1039/c9np00050j
.
- J. Piel and J. Cahn, Angew. Chem., Int. Ed., 2019 DOI:10.1002/anie.201900532
.
- J. A. Leeds, E. K. Schmitt and P. Krastel, Expert Opin. Invest. Drugs, 2006, 15, 211–226 CrossRef CAS
.
- D. A. Pereira and J. A. Williams, Br. J. Pharmacol., 2007, 152, 53–61 CrossRef CAS
.
- X. Liu, E. Ashforth, B. Ren, F. Song, H. Dai, M. Liu, J. Wang, Q. Xie and L. Zhang, J. Antibiot., 2010, 63, 415–422 CrossRef CAS
.
- O. Genilloud, I. Gonzalez, O. Salazar, J. Martin, J. R. Tormo and F. Vicente, J. Ind. Microbiol. Biotechnol., 2011, 38, 375–389 CrossRef CAS
.
- V. Knight, J. J. Sanglier, D. DiTullio, S. Braccili, P. Bonner, J. Waters, D. Hughes and L. Zhang, Appl. Microbiol. Biotechnol., 2003, 62, 446–458 CrossRef CAS
.
- W. Fenical and P. R. Jensen, Nat. Chem. Biol., 2006, 2, 666–673 CrossRef CAS
.
- A. L. Demain, Nat. Biotechnol., 2002, 20, 331 CrossRef
.
- J. F. Barrett, Curr. Opin. Microbiol., 2005, 8, 498–503 CrossRef
.
-
T. A. Okuda and G. Bills, in Handbook of Industrial Mycology, CRC Press/Taylor and Francis Group, 2004, ch. 6 Search PubMed
.
- F. Fujimori and T. Okuda, J. Antibiot., 1994, 47, 173–182 CrossRef CAS
.
- A. T. Bull, A. C. Ward and M. Goodfellow, Microbiol. Mol. Biol. Rev., 2000, 64, 573–606 CrossRef CAS
.
- P. Monciardini, M. Iorio, S. Maffioli, M. Sosio and S. Donadio, Microb. Biotechnol., 2014, 7, 209–220 CrossRef CAS
.
- C. M. Clark, M. S. Costa, L. M. Sanchez and B. T. Murphy, Proc. Natl. Acad. Sci. U. S. A., 2018, 115, 4981–4986 CrossRef CAS
.
- C. M. Clark, E. Conley, E. Li, L. M. Sanchez and B. T. Murphy, J. Visualized Exp., 2019, 147, e59219 Search PubMed
.
- J. Y. Yang, L. M. Sanchez, C. M. Rath, X. Liu, P. D. Boudreau, N. Bruns, E. Glukhov, A. Wodtke, R. de Felicio, A. Fenner, W. R. Wong, R. G. Linington, L. Zhang, H. M. Debonsi, W. H. Gerwick and P. C. Dorrestein, J. Nat. Prod., 2013, 76, 1686–1699 CrossRef CAS
.
- L. F. Nothias, M. Nothias-Esposito, R. da Silva, M. Wang, I. Protsyuk, Z. Zhang, A. Sarvepalli, P. Leyssen, D. Touboul, J. Costa, J. Paolini, T. Alexandrov, M. Litaudon and P. C. Dorrestein, J. Nat. Prod., 2018, 81, 758–767 CrossRef CAS
.
- R. Ueoka, A. Bhushan, S. I. Probst, W. M. Bray, R. S. Lokey, R. G. Linington and J. Piel, Angew. Chem., Int. Ed., 2018, 57, 14519–14523 CrossRef CAS
.
- J. C. Navarro-Muñoz, N. Selem-Mojica, M. W. Mullowney, S. A. Kautsar, J. H. Tryon, E. I. Parkinson, E. L. C. De Los Santos, M. Yeong, P. Cruz-Morales, S. Abubucker, A. Roeters, W. Lokhorst, A. Fernandez-Guerra, L. T. D. Cappelini, A. W. Goering, R. J. Thomson, W. W. Metcalf, N. L. Kelleher, F. Barona-Gomez and M. H. Medema, Nat. Chem. Biol., 2020, 16, 60–68 CrossRef
.
- J. R. Doroghazi, J. C. Albright, A. W. Goering, K. S. Ju, R. R. Haines, K. A. Tchalukov, D. P. Labeda, N. L. Kelleher and W. W. Metcalf, Nat. Chem. Biol., 2014, 10, 963–968 CrossRef CAS
.
- A. W. Goering, R. A. McClure, J. R. Doroghazi, J. C. Albright, N. A. Haverland, Y. Zhang, K. S. Ju, R. J. Thomson, W. W. Metcalf and N. L. Kelleher, ACS Cent. Sci., 2016, 2, 99–108 CrossRef CAS
.
- K. Chudejova, M. Bohac, A. Skalova, V. Rotova, C. C. Papagiannitsis, J. Hanzlickova, T. Bergerova and J. Hrabak, PLoS One, 2017, 12, e0190038 CrossRef
.
- Hindra, T. Huang, D. Yang, J. D. Rudolf, P. Xie, G. Xie, Q. Teng, J. R. Lohman, X. Zhu, Y. Huang, L. X. Zhao, Y. Jiang, Y. Duan and B. Shen, J. Nat. Prod., 2014, 77, 2296–2303 CrossRef CAS
.
- M. Crusemann, E. C. O'Neill, C. B. Larson, A. V. Melnik, D. J. Floros, R. R. da Silva, P. R. Jensen, P. C. Dorrestein and B. S. Moore, J. Nat. Prod., 2017, 80, 588–597 CrossRef CAS
.
- D. J. Floros, P. R. Jensen, P. C. Dorrestein and N. Koyama, Metabolomics, 2016, 12, 145 CrossRef
.
- R. Dieckmann, I. Graeber, I. Kaesler, U. Szewzyk and H. von Dohren, Appl. Microbiol. Biotechnol., 2005, 67, 539–548 CrossRef CAS
.
- Y. Hou, D. R. Braun, C. R. Michel, J. L. Klassen, N. Adnani, T. P. Wyche and T. S. Bugni, Anal. Chem., 2012, 84, 4277–4283 CrossRef CAS
.
- D. Forner, F. Berrue, H. Correa, K. Duncan and R. G. Kerr, Anal. Chim. Acta, 2013, 805, 70–79 CrossRef CAS
.
|
This journal is © The Royal Society of Chemistry 2021 |