DOI:
10.1039/D1GC03471E
(Paper)
Green Chem., 2021,
23, 10014-10029
Computer-aided solvent screening for the fractionation of wet microalgae biomass†
Received
22nd September 2021
, Accepted 9th November 2021
First published on 10th November 2021
Abstract
Microalgae have enormous potential as producers of fine and platform chemicals. However, sophisticated, energy-efficient separation strategies for fractionating the algal biomass are still under research and no economically viable biorefinery process exists to date. An obstacle for the feasibility of microalgal biorefineries is the energy-intense drying step. By fractionating wet algae biomass into the carbohydrate, protein, lipid, and pigment fractions, all valuable target molecules would be available for further purification and conversion while simultaneously eliminating energy expenses for biomass drying. This study presents a computational screening of more than 8000 molecules as solvent candidates for wet extraction and fractionation of microalgae using Phaeodactylum tricornutum as a model organism. In order to eliminate potentially hazardous solvents, environmental, health, and safety properties were considered. Our screening aimed at solvents being partially miscible with water in order to promote accessibility of the solvent to the target molecules contained in the algal cells and phase separation after extraction. Finally, green solvents identified by the computational approach were validated in extraction experiments. The proposed approach is a new computational method for solvent selection in biorefineries. In addition, it is the first study exploiting the water miscibility of solvents for biomass fractionation and wet extraction. 2-butanol was identified as highly effective, green solvent for the wet extraction of algae biomass and outperformed the typically used toxic solvent hexane.
1. Introduction
Climate change and the depletion of fossil resources are global challenges for generations now and in the future. Chemical industry is estimated to be the strongest driving force for increasing oil demand by 2030.1 In order to stop overexploitation of the earth's resources, but to simultaneously fulfil the demand for fine and platform chemicals, new sustainable production routes must be found and the emission of greenhouse gases from the chemical industry must be tremendously reduced. Microalgae have received much interest in the recent years as a potential source for the production of biofuels. In comparison to energy crops, such as maize or rapeseed, microalgae have much higher growth rates.2 Unlike terrestrial plants, algae cultivation does not require arable land and thus, does not compete with food production.3 In a typically proposed biodiesel process of microalgae, the biomass is harvested after cultivation, e.g. by centrifugation. The resulting paste is dried and the lipids are extracted by organic solvents. Often hexane is used for this purpose. However, this solvent is classified as hazardous by solvent selection guides and its substitution by greener alternatives is desirable.4 Several life-cycle and techno-economic assessments of such a process revealed that the drying step is extremely energy-consuming, proposing extraction from wet biomass as an economically more viable route for lipid recovery.5,6 Furthermore, the algal biodiesel process does not efficiently utilise the entire biomass. The lipid fraction can be converted to biodiesel at a cost level not competitive to fossil diesel. The other biomass fractions, namely proteins, carbohydrates and pigments remain unexploited: they often contain value-added molecules but are mostly converted to biogas. As a consequence, this study focuses on valorising all macromolecular fractions of the cell in the scope of a biorefinery concept in which the entire biomass is utilised for the production of fine and platform chemicals, animal feed compounds, neutraceuticals or food ingredients.7–9
In this study, P. tricornutum was chosen as a model alga. This species is a well-studied diatom with a balanced biomass composition containing, depending on cultivation conditions, 18–54% proteins, 3–31% carbohydrates, 14–54% lipids and pigments,10–12 and has large potential for use in a biorefinery.13 Unlike other diatoms, P. tricornutum is not surrounded by a heaviliy silicified cell wall, which facilitates cell disruption.14,15 This species is able to grow phototrophically as well as mixotrophically16 and is reported to grow even on waste streams.17,18P. tricornutum accumulates several high value molecules: fucoxanthin, a red pigment, is the most abundant carotenoid in P. tricornutum known for its anticancer, antihypertensive, anti-inflammatory, and anti-obesity effects.19,20 Another high-value molecule is chrysolaminarin, a polysaccharide accumulated in the vacuoles as a storage carbohydrate.21,22 Chrysolaminarin has immunostimulatory effects in fish,23–25 anticancer properties in human,26 and can be used as a natural plant protection agent.27 In addition, P. tricornutum synthesises the high-value fatty acid eicosapenatenoic acid (EPA) which is a polyunsaturated fatty acid (PUFA) important for human nutrition21,28 but traditionally obtained from unsustainable mass-fishery.29 Other molecules, such as carbohydrates and lipids could be used as starting materials for the synthesis of biofuels.13 Furthermore, algae are a viable protein source with high nutritional value.30P. tricornutum produces all essential amino acids required for human health and are therefore an alternative for meat products.12,30 Due to the variety of products and interesting applications thereof, P. tricornutum is an excellent microalga usable to derive innovative biorefinery concepts.
In order to make the macromolecular fractions available for purification of the high value products or for further conversion, the algal biomass must be separated into proteins, carbohydrates, lipids and pigments, without damaging one fraction during the recovery of another. Several methods are currently researched for that purpose, such as supercritical fluid extraction, aqueous two phase systems, membrane separation, three-phase partitioning and pressurized liquid extraction.31–35 In addition to purely solvent-based methods, also the cell disruption step can be exploited for biomass fractionation. Alavijeh et al. focused on a cell-disruption strategy using bead beating and enzymatic lysis to separate lipids from carbohydrates and proteins of Chlorella vulgaris.36 Also in P. tricornutum, cell disruption steps can be used to facilitate the selective separation bioactive-molecules from biomass.37
Attempts have been made to replace hexane for algae wet extraction with the aim of finding alternatives with greener environmental, health and safety (EHS) properties.38–40 Nevertheless, these studies did not consider the ability of the solvent for biomass fractionation and targeted only the lipid fraction. In addition, the solvent design space was searched experimentally and was therefore rather small, since experiments are consuming time, as well as financial and human resources.
In contrast to purely experimental screenings, computational approaches for solvent selection have the advantage of efficiently exploring a large solvent design space while also giving information about the underlying molecular thermodynamics of the separations. A popular approach for the prediction of thermodynamic properties is COSMO-RS.41,42 COSMO is a continuum solvation model and constructs the surface of a molecule as a cavity of charged, small surface segments. With quantum chemical calculations, their electrostatic charge can be predicted by the electron density. In the mixture, the charged segments of all molecules are paired according to the COSMO-RS approach in order to estimate chemical potential of components in nonideal mixtures.43–45 Instead of decomposing a molecule into several subgroups, as in group contribution methods such as UNIFAC, COSMO-RS treats the molecule as a whole entity and is especially applicable in the pharmaceutical industry and biotechnology where complex, highly functionalized molecules are used.46,47 COSMO-RS is also able to predict thermodynamic properties of ionic liquids (ILs) and deep eutectic solvents (DESs) which were proposed as new classes of green solvents with tunable properties in the recent years. DESs are usually composed of two solid substances which become liquid when mixed in a certain ratio. In the binary mixture, one molecule is serving as a hydrogen bond donor (HBD) and one as a hydrogen bond acceptor (HBA). The charge delocalization occurring through strong hydrogen bonding is responsible for the melting point depression.48,49 Often, molecules used in DESs are obtained from renewable resources, such as choline chloride, sugar or amino acids.50,51 Ionic liquids are salts with a melting point below 100 °C. Owing to their tunable properties they are considered as designer solvents. However, usually many steps are involved in their synthesis which should be critically taken into account.52
COSMO-RS has been recently applied in solvent screenings of biomolecules, e.g. for estimating the IL-solubilities of cellulose,53 partition coefficients of 5-hydroxymethylfurfural in different biphasic solvent systems,54 or extraction of lipids from yeast.55 Furthermore, COSMO-RS was used to predict partition coefficients in biocatalytic biphasic systems56 and was also applied for solvent screenings for lignin-based biorefineries.57,58 Rezaei Motlagh et al. screened solubilities of EPA and docosahexaenoic acid in ILs,59–61 but a solvent screening for microalgae in a biorefinery context has never been presented so far. Recently, Linke et al. developed a COSMO-RS-based solvent screening for catalyst recovery in thermomorphic solvent systems by using QSAR models from VEGA for the prediction of EHS properties and the selection of green solvents which served as a starting point of this study.62
Our goal is to identify solvents for the extraction and fractionation of wet algae biomass by a computer-guided approach. A database containing more than 8000 molecules was screened in silico in order to identify suitable solvents for each biomass fraction of the model microalga P. tricornutum. Among the screened molecules are also DESs and ILs. The screening uses COSMO-RS as the state-of-the-art model for thermodynamic predictions of biomolecules in mixtures. Similar to Linke et al., QSAR models from VEGA were applied to predict selected EHS properties of the solvent candidates.62 The most promising solvent candidates obtained from the computational predictions were then evaluated experimentally. Finally, a biorefinery process concept for the wet fractionation of P. tricornutum was proposed.
This work is structured as follows: in section 2, the connection of the target molecules in the cell is described and based thereon, an extraction strategy is proposed. In this context, the desired phase behaviour of the solvent candidates is described. Section 3 describes the computational details of the solvent screening. Representative biomolecules for each fraction of the algal biomass are defined and each step of the computational screening is explained. Subsequently, the results of the computational screening and the experimental validation are presented and discussed in section 4. From these results, a theoretical biorefinery process for P. tricornutum is concluded in section 5. In section 6, experimental details are briefly explained.
2. Solvent-based extraction strategy
Before suitable solvents for the wet extraction and fractionation of microalgae biomass can be identified, the location of the target molecules within the algal cell must be defined and the interplay of the molecules within the cell must be understood. In P. tricornutum, the target molecules of the extraction (see Table 1) are stored at different locations within the cell as shown in Fig. 1. Fucoxanthin is, together with chlorophyll, tightly connected to protein complexes in the chloroplast.63 In addition, fucoxanthin and other carotenoids are contained in triacylglycerol (TAG)-rich lipid droplets.64 Laminarin is accumulated as a storage carbohydrate in the vacuole.21 EPA is present in polar lipids often contained in membranes.65 It is obvious, that the macromolecular constituents are already fractionated on a cellular level by their accumulation in different organelles. Full cell disintegration disrupts the naturally given compartimentation of the cell. Therefore, no or mild cell disruption techniques should be performed and the extraction of the target molecules should be guided by a solvent selection strategy.
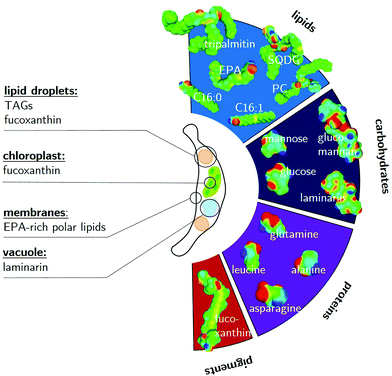 |
| Fig. 1 Representative algae molecules of the diatom P. tricornutum with their sigma surfaces. In accordance with Table 1, the lipid fraction is represented by neutral and polar lipids: tripalmitin, SQDG and PC, as well as the fatty acids C16:0, C16:1 and EPA. The pigment fraction is modeled using fucoxanthin, the most abundant carotenoid in P. tricornutum. Common molecules of the algal carbohydrate fraction are glucose and mannose monomers, as well as the polysaccharides laminarin and glucomannan. The polysaccharides were modeled by a representative chain of their corresponding monomers with similar thermodynamic properties. Proteins were approximated by the four most abundant amino acids in the fraction: asparagine and glutamine as hydrophilic representatives, as well as the more hydrophobic leucine and alanine. On the left-hand side, the most valuable target molecules and their locations within the algal cell are visualised. | |
Table 1 Representative molecules according to their fraction in the algal biomass of P. tricornutum and their reference solvents. Later in the screening, the solubilities of representative molecules in the solvent candidates is compared to the reference solvents. A solvent candidate will be eliminated in case of lower solubility than the reference
Fraction |
Representative molecules |
Reference solvent |
Carbohydrates |
Glucose |
Water |
Mannose |
Laminarin |
Glucomannan |
Proteins |
Leucine |
Water |
Alanine |
Asparagine |
Glutamine |
Pigments |
Fucoxanthin |
Ethanol |
Neutral lipids |
Glycerol tripalmitate |
Hexane |
Polar lipids |
Phosphatidyl choline (PC) |
Ethanol |
Sulfoquinovosyl diacyl glycerol (SQDG) |
Palmitic acid (C16:0) |
Palmitoleic acid (C16:1) |
Eicosapentaenoic acid (EPA) |
Extraction is a two-step process consisting of (i) extraction of the targets from the cell and (ii) separation of the extracted target molecules from the organic phase. Hence, for wet extraction, solvent selection must not only be limited to high solubilities of the target compounds but must also consider the accessibility of the solvent to the target molecules. Since the water content of wet microalgae can be up to 80 wt%,66 the phase behaviour of the solvent/water mixture is a crucial point for a successful wet extraction strategy. Commonly, practically immiscible solvents are used for lipid extraction of wet algae, such as hexane. However, solvents being practically immiscible with water usually attain low lipid yield without prior cell disintegration.38,67 It is proposed, that the resulting phase boundary and extracellular polysaccharides act as a barrier to wet cells such that the water-immiscible solvent has poor access to the target molecules.67,68 In contrast to practically immiscible solvents, Liu et al. experimentally identified a water-miscible solvent for wet lipid extraction69 enabling contact of the solvent to the target molecules. In this approach however, the solvent does not induce phase separation such that co-extracted hydrophilic molecules, for example carbohydrates or proteins, cannot be easily separated from the lipid compounds. This is detrimental for the application in a biorefinery approach. To the best of our knowledge, no study so far considered solvents being partially miscible with water for the extraction of algal cells. The proposed extraction strategy and the phase behaviour of partially miscible solvents is visualised in Fig. 2. Step 1 represents the target compounds in the wet biomass to which pure solvent is added (step 2). In contrast to practically immiscible solvents, partial miscibility with water enables proper penetration of the cell as long as the water content of the wet biomass does not exceed the water capacity of the partially miscible solvent. The solvent can be slowly saturated with water under extraction conditions (step 3 E) in order to step-wise increase the polarity of the mixture and hence, extract lipophilic compounds of different polarities. Exceeding the water capacity by adding additional water to the system enables phase separation (step 4 S). Thus, in contrast to fully miscible solvents, the hydrophilic fractions are transferred to the aqueous phase and the lipophilic fractions to the organic phase. In the depicted ternary system, the concentration of the target molecules is low compared to the solvent and water concentration. Hence, for the screening procedure it is reasonable to search for binary solvent/water mixtures and neglect the influence of the target compounds on the liquid–liquid-equilibrium (LLE). After phase separation of the binary solvent–water system, the solvent content in the aqueous phase xaqsolv should be low since this would complicate solvent recycling. The water content in the organic phase
should be high in order to promote accessibility of the solvent to the target compounds. Due to the advantages of such phase behaviour for extraction, this study focuses on the screening for partially miscible solvents.
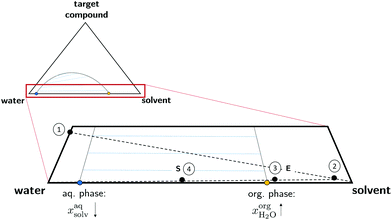 |
| Fig. 2 Proposed extraction strategy and phase behaviour of partially miscible solvents for the wet extraction of algae biomass. Initially (step 1) the target compounds are contained in the wet biomass (ca. 80 wt% water) to which pure solvent is given (step 2). Before reaching the extraction point (step 3 E), the solvent can be saturated with water. After extraction, the addition of surplus water leads to phase separation (step 4 S). Since the concentration of the target molecules is negligibly low, the assumption is made that the target molecules do not influence the solvent–water–LLE significantly. Consequently, the composition of the resulting aqueous and organic phase resembles that of the binary solvent/water mixture, as indicated by a blue and orange dot, respectively. The solvent content in the aqueous phase xaqsolv should be low whereas the water content in the organic phase should be high. | |
3. Computational methods
The screening was carried out by filtering molecules from a database of more than 8000 molecules according to favourable pure compound data, thermodynamic properties, and EHS criteria (Fig. 3). In a pre-screening step, solvent candidates with preferred pure compound properties, high solubilities of the algal biomolecules, and favorable EHS criteria were identified. The algal biomass was modeled by defining representative biomolecules for each major biomass fraction. In a second screening step, thermodynamic properties of the solvent candidates were considered. The solvent candidates must be partially miscibile with water to ensure penetration into the cell during extraction, and partitioning of the target molecules after phase separation. The LLE behaviour of the solvent candidates with water were predicted using COSMO-RS. The resulting LLE was used to parameterise COSMO-RS calculations for the partition coefficients of the representative algae molecules between the aqueous and organic phase to evaluate their potential for fractionation of the biomass. In order to be considered as a solvent candidate, the solvent must fulfill certain criteria in each step which are explained in more detail in sections 3.1 and 3.2 where also the modelling of the representative algal molecules is described. Computational details of the utilised hardware and software can be found in the ESI.†
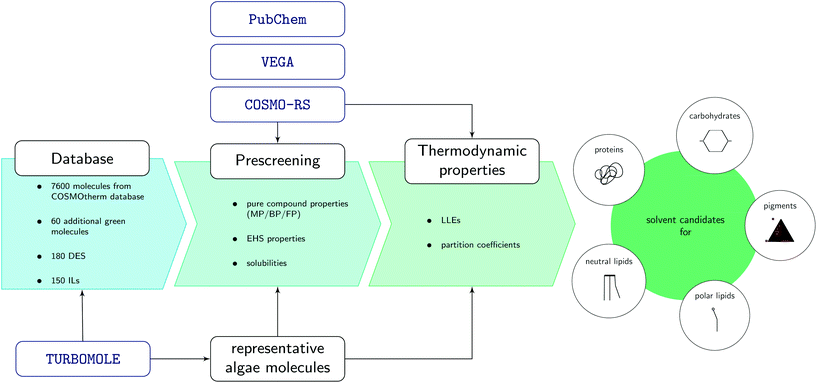 |
| Fig. 3 Approach applied in the computational solvent screening: a database containing more than 8000 molecules was first prescreened for suitable pure compound properties as automatically requested by PubChem, EHS properties predicted by VEGA models, and high solubilities of representative algal molecules calculated by COSMO-RS. For solvents passing the prescreening step, thermodynamic properties were predicted by COSMO-RS. For the wet extraction step, the solvents must be partially miscible with water. For solvents passing this screening step, partition coefficients of the representative algal molecules in the solvent–water system were predicted, resulting in a list of suitable solvents. | |
3.1. Representative biomolecules and reference solvent system
Biomass is a complex mixture of different biomacromolecules, which is far beyond to be modeled in all its details given by the variety of different molecules and their interactions. In order to reduce this complexity, molecules of each macromolecular fraction, namely carbohydrates, proteins, pigments, and lipids, were modeled individually. Each fraction was further divided into biochemical subgroups and representative molecules were chosen, taking into account
• all desired target molecules,
• the abundancy of the molecules from each biochemical class,
• storage organelles of the target molecules and implications thereof on the solvent (accessibility),
• computing effort.
Fig. 1 presents all representative molecules which are in total 15 substances.
3.1.1. Lipids.
The lipid fraction constitutes of two classes featuring different polarities: neutral and polar lipids.65 The most abundant neutral lipids in P. tricornutum are TAGs and are located inside lipid droplets.64,65 TAGs in P. tricornutum are mainly containing the fatty acids C16:0 and C16:1.64,65 Since the TAG tripalmitin contains three C16:0 fatty acid chains, it was chosen as a representative molecule. Polar lipids are often constituents of lipid organelle bilayers or precursor molecules in the lipid metabolism and contain high amounts of EPA.64,65 According to Yang et al., phosphatidylcholine (PC) and sulfoquinivosyl diacyl glycerol (SQDG) are highly abundant polar lipid classes in P. tricornutum65 and were therefore selected as representative molecules. Since fatty acids are also more polar than the neutral lipid fraction due to the influence of their –COOH group, palmitic acid (C16:0) was selected as a model for saturated free fatty acids, palmitoleic acid C16:1 as a representative for monounsaturated fatty acids (MUFAs) and EPA as a PUFA.
3.1.2. Pigments.
Pigments are located in the fucoxanthin-chlorophyll-complexes in the thylakoid membranes of the algal chloroplast.63 Furthermore, carotenoids, mostly fucoxanthin and beta-carotene are accumulated in lipid globules.64 Fucoxanthin is a value-added molecule and is the most abundant carotenoid in P. tricornutum.70,71 Although chlorophylls are even more abundant in P. tricornutum, fucoxanthin was selected as a representative molecule for the pigment fraction because it is located in both organelles, chloroplast and lipid droplets.
3.1.3. Carbohydrates.
The carbohydrate fraction consists of mono- and polysaccharides. The main monosaccharides are mannose and glucose which are therefore suitable representatives for the carbohydrate fraction.12,72 Polysaccharides are long and branched polymer chains, and can be found as exopolysaccharides surrounding the cell, as storage polysaccharides as in the case of chrysolaminarin, or as insoluble part of the cell wall. QM calculations or large biomolecules are very time-consuming and for this reason the polysaccharide fractions are approximated by model polysaccharides as by Chu et al.73 One suitable representative is a β(1→3)-glycosidic linked polysaccharide made of three glucose monomers, similar to chrysolaminarin stored in the vacuoles of P. tricornutum which contains β(1→3) and β(1→6) linked glucose monomers in a ratio of 11
:
1.74P. tricornutum is also known to produce immunostimulatory, water-soluble polysaccharides rich in glucose and mannose.75–77 A simplified model molecule, consisting of in total four glucose and mannose units connected via β(1→4) glycosidic linkages, was used as a representative molecule. Both chrysolaminarin, as well as the mannose and glucose-rich polymer are known to be water-soluble.22,76 This thermodynamic behaviour also applies to their representative model carbohydrates as used in this work. Our screening focuses only on water-soluble carbohydrates, since the insoluble part serves as stabilizing polymers in the cell wall78 and remains as cell debris at the end of the extraction process.
3.1.4. Proteins.
Proteins are the most challenging fraction of the cell to model. Proteins are polypeptides consisting of amino acid sequences linked via peptide-bonds and are folded due to various biochemical interactions. Depending on their amino acid side chains, they can be soluble or insoluble in water. In P. tricornutum, most of the proteins are water-soluble.79 Hence, the screening focuses on the water-soluble protein fraction. Although, it is possible to simulate an entire protein molecule using Turbomole80 such computations are very time-consuming. Di- or tripeptides constitute smaller building blocks of proteins. Their water-solubility, however, strongly depends on their amino acid composition.81 Single amino acids as building-blocks for proteins, on the contrary, can be modeled in much shorter time. Therefore, in this study, the four most abundant amino acids in P. tricornutum, namely glutamine, asparagine, alanine, and leucine were used to represent the protein fraction.12,79 The selected amino acids exhibit different thermodynamic behaviour: glutamine and asparagine are more hydrophilic than alanine and leucine.82 At neutral pH, as given for the majority of solvents in the screening procedure, the zwitterionic form of amino acids is present83 which was used in the QM calculations.
3.1.5. Reference solvent system.
In previously studied microalgal extraction processes, only the lipid fraction was targeted for biodiesel or colorant production, whereas the rest of the biomass had only low valued use. In these processes, the lipid fraction was often extracted with unpolar solvents, such as hexane. To enable better penetration of the wet cells, more polar solvents should be added, such as ethanol.68 Also water is still present in the system, since the drying step is to be omitted for energetic reasons. Common moisture contents after harvest range from 75 to 80 wt%.66 Hence, the reference solvent is either hexane, ethanol, or water. The solvent with the highest solibility of the representative molecule is assigned as the reference solvent. The reference solvents are later used to define the limits of the screening, especially with regard to EHS properties, and solubility thresholds. Table 1 summarises the molecules chosen for each fraction, and their reference solvent.
3.2. Solvent screening approach
3.2.1. Database.
The compiled database consists of the COSMOthermX19 integrated database, COSMObase13-01, and COSMObaseIL19-01. Additionally, green solvents from Moity et al.,84 and the green solvent Cyrene85 were added manually. Duplicate solvents were deleted. In this screening, 178 pairs of DESs48,86–95 and 143 commercially available IL pairs96,97 were added to the search space, leading to a total of 8011 molecules defining the solvent search space. A complete list of screened solvents can be found in the ESI.†
3.2.2. Prescreening.
From the database, molecules were pre-screened in several steps. First, single anions and cations were eliminated from the list since the single molecules were not used as solvents but only in combination as ILs. Subsequently, pure component properties, such as melting point (MP) and boiling point (BP) at atmospheric pressure were used as elimination criteria. The limits for eligible solvent candidates are summarised in Table 2. The MP must be higher than 25 °C to ensure liquid solvent candidates at extraction temperature (room temperature). The BP was restricted to be higher than 40 °C and lower than 120 °C in order to ensure facile downstream processing. In case that MP and BP were not already given in the database, an automated PubChem query retrieved missing experimental data. If there was still no data for the BP, missing data was predicted by COSMO-RS. Solvents with missing data were kept in the screening in order to prevent false exclusion. Subsequently, EHS properties were predicted using VEGA models.98 VEGA comprises different models to predict EHS properties based on quantitative structure–activity relationships (QSAR). VEGA is a useful tool for green solvent selection since also a reliability score is given in addition to the EHS property predictions in order to further evaluate the validity of the obtained results. Using the results and the reliability scores, an overall greeness score was predicted as proposed by Linke et al.62 Based on the score, the suitability of a solvent in terms of EHS criteria was estimated. Solvents with low overall greenness score were excluded in order to avoid time-consuming thermodynamic calculations of mixtures containing potentially hazardous substances. In brief, for each solvent candidate, different models were used to predict the EHS properties as given in Table 3. The EHS model results are represented by different data types, such as numeric values for fish toxicity or qualitative responses, such as “mutagenic” or “toxic”. In addition to the VEGA predictions, flash points (FPs) were calculated using COSMO-RS. These outputs were converted to a color code accompanied score. The score is element of the set {0, 0.25, 0.5, 0.75, 1}, where “green – 1” corresponds to desirable “green” EHS properties, over “green-yellow – 0.75”, “yellow – 0.5”, “yellow-red – 0.25”, to “red – 0”. Since several models can predict one EHS property, the model results were unified, taking also the model reliability into account. The score of each unified model result was averaged by the number of used models to generate the overall “greenness” score (OGS). VEGA is not applicable for ILs since these salt-like molecules were not included in the training-set of the VEGA models. Hence, their OGS was set to 1.0 in order to prevent false exclusion, and EHS characteristics were manually checked at the end of the screening. For DESs, the OGS for the HBA and HBD were predicted separately. In order to obtain the OGS for the whole DESs, the scores for the HBA and HBD were averaged and weighted by their molar fractions. VEGA models were recently applied for a screening of DESs by Song et al.99 and are a reasonable choice given the number of solvent candidates. However, recent studies regarding the toxicity of DESs suggest, that the toxicity of the DES might be higher than that of its single components.100 In the screening, a molecule must have an equal or a higher OGS compared to hexane which is the solvent candidate with the lowest OGS from the reference solvent system. Single molecules were falsely predicted to have unsuitable EHS properties due to model uncertainties, and were therefore manually re-added to the screening. These solvents are listed in the ESI.† Subsequently, the ability of the appropriate solvent candidates to solubilise the representative algal molecules was predicted using COSMO-RS. COSMOtherm outputs the solubility in terms of log10(xsolub) where a value of zero means that two substances are fully miscible: |  | (1) |
Table 2 Boundaries for the prescreening procedure and screening for partially miscible solvents. The limit for the overall greenness score (OGS) corresponds to the most toxic reference solvent hexane. Solubilities are averaged over all target molecules per fraction from Table 1
Property |
Boundaries |
Boiling point (BP) at 1 atm |
40 °C ≤ BP ≤ 120 °C |
Melting point (MP) |
MP ≤ 25 °C |
Overall greenness score (OGS) |
OGS > 0.79 |
Solubility  |
|
Water in org. phase  |
|
Solvent in aq. phase (xaqsolv) |
≤0.1 |
Table 3 QSAR models from VEGA used to predict selected EHS properties
Property |
Model |
Version |
Mutagenicity |
CONSENSUS |
1.0.3 |
CAESAR |
2.1.13 |
SarPy/IRFMN |
1.0.7 |
ISS |
1.0.2 |
KNN/read-across |
1.0.0 |
Carcinogenicity |
CAESAR |
2.1.9 |
ISS |
1.0.2 |
IRFMN/Antares |
1.0.0 |
IRFMN/ISSCAN-CSX |
1.0.0 |
Developmental toxicity |
CAESAR |
2.1.7 |
PG |
1.1.0 |
Endocrine disruptor potential |
IRFMN |
1.0.1 |
IRFMN/CERAPP |
1.0.0 |
Skin sensitization |
CAESAR |
2.1.6 |
Hepatoxicity |
IRFMN |
1.0.0 |
Fish acute toxicity |
SarPy/IRFMN |
1.0.2 |
KNN/read-across |
1.0.0 |
NIC |
1.0.0 |
EPA (96 h) |
1.0.7 |
Daphnia toxicity |
EPA (48 h) |
1.0.7 |
DEMETRA |
1.0.4 |
Bee acute toxicity |
KNN/IRFMN |
1.0.0 |
Bioaccumulation factor |
CAESAR |
2.1.14 |
Meylan |
1.0.3 |
KNN/read-across |
1.1.0 |
Biodegradability |
Arnot/Episuite |
1.0.0 |
IRFMN |
1.0.9 |
Persistence (sediment) |
IRFMN |
1.0.0 |
Persistence (soil) |
IRFMN |
1.0.0 |
Persistence (water) |
IRFMN |
1.0.0 |
log P (octanol/water) |
Meylan/Kowwin |
1.1.4 |
MlogP |
1.0.0 |
AlogP |
1.0.0 |
The chemical potential of the pure solute is denoted by μ(pure), the chemical potential of the solute at infinite dilution in the solvent as μ(solvent)(x∞). The free enthalpy of fusion is given as ΔGfus, which is zero for liquid compounds. For solid compounds, ΔGfus was estimated by a QSPR approach within COSMOtherm. COSMOtherm yields fast, approximative predictions of the solubility and is therefore a proper tool for the screening in large databases. The solubilities of all representative biomolecules of one fraction in one solvent were averaged to obtain the mean value of the overall fraction log10(
frac):
| 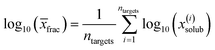 | (2) |
where
ntargets indicates the number of representative molecules per fraction. COSMO-RS predictions and experimental values for a subset of solvents were qualitatively well in line (see ESI, section 6
†). If log
10(
frac) in a potential solvent candidate was higher or equal to that of the reference solvent, the candidate passed the prescreening procedure. Finally, the appropriate solvent candidates are assigned to each fraction based on the solubilities of the corresponding fraction.
3.2.3. Multi-component thermodynamic property screening.
After the prescreening, thermodynamic properties of the solvent/water mixtures were predicted at normal temperature of 25 °C and atmospheric pressure. Binary LLEs of each solvent candidate with water were calculated using COSMO-RS. Only candidates with the desired phase behaviour passed this screening step. For the lipophilic fractions, namely polar lipids, pigments and neutral lipids, partial miscibility with water was a required property. In the context of this paper, partial miscibility is expressed as
, where
denotes the molar fraction of water in the organic phase. Furthermore, there should be only low solvent concentration in the aqueous phase xaqsolv < 0.1. All solvents within these boundaries passed this screening step.
The hydrophilic fractions, carbohydrates and proteins, are both water-soluble. There are two options for their separation from each other: first, either solvents with high solubility of the one, but low solubility of the other fraction can be identified. In this scenario, the fraction with high solubility in a first solvent is extracted into the organic solvent phase, while the other fraction remains in the biomass and can be extracted in a subsequent step. In the second scenario, no such solvent can be identified such that both fractions are simultaneously extracted from the biomass. Hence, separation of both fractions is only achievable by phase separation within the postulated separation strategy. Thus, one fraction would dissolve in the aqueous phase, while the other fraction is located in the organic phase. In order to account for both possible scenarios, in addition to the solubility calculations, also for the hydrophilic fractions binary LLEs of the solvent candidates with water were predicted.
For all biomass fractions, partition coefficients were calculated using COSMO-RS at infinite dilution of all single representative molecules. The calculation was parameterised by the phase composition of the previously predicted LLEs. If a partition coefficient of a single representative molecule had a different sign as compared to the other representatives of the fraction, meaning that this representative molecule would move to the other phase, the solvent candidate was excluded. After this first selection step, the average of the estimated partition coefficients for each fraction was calculated. Furthermore, solvents not able to separate hydrophilic from lipophilic fractions as indicated by their averaged partition coefficients were eliminated from the list of suitable solvent candidates. In the screening of solvents for the hydrophilic fractions, additionally solvents which cannot separate proteins from carbohydrates were excluded.
3.2.3.1. Solvent candidate lists.
The EHS properties and availability of the eligable solvent candidates were examined manually by checking their safety data sheets, price and possible suppliers. The solvents must be available at least in bulks larger than 100 ml with a price lower than 100€ and must not be toxic or eco-toxic according to their safety data sheets. Furthermore, LLE data predicted by COSMO-RS was compared to literature. In case that a solvent candidate did not meet the desired criteria, it was manually excluded. A list of candidates is given separately in section 4.2.
4. Results and discussion
4.1. Prescreening
The results of the prescreening are summarised in Fig. 4. At first, molecules with unsuitable structure were removed from the list of solvent candidates (structural constraints) as described in section 3.2.2. The given MP/BP criteria ruled out most molecules from the screening procedure. Especially a high number of DESs was excluded during this step due to their high BPs as predicted by COSMO-RS. BPs for all ILs were uncertain as stated in a warning of COSMOtherm, hence they were kept in the screening to eliminate false exclusion. However, DESs and also ILs are known for their low vapor pressure which contributes to their green properties, but also renders them difficult to be recovered by evaporation.101,102 After this step, around 28% of the solvent candidates were eligible. The screening for green EHS properties (green properties) reduced the number of solvent candidates from 2205 to 1112 suitable solvent candidates. In most cases, VEGA models were well in line with the corresponding information obtained from safety datasheets of the solvents. In the next step, solubilities of the representative algal molecules from Table 1 of the solvent candidates were predicted. There were only minor differences in the solubilities of the representatives in one fraction (e.g. between SQDG and C16:0 for polar lipids), such that an average solubility value for the whole fraction is a suitable measure. This furthermore justifies the classification of the representative molecules into fractions and shows that COSMO-RS is an appropriate prediction method for this study. All molecules with solubility higher than or equal to the reference solvent (see Table 1) were assigned to the corresponding biomass fraction. The highest number of solvent candidates was obtained for pigments and polar lipids. Pigments and polar lipids in ethanol have a lower solubility than proteins and carbohydrates in water or neutral lipids in hexane. Hence, the solubulity threshold given by the limits defined by the reference solvents to pass this screening step was lower than that for hexane and water. For the lipophilic fractions, nearly all ILs were ruled out in this step due to the low solubility. Many DESs contained in the database are predicted to have high solubilities for pigments and polar lipids. For neutral lipids, however, DESs could not compete with hexane in terms of solubility. The solvent candidates passing the prescreening step can be found in the ESI.†
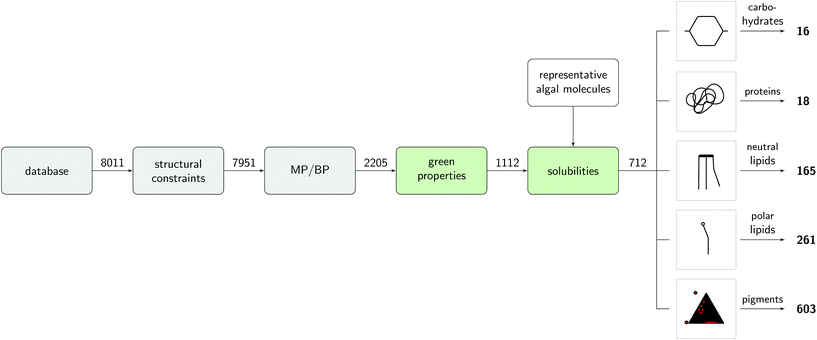 |
| Fig. 4 Prescreening procedure: from the database, solvents with unsuitable structure, pure compound properties and EHS criteria were eliminated. Subsequently, the solubilities of the representative algal molecules in each remaining solvent candidate were predicted. The solubilities were averaged over all candidates contained in each fraction. Each solvent with a higher solubility than the reference solvent of the fraction, finally passed the prescreening step. Numbers above the arrows indicated the number of solvents after each screening step. Note that after the last step, one solvent can be a suitable candidate for more than one fraction. | |
An additional, detailed screening for the extraction and separation of the carbohydrate and protein fraction is supplied in the ESI.† Within the screening of the hydrophilic fractions, several ILs with high carbohydrate solubility were identified. However, they were not selective enough to specifically extract carbohydrates without dissolving other biomass fractions. Furthermore, no solvent candidate could separate proteins from carbohydrates as expected. As a consequence, water is the solvent candidate identified for the hydrophilic fractions and other separation techniques might be more suitable to separate these two fractions. This result underlines the importance of water in wet biomass fractionation and hence, thermodynamic properties of solvent/water mixtures are crucial. The following screening steps focus on the lipophilic fractions as target molecules. Section 4.2 reports the applied procedure to screen appropriate solvents for the wet extraction of pigments, polar lipids and neutral lipids using partially miscible solvents.
4.2. Wet extraction and fractionation of the lipophilic fractions using partially miscible solvents
After the prescreening as described in section 4.1, we estimated the binary LLEs between the eligible solvent candidates for neutral lipids, polar lipids and pigments and water using COSMO-RS. For wet extraction, our goal was to identify solvents that are partially miscible with water. Fig. 5 summarises the results of the sequential screening steps: in the first step (LLE), solvents/water mixtures with miscibility gap were identified. In the next step (partial miscibility), the cross-solubility of the solvent candidates and water was further examined. Only solvent candidates exhibiting partial miscibility with water as defined in section 3.2.3 were passing this screening step. After this step, the number of solvents was nearly halved from 620 solvents after the LLE step to 328 after the partial miscibility step. Especially for neutral lipids, this step strongly reduced the number of suitable solvent candidates. A list of all solvent candidates can be found in the ESI.†
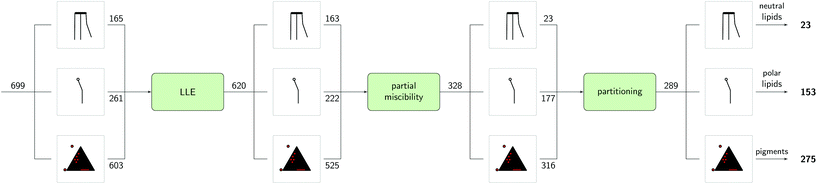 |
| Fig. 5 Thermodynamic property screening for wet extraction: after the prescreening step, 699 solvents for the lipophilic fractions remain for thermodynamic property screening. Subsequently, the solvent must form two phases with water (LLE). In the next screening step, partial miscibility with water was required. Next, partition coefficients were calculated. It was required, that lipophilic molecules preferably dissolve in the organic phase and the hydrophilic molecules in the aqueous phase. Numbers above the arrows indicate the respective number of solvent candidates after each screening step. | |
Certain solvents have a high water uptake. We estimated the solubilities of the representative molecules in the water-saturated solvents to evaluate the effect of the water capacity as expressed by
on the solubility of the lipophilic fractions. For COSMO-RS calculations, the solubilities of the representative molecules were computed at the phase compositions of the already calculated binary water/solvent LLE. The presence of water in the organic solvent strongly affects the solubilities of the representative molecules compared to those of pure solvents, as can be seen in Fig. 6. It shows that with increasing water content of the solvents, the solubilities of all lipophilic compounds decreased up to several log units. E.g. 2-butanol is predicted to have a mole fraction of
water in the organic phase. The high water content decreases the solubility of neutral lipids from log10(xsolub) = −2.25 to −14.87, whereas the carbohydrate solubility increases from log10(xsolub) = −7.51 to −2.24. These results indicate that partially miscible solvents with high water content in the organic phase are not able to sufficiently dissolve neutral lipids from the biomass and hence, they give the possibility to further separate the lipid fraction into polar lipids/pigments and neutral lipids. The neutral lipids remaining in the biomass after the first extraction step using partially miscible solvents could be extracted in a subsequent extraction stage. Since partially miscible solvents have a low solubility for neutral lipids when saturated with water, practically immiscible solvents with suitable EHS properties could be applied after complete cell disruption to extract the neutral lipids.
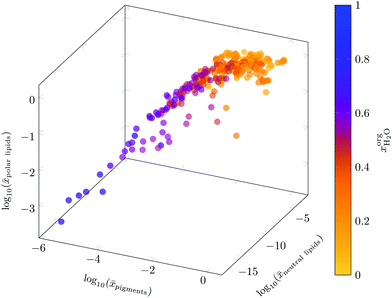 |
| Fig. 6 Solubilities of the lipophilic fractions in water-saturated solvents. The colorbar indicates the mole fraction of water in the organic phase . The solubility in water saturated solvents decreases for all lipophilic fraction with increasing water content, especially for neutral lipids. | |
While high solubilities ensure a high capacity of the target molecules, the partition coefficient describes the selectivity of the separation. Therefore, the results of the LLE calculations were subsequently used to parameterise predictions for the partition coefficients of the representative molecules between the aqueous and the organic phase. As visible in Fig. 5, 39 solvents were excluded during this screening step (partitioning). The dependence of the partition coefficients log
P on the water content in the organic phase
is exemplarily presented in Fig. 7 for carbohydrates pigments. It can be clearly seen that a high water content in the organic phase has a detrimental effect on the separation of hydrophilic and lipophilic molecules because partition coefficients approach zero. The log
P-plots for all other fractions which were extracted from the biomass by partially miscible solvents are contained in the ESI.†
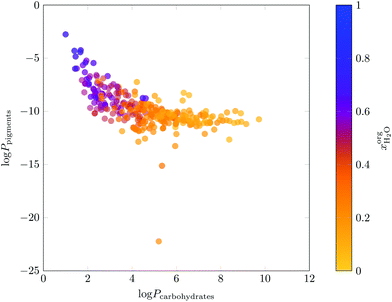 |
| Fig. 7 Partition coefficients log P for carbohydrates and pigments as predicted by COSMO-RS. The colorbar indicates the water content of the organic phase . Partition coefficients are approaching zero with increasing water content. | |
After compiling the final list of solvents as described in section 3.2.3.1, the final solvent candidates of the computational screening are listed in Table 4 remained. For experimental evaluation, five of these promising candidates were selected based on their water capacity as given by the experimental LLEs, and the predicted solubilities in the water saturated solvent.
Table 4 Solvent candidates for wet extraction identified by the computational screening procedure. BPs and FPs were taken from PubChem.103 The enthalpy of vaporization ΔHvap was taken from the NIST Webbook.104 LLE data is given at 25 °C and atmospheric pressure unless otherwise indicated. Solubilities log10(xsolub) of the fractions in the water saturated-solvents were predicted by COSMO-RS at 25 °C, whereby zero indicates full miscibility. The solvent/water-mixtures for the solubility calculations were parameterised by the LLE as predicted by COSMO-RS. EHS properties were estimated using VEGA models and validated by safety data sheets
|
Pure compound data |
LLE data |
log10(xsolub) |
|
Solvent |
BP [°C] |
FP [°C] |
ΔHvap [kJ mol−1] |
 (exp.) |
 (COSMO) |
Neutal lipids |
Polar lipids |
Pigments |
Protein |
Carbohydrates |
EHS |
2-Butanol |
99.5 |
24 |
49.86 |
0.71105 |
0.74 |
−14.87 |
−2.87 |
−5.03 |
−1.91 |
−2.24 |
Flammable, irritant |
1-Butanol |
117.7 |
29 |
52 |
0.49106 |
0.5 |
−9.92 |
−0.84 |
−2.82 |
−2.71 |
−3.17 |
Flammable, irritant, corrosive |
Isobutanol |
108 |
28 |
51 |
0.16107 (40 °C) |
0.48 |
−9.87 |
−0.89 |
−2.88 |
−2.78 |
−3.3 |
Flammable, irritant, corrosive |
Methyl acetate |
57 |
−13 |
33 |
0.26108 |
0.25 |
−8.65 |
−0.23 |
−1.36 |
−3.32 |
−3.99 |
Flammable, irritant |
Ethyl formate |
54.4 |
−20 |
32.2 |
0.17109 |
0.12 |
−7.22 |
−0.13 |
−1.06 |
−4.18 |
−5.05 |
Flammable, irritant |
2-MeTHF |
78 |
−10 |
34 |
0.16110 (19.3 °C) |
0.8 |
−15.3 |
−2.95 |
−5.2 |
−2.01 |
−1.75 |
Flammable, irritant, corrosive |
Ethyl acetate |
77.1 |
−4 |
35 |
0.13108 |
0.17 |
−6.35 |
0 |
−0.54 |
−4.24 |
−5.41 |
Flammable, irritant |
Methyl propionate |
−87.5 |
79.8 |
35.95 |
0.12111 (50 °C) |
0.13 |
−5.98 |
−0.01 |
−0.74 |
−4.71 |
−6.11 |
Flammable, irritant |
The 2-butanol/water mixture predicted by COSMO
has a low solubility for the neutral lipid fraction. This behaviour is especially interesting for the fractionation of the lipophilic fractions. 1-Butanol and isobutanol both have similar water capacities and are predicted to have comparable solubilities of the different fractions. Isobutanol has a lower BP and a slightly lower enthalpy of vaporization which is favorable regarding the energy consumption for solvent regeneration. However, more detailed phase equilibrium data with water is available for 1-butanol. Therefore, 1-butanol was chosen for experiments instead of isobutanol. Methyl acetate was selected due to its water capacity, which is in the medium range compared to the other solvents. This gives the possibility to study the dependency of the influence of the water capacity on the yield. COSMO-RS was over predicting the water capacity of 2-MeTHF. Since the experimental water capacity of 2-MeTHF is similar to that of ethyl formate, but ethyl formate has more favorable properties for evaporation, ethyl formate was the more interesting candidate for experimental evaluation. Ethyl formate requires the lowest energy for evaporation compared with all other solvents. In other studies, 2-MeTHF was already shown to be a suitable candidate for lipid extraction of other microalgal species after complete cell disruption.38,39 Ethyl acetate and methyl propionate have the lowest water capacity compared to the other identified solvents and high solubilities for pigments and polar lipids. LLE data for methyl propionate was only available at a temperature of 50 °C. In our experiments, it was not possible to obtain monophasic extraction conditions at room temperature for methyl propionate rendering it a practically immiscible solvent. Hence, only ethyl acetate was further studied in experiments. Note that although the solvents can be considered as green in contrast to hexane, they still require precautionary measures. If it comes to contact with the solvents they can cause eye damage, respiratory tract irritation, skin irritation and also have narcotic effects. Hence, protective clothes and a fume hood should be used.
The list of suitable DESs as given in the ESI† contains in total seven candidates of which only one has a known melting point. This DES is a mixture of decanoic and dodecanoic acid, and hence, it is made of compounds from renewable sources. The predicted water content in the DES phase of all seven candidates was rather low. Furthermore, the screening identified two trihexyltetradecylphosphonium-based ILs for the wet extraction of the lipophilic compounds. Both ILs were also predicted to have a high solubility of proteins and carbohydrates, however the hydrophilic fractions are predicted to be transferred to the aqueous phase after phase separation, as for the other solvents. The two identified ILs are known as Cyphos IL 103 and 104. The water capacity was determined to be around 20% by Fraser et al. and is lower than predicted by COSMO-RS.112 However, wet extraction is the central point of a biorefinery process and the products must be easily recoverable from the solvent. The recovery of poorly volatile or thermally labile products, such as pigments or fatty acids, is a known problem in IL and DES extraction. Advanced separation strategies are necessary for mild product recovery and are currently beeing researched.102,113
4.3. Experimental validation
Fig. 8 shows the yield of all lipophilic components after extraction of undisrupted wet algal biomass with the in the screening identified solvents. In comparison, extractions using hexane and hexane/ethanol were performed under the same conditions. Extraction with chloroform/methanol on completely disintegrated cells serves as a reference for the amount of total lipophilic compounds within the biomass. All solvents identified in the screening performed better than pure hexane and the hexane/ethanol mixture, both having a yield of only around 1%. Surprisingly, 2-butanol with the highest water capacity and the lowest solubilities for the lipophilic fractions as in accordance with predictions of COSMO-RS, attained the highest yield of 34%. We observed, that not only solubility, but mainly accessibility of the solvent to the target molecules as given by high water contents in the solvent are crucial for high yield of lipophilic compounds as prior hypothesised in section 2. See also ESI† for a more detailed analysis. In addition, FTIR spectra taken of each of the extracts confirmed that all extracts were rich in lipids as can be seen by the peaks at 1745 cm−1, see ESI.† Since 2-butanol has the most advantageous properties, the residual biomass after 2-butanol extraction was stained using BODIPY 505/515, a dye that bins specifically on neutral lipid compounds, as shown in Fig. 9. Several lipid globules are visible after extraction which indicates that neutral lipids indeed remain in the biomass which is in line with the COSMO-RS predictions in section 4.2. This is qualitative evidence that 2-butanol has not only a high yield, but also is able to fractionate the lipid fraction and is therefore the best solvent for wet extraction and fractionation in this study. All solvents with high yield, such as 2-butanol, 1-butanol methyl acetate or ethyl acetate, form azeotropes with water.114 This complicates solvent recovery by distillation. However, the predictions in this study show that water/solvent mixtures have beneficial properties as compared to the pure solvent. Hence, the solvent must not necessarily be a pure component.
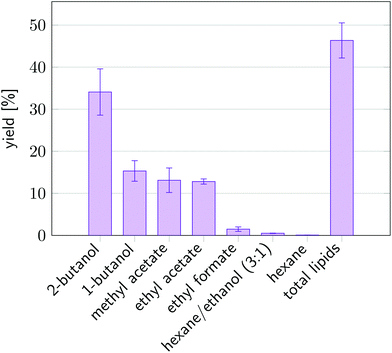 |
| Fig. 8 Yield of lipophilic compounds as extracted by the solvents identified in the screening, compared to hexane/ethanol and hexane on intact cells of P. tricornutum. Extraction with chloroform/methanol on completely disrupted biomass serves as a reference (total lipophilic compounds). All experiments were performed on wet biomass. | |
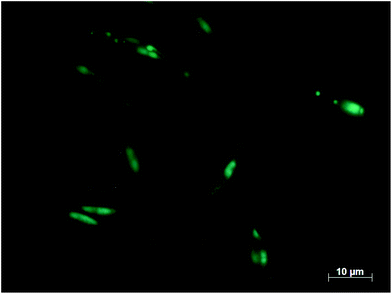 |
| Fig. 9 Neutral lipids of P. tricornutum after 2-butanol extraction stained with BODIPY 505/515. Neutral lipids remain in the cell after extraction and appear as blue-green color after staining. | |
4.4. Proposed process configuration
The goal of this study was to identify green solvents for the extraction and fractionation of wet algae biomass. Based on the results of this study, we propose a biorefinery process as shown in Fig. 10. After algae cultivation and harvest, a paste with a water content of around 80 wt% remains. In a first extraction step using a 2-butanol/water mixture, the major proportion of pigments and polar lipids are extracted. The residual biomass is rich in lipid droplets containing neutral lipids and carotenoids and is separated by filtration from the liquid phase. The main carotenoid in lipid droplets is fucoxanthin.64 The monophasic filtrate is transferred to a decanter where phase separation is induced by water addition. According to our predictions, polar lipids and pigments are transferred to the organic phase. The polar lipid fraction contains high amounts of EPA.65 The most abundant pigment in the organic phase would be chlorophyll a.71 In this way, the value-added products EPA, fucoxanthin and laminarin are separated into three different product streams and are available for further purification steps. Another extraction step can be applied after full cell disruption of the residual biomass, in order to separate the neutral lipids and remaining carotenoids from the algae cells. Also here a computational screening approach would facilitate solvent selection and reduce testing in the lab.
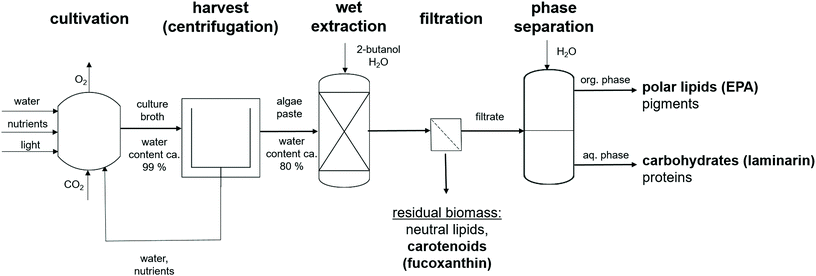 |
| Fig. 10 Proposed biorefinery process concept with 2-butanol extraction as central unit. Wet biomass of P. tricornutum with ca. 80 wt% moisture content is extracted with 2-butanol. According to our predictions, the biomass can be split up into three product streams, containing the value-added molecules fucoxanthin, laminarin and EPA. | |
5. Conclusions
In this study, more than 8000 molecules were screened for the fractionation of wet biomass from P. tricornutum. A new screening approach was presented in which representative molecules of the protein, carbohydrate, lipid and pigment fraction and screened molecules for structural constraints, pure compound information, such as MP and BP, EHS criteria, and thermodynamic behaviour as predicted by COSMO-RS. A new extraction strategy for wet biomass was proposed using solvents being partially miscible with water. A list of solvent molecules suitable for wet extraction was identified in silico so that experimental effort is only invested for the most promising candidates. Experiments revealed that 2-butanol without cell disruption attained 74% of all lipophilic compounds compared to extraction with chloroform/methanol and full cell disintegration. We demonstrated that solvents being practically immiscible with water, such as hexane, performed worse than all partially miscible solvents identified in the computational screening. Computational predictions show that due to the high water solubility of 2-butanol, the solubility of neutral lipids in the solvent/water-mixture is low which enables fractionation of the lipophilic compounds. After extraction using partially miscible solvents, neutral lipids remain in the biomass as could be shown by lipid staining. According to the screening results, water is the most favourable solvent for carbohydrate and protein extraction. Furthermore, no solvent was able to separate the two hydrophilic fractions. Hence, both fractions are solubilised in water. Based on these results, we propose a suitable process configuration for a the wet extraction. This concept can be easily extended by a subsequent neutral lipid extraction step in order to extract all valuable compounds from the algae. This study is a major step towards a biorefinery concept for P. tricornutum, tackling the problems of wet extraction and biomass fractionation at the same time. In the next studies, the amount of the target molcules in the product streams should be quantified and separation strategies for their purification should be developed. Furthermore, the screening approach can be applied to other microalgal species.
6. Experimental methods
6.1. Extraction
6.1.1. Determination of total lipohilic compounds.
All lipophilic compounds were quantified gravimetrically after a slightly modified procedure as proposed by Ryckebosch et al.115 In brief, wet biomass with a water content of 85.56 ± 0.69%, corresponding to 100 mg dry weight, was weighed into tubes and disrupted with an ultrasound probe (UIS250V, Hielscher Ultrasound Technology). 3 ml of methanol were added to the biomass, vortexed and incubated for 5 min. Subsequently, 3 ml of chloroform was added, vortexed, and incubated for 5 min. The cells were extracted until the biomass was decolored. 2 ml of water was added for phase separation, and the mixture was subsequently centrifuged. The chloroform-layer was given over a Whatman No. 1 filter filled with anhydrous sodium sulfate, and filtered to a dried, pre-weighed tube. The tube was placed in a heating block (ThermoMixer C, Eppendorf) over night at 60 °C to evaporate the solvent. The tubes containing the lipid extracts were dried and weighed. The extraction yield was determined as follows: | 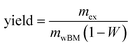 | (3) |
where mex is the determined mass of the extract, mwBM is the mass of the wet biomass, and W denotes the water content of the wet biomass. The analysis was performed in triplicate and the three results were averaged.
6.1.2. Extraction with partially miscible solvents, pure hexane, and hexane/ethanol mixture.
3500 mg of wet, undisrupted microalgal paste with a water content of 85.56 ± 0.69% were subjected to a 250 ml glass bottle, and extracted with 125 ml of the respective solvent candidate. The suspension was agitated by magnetic stirring. For the partially miscible solvents, the amount of water necessary to sature the solvent was readily available from LLE data in literature, see Table 4. From that amount, water contained as moisture in the biomass was substracted. The resulting volume of water was added in three steps of equal volume. Before the first water addition step, as well as after each water addition, the biomass was incubated 5 min with pure solvent, leading to a total incubation time of 20 min. All mixtures with the partially miscible solvents remained monophasic. For extractions with pure hexane and the extraction with a hexane/ethanol mixture (3
:
1, v/v), no water was added during extraction. The resulting suspension was vacuum filtered through a Buchner funnel equipped with a Whatman glass microfiber filter into 250 ml suction flasks. The content of the suction flask was subjected to a separatory funnel. The suction flask was rinsed with pure solvent which was subjected to the separatory funnel as well. Phase separation was achieved by adding 25 ml water. The organic phase was transferred to dried, pre-weighed round bottom flasks. Solvent and water were subsequently removed by rotary evaporation (Rotavapor R-205, Büchi). The extract containing flasks were dried in an oven and weighed again. The yield was calculated according to eqn (3). The analysis was performed in triplicate and the three results were averaged. To further examine the extracted compounds, FTIR spectra were recorded. Details about the cultivation and harvest procedure, FTIR spectroscopy, as well as a list containing all solvents, their supplier and the purity are given in the ESI.†
6.2. Neutral lipid staining
Neutral lipids contained in the biomass after extraction with 2-butanol were stained using BODIPY505/515 according to a method of Wu et al.116 In our study, BODIPY505/515 was dissolved in DMSO, pipetted to the residual biomass and incubated for 15 min in the dark at room temperature. Subsequently the cells were washed three times with 0.9% aqueous NaCl solution.
Conflicts of interest
There are no conflicts to declare.
Acknowledgements
The authors would like to thank Anne Christin Reichelt for assistance in microscopy and cultivation and Christine Meitzner for her help in the manual selection of solvent candidates. We also acknowledge funding by the Christiane Nüsslein-Volhard foundation. Open Access funding was provided by the Max Planck Society.
References
-
A. Fernandez Pales and L. Peter, The Future of Petrochemicals, 2018 Search PubMed.
-
A. C. Guedes, H. M. Amaro, I. Sousa-Pinto and F. X. Malcata, Biofuels from Algae, Elsevier, Burlington, USA, 1st edn, 2014, pp. 205–233 Search PubMed.
- E. M. Trentacoste, A. M. Martinez and T. Zenk, Photosynth. Res., 2015, 123, 305–315 CrossRef CAS PubMed.
- D. Prat, J. Hayler and A. Wells, Green Chem., 2014, 16, 4546–4551 RSC.
- L. Lardon, A. Hélias, B. Sialve, J.-P. Steyer and O. Bernard, Environ. Sci. Technol., 2009, 43, 6475–6481 CrossRef CAS PubMed.
- F. Ketzer, J. Skarka and C. Rösch, BioEnergy Res., 2018, 11, 95–105 CrossRef CAS.
-
Biorefineries, ed. K. Wagemann and N. Tippkötter, Springer International Publishing, Cham, 2019, vol. 166 Search PubMed.
- C. G. Khoo, Y. K. Dasan, M. K. Lam and K. T. Lee, Bioresour. Technol., 2019, 292, 121964 CrossRef CAS PubMed.
- R. Chandra, H. M. Iqbal, G. Vishal, H.-S. Lee and S. Nagra, Bioresour. Technol., 2019, 278, 346–359 CrossRef CAS PubMed.
- X. Bai, H. Song, M. Lavoie, K. Zhu, Y. Su, H. Ye, S. Chen, Z. Fu and H. Qian, Sci. Rep., 2016, 6, 25494 CrossRef CAS.
- I. Megía-Hervás, A. Sánchez-Bayo, L. F. Bautista, V. Morales, F. G. Witt-Sousa, M. Segura-Fornieles and G. Vicente, Processes, 2020, 8, 1072 CrossRef.
- M. R. Brown, J. Exp. Mar. Biol. Ecol., 1991, 145, 79–99 CrossRef CAS.
- M. Branco-Vieira, S. San Martin, C. Agurto, M. A. Freitas, A. A. Martins, T. M. Mata and N. S. Caetano, Fuel, 2020, 268, 117357 CrossRef CAS.
- B. Tesson, C. Gaillard and V. Martin-Jézéquel, Bot. Mar., 2009, 52, 104–116 Search PubMed.
- G. Francius, B. Tesson, E. Dague, V. Martin-Jézéquel and Y. F. Dufrêne, Environ. Microbiol., 2008, 10, 1344–1356 CrossRef CAS PubMed.
- J. Penhaul Smith, A. Hughes, L. McEvoy and J. Day, Bioresour. Technol. Rep., 2020, 9, 100321 CrossRef.
- X.-W. Wang, L. Huang, P.-Y. Ji, C.-P. Chen, X.-S. Li, Y.-H. Gao and J.-R. Liang, Bioresour. Technol., 2019, 289, 121681 CrossRef CAS PubMed.
- M. M. A. Nur, W. Muizelaar, P. Boelen and A. G. J. Buma, J. Appl. Phycol., 2019, 31, 111–122 CrossRef CAS.
- U. Neumann, F. Derwenskus, V. Flaiz Flister, U. Schmid-Staiger, T. Hirth and S. Bischoff, Antioxidants, 2019, 8, 183 CrossRef CAS.
- S. M. Kim, Y.-J. Jung, O.-N. Kwon, K. H. Cha, B.-H. Um, D. Chung and C.-H. Pan, Appl. Biochem. Biotechnol., 2012, 166, 1843–1855 CrossRef CAS PubMed.
- W. Zhang, F. Wang, B. Gao, L. Huang and C. Zhang, Algal Res., 2018, 32, 193–200 CrossRef.
- M. A. Caballero, D. Jallet, L. Shi, C. Rithner, Y. Zhang and G. Peers, Algal Res., 2016, 20, 180–188 CrossRef.
- B. Morales-Lange, J. Bethke, P. Schmitt and L. Mercado, Aquacult. Res., 2015, 46, 2707–2715 CrossRef CAS.
- R. A. Dalmo, B. Martinsen, T. E. Horsberg, A. Ramstad, C. Syvertsen, R. Seljelid and K. Ingebrigtsen, J. Fish Dis., 1998, 21, 459–462 CrossRef.
- M. Sakai, Aquaculture, 1999, 172, 63–92 CrossRef CAS.
- M. I. Kusaikin, S. P. Ermakova, N. M. Shevchenko, V. V. Isakov, A. G. Gorshkov, A. L. Vereshchagin, M. A. Grachev and T. N. Zvyagintseva, Chem. Nat. Compd., 2010, 46, 1–4 CrossRef CAS.
- M. Arena, D. Auteri, S. Barmaz, G. Bellisai, A. Brancato, D. Brocca, L. Bura, H. Byers, A. Chiusolo, D. Court Marques, F. Crivellente, C. De Lentdecker, M. De Maglie, M. Egsmose, Z. Erdos, G. Fait, L. Ferreira, M. Goumenou, L. Greco, A. Ippolito, F. Istace, S. Jarrah, D. Kardassi, R. Leuschner, C. Lythgo, J. O. Magrans, P. Medina, I. Miron, T. Molnar, A. Nougadere, L. Padovani, J. M. Parra Morte, R. Pedersen, H. Reich, A. Sacchi, M. Santos, R. Serafimova, R. Sharp, A. Stanek, F. Streissl, J. Sturma, C. Szentes, J. Tarazona, A. Terron, A. Theobald, B. Vagenende, A. Verani and L. Villamar-Bouza, EFSA J., 2017, 15, 16 Search PubMed.
- F. Russell and C. Bürgin-Maunder, Mar. Drugs, 2012, 10, 2535–2559 CrossRef CAS PubMed.
- X.-J. Ji, L.-J. Ren and H. Huang, Front. Bioeng. Biotechnol., 2015, 3, 158 Search PubMed.
- S. Bleakley and M. Hayes, Foods, 2017, 6, 33 CrossRef PubMed.
- S. Sarkar, M. S. Manna, T. K. Bhowmick and K. Gayen, Crit. Rev. Biotechnol., 2020, 40, 590–607 CrossRef CAS PubMed.
- C. A. Suarez Ruiz, J. Kwaijtaal, O. C. Peinado, C. van den Berg, R. H. Wijffels and M. H. Eppink, ACS Sustainable Chem. Eng., 2020, 8, 2441–2452 CrossRef CAS.
- J. H. P. M. Santos, J. P. Trigo, É. Maricato, C. Nunes, M. A. Coimbra and S. P. M. Ventura, ACS Sustainable Chem. Eng., 2018, 6, 14042–14053 CrossRef CAS.
- W. Zhao, M. Duan, X. Zhang and T. Tan, Renewable Energy, 2018, 118, 701–708 CrossRef CAS.
- A. d. P. Sánchez-Camargo, N. Pleite, J. A. Mendiola, A. Cifuentes, M. Herrero, B. Gilbert-López and E. Ibáñez, Electrophoresis, 2018, 39, 1875–1883 CrossRef PubMed.
- R. S. Alavijeh, K. Karimi, R. H. Wijffels, C. van den Berg and M. Eppink, Bioresour. Technol., 2020, 309, 123321 CrossRef CAS PubMed.
- R. Zhang, N. Lebovka, L. Marchal, E. Vorobiev and N. Grimi, Innovative Food Sci. Emerging Technol., 2020, 62, 102367 CrossRef CAS.
- E. Angles, P. Jaouen, J. Pruvost and L. Marchal, Algal Res., 2017, 21, 27–34 CrossRef.
- S. S. de Jesus, G. F. Ferreira, L. S. Moreira, M. R. Wolf Maciel and R. Maciel Filho, Renewable Energy, 2019, 143, 130–141 CrossRef CAS.
- V. C. A. Orr, N. V. Plechkova, K. R. Seddon and L. Rehmann, ACS Sustainable Chem. Eng., 2016, 4, 591–600 CrossRef CAS.
- F. Eckert and A. Klamt, AIChE J., 2002, 48, 369–385 CrossRef CAS.
- T. Zhou, K. McBride, S. Linke, Z. Song and K. Sundmacher, Curr. Opin. Chem. Eng., 2020, 27, 35–44 CrossRef.
- A. Klamt, J. Phys. Chem., 1995, 99, 2224–2235 CrossRef CAS.
- A. Klamt, V. Jonas, T. Bürger and J. C. W. Lohrenz, J. Phys. Chem. A, 1998, 102, 5074–5085 CrossRef CAS.
- A. Klamt and F. Eckert, Fluid Phase Equilib., 2000, 172, 43–72 CrossRef CAS.
- O. Spuhl, W. Arlt and A. del Río Hernández, Chem. Ing. Tech., 2003, 75, 58–62 CrossRef CAS.
- R. Franke, J. Krissmann and R. Janowsky, Chem. Ing. Tech., 2002, 5 Search PubMed.
- A. Paiva, R. Craveiro, I. Aroso, M. Martins, R. L. Reis and A. R. C. Duarte, ACS Sustainable Chem. Eng., 2014, 2, 1063–1071 CrossRef CAS.
- E. L. Smith, A. P. Abbott and K. S. Ryder, Chem. Rev., 2014, 114, 11060–11082 CrossRef CAS PubMed.
- F. M. Perna, P. Vitale and V. Capriati, Curr. Opin. Green Sustain. Chem., 2020, 21, 27–33 CrossRef.
- Y. Liu, J. B. Friesen, J. B. McAlpine, D. C. Lankin, S.-N. Chen and G. F. Pauli, J. Nat. Prod., 2018, 81, 679–690 CrossRef CAS PubMed.
- P. G. Jessop, Green Chem., 2011, 13, 1391–1398 RSC.
- J. Kahlen, K. Masuch and K. Leonhard, Green Chem., 2010, 12, 2172 RSC.
- Z. Wang, S. Bhattacharyya and D. G. Vlachos, Green Chem., 2020, 22, 8699–8712 RSC.
- C. Breil, A. Meullemiestre, M. Vian and F. Chemat, Molecules, 2016, 21, 196 CrossRef PubMed.
- A. C. Spieß, W. Eberhard, M. Peters, M. F. Eckstein, L. Greiner and J. Büchs, Chem. Eng. Process., 2008, 47, 1034–1041 CrossRef.
- Y. Chu and X. He, ACS Omega, 2019, 4, 2337–2343 CrossRef CAS PubMed.
- Z. Shen and R. C. Van Lehn, Ind. Eng. Chem. Res., 2020, 59, 7755–7764 CrossRef CAS.
- S. Rezaei Motlagh, R. Harun, D. Awang Biak, S. Hussain, W. Wan Ab Karim Ghani, R. Khezri, C. Wilfred and A. Elgharbawy, Molecules, 2019, 24, 713 CrossRef PubMed.
- S. Rezaei Motlagh, R. Harun, D. R. Awang Biak, S. A. Hussain, R. Omar and A. A. Elgharbawy, Mar. Drugs, 2020, 18, 108 CrossRef PubMed.
- S. Rezaei Motlagh, R. Harun, D. Radiah Awang Biak, S. A. Hussain, A. A. Elgharbawy, R. Khezri and C. D. Wilfred, Biomolecules, 2020, 10, 1149 CrossRef PubMed.
- S. Linke, K. McBride and K. Sundmacher, ACS Sustainable Chem. Eng., 2020, 10795–10811 CAS.
- X. Pi, S. Zhao, W. Wang, D. Liu, C. Xu, G. Han, T. Kuang, S.-F. Sui and J.-R. Shen, Science, 2019, 365, eaax4406 CrossRef CAS PubMed.
- J. Lupette, A. Jaussaud, K. Seddiki, C. Morabito, S. Brugière, H. Schaller, M. Kuntz, J.-L. Putaux, P.-H. Jouneau, F. Rébeillé, D. Falconet, Y. Couté, J. Jouhet, M. Tardif, J. Salvaing and E. Maréchal, Algal Res., 2019, 38, 101415 CrossRef.
- Y.-H. Yang, L. Du, M. Hosokawa, K. Miyashita, Y. Kokubun, H. Arai and H. Taroda, J. Oleo Sci., 2017, 66, 363–368 CrossRef CAS PubMed.
- A. I. Barros, A. L. Gonçalves, M. Simões and J. C. Pires, Renewable Sustainable Energy Rev., 2015, 41, 1489–1500 CrossRef.
- B. Yatipanthalawa, W. Li, D. R. Hill, Z. Trifunovic, M. Ashokkumar, P. J. Scales and G. J. Martin, J. Colloid Interface Sci., 2021, 589, 65–76 CrossRef CAS PubMed.
- F. Ghasemi Naghdi, L. M. González González, W. Chan and P. M. Schenk, Microb. Biotechnol., 2016, 9, 718–726 CrossRef CAS PubMed.
- C.-Z. Liu, S. Zheng, L. Xu, F. Wang and C. Guo, Appl. Energy, 2013, 102, 971–974 CrossRef CAS.
- J. E. Mann and J. Myers, J Phycol, 1968, 4, 349–355 CrossRef CAS PubMed.
- J. I. Carreto and J. A. Catoggio, Mar. Biol., 1976, 36, 105–112 CrossRef CAS.
- B. Gügi, T. Le Costaouec, C. Burel, P. Lerouge, W. Helbert and M. Bardor, Mar. Drugs, 2015, 13, 5993–6018 CrossRef PubMed.
- Y. Chu, X. Zhang, M. Hillestad and X. He, Fluid Phase Equilib., 2018, 475, 25–36 CrossRef CAS.
- A. Beattie, E. L. Hirst and E. Percival, Biochem. J., 1961, 79, 531–537 CrossRef CAS PubMed.
- S. Guzmán, A. Gato, M. Lamela, M. Freire-Garabal and J. M. Calleja, Phytother. Res., 2003, 17, 665–670 CrossRef PubMed.
- C. W. Ford and E. Percival, J. Chem. Soc., 1965, 7042–7046 RSC.
- C. W. Ford and E. Percival, J. Chem. Soc., 1965, 7035–7041 RSC.
- T. Le Costaouëc, C. Unamunzaga, L. Mantecon and W. Helbert, Algal Res., 2017, 26, 172–179 CrossRef.
- L. Grossmann, J. Hinrichs and J. Weiss, LWT–Food Sci. Technol., 2019, 105, 408–416 CrossRef CAS.
- M. P. Andersson, J. H. Jensen and S. L. S. Stipp, PeerJ, 2013, 1, e198 CrossRef PubMed.
- H. T. Do, Y. Z. Chua, J. Habicht, M. Klinksiek, S. Volpert, M. Hallermann, M. Thome, D. Pabsch, D. Zaitsau, C. Schick and C. Held, Ind. Eng. Chem. Res., 2021, 60, 4693–4704 CrossRef CAS.
-
Molecular Cell Biology (Online
Version), ed. H. Lodish, Freeman, New York, NY, 4th edn, 2002, ch. 3.2 Search PubMed.
-
CRC Handbook of Chemistry and Physics (Online Version), ed. J. R. Rumble, CRC Press/Taylor & Francis, Boca Raton, FL, 102nd edn, 2021, ch. 7 Search PubMed.
- L. Moity, M. Durand, A. Benazzouz, C. Pierlot, V. Molinier and J.-M. Aubry, Green Chem., 2012, 14, 1132–1145 RSC.
- J. E. Camp, ChemSusChem, 2018, 11, 3048–3055 CrossRef CAS PubMed.
- Q. Zhang, K. De Oliveira Vigier, S. Royer and F. Jérôme, Chem. Soc. Rev., 2012, 41, 7108 RSC.
-
R. Kohli, Developments in Surface Contamination and Cleaning: Applications of Cleaning Techniques, Elsevier, 2019, ch. 16, pp. 619–680 Search PubMed.
- E. Słupek, P. Makoś and J. Gebicki, Energies, 2020, 13, 3379 CrossRef.
- K. Jeong, M. Yang, Y. Jin, E. Kim, J. Ko and J. Lee, Molecules, 2017, 22, 2006 CrossRef PubMed.
- K. Radošević, N. Ćurko, V. Gaurina Srček, M. Cvjetko Bubalo, M. Tomašević, K. Kovačević Ganić and I. Radojčić Redovniković, LWT–Food Sci. Technol., 2016, 73, 45–51 CrossRef.
- T. Křížek, M. Bursová, R. Horsley, M. Kuchař, P. Tůma, R. Čabala and T. Hložek, J. Cleaner Prod., 2018, 193, 391–396 CrossRef.
- M. W. Nam, J. Zhao, M. S. Lee, J. H. Jeong and J. Lee, Green Chem., 2015, 17, 1718–1727 RSC.
- C. Florindo, L. C. Branco and I. M. Marrucho, ChemSusChem, 2019, 12, 1549–1559 CrossRef CAS PubMed.
- W. Tang, Y. Dai and K. H. Row, Anal. Bioanal. Chem., 2018, 410, 7325–7336 CrossRef CAS PubMed.
- H. Vanda, Y. Dai, E. G. Wilson, R. Verpoorte and Y. H. Choi, C. R. Chim., 2018, 21, 628–638 CrossRef CAS.
-
Iolitec, Iolitec Catalogue, https://iolitec.de/products/ionic_liquids/catalogue (accessed April 20, 2020) Search PubMed.
-
Sigma Aldrich, ChemFiles Vol. 5 No. 6, 2005 Search PubMed.
-
E. Benefenati, A. Manganaro and G. Gini, CEUR Workshop Proc., 2013, pp. 21–28.
- Z. Song, X. Hu, H. Wu, M. Mei, S. Linke, T. Zhou, Z. Qi and K. Sundmacher, ACS Sustainable Chem. Eng., 2020, 8, 8741–8751 CrossRef CAS.
- M. Hayyan, M. A. Hashim, A. Hayyan, M. A. Al-Saadi, I. M. AlNashef, M. E. Mirghani and O. K. Saheed, Chemosphere, 2013, 90, 2193–2195 CrossRef CAS PubMed.
- L. P. N. Rebelo, J. N. Canongia Lopes, J. M. S. S. Esperança and E. Filipe, J. Phys. Chem. B, 2005, 109, 6040–6043 CrossRef CAS PubMed.
- Y. Dai, J. van Spronsen, G.-J. Witkamp, R. Verpoorte and Y. H. Choi, J. Nat. Prod., 2013, 76, 2162–2173 CrossRef CAS PubMed.
- PubChem, https://pubchem.ncbi.nlm.nih.gov/, (accessed July 7, 2021).
- NIST Webbook, https://webbook.nist.gov/chemistry/, (accessed July 7, 2021).
- E. Lladosa, J. B. Montón, J. de la Torre and N. F. Martínez, J. Chem. Eng. Data, 2011, 56, 1755–1761 CrossRef CAS.
- M. Letcher, J. D. Sewry and D. Naran, Fluid Phase Equilib., 1989, 187–193 CrossRef.
- Y. Zhang, X. Guo, J. Xu, Y. Wu and M. Lu, J. Chem. Eng. Data, 2018, 63, 2775–2782 CrossRef CAS.
- S. Çehreli, D. Özmen and U. Dramur, Fluid Phase Equilib., 2006, 239, 156–160 CrossRef.
- M. Trofimova, A. Sadaev, A. Samarov, M. Toikka and A. Toikka, Fluid Phase Equilib., 2019, 485, 111–119 CrossRef CAS.
- R. M. Stephenson, J. Chem. Eng. Data, 1992, 37, 80–95 CrossRef CAS.
- C.-T. Hsieh, W.-Y. Ji, H.-M. Lin and M.-J. Lee, Fluid Phase Equilib., 2008, 271, 69–75 CrossRef CAS.
- K. J. Fraser and D. R. MacFarlane, Aust. J. Chem., 2009, 62, 309 CrossRef CAS.
- J. Zhou, H. Sui, Z. Jia, Z. Yang, L. He and X. Li, RSC Adv., 2018, 8, 32832–32864 RSC.
-
CRC Handbook of Chemistry and Physics (Online Version), ed. J. R. Rumble, CRC Press/Taylor & Francis, Boca Raton, FL, 102nd edn, 2021, ch. 6 Search PubMed.
- E. Ryckebosch, K. Muylaert and I. Foubert, J. Am. Oil Chem. Soc., 2012, 89, 189–198 CrossRef CAS.
- S. Wu, B. Zhang, A. Huang, L. Huan, L. He, A. Lin, J. Niu and G. Wang, J. Appl. Phycol., 2014, 26, 1659–1668 CrossRef CAS.
Footnote |
† Electronic supplementary information (ESI) available. See DOI: 10.1039/d1gc03471e |
|
This journal is © The Royal Society of Chemistry 2021 |
Click here to see how this site uses Cookies. View our privacy policy here.