DOI:
10.1039/D0FO02938F
(Paper)
Food Funct., 2021,
12, 4152-4164
A multi-omics approach for understanding the effects of moderate wine consumption on human intestinal health†
Received
7th November 2020
, Accepted 31st March 2021
First published on 16th April 2021
Abstract
The human gut is a highly diverse microbial ecosystem. Although showing a well-defined core of dominant taxa, an interindividual variability exists in microbiome arrangement patterns, and the presence and proportion of specific species, determining individual metabolic features-metabotypes-which govern the health effects of dietary interventions (i.e. polyphenol consumption). Starting with a 19-volunteer human intervention study, divided into low, medium, and high wine-polyphenol-metabolizers, we detected interindividual discrepancies on the effect of wine consumption in gut bacterial alpha-diversity, but a significant homogenization of beta-diversity among moderate wine consumers, independently of their metabotype. In addition, the abundance of key health-related taxa such as Akkermansia sp. increased after moderate wine intake in the group of high polyphenol-metabolizers. Regarding the metabolic activity, significant (p < 0.05) positive correlations in the production of SCFAs were observed after wine intake. Finally, we were able to correlate the microbiome and the metabolome of the three metabotypes, and to identify some metabolites-biomarker species, highlighting the genera Phascolarctobacterium, Pelotomaculum and Prevotella, as positively correlated with polyphenol concentration, and Prevotella, Zymophilus and Eubacterium as positively correlated with SCFAs concentration in faeces. Our results contribute to the evidence of the need of including the microbiome variable in personalized nutrition programs, as different metabotyes respond differently to dietary interventions.
1. Introduction
The human gut is a complex microbial ecosystem, where both environmental and dietary factors are the main drivers of its taxonomic and functional diversity.1–3 In a large survey, Zhang and collaborators reported that, although 9 bacterial genera co-occur as predominant taxa in all individuals (accounting for almost a 50% of the total bacterial biomass), subgenus taxonomic levels (species and Operational Taxonomic Units-OTUs) make it possible to discriminate the subjects according to their geographical origin and lifestyles.1
Thus, there is a great interest in understanding how diet can be used to modulate the composition and metabolic activity (functional adaption) of the human gut microbiota, which in turn can impact host health. Several comparative studies suggested that high fiber content diets may increase the taxonomic and functional diversity of gut microbiota, and may favour a significant enrichment in the abundance of certain microbial taxa (e.g. Prevotella and Xylanibacter species),3–6 altogether contributing to significant changes in the faecal and urinary microbial-derived metabolite profiles.7
Even being aware of the impact of diet on metabolic functions of the intestinal microbiota, it is important to note that the functional genetic profile expressed by the bacterial community is quite stable and similar in healthy individuals, thereby ensuring those essential functions for the survival of the host.8,9 Therefore, microbial species present in smaller quantities, but developing specific/determinant functions, could be the key to understanding the bioavailability and individual response to consumption of food bioactive compounds (i.e. polyphenols).
In recent decades, foods rich in polyphenols have been the object of study in gut health research. In particular, the moderate consumption of wine could have a beneficial effect on intestinal health, both by its direct impact on the microbiota, and the production of bioavailable metabolites.10,11 A higher microbial diversity has been associated to moderate regular consumption of red wine led,12 and the derived increased content in polyphenols may increase the abundance of health-related species such as Faecalibacterium prausnitzii and Akkermansia muciniphila.5,13,14
The phenolic profile in different physiological fluids (urine, plasma, faeces) after wine or phenolic-rich foods consumption is mainly composed of microbial-derived phenolic acids and other related metabolites.15,16 In the case of wine polyphenols, a tentative distribution of the individuals into low, medium, and high metabolizers by their capacity to metabolize wine polyphenols has been established.15,17 However, little is known about the bacterial diversity patterns, or specific species, associated with these metabotypes; but some differences in minority microbial groups related to the metabotypes were found after moderate wine consumption.12
Therefore, and considering that taxonomic and functional facets of microbiome are interdependent, an integrative approach should be considered to exploring taxa-function relationships in microbiome data. According with this, the main aim of this study was to associate previously-defined metabotypes with specific microbiological patterns of the intestinal microbiota and its response to a moderate wine intake. Thus, an integrative data analysis approach has been performed in this study, integrating metataxonomic and metabolomics information.
2. Materials and methods
2.1. Faecal samples collection and processing
The samples used in this study come from a previous randomized and controlled intervention study with a red wine in healthy volunteers.15 Since both, the previous work and this study claimed the same overall aim of evaluating the effects of moderate wine consumption at gut microbiological and metabolic level, the criteria for sample size calculation in this study was supported by the previous one. Additionally, we contemplated the results from the previous study15 that tentatively distributed individuals into low, medium, and high metabolizers (metabotypes) by their capacity to metabolize wine polyphenols. Therefore, faecal samples from 19 healthy volunteers (4 volunteers previously classified as high wine-polyphenol-metabolizers; 6 as medium metabolizers; 4 as low metabolizers; and 5 control volunteers) were used in this study. The participants (10 women and 9 men, aged on the 25–57 years-old range, age mean: 42 years) were not suffering from any disease or intestinal disorder, and were not receiving antibiotics or any other medical treatment for at least 6 months before the start of the study or during the study (including the washout period). All the participants were fully informed about the study and gave written informed consent. The study was approved by the Ethics Committee from CSIC (Madrid, Spain). Briefly, volunteers followed an initial washout period of 2 weeks (baseline) during which they did not consume any wine or any other alcoholic beverage and followed a low-polyphenols diet. After this period, the intervention group (8 women and 6 men) consumed 250 mL of red wine per day (439.5 mg of equivalents of polyphenols per day) divided into two doses, during 4 weeks. During this latter period, participants also maintained the restrictions for any other alcoholic beverages and followed a low-polyphenols diet. The control group followed the same pattern, with the exception that they ingested no wine during this 4-week period. Each participant provided samples of faeces at two points: (a) after the washout period (before wine intake) and (b) at the end of the study (after wine intake). Faeces were immediately frozen and stored at −80 °C awaiting analysis.
Before analysis samples were thawed, weighed, diluted in sterile saline solution (1
:
10 w/v), and vortexed. Later on, samples were centrifuged at 10
000 rpm for 10 min at 4 °C in order to separate the pellet (microbial analysis) from supernatant (metabolic analysis).
2.2. DNA extraction, sequencing and data processing
This study is a microbial amplicon-based survey created with previously unpublished data. DNA extraction from faeces samples were performed using bead-beating based DNA extraction kits such as DNeasy Powerlyzer Powersoil Kit (Qiagen, Hilden, Germany). The V4 region of the 16S rRNA gene was amplified by PCR using the primers 515F (GTGYCAGCMGCCGCGGTAA) and 806R (GGACTACNVGGGTWTCTAAT). Libraries were prepared following the two-step PCR Illumina® protocol and these were subsequently sequenced on Illumina® MiSeq instrument (Illumina®, San Diego, CA, USA) using 2 × 300 paired-end on the 16S rRNA V4 gene region using custom primers.18
The raw fastq sequences (available at NCBI bioproject PRJNA667844) were analyzed using Vsearch using default parameters.19 Briefly, raw paired-end fastq sequences were merged, filtered by expected error 0.25, dereplicated, and sorted by size. We filtered out chimera sequences and clustered non-singleton sequences into 97% identity OTUs. Finally, the taxonomic assignment of the OTUs was performed against the SILVA database.
2.3. Metabolic activity assessments
The study of metabolic activity included faecal phenolic metabolites, immune markers, short-chain fatty acids (SCFAs) and medium-chain fatty acids (MCFAs) (see Table S1† for a detailed list of the metabolites analysed and the individual and summatory data). Phenolic metabolites were analysed by UPLC-ESI-MS/MS using internal standard calibration15 and immune compounds were determined by using the Bio-Plex Pro Human Isotyping assay and the Human Cytokine group I and II assay kits (Bio-Rad Laboratories, Hercules, CA, USA).20 SCFAs and MCFAs in these samples have been analysed for the first time in this work.
Short-chain fatty acids (SCFAs: acetic, propionic, and butyric acids) and medium-chain fatty acids (MCFs: pentanoic, hexanoic, octanoic and decanoic acids) in faeces were analysed by SPME-GCMS following the method described by Cueva et al.21 Two hundred and ninety microliters of faecal solutions (1
:
10 w/v, faeces/PBS 0.1 M) or calibration stock solutions were added with 10 μL of internal standard solution of 2-methylvaleric acid (1 g L−1) and 30 μL of a 0.9 N H2SO4 solution (pH 2). Then, 100 μL of the acidified sample were transferred to a 20 mL hermetically closed vial. The extraction procedure was automatically performed by using a CombiPAL system (CTC Analytics AG, Zwingen, Switzerland) with a 50/30 μm DVB/CAR/PDMS SPME fibre of 2 cm length (Supelco, Bellefonte, PA). The chromatographic separation was performed in a DB-FFAP capillary column (30 m × 0.25 mm i.d. × 0.25 μm film thickness) (J&W, Agilent). Compounds identification was carried out by comparison of retention times and mass spectra of the reference compounds with those reported in the mass spectrum library NIST 2.0. Quantitative data were obtained by calculating the peak area of each compound in relation to that of the internal standard (2-methylvaleric acid). Calibration curves of each compound were obtained by diluting the original stock standard solution (from 5 g L−1 to 0.005 g L−1 of all volatile fatty acids) in PBS 0.1 M. Analyses were performed in duplicate.
2.4. Statistical analysis
Shapiro–Wilk test and Leven test were used to test normal distribution of the data and homogeneity of variances, respectively. One-way ANOVA was conducted to compare metabolic data (phenolic metabolites, immune markers, SCFAs, and MCFAs) obtained from volunteers after the washout period (before wine intake). The least significant differences were calculated by Tukeýs test (p < 0.05). A paired-samples t-test was also conducted to assess differences in metabolic data before and after wine consumption. Additionally, the relationships between metabolic parameters were investigated using the Pearson correlation coefficient. The IBM SPSS Statistic 23 program was used for processing these data.
All the statistical analysis which requires DNA sequences data (alpha- and beta-diversity calculations; Canonical Correspondence Analysis (CCpnA) and direct OTUs-metabolite correlations) were calculated in the R environment using the Vegan package and drawn in ggplot.22,23
3. Results and discussion
Currently, there is increasing scientific evidence suggesting that the correct approach to evaluate the effect of diet on the intestinal microbiota and biological outcomes must be done from a microbiological and functional perspective. According with this, this work has focused on the one hand, on evaluating the effect of wine consumption on the composition and the metabolic activity of the gut microbiota independently, and on the other hand, on integrating jointly the information of both approaches to try to associate previously-defined wine-polyphenols metabotypes with specific microbiological patterns.15 Also, we have intended to go a step further by describing the existence of certain groups of intestinal bacteria that could function as indicators/determinants of intestinal health in relation to the appearance of certain metabolites. The main findings are explained and discussed in the following sections.
3.1. Taxonomic diversity and its evolution after moderate wine intake
A total of 5
437
927 good quality 16S rRNA sequences were obtained from human faecal samples analysis, resulting in 1454 OTUs detected and a taxonomic assignation of 21 different phyla (Fig. 1A).
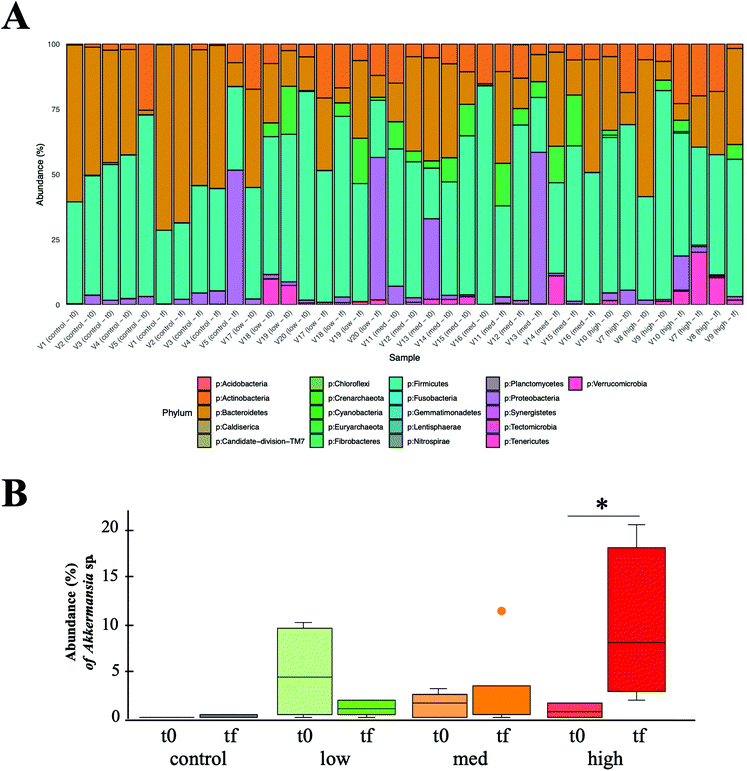 |
| Fig. 1 Descriptive analyses of bacterial diversity in the 19 volunteers (5 control volunteers; 4 low, 6 medium, and 4 high wine-polyphenol-metabolizers) at two sampling times (T0 and Tf; before and after wine intake, respectively). (A) General overview of the relative abundance of bacterial phyla detected in samples. (B) Relative abundance of Akkermansia spp. in the four groups, before and after moderate wine intake. * Means significant differences p < 0.05. | |
Considering as main phyla those whose mean abundance was higher than 1%, their average relative abundances in all volunteers and experimental conditions were as follows: Firmicutes 48.87% ± 16.05%, Bacteroidetes 28.57% ± 19.17%, Actinobacteria 8.72% ± 7.14%, Proteobacteria 7.16% ± 15.07%, Euryarchaeota 4.35% ± 5.99%, Verrucomicrobia 2.18% ± 4.25%. Except for samples V5(Tf), V20(Tf), V13(T0), V13(Tf), that showed an abnormally high abundance of Proteobacteria, there were no significant changes in the relative abundance of these phyla between groups (low, medium and high metabolizers, and control) and sampling times (before and after wine intake) (Kruskal–Wallis multiple comparison, p > 0.05), with the exception of Verrucomicrobia in the high metabolizers-Tf group compared with control samples (both T0 and Tf) (p < 0.05). A deeper exploration within the Verrucomicrobia phylum, allowed us to detect a significant increase in the abundance of Akkermansia (a mucin-degrading bacterium, related with positive effects in gut health25–27) after wine intake, but only in the high metabolizers group (p < 0.05) (Fig. 1B), suggesting a metabolism–microbiota interaction with a potential impact in gut health. Similar results of increasing A. muciniphila were reported by other authors, using black tea and red wine and a cranberry extract as sources of polyphenols.13,14 Finally, the Bacteroidetes/Firmicutes ratio was calculated as a marker of intestinal health,24 but no differences between groups or sampling times were detected (data not shown).
Fig. 2A shows no significant differences in terms of alpha-diversity between metabotypes (high, medium and low-wine-polyphenol metabolizers); and that there is no a clear effect of a moderate wine intake on it, but a great interindividual difference was observed (Fig. S1†). These results agree with the recent findings of Johnson et al. showing that similar foods and diets have different effects on different people's microbiomes, and that it is strongly dependent on their microbiome stability.28
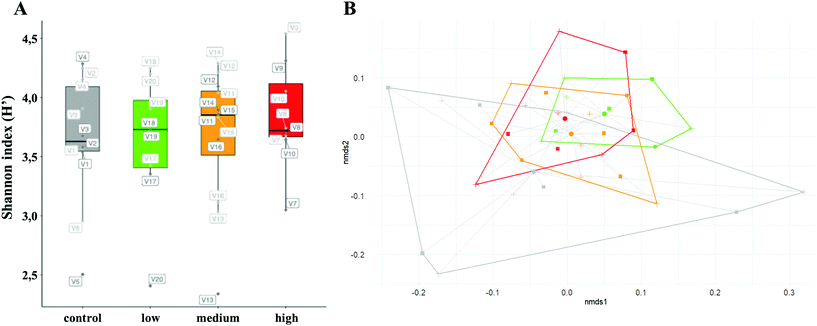 |
| Fig. 2 Bacterial diversity analysis. (A) Shannon (H′) diversity index as a measurement of alpha-diversity. Pairwise group comparisons confirmed no significant differences (Wilcoxon test) between groups and between sampling times (before and after wine consumption) at an intra-group level. (B) beta-diversity (nMDS) of bacterial communities and distance to the centroid (z parameter) of the experimental groups: control group (grey), and low (green), medium (yellow) and high (red) metabolizers, before-T0 (crosses) and after-Tf (squares) wine intake. | |
As regards to the beta-diversity, a great interspecific variability without clear patterns between groups or sampling times was observed (Fig. 2B and Fig. S2†). However, it is remarkable the greater dispersion (distance to centroid) of the control group compared with those experimental groups consuming wines (Fig. S3;† PERMANOVA, p = 0.002). These findings suggest that moderate wine consumption tends to homogenize the gut bacterial diversity between individuals; however, understanding the reasons under these interindividual stochastic variations are, nowadays, among the main challenges and bottlenecks in personalized nutrition.28 Thus, applying new conceptual frameworks, based in community- and functional-ecology concepts, is needed for a holistic understanding of gut microbiome functioning and its response to environmental, dietary and health perturbations.29
3.2 Metabolic functionality
The bacterial metagenome contributes to the production of metabolites that modulate human health in many ways.30 In this sense, diet plays a key role in the bacterial metabolome, since it is the major source of precursors for healthy metabolite production, as it would be the case for fatty acids and phenolic compounds produced through colonic fermentation. In order to stablish the relation between different metabolic parameters after red wine consumption, data from phenolic metabolites and immune markers from previous studies15,20 were used together with the fatty acid data obtained for the first time in this study. It should be clarified that, although in the previous studies,15,20 the statistical analysis of the data referring to phenolic metabolites was carried out, this new study did not contemplate the same number of volunteers based on the specific objectives of the work, which was the search for associations between the metabolome of the 3 metabotypes and the microbiome, as well as identifying some metabolites-biomarker species. Therefore, new analysis was required.
Significant differences before and after wine intake were observed for benzoic acid and decanoic acid after one-way ANOVA test (data not shown). In this sense, a high presence of benzoic acid before the intervention study with the same wine has previously reported,15 which could be related with the fact that it is a final metabolite of different metabolic pathways.31 In the case of decanoic acid, a medium-chain fatty acid (MCFA), is considered as non-microbial origin acid, that previously has demonstrated to remain in a constant level during an intervention study with a red grape pomace extract.32 Therefore, it seemed quite likely that the origin of these metabolites was closely related to the usual diet of the volunteers.
A paired-samples t-test was also conducted to assess differences in metabolic data in faeces before and after wine consumption. No significant differences (p > 0.05) were found in the content of metabolites tested except for 3-hydroxyphenylacetic acid (p = 0.03), syringic acid (p = 0.013), 4-hydroxy-5-(3′-hydroxyphenyl)-valeric acid (p = 0.024), 4-hydroxy-5-(phenyl)-valeric acid (p = 0.009) and decanoic acid (p = 0.037). The phenolic metabolites results are in accordance with the data published previously by Muñoz-González et al. who reported an increase in their concentration in faeces after wine intake period.15 As expected, some of them such as 4-hydroxy-5-(3′-hydroxyphenyl)-valeric acid and 4-hydroxy-5-(phenyl)-valeric acid, are characteristic metabolites exclusively derived from microbial catabolism of flavan-3-ols, one of the main class of phenolic compounds present in red wines and have been proposed as urinary biomarkers associated to wine consumption.17,33 Regard decanoic acid (p = 0.037), this result joined to the previously commented for this metabolite reinforce the idea of the influence of usual diet of volunteers.
In addition, Pearsońs correlations were calculated between different metabolites. In this sense, the most remarkable observed changes are depicted in the Fig. 3. In particular, after wine intake (Fig. 3B) the concentration of microbial phenolic metabolite 4-hydroxy-5-(phenyl)-valeric acid increases considerably and was positively correlated with decanoic acid (r = 0.927; p = 0.000) following a metabotype-depending pattern. Although we cannot provide any physiological explanation to this fact, the results obtained suggest that decanoic acid from diet may be involved in some way with phenolic metabolism.
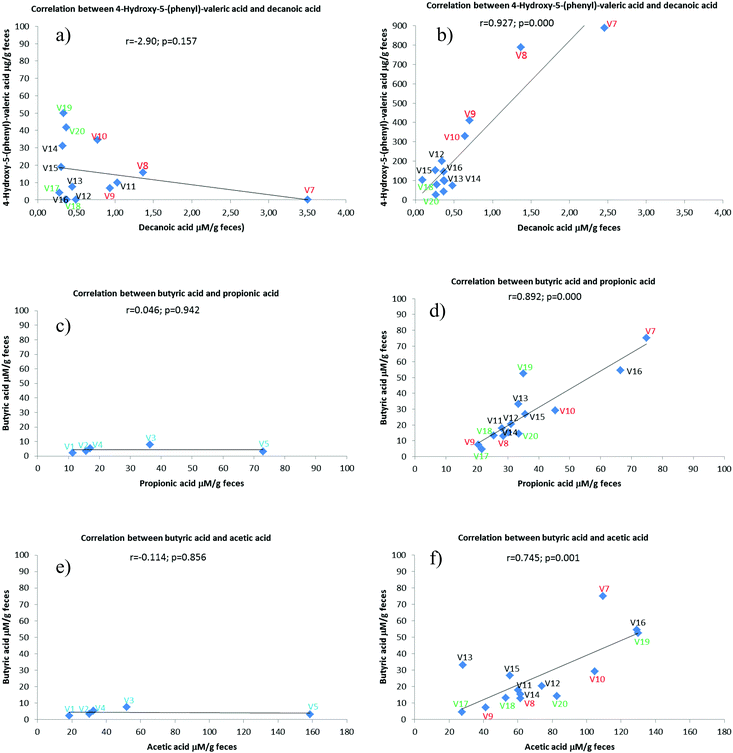 |
| Fig. 3 Pearsońs correlations among 4-hydroxy-5-(phenyl)-valeric acid and decanoic acid (n = 14) before (a) and after (b) the red wine administration. (c–f) show the Pearsońs correlation between butyric acid and propionic acid and butyric acid and acetic acid at the end of intervention period in the control volunteers (c and e) and after the red wine administration among different metabolizers groups (d and f; high, medium, and low wine-polyphenols-metabolizers are indicated as red, black and green colours, respectively). | |
Additionally, significant positive correlations between butyric and propionic acid (r = 0.892; p = 0.000) and butyric and acetic acid (r = 0.745; p = 0.001) were observed among different metabolizers groups (Fig. 3D and F) but not in control group at the end of intervention period. Among the factors that could affect this dynamic balance of SCFAs specially stand out the gut SCFA-producing bacteria, including the classification of the bacteria, their response to diet, the SCFAs metabolic pathways and the catalytic mechanisms of the main rate-limiting enzymes.34 Interestingly, the butyrate concentration in the three metabolizers groups was higher than in the control. Similar results were observed in studies carried out in dynamical gastrointestinal simulators after feeding with red wine or derivates.21,32
Overall, these results highlight that the main metabolic changes after wine consumption are microbiota-dependent, so an integrated microbiome–metabolome approach was required.
3.3. Microbiome–metabolome relationship
In recent years, numerous scientific studies have shown the bidirectional interaction between polyphenols and the intestinal microbiota: polyphenols are extensively metabolized giving rise to a large number of phenolic metabolites, which in turn they can exert a modulating activity of the microbiota35,36 and to confer a health benefit.25
As mentioned before, moderate wine consumption had a homogenising effect in the interindividual beta-diversity (Fig. 2B). In addition, although no differential beta-diversity pattern was detected between metabotypes, constringing microbial information with all total metabolites data (phenolic compounds, fatty acids, and immune markers) it is possible to cluster the bacterial profile of individuals, after wine intake but not before it, depending on their metabotype: from low to high polyphenol metabolizers, distributed in the first axis of the CCpnA analysis plot (Fig. 4B). This metabotype-driven clustering is also detectable when pooling together T0 and Tf samples (Fig. S4C†).
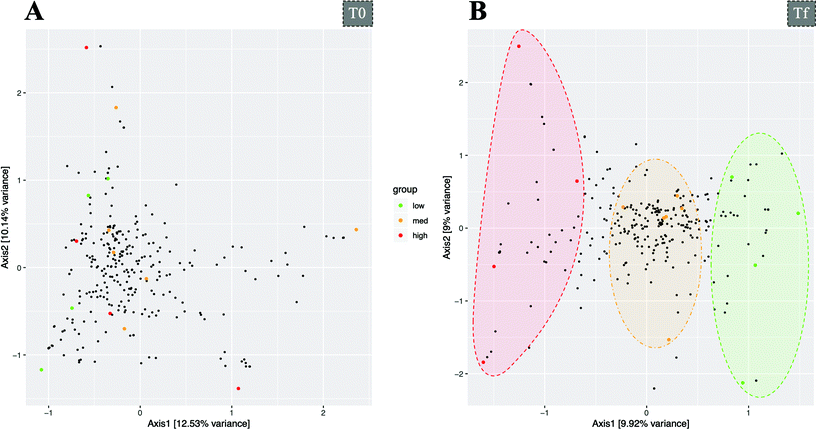 |
| Fig. 4 Canonical correspondence analysis (CCpnA) of bacterial diversity constrained by total metabolites data, before-T0 (A) and after-Tf (B) wine consumption. Samples are plotted as large dots and are coloured according to the metabolic groups they were classed as. Small black dots represent the key OTUs that drive the distribution of samples along the two primary axes (see ESI Fig. S4† for a detailed view of the taxonomic assignment of these OTUs). | |
This result demonstrates the different effect that a moderate wine consumption may have on the gut health status according to the categorization of individuals based on their capacity to metabolize polyphenols, the so-called metabotypes. In the same line, different metabotypes in the production of phenolic metabolites have been reported for other dietary sources of polyphenols.27,37–40 The importance of metabotypes relies on the effect that selective production of microbiota-derived metabolites may have on the health effects of certain foods or specific phenolic compounds.16 In this sense, it has been demonstrated that the individuaĺs urolithin metabotypes are associated with a different improve in cardiovascular biomarkers.41 Such observations were also noted with red wine whereby participants were clustered based on 4-hydroxyphenylacetate production.42
In this context, and taken into in consideration that minority and specific bacterial groups could be the responsible of defined metabotype profiles, another goal of this study was using the multi-omic tools to look for metabotype-related biomarkers species. Among them, we can highlight that Phascolarctobacterium sp., Flavobacterium sp., Pelotomaculum sp., Prevotella copri and Prolixibacter sp. appeared as positively correlated with the total faecal concentration of polyphenols (Fig. 5), but they also appeared as key species driving the metabotype-dependent clustering observed, after wine intake, in the microbiome ordination showed in Fig. 4B (Fig. S4B and S4C†). In line with this, an increase in Phascolarctobacterium, Barnesiella and Prevotellaceae groups was found in a recent observational study with red wine.43 Likewise, Phascolarctobacterium sp. has been found to be increased in patients successfully responding to weight loss in a volumetric diet-based intervention study in overweight adults, and it has also been reported as one of the main bacterial species responding to a vegetable-based diet.44 In the case of Flavobacterium sp., although its role in gut polyphenols metabolism is unknown, the ability of some strains of non-intestinal origin to produce the polyphenols quercetin, resveratrol and naringenin, it has been demonstrated.45 As regards to Pelotomaculum, it has been defined as a typical syntrophic propionate-oxidizing bacterium46 that, in complex microbial communities, is an important train for the stability of organic matter degradation processes.47 However, its specific role in human gut microbiota is still poorly understood, so here we provide some initial clues about its potential role in metabolizing diet polyphenols. In this sense, and given their metabolic capacities, a potential bacterial interaction networks between polyphenols and fatty acids production cannot be ruled out.48
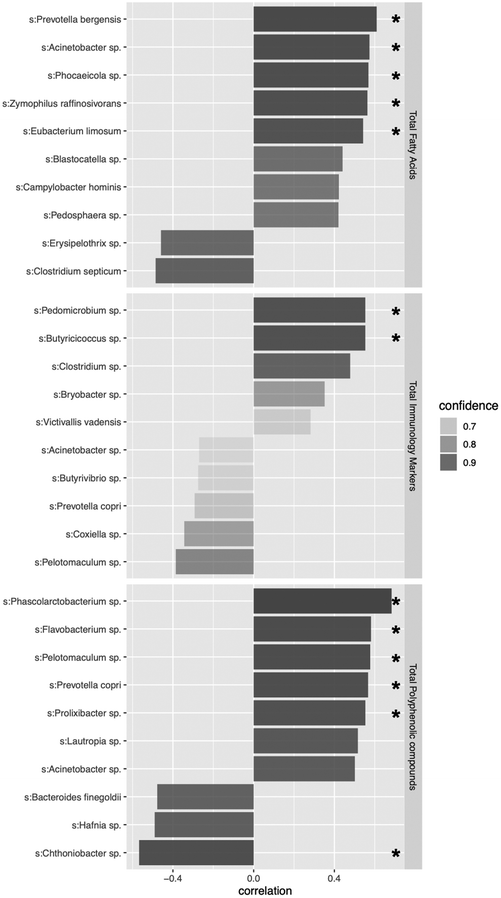 |
| Fig. 5 Top 10 bacteria species (s:) showing a positive or negative correlation with the total concentration of polyphenols, SCFAs, and total immunology markers (sum of immune markers). The top species from the CCA analysis had their correlation estimated. * Indicates significant Pearson's correlations (p < 0.05). | |
The human gut microbiota of Westernized populations is dominated by two bacterial phyla, the Firmicutes and the Bacteroidetes49 which they tend to mutually exclude one another.50 Gut microbiomes dominated by Prevotella (a dominant genus among Bacteroidetes) tend to occur in populations fed a fiber-rich diet,51 and it has also been associated with improvement in glucose metabolism.52 Here we show a positive correlation of the total polyphenol concentration and the relative abundance of P. copri in faeces (Fig. 5), one of the main species constituting the microbiota in healthy human gut,53 and which is considered as important biomarker for diet and lifestyle.54 An increase in P. copri was also observed after the administration of cocoa-enriched diet (a source of polyphenols) in a rat oral sensitization model.55 In line with this, de Filippis et al. noted that diet may select distinctive P. copri strains with distinguishable functions.51 This diversity may explain subject-specific responses to dietary interventions and variations in human health.
In the case of SCFAs, significant positive correlations with Prevotella bergensis, Acinetobacter sp., Phocaesicola sp., Zymophilus raffinosivorans and Eubacterium limosum were established. In this sense, it has been described that gut microbiomes with enriched concentrations of Prevotella, particularly in cases of dominance against versus Bacteroides species, tend to promotes a high production of total SCFAs from fiber-rich diets.56 This is also in agreement with our results where P. bergensis shows the highest positive correlation value with the total concentration of faecal SCFAs.
Regarding the significant correlation between Acinetobacter abundance and the total concentration of SCFAs, it has been already described the presence of a series of genes (originally described in E. coli) that are responsible for their contribution to the production of certain SCFAs-mainly butyrate-when present in gut microbiota.57 Similarly, two different Acinetobacter strains (Acinetobacter modestus CM11G and Acinetobacter radioresistens CM38.2) isolated from murine crypts, has demonstrated contain genes encoding for the presence of pili and secretion systems, which are considered as key factors in the protection of the intestinal crypts. In this sense, it is important to point out that some metabolites, such as SCFAs are important players to the maintenance of intestinal health through the microbiota-epithelium dialogue. This connection could be the responsible of positive correlation found with Acinetobacter sp. Furthermore, the potential to metabolize xenobiotics (i.e. polyphenols) has been found in the strains mentioned,58 although this was not the case in the results of our study. In contrast, it is well known that several strains of Acinetobacter baumanii species possess genes associated with bacterial virulence. These findings highlight the importance of species-to-strain level of identification in microbiome studies for a finer definition of the spectrum of roles that bacteria species can play in human gut.
In the same line, we should also highlight the abundance of Z. raffinosivorans (syn. Propionispira raffinosivorans) and E. limosum with a positive correlation with the total concentration of SCFAs, as they have been described as major propionic and/or butyric acid (in the Eubacterium genus) producers.59 Specifically, E. limosum, a gut commensal bacterium in some individuals, has been reported as a long life-associated biomarker, and its abundance is notably increased in the human gut of centenarians.60 In addition, it has been described the ability of this specie to metabolize hop polyphenols such as isoxanthohumol into 8-prenylnaringenin.61 Therefore, and although the results of our study only demonstrated a correlation with SCFAs, an interaction of this strain with the polyphenols metabolism would be also probable.
Butyricicocus species (i.e. B. pullicaecorum), have been also described as important SCFAs – mainly butyrate-producers in human gut.62 We did not find a significant correlation between Butyricicocus species and the total amount of SCFAs but, interestingly, we find a positive correlation of Butyricicocus sp. with the levels of immune markers detected in faeces. This contradicts the current association between Butyricicocus species, such as B. pullicaecorum, with a systematic reduction of immune marker levels, finding lower numbers of Butyricicoccus in patients with inflammatory bowel disease.63 Thus, once again, we detect the need for more precise microbiome profiling technologies (always reaching a species level definition) and we highlight the interest of understanding the potential range of roles that Butyricicocus species may be playing in human gut.
Finally, regarding the other taxa highlighted in Fig. 5, there is just a few to no scientific information on the role of Pedomicrobium in human gut, although here we found a significant positive correlation with the levels of immune markers detected in faeces, neither on the role of Flavobacterium and Prolixibacter, and Chthoniobacter species although here we found a significant positive and negative correlation, respectively, with the total concentration of polyphenols in faeces. Thus, further studies are needed for double-checking the relevance of these indicators and for a better comprehension on their role in human gut health.
Overall, these findings highlight the need of performing integrative multi-omics studies that allow facing, from a physiological point of view, the identification of subpopulations or specific groups of patients responding differently to a defined dietary intervention, such as moderate red wine consumption, promising better nutritional treatment at the metabotype group level. Likewise, strain-level biodiversity will also have to be considered in the near future in microbiome–metabolome-targeted nutritional interventions. Additionally, and in order to fully understand the role of different metabotypes in health, other considerations such as in particular studies with larger numbers of subjects as well as the influence of factors such genetic background, age, sex, habitual dietary patterns and (patho)physiological status should be also be investigated.
4. Conclusions
The metabolic response to a moderate and regular consumption of red wine, presents patterns dependent on the capacity of individuals to metabolize wine polyphenols (metabotypes). However, at level of microbial biodiversity, wine consumption led to interindividual discrepancies in alpha-diversity as well as a significant homogenization of beta-diversity, independently of their metabotypes. In addition, the abundance of key health-related taxa such as Akkermansia sp. was increased after moderate wine intake in the group of high wine-polyphenol-metabolizers. Regard the metabolic activity of the gut microbiota, the concentration of some microbial-derived metabolites (specially, SCFAs) appeared to be correlated and increased after wine intake. Considering that metabolic changes are most likely the result of polyphenol-microbiota interactions, an integrated microbiome–metabolome study of the different metabotypes was carried out, demonstrating a metabotype-dependent effect. Additionally, some metabolites-biomarker species were identified. In summary, our findings highlight the need of performing integrative multi-omics studies that allow approaching, in the near future, personalized nutrition strategies according to metabotypes and/or strain-level biodiversity.
Conflicts of interest
A. Acedo is co-founder and currently employed at Biome Makers, a company providing NGS and data analysis services. C. N. Ravarani was employed by Biome Makers during his contribution to this work. I. Belda was employed by Biome Makers during the initial part of the experimental work (contributing to this work under the framework of his postdoctoral Torres Quevedo Grant – PTQ08253) but he is currently working as an Assistant professor at the Complutense University of Madrid (Spain).
Acknowledgements
This work has been carried out within the framework of the URJC's “WINE MICROBIAL ECOLOGY AND BIOTECHNOLOGY” unit with CSIC. Research in our labs is funded by Grants AGL2015-64522-C2-R, PID2019-108851RB-C21 and PID2019-105834GA-I00 (Spanish Ministry of Science and Innovation), and ALIBIRD-CM 2020 P2018/BAA-4343 (Comunidad de Madrid, Spain).
References
- J. Zhang, Z. Guo, Z. Xue, Z. Sun, M. Zhang, L. Wang, G. Wang, F. Wang, J. Xu, H. Cao, H. Xu, Q. Lv, Z. Zhong, Y. Chen, S. Qimuge, B. Menghe, Y. Zheng, L. Zhao, W. Chen and H. Zhang, A phylo-functional core of gut microbiota in healthy young Chinese cohorts across lifestyles, geography and ethnicities, ISME J., 2015, 9, 1979 CrossRef PubMed.
- M. Arumugam, J. Raes, E. Pelletier, D. Le Paslier, T. Yamada, D. R. Mende, G. R. Fernandes, J. Tap, T. Bruls, J.-M. Batto, M. Bertalan, N. Borruel, F. Casellas, L. Fernandez, L. Gautier, T. Hansen, M. Hattori, T. Hayashi, M. Kleerebezem, K. Kurokawa, M. Leclerc, F. Levenez, C. Manichanh, H. B. Nielsen, T. Nielsen, N. Pons, J. Poulain, J. Qin, T. Sicheritz-Ponten, S. Tims, D. Torrents, E. Ugarte, E. G. Zoetendal, J. Wang, F. Guarner, O. Pedersen, W. M. de Vos, S. Brunak, J. Doré, MetaHIT Consortium, M. Antolín, F. Artiguenave, H. M. Blottiere, M. Almeida, C. Brechot, C. Cara, C. Chervaux, A. Cultrone, C. Delorme, G. Denariaz, R. Dervyn, K. U. Foerstner, C. Friss, M. van de Guchte, E. Guedon, F. Haimet, W. Huber, J. van Hylckama-Vlieg, A. Jamet, C. Juste, G. Kaci, J. Knol, O. Lakhdari, S. Layec, K. Le Roux, E. Maguin, A. Mérieux, R. M. Minardi, C. M'rini, J. Muller, R. Oozeer, J. Parkhill, P. Renault, M. Rescigno, N. Sanchez, S. Sunagawa, A. Torrejon, K. Turner, G. Vandemeulebrouck, E. Varela, Y. Winogradsky, G. Zeller, J. Weissenbach, S. D. Ehrlich and P. Bork, Enterotypes of the human gut microbiome, Nature, 2011, 473, 174 CrossRef CAS PubMed.
- G. D. Wu, J. Chen, C. Hoffmann, K. Bittinger, Y.-Y. Chen, S. A. Keilbaugh, M. Bewtra, D. Knights, W. A. Walters, R. Knight, R. Sinha, E. Gilroy, K. Gupta, R. Baldassano, L. Nessel, H. Li, F. D. Bushman and J. D. Lewis, Linking Long-Term Dietary Patterns with Gut Microbial Enterotypes, Science, 2011, 333, 105 CrossRef PubMed.
- C. De Filippo, D. Cavalieri, M. Di Paola, M. Ramazzotti, J. B. Poullet, S. Massart, S. Collini, G. Pieraccini and P. Lionetti, Impact of diet in shaping gut microbiota revealed by a comparative study in children from Europe and rural Africa, Proc. Natl. Acad. Sci. U. S. A., 2010, 107, 14691 CrossRef PubMed.
- A. Zhernakova, A. Kurilshikov, M. J. Bonder, E. F. Tigchelaar, M. Schirmer, T. Vatanen, Z. Mujagic, A. V. Vila, G. Falony, S. Vieira-Silva, J. Wang, F. Imhann, E. Brandsma, S. A. Jankipersadsing, M. Joossens, M. C. Cenit, P. Deelen, M. A. Swertz, LifeLines cohort study, R. K. Weersma, R. J. M. Feskens, M. G. Netea, D. Gevers, D. Jonkers, L. Franke, Y. S. Aulchenko, C. Huttenhower, J. Raes, M. H. Hofker, R. J. Xavier, C. Wijmenga and J. Fu, Population-based metagenomics analysis reveals markers for gut microbiome composition and diversity, Science, 2016, 352, 565 CrossRef CAS PubMed.
- L. A. David, C. F. Maurice, R. N. Carmody, D. B. Gootenberg, J. E. Button, B. E. Wolfe, A. V. Ling, A. S. Devlin, Y. Varma, M. A. Fischbach, S. B. Biddinger, R. J. Dutton and P. J. Turnbaugh, Diet rapidly and reproducibly alters the human gut microbiome, Nature, 2014, 505, 559 CrossRef CAS PubMed.
- G. D. Wu, C. Compher, E. Z. Chen, S. A. Smith, R. D. Shah, K. Bittinger, C. Chehoud, L. G. Albenberg, L. Nessel, E. Gilroy, J. Star, A. M. Weljie, H. J. Flint, D. C. Metz, M. J. Bennett, H. Li, F. D. Bushman and J. D. Lewis, Comparative metabolomics in vegans and omnivores reveal constraints on diet-dependent gut microbiota metabolite production, Gut, 2016, 65, 63 CrossRef CAS PubMed.
- M. Taguer and C. F. Maurice, The complex interplay of diet, xenobiotics, and microbial metabolism in the gut: Implications for clinical outcomes, Clin. Pharmacol. Ther., 2016, 99, 588 CrossRef CAS PubMed.
- V. Robles-Alonso and F. Guarner, Linking the gut microbiota to human health, Br. J. Nutr., 2013, 109, S21 CrossRef CAS PubMed.
- C. Cueva, I. Gil-Sanchez, B. Ayuda-Duran, S. Gonzalez-Manzano, A. Gonzalez-Paramas, C. Santos-Buelga, B. Bartolomé and M. V. Moreno-Arribas, An integrated view of the effects of wine polyphenols and their relevant metabolites on gut and host health, Molecules, 2017, 22, 99 CrossRef PubMed.
- V. Nash, C. S. Ranadheera, E. N. Georgousopoulou, D. D. Mellor, D. B. Panagiotakos, A. J. McKune, J. Kellett and N. Naumovski, The effects of grape and red wine polyphenols on gut microbiota - A systematic review, Food Res. Int., 2018, 113, 277 CrossRef CAS PubMed.
- E. Barroso, I. Muñoz-González, E. Jiménez, B. Bartolomé, M. V. Moreno-Arribas, C. Peláez, M. C. Martínez-Cuesta and T. Requena, Phylogenetic profile of gut microbiota in healthy adults after moderate intake of red wine, Mol. Nutr. Food Res., 2017, 61, 1600620 CrossRef PubMed.
- R. A. Kemperman, G. Gross, S. Mondot, S. Possemiers, M. Marzorati, T. Van de Wiele and E. E. Vaughan, Impact of polyphenols from black tea and red wine/grape juice on a gut model microbiome, Food Res. Int., 2013, 53, 659 CrossRef CAS.
- F. F. Anhê, D. Roy, G. Pilon, S. Dudonné, S. Matamoros, T. V. Varin, C. Garofalo, Q. Moine, Y. Desjardins, E. Levy and A. Marette, A polyphenol-rich cranberry extract protects from diet-induced obesity, insulin resistance and intestinal inflammation in association with increased Akkermansia spp. population in the gut microbiota of mice, Gut, 2015, 64, 872 CrossRef PubMed.
- I. Muñoz-González, A. Jiménez-Girón, P. J. Martín-Álvarez, B. Bartolomé and M. V. Moreno-Arribas, Profiling of microbial-derived phenolic metabolites in human feces after moderate red wine intake, J. Agric. Food Chem., 2013, 61, 9470 CrossRef PubMed.
- C. Morand, B. De Roos, M. T. Garcia-Conesa, E. R. Gibney, R. Landberg, C. Manach, D. Milenkovic, A. Rodriguez-Mateos, T. Van de Wiele and F. Tomas-Barberan, Why interindividual variation in response to consumption of plant food bioactives matters for future personalised nutrition, Proc. Nutr. Soc., 2020, 79, 225 CrossRef PubMed.
- A. Jiménez-Girón, C. Ibáñez, A. Cifuentes, C. Simó, I. Muñoz-González, P. J. Martín-Álvarez, B. Bartolomé and M. V. Moreno-Arribas, Faecal metabolomic fingerprint after moderate consumption of red wine by healthy subjects, J. Proteome Res., 2015, 14, 897 CrossRef PubMed.
-
A. A. Becares and A. F. Fernandez, Microbiome based identification, monitoring and enhancement of fermentation processes and products, US Patent App, 2018, 15/779531 Search PubMed.
- T. Rognes, T. Flouri, B. Nichols, C. Quince and F. Mahé, VSEARCH: a versatile open source tool for metagenomics, PeerJ, 2016, 4, e2584 CrossRef PubMed.
- I. Muñoz-González, I. Espinosa-Martos, J. M. Rodríguez, A. Jiménez-Girón, P. J. Martín-Álvarez, B. Bartolomé and M. V. Moreno-Arribas, Moderate consumption of red wine can modulate human intestinal inflammatory response, J. Agric. Food Chem., 2014, 62, 10567 CrossRef PubMed.
- A. Cueva, A. Jiménez-Girón, I. Muñoz-González, A. Esteban-Fernández, I. Gil-Sánchez, M. Dueñas, P. J. Martín-Álvarez, M. A. Pozo-Bayón, B. Bartolomé and M. V. Moreno-Arribas, Application of a new in vitro Gastrointestinal Simulator (simgi®) to study the impact of wine in colonic metabolism, Food Res. Int., 2015, 72, 149 CrossRef.
-
I. Oksanen, F. Guillaume-Blanchet, M. Friendly, R. Kindt, P. Legendre, D. McGlinn, P. R. Minchin, R. B. O'Hara, G. L. Simpson, P. Solymos, M. H. H. Stevens, E. Szoecs and H. Wagner, Vegan: community ecology package, R Packag. version 2.4-5, 2017 (https://CRAN.R-project.org/package=vegan) Search PubMed.
- H. Wickham, R for Data Science, J. Stat. Softw., 2017, 77, 1 Search PubMed.
- G. M. Barlow, A. Yu and R. Mathur, Role of the gut microbiome in obesity and diabetes mellitus, Nutr. Clin. Pract., 2015, 30, 787 CrossRef CAS PubMed.
- C. Lordan, D. Thapa, R. P. Ross and P. D. Cotter, Potential for enriching next-generation health-promoting gut bacteria through prebiotics and other dietary components, Gut Microbes, 2020, 11, 1 CrossRef PubMed.
- S. Y. Geerlings, I. Kostopoulos, W. M. De Vos and C. Belzer, Akkermansia muciniphila in the human gastrointestinal tract: When, Where, and How?, Microorganisms, 2018, 6, 75 CrossRef CAS PubMed.
- T. A. F. Corrêa, M. M. Rogero, N. M. A. Hassimotto and F. M. Lajolo, The two-way polyphenols-microbiota interactions and their effects on obesity and related metabolic diseases, Front. Nutr., 2019, 6, 188 CrossRef PubMed.
- A. J. Johnson, P. Vangay, G. A. Al-Ghalith, B. M. Hillmann, T. L. Ward, R. R. Shields-Cutler, A. D. Kim, A. K. Shmagel, A. N. Syed, Personalized Microbiome Class Students, J. Walter, R. Menon, K. Koecher and D. Knights, Daily sampling reveals personalized diet-microbiome associations in humans, Cell Host Microbe, 2019, 25, 789 CrossRef CAS PubMed.
- C. A. Lozupone, J. I. Stombaugh, J. I. Gordon, J. K. Jansson and R. Knight, Diversity, stability and resilience of the human gut microbiota, Nature, 2012, 489, 220 CrossRef CAS PubMed.
- G. Sharon, N. Garg, J. Debelius, R. Knight, P. C. Dorrestein and S. K. Mazmanian, Specialized metabolites from the microbiome in health and disease, Cell Metab., 2014, 20, 719 CrossRef CAS PubMed.
- P. Vernocchi, F. Del Chierico and L. Putignani, Gut microbiota profiling: metabolomics based approach to unravel compounds affecting human health, Front. Microbiol., 2016, 7, 1144 Search PubMed.
- I. Gil-Sánchez, A. Esteban-Fernández, D. González de Llano, M. Sanz-Buenhombre, A. Guadarrana, N. Salazar, M. Gueimonde, C. G. de los Reyes-Gavilán, L. Martín Gómez, M. L. García Bermejo, B. Bartolomé and M. V. Moreno-Arribas, Supplementation with grape pomace in healthy women: Changes in biochemical parameters, gut microbiota and related metabolic biomarkers, J. Funct. Foods, 2018, 45, 34 CrossRef.
- A. Esteban-Fernández, C. Ibañez, C. Simó, B. Bartolomé and V. Moreno-Arribas, Metabolome-based clustering after moderate wine consumption, Oeno One, 2020, 3, 455 CrossRef.
- Y. Xu, Y. Zhu, X. Li and B. Sun, Dynamic balancing of intestinal short-chain fatty acids: the crucial role of bacterial metabolism, Trends Food Sci. Technol., 2020, 100, 118 CrossRef CAS.
- I. Zorraquín, E. Sánchez-Hernández, B. Ayuda-Durán, M. Silva, A. M. González-Paramás, C. Santos-Buelga, M. V. Moreno-Arribas and B. Bartolomé, Current and future experimental approaches in the study of grape and wine polyphenols interacting gut microbiota, J. Sci. Food Agric., 2020, 100, 3789 CrossRef PubMed.
- J. R. Marchesi, D. H. Adams, F. Fava, G. D. A. Hermes, G. M. Hirschfield, G. Hold, M. N. Quraishi, J. Kinross, H. Smidt, K. M. Tuohy, L. V. Thomas, E. G. Zoetendal and A. Hart, The gut microbiota and host health: a new clinical frontier, Gut, 2016, 65, 330 CrossRef PubMed.
- A. Cortés-Martín, R. Garcia-Villalba, A. Gonzalez-Sarrias, M. Romo-Vaquero, V. Loria-Kohen, A. Ramirez-de-Molina, F. A. Tomás-Barberán, M. V. Selma and J. C. Espín, The gut microbiota urolithin metabotypes revisited: the human metabolism of ellagic acid is mainly determined by aging, Food Funct., 2018, 9, 4100 RSC.
- M. V. Selma, A. González-Sarrías, J. Salas-Salvadó, C. Andrés-Lacueva, C. Alasalvar, A. Örem, F. A. Tomás-Barberán and J. C. Espín, The gut microbiota metabolism of pomegranate or walnut ellagitannins yields two urolithin-metabotypes that correlate with cardiometabolic risk biomarkers: Comparison between normoweight, overweight-obesity and metabolic syndrome, Clin. Nutr., 2018, 37, 897 CrossRef CAS PubMed.
- P. Mena, I. A. Ludwig, V. B. Tomatis, A. Acharjee, L. Calani, A. Rosi, F. Brighenti, S. Ray, J. L. Griffin, L. J. Bluck and D. Del Rio, Inter-individual variability in the production of flavan-3-ol colonic metabolites: preliminary elucidation of urinary metabotypes, Eur. J. Nutr., 2019, 58, 1529 CrossRef CAS PubMed.
- F. A. Tomás-Barberán and J. C. Espín, Effect of food structure and processing on (poly)phenol-gut microbiota interactions and the effects on human health, Annu. Rev. Food Sci. Technol., 2019, 10, 221 CrossRef PubMed.
- A. González-Sarrías, R. García-Villalba, M. Romo-Vaquero, C. Alasalvar, A. Örem, P. Zafrilla, F. A. Tomás-Barberán, M. V. Selma and J. C. Espín, Clustering according to urolithin metabotype explains the interindividual variability in the improvement of cardiovascular risk biomarkers in overweight-obese individuals consuming pomegranate: A randomized clinical trial, Mol. Nutr. Food Res., 2017, 61, 1600830 CrossRef PubMed.
- R. Vázquez-Fresno, R. Llorach, A. Perera, R. Mandal, M. Feliz, F. J. Tinahones, D. S. Wishart and C. Andres-Lacueva, Clinical phenotype clustering in cardiovascular risk patients for the identification of responsive metabotypes after red wine polyphenol intake, J. Nutr. Biochem., 2016, 28, 114 CrossRef PubMed.
- C. I. Le Roy, P. M. Wells, J. Si, J. Raes, J. T. Bell and T. D. Spector, Red wine consumption associated with increased gut microbiota α-diversity in 3 independent cohorts, Gastroenterology, 2020, 158, 270 CrossRef CAS PubMed.
- A. Muñiz-Pedrogo, M. D. Jensen, C. T. Van Dyke, J. A. Murray, J. A. Woods, J. Chen, P. C. Kashyap and V. Nehra, Gut microbial carbohydrate metabolism hinders weight loss in overweight adults undergoing lifestyle intervention with a volumetric diet, Mayo Clin. Proc., 2018, 93, 1104 CrossRef PubMed.
- V. Enisoglu-Atalay, B. Atasever-Arslan, B. Yaman, R. Cebecioglu, A. Ku, S. Ozilhan, F. Ozen and T. Catal, Chemical and molecular characterization of metabolites from Flavobacterium sp., PLoS One, 2018, 13, e0205817 CrossRef PubMed.
- B. E. L. Morris, R. Henneberger, H. Huber and C. Moissl-Eichinger, Microbial syntrophy: interaction for the common good, FEMS Microbiol. Rev., 2013, 37, 384 CrossRef CAS PubMed.
- A. Lykidis, C.-L. Chen, S. G. Tringe, A. C. McHardy, A. Copeland, N. C. Kyrpides, P. Hugenholtz, H. Macarie, A. Olmos, O. Monroy and W.-T. Liu, Multiple syntrophic interactions in a terephthalate-degrading methanogenic consortium, ISME J., 2011, 5, 122 CrossRef CAS PubMed.
- H. Li, L. M. Christman, R. Lia and L. Gu, Synergic interactions between polyphenols and gut microbiota in mitigating inflammatory bowel diseases, Food Funct., 2020, 11, 4878 RSC.
- S. P. Claus, The Strange Case of Prevotella copri: Dr. Jekyll or Mr. Hyde?, Cell Host Microbe, 2019, 26, 577 CrossRef CAS PubMed.
- P. I. Costea, F. Hildebrand, M. Arumugam, F. Backhed, M. J. Blaser, F. D. Bushman, W. M. de Vos, S. D. Ehrlich, C. M. Fraser, M. Hattori, C. Huttenhower, I. B. Jeffery, D. Knights, J. D. Lewis, R. E. Ley, H. Ochman, P. W. O'Toole, C. Quince, D. A. Relman, F. Shanahan, S. Sunagawa, J. Wang, G. M. Weinstock, G. D. Wu, G. Zeller, L. Zhao, J. Raes, R. Knight and P. Bork, Enterotypes in the landscape of gut microbial community composition, Nat. Microbiol., 2018, 3, 8 CrossRef CAS PubMed.
- F. De Filippis, E. Pasolli, A. Tett, S. Tarallo, A. Naccarati, M. De Angelis, E. Neviani, L. Cocolin, M. Gobbetti, N. Segata and D. Ercolini, Distinct genetic and functional traits of human intestinal Prevotella copri strains are associated with different habitual diets, Cell Host Microbe, 2019, 25, 444 CrossRef CAS PubMed.
- P. Kovatcheva-Datchary, A. Nilsson, R. Akrami, Y. S. Lee, F. De Vadder, T. Arora, A. Hallen, E. Martens, I. Björck and F. Backhed, Dietary fiber-induced improvement in glucose metabolism is associated with increased abundance of Prevotella, Cell Metab., 2015, 22, 971 CrossRef CAS PubMed.
- H. M. Eid, M. L. Wright, N. V. A. Kumar, A. Qawasmeh, S. T. S. Hassan, A. Mocan, S. M. Nabavi, L. Rastrelli, A. G. Atanasov and P. S. Haddad, Significance of microbiota in obesity and metabolic diseases and the modulatory potential by medicinal plant and food ingredients, Front. Pharmacol., 2017, 8, 387 CrossRef PubMed.
- G. Precup and D.-C. Vodnar, Gut Prevotella as a possible biomarker of diet and its eubiotic versus dysbiotic roles: a comprehensive literature review, Br. J. Nutr., 2019, 122, 131 CrossRef CAS PubMed.
- M. Camps-Bossacoma, F. J. Pérez-Cano, A. Franch and M. Castell, Gut Microbiota in a Rat Oral Sensitization Model: Effect of a Cocoa-Enriched Diet, Oxid. Med. Cell. Longevity, 2017, 2017, 7417505 Search PubMed.
- T. Chen, W. Long, C. Zhang, S. Liu, L. Zhao and B. R. Hamake, Fiber-utilizing capacity varies in Prevotella- versus Bacteroides-dominated gut microbiota, Sci. Rep., 2017, 7, 2594 CrossRef PubMed.
- C. Zhao, H. Dong, Y. Zhang and Y. Li, Discovery of potential genes contributing to the biosynthesis of short-chain fatty acids and lactate in gut microbiota from systematic investigation in E. coli, NPJ Biofilms Microbiomes, 2019, 5, 19 CrossRef PubMed.
- A. Saffarian, M. Touchon, C. Mulet, R. Tournebize, V. Passet, S. Brisse, E. P. C. Rocha, P. J. Sansonetti and T. Pédron, Comparative genomic analysis of Acinetobacter strains isolated from murine colonic crypts, BMC Genomics, 2017, 18, 525 CrossRef PubMed.
- A. Braune, W. Engst, P. W. Elsinghorst, N. Furtmann, J. Bajorath, M. Gütschow and M. Blaut, Chalcone isomerase from Eubacterium ramulus catalyzes the ring contraction of flavanonols, J. Bacteriol., 2016, 198, 2965 CrossRef CAS PubMed.
- A. M. Vaiserman, A. K. Koliada and F. Marotta, Gut microbiota: a player in aging and a target for anti-aging intervention, Ageing Res. Rev., 2017, 35, 36 CrossRef CAS PubMed.
- M. Quesada-Molina, A. Muñoz-Garach, F. J. Tinahones and I. Moreno-Indias, A new perspective on the health benefits of moderate beer consumption: involvement of the gut microbiota, Metabolites, 2019, 9, 272 CrossRef CAS PubMed.
- A. Geirnaert, A. Steyaert, V. Eeckhaut, B. Debruyne, J. B. A. Arends, F. Van Immerseel, N. Boon and T. Van de Wiele, Butyricicoccus pullicaecorum, a butyrate producer with probiotic potential, is intrinsically tolerant to stomach and small intestine conditions, Anaerobe, 2014, 30, 70 CrossRef CAS PubMed.
- V. Eeckhaut, K. Machiels, C. Perrier, C. Romero, S. Maes, B. Flahou, M. Steppe, F. Haesebrouck, B. Sas, R. Ducatelle, S. Vermeire and F. Van Immersee, Butyricicoccus pullicaecorum in inflammatory bowel disease, Gut, 2013, 62, 1745 CrossRef CAS PubMed.
Footnote |
† Electronic supplementary information (ESI) available. See DOI: 10.1039/d0fo02938f |
|
This journal is © The Royal Society of Chemistry 2021 |
Click here to see how this site uses Cookies. View our privacy policy here.