Role of structural rigidity and collective behaviour in the molecular design of gas hydrate anti-agglomerants†
Received
29th December 2020
, Accepted 14th April 2021
First published on 14th April 2021
Abstract
Anti-agglomerants (AAs) are surface active molecules widely used in the petroleum industry, among others. It is believed that AAs strongly adsorb onto the surface of hydrate particles to prevent the growth of clathrate hydrate within oil pipelines. Small changes in their molecular structures can strongly affect the thermodynamic and kinetic stability of the system as a whole. Here we employ molecular dynamics simulations to study the interplay between the modification of the molecular structure, rigidity and collective effects of AAs designed to prevent hydrate agglomeration under the conditions encountered in rocking cell experiments. The AAs are surface-active compounds with a complex hydrophilic head and three hydrophobic tails whose structural rigidity is enhanced with the attachment of an aromatic group. Extrapolating from our simulation results, we predict that the aromatic group can positively or negatively affect the performance of the AAs, depending on its location along the hydrophobic tail. Our approach is based on first quantifying the molecular mechanisms responsible for the macroscopic performance and then altering the AA molecular structure to amplify said molecular mechanisms. Although the mechanisms at play depend on the application, the methodology implemented could be applicable to other high-tech industries, where the agglomeration of small particles must be controlled.
Design, System, Application
Gas hydrates have attracted significant practical attention over the last decade due to their possible application in various high-tech and sustainable technologies. Among available strategies to control and sometimes prevent hydrate growth is the use of surface-active low-molecular-weight compounds, known as anti-agglomerants (AAs). Towards designing novel synthetic AAs for hydrate management, it is crucial to better understand the relationship between molecular-level properties and collective effects at the hydrate–oil interface. To aid future system design and engineering for such systems, we conduct computational studies to quantify the molecular driving forces responsible for the transport of methane molecules across interfacial AA layers. We focus on AAs with a complex hydrophilic head and three hydrophobic tails whose structural rigidity is selectively enhanced with the attachment of a simple aromatic group. Our results yield a comprehensive and strategic approach to design new synthetic AAs based on the study of the transport properties of solute molecules through the AA layers. They suggest that a balance between the molecular structural rigidity and collective effects of AA layers is essential not only to continue to improve flow assurance but also to develop other high-tech technologies, where the agglomeration of small particles must be controlled.
|
1 Introduction
Gas hydrates, also known as clathrate hydrates, are ice-like inclusion compounds consisting of polyhedral hydrogen-bonded water cages stabilized by guest gas molecules.1–3 They are formed under high-pressure and low-temperature conditions such as those found in deep oceans and pipelines.4 Clathrate hydrates are relevant in a variety of scientific and industrial contexts, including climate change modeling,5 carbon dioxide sequestration,6 hydrocarbon extraction,7 hydrogen and natural gas storage,7–9 separation and refrigeration technologies,10 marine biology,11 and planetary surface chemistry.12 Of particular interest here are the hydrocarbon hydrates that can form blockages in oil and gas pipelines. This phenomenon can severely affect the safety of pipeline transport, potentially leading to large negative environmental consequences.1,3,13,14
Traditional gas hydrate management methods, including the injection of thermodynamic hydrate inhibitors,7 dehydration,15 and others,14 attempt to avoid hydrate formation. The focus has recently shifted towards allowing hydrates to form, but preventing the formation of large hydrates plugs. Within this strategy, low dosage hydrate inhibitors,2 such as kinetic hydrate inhibitors and surfactant anti-agglomerants (AAs), do not influence the thermodynamics of hydrate formation but affect its kinetics. AAs are optimized to prevent hydrate plug formation in flow assurance.2,16–18 AAs are believed to adsorb on hydrate particles by their hydrophilic head groups, while the AA tail groups are soluble in the hydrocarbon phase. The hydrate particles are expected to be covered by a film of AAs and oil, making them repel each other and remain dispersed.19–23 Laboratory and field observations alike show that many phenomena determine the performance of AAs. For instance, small changes in the molecular structure of the surfactants can strongly affect their ability to prevent hydrate plug formation.23 Phan et al. showed that the adhesion of AAs on hydrate particles is strongly dependent on the molecular features of the AA headgroups, thus explaining the experimental observations.19 Trout and his group showed that the adhesion between monomers and hydrate surfaces is related to the performance of kinetic hydrate inhibitors,24 and recent results show that the adsorption of AAs at the hydrate–water interface is strongly affected by the presence of salts in the system.25,26 To gain better predictive control of how AAs adsorb at solid–liquid interfaces characterized by surface roughness and chemical heterogeneity at the molecular scale, computation-accelerated design has become essential in unraveling the complexity of relevant interfacial phenomena. For example, modelling can help quantify how much AAs adsorb on nanostructured surfaces and how AA aggregates vary as the degree of lateral confinement changes.27 Of particular interest in the present work is the fact that the stability of water-in-oil emulsions is dependent on the rigidity of the interfacial film, which in turn can be determined by the chemical structure and the collective effects of the AAs.20,22,23
Here, we employ molecular dynamics (MD) simulations and enhanced sampling techniques to study the molecular-level properties along with the thermodynamic and kinetic information of AAs specifically designed to prevent hydrate agglomerations under the conditions encountered in rocking cell experiments. The AAs considered are based on a compound, recently developed, which has shown good laboratory performance in preventing hydrate formation in light oils.20,22,23 The molecular structure of the AA tail groups was computationally modified to improve the rigidity of the compound, while maintaining the ability of the polar head group to adsorb on the hydrate surface. We analyse the behaviour of the newly designed AAs at the hydrate–oil interfaces (Fig. 1), in terms of density profiles and preferential orientation, and quantify the thermodynamic and kinetic properties associated with the transport of the methane molecules across the AA film. Details regarding the simulation models and algorithms are reported in the Methods section. Based on our hypothesis according to which the flexibility of the interfacial film is related to macroscopic performance, we observe that, at AA densities similar to those considered in rocking cell experiments, the aromatic group can positively or negatively affect the performance of the AAs depending on its location along the hydrophobic tail. In particular, collective and synergistic effects between the new AAs and the liquid hydrocarbon can yield a free energy (FE) barrier for methane transport through the surfactant layer, whose height is significantly higher than the one computed for synthetic AAs used in the oil and gas industry. Based on our interpretation of the simulation results, this suggests that the engineered AAs could have much higher performance in preventing methane capture and thereby the formation of hydrate plugs than existing synthetic AAs, which should result in good performance in preventing hydrate formation in light oils. Experimental verification is required to test our expectations.
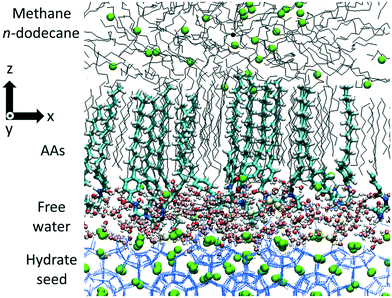 |
| Fig. 1 Representative simulation snapshot of the AA layer at the hydrate–oil interface obtained after equilibration for an AA surface density ≈0.67 molecules per nm2. Green spheres represent methane molecules either in the sII methane hydrate or in the hydrocarbon phase. Blue dotted lines represent water molecules in the hydrate substrate. Silver lines represent n-dodecane molecules, either in the bulk or trapped within the AA layer. Yellow, red, blue, white, and cyan spheres represent chloride ions, and oxygen, nitrogen, hydrogen, and carbon atoms in AA molecules, respectively. Only half of the simulation box is shown here for clarity. | |
2 Methods
2.1 Simulation details
MD simulations are performed with the GROMACS software package, version 5.1.4 (ref. 28) using the TIP4P/ice water model.29–33 Biased simulations are performed using version 2.3 of the plugin for free energy (FE) calculations, PLUMED.34 The simulation methods are obtained from previous studies20,22 and further details on the parameters can be found there. Methane and n-dodecane are represented within the united-atom version of the TraPPE-UA force field.35 AAs are modeled using the general Amber force field (GAFF).36 Atomic charges are calculated with the AM1-BCC method employed in Antechamber from the Amber 14 suite.37 The chloride counterions (Cl−) are modeled as charged Lennard-Jones (LJ) spheres with the potential parameters taken from Dang,38 without polarizability. The sII hydrates are considered to be solid, and they are not allowed to vibrate. AAs, chloride counterions, n-dodecane, and methane compose the liquid phase. Dispersive and electrostatic interactions are modeled by the 12-6 LJ and Coulombic potentials, respectively. The Lorentz–Berthelot mixing rules39,40 are applied to determine the LJ parameters for unlike interactions from the parameters of the pure components. The distance cutoff for all non-bonded interactions is set to 1.4 nm. Long-range corrections to the electrostatic interactions are described using the particle mesh Ewald (PME) method41–43 with a Fourier grid spacing of 0.12 nm, a tolerance of 10−5, and fourth-order interpolation. Periodic boundary conditions are applied in three dimensions for all simulations.
2.2 Unbiased MD simulations
To construct the initial configurations, we follow the procedure described in previous work.20,22 The sII methane hydrate is chosen to represent the features of the experimental system considered, in which a small amount of gases other than methane is present. The underlying assumption is that the host gas does not affect the properties of the AA film, which is the subject matter of this investigation. One unit cell of sII methane hydrates is adapted from the study of Takeuchi et al.44 The sII methane hydrate unit cell is replicated three times in the X and Y directions (5.193 nm) and two times in the Z direction (3.462 nm). It is then flanked by a thin liquid water film of approximately 0.5 nm on both sides along the Z direction. This represents a quasi-liquid interfacial layer of water molecules, which remains disordered at the hydrate–hydrocarbon interface under the temperature and pressure conditions chosen in this study, as identified in the experiments of Aman and coworkers.45 The desired number of AA molecules is arranged near both sides of the hydrate substrate. The chloride counterions (Cl−) are placed next to the AA headgroups. The n-dodecane and methane molecules are placed within the remainder of the simulation box. The time step used in all the simulations is 0.001 ps, and the list of neighbors is updated every 0.01 ps with the grid method and a cutoff radius of 1.4 nm. It should be noted that no free water dispersed through the hydrocarbon phase is considered here. This perhaps could limit the applicability of the simulation results to “low water cut” conditions.
The initial configuration is first relaxed using the steepest descent minimization algorithm to remove high-energy configurations, which might be related to steric hindrance between AAs and the hydrocarbon phase. During this step, the gas hydrate structure remains unaltered. Subsequently, to minimize the possibility that the initial configuration biased the simulation results, an NVT temperature-annealing procedure, as implemented in GROMACS,28 is conducted. The algorithm linearly decreases the system temperature from 1000 K to 277 K in 500 ps. In these simulations, the hydrate substrate and chloride ions are kept fixed in position. To relax the structure of n-dodecane and AAs, an NVT simulation is then conducted at 277 K for 2 ns using the Berendsen thermostat,46 with the sII hydrate structure kept fixed in position. The equilibration phase is then conducted within the isobaric–isothermal (NPT) ensemble under thermodynamic conditions favorable for hydrate formation (T = 277 K and P = 20 MPa) to equilibrate the fluid density. During the NPT simulation, all molecules in the system are allowed to move, including water and methane in the hydrate substrate. The pressure coupling is applied only along the Z direction of the simulation box, which allows the X and Y dimensions to be maintained constant. The temperature and pressure are maintained at 277 K and 20 MPa, respectively, using the Berendsen thermostat and barostat46,47 for 5 ns. We then switch to the Nose–Hoover thermostat48 and the Parrinello–Rahman barostat49 for 100 ns, which are considered more thermodynamically consistent algorithms.47 This numerical protocol allows the AAs to assemble and orient to form the interfacial layer depicted in Fig. 1.20,22 The system is then equilibrated for 3 ns under NVT conditions coupling with the v-rescale thermostat50 (T = 277 K, τT = 0.1 ps). The simulation is then continued under NVT conditions holding in place the methane molecule enclathrated into the water cages defining the sII hydrate structure. One representative configuration of the whole system obtained after equilibration and two sequences of simulation snapshots showing the behaviour of the system for 2 AA concentrations are given in the ESI.†
2.3 Biased MD simulations
The phenomenon of interest (i.e. the transport of methane across the interfacial layer) occurs on time scales that are orders of magnitude longer than those accessible with classical MD simulations.20,22 To overcome this limitation, we use the numerical method based on constrained MD employed by Sicard and coworkers,22,51 which combined the adiabatic biased molecular dynamics (ABMD)52–55 and umbrella sampling (US)56 frameworks. To design the US windows, we use the projection of the Cartesian position of the methane molecules along the Z-direction as a reaction coordinate (RC). The starting configurations for the US simulations are obtained by pulling adiabatically the system along the RC, generating 40 windows. Each US window is subsequently run for 2 ns to allow equilibration, followed by additional 16 ns of the production run. To control the accuracy of the sampling with respect to the RC orthogonal degrees of freedom (either the X- or Y-direction), we implement the flat-bottomed potential,57–59 as already described in previous work.22 Upon completion of the US simulations, we obtain the free energy profile (FEP) associated with the transport of the methane molecules across the AA film using the dynamic histogram analysis method (DHAM).60 This unbiasing method uses a maximum likelihood estimate of the Markov state model (MSM) transition probabilities given the observed transition counts during each biased trajectory. To produce MSMs from the biased simulations, we discretize the RC into bins and count the number of transitions between each pair of bins i and j in the US simulation k at the chosen lag time t, Ckij(t), as well as the number of times each bin is occupied during each US simulation k,
. These values provide the conditional probabilities Mij(t) = P(j,t|i,0). For biased simulations, where a biasing energy of uki is applied to state i during simulation k, we compute the unbiased MSM from the biased data, as given by | 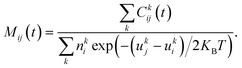 | (1) |
Once the MSM is constructed from the simulation data, the equilibrium probabilities are calculated as the eigenvector corresponding to eigenvalue 1 of the transition matrix Mij obtained in eqn (1). To estimate the position-dependent diffusion coefficient associated with the methane transport across the interfacial layer, we extend the standard scope of the US framework considering the method developed by Berne and co-workers61 and elaborated by Hummer,62 where the diffusion coefficient is calculated from the position autocorrelation function (PACF) obtained from harmonically restrained simulations | 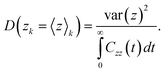 | (2) |
In eqn (2), 〈z〉k is the average of the RC in the US window k, var(z) = 〈z2〉 − 〈z〉2 is its variance, and Czz(t) = 〈δz(0)δz(t)〉 is the PACF calculated directly from the time series.
3 Results and discussion
The two AA molecular structures considered throughout this work are shown in Fig. 2. The short tail R2 is a linear hydrocarbon of four carbon atoms (n-butyl). The headgroup including both the amide and tertiary ammonium cation group is known for its ability to adsorb on the hydrate surface.20–23 The two long R1 tails are composed of a linear hydrocarbon of twelve carbon atoms with an aromatic ring (benzene) positioned either at the bottom (Fig. 2b) or in the middle (Fig. 2c) of the chain. The aromatic ring is intended to rigidify the AA tails thus providing significantly stronger mechanical strength and thermal stability.63 The simulations are run at the temperature and pressure maintained at 277 K and 20 MPa, similar to those encountered in laboratory experiments.20 Thus, the conditions chosen in this study are well within the gas hydrate stability zone and correspond to subcooled systems.21
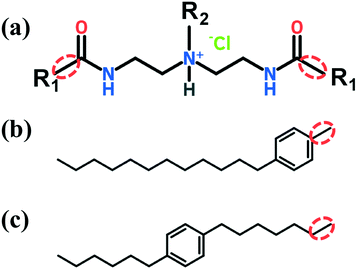 |
| Fig. 2 (a) Molecular structure of the AAs considered in this work, which contain a headgroup including both amide and tertiary ammonium cation groups, two hydrophobic tails R1, and one short hydrophobic tail R2. The R1 tail is composed of a linear hydrocarbon of twelve carbon atoms with an aromatic ring (benzene) positioned either at the bottom (b) or in the middle (c) of the chain. The short tail R2 is composed of a linear hydrocarbon of four carbon atoms (n-butyl). The bond connecting the headgroup to the long tails is highlighted in red. | |
3.1 Visual observation of simulation snapshots
We first consider in Fig. 3 the AA structure given in Fig. 2b, where the aromatic ring is positioned at the bottom of the tail R1. Representative simulation snapshots of the AA configurations adsorbed on the hydrate surface are shown in Fig. 3b at low (0.33 molecules per nm2) and high (0.67 molecules per nm2) surface densities. The snapshots are taken at the end of our simulations. We observe that the headgroups of the AAs adsorb on the hydrate surface, as noticed previously.20–23 The long tails of AAs are instead more likely to extend towards the alkane bulk phase. The snapshots shown in Fig. 3b suggest that different AA densities yield differences in the thin-film structure. The increase of the AA surface density yields a transition between the disordered and ordered orientation of the AA long tails with a thin film within which the long tails of AAs and n-dodecane align parallel to each other and orient perpendicularly to the hydrate surface. This transition comes with the configurational change of the n-dodecane molecules from a gauche conformation in the bulk phase to a nearly all-trans conformation within the AA film (cf. details in the ESI†).
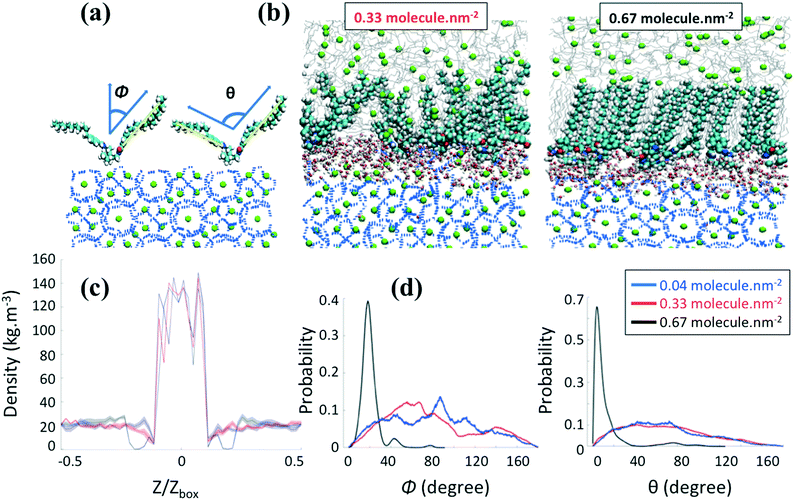 |
| Fig. 3 (a) Schematic representation of the orientational angle Φ formed by the vector connecting the first to the last carbons of the hydrocarbon tails and the surface normal (Z direction) and the conformational angle θ between two long tails of one AA molecule. (b) Representative simulation snapshots for the system containing AAs with the aromatic ring positioned at the bottom of the hydrophobic tails R1 at two surface densities. Methane, green spheres; n-dodecane, silver lines; water connected by hydrogen bonds, blue lines; chloride ions, yellow spheres. AAs and free water: hydrogen, carbon, oxygen, and nitrogen atoms are represented by white, cyan, red, and blue spheres, respectively. (c) Density profiles of methane along the Z direction of the simulation box and (d) probability distributions of orientational (Φ) and conformational (θ) angles at increasing AA surface density. | |
Although changing the position of the aromatic ring from the bottom to the middle in the long tails does not seem to affect the ability of the AAs to adsorb on the hydrate surface, it significantly affects the ordering of the thin film, even at the relatively high surface density (0.67 molecule nm−2) studied here, as shown in Fig. 4a. The lack of ordering of the AA film also comes with n-dodecane molecules in a gauche conformation either in the bulk phase or within the surfactant layer.
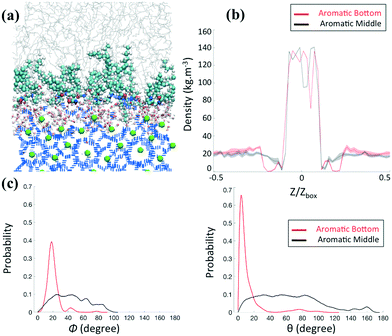 |
| Fig. 4 (a) Representative simulation snapshot for the system containing AAs with the aromatic ring positioned in the middle of the hydrophobic long tails at high surface density (0.67 molecule nm−2). The density profiles of the methane along the Z direction (b) and the probability distributions of orientational (left) and conformational (right) angles, as defined in Fig. 3, (c) at high density for systems composed of AAs with the aromatic ring positioned at the bottom (red) or in the middle (black) of the hydrophobic long tails are compared. | |
3.2 Density profiles
To quantify the influence of AAs on the distribution of methane in the system, we calculate the mass density profiles along the z-axis of the simulation box for increasing AA surface density. In the following, Z and Zbox represent the projection of the Cartesian position of the methane molecules and the size of the simulation box along the z-axis, respectively. Fig. 3c and 4b show a high and constant density between Z/Zbox = −0.1 and Z/Zbox = 0.1, which corresponds to the methane molecules trapped in the hydrate cages. For Z/Zbox > 0.35 and Z/Zbox < −0.35 the results show a uniform density representative of the fluid hydrocarbon phase. The methane density profile in the thin region between the layer of AA headgroups and the bulk liquid hydrocarbon phase shows a pronounced dependence on the AA type and surface density. At low AA surface densities, the density of methane near the hydrate surface for systems containing both AAs (Fig. 3c and 4b) is similar to those found in the bulk. When the aromatic ring is positioned at the bottom of the AA long tails (Fig. 3c), the results show a pronounced depletion of methane at the interface (Z/Zbox < 0.35) as the AA surface density increases to 0.67 molecules per nm2, with the density profile being nearly 0. Combined with a visual observation of the simulation snapshots, these results suggests that the ordered AA layer successfully expels methane from the interfacial region. This phenomenon is similar to the one discussed in previous work for synthetic AAs used in the oil and gas industry,20,22 which was explained by the collective and synergistic effects between AAs and the hydrocarbon phase.
3.3 AA orientation
To quantify the orientation of AAs at the interface, we consider the orientational angle, ϕ, formed between each tail and the direction perpendicular to the hydrate surface. We calculate the probability distribution of this angle as well as that of the conformational angle, θ, between the two long tails of one AA molecule. In Fig. 3d and 4c, we report the probability distribution of the orientational and configurational angles for the two AA structures represented in Fig. 2b and c at various surface densities. At low surface density, the orientational angle shows a wide probability distribution, from 0 to 90° and above, irrespective of the position of the aromatic ring in the hydrophobic long tail. Similarly, the conformational angle does not show preferential values at low surface coverage for either AA. These results suggest that the AAs are rather disordered. When the AA surface density increases, the results show significant variations. While the results obtained for the compound with the aromatic ring positioned in the middle of the long tails do not show substantial changes compared to those obtained at low surface density (Fig. 4c), the compound with the aromatic ring at the bottom shows a pronounced order. As shown in Fig. 3d, the orientational distribution shows a narrow peak at Φ ≈ 20° when the surface density increases to 0.67 molecules per nm2, suggesting that the AA tails become almost perpendicular to the hydrate surface. At the same surface density, the conformational distribution shows a pronounced peak at θ ≈ 10°, suggesting that the AAs maintain their long tails almost parallel to each other.
As the only difference between the two AA structures simulated is the position of the aromatic ring in the hydrophobic tail, the differences highlighted in Fig. 3 and 4 are likely due to the collective effects and preferential interactions between the AA long tail and the hydrocarbon molecules in the fluid phase due to attractive chain–chain lateral van der Waals interactions.
3.4 Thermodynamic properties
We quantify the thermodynamic characteristics of the AA layer via the free energy profile (FEP) associated with the transport of the methane molecules. Following previous work,22 we focus on the diffusion of methane through an interfacial region made up of the largest cluster of hydrocarbons (characteristic size ≈20 Å). In this situation, the methane molecules interact mainly with hydrocarbon molecules trapped in the AA film. Fig. 5a shows the FEP obtained within the US/ABMD framework,22,51 and using the dynamic histogram analysis method (DHAM)60 plotted along the reduced units, Z/Zbox. Uncertainties were determined by dividing the data into four equal sections, determining the profiles independently, and calculating the standard error. We measured a difference in FE between the global (Z/Zbox ≈ 0.36) and local (Z/Zbox ≈ 0.26) minima ΔF0 ≈ 8.5 kJ mol−1. These two basins are well separated by activation energies associated with methane capture and escape, ΔFC ≈ 35 kJ mol−1 and ΔFE ≈ 26.5 kJ mol−1, respectively. As shown in Fig. 5c, the free methane molecule is initially in the bulk hydrocarbon phase, above the AA layer. When it comes closer to the interface, it is first trapped in a FE minimum (Z/Zbox ≈ 0.36). This minimum corresponds to a transition region between oil molecules isotropically oriented in the bulk and oil molecules parallel to the AA tails. The methane molecule then enters the interfacial film. As the methane travels farther across the interfacial layer, an energy barrier arises as the oil molecules are displaced from the methane pathway. The transport proceeds until one oil molecule cannot be pushed farther down (0.31 ≤ Z/Zbox ≤ 0.36). Under these conditions, the oil molecule bends, eventually forming a cage surrounding the methane (Z/Zbox ≈ 0.31). This corresponds to the high-energy transition region in the FEP. Once the methane molecule overcomes this transition state, it is pushed underneath the AA layer. The methane molecule then reaches the FE minimum corresponding to the water layer between the AA layer and the hydrate (Z/Zbox ≈ 0.26).
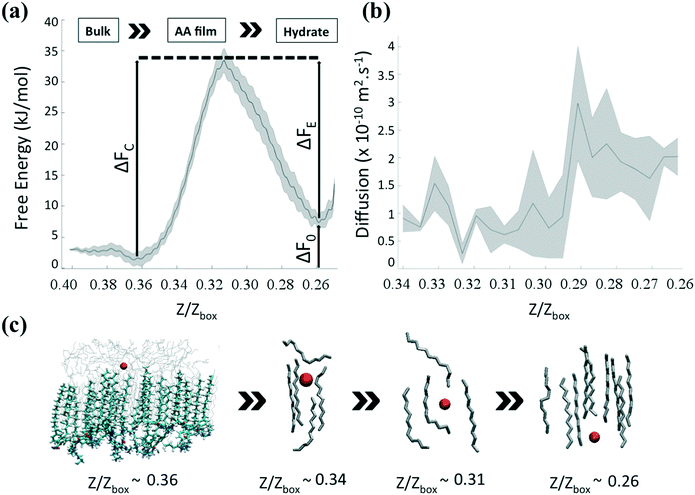 |
| Fig. 5 (a) FEP associated with the transport of the free methane molecule from the hydrocarbon phase (Z/Zbox > 0.36), across the interfacial layer (0.26 < Z/Zbox < 0.36), to the aqueous film near the hydrate (Z/Zbox < 0.26), obtained within the US/ABMD framework and calculated with DHAM and 200 bins. The AA surface density is 0.67 molecules per nm2. The x-axis corresponds to the Z-Cartesian coordinate of methane expressed in reduced units, Z/Zbox, with Zbox the size of the simulation box along the Z direction. The activation energies associated with methane capture and escape, ΔFC and ΔFE are ≈35 kJ mol−1 and ≈26.5 kJ mol−1, respectively. Uncertainties are represented by the shaded area. (b) Position-dependent diffusion coefficient calculated from the PACF obtained within the US/ABMD framework. The system shows a diffusion profile with two distinct plateaus at D(Z/Zbox > 0.31) ≈ 0.6 × 10−10 m2 s−1 and D(Z/Zbox < 0.31) ≈ 2.2 × 10−10 m2 s−1 on both sides of the transition state Z/Zbox ≈ 0.31. Uncertainties are represented by the shaded area. (c) Sequence of simulation snapshots representing the transport mechanism of methane (red sphere) across the interfacial layer composed of AAs and hydrocarbons (silver molecules). The bulk hydrocarbon phase and the sII hydrate are not shown for clarity. The AA layer is only shown in the first snapshot. The methane molecule starts in the bulk hydrocarbon phase, above the AA layer (Z/Zbox ≈ 0.36). The methane then enters the interfacial layer between two oil molecules. As the methane goes farther across the interfacial layer, the oil molecules bend (Z/Zbox ≈ 0.34), eventually forming a cage surrounding the methane (Z/Zbox ≈ 0.31). As the methane travels farther down, one oil molecule begins pushing the methane. Eventually, methane is driven underneath the AA film (Z/Zbox ≈ 0.26). | |
3.5 Kinetic properties
To complement the thermodynamic analysis, we estimate the position-dependent diffusion profile, which provides a molecular understanding of the transport of solutes across three-dimensional heterogeneous media.64–66 In our system, the variation of the solute diffusivity can be impacted by the variation of the frictional environment as the solute moves from bulk hydrocarbon through the interface, and into the water layer. In Fig. 5b, we show the position-dependent diffusion profile, D(Z), as given in eqn (2), along the Z direction for methane travelling across the AA layer. Uncertainties were determined by dividing the data into four equal sections, determining the profiles independently, and calculating the standard error. The system shows a diffusion profile with two distinct plateaus located at D(Z/Zbox > 0.31) ≈ 0.6 × 10−10 m2 s−1 and D(Z/Zbox < 0.31) ≈ 2.2 × 10−10 m2 s−1 on both sides of the transition state Z/Zbox ≈ 0.31. When methane enters the interfacial layer, the effective diffusion coefficient is similar to the one measured experimentally in bulk hydrocarbons67 (≈5.10−11 m2 s−1 at 277 K and 20 MPa). As the methane goes farther across the interfacial AA layer, the hydrocarbon molecules surrounding it start to push it underneath the AA layer, increasing the effective diffusion coefficient by almost an order of magnitude.
4 Conclusions
The extensive simulations discussed above highlight the role of the structural rigidity and collective behaviour in the molecular design of new AAs for managing gas hydrates. Based on our simulation results, we studied the molecular-level properties of a new synthetic AA specifically designed in silico to prevent hydrate plug formation in rocking cell experiments. We studied the thermodynamic and kinetic characteristics of the system and quantified the FEP experienced by one methane molecule travelling across the interfacial film along with the position-dependent diffusion coefficient, using a combination of MD simulations, enhanced sampling techniques, and Markov state model analysis. We showed that the FE barrier associated with methane transport across the AA film is due to the collective and synergistic effects between the AAs and the liquid hydrocarbon trapped in the interfacial layer.
Interestingly, the transport properties due to the AAs specifically designed in this work can be compared with those associated with synthetic AAs, which show good laboratory performance in preventing hydrate formation in light oils.20,22 In the latter, the calculated FE of activation for methane capture was significantly lower (≈15 kJ mol−1). This suggests that tuning the molecular rigidity of AAs, while preserving their ability to interact with the hydrocarbon molecules, can significantly improve their collective performance in preventing hydrate formation. To extend the conditions of applicability of the proposed putative AA, one could study how the thermodynamic and kinetic properties of the system are impacted when the ratio of free water over clathrate water increases. One could also consider an initial configuration without methane molecules in the hydrocarbon phase, which would allow researchers to study the role played by the AA layer in clathrate hydrate dissolution.
The results presented here follow from the hypothesis that transport through the AA film determines the AA performance in flow assurance. The computational methodology developed could be useful for a variety of high-tech technologies in the petroleum, rubber latex, ink, and paint and coatings industries, where the agglomeration and flocculation of small particles must be controlled.27,68,69 Although the mechanisms by which AAs are effective depends on the application of interest, several research and industrial groups focus on the interplay between the design and performance of AAs at the microscopic scale.70–75 Accounting for the interplay between the structural rigidity and the collective effects of new synthetic AAs could allow us to infer their use in practical applications, which addresses current industrial needs.
Conflicts of interest
There are no conflicts to declare.
Acknowledgements
FS thanks Jhoan Toro-Mendoza, Denes Berta, and Tai Bui for useful discussions. Via our membership of the UK's HEC Materials Chemistry Consortium, which is funded by EPSRC (EP/L000202), this work used the ARCHER UK National Supercomputing Service (http://www.archer.ac.uk). Financial support was graciously provided by the EPSRC to AS under grant number EP/T004282/1. The project was initiated under the support of EPSRC, via grant number EP/N007123/1.
Notes and references
- C. Koh, R. Westacott, W. Zhang, K. Hirachand, J. Creek and A. Soper, Fluid Phase Equilib., 2002, 194–197, 143–151 Search PubMed.
- M. Kelland, Energy Fuels, 2006, 20, 825–847 Search PubMed.
-
E. Sloan and C. Koh, Clathrate hydrates of natural gases, CRC Press, Boca Raton, Florida, 3rd edn, 2008 Search PubMed.
- P. Brewer, F. M. Orr Jr, G. Friederich, K. Kvenvolden, D. Orange, J. McFarlane and W. Kirkwood, Geology, 1997, 25, 407–410 Search PubMed.
- K. Kaiho, T. Arinobu, R. Ishiwatari, H. Morgans, H. Okada, N. Takeda, K. Tazaki, G. Zhou, Y. Kajiwara, R. Matsumoto, A. Hirai, N. Niitsuma and H. Wada, Paleoceanography, 1996, 11, 447 Search PubMed.
- K. Park, Z. Ni, A. Côté, J. Choi, R. Huang, F. Uribe-Romo, H. Chae, M. O'Keeffe and O. Yaghi, Proc. Natl. Acad. Sci. U. S. A., 2006, 103, 12690 Search PubMed.
- E. Sloan, Nature, 2003, 426, 353 Search PubMed.
- L. Florusse, C. Peters, J. Schoonman, K. Hester, C. Koh, S. Dec, K. Marsh and E. Sloan, Science, 2004, 306, 469 Search PubMed.
- W. Mao, H. Mao, A. Goncharov, V. Struzhkin, Q. Guo, J. Hu, J. Shu, R. Hemley, M. Somayazulu and Y. Zhao, Science, 2002, 297, 2247 Search PubMed.
- T. Ogawa, T. Ito, K. Watanabe, K. I. Tahara, R. Hiraoka, J. I. Ochiai, R. Ohmura and Y. Mori, Appl. Therm. Eng., 2006, 26, 2157 Search PubMed.
- C. Fisher, I. MacDonald, R. Sassen, C. Young, S. Macko, S. Hourdez, R. Carney, S. Joye and E. McMullin, Naturwissenschaften, 2000, 87, 184 Search PubMed.
- D. Milton, Science, 1974, 183, 654 Search PubMed.
- E. Hammerschmidt, Ind. Eng. Chem., 1934, 26, 851–855 Search PubMed.
- A. Striolo, A. Phan and M. Walsh, Curr. Opin. Chem. Eng., 2019, 25, 57–66 Search PubMed.
- A. Aspelund and K. Jordal, Int. J. Greenhouse Gas Control, 2007, 1, 343–354 Search PubMed.
- M. Kelland, T. Svartaas, J. Ovsthus, T. Tomita and J. Chosa, Chem. Eng. Sci., 2006, 61, 4048–4059 Search PubMed.
- M. Kelland, T. Svartaas and L. Andersen, J. Pet. Sci. Eng., 2009, 64, 1–10 Search PubMed.
-
M. Kelland, Production Chemicals for the Oil and Gas Industry, CRC Press, Boca Raton, Florida, 2nd edn, 2014 Search PubMed.
- A. Phan, T. Bui, E. Acosta, P. Krishnamurthy and A. Striolo, Phys. Chem. Chem. Phys., 2016, 18, 24859–24871 Search PubMed.
- T. Bui, A. Phan, D. Monteiro, Q. Lan, M. Ceglio, E. Acosta, P. Krishnamurthy and A. Striolo, Langmuir, 2017, 33, 2263–2274 Search PubMed.
- T. Bui, F. Sicard, D. Monteiro, Q. Lan, M. Ceglio, C. Burress and A. Striolo, J. Phys. Chem. Lett., 2018, 9, 3491–3496 Search PubMed.
- F. Sicard, T. Bui, D. Monteiro, Q. Lan, M. Ceglio, C. Burress and A. Striolo, Langmuir, 2018, 34, 9701–9710 Search PubMed.
- T. Bui, D. Monteiro, L. Vo and A. Striolo, Sci. Rep., 2020, 10, 5496 Search PubMed.
- B. Anderson, J. Tester, G. Borghi and B. Trout, J. Am. Chem. Soc., 2005, 127, 17852–17862 Search PubMed.
- H. Mehrabian and B. Trout, J. Phys. Chem. C, 2020, 124, 18983–18992 Search PubMed.
- F. Jiménez-Ángeles and A. Firoozabadi, ACS Cent. Sci., 2018, 4, 820–831 Search PubMed.
- A. Striolo and B. Grady, Langmuir, 2017, 33, 8099–8113 Search PubMed.
- M. Abraham, T. Murtola, R. Schulz, S. Páll, J. Smith, B. Hess and E. Lindahl, SoftwareX, 2015, 1–2, 19–25 Search PubMed.
- J. Abascal, E. Sanz, R. F. Garcia and C. Vega, J. Chem. Phys., 2005, 122, 234511 Search PubMed.
- M. Walsh, C. Koh, E. Sloan, A. Sum and D. Wu, Science, 2009, 326, 1095–1098 Search PubMed.
- M. Conde and C. Vega, J. Chem. Phys., 2010, 133, 064507 Search PubMed.
- L. Jensen, K. Thomsen, N. von Solms, S. Wierzchowski, M. Walsh, C. Koh, E. Sloan, D. Wu and A. Sum, J. Phys. Chem. B, 2010, 114, 5775–5782 Search PubMed.
- S. A. Bagherzadeh, S. Alavi, J. Ripmeester and P. Englezos, Phys. Chem. Chem. Phys., 2015, 17, 9984–9990 Search PubMed.
- G. Tribello, M. Bonomi, D. Branduardi, C. Camilloni and G. Bussi, Comput. Phys. Commun., 2014, 185, 604–613 Search PubMed.
- M. Martin and J. Siepmann, J. Phys. Chem. B, 1998, 102, 2569–2577 Search PubMed.
- J. Wang, R. Wolf, J. Caldwell, P. Kollman and D. Case, J. Comput. Chem., 2004, 25, 1157–1174 Search PubMed.
-
D. Case, J. Berryman, R. Betz, Q. Cai, D. Cerutti, T. Cheatham, T. Darden, R. Duke, H. Gohlke, A. Goetz, S. Gusarov, N. Homeyer, P. Janowski, J. Kaus, I. Lolossváry, A. Kovalenko, T. Lee, S. LeGrand, T. Luchko, R. Luo, B. Madej, K. Merz, F. Paesani, D. Roe, A. Roitberg, C. Sagui, R. Salomon-Ferrer, G. Seabra, C. Simmerling, W. Smith, J. Swails, R. Walker, J. Wang, R. Wolf, X. Wu and P. Kollman, AMBER 14, University of California, San Francisco, California, 2014 Search PubMed.
- D. Smith and L. Dang, J. Chem. Phys., 1994, 100, 3757–3766 Search PubMed.
- H. Lorentz, Ann. Phys., 1881, 248, 127–136 Search PubMed.
- D. Berthelot, C. R. Acad. Sci., 1898, 126, 1703–1706 Search PubMed.
- T. Darden, D. York and L. Pedersen, J. Chem. Phys., 1993, 98, 10089–10092 Search PubMed.
- U. Essmann, L. Perera and M. Berkowitz, J. Chem. Phys., 1995, 103, 8577 Search PubMed.
- M. Kawata and U. Nagashima, Chem. Phys. Lett., 2001, 340, 165–172 Search PubMed.
- F. Takeuchi, M. Hiratsuka, R. Ohmura, S. Alavi, A. Sum and K. Yasuoka, J. Chem. Phys., 2013, 138, 124504 Search PubMed.
- Z. Aman, E. Brown, E. Sloan, A. Sum and C. Koh, Phys. Chem. Chem. Phys., 2011, 13, 19796–19806 Search PubMed.
- H. Berendsen, J. Postma, W. van Gunsteren, A. DiNola and J. R. Haak, J. Chem. Phys., 1984, 81, 3684–3690 Search PubMed.
- S. Pronk, S. Páll, R. Schulz, P. Larsson, P. Bjelkmar, R. Apostolov, M. Shirts, J. Smith, P. Kasson, D. van der Spoel, B. Hess and E. Lindahl, Bioinformatics, 2013, 29, 845–854 Search PubMed.
- D. Evans and B. Holian, J. Chem. Phys., 1985, 83, 4069 Search PubMed.
- M. Parrinello and A. Rahman, J. Appl. Phys., 1981, 52, 7182–7190 Search PubMed.
- G. Bussi, D. Donadio and M. Parrinello, J. Chem. Phys., 2007, 126, 014101 Search PubMed.
- F. Sicard, J. Toro-Mendoza and A. Striolo, ACS Nano, 2019, 13, 9498–9503 Search PubMed.
- E. Paci and M. Karplus, J. Mol. Biol., 1999, 288, 441–459 Search PubMed.
- M. Marchi and P. Ballone, J. Chem. Phys., 1999, 110, 3697–3702 Search PubMed.
- C. Camilloni, R. Broglia and G. Tiana, J. Chem. Phys., 2011, 134, 045105 Search PubMed.
- F. Sicard and A. Striolo, Faraday Discuss., 2016, 191, 287–304 Search PubMed.
- J. Kastner, WIREs Comput. Mol. Sci., 2011, 1, 932–942 Search PubMed.
- T. Allen, O. Andersen and B. Roux, Proc. Natl. Acad. Sci. U. S. A., 2004, 101, 117–122 Search PubMed.
- Y. Zhang and G. Voth, J. Chem. Theory Comput., 2011, 7, 2277–2283 Search PubMed.
- F. Zhu and G. Hummer, J. Chem. Theory Comput., 2012, 8, 3759–3768 Search PubMed.
- E. Rosta and G. Hummer, J. Chem. Theory Comput., 2015, 11, 276–285 Search PubMed.
- B. Berne, M. Borkovec and J. Straub, J. Phys. Chem., 1988, 92, 3711 Search PubMed.
- G. Hummer, New J. Phys., 2005, 7, 34 Search PubMed.
- A. Mohanty and C. Bae, Adv. Organomet. Chem., 2015, 64, 1–39 Search PubMed.
- C. Lee, J. Comer, C. Herndon, N. Leung, A. Pavlova, R. Swift, C. Tung, C. Rowley, R. Amaro, C. Chipot, Y. Wang and J. Gumbart, J. Chem. Inf. Model., 2016, 56, 721–733 Search PubMed.
- K. Gaalswyk, E. Awoonor-Williams and C. Rowley, J. Chem. Theory Comput., 2016, 12, 5609–5619 Search PubMed.
- F. Sicard, V. Koskin, A. Annibale and E. Rosta, J. Chem. Theory Comput., 2021, 17(4), 2022–2033 Search PubMed.
-
S. Granick, Fundamentals of Friction, Macroscopic and Microscopic Processes, ed. I. L. Singer and H. Pollock, Springer Netherlands, 3rd edn, 1992, pp. 387–401 Search PubMed.
- L. Karlson, M. Olsson, G. Bostrom and L. Piculell, J. Coat. Technol. Res., 2008, 5, 447–454 Search PubMed.
- T. Ardyani, A. Mohamed, S. AbuBakar, M. Sagisaka, Y. Umetsu, M. Mamat, M. Ahmad, H. A. Khalil, S. King, S. Rogers and J. Eastoe, J. Colloid Interface Sci., 2019, 545, 184–194 Search PubMed.
- N. Choudhary, S. Das, S. Roy and R. Kumar, Fuel, 2016, 186, 613–622 Search PubMed.
-
D. Thompson and C. Lund, US20170158827A1, 2018.
-
D. Thompson and C. Lund, US10385200B2, 2019.
-
Q. Lan, D. Monteiro, M. Ceglio, E. Acosta and P. Krishnamurthy, WO2017105507A1, 2017.
-
P. Prince, L. Vo, D. Monteiro, T. Bui and A. Striolo, US20200087566A1, 2020.
-
Q. Lan and D. Monteiro, WO2020068046A1, 2020.
Footnote |
† Electronic supplementary information (ESI) available. See DOI: 10.1039/d0me00174k |
|
This journal is © The Royal Society of Chemistry 2021 |
Click here to see how this site uses Cookies. View our privacy policy here.