DOI:
10.1039/D0LC00801J
(Paper)
Lab Chip, 2021,
21, 122-142
Microdissected “cuboids” for microfluidic drug testing of intact tissues†
Received
7th August 2020
, Accepted 29th October 2020
First published on 11th November 2020
Abstract
As preclinical animal tests often do not accurately predict drug effects later observed in humans, most drugs under development fail to reach the market. Thus there is a critical need for functional drug testing platforms that use human, intact tissues to complement animal studies. To enable future multiplexed delivery of many drugs to one small biopsy, we have developed a multi-well microfluidic platform that selectively treats cuboidal-shaped microdissected tissues or “cuboids” with well-preserved tissue microenvironments. We create large numbers of uniformly-sized cuboids by semi-automated sectioning of tissue with a commercially available tissue chopper. Here we demonstrate the microdissection method on normal mouse liver, which we characterize with quantitative 3D imaging, and on human glioma xenograft tumors, which we evaluate after time in culture for viability and preservation of the microenvironment. The benefits of size uniformity include lower heterogeneity in future biological assays as well as facilitation of their physical manipulation by automation. Our prototype platform consists of a microfluidic circuit whose hydrodynamic traps immobilize the live cuboids in arrays at the bottom of a multi-well plate. Fluid dynamics simulations enabled the rapid evaluation of design alternatives and operational parameters. We demonstrate the proof-of-concept application of model soluble compounds such as dyes (CellTracker, Hoechst) and the cancer drug cisplatin. Upscaling of the microfluidic platform and microdissection method to larger arrays and numbers of cuboids could lead to direct testing of human tissues at high throughput, and thus could have a significant impact on drug discovery and personalized medicine.
Introduction
Preclinical animal tests fall short as predictors of efficacy, toxic doses, and drug metabolism later observed in human trials.1 In its Provocative Questions in Cancer, the National Cancer Institute (NCI) highlights the potential impact of direct-in-human drug testing approaches: “If systems can be developed that accurately predict drug responses in human, advances in drug treatment or prevention would be dramatically streamlined, and time frames for drug development shortened considerably. The ultimate benefit for patients would be immense”.2 Functional assays can potentially complement and extend genomics-based approaches for personalized oncology by capturing key determinants of therapeutic response such as tissue architecture, tumor heterogeneity, and the tumor microenvironment (TME).3 Microfluidic technology is generally well suited for the challenge of delivering minute amounts of drugs to small tissue biopsies, such as live human tissue, which is almost always available only in very limited amounts. We have addressed the NCI Provocative Question by developing a microfluidic platform that miniaturizes functional drug testing on large numbers of live, intact tumor tissue fragments. Here we demonstrate its utility with mouse tumors, but the approach is directly applicable to human tumors.
Researchers have developed diverse functional assay platforms to assess drug responses in live tumor samples. One of the most acute challenges in functional precision cancer medicine arises from the fact that dissociated cells are insufficient for the functional assays – to preserve the TME, these assays should be performed on intact tissue, whose availability is scarce. The small size of clinical samples has prompted many groups (including ours) to use various approaches. The following live tissue assay approaches are listed from less to more preservation and faithful representation of the TME. 1) Tumor spheroids (small spheres or “organoids” formed from patient-derived, dissociated cells4–11) create cell–cell and cell–matrix 3-D interactions that more closely resemble in vivo interactions and has been used for high-throughput drug screening assays12 that can be predictive of the patient's responses.4,5 However, these spheroids retain only a limited amount of the original TME because they rely on an amplification or growth step. 2) Microdissected tumors derived from cutting of tumors into submillimeter tissue pieces (confusingly, often termed “spheroids” as well), maintain the original TME relatively intact.13–19 3) Tumor slices20,21 is an approach sensitive to tissue scarcity, but this limitation is potentially addressed by a microfluidic approach that miniaturizes multiplexed drug delivery to individual slices.22–24 4) Patient-derived xenograft (PDX) mouse models permit the study of drug responses in an intact organism (including immune checkpoint blockade in humanized PDX25), but with the caveats that all or most of the TME is from the host mouse, and PDX from individual patients grow too slowly to inform initial post-operative therapeutic decisions. Lastly, 5) implantable or needle microdelivery devices26,27 locally deliver small doses of (up to 16) drugs to the tumor in vivo, with maximal preservation of the TME, but subject to limitations of tumor accessibility and patient safety.
Microdissected tissues (μDTs) from tumors have shown promise recently as an approach that preserves the TME.15,19,28–30 In 2014, Jahnke et al. demonstrated drug responses in roughly 400 μm fragments manually prepared from patient melanomas (primary and metastases).29 Two groups showed responses to immune checkpoint inhibitors in multiple cancer types with μDTs created by manually mincing with a scalpel. Kuo's group re-suspended the μDTs in collagen gel and cultured them in Transwells.17–19 Jenkins and co-workers also re-suspended the μDTs in collagen gel but cultured them in microchannels.14–16 However, mincing has shortcomings: a) as a manual technique, it results in very heterogeneous μDT sizes; while filtering is an option, it severely reduces the tumor mass available for testing; and b) the μDTs are typically seeded at random, which potentially confounds results (e.g., in secretion assays) because different wells or chambers can have different amounts of μDTs. To address these two issues, Astolfi et al. prepared regularly-sized cylindrical μDTs (380 μm diam. cores punched from 300 μm-thick PDX tumor slices) and tested their response to a cytotoxic chemotherapy drug within a microfluidic device; they presented a metabolite transport model for μDTs cultured under flow (no hydrogel) in a design with 5 manually-loaded traps per microchannel and 4 channels per device.13 They recently integrated their device (increased to 8 traps per microchannel) with tissue microarray histological analysis and confirmed the integrity of the μDT TME for up to 15 days in culture.30 None of the above studies/approaches can be easily upscaled to drug testing of large numbers (hundreds) of drug conditions with homogeneously sized μDTs.
While microfluidic approaches to drug testing that use “reconstituted” organs or tissues from dissociated cells (i.e., organ-on-a-chip) have become quite sophisticated,31–33 microfluidic approaches that use intact tissues remain less advanced.34,35 Our microfluidic trap design is based on the hydrodynamic trap invented by Takeuchi to create arrays of beads,36 and then adapted to trap single cells,37 spheroids,38 or small μDTs39 at high efficiency with PDMS devices. However, PDMS is not adequate for drug-based studies; both ![[a with combining low line]](https://www.rsc.org/images/entities/char_0061_0332.gif)
sorption ![[i with combining low line]](https://www.rsc.org/images/entities/char_0069_0332.gif)
![[n with combining low line]](https://www.rsc.org/images/entities/char_006e_0332.gif)
![[t with combining low line]](https://www.rsc.org/images/entities/char_0074_0332.gif)
PDMS40–47 and ![[a with combining low line]](https://www.rsc.org/images/entities/char_0061_0332.gif)
sorption ![[o with combining low line]](https://www.rsc.org/images/entities/char_006f_0332.gif)
![[n with combining low line]](https://www.rsc.org/images/entities/char_006e_0332.gif)
![[t with combining low line]](https://www.rsc.org/images/entities/char_0074_0332.gif)
PDMS48 can potentially alter experimental outcomes by changing the target concentrations and by partitioning molecules in undesired regions of a microfluidic device. Thus, we fabricated Takeuchi traps in poly(methyl methacrylate) (PMMA) laminates by laser cutting, an approach made feasible by the larger dimensions of our microchannels and traps. Furthermore, existing microchannel trap devices for cells or organoids remain closed after trapping,34,49 and thus generally only permit treatment of all trapped elements with the same medium/drug condition. While one could create multiple treatment conditions with valves and additional microfluidic circuitry to permit delivery of different solutions, these approaches create even more complex devices and operation. In our device, the traps are only closed during loading; after trapping, removal of the trap roof layer opens a window above each cuboid. Subsequent application of a multi-well grid allows for top-loading each well with a different drug treatment by simple pipetting. Here, we demonstrate the feasibility of the approach with an 8-well design capable of trapping 3 cuboids per well (total 24 cuboids). The design is potentially scalable to a 96-well (or larger) format, opening the possibility for large-scale drug testing with human donor tissue in the near future.
Results and discussion
Cuboid microdissection procedure
We have developed a procedure that facilitates the microdissection of live biopsy specimens (e.g., a tumor resection) into thousands of similarly-sized, cuboidal-shaped μDTs, or “cuboids”. Such regularly-sized, symmetrical tissue pieces critically enable their use in microfluidic devices and reduce size variability to improve biological assays, such as cytotoxicity testing.13,50 The procedure is schematically depicted in Fig. 1. Using a mechanical (McIlwain-type) tissue chopper, we perform three series of cuts. First, we generate slices from the biopsy that has been glued onto a PMMA disc (Fig. 1a). Next, we cut those slices, laid down flat (Fig. 1b), in one direction (Fig. 1c), then again in the orthogonal direction, generating cuboids (Fig. 1d). Due to the rapid operation of the tissue slicer blade, the chopping procedure takes only a few minutes (improving tissue viability) to potentially produce hundreds of cuboids per slice of similar size. The total number of cuboids obtained depends on the initial biopsy volume and the target size of the cuboids. The tissue chopper performs best with pieces of a low aspect ratio, such that in the initial dissection we would prepare small pieces ∼0.25–0.5 cm in height, and 0.5–1 cm in the lateral dimension, sizes comparable to that of a small patient biopsy.
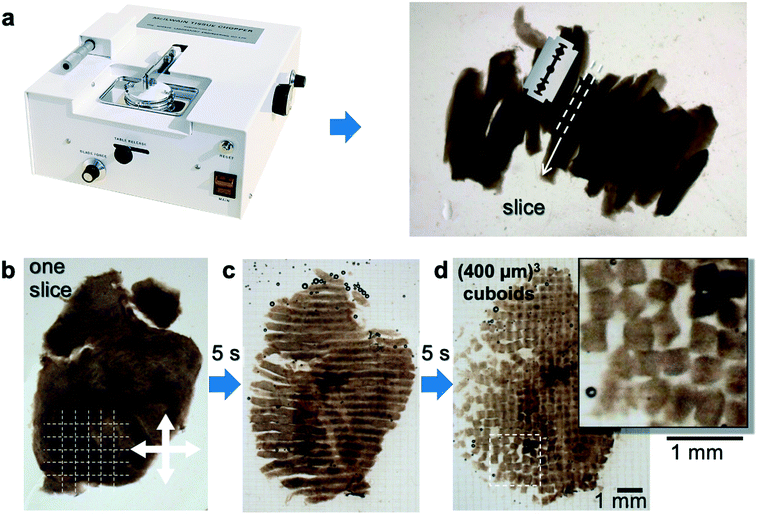 |
| Fig. 1 “Cuboid” sectioning procedure. (a) McIlwain-type tissue chopper and example of ∼400 μm-thick glioma slices. (b–d) Cuboid sectioning procedure showing a consecutive orthogonal slicing procedure starting with a single 400 μm-thick slice. | |
We have focused on cuboids that measure ∼400 μm × 400 μm × 400 μm for several reasons. 400 μm lies within the range (50–800 μm) previously used with organoids12,51 and μDTs13,15,29 from a variety of solid tumor types.12,51 Critically, this size strikes a balance between a larger size that better preserves the TME, and a smaller size that better maintains nutrient delivery and viability, thus minimizing the development of necrotic cores.13,52 Larger and smaller cuboids are possible and could also be of interest, e.g., to understand the effect of hypoxia (larger cuboids) and to obtain higher throughput (smaller cuboids). As guidance, Table 1 shows the maximum number of expected cuboids per cm2 as a function of their size, when cut from slices of the corresponding thickness; these maxima range from 2500 cuboids per cm2 for (200 μm)3 cuboids to 400 cuboids per cm2 for (500 μm)3 cuboids. Theoretically, a small tumor from which we could obtain 4 or more 1 cm diam. slices on the first cut could yield up to a few thousand cuboids that are (400 μm)3 in volume. In practice, the debris caused by the dissection procedure and other irregularities make a filtering step highly desirable. Passage through mesh filters of defined dimensions can enable enrichment for pieces of a particular size range because it removes pieces that are too big (e.g., remain attached to each other) or too small (e.g., cut from the edges or from pieces that break up during processing).
Table 1 Total number of cuboids per unit area
Cuboid size |
(200 μm)3 |
(300 μm)3 |
(400 μm)3 |
(500 μm)3 |
# cuboids per cm2 |
2500 |
1111 |
625 |
400 |
Distribution in cuboid sizes
We evaluated the size distribution and sample loss for the cutting and filtering/enrichment steps of our cuboid microdissection process (Fig. 2a). We analyzed freshly microdissected cuboids before and after filtering both from mouse liver (Fig. 2b–e) and from U-87MG (U87) glioma cell-derived xenograft flank tumors (Fig. 2f–i). The liver and glioma tissues yielded different size distributions, probably due to the differences in consistency and density between liver and glioma. For mouse liver samples before filtering, we observed large numbers of liver tissue fragments below the desired size range (39% of the cuboids <300 μm), though the majority of cuboids were in the desired size range (59% were 300–600 μm) (Fig. 2b and c). The filtering steps improved the size distribution, reducing the small liver cuboids and fragments to ∼28%, and increasing cuboids of the desired sizes to 70% (Fig. 2d and e). We only observed a small percentage of liver cuboids bigger than 600 μm, both before filtering (1.5%) and after filtering (1.8%). For glioma cuboids before filtering (Fig. 2f and g), we observed a smaller percentage of small pieces (20% were smaller than 300 μm and 78% were in the desired size range) than we had with liver, suggesting less tissue fragmentation during the microdissection process. As with the liver, the filtering steps improved the size distribution, with 88% of the glioma cuboids in the desired 300–600 μm size range and only 10% of the cuboids smaller than 300 μm. As with liver cuboids, only a small percentage of glioma cuboids were larger than 600 μm, both before (0.9%) and after (1.3%) filtering.
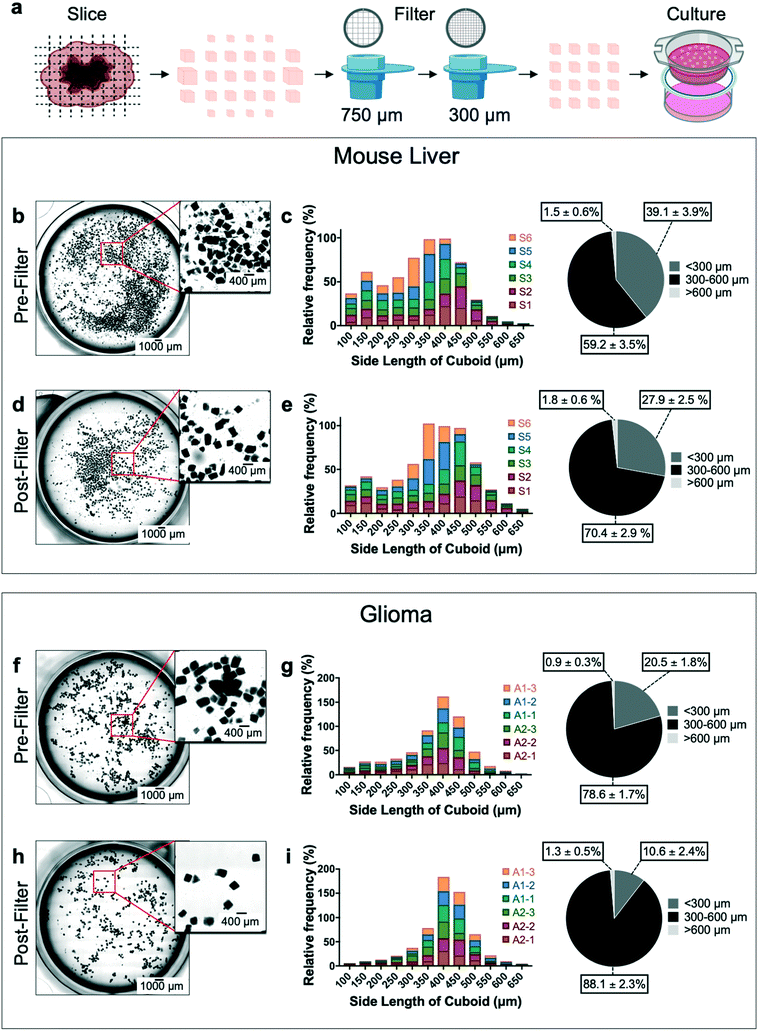 |
| Fig. 2 Mouse liver and glioma cuboid size analysis. (a) Cuboid slicing and filtering process overview. Size distribution analysis of mouse liver (b–e) and glioma cuboids (f–i). Sample images showing cuboids before ((b) mouse liver, (f) glioma) and after filtering ((d) mouse liver, (h) glioma). Summary of size distribution shown as relative frequency (%, histogram) and as frequency average of all samples (%, pie chart) for sizes <300 μm, 300–600 μm, >600 μm before ((c) mouse liver, (g) glioma) and after ((e) mouse liver, (i) glioma). Each “sample” consisted of a set of 11–17 slices (∼1 cm2 total area) that were processed together, from cutting further into cuboids and to filtering, with up to 3 samples per tumor/liver. The total number of cuboids pre-filtering per sample ranged from 650–1150 for liver, and from 500–900 for glioma. N = 6 samples each for both mouse liver and glioma. Pie chart data shows averages ± SEM. | |
In addition to size distribution, we investigated how much of the initial liver and glioma tissue sample loss occurs in each microdissection step of the process (ESI† Fig. S1). We found that, at the end, cuboids of the desired 300–600 μm size range represented ∼34% and ∼48% of the initial liver and glioma tissue slice samples, respectively (ESI† Fig. S1b and c). The tissue loss due to mechanical microdissection was similar for liver (∼20%) and glioma (∼21%). Likewise, tissue loss due to the filtering steps was similar for liver (∼32%) and for glioma (∼25%). Together, the tissue loss from the dissection and filtering steps totaled ∼53% for liver and 46% for glioma. Finally, to better understand the effectiveness of the filtering process for our cuboid size of interest, we calculated the loss of the initial pool of 300–600 μm cuboids with filtration, which was higher for liver (∼49%) than for glioma (∼30%), perhaps due to the fragility of the liver cuboids. From each of the two small glioma tumors analyzed we obtained ∼4 cm2 of slices and ∼1300 total cuboids after filtering. We could potentially improve our microdissection process. An additional 300 μm filtering step to remove more of the smallest cuboids would increase the percentage of cuboids of the desired size range, but at the cost of a lower yield. We found that much of the sample loss, and some of the cuboids measured as larger than 600 μm, resulted from incomplete separation of cut cuboids. This problem could potentially be addressed by gentle enzymatic treatments such as collagenase or DNase.12,19
Cuboids that are too small or too large impair the performance of our microfluidic device, which has flow-driven cuboid traps (see below). While the smallest tissue fragments just flow straight through the device, tissue fragments between 100–150 μm can directly obstruct and/or clog the small microchannels, thus disabling individual traps. Cuboids between 150–250 μm would potentially allow trapping of multiple cuboids because they are not big enough to fill the traps. On the other hand, cuboids larger than 600 μm that may have difficulty flowing through the bypass channel curves could result in cuboid congestion or even blockage.
Characterization of the cuboid tissue microenvironment
To visualize the 3D tissue microenvironment inside the cuboids, we performed open-top light-sheet (OTLS) microscopy of fixed and optically-cleared liver cuboids (Fig. 3a–f and ESI† Movie S1). OTLS microscopy offers high-throughput 3D imaging in a configuration easily compatible with culture dishes or devices.53–55 Vibratome slices of liver were cut into cubes with the tissue chopper, suspended in collagen, and fixed after gelation. Staining with far-red fluorescent TO-PRO 3 nuclear dye and fluorescent eosin, ethyl cinnamate clearing, and OTLS microscopy were performed as according to ref. 54–56. Note that optical clearing, which often involves tissue dehydration and solvent exchange, can lead to significant shrinkage of the tissue (potentially ∼50% (ref. 57)). The fluorescent images revealed that while the outer edges of the cuboid had ∼1–3 layers of dead or dying cells (an expected acute response of the fragile liver to the cutting procedure), most of the cuboid interior appears undamaged (Fig. 3a). All sides were similar, suggesting that chopping did not cause worse tissue damage than the gold-standard vibratome. Using simple image processing, one may generate false-colored images that mimic the traditional H&E (hematoxylin and eosin) stain58 (Fig. 3b). The large hepatocytes (parenchymal cells), nuclei of smaller non-parenchymal cells (including Kupffer cells, stellate cells, and endothelial cells, among others), along with the liver sinusoids and other vascular structures, can all be observed (Fig. 3a and b and ESI† Movie S1). By 3D image analysis of three cuboids with a combination of FIJI and Imaris, we identified the cuboid surface, vascular spaces, and cell nuclei (Fig. 3c–f and ESI† Movie S2). For the vascular spaces and nuclei, we limited our analysis to the central regions as the morphology was altered in the areas nearer the surface. With this approach, we could quantify not only the number of cells (nuclei) per cuboid, which could be valuable for normalizing the mass in pharmacological assays, but also their relative location, which could be exploited for spatial assays with labeled cells. Using the Imaris surface module, we calculated an average volume of the three cuboids of 0.025 ± 0.002 mm3. In the smaller central regions of the cuboids (0.0028 ± 0.00014 mm3), the vasculature occupied 7.9 ± 2.2% of the volume, and the nuclear density was 311
000 ± 17
000 nuclei per mm2. Using the bimodal size distribution of the nuclei from the two cuboids with best-preserved morphology (Fig. 3g), we classified the larger nuclei as hepatocytes (parenchymal cells) and the smaller nuclei as non-parenchymal cells (size threshold of 7.5 μm). This division was consistent with the nuclear size ranges and locations we observed for hepatocyte and non-parenchymal cells in the original 2D images. For the 3 cuboids, hepatocyte nuclei represented 44 ± 4% of the nuclei, not far from the ∼52% hepatocytes of total cells reported in a 2D study of mouse liver sections.59 The percent of binuclear cells for the cuboids (counted visually on 3 optical sections per cuboid) was 6.0 ± 0.3%; thus, our nuclear count represents an overestimate of the hepatocyte cell number. Note that hepatocytes have age-dependent changes in the percentage of diploid and polyploid cells.60 More in-depth image analysis of locations and DNA signal intensity could provide more insight into this aspect of hepatocyte analysis. In the future, fluorescently labeled cells and/or other fluorescent labels (endogenous or exogenous) can be added to provide additional biological readouts, both for fluorescent 3D analysis of fixed tissue (as performed here) or for two-photon/confocal microscopy of living tissues.
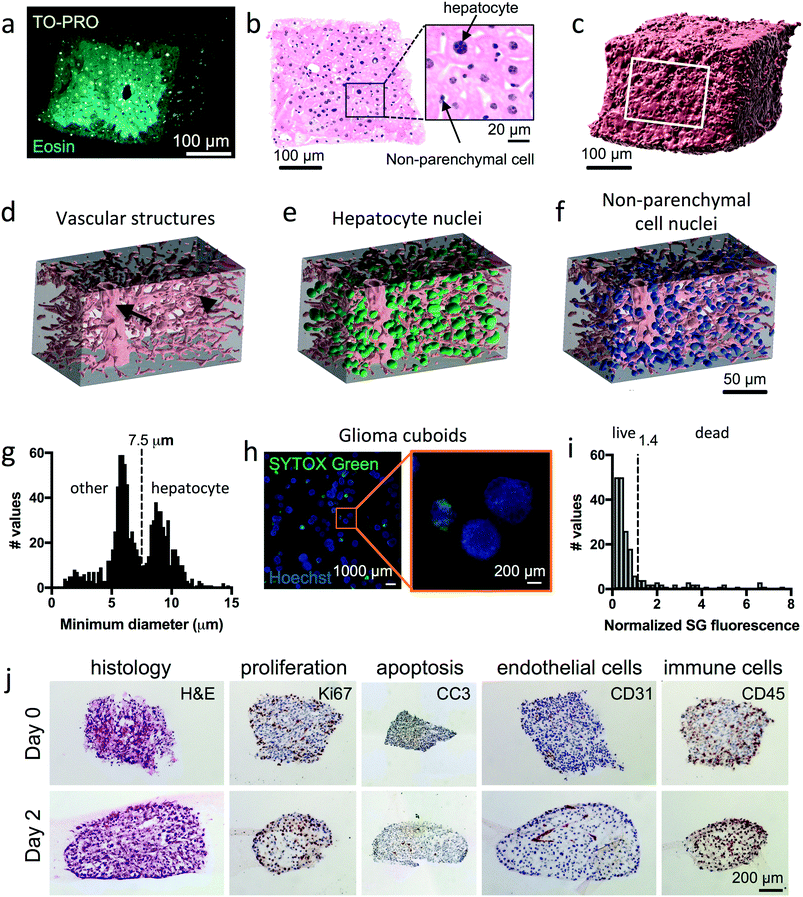 |
| Fig. 3 Characterization of the cuboid microenvironment. (a–f) 3D imaging of liver cuboid by light sheet microscopy (TO-PRO, yellow; eosin, blue). (a) 3D rendering. (b) False-colored H&E of top view slice showing parenchymal cell hepatocytes (arrows) and smaller, non-parenchymal cells in inset. (c) 3D rendering of the surface of a cuboid. (d) 3D rendering of the vascular space (“vascular” structures) within a central region of the cuboid as indicated by a white box in (c). Large vessel (arrow) and smaller vascular structures (arrowheads). (e) 3D rendering of the larger nuclei (“hepatocyte”) superimposed on the vascular space. (f) 3D rendering of the smaller nuclei (“non-parenchymal”) superimposed on the vascular space. (g) Histogram of nuclear size distribution from the same liver cuboid, using the diameter along the shortest axis of a bounding box. (h) Viability staining of live U87 glioma cuboids after 3 days in culture. Dead nuclei stain with SYTOX green (SG, green) and all nuclei stain with Hoechst (blue). (i) Histogram of mean SG fluorescence after normalization to average (N = 188). The dotted line indicates fluorescence threshold of 1.4. (j) U87 glioma cuboids before (day 0) and after time in culture in collagen hydrogel (day 2), showing histology and immunostaining for proliferation, apoptosis (cleaved-caspase 3, CC3), endothelial cells, and immune cells. Representative images from experiments on 3 different tumors. | |
We next evaluated the viability and microenvironment of U87 glioma cuboids after time in culture. For these experiments, we cultured the cuboids within a collagen hydrogel layer on top of a Transwell insert, with an air interface above and medium below, as described by Neal et al. for microdissected tumor pieces.19 By day 2, the cuboid shape relaxed to that of a spheroid. First, we performed live viability staining of the cuboids using the green fluorescent dead nuclear stain, SYTOX green (SG), and the blue fluorescent pan-nuclear stain, Hoechst (H), after 3 days in culture (Fig. 3h). Most of the cuboids show minimal SG dead stain (fluorescence normalized to the mean), but ∼15% were relatively green (dead) (two separate experiments with ∼16% at day 3 or at day 4) (Fig. 3h and i and ESI† Fig. S2). The threshold for death was the mean increase (1.4) seen after a 2 day maximal drug treatment (100 μM cisplatin) (ESI† Fig. S2). Next, after fixation of cuboids cultured for 2 days, staining of thin histological sections also revealed preservation of the cuboid viability (Fig. 3j). Traditional H&E staining showed similar histology between the cuboids and the initial tumor. Immunostaining revealed continued proliferation (Ki-67) and minimal apoptosis (anti-cleaved caspase 3, CC3), usually central if present. Finally, immunostaining for different cells of the microenvironment demonstrated continued presence of endothelial cells (CD31) and immune cells (CD45) (Fig. 3j). These results support the potential of cuboids as an intact tissue model for drug testing.
Microfluidic device design and operation
The regular, small size of the cuboids makes them amenable to microfluidic hydrodynamic trapping. To trap the cuboids, we chose the Takeuchi design36 because it traps nearly every particle entering the network until all of the traps are filled (Fig. 4a and b). Flow diverts particles from the main channel into individual trap channels when the traps are empty and does not divert particles when they are filled. Later versions by others, all made in PDMS, have trapped cells, spheroids, or μDTs (of less than 300 μm diameter when trapped).37–39,61 To iterate the design of our prototypes and to rapidly test various trap and chip layouts, we used stereolithographic 3D printing until we settled on our final trap and chip design (see ESI† Fig. S3). To avoid resin leaching issues and to facilitate the integration of removable layers (which are needed for hydrodynamic seeding of the cuboids), we fabricated the final design in PMMA laminates, following our published laser-cutting and PMMA bonding protocols.62 A 3D CAD drawing (Fig. 4c) shows the basic design of the PMMA microfluidic channel network and traps.
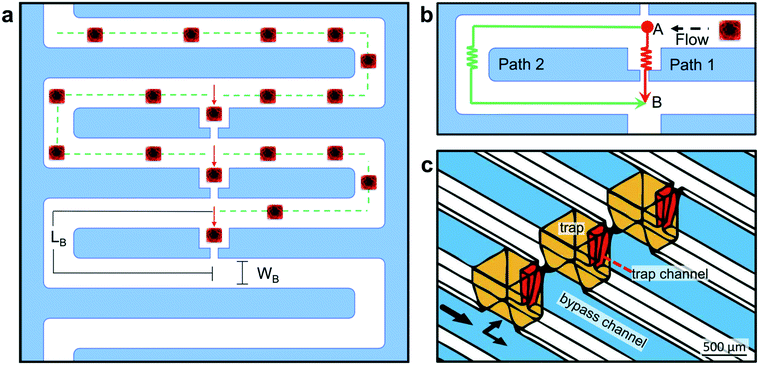 |
| Fig. 4 Microfluidic trap design. (a) Schematic representation of the trap design corresponding to one well and its three traps. The flow resistance of the traps (red arrow) is lower than that of the bypass channel (green dotted line), but if a cuboid (red) becomes trapped, the next cuboid in the flow will continue through the bypass channel. The width and length of the bypass channel are WB and LB, respectively. (b) Close-up of one trap. Lower hydraulic resistance through path 1 (RT in the trapping channel, red) versus through path 2 (RB in the bypass channel, green) leads to greater flow from point A to point B through the trap. (c) 3D CAD drawing of the traps and microchannels in the PMMA device, with a close-up view of three adjacent traps. | |
Our microfluidic device enables the trapping of intact cuboidal micro-tissues into addressable wells/traps for culture and multi-drug exposures (including cell-based therapies). The multi-stage design consists of a loading stage and a culture stage (Fig. 5a). For both stages of operation, the device is composed of 4 functional components, not including the lid and a base for culture. These components are: 1) a removable polysil silicone adhesive roof (for the loading stage) or a bottomless 8-well plate (for the culture stage, attached after loading), 2) a microfluidic channel layer, 3) a sealing layer for the bottom surface of the channel network, and 4) a set of inlets.
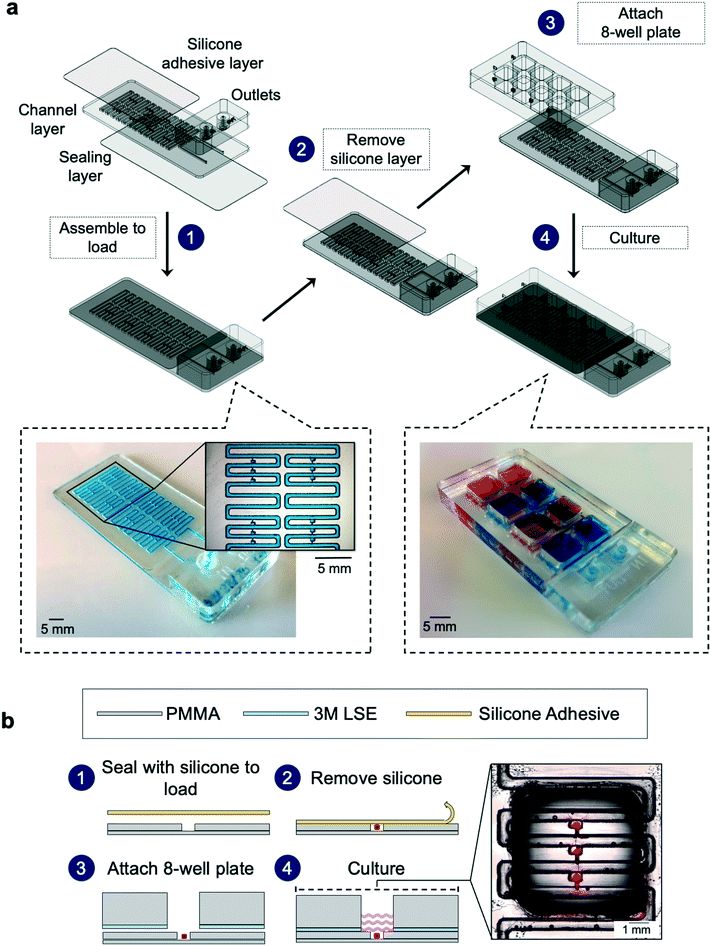 |
| Fig. 5 Microfluidic device design and operation. a) Multistage design of the microfluidic device, with a loading stage (1–2) and a culture stage (3–4). 3D renderings of device components, as well as micrographs of a device ready for loading (filled with blue dye) and of a device ready for culture (wells filled with red and blue dyes). (b) Cross-sectional view of the same multi-step operational procedure as in (a). Inset shows a top view of trapped glioma cuboids (fluorescently labeled with CellTracker-red) in a single well of the microfluidic device. | |
We fabricated the device using a combination of CO2 laser micromachining, solvent bonding, thermal bonding, and transfer adhesive techniques. For CO2 laser micromachining, we determined different optimal CO2 laser power and speeds for each specific width and depth of the cuboid traps and microchannels (ESI† Fig. S4). Chloroform treatment before bonding not only promoted bonding of the PMMA components, but also improved the optical clarity and reduced surface roughness (ESI† Fig. S5 and S6).63,64 The bonding procedure for the PMMA components (irreversible except for the silicone adhesive) produced a leak-proof platform.
The microfluidic device design necessitates two stages of operation. The main microchannel connects the 8 wells in series. In the final culture configuration, each well contains 3 cuboid traps that each open from the top to the well and remain connected to the main microchannel. Due to this incompletely closed channel architecture (the traps are open to the atmosphere), effective flow through the microchannels necessitates closure of the trap roofs. Therefore, before we load the traps with cuboids via the microchannels, we temporarily seal the open traps with removable silicone adhesive tape to enable flow (Fig. 5a). We then load the cuboids in a collagen suspension, which, once gelled, immobilizes them and creates the collagen/hydrogel culture environment. After loading, we remove the silicone tape and replace it with the bottomless 8-well plate (containing adhesive), leaving the traps open. Note that, while it would be technically possible to load each trap manually in our small prototype device by pipetting into each trap, we seek a high-throughput solution that could potentially solve the problem of loading thousands of cuboids.
We based our microfluidic approach for cuboid entrapment on Takeuchi's design, which exploits hydrodynamic principles to achieve the serial entrapment of particles.65 Our microfluidic design (Fig. 4a and b) consists of a continuous bypass channel (Wb = 315 ± 9 μm, Wt = 721 ± 12 μm, h = 672 ± 10 μm) and a series of lower resistance trapping channels. These trapping channels contain a trapping section/well (W = 737 μm, h = 1000 μm) and a narrower channel (Wb = 99 ± 5 μm, Wt = 181.6 ± 0.1 μm, h = 712 ± 54 μm). The top and bottom dimensions reflect the approximately trapezoidal profiles created by our laser-cutting protocol (ESI† Fig. S6). With this microfluidic network design, flow “prefers” to go through the open traps (Fig. 4b, path 1) but, when flow brings a particle into a trap, the particle blocks the flow and diverts the flow to the next trap (Fig. 4b, path 2). The hydraulic resistance ratio of the two fluidic paths governs the relative flow rates (QB & QT) through the fluidic circuits. The hydraulic resistance (R) is directly related to the geometric parameters of the channel. Channels made with CO2 laser engraving have a trapezoidal geometry with a constant depth (h), defined by the power and speed parameters of the laser cutter. After applying the Darcy–Weisbach equation (assuming fully developed, steady state and incompressible flow, see ESI† Methods), we calculated the hydraulic resistance along the bypass channel (RB) and along the trapping channel (RT). Their ratio, RB/RT, was 1.26. Since ΔP = Q × R, and ΔP is the same for path 1 and path 2, then it must be that QB × RB = QT × RT or, in other words, that QT/QB ∼ 1.26, i.e., the flow rate through the trapping path (QT) will be ∼1.26× higher than the flow rate through the bypass path (QB).
Because laser cutting yields channels with complex geometry, we turned to more realistic finite volume modeling of fluid flow in the device to inform key decisions related to the design and operation of the device. In particular, we wanted to more accurately assess the path taken by the cuboids for each of the three traps (Fig. 6a–c). We created a 3D AutoCAD model of our device (Fig. 6a) using measurements from assembled devices (ESI† Fig. S6). Using Ansys Fluent, we modeled the velocity and flow profiles over the whole device assuming a trap configuration with groups of three adjacent traps (Fig. 6a), as well as a control configuration with traps separated by an extra length of microchannel (Fig. 6b). Using the Ansys model, we incorporated the complex 3D geometry and hydrodynamic properties of the fluid, including viscosity and flow rate. Prior studies that utilized the Takeuchi method calculated the ratio of resistance in the two paths (bypass and trap) to predict the relative flow ratios36,61,65 – an approach that did not take into account the influence of downstream traps. Calculation of the flow ratio QT/QB for the first three traps revealed dramatically different results for the two different configurations (Fig. 6c and d). The QT/QB ratio for the control configuration was 0.72 for all three traps, which suggested that the traps would not function. In contrast, the QT/QB ratios for the adjacent-trap configuration were over 1 (1.16, 1.39, 1.22), which suggested functional traps and that the second trap would be the most efficient of the three traps. A key factor for experiments with intact-tissue cuboids is their high density (higher than that of cells), which causes a high speed of sedimentation and can lead to settling and friction at the bottom of the microchannel. Note that because of their rectangular profiles they do not roll, as would spheroids. Therefore, use of denser solutions (which also tend to be more viscous), such as collagen (1.013 g mL−1 and ∼50 mPa s (ref. 66)) or polyethylene glycol (PEG) solutions (20% PEG is 1.04 g mL−1, μ = 15 mPa s (ref. 67)), might minimize sedimentation and friction and improve trapping efficiency. If high viscosity becomes a problem, Percoll solutions could, in principle, be used for trapping to make solutions with high-density and relatively low-viscosity (1.05 g mL−1 and μ ≈ 2.5 mPa s (ref. 68)), as used previously to hydrodynamically trap stem cell-derived cellular aggregates.69
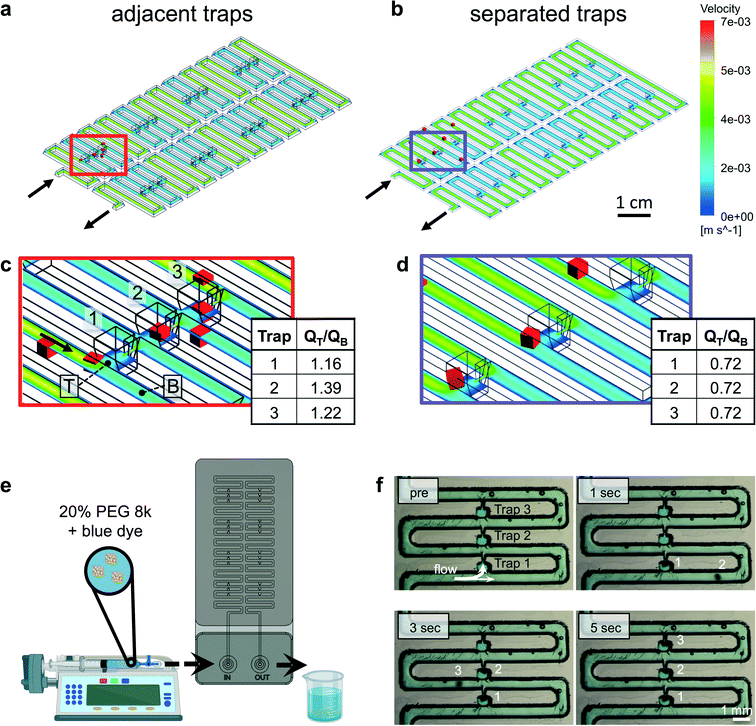 |
| Fig. 6 Finite volume modeling and cuboid loading into the microfluidic device. (a and b) 3D schematics of the microfluidic device with adjacent traps (a) and version with separated traps (b), showing a 2D cross-section of the 3D velocity profile performed with Ansys Fluent (point particles in red). First well (three traps) outlined with a box. (c and d) Close-up views of the first well region with locations at which flow was measured in a plane perpendicular to flow indicated for the trap (T) and for the bypass channel (B). Flow (Q) ratios are given for each trap. (e) Experimental setup. (f) Still images of device loading with fixed U87 cuboids (labeled 1, 2, 3) in a 20% PEG solution with blue dye. Full movie in ESI.† The flow rate was 20 mL h−1. | |
We next experimentally evaluated the loading efficiency of cuboids into the trap under relatively controlled conditions. For these experiments (setup in Fig. 6c), we used sparse, fixed U87 glioma cuboids manually selected for similar size (∼300–500 μm). In preliminary experiments, we observed that cuboids tended to rapidly settle to the bottom of the channels, which affected the trapping efficiency and could cause clogging. We could only achieve successful trap loading with medium or PBS if we loaded manually using a syringe while applying transient bursts of pressure. In contrast, manual loading with a collagen solution worked relatively smoothly without clogging. However, syringe pump loading with collagen proved impractical due to its high cost (limiting the volume we could use) and its irreversible gelation (a threat during extended loading if the solution is not kept cold in the whole fluid path, including the syringe, tubing, and device). Therefore, we suspended the cuboids in a 20% PEG solution (lightly colored with blue dye), which was dense enough to slow the settling of cuboids at rest. For these experiments, we controlled the flow rate (20 mL h−1) with a syringe pump. We found that cuboids would indeed fill the traps (Fig. 6d and ESI† Movie), but with less than 100% efficiency and with occasional sticking (that could be overcome by a brief, abrupt change in flow rate). Therefore, to quantitate trapping efficiency, for each of the two devices, we evaluated the filling of the first trap while all other traps were empty (to minimize any confounding effects on resistance). Cuboid removal by manual flow in reverse permitted us to perform multiple trials on the same trap. We found that the trapping efficiency was 84% (26/31) for device 1, and 69% (22/32) for device 2. Interestingly, for the cuboids not trapped by the first well, the trapping efficiency was 100% at the second well (5/5 for device 1 and 10/10 for device 2), consistent with the prediction by finite volume modeling that trap 2 would have a higher efficiency than trap 1.
When we performed manual loading of live cuboids in collagen (as in Fig. 5b), cuboids filled 83 ± 4% of the 24 traps of each of 4 different devices (AVE ± S.E.M.). Cuboids were at a low concentration to minimize interference between them, with excess cuboids to maximize filling (∼300 cuboids in 1.5 mL, not accounting for loss from manipulation). All but one of the unfilled traps (17%) were blocked by air bubbles present during loading. Furthermore, air bubbles nucleate and grow in the collagen solution (initially at 4 °C) because as it warms up, dissolved gases lose their solubility. These problems could potentially be mitigated by improvements in fluid handling or by addition of a bubble trap element. Despite filtering, one empty trap was plugged by a tissue piece too large to pass through the trap channels and too small to be in the target size range. Of the traps filled with cuboids, 17 ± 3% were filled by more than one cuboid. These touching cuboids fused over time in culture and were thus considered as one cuboid for later analysis. Removal of the silicone layer did not disturb the cuboids, which were held in place by collagen gel. In these preliminary experiments, our goal was to demonstrate the successful loading of a multi-trap device. This loading procedure was arguably wasteful, but we envision that in the future, one could recycle most of the untrapped cuboids and load multiple devices with the result of one micro-dissection.
We designed our microfluidic trapping device and loading procedure to preserve as much precious tissue material as possible. One can compare our microfluidic traps (with 3 traps per well) to a straightforward alternative, random seeding into multi-well plates. In principle, the microfluidic design would trap every cuboid, though variability can arise from occasional multiple cuboids caught in a trap or from bubbles blocking traps (as discussed above). Using a Poisson distribution with an expected rate of occurrences of 3 cuboids, we estimate that random seeding into multi-well plates should yield 22% of wells with 3 cuboids, 42% with less than 3, and 35% with more than 3. With this first iteration of our microfluidic device, counting traps occupied by 1–2 cuboids, we observed that our microfluidic devices yielded 63% of wells with 3 traps occupied per well (calculated for 32 wells over 4 devices). Looking at total cuboids per well, 85% had 2–4 cuboids per well, and 100% had 1–4 cuboids per well, as compared to 61% and 76% predicted for random seeding; none had more than 4 total cuboids per well in all traps, and none had no cuboids per well, as compared to 18% and 5% predicted for random seeding. Thus, the microfluidic device had a 39% better yield of wells within a target range of 2–4 cuboids. Further improvements with reductions of bubbles such as bubble traps would improve the yield. Additionally, while random seeding places cuboids haphazardly throughout the well, the microfluidic traps arrange the cuboids into an array with defined positions that should facilitate improved readouts, notably of single cuboids or for imaging.
Characterization of diffusion crosstalk
In order to evaluate potential crosstalk between wells of the device, we performed experiments with a fluorescent dye (fluorescein, MW = 332 g mol−1). An open channel ∼30 mm long (x) connects the last trap of one well to the narrow opening of the first trap of the next well. We measured the diffusion of fluorescein (1 mM) from one well in a device filled with collagen over 72 h (Fig. 7). To prevent bulk flow of fluid that would confound results, we sealed the openings of all other traps to other wells with silicone tape, plugged the inlet and outlet, and sealed the top of the fluorescein well with tape. From images taken at different time intervals, we measured the fluorescence intensity at different distances from the last open trap of the fluorescein well towards both sides (Fig. 7b–d). After 42 h, we found that at ∼30 mm along the microchannel from the last trap under the fluorescein well (ending at a point halfway between the small and large openings of the closest trap of the neighboring well), the fluorescence reached approximately 10% of the initial value. Beyond the first neighboring trap, the levels were much lower, with no apparent increase in fluorescence.
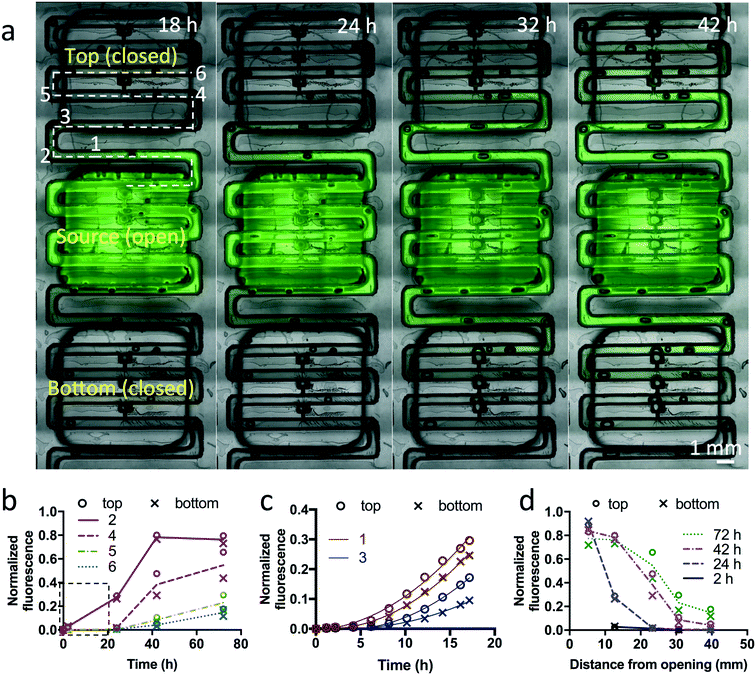 |
| Fig. 7 Dye diffusion between wells on the device. (a) Overlay of fluorescent and brightfield images of a collagen-filled device loaded with fluorescein (green) dye in a source well. Only traps in that well are open; the rest are sealed. At different times, fluorescent measurements were taken at different distances in both the “top” and the “bottom” directions, as indicated by the numbers along the dotted line. (b) Graph of fluorescence versus time, measured at different locations (numbers in (a)). The line represents the average value of the top and bottom directions. (c) Graph of fluorescence over the first 16 h (boxed area in (b)) as measured in two locations, as indicated in (a). A quadratic fit is shown. (d) Graph of fluorescence versus distance along the dotted line in (a), measured at different times. The line represents the average value in the top and bottom directions. After background subtraction, fluorescence was normalized to maximal fluorescence, as measured in the channels between the traps of the source well. | |
Next, we applied Fick's second law of diffusion to calculate for our experiments the effective diffusivity of fluorescein in collagen-filled microchannels:
A solution to Fick's second law of diffusion in semi-infinite media and a constant concentration source is given by:
where
D represents the effective diffusivity of fluorescein in collagen,
C(
x =
0) =
Cs is the concentration of fluorescein at the source, and
C(
x = ∞) =
C0 corresponds to the concentration at the first trap of the next well. We assume that
Cs remains constant over time, and
C0 = 0. The characteristic diffusion length (
L) at a given time (
t) is defined as the distance at which the concentration of the diffusing species reaches 50% of the source concentration (
Cs) and can be approximated by

. With a similar rationale, the solution for
D can be approximated using 15% of the source concentration instead, with

. Using this relationship, we used a quadratic fit to estimate the effective diffusivity of fluorescein at
C(
x,
t)
= 0.15 using the experimental ∂
C/∂
t curves for different locations (10 and 12.5 mm away from the source) over the first 16 hours (
Fig. 7c). These calculations yielded an effective diffusivity of fluorescein in collagen for our system of 6.04 × 10
−10 m
2 s
−1, close to the reported diffusion constant of fluorescein (
D = 4.25 × 10
−10 m
2 s
−1).
70 However, at longer time points (>16 hours), the movement of fluorescein appeared to be approximately 4-fold faster. Thus, we suspect there must be an alternative transport mechanism, such as residual flow caused by hydrostatic pressure or evaporation (the sealing of outlet and the open well was incomplete), that disrupted the assumption of a stationary medium in Fick's laws of diffusion. In future devices, we could ensure a stationary medium at any given point in time with physical barriers such as valves.
Selective dye delivery to cultured cuboids in the device
We next performed experiments to demonstrate the culture of cuboids and selective dye application in the device (Fig. 8). For these experiments we manually loaded U87 glioma cuboids in a collagen solution, immediately after cutting. After gelation of the collagen in the incubator, we labeled live cells in the cuboids for one hour with alternating patterns of green and red live fluorescent dyes (CellTracker Green and CellTracker Orange). Imaging after 2 days in culture (Fig. 8b) showed robust labeling of both dyes, indicating continued viability of the cuboids and no evidence for crosstalk between wells. To further confirm cuboid viability, we then stained the CellTracker Orange-labeled wells with the cell death nuclear marker, SYTOX green, and the pan-nuclear marker, Hoechst. We also stained the CellTracker Green-labeled wells with Hoechst alone. As shown in Fig. 8c–f, SG staining showed minimal cell death. If desired, confocal imaging or similar (potentially combined with tissue clearing analysis of the whole cuboid) could give a cellular level analysis of viability.
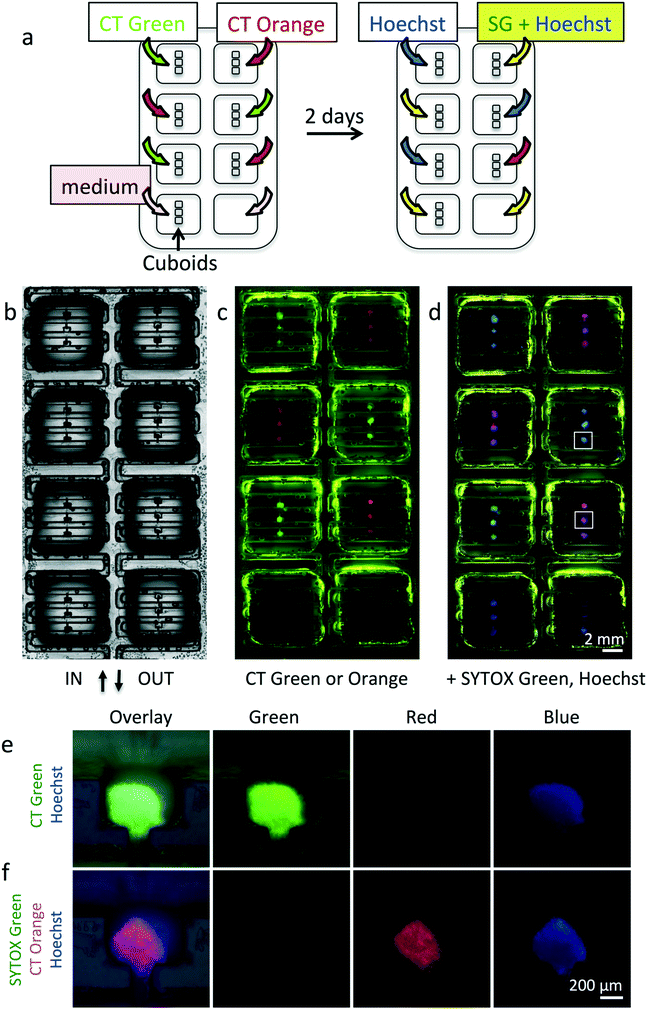 |
| Fig. 8 Cuboid culture and selective dye labeling in the device. (a) Schematic of the procedure. U87 cuboids were labeled with either green or orange fluorescent CellTracker (CT) dyes in the pattern indicated, for two devices in parallel with similar results. The CT dyes label live cells. The bottom wells contained only medium. After 2 days in culture, the cuboids were imaged. Then all wells were treated with the pan-nuclear blue dye Hoechst, and wells with CT Orange were additionally treated with the nuclear cell death indicator SYTOX Green (SG). (b) Brightfield image, with arrows indicating the direction of cuboid loading. (c) Continued strong CT fluorescence after 2 days indicates cell viability. (d) Subsequent cell death staining with Hoechst ± SG. (e and f) Close-ups of boxed regions in (d). | |
Drug treatment on the microfluidic device
As a first test of drug treatment with the device, we exposed U87 cuboids in the device with different concentrations of a cytotoxic chemotherapy drug, cisplatin (Fig. 9). In each of two devices, we treated two wells with each concentration of cisplatin (0, 10, 30, 100 μM) for 2 days from day 1 to day 3 (Fig. 9a). As a straightforward, simple measure of cell viability, we measured the mean fluorescence ratio in epifluorescence images of SG dead nuclear fluorescence to Hoechst pan-nuclear fluorescence (Fig. 9b). We found a statistically significant response to 30 μM cisplatin, with 100 μM showing a trend. The weak SG staining at higher concentrations was accompanied by concomitant weak Hoechst staining, consistent with breakdown of nuclei in later stages of cell death. Note the variability in responses, not unexpected due to the small size of the cuboids as well as some of the baseline viability noted without drug treatment (∼15% as seen in Fig. 3i and S2†). Off-device experiments performed in parallel with 100 μM cisplatin also showed cell death (Fig. S2†). We did not exclude any statistical outliers here. However, future experiments could increase the sensitivity of the assay by incorporating pre-treatment viability tests to exclude from analysis the already less viable cuboids without treatment. These results were similar to our previous experiments with U87 glioma slices on and off a microfluidic device that showed cell death in response to 30 and 100 μM cisplatin by SG/H live viability staining.23 These high cisplatin concentrations reflect the known sensitivity of the U87 cell line and are at least 10 fold higher than clinical doses in patients.71 While confocal imaging could provide a more precise evaluation, it is more time consuming. This device platform is also compatible with analysis of the supernatant in the wells for cell death, e.g., LDH levels by fluorescent assay, but would require more manipulation and would lose the independence of measurements for individual cuboids. Lastly, tumor heterogeneity poses a challenge to drug testing because different parts of the tumor respond differently to drugs. By histological measures on their μDT microfluidic platform, Simeone et al. determined that 15 PDX μDTs could encompass the heterogeneity of the original tumor.30 Their methodology could help inform the minimum number of cuboids and wells required in upscaling our device to a high-throughput multi-well platform.
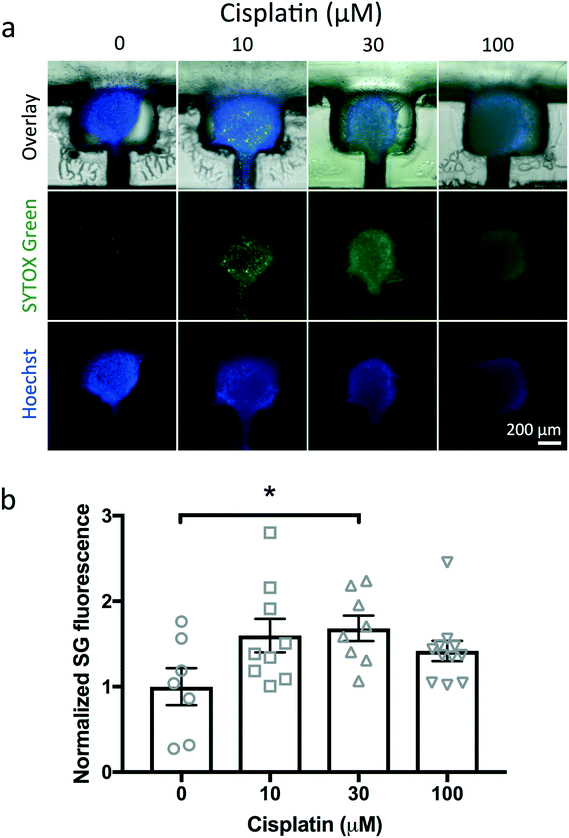 |
| Fig. 9 Drug treatment with the device. (a) Cell death in U87 cuboids after treatment for 2 days with different concentrations of cisplatin. Representative images from two devices with two wells each per concentration. SYTOX Green (green nuclear death stain) and Hoechst (blue pan-nuclear stain). (b) Quantitation of cell death by SYTOX Green (death) fluorescence. Mean fluorescence was normalized to the average value of control conditions. Individual points and average ± S.E.M. N = 7–11. One-way ANOVA versus control, Dunnett's multiple comparison test. *p < 0.05. | |
Conclusions
The combination of micro-dissected tumor cuboids and microfluidics should facilitate the applicability of functional drug testing to intact tissues that better preserve the tumor microenvironment. Here, we performed proof-of-concept dye labeling and drug testing using cuboids from a xenograft tumor model and an 8-well microfluidic device. The laser-cutting approach used here should facilitate the upscaling of the PMMA device to a higher-throughput 96-well format, to which valves may be integrated. More sophisticated readouts, including extraction of the cuboid cells and/or lysate, could include most types of analysis, from cDNA, to Western blot, to multi-omic analyses.
Experimental
Cell culture and drug screening
U-87 MG (U87) cells (ATCC) were grown in DMEM/F12 (Invitrogen) supplemented with 10% fetal bovine serum (VWR) and penicillin/streptomycin (Invitrogen). We passaged the cells twice a week.
Mouse tumor models
Mice were handled in accordance with institutional guideline and under protocols approved by the Animal Care and Use Committee at the University of Washington (Seattle, USA). To generate xenograft tumor mice, male athymic nude mice (Taconic, Foxn1nu) aged 4–10 weeks were injected subcutaneously in the flank (0.5–1 million cells in 200 μL of DMEM-F12 serum and antibiotic-free medium). Mice were sacrificed before tumor volume reached 2 cm2 (2–4 weeks). Livers from male nude mice without tumors (2–4 months old) were used for the intact cuboid imaging. For the chopping quantitation experiments, we used livers from adult (3 months old) C57BL6-derived mice (C57BL6-Sntb1tm2fl/fl SCF, functionally wild-type), kindly provided by M. Adams and S. Froehner, University of Washington.
Microdissection procedure and cuboid culture
To microdissect tissue cuboids, we used a standard McIlwain tissue chopper (Ted Pella, Inc.) set to 400 μm slice thickness. We first cut 400 μm-thick slices by mounting ∼1 cm × 1 cm tissue samples (mouse liver or glioma) onto a 1/4′′ PMMA disc using cyanoacrylate glue (Krazy glue). This procedure resulted in ∼20–25 tissue slices, which we manually separate as needed. Then, we manually transferred the slices into ice-cold solution in a dish using a razor blade. When possible, we kept the live tissue in ice-cold solutions, with mouse liver and slices in Belzer UW Cold Storage Solution (Bridge to Life), and with glioma tissue in DMEM/F12 supplemented with 10 mM HEPES (Invitrogen). Note that the blade leaves indentations in the PMMA surface with each cut. After leveling the blade again to ensure maximum contact, we cut the slices into cuboids as follows. We placed 400 μm-thick slices onto a relatively smooth (unused or used only once prior) 1/4′′ PMMA disc and removed excess fluid to prevent movement. After the first series of cuts, we rotated the disc 90° and repeated the cutting procedure. After cutting, we transferred the cuboids to a dish. To separate the cuboids, we gently pipetted up and down and used other tools if necessary (i.e., dissecting scissors). Then, we filtered the cuboid solution through a 750 μm filter followed by a 300 μm filter (Pluriselect, USA), keeping the cuboids retained by the smaller filter. Cuboids were maintained and washed with DMEM-F12 with HEPES. Before loading into devices, we manually removed unwanted particles (i.e., excess glue, unwanted pieces). For experiments with liver cuboids for intact imaging, we did the first cut of the liver into 400 μm-thick slices using a 5100mz vibratome (Lafayette Instrument) in ice-cold Krebs–Hensley solution (Alfa Aesar), bubbled with carbogen (95% O2, 5% CO2).
U87 cuboids were cultured in collagen hydrogel. We prepared 80% collagen (Corning rat tail collagen type 1, 354
236, 3–4 mg mL−1), 10% 10× PBS, and 10% serum-free medium. We then used filtered-sterilized 1 M NaOH to neutralize the pH to ∼7.2. For culture in 6 well plates, cuboids were cultured in 1 mL collagen on top of a 0.4 μm filter Transwell PFTE membrane insert (PICMORG50, Millipore) with 1.3 mL culture medium placed below. Cuboids were cultured both with and without an additional layer of pre-gelled 1 mL collagen below with similar histology and staining observed, apart from a relatively flatter shape often seen without the extra collagen layer. The culture medium contained Neurobasal-A medium (Invitrogen) with 25% heat-inactivated horse serum (Sigma), glutamax (Invitrogen), 2× penicillin/streptomycin (Invitrogen), and growth factors (EGF 20 ng mL−1 and FGF 20 ng mL−1, Preprotech or Invitrogen). Cisplatin (3 mM stock in dH20, MedChem Express) was diluted in cell culture medium for the drug treatment experiment.
Cuboid size and sample yield analyses
During microdissection, each set of slices cut at once (400 μm-thick, 11–17 slices, ∼100 mm2) represented one set of cuboids. After dissecting each set of slices (as described above), we isolated the resulting cuboids in a 6-well plate. We took tiled 2× images of each set of cuboids before and after filtering.
We utilized the free Fiji72 image analysis program to investigate cuboid size distribution and sample yield for intact tissues from both mouse liver and glioma. First, we converted each image to an 8-bit binary format. After binary conversion, we did background subtraction (100–200 pixel rolling ball radius) and manually adjusted brightness and contrast, if necessary. In addition to background subtraction, bubbles and other imperfections (such as objects out of focus) were eliminated from the images utilizing a background-colored brush. After cleaning, we adjusted the threshold to isolate the tissues (cuboids and slices, black) from the background (white, ESI† Fig. S1a).
To isolate cuboid aggregations, we converted binary images to masks and performed a watershed (1 px line width). Each image was carefully inspected to ensure proper watershed. By comparing to the original image, we distinguished areas of imperfections and made manual corrections as necessary. We adjusted the scale (μm px−1) of each image and analyzed the area (μm2) of cuboids/slices by setting a size range of (100 μm)2–(650 μm)2 and >(300 μm),2 respectively.
To estimate the dimensions of each cuboid, we assumed that cuboids had a cuboidal shape and took the square root of the obtained area value. We utilized GraphPad Prism 8 to investigate the size distribution (relative frequency) of each cuboid set using a 100–650 μm bin range with a 50 μm bin width. Similarly, for cumulative size range distribution analyses, we utilized a 100–750 μm bin range with a 300 μm bin width and averaged the relative frequency for all data sets (n = 6, for both mouse liver and glioma).
To estimate the % of the original sample left after dissection and filtering (ESI† Fig. S1b and c), we computed the total area of each slice set. Then, we aggregated the total cuboid tissue area for each set before and after the filtering step and the total area of cuboids within the range of 300–600 μm. Finally, we divided each data set by the total slice area. The same process was performed for both mouse liver and glioma tissues.
Live staining and microscopy
Live cuboids were stained for 1 hour, 37 °C, with the following dyes (individually or in combination) diluted in culture medium: Cell Tracker Green CMFDA (Invitrogen, 10 μM), Cell Tracker Orange CMRA (Invitrogen, 10 μM), Hoechst (H; Invitrogen, 16 μM), and/or SYTOX green (SG; Invitrogen, 0.01 μM). We performed epifluorescence and brightfield microscopy of the cuboids with a Nikon Eclipse Ti inverted microscope (Nikon Instruments, Melville, NY) at 2× and/or at 4×. For SG analysis, we used FIJI as follows. We performed background subtraction from empty areas. Cuboid regions were created from the Hoechst channels by thresholding, watershed on a binary image, followed by Analyze Particles. Mean SG fluorescence was normalized to the average value of untreated cuboids.
Immunostaining
After imaging, off-device cuboids were fixed with 4% paraformaldehyde overnight then cryoprotected with 30% sucrose/PBS overnight two times. Cryosections (14 μm thickness) were then processed for H&E or for immunostaining. For immunostaining, we pretreated tissue sections with 0.6% hydrogen peroxide in methanol for 30 min, washed, and then for some antibodies we processed for antigen retrieval by steaming for 30 min in 10 mM sodium citrate, 0.05% Tween 20 (Sigma), pH 6.0. After at least 30 min incubation in blocking solution (Tris-NaCl-blocking buffer or TNB buffer, Perkin Elmer, with 0.1% Triton X-100), we incubated the tissues with rabbit primary antibodies (diluted in TNB) overnight at 4 °C: active cleaved caspase 3 (CC3, 1/600, Cell Signaling), Ki-67 (1/1000, AbCAM, ab15580), CD31 (1/200, AbCAM ab28364), or CD45 (1/1000, AbCAM, ab10558). Finally, we incubated the tissues with anti-rabbit peroxidase polymer for 30 min (Vector Labs MP7401), then with the chromogen 3,3′-diaminobenzidine (DAB, Vector Labs), and lightly counterstained with hematoxylin. We performed all tissue washes with PBS.
Clearing and staining for light-sheet microscopy
Liver cuboids embedded in collagen were stored in 30% sucrose solution prior to staining and clearing. Cuboids were first dehydrated in graded steps to a 70% ethanol solution (v/v in water). Samples were then stained with a fluorescent H&E analog consisting of a 1
:
500 dilution of TO-PRO3 Iodide (Cat: T3605, Thermo-Fisher) and 1
:
4000 dilution of Eosin-Y (Cat: 3801615, Leica Biosystems).55 Following staining, samples were fully dehydrated in 100% ethanol and then cleared and stored in ethyl cinnamate at room temperature before and after imaging (Cat: 112372, Sigma-Aldrich).57
Open-top light-sheet (OTLS) microscopy imaging
Collagen-embedded liver cuboids were imaged on a multi-resolution OTLS microscope.54,55 Embedded cuboids cleared in ethyl cinnamate were mounted intact on a flat sample holder (Ultem 1000), mounted onto a motorized XYZ stage, and immersed in an ethyl cinnamate-based immersion bath. Specimens were illuminated at a 45 degree angle with a Gaussian light sheet (NA = 0.09, 2.75 μm FWHM light-sheet thickness) at 488 nm and 660 nm to excite eosin and TO-PRO3, respectively. The specimen was translated through the light sheet such that a series of adjacent 2D “optical sections” were imaged to generate a 3D dataset (0.65 μm lateral resolution). Fluorescence signal was imaged through a band-pass filter onto an sCMOS camera (ORCA Flash 4.0). A volume of approximately 0.10 mm3 was imaged for each cuboid at an approximate volumetric imaging rate of 0.07 mm3 min−1 (for a 2-channel dataset). Raw images were de-skewed by 45 degrees and subsequently stored in a Hierarchical Data Format (HDF5) with a B3D compression filter, as has been previously described.55,73 BigStitcher was utilized to fuse these datasets, which were then visualized volumetrically in Imaris.74 Additionally, to visualize datasets in a palette that mimics H&E histology, a false-coloring algorithm (based on Beer–Lambert law absorption) was applied using a Python script.58,75 The TIFF images of cleared cuboids obtained from OTLS microscopy were imported to Imaris software (Imaris 9.5.0, Bitplane AG) to perform 3D reconstruction. Imaris analysis was done using three channels: the original TO-PRO3 (nuclei), the original eosin (for surface volume), and a binary version of the eosin channel representing the voids (vasculature). For the analysis using the central region of the cuboid (vasculature and nuclei), we cropped the images to remove the outer regions with signs of unhealthy tissue (condensed nuclei and pale eosin staining). For nuclear analysis, nuclei labeled with TO-PRO3 were reconstructed into 3D surfaces using the ‘surpass module’ and “surface creation” feature, which included steps of region-of-interest (ROI) selection, background subtraction, thresholding, and water-shedding. The diameter for each nucleus was measured for the 3 sides of the bounding cube, and the shortest length was used to segregate nuclei according to their size distribution. We removed from analysis the nuclei on the edges (inaccurate size as cut by the border) and the few “nuclei” that were too large (>15 μm) or too small (<1 μm with volume <5 μm3) to be nuclei. For the vasculature analysis, with Fuji we converted the eosin images from 32 bit-TIFF to 8-bit, used Auto-local-threshold to isolate the vasculature, including gaps between the cell and sinusoids, and saved as a binary image (inverted). After importing to Imaris with the other two channels, we used the ‘surface creation’ feature to construct a 3D cuboid vasculature. Thresholding was performed on each cuboid individually in order to optimize capture of the nuclear and vascular features.
CO2 laser micromachining
The current version of our microfluidic consists of an 8-well plate with an integrated channel network layer. We fabricated the device by laser micromachining of PMMA substrates, thermal fusion, and adhesive bonding. The device is composed of four layers: a 1000 μm-thick PMMA channel network layer (Astra Products, Baldwin, NY (11510103)) containing the trapping microchannel connected in series to 8 sets with 3 traps per set, a 200 μm-thick PMMA sealing layer (AFT00, SPolytech, Chungbuk, Korea), a 76 μm-thick removable polysil double-coated silicone adhesive tape (S1001-1DC1, Adhesive Applications, Easthampton, MA), and an insertable 6.35 mm-thick PMMA bottomless 8-well “well plate” (1227T569, McMaster-Carr, Elmhurst, IL) lined with a 50 μm-thick 3M™ High-Strength Acrylic Adhesive 300LSE. In addition to the main components, the device also has a customized base and a lid (1227T569, McMaster-Carr, Elmhurst, IL). The base raises the device from the surface to avoid scratches (thus maintaining optical clarity) and makes its dimensions compatible with conventional imaging slide stages. The lid prevents contamination and allows proper airflow for tissue culture.
The CO2 laser system used (VLS3.60, Scottsdale, USA) has a wavelength of 10.6 μm and a maximum power of 30 W. We utilized AutoCAD 2017 for device design (ESI† Fig. S3) and optimized the power and speed settings of the CO2 laser to achieve specific widths and depths for the microchannels and to cut the outlines of the channel network and sealing layers. For optimal alignment, we manually lined the bottomless well-plate with the high-strength acrylic 300LSE adhesive prior to laser cutting.
Post-ablation processing
Laser ablation of PMMA generates polymer debris and polymer reflow. To remove debris from the laser-cut substrates, we rinsed each of the device components with DI water and sonicated them in an IPA bath for 30 s. To reduce surface roughness and improve the optical quality, we exposed the channel network to chloroform vapor. We used a glass container (264 mm (L) × 213 mm (W) × 165 (T) mm) filled with 50 mL of chloroform and steel standoffs (6 mm) to elevate the laser-micromachined layers 3 mm above the chloroform surface (ESI† Fig. S2). We concurrently exposed the channel network layer and the sealing layer to chloroform for 5 min.
Bonding procedures
We performed a combination of thermal bonding and solvent bonding (which we refer to as “thermal solvent bonding”) as well as adhesive bonding. Exposure to chloroform vapor also causes the PMMA to become slightly adhesive by inducing polymer reflow.64,76 After chloroform vapor treatment, the surface of the PMMA substrates becomes soft due to polymer solvation. When two treated surfaces are placed in contact with each other, a cohesive molecular bond is formed while excess vapor evaporates from the interface. For assembly, we exposed the channel network layer and the sealing layer to chloroform vapor. For “thermal solvent bonding”, we first hand-pressed the sealing layer onto the channel network layer to form a weak bond. Then, to ensure uniform bonding, we sandwiched the two layers between two ∼3 mm thick PDMS slabs with the same outer dimensions as the channel network layer. Finally, we placed the whole ensemble in the heat press for 4 min at 150 psi and 60 °C.
Before cuboid loading, we sealed the trap openings with the removable polysil double-coated silicone adhesive tape using the same pressing setup, at room temperature. After loading, we manually removed the silicone adhesive from the channel network. To bond the bottomless 8-well plate to the channel layer, we removed the 3M300LSE liner and manually pressed both components together.
Hydrophilization and bubble removal
After device assembly and prior to use, we treated each device with oxygen plasma for 5 min at ∼950 mTorr (60 watts, Diener RF plasma oven) to increase the hydrophilicity of the PMMA surfaces. Then, to prepare the device for use, we manually filled the device with 100% ethanol to remove bubbles from trapping areas. Once the device was bubble-free, we manually injected sterile DI water into the microchannels, followed by sterile PBS.
Computational fluid dynamics (CFD) modeling
CFD modeling was performed using Ansys 2019 R1 software package available at the Department of Mechanical and Aeronautical Engineering, University of Pretoria, in South Africa. To do the numerical simulation, the CAD file shown in Fig. 4c was first generated in Design Modeler from Ansys workbench. Then the unstructured quadrilateral mesh was generated in Ansys ICEM meshing software with 380
000 grids. Finally, using Ansys Fluent 2019 R1 software, the numerical results shown in Fig. 6a and b were generated. The Ansys Fluent software uses the finite volume method (FVM) for solving the governing equations. In this work, the coupled method was employed for coupling of pressure and velocity, and the QUICK discretization method was employed to discretize continuity and momentum equations on the computational grids.
Device operation
The device is designed to be operated in two main stages: 1) cuboid loading with the polysil double-coated silicone adhesive tape; and 2) cuboid culture after manual removal of the silicone adhesive tape, and attachment of the bottomless 8-well plate. Prior to loading, we ensured that the trap openings were completely bubble-free and filled the device with ethanol, then water, then PBS. Devices to be utilized for culture were sterilized with 70% ethanol and followed by use of sterile solutions. Cuboid loading was accomplished by manual injection or by pump infusion.
For our loading efficiency experiments, we manually suspended fixed glioma cuboids in a 5 mL syringe containing 20% 8k-PEG (P2139, Sigma-Aldrich). To load the cuboids, we connected the inlet of the device to the 5 mL syringe and used a syringe pump (Fusion 200, Chemyx Inc., Stafford, TX) at a flow rate of 20 mL h−1. For our live glioma cuboid experiments, we suspended glioma cuboids in a 5 mL syringe containing collagen. We manually loaded the cuboids after connecting the inlet of the device to the 5 mL syringe. After loading, we peeled the silicone adhesive from the channel layer and bonded the bottomless 8-well plate using the adhesive on its bottom surface and firm manual pressure. Then we carefully added reagents to each well (usually 0.2 mL per well).
Dye diffusion experiment
We manually filled the device with collagen as above. After gelation of the collagen, we replaced the silicone adhesive tape with tape that had a window cut over only one well, keeping all other wells closed. Then we glued the wells on top and clamped the inlet and outlet shut with clips. After pipetting 200 μL of 1 mM fluorescein into the one well with exposed traps, we covered the well with clear tape to prevent evaporation. We left the device on the microscope at room temperature and took tiled 2× brightfield and fluorescent images at different intervals over 3 days. We subtracted the fluorescence background over similar regions not exposed to fluorescein from the mean fluorescence measured at intervals along the diffusion path.
Author contributions
L. F. H. and A. D. R. designed, performed, and analyzed experiments and wrote the paper. A. A. designed and performed experiments and wrote the paper. K. W. B. designed and performed experiments, and wrote the paper. L. A. B. performed and analyzed experiments and wrote the paper. G. M. analyzed experiments and wrote the paper. A. R. and P. D. performed experiments. T. G. wrote the paper. M. M. designed, analyzed, and performed the numerical simulations and wrote the paper. J. T. C. L. designed experiments and wrote the paper. M. Y. and R. H. P. designed experiments. A. F. designed and analyzed experiments and wrote the paper.
Conflicts of interest
L. F. H. and A. F. are the founders of OncoFluidics, a startup that seeks to commercialize microfluidic drug tests using intact tissues. L. F. H., A. F., A. D. R., P. D., and A. A. Y. are listed as inventors in International Patent Application no. PCT/US2020/51536, which describes the cuboids method and device.
Acknowledgements
This work was partially supported by a grant from the National Cancer Institute R01 CA181445, a pilot grant from Juno Therapeutics, and an Innovation grant from CoMotion at the University of Washington. This work was also funded in part by a Hong Kong Research Grant Council (CityU_11319516 and R1020-18F) for A. A. Y. and M. Y., an International Scholars award from the Consejo Nacional de Ciencia y Tecnología of Mexico for A. R., a Department of Defense Prostate Cancer Research Program W81XWH-18-10358 for J. T. C. L., and the National Science Foundation Graduate Research Fellowship Program for L. A. B. and K. W. B. We thank Paul Yager's group for the use of their laser cutting facility.
Notes and references
- N. Shanks, R. Greek and J. Greek, Are animal models predictive for humans?, Philos. Ethics, Humanit. Med., 2009, 4, 1–20 CrossRef.
- Provocative Questions, 2011, Available at: https://provocativequestions.cancer.gov/expired-rfas-and-pqs/2011-archived-rfas-and-pqs.
- A. Letai, Functional precision cancer medicine—moving beyond pure genomics, Nat. Med., 2017, 23, 1028–1035 CrossRef CAS.
- N. Sachs,
et al., A Living Biobank of Breast Cancer Organoids Captures Disease Heterogeneity, Cell, 2018, 172, 373–386 CrossRef CAS.
- M. van de Wetering,
et al., Prospective Derivation of a Living Organoid Biobank of Colorectal Cancer Patients, Cell, 2015, 161, 933–945 CrossRef CAS.
- L. A. Baker, H. Tiriac, H. Clevers and D. A. Tuveson, Modeling Pancreatic Cancer with Organoids, Trends Cancer, 2016, 2, 176–190 CrossRef.
- S. F. Boj,
et al., Organoid models of human and mouse ductal pancreatic cancer, Cell, 2015, 160, 324–338 CrossRef CAS.
- H. Clevers, Modeling Development and Disease with Organoids, Cell, 2016, 165, 1586–1597 CrossRef CAS.
- D. Gao,
et al., Organoid cultures derived from patients with advanced prostate cancer, Cell, 2014, 159, 176–187 CrossRef CAS.
- L. Huang,
et al., Ductal pancreatic cancer modeling and drug screening using human pluripotent stem cell– and patient-derived tumor organoids, Nat. Med., 2015, 21, 1364–1371 CrossRef CAS.
- C. E. Nash,
et al., Development and characterisation of a 3D multi-cellular in vitro model of normal human breast: a tool for cancer initiation studies, Oncotarget, 2015, 6, 13731–13741 CrossRef.
- C. Pauli,
et al., Personalized in vitro and in vivo cancer models to guide precision medicine, Cancer Discovery, 2017, 7, 462–477 CrossRef.
- M. Astolfi,
et al., Micro-dissected tumor tissues on chip: An ex vivo method for drug testing and personalized therapy, Lab Chip, 2016, 16, 312–325 RSC.
- A. R. Aref,
et al., Screening therapeutic EMT blocking agents in a three-dimensional microenvironment, Integr. Biol., 2013, 5, 381–389 CrossRef CAS.
- R. W. Jenkins,
et al., Ex vivo profiling of PD-1 blockade using organotypic tumor spheroids, Cancer Discovery, 2018, 8, 196–215 CrossRef CAS.
- A. R. Aref,
et al., 3D microfluidic ex vivo culture of organotypic tumor spheroids to model immune checkpoint blockade, Lab Chip, 2018, 18, 3129–3143 RSC.
- A. Ootani,
et al., Sustained in vitro intestinal epithelial culture within a Wnt-dependent stem cell niche, Nat. Med., 2009, 15, 701–706 CrossRef CAS.
- X. Li,
et al., Oncogenic transformation of diverse gastrointestinal tissues in primary organoid culture, Nat. Med., 2014, 20, 769–777 CrossRef CAS.
- J. T. Neal,
et al., Organoid Modeling of the Tumor Immune Microenvironment, Cell, 2018, 175, 1972–1988.e16 CrossRef CAS.
- V. Vaira,
et al., Preclinical model of organotypic culture for pharmacodynamic profiling of human tumors, Proc. Natl. Acad. Sci. U.S.A., 2010, 107, 8352–8356 CrossRef CAS.
- B. P. Murry, B. E. Blust, A. Singh, T. P. Foster and D. Marchetti, Heparanase mechanisms of melanoma metastasis to the brain: Development and use of a brain slice model, J. Cell. Biochem., 2006, 97, 217–225 CrossRef CAS.
- T. C. Chang,
et al., Parallel microfluidic chemosensitivity testing on individual slice cultures, Lab Chip, 2014, 14, 4540–4551 RSC.
- L. F. Horowitz,
et al., Multiplexed drug testing of tumor slices using a microfluidic platform, npj Precis. Oncol., 2020, 4, 1–15 CrossRef.
- A. D. Rodriguez,
et al., A microfluidic platform for functional testing of cancer drugs on intact tumor slices, Lab Chip, 2020, 20, 1658–1675 RSC.
- M. Wang,
et al., Humanized mice in studying efficacy and mechanisms of PD-1-targeted cancer immunotherapy, FASEB J., 2018, 32, 1537–1549 CrossRef CAS.
- O. Jonas,
et al., An implantable microdevice to perform high-throughput in vivo drug sensitivity testing in tumors, Sci. Transl. Med., 2015, 7, 284ra57 CrossRef.
- R. A. Klinghoffer,
et al., A technology platform to assess multiple cancer agents simultaneously within a patient's tumor, Sci. Transl. Med., 2015, 7, 284ra56 CrossRef.
- J. M. Balko and J. A. Sosman, A critical need for better cancer immunotherapy models: Are organotypic tumor spheroid cultures the answer?, Cancer Discovery, 2018, 8, 143–145 CrossRef.
- H. G. Jahnke,
et al., Direct chemosensitivity monitoring ex vivo on undissociated melanoma tumor tissue by impedance spectroscopy, Cancer Res., 2014, 74, 6408–6418 CrossRef CAS.
- K. Simeone,
et al., Paraffin-embedding lithography and micro-dissected tissue micro-arrays: tools for biological and pharmacological analysis of ex vivo solid tumors, Lab Chip, 2019, 19, 693–705 RSC.
- E. W. Esch, A. Bahinski and D. Huh, Organs-on-chips at the frontiers of drug discovery, Nat. Rev. Drug Discovery, 2015, 14, 248–260 CrossRef CAS.
- A. Skardal, T. Shupe and A. Atala, Organoid-on-a-chip and body-on-a-chip systems for drug screening and disease modeling, Drug Discovery Today, 2016, 21, 1399–1411 CrossRef CAS.
- K. Ronaldson-Bouchard and G. Vunjak-Novakovic, Organs-on-a-Chip: A Fast Track for Engineered Human Tissues in Drug Development, Cell Stem Cell, 2018, 22, 310–324 CrossRef CAS.
- I. C. McLean, L. A. Schwerdtfeger, S. A. Tobet and C. S. Henry, Powering ex vivo tissue models in microfluidic systems, Lab Chip, 2018, 18, 1399–1410 RSC.
- L. F. Horowitz, A. D. Rodriguez, T. Ray and A. Folch, Microfluidics for interrogating live intact tissues, Microsyst. Nanoeng., 2020, 6, 69 CrossRef CAS.
- W. H. Tan and S. Takeuchi, A trap-and-release integrated microfluidic system for dynamic microarray applications, Proc. Natl. Acad. Sci. U. S. A., 2007, 104, 1146–1151 CrossRef CAS.
- S. Kobel, A. Valero, J. Latt, P. Renaud and M. Lutolf, Optimization of microfluidic single cell trapping for long-term on-chip culture, Lab Chip, 2010, 10, 857–863 RSC.
- T. Das,
et al., Empirical chemosensitivity testing in a spheroid model of ovarian cancer using a microfluidics-based multiplex platform, Biomicrofluidics, 2013, 7, 011805 CrossRef.
-
M. Astolfi
et al., On-chip trapping and viability assessment of submicroliter primary tissues for personalized treatment of ovarian cancer, in Proceedings of the 17th International Conference on Minitiarization in Chemistry and the Life Sciences, Freiburg, Germany, 2013, pp. 422–424 Search PubMed.
- E. Berthier, E. W. K. Young and D. Beebe, Engineers are from PDMS-land, Biologists are from Polystyrenia, Lab Chip, 2012, 12, 1224 RSC.
- M. W. Toepke and D. J. Beebe, PDMS absorption of small molecules and consequences in microfluidic applications, Lab Chip, 2006, 6, 1484 RSC.
- K. J. Regehr,
et al., Biological implications of polydimethylsiloxane-based microfluidic cell culture, Lab Chip, 2009, 9, 2132 RSC.
- T. A. Moore, P. Brodersen and E. W. K. Young, Multiple Myeloma Cell Drug Responses Differ in Thermoplastic vs PDMS Microfluidic Devices, Anal. Chem., 2017, 89, 11391–11398 CrossRef CAS.
- S. Halldorsson, E. Lucumi, R. Gómez-Sjöberg and R. M. T. Fleming, Advantages and challenges of microfluidic cell culture in polydimethylsiloxane devices, Biosens. Bioelectron., 2015, 63, 218–231 CrossRef CAS.
- J. D. Wang, N. J. Douville, S. Takayama and M. ElSayed, Quantitative Analysis of Molecular Absorption into PDMS Microfluidic Channels, Ann. Biomed. Eng., 2012, 40, 1862–1873 CrossRef.
- B. J. van Meer,
et al., Small molecule absorption by PDMS in the context of drug response bioassays, Biochem. Biophys. Res. Commun., 2017, 482, 323–328 CrossRef CAS.
- V. S. Shirure and S. C. George, Design considerations to minimize the impact of drug absorption in polymer-based organ-on-a-chip platforms, Lab Chip, 2017, 17, 681–690 RSC.
- E. Delamarche,
et al., Microfluidic networks for chemical patterning of substrates: Design and application to bioassays, J. Am. Chem. Soc., 1998, 120, 500–508 CrossRef CAS.
- K. Moshksayan,
et al., Spheroids-on-a-chip: Recent advances and design considerations in microfluidic platforms for spheroid formation and culture, Sens. Actuators, B, 2018, 263, 151–176 CrossRef CAS.
- M. Zanoni,
et al., 3D tumor spheroid models for in vitro therapeutic screening: A systematic approach to enhance the biological
relevance of data obtained, Sci. Rep., 2016, 6, 19103 CrossRef CAS.
- C. Pauli,
et al., An emerging role for cytopathology in precision oncology, Cancer Cytopathol., 2016, 124, 167–173 CrossRef.
- K. Groebe and W. Mueller-Klieser, On the relation between size of necrosis and diameter of tumor spheroids, Int. J. Radiat. Oncol., Biol., Phys., 1996, 34, 395–401 CrossRef CAS.
- A. K. Glaser,
et al., Light-sheet microscopy for slide-free non-destructive pathology of large clinical specimens, Nat. Biomed. Eng., 2017, 1, 84 CrossRef.
- L. A. Barner, A. K. Glaser, L. D. True, N. P. Reder and J. T. C. Liu, Solid immersion meniscus lens (SIMlens) for open-top light-sheet microscopy, Opt. Lett., 2019, 44, 4451 CrossRef CAS.
- A. K. Glaser,
et al., Multi-immersion open-top light-sheet microscope for high-throughput imaging of cleared tissues, Nat. Commun., 2019, 10, 2781 CrossRef.
- L. A. Barner, A. K. Glaser, H. Huang, L. D. True and J. T. C. Liu, Multi-resolution open-top light-sheet microscopy to enable efficient 3D pathology workflows, Biomed. Opt. Express, 2020, 11, 6605 CrossRef.
- A. Klingberg,
et al., Fully automated evaluation of total glomerular number and capillary tuft size in nephritic kidneys using lightsheet microscopy, J. Am. Soc. Nephrol., 2017, 28, 452–459 CrossRef CAS.
-
R. Serafin, W. Xie, A. K. Glaser and J. T. C. Liu, FalseColor-Python: a rapid intensity-leveling and digital-staining package for fluorescence-based slide-free digital pathology, bioRxiv, 2020, DOI:10.1101/2020.05.03.074955.
- J. L. Baratta,
et al., Cellular organization of normal mouse liver: A histological, quantitative immunocytochemical, and fine structural analysis, Histochem. Cell Biol., 2009, 131, 713–726 CrossRef CAS.
- G. Gentric and C. Desdouets, Polyploidization in liver tissue, Am. J. Pathol., 2014, 184, 322–331 CrossRef CAS.
- J. Ruppen,
et al., A microfluidic platform for chemoresistive testing of multicellular pleural cancer spheroids, Lab Chip, 2014, 14, 1198–1205 RSC.
- A. Rodriguez,
et al., A microfluidic platform for the delivery of panels of drugs to live tumor slices, Lab Chip, 2020, 20, 1658–1675 RSC.
- J. Jiang,
et al., A single low-cost microfabrication approach for polymethylmethacrylate, polystyrene, polycarbonate and polysulfone based microdevices, RSC Adv., 2015, 5, 36036–36043 RSC.
- I. R. G. Ogilvie,
et al., Reduction of surface roughness for optical quality microfluidic devices in PMMA and COC, J. Micromech. Microeng., 2010, 20, 065016 CrossRef.
- T. Teshima, H. Ishihara, K. Iwai, A. Adachi and S. Takeuchi, A dynamic microarray device for paired bead-based analysis, Lab Chip, 2010, 10, 2443 RSC.
- H. Noda, Physico-chemical studies on the soluble collagen of rat-tail tendon, Biochim. Biophys. Acta, 1955, 17, 92–98 CrossRef CAS.
- P. Gonzalez-Tello, F. Camacho and G. Blazquez, Density and Viscosity of Concentrated Aqueous Solutions of Polyethylene Glycol, J. Chem. Eng. Data, 1994, 39, 611–614 CrossRef CAS.
- W. R. Knocke, C. M. Dishman and G. F. Miller, Measurement of chemical sludge floc density and implications related to sludge dewatering, Water Environ. Res., 1993, 65, 735–743 CrossRef CAS.
- E. L. Jackson-Holmes, T. C. McDevitt and H. Lu, A microfluidic trap array for longitudinal monitoring and multi-modal phenotypic analysis of individual stem cell aggregates, Lab Chip, 2017, 17, 3634–3642 RSC.
-
P. Kapusta, Absolute Diffusion Coefficients: Compilation of Reference Data for FCS Calibration, 2010, DOI:10.1209/0295-5075/83/46001.
- S. Urien and F. Lokiec, Population pharmacokinetics of total and unbound plasma cisplatin in adult patients, Br. J. Clin. Pharmacol., 2004, 57, 756–763 CrossRef CAS.
- J. Schindelin,
et al., Fiji: An open-source platform for biological-image analysis, Nat. Methods, 2012, 9, 676–682 CrossRef CAS.
-
B. Balázs, J. Deschamps, M. Albert, J. Ries and L. Hufnagel, A real-time compression library for microscopy images, bioRxiv, 2017, DOI:10.1101/164624, p. 164624.
- D. Hörl,
et al., BigStitcher: reconstructing high-resolution image datasets of cleared and expanded samples, Nat. Methods, 2019, 16, 870–874 CrossRef.
- M. G. Giacomelli,
et al., Virtual hematoxylin and eosin transillumination microscopy using epi-fluorescence imaging, PLoS One, 2016, 11, 1–13 CrossRef.
- S. P. Ng, F. E. Wiria and N. B. Tay, Low Distortion Solvent Bonding of Microfluidic Chips, Procedia Eng., 2016, 141, 130–137 CrossRef CAS.
Footnotes |
† Electronic supplementary information (ESI) available: Supplementary methods, Fig. S1–S6, and Movies S1–S3. See DOI: 10.1039/d0lc00801j |
‡ These two authors contributed equally to this work. |
|
This journal is © The Royal Society of Chemistry 2021 |
Click here to see how this site uses Cookies. View our privacy policy here.