DOI:
10.1039/D0MO00173B
(Review Article)
Mol. Omics, 2021,
17, 357-364
Genetic and structural analyses of ssRNA viruses pave the way for the discovery of novel antiviral pharmacological targets
Received
1st December 2020
, Accepted 18th February 2021
First published on 19th February 2021
Abstract
In the era of big data and artificial intelligence, a lot of new discoveries have influenced the fields of antiviral drug design and pharmacophore identification. Viruses have always been a threat to society in terms of public health and economic stability. Viruses not only affect humans but also livestock and agriculture with a direct impact on food safety, economy and environmental imprint. Most recently, with the pandemic of COVID-19, it was made clear that a single virus can have a devastating impact on global well-being and economy. In this direction, there is an emerging need for the identification of promising pharmacological targets in viruses. Herein, an effort has been made to discuss the current knowledge, state-of-the-art applications and future implications for the main pharmacological targets of single-stranded RNA viruses.
Introduction
In the last 50 years, more than 50 new viruses have been identified that are responsible for several human diseases. However, the development of antiviral drugs is not an easy task. It took about 60 years from the initial development of the antiviral drug to achieve its current state of efficacy. In contrast to the development of antiviral drugs, the development of antibiotics reached a fairly advanced therapeutic stage over a period of 34 years, as the first Salvarsan antibacterial molecule was described by Ehlrich in 1910,1 followed by the discovery of penicillin by Fleming in 19292 and the discovery of prontosil, a precursor of sulfonamides, by Domagk in 1935,3 resulting in the isolation of streptomycin, chloramphenicol, erythromycin, and tetracycline by Waksman in 1944.4,5
Although the mainstay of the treatment of viral diseases has been the development of vaccines for several years, a new era of antiviral therapy began in 1963 with the development and approval of the first human antiviral drug, idoxuridine.6 Since then, almost a hundred FDA-approved drugs have indication for antiviral treatment, with the latest being remdesivir for the treatment of COVID-19 (Fig. 1).7 The disadvantage of antiviral drugs is the fact that they can affect the functioning of the molecular pathways of the host, thus posing a high risk of toxicity. The reason for this disadvantage is the fact that the life cycle and reproduction of viruses involve the use of biochemical pathways of the host cells.8 For this reason, the identification of specific and specialized viral targets with increased selectivity and reduced side effects is a major concern in the antiviral drug development process. Overcoming toxicity remains challenging, and targeted delivery systems, such as nanoparticles, are being considered for their enhanced biocompatibility and low toxicity.9 Another direction is the combination of multiple drug entities, where synergism between the compounds can minimize drug resistance and toxicity, as is the case for first-line HIV treatment.10
 |
| Fig. 1 Timeline of FDA-approved antiviral drugs since 1963. | |
Rational drug design is one of the most common approaches to the discovery and development of antiviral drugs. This approach is based on understanding the structures and functions of target molecules and includes three steps: (1) identifying the enzyme or receptor responsible for the disease being studied, (2) finding the structure and function of the enzyme or the receptor of interest, and (3) the use of the above information to design a drug molecule that would interact with the receptor or enzyme in a therapeutically beneficial manner. A typical example of this approach is the drug used against the human immunodeficiency virus (HIV), azidothymidine (AZT), which acts as an inhibitor of HIV reverse transcription. It is interesting to note that AZT was originally developed to target the reverse transcription of cancer-causing avian retroviruses and was later used successfully in the treatment of HIV.8
High-throughput screening (HTS) methods are another widely used approach in the development of antiviral drugs. These methods enable the validation of a number of biological regulators against a selected set of defined targets, delivering rich data sets in a short time as they combine fluid handling specialization and robotic automation, multi-format plate reading, and high-performance imaging. Similar to the rational drug design approach, the HTS pipeline also includes three basic steps: (1) sample preparation, (2) sample handling, and (3) readings and data acquisition. Of the HTS methods, the most widely used are targeted/selected screening, diversity and high content screening, and RNAi screening.11
The basis of targeted or selected screening is the identification of molecules or compounds that have the ability to inhibit or selectively bind to a particular protein of interest. In silico three-dimensional (3-D) modeling is used in the case where the 3-D structure of the protein of interest is known, while in the case where the ligand of the protein of interest is known, a software that can search in libraries to find molecules or compounds with similar characteristics and binding properties is used. This method has been used to identify antiviral compounds for HIV, filoviruses, poxviruses, and arenaviruses.12
Diversity screening is another widely used screening method based on discovering and identifying compounds that arrest viral proliferation or pathogenesis at the ground level. The difference between this method and the aforementioned one is that diversity screening does not focus on a specific protein of interest but includes a broader base of targets and has been applied to finding potential small-molecule inhibitors against dengue virus, yellow fever virus, and New World arenaviruses.13 A subcategory of this method is the high content screening (HCS) method developed through the automation of cell imaging and analysis techniques. HCS enables simultaneous multi-cell imaging and simultaneous measurement of multiple parameters such as shape, texture, total cell number, nucleus size, and percentage of virus-positive cells.14
RNAi screening is an additional screening method for the detection of antiviral drugs, in which RNA molecules inhibit the expression or translation of genes by neutralizing targeted mRNA molecules. In this method, small interfering RNAs (siRNAs) are incorporated into the RNA-induced silencing complex (RISC) and bind to the target mRNA through the complementarity of the nucleotide bases, thereby causing degradation of mRNA and preventing its translation into protein. The short hairpin RNAs (shRNAs), on the other hand, are RNA molecules with a tight hairpin folding through which they silence the protein. This method has been used to study the pathogenesis of a wide range of viruses such as HIV, influenza virus, West Nile virus, Ebola virus, respiratory syncytial virus, hepatitis B (HBV) or C virus (HCV), and dengue virus (DENV).15,16
In addition to the methods mentioned above, recent developments in genomics and bioinformatics offer new opportunities in the discovery of antiviral drugs. Nowadays, computer-generated databases contain information related to the biological functions, chemical structures, biological activities, and many other properties of possible antiviral compounds, thus helping to identify new lead bioactive entities.17 Particularly, during the last few years and the recent pandemic, omics technologies have been applied for the rapid and more efficient drug discovery and the elucidation of viral pathogenesis.18,19
Structural conservation of viral proteins
It has been widely evidenced that the three-dimensional structure of a protein is more related to its function than its primary amino acid sequence.20 Hence, the three-dimensional arrangement of the polypeptide chains can appear generally more conserved, while primary sequences can diverge. Structural conservation, and the notion of the homology of proteins based on their shared structural conformation, has been extensively used in the study of viruses.21 More specifically, it has provided valuable insights into the evolutionary origins of viral proteins as well as of the viral families themselves, something that would have been impossible with the use of sequence analysis alone. The structural features of a viral protein are naturally tied to the molecular function that the viral protein is expected to carry out. Hence, viral protein families are often found to exhibit structural similarities. For example, viral helicases, motor proteins that use the free energy of nucleoside triphosphate (NTP) hydrolysis in order to unwind duplexes of nucleic acids, demonstrate a common catalytic core conformation. The core topology consists of four strands flanked by a helix and two helices on either side, respectively, allowing the binding of nucleotides to the Walker A and B motifs.22 Viral polymerases, much like host cell polymerases, possess the common structural framework of the finger, palm and thumb domains, which contain the sequence motifs A–G that characterize polymerase sequences.23 Viral proteases in the PA clan of proteases, which contains cysteine and serine proteases, exhibit a conserved double β-barrel core, while catalysis occurs at the interface of the two barrels with the aid of the catalytic triad motif.24 Viral proteases additionally exhibit a flexible C-terminal loop that creates a binding tunnel.25 For a number of negative-stranded RNA viruses from families such as Filoviridae and Rhabdoviridae, viral RNA synthesis is dependent on a phosphoprotein in an assembly of three domains: a central oligomerization domain, a conserved C-terminal domain and a disordered N-terminal domain.26 In spite of the low sequence homology of this protein across the aforementioned viral families, the C-terminal domain contains a common α-helical core. This structural conservation appears to correlate with an evolutionary conserved function in mediating the binding of P to the nucleocapsid protein.27 A thorough study of the various levels of structural conservation can provide invaluable information in terms of understanding the life cycle of the virus and assembly mechanisms, especially in the case of emerging viruses. In a recent study, Diaz-Valle and associates have described a conservation-based methodology to identify interface residues of key importance for the self-assembly and the thermodynamic stability of the viral capsid.28 Furthermore, structural information regarding conserved binding sites can guide the process of constructing anti-viral strategies, as demonstrated in a study by Darapaneni et al., where conserved sites on the HIV-1 viral protein U were characterized as novel binding sites and, by extension, as possible anti-HIV-1 drug targets.29 Finally, structural conservation of viral proteins can allow the repurposing of existing inhibitors or other molecules that have been found to target the conserved sites, speeding up the process of constructing an anti-viral strategy. The most important pharmacological targets in antiviral drug design include non-structural viral proteins, while most of the approved antivirals target proteases and polymerases, and potential therapeutic effects for helicase and methyltransferase inhibition are being widely researched (Fig. 2).30,31
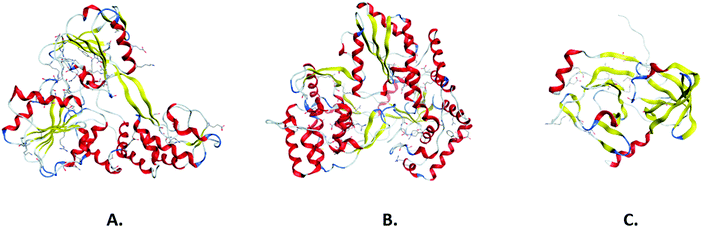 |
| Fig. 2 3-D structure of non-structural HCV helicase (A), polymerase (B), and protease (C) in ribbon representation. | |
The most important pharmacological targets
Proteases
Viruses of the Togaviridae family have a positive-sense, single-stranded (ss) RNA genome, which codes for various structural and non-structural (ns) proteins, including two proteases, capsid protease (CP) and nsP2 protease.32 The function of CP is essential in order for the processing of the structural polyprotein to begin, while nsP2 protease activity is critical for the maturation of the ns polyprotein, both of which are essential steps in the viral replication cycle. The C-terminal region of CP comprises a chymotrypsin-like protease fold, thus allowing for serine protease activity, with the catalytic triad of residues Ser–His–Asp forming the active site.33 Conversely, nsP2 contains a papain-like cysteine protease in its C-terminal region. This protease is responsible for the processing of the ns polyprotein, through the recognition of conserved motifs. In addition, the Togaviridae protease participates in other processes, such as the suppression of host antiviral responses.34 Crystallographic evidence showed that residues in the protein's active site appear highly conserved.35 Furthermore, structural studies between members of the viral family have shown high conservation of folds, despite low sequence identity, reinforcing the idea of the protease domain of nsP2 as a potential pharmacological target.36 Viruses of the important families such as Picornaviridae, Caliciviridae and Coronaviridae are positive-sense, ssRNA viruses with a common feature, which is the possession of a 3C or 3C-like protease (3Cpro or 3CLpro, respectively) responsible for the cleavage of the viral polyprotein.37 A chymotrypsin-fold and a catalytic triad of Cys–His–Glu/Asp residues are common features between 3Cpro and 3CLpro.38 Similarly, in the Togaviridae family, structural studies have elucidated the conservation of substrate-binding pockets as well as active sites,35,39 evidencing their functional significance and their potential use in the scope of developing antiviral drugs. Viruses in the widely studied Flaviviridae family code for the NS3 protein, which exhibits protease activity, among others. Flavivirus protease is a complex between the NS3 protein and a hydrophobic core within the viral NS2B protein, which acts as an essential cofactor. The function of this complex, similar to the previously mentioned viral families, is the proteolytic cleavage of the precursor polyprotein that the viral genome encodes, allowing the release of mature non-structural proteins.40 Early studies showed the essential role of the Flaviviridae protease by reporting the absence of infectious virions in mutants with inactivated viral proteases.41 While the NS3 active site remains a standard pharmacological target against flaviviruses, inherent difficulties in targeting this specific feature of the complex have led to a new interest in the association between NS2B and NS3.40 More specifically, two regions of the NS2B, Nter constituting aa 53–61 and Cter constituting aa 74–86, have been shown to be essential for the function of the complex.42 As a result, the disruption of the NS2B–NS3 interaction emerges as a possible alternative approach in the design of anti-flaviviral drugs. Regardless of the differences between viral families with regard to protease characteristics, the role of the protein in the maturation of the viral proteins and, by extension, in the viral replication remains pivotal, establishing it as a promising target against pathogenic ssRNA viruses.
Helicases
In general, helicases use energy from ATP hydrolysis in order to separate nucleic acid strands.43 Cells need RNA helicases for mRNA transcription and translation, and to assemble or disassemble RNA–protein complexes. Viruses with RNA genomes also use helicases to separate RNA duplexes formed after replication. Some types of helicases assemble into oligomeric rings,44 while non-ring helicases carry out their function as oligomers of various orders.45 To separate a double strand, helicases need to load on a single-stranded RNA (or DNA), and then proceed to move either from 5′ to 3′-end or in the opposite direction.46 Apart from differences in the direction of movement and the oligomeric state, the basis of amino acid sequence and motif conservation can be used to divide helicases into three superfamilies (SF1, SF2, and SF3).47 Non-ring helicases mostly belong to helicase superfamily 1 (SF1) or SF2, while ring helicases belong either to SF3 or other families.48 As a general mode of action, helicases bind NTP by using two amino acid patterns, the Walker A and B motifs, which form a phosphate-binding P-loop, with a conserved Lys, and a Mg2+ co-factor binding loop, respectively.49 A NTP-binding pocket forms when the helicase domain 2 approaches domain 1, leading to ATP hydrolysis, while a specific motif between the two domains functions as a hinge, allowing for the movement of domain 2. The existence of these domains and the dynamically changing conformation, characterized as “closed” or “open”, are essential for the enzyme function.47 Additionally, many of the conserved residues critical for the helicase activity appear to be located on the interior face between the two aforementioned domains.50 Functionally important residues and structural features that enable enzyme activity can guide the search for effective drugs that target viral helicases. Competition with NTP binding, inhibition of NTP hydrolysis, inhibition of RNA binding, blocking of the domain 2 movements for the achievement of the “closed” conformation, and sterically blocking the helicase translocation to prevent unwinding of the nucleic acid are but a few of the strategies that can be drawn from the principles of the NTPase function of the helicase.50 A major concern when reviewing viral helicases as a pharmacological target is the likelihood of toxicity after the employment of potential helicase-inhibiting compounds, which is in turn caused by the high level of conservation that has been documented among helicases. Nevertheless, the differences in tertiary structure and sequence between the cell and the viral RNA helicases can provide a window of opportunity for the specific, safe targeting of virally encoded helicases, while avoiding unwanted effects on the cell enzymes that catalyze similar reactions. This was evidenced in a study on hepatitis C virus (HCV) helicase by Frick and associates, which sought specific motifs of domain 2 conserved in HCV isolates but not in the related proteins. Since similar sites would not be present in related cellular helicases, targeting them would potentially not cause toxic effects within the cells.48
Methyltransferases
RNA capping, the chemical modification at the 5′-end of the nascent mRNA, is a crucial process for the stability of mRNA molecules, both for eukaryotes and for viruses.51 In general, viruses replicate in the cytoplasm, while most capping mechanisms take place within the nucleus.52 In broad strokes, the steps for the addition of the cap structure are as follows: first, the 5′-triphosphate is hydrolyzed by an RTPase, then a GTase adds the structure of the cap as a guanosine 5′-monophosphate in a 5′–5′ orientation. This is followed by the activity of an N-7 methyltransferase, which methylates the cap onto the N-7 position of its guanine, and finally, methylation by ribose 2′-O-methyl-transferase (2′-O MTase) takes place at the 2′-position of the riboses to produce cap-1 and cap-2 structures.53 The methyl donor for the last two methylation processes is S-adenosyl-L-methionine (SAM). The last two methylation processes can be carried out by specific domains or proteins (e.g. in the Coronaviridae viral family) or by a single protein or domain (e.g. in the Flaviviridae viral family).53 The methyltransferase (MTase) domain of the Flavivirus non-structural 5 (NS5) protein is a prime example of a single-domain MTase enzyme. The Flavivirus NS5 protein contains an RdRp domain in the C-terminal and an MTase domain in the N-terminal.54 It has been shown that the MTase domain carries out both the N-7 MTase and the 2′-O MTase activities.55 The structure of the domain comprises four α-helices which surround the 7-stranded β-sheet, while the catalytic site harbors the K-D-K-E tetrad.53 It was discovered that the two methylation processes take place in sequence, and a control over the reaction is achieved through a steric constraint for the substrates.55 More specifically, wild-type nucleotides are required at the 2nd and 3rd positions for N-7 methylation, while the prerequisite for 2′-O methylation is wild-type nucleotides at the 1st and 2nd positions, with a specific minimum of viral RNA nucleotides.53 Hindering the N-7 MTase activity leads to the inhibition of viral RNA translation, which is a crucial step in viral replication and, by extension, in the life cycle of the virus, as evidenced in several studies.54,55 Additionally, it has been shown that the lack of 2′-O methylation on the RNA structure can trigger antiviral responses,56 while mutations in the 2′-O MTase gene have been shown to cause reduced replication in animal models.57 The critical importance of the MTase function in the successful capping of viral RNA, ensuring its stability and successful use within the cell, supports its potential as a pharmacological target towards the inhibition of the replication of ssRNA viruses. Towards this goal, new crystallographic data and mechanistic or array studies continue to shed light on potentially useful structural features of the methyltransferases, such as a conserved hydrophobic binding pocket next to the SAM-binding site of Flavivirus MTase.31 In summary, there is a dual benefit in examining methyltransferases as a pharmacological target against ssRNA viruses. RNA caps that are incomplete or immature may first present modified expression profiles, affecting the replication cycle of the virus. Second, a broad spectrum of sensors of innate cellular immune response can be triggered by the absence of an RNA cap, essentially aiding any efforts to hinder the viral infection.58
Polymerases
During their life cycle, viruses need to convert their genomes into mRNAs which can be translated into viral proteins, as well as generate accurate copies of their genomes, which will be packaged into the newly assembled virions.59 RNA viruses replicate and transcribe their genomes with the use of RNA-dependent RNA polymerase (RdRp) enzymes.23 In the case of positive-sense, single-stranded RNA viruses, a single-stranded genomic RNA is used as an mRNA inside the infected cell, whereas the replication of the viral RNA genome is a process carried out by the viral RNA-dependent RNA polymerase. RNA-dependent RNA polymerase transcribes the genomic positive-sense RNA strand into the complementary negative-sense RNA, which then serves as the template for the production of multiple copies of positive-sense viral RNA.60 The main catalytic activity of the polymerase is the transfer of a nucleotidyl moiety of an incoming NTP complementary to the template strand, to the 3′-hydroxyl end of a growing daughter strand of RNA.23 The general steps of the process are the binding of the template and the NTP, the addition of the nucleoside monophosphate to the growing daughter strand and the translocation of the enzyme along the template under use.61 The structure of RNA-dependent RNA polymerases follows the general conformation shared by all polymerases, described as a cupped right hand, with domains described as “palm”, “thumb” and “fingers”.60 The catalytic palm domain of the enzyme is composed of a β-sheet located between α-helices.62 In general, the size of the catalytic module varies, depending on the nature of the RdRp initiation type, which can be primer-dependent initiation or de novo initiation. The interior faces of the thumb and finger domains create the template-binding channel that ends in the catalytic palm.23 RNA-dependent RNA polymerases have been found to share conserved sequence motifs named A, B, C and D. More specifically, motifs A and C possess the catalytic Asp residues that bind two metal ions in the active site of the enzyme.63 RNA-dependent RNA polymerases present a unique feature in the shape of “fingertips”, that form the finger domain, which interacts with the thumb domain.64 These “fingertips” aid in forming a template-binding channel and an NTP-binding channel at the “front” and the “back” facets of the enzyme structure, respectively. The size of the thumb domain tends to vary between RNA-dependent RNA polymerases, presumably correlating to the initiation mechanism used by the enzyme.23 In the case of negative-sense ssRNA viruses, the viral negative-sense genomes cannot be used to produce viral proteins and must be converted into positive-sense RNA. Since RNA-dependent RNA polymerization cannot be executed by host cell polymerases, the viral RNA-dependent RNA polymerase is packaged within the virion and is responsible for the transcription of the viral genome, as well as for its replication.65 In general, the RNA-dependent RNA polymerase plays a key role in the life cycle of the virus, given the fact that it carries out the essential processes of replication and transcription. Furthermore, the catalytic core of the enzyme appears to be strongly conserved as a region, as well as relatively accessible, allowing its potential use in the efforts to inhibit the function of the enzyme.66 Finally, the well-documented RNA-dependent RNA-polymerase conservation between various RNA viruses is a very attractive feature in terms of its potential as a pharmacological target. This level of conservation could allow the design of inhibitors or other therapeutic strategies on a broad spectrum for different ssRNA viruses.
The future of drug design is in big data and learning
A widely used approach in the field of anti-viral drug design is computational structure-based drug discovery.67 In this approach, trial compounds are docked in silico into binding sites that have been elucidated in the three-dimensional structures of the protein targets.68 Through the implementation of physics-based equations that ground the interactions between the target and the candidate drug to quantifiable results, the binding affinities of the compounds are calculated. The compounds that present the best scores can be subsequently validated in vitro, to ascertain whether they indeed bind to the target and have the desired effects and the simultaneous absence of toxicity, both in cell cultures and in animal models.69 The expensive and time-consuming process of conventional, lab-based, high-throughput screening has been steadily outperformed by these virtual screening methods. Molecular dynamics simulations can be executed in parallel, towards the calculation of protein motions, and candidate compounds can undergo screening through a process called “ensemble docking”, which takes into account the different shapes that are formed by the binding site and has been shown to be more precise than the standard rigid docking.70 The detailed exploration of the conformational space during the molecular dynamics simulations requires increasing amounts of computational speed and efficacy. Computational systems that proved sufficient for the standard rigid docking experiments of the earlier years cannot yield the results that the current viral epidemics call for. Advanced computational systems, reaching as far as quantum computing, are the ones that will possess the parallel processing capabilities needed for the simulations and the docking of vast compound databases.71 Such a cutting-edge approach can be expected to accelerate the drug discovery process. Furthermore, newly developed methods, such as atomic force microscopy, are offering novel insights into the molecular interactions during viral infection and/or inhibition, and can be used for a more direct and accurate estimation of protein–protein interactions and affinity, enhancing the drug design process.72 Unwanted side effects and reduced efficiency of candidate compounds have been steadily leading to a decline in the number of FDA-approved drugs, along with a significant delay in the manufacturing of new molecular entities.73 Artificial intelligence (AI) approaches can provide an alternative path in the process of anti-viral drug design, and a way to utilize the new scale of scientific data size and complexity.74 Through the use of algorithms to process data and the identification of patterns of functional properties, the artificial intelligence machine predicts outcomes related to drug discovery. This process, described as machine learning, can be taken to higher levels, such as deep learning or neural networks approach, making use of vast datasets in order to reach multiple layers of learning.75 AI strategies can prove immensely useful in various stages of anti-viral drug design, such as the selection of drug candidates with desired properties, which include physical properties that can be predicted by an AI system, and predictions of a molecule's bioactivity or toxicity. Additional examples of AI applications include the use of recurrent neural networks and reinforcement learning for de novo molecular design,76 or the implementation of neural networks towards the establishment of a scoring function for protein and ligand interactions, or the prediction of ligand-binding affinity.75 AI technologies have also been successfully implemented in the in silico prediction of protein structures. Innovative programs, based on deep learning algorithms, such as AlphaFold, have outperformed conventional methods reaching higher levels of accuracy, comparable to crystallographic data, empowering structure-based drug design research.77
Conclusion
The most prevalent pharmacological targets of single-stranded RNA viruses are the viral protease, helicase, methyltransferase and polymerase. A lot of research has already been conducted in an effort to tackle these viruses by intercepting and hijacking the abovementioned pharmacological targets. Mercifully, the ever-evolving fields of the state-of-the-art virology and antiviral drug design are now flanked by big data and efficient informatics for the management and analytics that come with them. Modern technologies such as artificial intelligence or advanced supercomputing systems have the potential to provide the next step for anti-viral drug design, in a manner that is both time- and cost-efficient. Hence, the arsenal of the virologist has been hugely reinforced, and novel and very efficient antiviral strategies are expected to be developed based on the well-understood and well-described antiviral pharmacological targets of ssRNA viruses.
Conflicts of interest
There are no conflicts to declare.
References
- J. G. Fitzgerald, Ehrlich-Hata Remedy for Syphilis, Can. Med. Assoc. J., 1911, 1(1), 38–46 CAS.
- A. E. Porritt, The discovery and development of penicillin, Med. Press, 1951, 225(19), 460–462 CAS.
- G. Domagk, [Sulfonamides in the past, present and future], Minerva Med, 1950, 41(35), 41–47 CAS.
- O. L. Bryan-Marrugo, J. Ramos-Jiménez, H. Barrera-Saldaña, A. Rojas-Martínez, R. Vidaltamayo and A. M. Rivas-Estilla, History and progress of antiviral drugs: From acyclovir to direct-acting antiviral agents (DAAs) for Hepatitis C, Medicina Universitaria, 2015, 17(68), 165–174 CrossRef.
-
H. C. Neu and T. D. Gootz, Antimicrobial Chemotherapy. in Medical Microbiology, ed. S. Baron, Galveston (TX), 1996 Search PubMed.
- E. De Clercq and G. Li, Approved Antiviral Drugs over the Past 50 Years, Clin. Microbiol. Rev., 2016, 29(3), 695–747 CrossRef PubMed.
- D. Rubin, K. Chan-Tack, J. Farley and A. Sherwat, FDA Approval of Remdesivir - A Step in the Right Direction, N. Engl. J. Med., 2020, 383(27), 2598–2600 CrossRef CAS PubMed.
- F. Dal Pozzo and E. Thiry, Antiviral chemotherapy in veterinary medicine: current applications and perspectives, Rev. Sci. Technol., 2014, 33(3), 791–801 CrossRef CAS PubMed.
- D. Lembo and R. Cavalli, Nanoparticulate delivery systems for antiviral drugs, Antiviral Chem. Chemother., 2010, 21(2), 53–70 CrossRef CAS PubMed.
- E. Paintsil and Y.-C. Cheng, Antiviral Agents, Encycl. Microbiol., 2019, 176–225 Search PubMed.
- P. Szymanski, M. Markowicz and E. Mikiciuk-Olasik, Adaptation of high-throughput screening in drug discovery-toxicological screening tests, Int. J. Mol. Sci., 2012, 13(1), 427–452 CrossRef CAS PubMed.
- D. P. Marriott, I. G. Dougall, P. Meghani, Y. J. Liu and D. R. Flower, Lead generation using pharmacophore mapping and three-dimensional database searching: application to muscarinic M(3) receptor antagonists, J. Med. Chem., 1999, 42(17), 3210–3216 CrossRef CAS PubMed.
- M. J. Valler and D. Green, Diversity screening versus focussed screening in drug discovery, Drug Discovery Today, 2000, 5(7), 286–293 CrossRef PubMed.
- P. Brodin and T. Christophe, High-content screening in infectious diseases, Curr. Opin. Chem. Biol., 2011, 15(4), 534–539 CrossRef CAS PubMed.
- R. Badia, E. Ballana, J. A. Este and E. Riveira-Munoz, Antiviral treatment strategies based on gene silencing and genome editing, Curr. Opin. Virol., 2017, 24, 46–54 CrossRef CAS PubMed.
- A. J. Hirsch, The use of RNAi-based screens to identify host proteins involved in viral replication, Future Microbiol., 2010, 5(2), 303–311 CrossRef CAS PubMed.
-
M. N. Prichard, New approaches to antiviral drug discovery (genomics/proteomics), in Human Herpesviruses: Biology, Therapy, and Immunoprophylaxis, ed. A. Arvin, G. Campadelli-Fiume, E. Mocarski, P. S. Moore, B. Roizman and R. Whitleyet al., Cambridge, 2007 Search PubMed.
- D. Barh, S. Tiwari, M. E. Weener, V. Azevedo, A. Goes-Neto and M. M. Gromiha,
et al., Multi-omics-based identification of SARS-CoV-2 infection biology and candidate drugs against COVID-19, Comput. Biol. Med., 2020, 126, 104051 CrossRef CAS PubMed.
- K. A. Overmyer, E. Shishkova, I. J. Miller, J. Balnis, M. N. Bernstein and T. M. Peters-Clarke,
et al., Large-Scale Multi-omic Analysis of COVID-19 Severity, Cell Syst., 2021, 12(1), 23–40 CrossRef CAS PubMed.
- K. Illergard, D. H. Ardell and A. Elofsson, Structure is three to ten times more conserved than sequence–a study of structural response in protein cores, Proteins, 2009, 77(3), 499–508 CrossRef CAS PubMed.
- D. H. Bamford, J. M. Grimes and D. I. Stuart, What does structure tell us about virus evolution?, Curr. Opin. Struct. Biol., 2005, 15(6), 655–663 CrossRef CAS PubMed.
- K. E. Medvedev, L. N. Kinch and N. V. Grishin, Functional and evolutionary analysis of viral proteins containing a Rossmann-like fold, Protein Sci., 2018, 27(8), 1450–1463 CrossRef CAS PubMed.
- K. H. Choi, Viral polymerases, Adv. Exp. Med. Biol., 2012, 726, 267–304 CrossRef CAS PubMed.
- W. G. Dougherty, T. D. Parks, S. M. Cary, J. F. Bazan and R. J. Fletterick, Characterization of the catalytic residues of the tobacco etch virus 49 kDa proteinase, Virology, 1989, 172(1), 302–310 CrossRef CAS PubMed.
- A. Sharma and S. P. Gupta, Fundamentals of Viruses and Their Proteases, Viral Proteases Their Inhib., 2017, 1–24 Search PubMed.
- N. Martinez, E. A. Ribeiro Jr., C. Leyrat, N. Tarbouriech, R. W. Ruigrok and M. Jamin, Structure of the C-terminal domain of lettuce necrotic yellows virus phosphoprotein, J. Virol., 2013, 87(17), 9569–9578 CrossRef CAS PubMed.
- T. J. Green and M. Luo, Structure of the vesicular stomatitis virus nucleocapsid in complex with the nucleocapsid-binding domain of the small polymerase cofactor, Proc. Natl. Acad. Sci. U. S. A., 2009, 106(28), 11713–11718 CrossRef CAS PubMed.
- A. Díaz-Valle, J. M. Falcón-González and M. Carrillo-Tripp, Hot Spots and Their Contribution to the Self-Assembly of the Viral Capsid: In Silico Prediction and Analysis, Int. J. Mol. Sci., 2019, 20(23), 5966 CrossRef PubMed.
- V. C. Darapaneni, J. Sakhamuri and V. Darapaneni, Conservation Analysis of HIV-1 Protein Sequences Reveal Potential Drug Binding Sites: A Case of Viral Protein U and Protease, Am. J. Curr. Virol., 2015, 1(1), 1–12 Search PubMed.
- S. Chaudhuri, J. A. Symons and J. Deval, Innovation and trends in the development and approval of antiviral medicines: 1987-2017 and beyond, Antiviral Res., 2018, 155, 76–88 CrossRef CAS PubMed.
- H. Dong, B. Zhang and P. Y. Shi, Flavivirus methyltransferase: a novel antiviral target, Antiviral Res., 2008, 80(1), 1–10 CrossRef CAS PubMed.
- J. H. Strauss and E. G. Strauss, The alphaviruses: gene expression, replication, and evolution, Microbiol. Rev., 1994, 58(3), 491–562 CrossRef CAS PubMed.
-
S. Tomar and M. Aggarwal, Structure and Function of Alphavirus Proteases, in Viral Proteases and Their Inhibitors, ed. S. P. Gupta, Academic Press, 2017, ch. 5, pp. 105–135 Search PubMed.
- A. Lulla, V. Lulla, K. Tints, T. Ahola and A. Merits, Molecular determinants of substrate specificity for Semliki Forest virus nonstructural protease, J. Virol., 2006, 80(11), 5413–5422 CrossRef CAS PubMed.
- A. T. Russo, M. A. White and S. J. Watowich, The crystal structure of the Venezuelan equine encephalitis alphavirus nsP2 protease, Structure, 2006, 14(9), 1449–1458 CrossRef CAS PubMed.
- F. Abu Bakar and L. F. P. Ng, Nonstructural Proteins of Alphavirus-Potential Targets for Drug Development, Viruses, 2018, 10(2), 71 CrossRef PubMed.
- L. Tong, Viral proteases, Chem. Rev., 2002, 102(12), 4609–4626 CrossRef CAS PubMed.
- I. Schechter and A. Berger, On the size of the active site in proteases. I. Papain, Biochem. Biophys. Res. Commun., 1967, 27(2), 157–162 CrossRef CAS PubMed.
- K. Nakamura, Y. Someya, T. Kumasaka, G. Ueno, M. Yamamoto and T. Sato,
et al., A norovirus protease structure provides insights into active and substrate binding site integrity, J. Virol., 2005, 79(21), 13685–13693 CrossRef CAS PubMed.
-
Z. Li, J. Zhang and H. LiFlavivirus NS2B/NS3 Protease: Structure, Function, and Inhibition, in Viral Proteases and Their Inhibitors, ed. S. P. Gupta, Academic Press, 2017, ch. 7, pp. 163–188 Search PubMed.
- T. J. Chambers, A. Nestorowicz, S. M. Amberg and C. M. Rice, Mutagenesis of the yellow fever virus NS2B protein: effects on proteolytic processing, NS2B-NS3 complex formation, and viral replication, J. Virol., 1993, 67(11), 6797–6807 CrossRef CAS PubMed.
- W. Y. Phong, N. J. Moreland, S.
P. Lim, D. Wen, P. N. Paradkar and S. G. Vasudevan, Dengue protease activity: the structural integrity and interaction of NS2B with NS3 protease and its potential as a drug target, Biosci. Rep., 2011, 31(5), 399–409 CrossRef PubMed.
- P. Soultanas and D. B. Wigley, Unwinding the 'Gordian knot' of helicase action, Trends Biochem. Sci., 2001, 26(1), 47–54 CrossRef CAS PubMed.
- M. R. Singleton, M. R. Sawaya, T. Ellenberger and D. B. Wigley, Crystal structure of T7 gene 4 ring helicase indicates a mechanism for sequential hydrolysis of nucleotides, Cell, 2000, 101(6), 589–600 CrossRef CAS PubMed.
- B. Sikora, Y. Chen, C. F. Lichti, M. K. Harrison, T. A. Jennings and Y. Tang,
et al., Hepatitis C virus NS3 helicase forms oligomeric structures that exhibit optimal DNA unwinding activity in vitro, J. Biol. Chem., 2008, 283(17), 11516–11525 CrossRef CAS PubMed.
- D. N. Frick and A. M. Lam, Understanding helicases as a means of virus control, Curr. Pharm. Des., 2006, 12(11), 1315–1338 CrossRef CAS PubMed.
- A. E. Gorbalenya and E. V. Koonin, Helicases: amino acid sequence comparisons and structure-function relationships, Curr. Opin. Struct. Biol., 1993, 3(3), 419–429 CrossRef CAS.
- D. N. Frick, The hepatitis C virus NS3 protein: a model RNA helicase and potential drug target, Curr. Issues Mol. Biol., 2007, 9(1), 1–20 CAS.
- H. S. Subramanya, L. E. Bird, J. A. Brannigan and D. B. Wigley, Crystal structure of a DExx box DNA helicase, Nature, 1996, 384(6607), 379–383 CrossRef CAS PubMed.
- A. D. Kwong, B. G. Rao and K. T. Jeang, Viral and cellular RNA helicases as antiviral targets, Nat. Rev. Drug Discovery, 2005, 4(10), 845–853 CrossRef CAS PubMed.
- J. E. Darnell, Jr., Transcription units for mRNA production in eukaryotic cells and their DNA viruses, Prog. Nucleic Acid Res. Mol. Biol., 1979, 22, 327–353 Search PubMed.
- D. R. Schoenberg and L. E. Maquat, Re-capping the message, Trends Biochem. Sci., 2009, 34(9), 435–442 CrossRef CAS PubMed.
- F. Ferron, E. Decroly, B. Selisko and B. Canard, The viral RNA capping machinery as a target for antiviral drugs, Antiviral Res., 2012, 96(1), 21–31 CrossRef CAS PubMed.
- Y. Zhou, D. Ray, Y. Zhao, H. Dong, S. Ren and Z. Li,
et al., Structure and function of flavivirus NS5 methyltransferase, J. Virol., 2007, 81(8), 3891–3903 CrossRef CAS PubMed.
- D. Ray, A. Shah, M. Tilgner, Y. Guo, Y. Zhao and H. Dong,
et al., West Nile virus 5′-cap structure is formed by sequential guanine N-7 and ribose 2′-O methylations by nonstructural protein 5, J. Virol., 2006, 80(17), 8362–8370 CrossRef CAS PubMed.
- R. Zust, L. Cervantes-Barragan, M. Habjan, R. Maier, B. W. Neuman and J. Ziebuhr,
et al., Ribose 2′-O-methylation provides a molecular signature for the distinction of self and non-self mRNA dependent on the RNA sensor Mda5, Nat. Immunol., 2011, 12(2), 137–143 CrossRef PubMed.
- S. Daffis, K. J. Szretter, J. Schriewer, J. Li, S. Youn and J. Errett,
et al., 2′-O methylation of the viral mRNA cap evades host restriction by IFIT family members, Nature, 2010, 468(7322), 452–456 CrossRef CAS PubMed.
- S. Koyama, K. J. Ishii, C. Coban and S. Akira, Innate immune response to viral infection, Cytokine, 2008, 43(3), 336–341 CrossRef CAS PubMed.
- J. H. Strauss and E. G. Strauss, Overview of Viruses and Virus Infection, Viruses and Human Disease, 2008, 1–33 Search PubMed.
- S. Venkataraman, B. V. L. S. Prasad and R. Selvarajan, RNA Dependent RNA Polymerases: Insights from Structure, Function and Evolution, Viruses, 2018, 10(2), 76 CrossRef PubMed.
- T. A. Steitz, A mechanism for all polymerases, Nature, 1998, 391(6664), 231–232 CrossRef CAS PubMed.
- B. Shu and P. Gong, Structural basis of viral RNA-dependent RNA polymerase catalysis and translocation, Proc. Natl. Acad. Sci. U. S. A., 2016, 113(28), E4005–14 CrossRef CAS PubMed.
- J. Cerny, B. Cerna Bolfikova, J. J. Valdes, L. Grubhoffer and D. Ruzek, Evolution of tertiary structure of viral RNA dependent polymerases, PLoS One, 2014, 9(5), e96070 CrossRef PubMed.
- H. Jia and P. Gong, A Structure-Function Diversity Survey of the RNA-Dependent RNA Polymerases From the Positive-Strand RNA Viruses, Front. Microbiol., 2019, 10, 1945 CrossRef PubMed.
- E. Muhlberger, Filovirus replication and transcription, Future Virol., 2007, 2(2), 205–215 CrossRef PubMed.
- A. J. te Velthuis, Common and unique features of viral RNA-dependent polymerases, Cell. Mol. Life Sci., 2014, 71(22), 4403–4420 CrossRef CAS PubMed.
- V. Devadasan, S. Udhayasuriyan Malar, M. Udhayakumar, R. Kutumba and R. Ramaiah, Sangeetha. Structure-Based Discovery of Anti-Viral Compounds for Hepatitis B & C, Human Immunodeficiency, and Dengue Viruses, Curr. Bioinf., 2012, 7(2), 187–211 CrossRef.
- G. I. Makrynitsa, M. Lykouras, G. A. Spyroulias and M.-T. Matsoukas, In silico Drug Design, eLS, 2018, 1–7 Search PubMed.
- M. S. Murgueitio, M. Bermudez, J. Mortier and G. Wolber, In silico virtual screening approaches for anti-viral drug discovery, Drug Discovery Today Technol., 2012, 9(3), e219–e225 CrossRef CAS PubMed.
- R. E. Amaro, J. Baudry, J. Chodera, O. Demir, J. A. McCammon and Y. Miao,
et al., Ensemble Docking in Drug Discovery, Biophys. J., 2018, 114(10), 2271–2278 CrossRef CAS PubMed.
- T. Liu, D. Lu, H. Zhang, M. Zheng, H. Yang and Y. Xu,
et al., Applying high-performance computing in drug discovery and molecular simulation, Natl. Sci. Rev., 2016, 3(1), 49–63 CrossRef PubMed.
- W. Li, X. Kou, J. Xu, W. Zhou, R. Zhao and Z. Zhang,
et al., Characterization of Hepatitis C Virus Core Protein Dimerization by Atomic Force Microscopy, Anal. Chem., 2018, 90(7), 4596–4602 CrossRef CAS PubMed.
- G. A. Van Norman, Drugs, Devices, and the FDA: Part 1: An Overview of Approval Processes for Drugs, JACC Basic Transl Sci, 2016, 1(3), 170–179 CrossRef PubMed.
- G. Hessler and K. H. Baringhaus, Artificial Intelligence in Drug Design, Molecules, 2018, 23(10), 2520 CrossRef PubMed.
- H. C. S. Chan, H. Shan, T. Dahoun, H. Vogel and S. Yuan, Advancing Drug Discovery via Artificial Intelligence, Trends Pharmacol. Sci., 2019, 40(10), 801 CrossRef CAS PubMed.
- M. Olivecrona, T. Blaschke, O. Engkvist and H. Chen, Molecular de-novo design through deep reinforcement learning, J. Cheminfor., 2017, 9(1), 48 CrossRef PubMed.
- E. Callaway, ‘It will change everything’: DeepMind's AI makes gigantic leap in solving protein structures, Nature, 2020, 588(7837), 203–204 CrossRef CAS PubMed.
|
This journal is © The Royal Society of Chemistry 2021 |
Click here to see how this site uses Cookies. View our privacy policy here.