Cast iron drinking water pipe biofilms support diverse microbial communities containing antibiotic resistance genes, metal resistance genes, and class 1 integrons†
Received
1st December 2020
, Accepted 23rd January 2021
First published on 1st February 2021
Abstract
Antimicrobial resistance is a well-documented public health concern. The role that drinking water distribution pipes have as sources of antibiotic resistance genes (ARGs) is not well known. Metals are a known stressor for antibiotic resistance development, implying that aging metal-pipe infrastructure could be a source of ARGs. The objective of this study was to determine if ARGs, metal resistance genes (MRGs), and intI1 were pervasive across various pipe biofilm sample types (biomass surfaces, pipe surfaces, corrosion tubercles, and under corrosion tubercles) and if the resistance genes associated with particular microbial taxa. Eight sample types in triplicate (n = 24) were taken from inside a >100 year-old, six ft. section of a full-scale chloraminated cast iron drinking water main. Droplet digital PCR (ddPCR) was employed as a novel approach to quantify ARGs in pipes from full-scale drinking water distribution systems (DWDS) because it yielded higher detection frequencies than quantitative PCR (qPCR). Illumina sequencing was employed to characterize the microbial community based on 16S rRNA genes. ARGs and MRGs were detected in all 24 pipe samples. Every sample contained targeted genes. Interestingly, the mean absolute abundances of ARGs and MRGs only varied by approximately one log value across sample types, but the mean relative abundances (copy numbers normalized to 16S rRNA genes) varied by over two log values. The ARG and MRGs concentrations were not significantly different between sample types, despite significant changes in dominant microbial taxa. The most abundant genera observed in the biofilm communities were Mycobacterium (0.2–70%), and β-lactam resistance genes blaTEM, blaSHV, and the integrase gene of class 1 integrons (intI1) were positively correlated with Mycobacterium. The detection of ARGs, MRGs, and class 1 integrons across all sample types within the pipe indicates that pipes themselves can serve as sources for ARGs in DWDS. Consequently, future work should investigate the role of pipe materials as well as corrosion inhibitors to determine how engineering decisions can mitigate ARGs in drinking water that stem from pipe materials.
Water impact
Aging drinking water infrastructure, particularly when made of metals, can have significant impacts on microbial water quality, and specifically antibiotic resistance genes. This research revealed that various types of biofilm samples in a cast iron pipe can serve as sources of antibiotic resistance genes in drinking water distribution systems. Infrastructure material decisions can be part of antibiotic resistance mitigation strategies.
|
1. Introduction
Antibiotic resistance is a major public health concern stemming from the microbial response to the widespread occurrence of antibiotics and other physiological stressors in the environment.1,2 Approximately 2.8 million people are diagnosed with infections caused by antibiotic-resistant bacteria (ARB), and over 35
000 deaths are attributed to antibiotic resistance annually in the U.S. alone.3 Antibiotic resistance genes (ARGs) on mobile genetic elements can be acquired by pathogens in the human gut,4–6 creating risks for vulnerable populations that are exposed to ARGs.7 ARGs have been detected in various water environments including groundwater,8 surface water,2,9 drinking water treatment plants,10,11 and tap drinking water12–14 at concentrations up to 1010 copies per L.11 Consequently, it is becoming increasingly important to quantify ARGs in exposure routes that directly convey ARGs to people, including drinking water distribution systems (DWDS).15–17
ARGs in tap water have been shown to increase from the drinking water treatment plant effluent to the tap.10 While residual disinfectants can select for antibiotic resistance,18,19 the actual infrastructure of DWDS, i.e. the pipe materials used, could also be an important factor that impacts microbial ecology and consequently ARG profiles.20–24 DWDS are comprised of a variety of metallic pipe materials (e.g., copper, iron, and lead) and additional metals in treated drinking water can accumulate in biofilms and corrosion scales.25–28 Metals select for antibiotic resistance through co-resistance and cross-resistance mechanisms.29,30 Additionally, microorganisms have evolved detoxification strategies, such as metal resistance genes (MRGs) and efflux pumps, to mitigate the toxic effects of metals.31,32 Exposing bacteria to metals in DWDS may promote the survival of bacteria resistant to metals and antibiotics.30,33 While studies have documented the occurrence of ARGs in tap drinking water and in biofilms,34,35 to the best of our knowledge, no research efforts have quantified ARGs, MRGs, and mobile genetic elements from different biofilm sample locations (e.g., surface biofilms, tubercles, under tubercles) in a single pipe to understand if drinking water pipes can serve as sources of ARGs.
The objective of this research was to determine if ARGs, MRGs, and class 1 integrons (intI1) were quantifiable across multiple sample types in a chloraminated cast iron water main and to determine if microbial taxa were correlated to resistance gene concentrations. It was hypothesized that ARGs, MRGs, and intI1 would be detected regardless of sample type and location. The abundance of bacterial biomass (measured by 16S rRNA gene copies), ARGs, MRGs, and the integrase gene of the class 1 integron, intI1, were quantified in samples collected from different microenvironments using droplet digital PCR (ddPCR) and quantitative PCR (qPCR). Microbial communities were analyzed using PCR-amplified 16S rRNA gene sequences from each pipe sample (n = 24). This is the first research to determine if various types of biofilm samples from a single full-scale DWDS pipe can serve as potential sources for ARGs.
2. Materials and methods
2.1 Pipe collection, sampling, and DNA extraction
A six ft section of cast-iron water main (18′′ ID, 105 years in operation) that transported chloraminated water was extracted, covered with sterile plastic sheeting, and immediately transported to the laboratory for sampling and analysis. The water main was collected as part of planned maintenance to replace old water mains throughout the distribution system. Pipe samples were collected from i) a visible biofilm surface, referred to as “biomass surface” (n = 6), ii) a pipe surface that did not have biofilm visible to the naked eye, referred to as “pipe surface” (n = 6), iii) from three-dimensional corrosion tubercles that could be removed, referred to as “corrosion tubercles” (n = 6), and iv) from the pipe surface on the location where the tubercle was removed, referred to as “under corrosion tubercles” (n = 6) (ESI†Fig. 1). Broadly speaking, all samples were microbial biofilms, not bulk water samples, and they were subcategorized into the four categories listed for comparison and statistical analysis. Pipe surface, biomass surface, and under tubercle swabs were collected by firmly pressing a sterile cotton-tipped applicator (Fisher Scientific, Waltham, MA) on the biofilm surface and swabbing an area of approximately 2–5 cm2. For each of the microenvironment types sampled, top (n = 3) and bottom (n = 3) samples of the cast iron water main were collected. Each swab was transferred directly to a sterile DNA extraction lysing tube and the stem was snapped and severed to preserve only the sample end of the swab.36 Tubercle samples were collected into plastic tubes using a flame sterilized spatula, and approximately 0.2 g of corrosion tubercle was sub-sampled for DNA extraction. Samples were immediately frozen at −20 °C until DNA extraction was performed. DNA was extracted using the FastDNA Spin Kit (MP Biomedicals, Solon, OH). The manufacturer's protocol was followed with the exception that initial cell lysis was conducted using liquid nitrogen freeze thaw cycling (3×).37–40 DNA concentrations in resulting extracts were quantified by microspectrophotometry using a Nano-Drop (Nano-Drop™ Lite, Thermo Scientific, Waltham, MA) and stored at −20 °C.
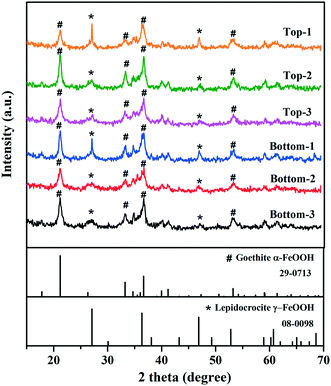 |
| Fig. 1 X-ray diffraction (XRD) patterns of biofilm tubercle samples (n = 6) collected from the chloraminated cast-iron water main. The reference XRD patterns for goethite (JCPDS 29-0713) and lepidocrocite (JCPDS 08-0098) are also shown for comparison. | |
2.2 Quantification of resistance genes
Droplet digital PCR (ddPCR) assays were conducted to quantify gene copies. A subset of samples was initially analyzed at 5, 10, 50, and 100-fold dilutions to test for inhibition during gene quantification. Based on these results, a 10-fold dilution was selected to minimize inhibition for all samples. Reaction mixtures consisted of a total volume of 22 μL with 11 μL of QX200 ddPCR EvaGreen Supermix (final concentration 1×) (Bio-Rad Laboratories Inc., Hercules, CA), 2 μL each of forward and reverse primers (final concentration 250 nM each), 4 μL of diluted DNA extract, and 3 μL molecular grade water. The ddPCR reaction mixture was added to a 96-well plate, sealed with foil, homogenized by vortexing, and centrifuged briefly to ensure that all reaction components were at the bottom of the wells. The 96-well plate was equilibrated at room temperature for 3 minutes prior to droplet generation. Aliquots of 20 μL for each reaction were dispensed into a separate well of an eight-channel droplet generator cartridge (DG8 Cartridge, Bio-Rad) followed by 70 μL of QX200 Droplet Generation Oil for EvaGreen into the oil wells for subsequent droplet generation using the QX100 Droplet Generator. Oil-droplet mixtures were transferred to a 96-well plate and sealed at 180 °C using the PX1 PCR plate sealer. The 96-well plate was transferred to the C1000 touch thermal cycler for PCR thermal cycling with the following conditions: 5 min at 95 °C for activation of DNA polymerase, 39 cycles of 95 °C for 30 s and 60 °C for 60 s, followed by signal stabilization at 4 °C for 5 min and 90 °C for 5 min. Thermal cycling conditions were modified for genes with annealing temperatures varying from 60 °C (ESI† Table S1). After thermal cycling, plates were transferred to the QX200 Droplet Reader for absolute quantification of target genes.
Data analysis was performed using the QuantaSoft Analysis Pro software and expressed as gene copies μL−1 (V 1.0.596, Bio-Rad). Positive controls were included with each ddPCR assay and were produced by ten-fold serial dilution of plasmid DNA yielding 104 to 100 copies per reaction. No-template (i.e., reagent only) controls were included with each ddPCR assay. All ddPCR negative controls failed to yield amplification above the limit of quantification for each assay. Thresholds to discriminate between positive and negative droplets were manually applied to each sample and only samples with ≥3 positive droplets were considered as positive.41 Furthermore, only reactions with greater than 10
000 accepted droplets were used for subsequent analysis.41 The limit of the detection (LOD) and limit of quantification (LOQ) for each tested gene were determined according to the MIQE guidelines.42,43 Additional MIQE guidelines were followed and are shown in ESI† Table S2. DNA extract from each sample was analyzed in triplicate for each target gene, and the average value from analyzing each DNA sample three times was used for each sample for further analysis.
Target gene copies were also quantified in triplicate from DNA extracts using qPCR with previously published protocols for the 16S rRNA gene,44 ARGs (blaSHV,10blaTEM,45sul1,46 MRGs (czcD, copA),47 and the integrase gene of class 1 integrons (intI1).48 Additional information on qPCR methodology, specific primer sets, amplicon sizes, annealing temperatures, R2 values, efficiencies, and quantification limits are described in the ESI† Methods and Table S1. β-lactam resistance genes such as blaTEM and blaSHV are grouped in the most common types of β-lactamases belonging to Enterobacteriaceae and encode resistance to β-lactam antibiotics such as penicillins and cephalosporins.49 Sulfonamide resistance gene (sul1) and the integrase gene of class 1 integrons (intI1) are frequently detected in various natural and engineered environments and are considered a good proxy for ARG abundance and anthropogenic pollution.50,51 MRGs quantified in biofilm samples from the cast iron water main included the copper resistance gene copA and czcD, which is a part of the cation diffusion facilitator mediating resistance to cadmium, zinc, and cobalt.52,53 These genes were selected based on their abundance in an initial qPCR assay conducted with over 20 different MRGs including genes encoding resistance to metals such as arsenic, copper, iron, lead, and zinc.
2.3 PCR and Illumina sequencing of 16S rRNA gene amplicons
Microbial communities from biofilm samples were prepared for analysis by triplicate PCR-amplifying and pooling V4 hypervariable regions of 16S rRNA genes.54 One extraction blank and mock community (#HM-782D, BEI Resources) were included in the sample set. PCR amplicons were sequenced with Illumina MiSeq 2 × 250 paired-end chemistry at the Great Lakes Genomic Center (http://greatlakesgenomics.uwm.edu). Primer and barcode sequences were removed from reads using cutadapt.55 Reads were processed, including filtered, merged, error-corrected, and chimera-checked, into amplicon sequence variants (ASVs) using the R package DADA2.56 Taxonomy was assigned using DADA2 from the SILVA v. 132 reference database.57 ASVs that were classified as mitochondria, chloroplast, or eukaryota were removed. Additional thresholds were set to identify and remove ASVs potentially derived from the mock community, extraction/PCR blank, and non-target samples that were included in the sequencing run. Additional description of sequencing methods is included in the ESI† Methods. Raw sequences have been uploaded to NCBI Sequence Read Archive (SRA) under BioProject ID PRJNA692495.
2.4 Corrosion tubercle characterization
X-ray diffraction (XRD) analysis was conducted on corrosion tubercles that were sampled from the cast iron water main (n = 6) to identify the dominant crystalline phases. XRD was performed on a Bruker D8 Discover A25 diffractometer using copper Kα radiation with step scanning from 2θ of 15–70°. The scan speed and step size were 3° per min and 0.02°, respectively. XRD patterns of each corrosion tubercle were compared to standard patterns from International Centre for Diffraction Data (ICDD).
The inorganic elemental composition of corrosion tubercles was determined using inductively coupled plasma mass spectrometry (ICP-MS).58 Approximately 0.1 g of each corrosion tubercle was subsampled for elemental analysis. Each tubercle sample was homogenized using a sterile mortar and pestle prior to acid digestion with nitric acid (2%) and hydrochloric acid (1%).59 An Agilent Technologies 7700 Series ICP-MS (Agilent Technologies Inc., Santa Clara, CA) was used for elemental composition determination. Standard reference materials for elements including Ag, Al, As, Ba, Be, Ca, Cd, Co, Cr, Cu, Fe, Hg, Mg, Mn, Mo, Na, Ni, Pb, Sb, Se, Th, Tl, U, V, and Zn were purchased from Agilent Technologies.
2.5 Statistical analysis
Statistical analyses were conducted using RStudio in the open-sourced statistical program R (V 3.6.1).60,61 One-way analysis of variance (ANOVA) was conducted using the ‘aov’ function to determine statistical differences between abundances of target genes across groups of samples. A significant cutoff of α = 0.05 was used for all analyses. For sequence analysis, the BIOM file generated via DADA2 was imported into R using the phyloseq package.62 R packages ‘phyloseq’ and ‘ggplot2’ were utilized for general visualization of sequence data. Alpha and beta diversity metrics and plots were generated using the ‘vegan’ and ‘ggplot2’ packages. ANOVA was used to determine significance among the alpha diversity metrics. Principal coordinate analysis (PCoA) was performed using the ‘ape’ package to visualize differences between samples using the Bray–Curtis dissimilarity matrix generated in ‘phyloseq’. Canonical correspondence analysis (CCA) was conducted in R using the vegan package to identify correlations between the bacterial community structure and biofilm sample location. Spearman's rank sum correlation coefficients were calculated in R to assess correlations between ARGs, MRGs, abundant taxa, and biofilm sample location. Indicator taxa were identified for each sample location using the multi-level pattern (indicator species) analysis in the package ‘indicspecies’.63 Rarefaction curves were generated using the ‘ggrare’ function from the phyloseq-extended package of scripts.64
3. Results and discussion
3.1 Physical characterization of corrosion tubercles
XRD analysis indicated that the mineral phases present in the interior surfaces of the cast iron pipe primarily consisted of goethite (α-FeOOH) and lepidocrocite (γ-FeOOH) (Fig. 1). Goethite has been widely found as a main corrosion product in cast iron pipes65–70 and several previous studies also reported the observation of lepidocrocite in iron pipes from full-scale DWDS.71,72 The inorganic elemental composition of corrosion tubercles was characterized by ICP-MS. Iron was the dominant element in corrosion tubercles representing approximately 98.6% of the measured mass. Other elements detected in quantities ranging from 0.1% to 1.0% in the tubercles included Ca (0.64%), Al (0.38%), Mg (0.12%), and Mn (0.10%). Elements detected below 0.1% included Na, K, Co, Cu, Pb, Zn, V, As, Se, Mo, Ag, Cd, Hg, Ni, and Be. Metals present in corrosion scales and tubercles in the cast iron water main may have originated from the pipe material itself, especially Fe, but other trace elements were likely deposited over time from the bulk drinking water.
3.2 Quantification of bacterial biomass
16S rRNA genes were detected above quantification limits in all 24 samples from the cast iron water main (ESI† Fig. S2). The mean concentration in corrosion tubercles was 4.4 × 107 16S rRNA gene copies per g tubercle. The mean concentration in biofilms collected under corrosion tubercles was 3.5 × 105 16S rRNA copies per cm2. Previous studies have reported similar levels of 16S rRNA in biofilms from chloraminated water mains with averages ranging from 3.2 × 105 to 2.5 × 107 copies per cm2.73–75
3.3 Quantification of resistance genes in pipe samples
3.3.1 Detection frequency by ddPCR and qPCR.
The frequency of gene detects for every gene analyzed across the 24 samples using ddPCR was equal to or higher than that for qPCR (Table 1; ESI† Fig. S3). The presence of inhibiting substances such as metals or humic acids in the biofilm samples are known to impact amplification and primer annealing in qPCR assays.76,77 Previous studies have also demonstrated that ddPCR, as compared to qPCR, can have increased precision and accuracy for quantifying low concentrations of DNA in variably contaminated samples.76–78 Our findings in conjunction with previous studies suggest that ddPCR is favorable for detecting ARGs in DWDS, particularly because these samples often contain low concentrations of DNA and contaminants that may interfere with qPCR. Reporting limit of detection and limit of quantification will be key for comparing across studies, as these values can differ significantly among quantification methods.
Table 1 Summary of detections of ARGs, MRGs, and intI1 with ddPCR and qPCR
Gene |
Mechanism/mode of action |
ddPCR detections |
ddPCR LOQ (CN μL−1) |
ddPCR LOQ (CN cm−2) |
qPCR detections |
qPCR LOQ (CN μL−1) |
qPCR LOQ (CN cm−2) |
Notes: CN – copy numbers, LOQ – limit of quantification, NA – not applicable.
|
16S rRNA |
NA |
24 (100%) |
5 |
5 × 103 |
24 (100%) |
500 |
5 × 105 |
bla
SHV
|
Beta lactam resistance |
24 (100%) |
2 |
2 × 103 |
20 (83%) |
50 |
5 × 104 |
bla
TEM
|
Beta lactam resistance |
24 (100%) |
2 |
2 × 103 |
24 (100%) |
5 |
5 × 103 |
copA |
Copper resistance |
11 (45%) |
4 |
4 × 103 |
3 (12.5%) |
5 |
5 × 103 |
czcD |
Cobalt, zinc, cadmium efflux |
20 (83%) |
3 |
3 × 103 |
19 (79%) |
5 |
5 × 103 |
intI1 |
Integrase gene of class 1 integrons |
10 (42%) |
4 |
4 × 103 |
8 (33%) |
5 |
5 × 103 |
sul1 |
Enzymatic modification |
17 (71%) |
3 |
3 × 103 |
4 (17%) |
5 |
5 × 103 |
tet(L) |
Tetracycline efflux |
0 (0%) |
6 |
6 × 103 |
0 (0%) |
50 |
5 × 104 |
3.3.2 Abundance of antibiotic resistance genes (ARGs) and intI1.
The ARGs blaSHV, blaTEM, and sul1, and intI1 were detected in biofilm samples from the chloraminated cast-iron drinking water main at concentrations up to nearly 6 log gene copies cm−2, with the highest mean value belonging to gene blaTEM at approximately 4.8 log gene copies cm−2 (Fig. 2). A previous study reported the mean absolute abundance of ARGs (blaTEM, sul1, qnrA, vanA) and intI1 in biofilms from undefined pipe materials to range from <LOQ to 4.2 log copies swab−1.34 Mean absolute abundances of ARGs and intI1 in different biofilm microenvironments varied by over one log unit, but the mean differences of the sample types were not significantly different from each other (one-way ANOVAs, p values > 0.05), indicating that sample type did not impact absolute gene abundances. Relative gene abundances (absolute normalized to 16S rRNA gene copies) demonstrated higher variability (>2-log units) between the different microenvironments sampled, but the mean relative abundance values were not significantly different based on sample type (one-way ANOVA, p > 0.05) (ESI† Fig. S4). The variability in relative abundance values for ARGs in the different microenvironments was primarily due to differences in levels of bacterial 16S rRNA genes between sample locations rather than changes in ARG abundance. Indeed, these results indicate that various pipe biofilm samples, and thus pipe infrastructure, could serve as sources of ARGs into tap drinking water.
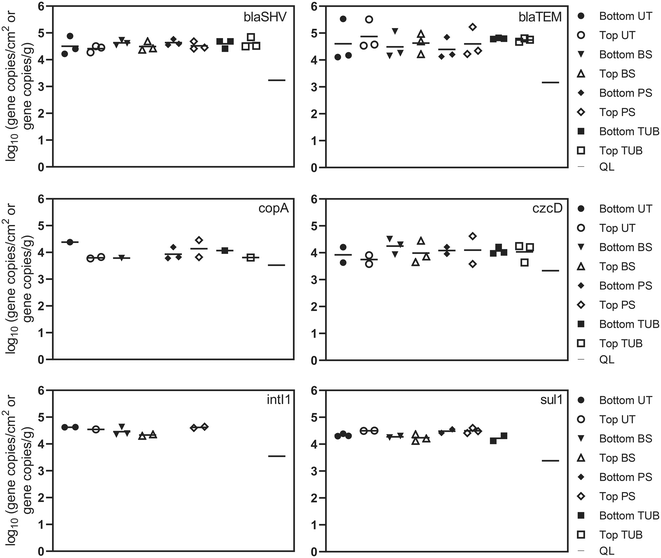 |
| Fig. 2 Absolute abundance of antibiotic resistance genes, metal resistance genes, and intI1 in different biofilm microenvironments from a cast iron drinking water main as measured with ddPCR. The biofilm microenvironments include biomass surface (BS), pipe surface (PS), tubercle (TUB), and under tubercle (UT). Each biofilm sample is also categorized by top or bottom pipe sample location. Sample categories (e.g. bottom UT, top BS) are plotted when 1–3 of the samples yielded a quantifiable result. Sample categories with no positive detections were left blank. Each symbol represents the average of 3 ddPCR technical replicates from a single biofilm sample. All biofilm swab samples are plotted as log10 gene copies per cm2. Corrosion tubercle samples are plotted as log gene copies per g. The quantification limit (QL) is also plotted for each gene as the dash on the right side of the plot. | |
Absolute concentrations of ARGs remained relatively consistent in the different biofilm sample locations. ARGs were detected more frequently in surficial biofilm environments but demonstrated similar absolute abundance compared to sub-surface environments. One explanation could be that corrosion deposits and tubercles can provide relief from disinfectants, advective flow, and shear stress which allows additional biofilm development to occur.80 Observed relative abundance values for ARGs in the current study suggest that microbes in sub-surface communities may harbor ARGs at similar levels compared to surficial microbes. The presence of ARGs in each of the different biofilm sample locations suggests that potential selection for antibiotic resistance exerted by disinfectants, metals and other dissolved contaminants exists throughout the cast iron biofilm communities inside the pipe. Additionally, the detection of ARGs and class 1 integrons in the biofilm communities suggests that horizontal gene transfer may be one plausible explanation for the proliferation of ARGs inside the cast iron biofilm communities due to the close proximity of microbial species within biofilms.30,79 Average and median chloramine concentrations inside the distribution system were 1.0 to 1.3 mg L−1. Residual disinfectants such as chloramines present in DWDS at subinhibitory levels have been shown to stimulate horizontal gene transfer of ARGs through multiple pathways including reactive oxygen species response systems and the SOS response.18
Beta-lactamase resistance genes, blaSHV and blaTEM, were detected in all 24 biofilm samples ranging from 4.1 to 5.3 log gene copies per cm2. A previous study detected blaTEM in drinking water biofilms at lower absolute abundance (mean = 1.5 log gene copies swab−1) but with similar relative abundance (mean = −2.3 log ARG copies/16S rRNA copies) as in this study.34 These results demonstrate that different microenvironments or niches in pipes can serve as reservoirs for bacteria harboring resistance genes, indicating that biofilms on DWDS pipes can serve as sources of ARGs when biofilms shed viable cells into tap drinking water.79 The sulfonamide resistance gene (sul1) and the integrase gene of class 1 integrons (intI1) are frequently detected in various natural and engineered environments and are considered potential indicators of horizontal gene transfer of ARGs.50,51 The intI1 gene was detected in 10 biofilm samples at an average concentration of 4.5 log gene copies per cm2. The intI1 gene was detected at a higher frequency in biofilm surface samples (83%) and was not detected in tubercle samples. The frequency of sul1 detections for biofilm surface, pipe surface, and under tubercle samples was 83%, compared to 33% for corrosion tubercles. Additional variation in ARG abundance was observed between samples collected from the top and bottom of the water main, but the differences were not statistically different (one-way ANOVA, p values >0.05). The distance of biofilm sample collection inside the water main (12 in., 18 in., and 24 in.) also did not have a significant effect on ARG concentrations.
3.3.3 Abundance of metal resistance genes (MRGs).
MRGs quantified in biofilm samples from the cast iron water main included the copper resistance gene copA and czcD. The czcD gene was detected in 83% of biofilm samples at concentrations ranging from above LOQ to 4.6 log gene copies cm−2. The mean czcD absolute abundance in surficial samples (BS, PS) was not significantly different compared to sub-surface samples (TUB, UT) according to One-way ANOVA results (p > 0.05). The czcD gene is part of the cation diffusion facilitator mediating resistance to cadmium, zinc, and cobalt52,53 and has previously been documented in source drinking water treated with the corrosion inhibitor zinc orthophosphate.58 The copper resistance gene copA was detected above the LOQ in 45% of samples with ddPCR assays. The copA gene encodes an ATPase efflux pump that extrudes copper ions, making it one of the main mechanisms mediating copper resistance.81 The detection of czcD and copA may be related to the presence of copper, zinc, cadmium, and cobalt ions in the drinking water and corrosion deposits. Previous studies have demonstrated that exposure to sub-inhibitory levels of Cu(II) and Zn(II) can contribute to horizontal gene transfer of ARGs.29 This is the first study to quantify clinically-relevant ARGs and MRGs in multiple types of biofilm samples from a chloraminated cast iron water main collected from a full-scale distribution system.
3.4 Microbial community composition of pipe samples
Corrosion tubercles, especially from the bottom of the water main, exhibited the most unique microbial community composition compared to the biomass surface, pipe surface, and under tubercle samples (Fig. 3a). Biofilm microbial communities exhibited similar Shannon diversity (1.8–4.4) to previous observations of biofilm communities in cast iron drinking water mains73 (ESI† Fig. S5). A total of 469 microbial genera corresponding to 47 different phyla were identified in the biofilm microbial communities from the cast iron water main. The most abundant genera observed in the biofilm communities included Mycobacterium (0.2–70%), Geobacter (0–57%), Gallionella (0–40%), Phreatobacter (0–25%), Desulfovibrio (0–21%), Undibacterium (0–18%), Streptococcus (0–17%), and Sphingomonas (0–17%) (Fig. 3b). Previous studies have also observed high abundance of Mycobacterium, Geobacter, Gallionella, Sphingomonas, and Undibacterium in corrosion deposits and biofilms in DWDS.68,82–84 Other abundant genera observed in the cast iron biofilm communities included Hydrogenophaga (0–15%), Rhodoferax (0–15%), Galbitalea (0–12%), Corynebacterium (0–11%), Ralstonia (0–8%), and Geothrix (0–6%).
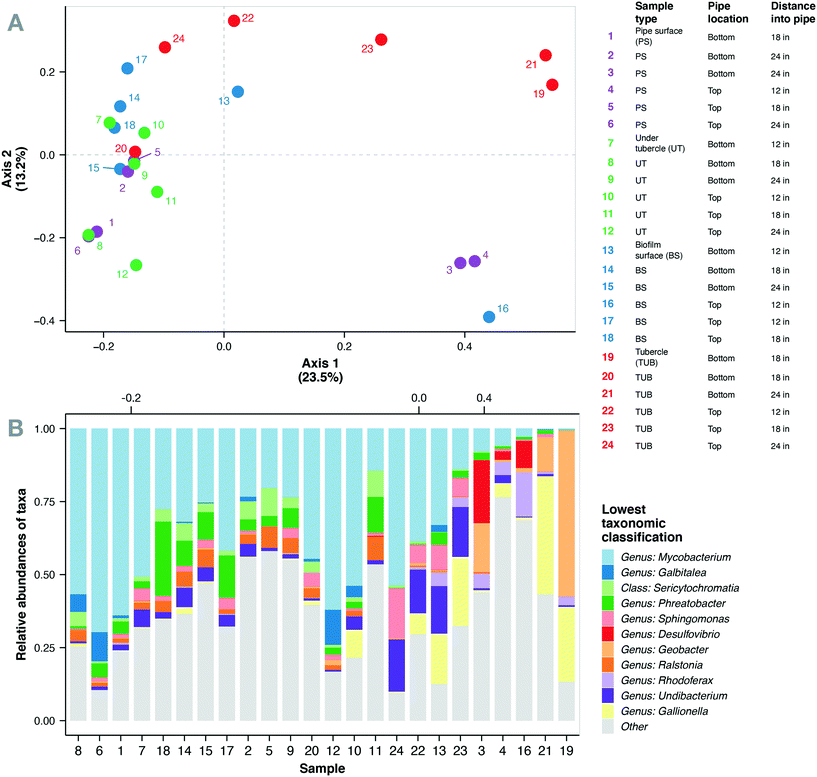 |
| Fig. 3 (A) Principal coordinate analysis (PCoA) using Bray–Curtis dissimilarity of microbial communities in biofilm samples from the chloraminated cast-iron drinking water main (n = 24). Color of points denote sample type and are as described in table on top right. Point labels refer to sample number in table. (B) Relative abundance of 11 most abundant taxa combined down to lowest classification. Samples on x-axis ordered along axis 1 of PCoA. | |
Mycobacterium represented the most abundant genus in the current study with 22 unique Mycobacterium ASVs detected in the biofilm communities. These ASVs comprised 32% of the total sequences in the biofilm samples and were detected in all 24 biofilm samples. Mean relative abundance of Mycobacterium spp. was highest in under tubercle samples (43%) compared to other sample locations (25–32%), but the differences were not statistically significant (one-way ANOVA, p > 0.05). Although the mean values were not significantly different, relative abundance of Mycobacterium exhibited wide variability across individual samples ranging from 0.2 to 70%. These findings are consistent with previous studies that have reported Mycobacterium as the dominant genus in drinking water biofilms from chloraminated cast iron water mains.73,75,82Mycobacterium spp. are frequently detected in DWDS85–87 and have several characteristics that give them a competitive advantage in chloraminated DWDS including the ability to form biofilms, resistance to residual disinfectants,88 and the ability to survive in nutrient-deficient environments.89,90 The prevalence of Mycobacterium in full-scale DWDS is a potential concern because the genus contains several opportunistic pathogens.90–93
The abundance patterns for the most abundant taxa are shown in Fig. 4. Several corrosion-related bacterial genera including Sphingomonas, Desulfovibrio, Gallionella, Geobacter, Hydrogenophaga, and Rhodobacter were observed in the biofilm communities and have been previously documented in cast-iron pipe biofilms and corrosion scales.67,68,73,94–96 Bacteria inhabiting distribution systems primarily perform functions related to carbon source utilization and nitrification, as well as microbial induced corrosion processes.97 Corrosion tubercles contained increased abundance of microorganisms related to microbial induced corrosion and redox cycling processes in cast iron pipes including genera such as Gallionella, Geobacter, Geothrix, and Undibacterium.67,68,73 Previous studies have demonstrated that the abundance of microorganisms in DWDS may be impacted by the presence of corrosion tubercles, suspended solids, and other loose corrosion deposits.98,99 Additionally, the bottom of water mains can accumulate higher densities of bacteria and corrosion deposits, which may also support increased abundance of nitrifying bacteria.100 Similarly, the biofilm samples from the bottom of the cast iron water main displayed increased relative abundance of bacterial genera related to iron and nutrient cycling.
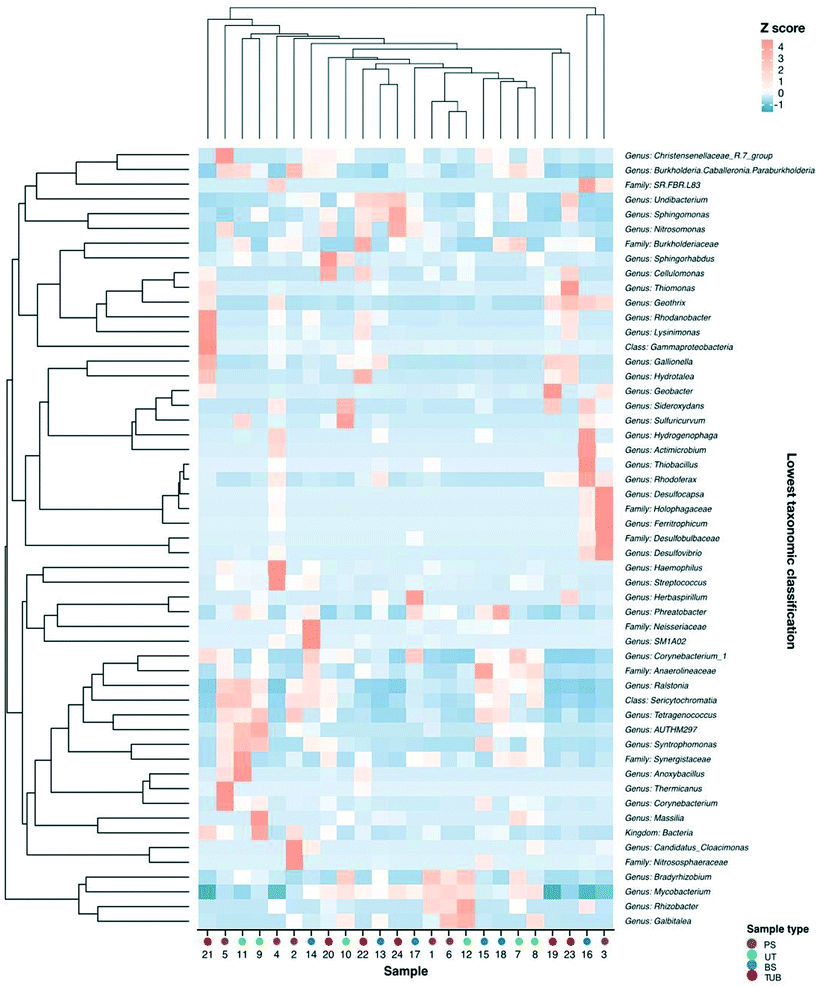 |
| Fig. 4 Heatmap of normalized (Z score) abundances of most abundant (maximum relative abundance >2% in at least one sample) taxa combined to lowest classification. Samples along x-axis and taxa along y-axis were clustered into dendrograms using Bray–Curtis dissimilarity. Colors of points on x-axis denote sample type. | |
Spearman correlation analysis revealed significant relationships between the co-occurrence of several corrosion-related bacteria in the biofilm communities (ESI† Fig. S6). The genus Ralstonia demonstrated the most significant relationships with other taxa, and it is considered an emerging global opportunistic pathogens in municipal water supplies.101 Positive relationships were observed between the co-occurrence of corrosion-related bacteria from Geobacter and Gallionella genera, which also made up a large proportion of the communities in corrosion tubercles. Geobacter were most prevalent in corrosion tubercles (11.7%) but were also identified in lower abundance in pipe surface (3.1%), biofilm surface (0.4%), and under tubercle environments (0.4%). The Geobacter genus contains iron-reducing species, and previous studies have reported that Geobacter spp. were among the most resistant bacteria to monochloramine disinfection in lab-scale disinfection experiments.102 Similarly, Gallionella spp. were more abundant in corrosion tubercles (16.5%) compared to biofilm surface (3.6%), pipe surface (1.0%), and under tubercle environments (1.9%). Gallionella spp. are neutrophilic iron oxidizing bacteria (IOB) that can promote the precipitation of iron oxides by converting ferrous iron to ferric iron and have previously been observed in association with severe iron corrosion release or “red water” events in distribution systems.96,103 Bottom tubercle samples demonstrated increased relative abundance of Gallionella and Geobacter (22.5% and 23%) compared to top tubercle samples (10.5% and 0.4%), but these differences were not significant (one-way ANOVA, p values >0.05).
Indicator species analysis was employed to identify the most abundant indicator organisms in each sample location from the water main. Several corrosion-related genera were significant indicator organisms for corrosion tubercle samples including Geothrix, Gallionella, Phreatobacter, Thiomonas, and Rhodovastum (all p values <0.05). Methylobacterium were identified as significant indicators for biofilm surface communities, while Bradyrhizobium were identified as indicators of pipe surface and under tubercle communities. Phreatobacter and Ralstonia genera were identified as indicators of communities in samples collected from pipe surfaces, biofilm surfaces, and under tubercles. Several of the identified indicator organisms in our study have been reported in previous studies analyzing cast iron biofilm communities.68,83,104
3.5 Relationships between sample location, resistance genes, and microbial community composition
Microbial communities and abundances of resistance genes were compared between sample locations to determine potential host bacteria harboring ARGs, MRGs, and intI1.105 Additionally, the relationships among bacterial community composition and different sample types were evaluated using CCA. Biofilm sample type significantly influenced microbial community composition and explained 32% of the community variability (CCA, p = 0.009). Biofilm samples were taken from 12, 18, and 24 in. inside of the water main to assess impact of lateral sampling distance. CCA indicated that sampling distance into the pipe explained 36% of the variability in bacterial community composition (p = 0.001). These results indicate that biofilm sample location was a significant variable influencing microbial communities.
Spearman correlation analysis was conducted to determine relationships between bacterial community composition and abundance of resistance genes. Significant correlations were observed between several microbial genera and the abundance of resistance genes in the biofilm samples (Fig. 5). The occurrence of czcD was significantly correlated with genera including Desulfovibrio, Ferritrophicum, Herbaspirillum, and Rhodoferax (all p values <0.05). Positive relationships were also observed between czcD and corrosion-related genera such as Geothrix, Gallionella, Sphingomonas, and Undibacterium.
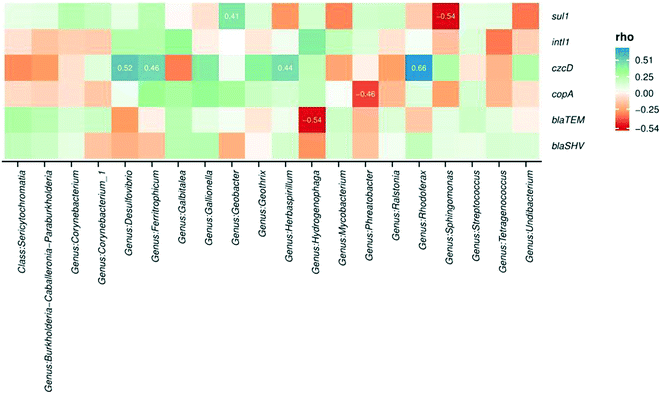 |
| Fig. 5 Relationships between relative abundance of most abundant taxa and absolute abundance of ARGs, MRGs, and intI1 in biofilm samples from cast iron water main. Color denotes the result from correlation analysis using Spearman's rank sum correlation with Spearman's rho value plotted for each comparison. The rho value for statistically significant relationships are also included (p values <0.05). The lowest available taxonomic classification for each observed ASV is provided. | |
The copper resistance gene copA was positively associated with the occurrence of several genera including Galbitalea, Gallionella, Geobacter, Geothrix, and Ferritrophicum. A significant negative relationship was observed between copA and Phreatobacter. β-Lactam resistance genes blaTEM and blaSHV were positively correlated with taxa including Burkholderia, Galbitalea, and Mycobacterium. A significant negative relationship was observed between blaTEM and the genus Hydrogenophaga (p < 0.05). The integrase gene of class 1 integrons (intI1) was positively correlated with genera including Desulfovibrio, Galbitalea, Hydrogenophaga, and Mycobacterium. The sulfonamide resistance gene sul1 demonstrated a significant positive relationship with the Geobacter genus.106 The presence of statistically significant correlations between microbial taxa and resistance genes in the biofilm samples implies that at least some of the shifts observed in gene abundance could have resulted from shifts in abundances or types of host bacteria.34
4. Conclusions
This research established that drinking water main biofilms in a chloraminated cast iron water main can serve as sources of resistance genes, regardless of sample type or location within the pipe. This is the first research to use ddPCR and qPCR to quantify ARGs, MRGs, and class 1 integrons in drinking water biofilms from a full-scale distribution system. Future research should be conducted to determine the distribution and concentrations of resistance genes in different pipe materials and in different locations of the same distribution system. Additional research is needed to quantify resistance genes and microbial communities in other pipe materials including copper, lead, and plastic that are commonly used in full-scale DWDS to understand how engineering management decisions can impact sources of antibiotic resistance.24 Further, full-scale and laboratory scale studies should be conducted to determine the impacts of corrosion inhibitors and corrosion products on the prevalence of antibiotic resistance in DWDS. Previous studies have suggested that drinking water biofilms may facilitate horizontal transfer of the ARGs from one host to another due to the presence of nutrients and high bacterial density and diversity.8 In the current study, genera containing opportunistic pathogens detected in the biofilm samples included Mycobacterium, Ralstonia, Staphylococcus, and Sphingomonas. Further research targeting these specific bacterial genera would be necessary to determine the presence of any potential opportunistic pathogens. Human exposure routes relevant for potable water include consumption of tap drinking water, dermal contact, and inhalation of aerosolized drinking water during showering or bathing.16 Studies documenting the occurrence and distribution of ARGs and MRGs in DWDS are critical for human health risk assessments evaluating the potential for the transfer and development of antibiotic resistance in engineered systems and in the environment.105,107 Given the potential for bacterial growth in DWDS, it is essential to continue to seek water treatment and management options that minimize levels of antibiotic resistance. Specific conclusions from key findings are as follows:
• ARGs (blaTEM, blaSHV, sul1), MRGs (copA, czcD), and/or class 1 integrons (intI1) were detected in every biofilm sample type studied within a chloraminated cast iron drinking water main, indicating that pipes could serve as sources for ARGs.
• ddPCR assays resulted in more positive detections and lower detection limits for target genes compared to qPCR assays. Future studies should consider ddPCR for environmental samples containing inhibiting substances such as metals, humic acids, and other contaminants.
• Microbial communities varied between different biofilm sample locations and were dominated by corrosion-related genera including Mycobacterium, Geobacter, Gallionella, and Sphingomonas.
• Significant relationships were observed for the co-occurrence of ARGs, MRGs, intI1 and several microbial taxa.
• Further research is warranted to determine the impacts of different pipe materials on the abundance of ARGs, MRGs, and intI1 in biofilms inhabiting full-scale DWDS.
Conflicts of interest
There are no conflicts to declare.
Acknowledgements
This material is based upon work supported by the National Science Foundation under Grant Numbers 2027288 and 2027233. Any opinions, findings, and conclusions or recommendations expressed in this material are those of the author(s) and do not necessarily reflect the views of the National Science Foundation. We would like to thank Dr. Walter McDonald for his assistance with transporting the pipe to the lab. We also thank Mike Dollhopf and the Marquette University (MU) Water Quality Center for analysis of metals using the ICP-MS. L. K. K. was supported by an Arthur J. Schmitt Fellowship from Marquette University. E. L. M. was supported by a student research fellowship from the Milwaukee Metropolitan Sewerage District. This research was supported by a Regular Research Grant from the MU Office of Innovation for ddPCR equipment and supplies. The Way Klinger Young Scholar Sabbatical Award supported P. J. M. and sequencing costs. We acknowledge the use of XRD at the Advanced Analysis Facility at the University of Wisconsin-Milwaukee. The authors acknowledge the use of the LightCycler 96 for qPCR from Marquette University, funded by the GHR Foundation.
References
- S. B. Levy, Antibiotic Resistance: Consequences of Inaction, Clin. Infect. Dis., 2001, 33(s3), S124–S129, DOI:10.1086/321837.
- A. Pruden, R. Pei, H. Storteboom and K. H. Carlson, Antibiotic resistance genes as emerging contaminants: Studies in northern Colorado, Environ. Sci. Technol., 2006, 40(23), 7445–7450 CrossRef CAS.
-
CDC, Antibiotic resistance threats in the United States, Centers Dis Control Prev, 2019, p. 114 Search PubMed.
- J. W. Beaber, B. Hochhut and M. K. Waldor, SOS response promotes horizontal dissemination of antibiotic resistance genes, Nature, 2004, 427(6969), 72–74 CrossRef CAS.
- M. A. Kohanski, D. J. Dwyer, B. Hayete, C. A. Lawrence and J. J. Collins, A Common Mechanism of Cellular Death Induced by Bactericidal Antibiotics, Cell, 2007, 130(5), 797–810 CrossRef CAS.
- A. A. Salyers, A. Gupta and Y. Wang, Human intestinal bacteria as reservoirs for antibiotic resistance genes, Trends Microbiol., 2004, 12(9), 412–416 CrossRef CAS.
- D. Bor and P. Epstein, Pathogenesis of respiratory infection in the disadvantaged, Semin. Respir. Infect., 1991, 6(4), 194–203 CAS.
- X. X. Zhang, T. Zhang and H. H. P. Fang, Antibiotic resistance genes in water environment, Appl. Microbiol. Biotechnol., 2009, 82(3), 397–414 CrossRef CAS.
- A. D. Kappell, M. S. De Nies, N. H. Ahuja, N. A. Ledeboer, R. J. Newton and K. R. Hristova, Detection of multi-drug resistant Escherichia coli in the urban waterways of Milwaukee, WI, Front. Microbiol., 2015, 6, 1–12 Search PubMed.
- C. Xi, Y. Zhang, C. F. Marrs, W. Ye, C. Simon and B. Foxman,
et al., Prevalence of antibiotic resistance in drinking water treatment and distribution systems, Appl. Environ. Microbiol., 2009, 75(17), 5714–5718 CrossRef CAS.
- L. Xu, W. Ouyang, Y. Qian, C. Su, J. Su and H. Chen, High-throughput profiling of antibiotic resistance genes in drinking water treatment plants and distribution systems, Environ. Pollut., 2016, 213, 119–126 CrossRef CAS.
- H. C. Su, Y. S. Liu, C. G. Pan, J. Chen, L. Y. He and G. G. Ying, Persistence of antibiotic resistance genes and bacterial community changes in drinking water treatment system: From drinking water source to tap water, Sci. Total Environ., 2018, 616–617, 453–461, DOI:10.1016/j.scitotenv.2017.10.318.
- S. Bergeron, R. Boopathy, R. Nathaniel, A. Corbin and G. LaFleur, Presence of antibiotic resistant bacteria and antibiotic resistance genes in raw source water and treated drinking water, Int. Biodeterior. Biodegrad., 2015, 102, 370–374, DOI:10.1016/j.ibiod.2015.04.017.
- E. Garner, M. Inyang, E. Garvey, J. Parks, C. Glover and E. Dickenson,
et al. Impact of blending for direct potable reuse on premise plumbing microbial ecology and regrowth of opportunistic pathogens and antibiotic resistant bacteria, Water Res., 2019, 151, 75–86, DOI:10.1016/j.watres.2018.12.003.
- WHO, Antimicrobial resistance, Global Report on Surveillance, Bull. W. H. O., 2014, 61(3), 383–394 Search PubMed , Available from: http://www.ncbi.nlm.nih.gov/pubmed/22247201%5Cnhttp://www.pubmedcentral.nih.gov/articlerender.fcgi?artid=2536104&tool=pmcentrez&rendertype=abstract.
- E. J. Anaissie, S. R. Penzak and M. C. Dignani, The hospital water supply as a source of nosocomial infections: a plea for action, Arch. Intern. Med., 2002, 162(13), 1483–1492 CrossRef.
- A. Pruden, R. E. Alcalde, A. PJJ, N. Ashbolt, H. Bischel and N. L. Capiro,
et al. An Environmental Science and Engineering Framework for Combating Antimicrobial Resistance, Environ. Eng. Sci., 2018, 35(10), ees.2017.0520, DOI:10.1089/ees.2017.0520.
- Y. Zhang, A. Z. Gu, M. He, D. Li and J. Chen, Subinhibitory Concentrations of Disinfectants Promote the Horizontal Transfer of Multidrug Resistance Genes within and across Genera, Environ. Sci. Technol., 2017, 51(1), 570–580 CrossRef CAS.
- S. Jia, P. Shi, Q. Hu, B. Li, T. Zhang and X. X. Zhang, Bacterial Community Shift Drives Antibiotic Resistance Promotion during Drinking Water Chlorination, Environ. Sci. Technol., 2015, 49(20), 12271–12279 CrossRef CAS.
- C. R. Proctor, M. Gächter, S. Kötzsch, F. Rölli, R. Sigrist and J. C. Walser,
et al. Biofilms in shower hoses-choice of pipe material influences bacterial growth and communities, Environ. Sci.: Water Res. Technol., 2016, 2(4), 670–682 RSC.
- S. Aggarwal, C. K. Gomez-Smith, Y. Jeon, T. M. Lapara, M. B. Waak and R. M. Hozalski, Effects of Chloramine and Coupon Material on Biofilm Abundance and Community Composition in Bench-Scale Simulated Water Distribution Systems and Comparison with Full-Scale Water Mains, Environ. Sci. Technol., 2018, 52(22), 13077–13088 CrossRef CAS.
- I. Douterelo, B. E. Dutilh, K. Arkhipova, C. Calero and S. Husband, Microbial diversity, ecological networks and functional traits associated to materials used in drinking water distribution systems, Water Res., 2020, 173, 115586, DOI:10.1016/j.watres.2020.115586.
- M. C. Cruz, Y. Woo, H. Flemming and S. Wuertz, Nitrifying niche differentiation in biofilms from full-scale chloraminated drinking water distribution system, Water Res., 2020, 115738, DOI:10.1016/j.watres.2020.115738.
- L. K. Kimbell, Y. Wang and P. J. McNamara, The impact of metal pipe materials, corrosion products, and corrosion inhibitors on antibiotic resistance in drinking water distribution systems, Appl. Microbiol. Biotechnol., 2020, 7673–7688 CrossRef CAS.
- D. A. Lytle, T. J. Sorg and C. Frietch, Accumulation of arsenic in drinking water distribution systems, Environ. Sci. Technol., 2004, 38(20), 5365–5372 CrossRef CAS.
- C. Y. Peng and G. V. Korshin, Speciation of trace inorganic contaminants in corrosion scales and deposits formed in drinking water distribution systems, Water Res., 2011, 45(17), 5553–5563, DOI:10.1016/j.watres.2011.08.017.
- C. Y. Peng, A. S. Hill, M. J. Friedman, R. L. Valentine, G. S. Larson and A. M. Y. Romero,
et al. Occurrence of trace inorganic contaminants in drinking water distribution systems, J. - Am. Water Works Assoc., 2012, 104(3), 53–54 CrossRef CAS.
- M. R. Schock, R. N. Hyland and M. M. Welch, Occurrence of contaminant accumulation in lead pipe scales from domestic drinking-water distribution systems, Environ. Sci. Technol., 2008, 42(12), 4285–4291 CrossRef CAS.
- Y. Zhang, A. Z. Gu, T. Cen, X. Li, M. He and D. Li,
et al. Sub-inhibitory concentrations of heavy metals facilitate the horizontal transfer of plasmid-mediated antibiotic resistance genes in water environment, Environ. Pollut., 2018, 237, 74–82, DOI:10.1016/j.envpol.2018.01.032.
- C. Baker-Austin, M. S. Wright, R. Stepanauskas and J. V. McArthur, Co-selection of antibiotic and metal resistance, Trends Microbiol., 2006, 14, 176–182 CrossRef CAS.
- C. Pal, K. Asiani, S. Arya, C. Rensing, D. J. Stekel and D. G. J. Larsson,
et al. Metal Resistance and Its Association With Antibiotic Resistance, Adv. Microb. Physiol., 2017, 70, 261–313 CrossRef CAS.
- K. Poole, At the Nexus of Antibiotics and Metals: The Impact of Cu and Zn on Antibiotic Activity and Resistance, Trends Microbiol., 2017, 25(10), 820–832, DOI:10.1016/j.tim.2017.04.010.
- J. O. N. J. Calomiris, J. L. Armstrong and R. J. Seidler, Association of Metal Tolerance with Multiple Antibiotic Resistance of Bacteria Isolated from Drinking Water, Appl. Environ. Microbiol., 1984, 47(6), 1238–1242 CrossRef CAS.
- E. Garner, C. Chen, K. Xia, J. Bowers, D. Engelthaler and J. McLain,
et al. Metagenomic characterization of antibiotic resistance genes in full-scale reclaimed water distribution systems and corresponding potable systems, Environ. Sci. Technol., 2018, acs.est.7b05419, DOI:10.1021/acs.est.7b05419.
- H. Hao, D. Y. Shi, D. Yang, Z. W. Yang, Z. G. Qiu and W. L. Liu,
et al. Profiling of intracellular and extracellular antibiotic resistance genes in tap water, J. Hazard. Mater., 2019, 365, 340–345, DOI:10.1016/j.jhazmat.2018.11.004.
- E. Garner, J. Mclain, J. Bowers, D. M. Engelthaler, M. A. Edwards and A. Pruden, Microbial Ecology and Water Chemistry Impact Regrowth of Opportunistic Pathogens in Full-Scale Reclaimed Water Distribution Systems, Environ. Sci. Technol., 2018, 52(16), 9056–9068, DOI:10.1021/acs.est.8b02818.
- G. W. Li, E. Oh and J. S. Weissman, The anti-Shine-Dalgarno sequence drives translational pausing and codon choice in bacteria, Nature, 2012, 484(7395), 538–541, DOI:10.1038/nature10965.
- L. K. Kimbell, A. D. Kappell and P. J. McNamara, Effect of pyrolysis on the removal of antibiotic resistance genes and class I integrons from municipal wastewater biosolids, Environ. Sci.: Water Res. Technol., 2018, 4, 1807–1818 RSC , Available from: http://xlink.rsc.org/?DOI=C8EW00141C.
- A. D. Kappell, L. K. Kimbell, M. D. Seib, D. E. Carey, M. J. Choi and T. Kalayil,
et al., Removal of antibiotic resistance genes in an anaerobic membrane bioreactor treating primary clarifier effluent at 20 °C, Environ. Sci.: Water Res. Technol., 2018, 4(11), 1783–1793 RSC , Available from: http://xlink.rsc.org/?DOI=C8EW00270C.
- K. R. Harrison, A. D. Kappell and P. J. McNamara, Benzalkonium chloride alters phenotypic and genotypic antibiotic resistance profiles in a source water used for drinking water treatment, Environ. Pollut., 2020, 257, 113472, DOI:10.1016/j.envpol.2019.113472.
- A. Di Cesare, S. Petrin, D. Fontaneto, C. Losasso, E. M. Eckert and G. Tassistro,
et al. ddPCR applied on archived Continuous Plankton Recorder samples reveals long-term occurrence of class 1 integrons and a sulphonamide resistance gene in marine plankton communities, Environ. Microbiol. Rep., 2018, 10(4), 458–464 CrossRef CAS.
- S. A. Bustin, V. Benes, J. A. Garson, J. Hellemans, J. Huggett and M. Kubista,
et al., The MIQE guidelines: Minimum information for publication of quantitative real-time PCR experiments, Clin. Chem., 2009, 55(4), 611–622 CrossRef CAS.
- J. F. Huggett, C. A. Foy, V. Benes, K. Emslie, J. A. Garson and R. Haynes,
et al. The digital MIQE guidelines: Minimum information for publication of quantitative digital PCR experiments, Clin. Chem., 2013, 59(6), 892–902 CrossRef CAS.
- G. Muyzer, E. Waal and A. G. Uitierlinden, Profiling of complex microbial populations by denaturing gradient gel electrophoresis analysis of polymerase chain reaction-amplified genes coding for 16S rRNA, Appl. Environ. Microbiol., 1993, 59(3), 695–700 CrossRef CAS , Available from: http://aem.asm.org/content/59/3/695.short.
- E. Marti, J. Jofre and J. L. Balcazar, Prevalence of Antibiotic Resistance Genes and Bacterial Community Composition in a River Influenced by a Wastewater Treatment Plant, PLoS One, 2013, 8(10), e78906, DOI:10.1371/journal.pone.0078906.
- T. R. Burch, M. J. Sadowsky and T. M. LaPara, Air-drying beds eliminate antibiotic resistance genes and class 1 integrons in residual municipal wastewater solids, Environ. Sci. Technol., 2013, 47, 9965–9971 CrossRef CAS.
- S. Roosa, R. Wattiez, E. Prygiel, L. Lesven, G. Billon and D. C. Gillan, Bacterial metal resistance genes and metal bioavailability in contaminated sediments, Environ. Pollut., 2014, 189, 143–151, DOI:10.1016/j.envpol.2014.02.031.
- C. Goldstein, M. D. Lee, S. Sanchez, B. Phillips, B. Register and M. Grady,
et al. Incidence of Class 1 and 2 Integrases in Clinical and Commensal Bacteria from Livestock, Companion Animals, and Exotics, Antimicrob. Agents Chemother., 2001, 45(3), 723–726 CrossRef CAS.
- K. Bush and G. A. Jacoby, Updated functional classification of β-lactamases, Antimicrob. Agents Chemother., 2010, 54(3), 969–976 CrossRef CAS.
- A. Pruden, M. Arabi and H. N. Storteboom, Correlation between upstream human activities and riverine antibiotic resistance genes, Environ. Sci. Technol., 2012, 46(21), 11541–11549 CrossRef CAS.
- M. R. Gillings, W. H. Gaze, A. Pruden, K. Smalla, J. M. Tiedje and Y. G. Zhu, Using the class 1 integron-integrase gene as a proxy for anthropogenic pollution, ISME J., 2015, 9(6), 1269–1279, DOI:10.1038/ismej.2014.226.
- T. G. Kloosterman, M. M. Van Der Kooi-Pol, J. J. E. Bijlsma and O. P. Kuipers, The novel transcriptional regulator SczA mediates protection against Zn2+ stress by activation of the Zn2+−resistance gene czcD in Streptococcus pneumoniae, Mol. Microbiol., 2007, 65(4), 1049–1063 CrossRef CAS.
- F. W. Outten, D. L. Huffman, J. A. Hale and T. V. O'Halloran, The Independent cue and cus Systems Confer Copper Tolerance during Aerobic and Anaerobic Growth in Escherichia coli, J. Biol. Chem., 2001, 276(33), 30670–30677 CrossRef CAS.
- J. G. Caporaso, C. L. Lauber, W. A. Walters, D. Berg-Lyons, J. Huntley and N. Fierer,
et al. Ultra-high-throughput microbial community analysis on the Illumina HiSeq and MiSeq platforms, ISME J., 2012, 6(8), 1621–1624, DOI:10.1038/ismej.2012.8.
- M. Martin, Cutadapt removes adapter sequences from high-throughput sequencing reads, EMBnet.journal, 2011, 17(1), 10–12 CrossRef.
- B. J. Callahan, P. J. McMurdie, M. J. Rosen, A. W. Han, A. J. A. Johnson and S. P. Holmes, DADA2: High-resolution sample inference from Illumina amplicon data, Nat. Methods, 2016, 13(7), 581–583 CrossRef CAS.
- C. Quast, E. Pruesse, P. Yilmaz, J. Gerken, T. Schweer and P. Yarza,
et al. The SILVA ribosomal RNA gene database project: Improved data processing and web-based tools, Nucleic Acids Res., 2013, 41(D1), 590–596 CrossRef.
- A. D. Kappell, K. R. Harrison and P. J. McNamara, Effects of zinc orthophosphate on the antibiotic resistant bacterial community of a source water used for drinking water treatment, Environ. Sci.: Water Res. Technol., 2019, 5(9), 1523–1534 RSC , Available from: http://xlink.rsc.org/?DOI=C9EW00374F.
-
American Public Health Association(APHA), Standard Methods for Examination of Water and Wastewater, 20th edn, 1975 Search PubMed.
-
R. C. Team, R: A language and environment for statistical computing, R Foundation for Statistical Computing, Vienna, Austria, 2018, Available from: https://www.r-project.org/ Search PubMed.
- J. S. Racine, RStudio: A Platform-Independent IDE for R and Sweave, J. Appl. Econom., 2012, 27, 167–172 CrossRef.
- P. J. McMurdie and S. Holmes, Phyloseq: An R Package for Reproducible Interactive Analysis and Graphics of Microbiome Census Data, PLoS One, 2013, 8(4), e61217 CrossRef CAS.
- M. Dufrêne and P. Legendre, Species assemblages and indicator species: The need for a flexible asymmetrical approach, Ecol. Monogr., 1997, 67(3), 345–366 Search PubMed.
-
M. Mariadassou, Phyloseq-extended, 2017, Available from: https://github.com/mahendra-mariadassou/phyloseq-extended Search PubMed.
- H. Wang, C. Hu, S. Zhang, L. Liu and X. Xing, Effects of O3/Cl2disinfection on corrosion and opportunistic pathogens growth in drinking water distribution systems, J. Environ. Sci., 2018, 1–9, DOI:10.1016/j.jes.2018.01.009.
- H. Wang, C. Hu, X. Hu, M. Yang and J. Qu, Effects of disinfectant and biofilm on the corrosion of cast iron pipes in a reclaimed water distribution system, Water Res., 2012, 46(4), 1070–1078, DOI:10.1016/j.watres.2011.12.001.
- H. Wang, C. Hu, L. Zhang, X. Li, Y. Zhang and M. Yang, Effects of microbial redox cycling of iron on cast iron pipe corrosion in drinking water
distribution systems, Water Res., 2014, 65, 362–370, DOI:10.1016/j.watres.2014.07.042.
- Y. Zhu, H. Wang, X. Li, C. Hu, M. Yang and J. Qu, Characterization of biofilm and corrosion of cast iron pipes in drinking water distribution system with UV/Cl2disinfection, Water Res., 2014, 60, 174–181, DOI:10.1016/j.watres.2014.04.035.
- H. Wang, C. Hu, L. Han and M. Yang, Effects of microbial cycling of Fe(II)/Fe(III) and Fe/N on cast iron corrosion in simulated drinking water distribution systems, Corros. Sci., 2015, 100, 599–606, DOI:10.1016/j.corsci.2015.08.037.
- L. Chen, J. Rui-Bao and L. Li, Bacterial community of iron tubercles from a drinking water distribution system and its occurrence in stagnant tap water, Environ. Sci.: Processes Impacts, 2013, 15(7), 1332–1340 RSC.
- P. Sarin, V. L. Snoeyink, J. Bebee, W. M. Kriven and J. A. Clement, Physico-Chemical Characteristics of Corrosion Scales in Old Iron Pipes, Water Res., 2001, 35(12), 2961–2969 CrossRef CAS.
- F. Yang, B. Shi, J. Gu, D. Wang and M. Yang, Morphological and physicochemical characteristics of iron corrosion scales formed under different water source histories in a drinking water distribution system, Water Res., 2012, 46(16), 5423–5433, DOI:10.1016/j.watres.2012.07.031.
- C. K. Gomez-Smith, T. M. Lapara and R. M. Hozalski, Sulfate reducing bacteria and mycobacteria dominate the biofilm communities in a chloraminated drinking water distribution system, Environ. Sci. Technol., 2015, 49(14), 8432–8440 CrossRef CAS.
- M. Waak, T. M. Lapara, C. Halle and R. M. Hozalski, Occurrence of Legionella spp . in Water-Main Biofilms from Two Drinking Water Distribution Systems, Environ. Sci. Technol., 2018, 52, 7630–7639 CrossRef CAS.
- M. B. Waak, R. M. Hozalski, C. Hallé and T. M. Lapara, Comparison of the microbiomes of two drinking water distribution systems - With and without residual chloramine disinfection, Microbiome, 2019, 7(1), 1–14 CrossRef.
- L. Cavé, E. Brothier, D. Abrouk, P. S. Bouda, E. Hien and S. Nazaret, Efficiency and sensitivity of the digital droplet PCR for the quantification of antibiotic resistance genes in soils and organic residues, Appl. Microbiol. Biotechnol., 2016, 100(24), 10597–10608, DOI:10.1007/s00253-016-7950-5.
- S. C. Taylor, G. Laperriere and H. Germain, Droplet Digital PCR versus qPCR for gene expression analysis with low abundant targets: From variable nonsense to publication quality data, Sci. Rep., 2017, 7(1), 1–8 CrossRef CAS.
- B. Verhaegen, K. De Reu, L. De Zutter, K. Verstraete, M. Heyndrickx and E. Van Coillie, Comparison of droplet digital PCR and qPCR for the quantification of shiga toxin-producing Escherichia coli in bovine feces, Toxins, 2016, 8(5), 1–11 CrossRef.
- J. L. Balcázar, J. Subirats and C. M. Borrego, The role of biofilms as environmental reservoirs of antibiotic resistance, Front Microbiol., 2015, 6, 1–9 Search PubMed.
- M. J. Lehtola, M. Laxander, I. T. Miettinen, A. Hirvonen, T. Vartiainen and P. J. Martikainen, The effects of changing water flow velocity on the formation of biofilms and water quality in pilot distribution system consisting of copper or polyethylene pipes, Water Res., 2006, 40(11), 2151–2160 CrossRef CAS.
- Y. Yin, J. Gu, X. Wang, W. Song, K. Zhang and W. Sun,
et al. Effects of copper addition on copper resistance, antibiotic resistance genes, and intl1 during swine manure composting, Front Microbiol., 2017, 8, 1–10 Search PubMed.
- M. B. Waak, T. M. Lapara, C. Halle and R. M. Hozalski, Non-tuberculous mycobacteria in two drinking water distribution systems and the role of residual disinfection, Environ. Sci. Technol., 2019, 53(15), 8563–8573 CrossRef CAS.
- H. Sun, B. Shi, D. A. Lytle, Y. Bai and D. Wang, Formation and release behavior of iron corrosion products under the influence of bacterial communities in a simulated water distribution system, Environ. Sci.: Processes Impacts, 2014, 16(3), 576–585 RSC.
- H. T. Wu, Z. L. Mi, J. X. Zhang, C. Chen and S. G. Xie, Bacterial communities associated with an occurrence of colored water in an urban drinking water distribution system, Biomed. Environ. Sci., 2014, 27(8), 646–650 Search PubMed , Available from: http://www.ncbi.nlm.nih.gov/pubmed/25189613.
- E. Torvinen, M. J. Lehtola, I. T. Miettinen, O. Zacheus, S. Suomalainen and L. Paulin,
et al. Mycobacteria in Water and Loose Deposits of Drinking Water Distribution Systems in Finland, Appl. Environ. Microbiol., 2004, 70(4), 1973–1981 CrossRef CAS.
- M. Sevillano, Z. Dai, S. Calus, Q. M. Bautista-de los Santos, A. M. Eren and P. W. J. J. van der Wielen,
et al. Differential prevalence and host-association of antimicrobial resistance traits in disinfected and non-disinfected drinking water systems, Sci. Total Environ., 2020, 749, 141451, DOI:10.1016/j.scitotenv.2020.141451.
- Q. M. Bautista-De los Santos, J. L. Schroeder, M. C. Sevillano-Rivera, R. Sungthong, U. Z. Ijaz and W. T. Sloan,
et al., Emerging investigators series: Microbial communities in full-scale drinking water distribution systems-a meta-analysis, Environ. Sci.: Water Res. Technol., 2016, 2(4), 631–644 RSC.
- W. Lin, Z. Yu, H. Zhang and I. P. Thompson, Diversity and dynamics of microbial communities at each step of treatment plant for potable water generation, Water Res., 2014, 52, 218–230, DOI:10.1016/j.watres.2013.10.071.
- L. Hall-Stoodley, C. W. Keevil and H. M. Lappin-Scott, Biofilm Formation Under High and Low Nutrient Conditions, J. Appl. Microbiol., 1999, 85, 60–69 CrossRef.
- T. P. Primm, C. A. Lucero and J. O. Falkinham III, Health Impacts of Environmental Mycobacteria, Clin. Microbiol. Rev., 2004, 17(1), 98–106 CrossRef.
- K. Dowdell, S. J. Haig, L. J. Caverly, Y. Shen, J. J. LiPuma and L. Raskin, Nontuberculous mycobacteria in drinking water systems– the challenges of characterization and risk mitigation, Curr. Opin. Biotechnol., 2019, 57, 127–136, DOI:10.1016/j.copbio.2019.03.010.
- S.-J. Haig, N. Kotlarz, J. J. LiPuma and L. Raskin, A High-Throughput Approach for Identification of Nontuberculous Mycobacteria in Drinking Water Reveals Relationship between Water Age and Mycobacterium avium, MBio, 2018, 9(1), 1–13 CrossRef.
- B. A. Brown-Elliott, R. J. Wallace, C. Tichindelean, J. C. Sarria, S. McNulty and R. Vasireddy,
et al., Five-year outbreak of community- and hospital-acquired Mycobacterium porcinum infections related to public water supplies, J. Clin. Microbiol., 2011, 49(12), 4231–4238 CrossRef CAS.
- X. Li, H. Wang, Y. Zhang, C. Hu and M. Yang, Characterization of the bacterial communities and iron corrosion scales in drinking groundwater distribution systems with chlorine/chloramine, Int. Biodeterior. Biodegrad., 2014, 96, 71–79, DOI:10.1016/j.ibiod.2014.09.014.
- H. Sun, B. Shi, Y. Bai and D. Wang, Bacterial community of biofilms developed under different water supply conditions in a distribution system, Sci. Total Environ., 2014, 472, 99–107, DOI:10.1016/j.scitotenv.2013.11.017.
- D. Li, Z. Li, J. Yu, N. Cao, R. Liu and M. Yang, Characterization of bacterial community structure in a drinking water distribution system during an occurrence of red water, Appl. Environ. Microbiol., 2010, 76(21), 7171–7180 CrossRef CAS.
- D. Berry, C. Xi and L. Raskin, Microbial ecology of drinking water distribution systems, Curr. Opin. Biotechnol., 2006, 17(3), 297–302 CrossRef CAS.
- G. Liu, G. L. Bakker, S. Li, J. H. G. Vreeburg, J. Q. J. C. Verberk and G. J. Medema,
et al. Pyrosequencing reveals bacterial communities in unchlorinated drinking water distribution system:
An integral study of bulk water, suspended solids, loose deposits, and pipe wall biofilm, Environ. Sci. Technol., 2014, 48(10), 5467–5476 CrossRef CAS.
- G. Liu, Y. Zhang, E. van der Mark, A. Magic-Knezev, A. Pinto and B. van den Bogert,
et al. Assessing the origin of bacteria in tap water and distribution system in an unchlorinated drinking water system by SourceTracker using microbial community fingerprints, Water Res., 2018, 138, 86–96, DOI:10.1016/j.watres.2018.03.043.
- G. Liu, Y. Zhang, X. Liu, F. Hammes, W. T. Liu and G. Medema,
et al. 360-Degree Distribution of Biofilm Quantity and Community in an Operational Unchlorinated Drinking Water Distribution Pipe, Environ. Sci. Technol., 2020, 54(9), 5619–5628 CrossRef CAS.
- M. P. Ryan and C. C. Adley, Ralstonia spp.: Emerging global opportunistic pathogens, Eur. J. Clin. Microbiol. Infect. Dis., 2014, 33(3), 291–304 CrossRef CAS.
- T. H. Chiao, T. M. Clancy, A. Pinto, C. Xi and L. Raskin, Differential resistance of drinking water bacterial populations to monochloramine disinfection, Environ. Sci. Technol., 2014, 48(7), 4038–4047 CrossRef CAS.
- C. Volk, E. Dundore, J. Schiermann and M. Lechevallier, Practical evaluation of iron corrosion control in a drinking water distribution system, Water Res., 2000, 34(6), 1967–1974 CrossRef CAS.
- X. Ma, G. Zhang, G. Li, Y. Wan, H. Sun and H. Wang,
et al. Biofilm bacterial community transition under water supply quality changes in drinking water distribution systems, Environ. Sci.: Water Res. Technol., 2018, 4(5), 644–653 RSC.
- E. W. Rice, P. Wang, A. L. Smith and L. B. Stadler, Determining Hosts of Antibiotic Resistance Genes: A Review of Methodological Advances, Environ. Sci. Technol. Lett., 2020, 7(5), 282–291 CrossRef CAS.
- H. Wang, C. Hu and X. Hu, Effects of combined UV and chlorine disinfection on corrosion and water quality within reclaimed water distribution systems, Eng. Failure Anal., 2014, 39, 12–20, DOI:10.1016/j.engfailanal.2014.01.009.
- N. J. Ashbolt, A. Amèzquita, T. Backhaus, P. Borriello, K. K. Brandt and P. Collignon,
et al. Human health risk assessment(HHRA) for environmental development and transfer of antibiotic resistance, Environ. Health Perspect., 2013, 121(9), 993–1001 CrossRef.
Footnotes |
† Electronic supplementary information (ESI) available. See DOI: 10.1039/d0ew01059f |
‡ Current affiliation: Signature Science, Austin, TX, USA. |
|
This journal is © The Royal Society of Chemistry 2021 |