Effect of deposition, detachment and aggregation processes on nanoparticle transport in porous media using Monte Carlo simulations†
Received
11th January 2021
, Accepted 4th June 2021
First published on 8th June 2021
Abstract
A novel off-lattice three-dimensional coarse-grained Monte Carlo model is developed to study engineered nanoparticle (ENP) behavior in porous media. Based on individual particle tracking and on the assumption that different physicochemical processes may occur with different probabilities, our model is used to independently evaluate the influence of homoaggregation, attachment and detachment processes on ENP transport and retention inside porous media made of colloidal collectors. The possibility of straining, i.e. trapping of ENPs or aggregates that are too large to pass pore necks, is also included in the model. The overall probability of ENP retention as a function of the above mentioned processes is quantified using functional tests in the form of a αglobal(tref) retention parameter. High αglobal(tref) values were obtained for moderate probabilities of homoaggregation between ENPs (αENP–ENP) and very small probabilities of attachment between ENPs and collectors (αatt), thus indicating the important role of homoaggregation and attachment in ENP retention. Moreover, attaching ENPs and large aggregates was found to cause pore neck enclosure and thus largely contributed to the straining of unbound ENPs. An analysis of depth distribution of retained ENPs revealed that, depending on the dominating conditions, the number of ENPs was decreasing monotonously or exponentially with depth. The introduction of the ENP detachment probability (αdet) from collectors resulted in an increased ENP occurrence at the porous media matrix outlet. It was also found that different sets of αdet and αatt values, reflecting different ENPs and collector physicochemical properties and inter-particle forces, lead to identical αglobal(tref) values. This constitutes an important outcome indicating that αglobal(tref) values determined from functional tests are not mechanistic but operationally defined parameters and thus cannot be deemed predictive beyond these tests.
Environmental significance
Parameters describing the fate and transport of nano-objects (e.g. natural and engineered nanoparticles, nanoplastics, viruses) in natural porous media are required for appropriate exposure predictions during risk assessments, but also for predicting the efficiency of terrestrial nanotechnology such as nanozerovalent iron for soil remediation, nanopesticides and nanofertilizers. In this study, nanoparticle transport in modelled porous media is described by the experimentally accessible αglobal parameter. Its variation is investigated as a function of the key porous media characteristic – porosity – and commonly occurring processes such as deposition, homoaggregation and detachment. Our results allow a better understanding of the mechanisms controlling nanoparticle transport in porous media and demonstrate the relative importance of homoaggregation, detachment and straining processes on the retention profiles.
|
1. Introduction
Engineered nanoparticles (ENPs) are typically defined as unbound or aggregated particles with at least one external dimension less than 100 nm.1 Demonstrating unique physicochemical properties, they have rapidly found many applications in automotive, pharmaceutical, textile and food processing industries.2,3 Unfortunately, the growing production, manufacture and use of numerous ENP-containing products are inevitably resulting in their higher emissions into the environment and porous media, such as natural soils, are particularly exposed.4
Natural soils may be exposed to ENP through the use of (nano)fertilizers and nanopesticides,5,6 remediation of contaminated zones,7 application of sewage sludge for agricultural purposes,8 landfill disposal,9 influx of surface water runoff10 or accidental spills.11 It should be noted that not only ENPs can be introduced into soils in such a way but also other nanoscale particles such as nanoplastics and viruses.12,13 The fate and transport of ENPs in porous media will strongly depend on different physicochemical processes, such as aggregation (homo- and heteroaggregation), deposition, straining and sedimentation, but also on intrinsic ENP properties (size, surface charge, coating) and the chemistry of the surrounding medium (pH, ionic strengths, presence of natural organic matter and nature of the collectors).14–19
Terrestrial nanotechnology applications, such as remediation by nano-scale zero-valent iron (nZVI) and crop protection by nanopesticides, are seen as promising solutions due to assumed ENP mobility in soils and high potential of reaching respective targets.5,20,21 However, limited mobility of, for example, nZVI particles was observed due to their homoaggregation or heteroaggregation with carbonate minerals.21–23 Released ENPs are also expected to migrate between different environmental compartments and be present in surface water bodies, often used for drinking water production.24,25 Thus, the ENP transport to porous media systems used in drinking water treatment (or wastewater treatment) plants, such as micro- and nanomembranes, sand and activated carbon filters, is very probable. The removal or retention of ENPs from drinking water depends on the efficiency of different treatments including coagulation, flocculation, sedimentation and filtration. Recent studies showed that combination of coagulation, flocculation and sedimentation processes guarantees high but not complete removal of ENPs from drinking water.26–28 This underlines the importance of filtration processes and that the understanding of ENP behavior in porous media also needs careful examination.
In the above context, a thorough comprehension of the physicochemical processes at the micro and nanoscale levels controlling the fate and transport of ENPs in porous media is needed to evaluate retention times, develop ENP transport models, etc. The most frequently used ENP fate indicator in porous media is the attachment efficiency (α) also termed collision efficiency, deposition efficiency or sticking probability. This dimensionless parameter has been developed within the colloid filtration theory (CFT) framework to accurately estimate colloid deposition rates under environmentally relevant conditions. It is defined as the ratio of the number of collisions that succeed in producing adhesion to the total number of collisions occurring between colloids and collector surfaces.29 An analogous particle–particle attachment efficiency α is used to describe homoaggregation of ENPs and heteroaggregation between ENPs and suspended colloids.30 Reflecting average chemical characteristics of the system such as surface charges, pH and ionic strength, it is comprised between 1 (chemical–colloidal interactions favorable for deposition due to the lack of repulsion forces or presence of strong attractive forces) and 0 (chemical–colloidal interactions unfavorable for deposition due to the presence of strong repulsion forces).31 Attachment efficiency under unfavorable conditions can be predicted using theoretical models or semi-empirical equations.31–34 However, as calculating attachment efficiency from theory requires detailed characterization of both porous medium and dominating hydrodynamic conditions, it is very often assessed experimentally using laboratory column studies. Particles in the effluent concentration are measured as a function of time at the column outlet with a variety of techniques to obtain breakthrough curves (BTC). The attachment efficiency can then be calculated from the BTC using mathematical expressions presented by Tufenkji and Elimelech35 or Yao et al.29
An essential characteristic of the attachment efficiency, be it a calculated or experimentally determined one, is that it relies on a continuum model, i.e. the porous medium is considered chemically and hydrodynamically homogeneous. Moreover, deposition is often the only mechanism assumed to occur and is characterized by a single attachment efficiency even though it is generally acknowledged that porous media are seldom homogeneous and the effects of heterogeneity on accuracy of predictions based on a single attachment efficiency are poorly known. This heterogeneity comprises both variations in hydrodynamic, physical and chemical conditions,36,37 and also occurrence of processes other than deposition, such as homoaggregation, detachment and straining.15,38 Investigating the conditions under which the latter processes occur requires more complex continuum models that have several parameters to be fitted to the BTC of column tests in addition to the attachment efficiency. This approach is prone to singularity, i.e. many different explanations that are empirically equivalent may explain the BTC shapes, which in turn undermines the accuracy of more complex continuum models. Continuum models using the attachment efficiency as a single parameter are, despite their shortcomings, widely used, but mostly in research contexts that approach ideal conditions of a homogeneous medium and rarely to predict transport under highly complex field conditions.39
Particle-tracking models, i.e. models in which particles are explicitly tracked in a simulated porous medium can consider the heterogeneity under hydrodynamic and chemical conditions in porous media much better than continuum models. Mechanistic particle tracking models have been developed where the interaction energy of individual particles are calculated using a Derjaguin, Landau, Verwey and Overbeek (DLVO) approach and local hydrodynamic conditions are computed using computational fluid dynamics (CFD) (e.g. ref. 40). Particles thus interact with surfaces or other particles whenever their kinetic energy exceeds the local energy barrier. Such models are computationally heavy and/or can only simulate a relatively small volume of porous medium. In addition, CFD-DLVO models only consider one process at a time, e.g. deposition.40 Moreover, outcomes of DLVO calculations depend strongly on a range of conditions that are difficult to characterize in porous media such as the concentration and type of organic surface coatings, local roughness, and determination of Hamaker constants.
Hybrid models based on an Eulerian finite difference (FD) method41 and a Lagrangian (random-walk particle-tracking (RWPT)) approach42 can also be considered. These models track a large number of individual particles to describe the reactive transport of ENP dissolved species and capture ENP transport and aging.43 As the dissolution process proceeds, changes in the model particle size, mass and surface area are tracked and corresponding attachment, detachment and dissolution parameters are updated. Accounting for ENP capture and release as well as tracking of changes in ENP morphology make these models more advantageous than the continuum ones. However, as these models work only in one dimension, they do not allow certain parts of porous media (e.g. collectors) to be explicitly modeled and porous media characteristics (e.g. porosity) to be adjusted as well as they do not take into account specific phenomena such as homoaggregation and straining.
In this context, we develop, for the first time, a Monte Carlo approach to increase the understanding of how occurrence of different processes (homoaggregation, attachment, detachment and straining) and variations in porous media characteristics can affect the overall probability of ENP retention within three-dimensional (3D) porous media, here denoted as αglobal(tref). Our approach is based on the tracking of individual particles, but instead of using mechanistic DLVO and local hydrodynamics calculations, we made the assumption that different interactions between ENPs and colloidal collectors occur with different probabilities. These probabilities can be varied without specifying the exact chemical and hydrodynamic conditions and they do not apply only to attachment and detachment, as it was done before, but also to the homoaggregation process. Moreover, the possibility of straining of single particles or larger homoaggregates is also included in this model. Our study aims particularly to 1) model ENP transport through 3D porous media made of colloidal collectors based on a Monte Carlo approach, 2) independently evaluate the influence of porosity, homoaggregation, deposition, detachment and straining processes on BTC shapes and ENP porous media retention profiles (depth concentrations) and 3) calculate the overall probability of ENP retention (αglobal(tref)) as a function of the balance between ENP homoaggregation, deposition, detachment and straining processes.
2. Model
2.1 Model description
The simulation model is initially composed of two off-lattice three-dimensional coarse-grained model boxes, one representing a reservoir of a given number of mobile ENPs and a second one representing the fixed porous media matrix (Fig. 1). The ENP reservoir box is placed at the top of the porous media matrix. The ENPs are represented as rigid and impermeable spheres with an arbitrary diameter of 20 nm. As the ENP diameter is an input parameter, it can be adapted during future simulations. The ENPs are initially randomly placed in a cubic and periodic simulation box with an (x, y, z) size of 2000 nm with respect to the excluded volume criteria, i.e. without any overlap, and with a volume concentration equal to 1%.
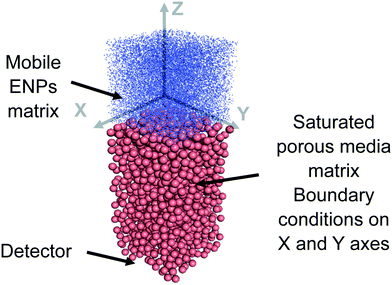 |
| Fig. 1 Simulation model composed of mobile ENPs, a saturated porous media matrix and one detector. The ENPs and grains (collectors) are represented as rigid spheres with a diameter of 20 and 200 nm, respectively. The ENP solution is initially placed in a cubic simulation box with a size of 2000 nm. The length and depth of the porous media simulation box are the same as those of the ENP matrix whereas its height is equal to 4000 nm. The boundary conditions are applied in the x and y lateral directions. During simulation, ENPs undergo elementary random movements based on a random angle and a random distance of a maximum radius of 5 nm, with limitative constraints for backward movement in the z (length) direction. | |
The porous media simulation box has an (x, y) size of 2000 nm and 4000 nm of depth (z). Due to its finite size, the lateral boundary conditions and minimum image convention44–46 are applied in the x and y directions to avoid any side effects. The collectors are represented by impenetrable spheres with an arbitrary diameter of 200 nm. The collector diameter is an input parameter and can be adapted in the colloidal size range. Due to model restrictions related to the size of the simulation box, the number of ENPs to consider, the computer time, and the larger collectors cannot be considered at the moment. Therefore, the model is limited to the modeling of the influence of colloidal collectors, which are also expected to play key roles in natural porous media. The collectors are positioned inside the porous media simulation box using the random sequential packing (RSP) of spheres in a 3D model.47 The water phase is represented as a continuum and the presence of air bubbles is not considered. To generate a porous media matrix of given porosity, the number of grains is derived directly from the porosity definition:
|  | (1) |
where
N is the number of grains,
P the porosity,
VB the volume of the simulation box and
VG the volume of a single grain. It should be noted that the RSP model based on the use of uniform spheres has a jamming coverage estimate of 0.38, corresponding to a limiting porosity of 0.62, and does not allow low porosities such as those found in natural soils to be generated. This is the reason why in this study, we are considering high porosity situations with values ranging between 0.7 and 1. The (
x,
y,
z) positions of the mobile ENPs passing through the porous media are recorded allowing a detailed ENP position tracking analysis during a simulation run. A detector, which thickness is set to three nanoparticle radii, is placed at the output of the porous media matrix and allows determination of the BTCs.
2.2 Off-lattice Monte Carlo coarse grained procedure
Monte Carlo (MC) simulations have been already successfully applied to study the fate and transport of ENPs in wastewater treatment plants to calculate variability and uncertainty of ENP predicted environmental concentrations (PECs) as well as to evaluate PEC changes as a function of ENP physicochemical properties.48–51 In this research, they are performed to follow ENP distribution and transport as a function of the MC steps inside porous media. Every MC step, ENPs are allowed to undergo elementary random movements based on a random angle and a random distance of a maximum radius of 5 nm, with limitative constraints for backward movement in the z (length) direction. The angle is random in order to simulate the random Brownian motion (diffusion), whereas advection is modelled by making the negative z-direction dominant. A new position is accepted if the excluded volume criteria between ENPs and with collectors are fulfilled. Otherwise, the movement is rejected and the nanoparticle stays in its previous position until the next MC step. An example of ENP trajectories in the absence of homoaggregation, attachment and detachment processes is presented in Fig. S1 (ESI†). A contact between ENPs occurs when a distance between centers of the spheres is smaller or equal to the sum of radii of interacting ENPs. A contact between the ENP and collector occurs when a distance between centers of the spheres is equal to the sum of radii of interacting ENPs and collectors reduced by a value of imposed mean displacement. If the displacement leads to a contact with another nanoparticle or a collector, a uniformly distributed random number between 0 and 1 is generated and compared to the corresponding attachment efficiencies (αENP–ENP or αatt). Different values of αENP–ENP and αatt are chosen at the beginning of each simulation in order to model ENP homoaggregation and deposition on grains, respectively. If the generated number is lower or equal to the imposed efficiencies, the nanoparticle status changes from mobile to fixed and it is considered as attached to the other nanoparticles or collectors until the end of the simulation. The ENPs can form mobile homoaggregates as they attach to other mobile ENPs, or they can form fixed homoaggregates when ENPs attach to ENPs already deposited at the surface of grains or, finally, ENPs are deposited if they attach to grains. The straining is modelled by the constant application of excluded volume criteria. If ENP detachment is introduced and that an attached nanoparticle is chosen to be moved, a random number between 0 and 1 is first generated and compared to the imposed detachment efficiencies (αdet). Different values of αdet are determined at the beginning of each simulation in order to simulate the ENP detachment process. If the generated number is lower or equal to them, the nanoparticle status changes from fixed to mobile and it is allowed to change its position during the next MC step. Each ENP that manages to pass through the porous media matrix is registered by the detector. A BTC is generated by calculating the ratio between the number of ENPs registered by the detector at a given MC step (Nt) and the initial number of ENPs (N0). The simulation ends when all ENPs pass through the detector or after 50
000 MC steps if some ENPs are definitively blocked (strained). In most cases, this number of MC steps is found to be sufficient to generate ascending segments, plateaus and descending segments of each BTC.
2.3 Retention probability, αglobal(tref), definition and calculation
In this study, a global attachment efficiency or retention probability (αglobal(tref)) is defined as the probability that ENPs remain retained within the porous media. The suggested definition might be similar to the definition of removal efficiency used in experimental studies. αglobal(tref) is calculated for a given time step (tref) from the following equation: |  | (2) |
where Nt represents the number of ENPs at a given time t, N0 is the initial number of ENPs at time t0 and Nt (tref) is the number of ENPs measured by the detector at the outlet of the porous media matrix at time tref. The values of Nt (tref)/N0 can be derived directly from BTCs. The time tref is calculated from the BTC obtained for a reference case (porosity = 0.7, αatt = 0, αdet = 0, αENP–ENP = 0) and corresponds to the time when less than 1% of all mobile ENPs remain inside the porous media matrix.
3. Results and discussion
3.1 Influence of media porosity
The influence of media porosity on the transport of ENPs was first investigated. A porous media matrix of randomly placed non-overlapping monodispersed spheres is generated at the beginning of MC simulations. The number of grains is respectively adjusted to 1146, 764, 382, and 0 in order to obtain matrices with porosity values ranging from 0.7 to 1. While these porosities are related to model restrictions, we would like to point out that, even in column experiments, there will be many regions occurring having local porosities as high as 0.7–1 whereas there will be other regions having virtually zero porosity. The porous media matrix which is characterized by a porosity of 1, i.e. having no grains, is considered as a reference case. Then, 19
098 mobile ENPs are randomly distributed with respect to the excluded volume criteria inside a simulation box located at the top of each porous media matrix. When the simulation is started, ENPs move downwards and enter the pore space. In this case, αENP–ENP, αatt and αdet are set to 0.
As shown in Fig. 2, the temporal ENP occurrence at the outlet of the porous media matrix is affected by changes in porosity. A decrease in porosity leads to a rightward shift of BTCs and emergence of a moderate tailing. Such retardation and modification of BTC shapes are due to the higher residence and transport time of ENPs. Decreasing porosity causes an increase of tortuosity and length of the pores and thereby makes ENP diffusion more constraint and time-consuming (Fig. 3). The straining effects, i.e. trapping of particles in pores that are too narrow to allow particle passage, are not observed here. This is in agreement with the fact that straining effects are thought to be significant when the ratio between the particle diameter and grain diameter, here equal to 0.1, is smaller than 0.02.52
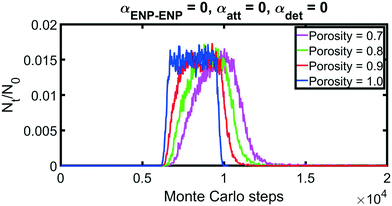 |
| Fig. 2 Breakthrough curves obtained for various values of media porosity. Attachment efficiencies αENP–ENP and αatt as well as detachment efficiencies αdet were set to 0. | |
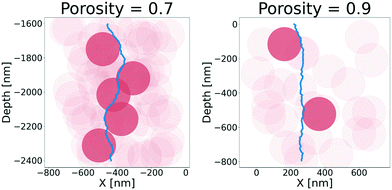 |
| Fig. 3 Trajectory of an arbitrary ENP through porous media with a porosity of 0.7 and 0.9, respectively. Highlighted grains are at a distance smaller or equal to 10 nm from passing ENPs. Homoaggregation, attachment and detachment effects are not considered here. When the porosity is decreasing, the ENP trajectory becomes more tortuous and the ENP has more chance to be in contact with grains. | |
3.2 Influence of homoaggregation
Homoaggregation is generally considered to have an effect on transport processes and accordingly retention via straining effects and deposition at the surface of the collectors. Some studies suggest that, depending on the physicochemical conditions, the impact of homoaggregation and deposition on BTCs may be comparable.53 The influence of homoaggregation on ENP transport is here investigated by considering a porous media matrix with a porosity of 0.7 and in the absence of deposition and detachment processes (αatt = 0, αdet = 0). A large set of homoaggregation attachment efficiencies αENP–ENP, ranging from 0 to 1, is chosen to consider different ENP and grain physicochemical properties (for instance surface charges and Hamaker constants) as well as variations of characteristics of the interstitial water properties (pH and ionic strength) playing important roles in the DLVO interaction energies. The case where αENP–ENP is equal to 0 is considered as the reference case.
BTCs obtained for different homoaggregation attachment efficiencies αENP–ENP are presented in Fig. 4a. A significant decrease in ENP concentration in the effluent is observed even for very small αENP–ENP values such as 0.005. Moreover, changes in BTC shapes become more visible with an increase of αENP–ENP values. Only BTC shapes obtained for αENP–ENP of 0.005 and 0.01 are similar to the one modelled for the reference case. Other BTC shapes are more extended and flattened due to the increase of homoaggregate formation and subsequent straining inside the pore space. High values of ENP–ENP collision efficiency lead rapidly to the formation of large aggregates. This phenomenon is expected to be enhanced with decreasing porosity because the same number of particles is confined in more narrow pores and the longer travel distances in more tortuous pores will increase the probability that particles can interact. Increasing the aggregate size also increases the probability of straining effects.54 Aggregates become too large to be transported through tight pores and are strained at various depths. This causes local and global changes in soil effective porosity which globally decreases from 0.7 down to 0.25 for αENP–ENP values greater than 0.1 as shown in Fig. S2 (ESI†). At the end of the simulation, for αENP–ENP values equal or greater than 0.3, as much as 90% of ENPs are strained inside the pores (Fig. 4b). Darlington et al. (2009)55 studied transport of aluminum oxide ENPs of various sizes through natural soils. They also observed that homoaggregate formation and their further accumulation inside the soil matrix was changing the effective pore size as well as reducing ENP relative peak effluent concentrations.
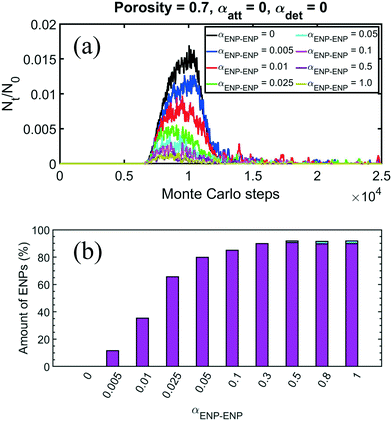 |
| Fig. 4 a) Breakthrough curves modelled for different homoaggregation attachment efficiencies (αENP–ENP). Attachment (αatt) and detachment (αdet) processes were not considered here. Porous media matrix porosity was equal to 0.7. b) Percentage of unbound or aggregated ENPs retained inside the porous media matrix (violet) as well as ENPs blocked at the top of the porous media matrix (light blue) at the end of the simulation (after 50 000 MC steps). Attachment (αatt) and detachment (αdet) processes were not considered here. Porous media matrix porosity was equal to 0.7. | |
A vertical distribution of ENPs inside the porous media was calculated according to the method described in the ESI† (SI3) and is presented in Fig. 5a and b. When homoaggregation attachment efficiency αENP–ENP values are smaller than 0.05, the number of retained ENPs tends to be more or less constant with the distance from the inlet of the porous media matrix. These profiles resemble to a non-monotonic distribution of ENPs that has already been reported in the literature.56 While interactions between ENPs are increasing, the number of retained ENPs achieves a maximum at the top of the porous media matrix, i.e. at depths varying from 250 to 500 nm. This enhanced retention at the column inlet is caused by homoaggregation and resulting straining effects. Pores which are blocked by aggregates act as dead ends for the rest of the mobile or aggregated ENPs and contribute to their local accumulation. The presence of ENPs at significant depths, even at high homoaggregation rates αENP–ENP, can be explained by transport in large continuous pore networks. Even though the retention process is homoaggregation in combination with straining, these curves could be described by continuum particle transport models considering deposition, remobilization and blocking processes.57 This exposes the weakness of continuum models as a tool for investigating dominating processes occurring in porous media.
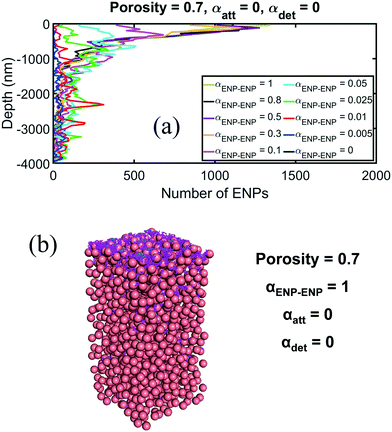 |
| Fig. 5 a) Final distribution of unbound and aggregated ENPs inside the porous media matrix obtained at the end of the MC simulation run (after 50 000 MC steps). Attachment (αatt) and detachment (αdet) processes were not considered here. Porous media matrix porosity is equal to 0.7. b) Porous media matrix at the end of the simulation (αENP–ENP = 1, αatt = 0, αdet = 0, porosity = 0.7). Blue ENPs are unbound whereas the violet ones are aggregated. Some of the ENPs are blocked at the top of the matrix due to physical blockage of larger aggregates. | |
3.3 Influence of ENP–collector attachment efficiencies
In this section, the influence of different attachment efficiencies αatt on the overall ENP retention and transport is evaluated specifically by considering a porous media matrix with a porosity of 0.7 without homoaggregation and detachment processes. Straining could still occur, because deposition of ENPs effectively narrowed pore diameters preventing subsequent ENPs from passing pore necks.
Fig. 6a shows BTCs obtained for different attachment efficiencies αatt. As can be noted, ENP breakthrough is only observable for αatt values equal or smaller than 0.005. BTCs become more flattened and wide as αatt values are increasing and disappear completely for αatt values greater than 0.1. Observed BTC modifications are in good agreement with previous theoretical results. Babakhani et al. (2017) modelled BTCs of nanoparticles by solving the colloid transport model equation accounting for irreversible attachment and showed that increasing values of deposition rate coefficient Katt also cause a flat reduction of BTC plateau and increase in removal efficiency by the porous matrix.59
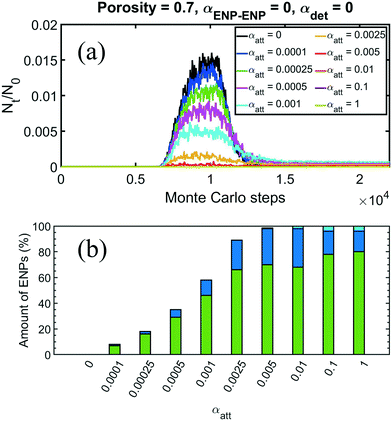 |
| Fig. 6 a) Breakthrough curves obtained for different attachment efficiencies αatt. Homoaggregation (αENP–ENP) and detachment (αdet) processes were not considered here. Porous media matrix porosity is equal to 0.7. b) Amount of ENPs attached to the grains inside the porous media matrix (green), of ENPs blocked physically inside due to straining effects (dark blue) and of ENPs strained at the top of the porous matrix (light blue). Straining effects are more and more significant by increasing the attachment efficiency between ENPs and soil grains. Porosity of the porous media matrix is equal to 0.7. Homoaggregation and detachment processes were not considered (αENP–ENP = 0, αdet = 0). | |
Fractions of ENPs strained and deposited on collector surfaces inside and outside of the porous media matrix at very low and very high αatt values were calculated according to the method described in the ESI† (SI4) and are presented in Fig. 6b. For the αatt value equals to 0.005, i.e. for the greatest value for which BTCs could be recorded, at the end of the simulation, 70% of ENPs inside the porous media matrix were attached to the collectors, approximately 28% were strained inside the porous media matrix whereas 2% were strained at the top of it. In the case of the highest αatt value (αatt = 1), 80% of ENPs were attached to the collector surfaces, 16% were strained inside the porous media matrix whereas 4% were strained at the top of it. This indicate that not only the presence of inter-particle forces but also physical straining occurring because of narrowing pore necks result in an important immobilization of ENPs, even though the small diameter of ENPs as well as high porosity would suggest otherwise. This mechanism is, however, only expected to occur when relatively high ENP concentrations are added to the porous media. Attached particles cause an increase of tortuosity and pore length as well as pore clogging. Back to Fig. S2 (ESI†), as the number of attached ENPs is increasing with increasing αatt values, the effective porosity is found to rapidly decrease from 0.7 to approximately 0.2. Depending on the attachment efficiency between ENPs and collectors, the vertical distributions (Fig. 7a and b) rather follow an exponential decay and always monotonously decrease as predicted by continuum models.57
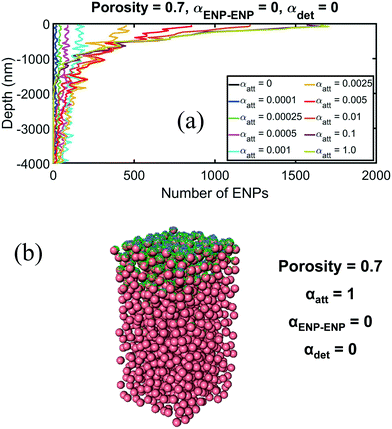 |
| Fig. 7 a) Final ENP distribution inside the porous media matrix at the end of the simulation. Homoaggregation (αENP–ENP) and detachment (αdet) processes are not considered here. Porous media matrix porosity is equal to 0.7. b) Porous media matrix at the end of the simulation (αatt = 1, αENP–ENP = 0, αdet = 0, porosity = 0.7). Blue ENPs are unbound whereas the green ones are attached. Most of the ENPs are blocked at the top of the matrix due to collector saturation and straining effects. | |
3.4 Influence of detachment
In the previous section, ENP–collector deposition was considered irreversible. However, sudden decreases in ionic strength, strong pH changes or the addition of phosphate or organic matter that increases surface charges may cause a significant release of already attached ENPs,58 in particular ENPs that are attached in a secondary energy minimum. Here, the porous media matrix has a porosity of 0.7 and the case where αdet and αatt are equal to 0 is set as the reference case. The homoaggregation process is omitted here.
Fig. 8a–c present BTCs obtained for αatt values equal to 0.05, 0.5 and 1, respectively, and detachment efficiencies ranging from 0 to 1. As indicated in the previous section, in the absence of detachment, all ENPs remain attached to the collectors and no breakthrough is observable for αatt greater than 0.005. As shown here, the introduction of even a small deposition reversibility to the system results in significant changes in the BTC and ENP retention, thus indicating the importance of detachment processes for ENP transport in porous media.
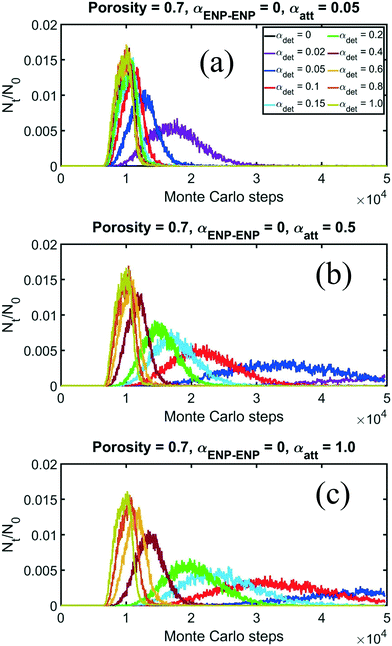 |
| Fig. 8 BTCs obtained at different attachment efficiencies equal to 0.05 (a), 0.5 (b) and 1 (c) and different values of the detachment efficiency. Homoaggregation (αENP–ENP) and straining processes are not considered here. Porous media matrix porosity is equal to 0.7. Retardation effects are particularly important at high attachment efficiency and low detachment values. | |
When αatt values are very small (Fig. 8a, with αatt = 0.05), BTCs modelled for αdet values higher than 0.1 are similar to the ones obtained for the porous media matrix with αatt, αdet and αENP–ENP values equal to 0 (reference case). Although ENPs are subjected to an important number of attachment–detachment processes during their transport, such interactions are not significantly changing the BTC shapes. On the other hand, an important retardation and widening of BTCs (retardation and spreading effect) can be observed when αdet is smaller than or equal to 0.1. Here, sequences of attachment–detachment processes increase the retention–re-entrainment of ENPs. Moreover, the straining process is not observable in this case.
When deposition rates are moderately high-to-high (Fig. 8b, with αatt = 0.5 and Fig. 8c with αatt = 1), BTCs shift and widening is more pronounced. Retardation and spreading effects are especially important in the case of small detachment values. ENPs are first attached to the soil surface but, due to the strong inter-particle interactions, they are slowly detached over time. Consequently, all ENPs leave the porous media matrix over the simulation time. Only scenarios where αdet is equal to or smaller than 0.1 are an exception. It is worth noting that, for the αdet value of 0.02, BTCs emerge at 35
000 MC steps for αatt equal to 0.5 and do not emerge during the simulation time for αatt equal to 1. Moreover, similarly to the case presented above, the straining process was not observable here.
The above results are in good agreement with previous findings in the literature. Babakhani et al. (2017)59 solved the colloid transport model equation accounting both for deposition and detachment, by maintaining the deposition rate coefficient Katt constant and varying the detachment rate coefficient Kdet. It was observed that increasing detachment causes an emergence of BTC tailing and a rise of the BTC plateau at the side of the descending segment. It should be noted that an asymmetric shape of the BTC plateau was not observed in our study because the considered porous media matrix depth was not sufficiently large to allow a visible plateau to be generated.
3.5 Influence of homoaggregation, deposition and detachment processes on the probability of ENP retention αglobal(tref)
This section examines the influence of homoaggregation, deposition and detachment on αglobal(tref) values, i.e. the probability that ENPs remain inside the porous media matrix. αglobal(tref) values are calculated for 17
670 MC time steps. This reference time (tref) is derived from the BTC obtained for the case where the matrix porosity is 0.7 and αENP-ENP, αatt and αdet are equal to 0. This reference time allows the isolation of the effects of homoaggregation, deposition and detachment processes from the geometrical constraints of the porous matrix.
α
global(tref) values as a function of different αatt (effect of deposition) and αENP–ENP (effect of homoaggregation) are given in Fig. 9. In this case, αdet is set to 0. It can be seen that αglobal(tref) values were obtained between 1, corresponding to a situation for which all ENPs are retained inside the soil pores, and 0, corresponding to a situation for which all ENPs are transported through the porous media matrix. Interestingly, on the one hand, lower αatt values are needed to reach high αglobal(tref) values and plateau close to 1. On the other hand, higher values of αENP–ENP are required to achieve a plateau close to 0.9 for αglobal(tref). This finding indicates the dominating effect of the deposition process on the overall transport distances of ENPs. Moreover, and surprisingly, in the presence of homoaggregation only, αglobal(tref) is never equal to unity and remains close to 0.9. This indicates that in most cases, single non-aggregated particles or very small aggregates manage to go through the porous media matrix via sufficiently large and unblocked pores.
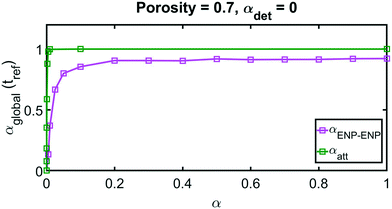 |
| Fig. 9 Variation of the global attachment efficiency (αglobal(tref)) as a function of individual attachment efficiencies between ENPs and collectors (αatt) and ENP–ENP homoaggregation rates (αENP–ENP). | |
Variation of αglobal(tref) as a function of a set of αdet and αatt values equal respectively to 0.05, 0.1, 0.2, 0.3, 0.5, 0.7 and 1.0 is presented in Fig. 10a. Results indicate that an increase of αdet values causes a decrease of αglobal(tref) values from 1 to 0 and consequently decrease the probability of ENPs to be retained within the porous matrix. On the other hand, decreasing the αdet values leads to a significant increase of αglobal(tref) and therefore ENP retention. Fig. 10b shows the relationship between attachment and detachment efficiencies and αglobal(tref) values. It is shown that a αglobal(tref) value close to one may be achieved only if deposition values are very high and detachment efficiencies do not exceed a value of approximately 0.5. In reality, however, both reversible and irreversible attachment occur simultaneously, which again reduces the effect of detachment on the overall transport of ENPs.
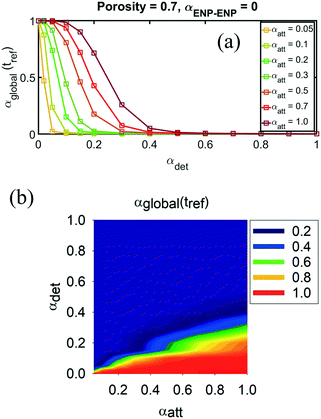 |
| Fig. 10 a) Global attachment efficiency as a function of different attachment and detachment efficiencies. b) Relationship between the detachment and attachment efficiencies and the global attachment efficiency. Red regions indicate a high retention rate of ENPs inside the porous media matrix, whereas blue regions indicate their significant transport. | |
It should be noted that different sets of αdet and αatt values, reflecting different ENPs and collector physicochemical properties and inter-particle forces, can lead to similar αglobal(tref) values (Fig. 10a). For instance, αglobal(tref) equal to 0.5 corresponds to a set of αatt = 1.0 and αdet = 0.3 but also to a set of αatt = 0.5 and αdet = 0.2. An additional example for αglobal(tref) equal to 0.2 indicates that such a value may be obtained when αatt = 0.7 and αdet = 0.35, and αatt = 0.3 and αdet = 0.17. This constitutes an important outcome indicating that αglobal(tref) values cannot reflect the importance and balance of local effects such as deposition and detachment processes.
4. Conclusions
Monte Carlo simulations were carried out to investigate the influence of homoaggregation, deposition and detachment processes as well as porosity on ENP retention and mobility in porous media. The coarse-grained and discrete model presented here indicates that porosities varying from 1 to 0.7 already significantly affect the ENP residence time inside the granular medium. Nonetheless, it was demonstrated that porous media matrices of such high porosities do not have sufficiently narrow pores to cause ENP straining. A subsequent introduction of ENP–ENP and ENP–collector interactions into the system revealed that these processes play a key role in ENP retention and distribution inside the porous media. In both cases, interparticle interactions led to smaller ENP effluent concentration and thus to flattening and broadening of BTCs. It was found that 90% of ENPs are retained inside the porous media matrix for homoaggregation attachment efficiency values αENP–ENP equal to or greater than 0.3. It was also observed that almost all ENPs are retained inside the porous media matrix for attachment efficiency values αatt equal to or greater than 0.005. It was also demonstrated that the homoaggregation process contributes slightly less to ENP retention than deposition on collectors because unbound ENPs or small aggregates manage to pass through sufficiently large pores. As for the ENP retention profiles, depending on the strength of interactions between ENPs and grains, the number of attached or physically blocked ENPs decreased monotonously or exponentially with depth. As for the straining process, both ENP attachment to collectors and aggregate formation contributed to the pore enclosure and subsequent straining of free ENPs. A complementary study taking into consideration the attachment–detachment effects showed that a slight increase of detachment efficiencies causes a significant release of attached ENPs. The αglobal(tref) parameter has been calculated to describe ENP retention in porous medium. This allowed us to reveal how probability of ENP retention changes not only as a function of deposition but also as a function of different processes and porous medium characteristics. It was found that even small affinities between ENPs or ENPs and collectors contribute to high global attachment efficiency values and thereby result in the increase of ENP retention. By subtracting the geometrical constraints of the porous matrix it was also observed that different sets of attachment–detachment values, reflecting different physicochemical conditions, can correspond to the same αglobal(tref) values suggesting that the αglobal(tref) parameter, often obtained from column tests, is not relevant to describe the importance of attachment and detachment processes taking place at the collector surface. This constitutes an important outcome indicating that αglobal(tref) values determined from functional tests are not mechanistic but operationally defined parameters and thus cannot be deemed predictive beyond the functional test in which they were determined. Moreover, for continuum models calibrated to column test, breakthrough curves will poorly reflect effective deposition and detachment rates in those cases where there are many simultaneously occurring processes.
Conflicts of interest
There are no conflicts to declare.
Acknowledgements
The authors acknowledge support received from the European Commission within the Horizon 2020 research and innovation program and the Swiss Secrétariat d'Etat à la formation, à la Recherche et à l'Innovation (SEFRI) under grant agreements NanoFASE-646002 and 15.0183-2 and the University of Geneva. The computations were performed at the University of Geneva on the Baobab cluster. The authors acknowledge COINF, Bastien Chopard, Yann Sagon and all the Baobab team.
References
- C. European, Commission recommendation of 18 October 2011 on the definition of nanomaterial (2011/696/EU), Official Journal of the European Union, 2011, 275, 38–40 Search PubMed.
- A. R. Petosa, D. P. Jaisi, I. R. Quevedo, M. Elimelech and N. Tufenkji, Aggregation and Deposition of Engineered Nanomaterials in Aquatic Environments: Role of Physicochemical Interactions, Environ. Sci. Technol., 2010, 44(17), 6532–6549 CrossRef CAS PubMed.
- T. Tsuzuki, Commercial Scale Production of Inorganic Particles, Int. J. Nanotechnol., 2009, 6(5), 567–578 CrossRef CAS.
- F. Gottschalk and B. Nowack, The release of engineered nanomaterials to the, environment, J. Environ. Monit., 2011, 13(5), 1145 RSC.
- M. C. DeRosa, C. Monreal, M. Schnitzer, R. Walsh and Y. Sultan, Nanotechnology in fertilizers, Nat. Nanotechnol., 2010, 5(2), 91 CrossRef CAS PubMed.
- M. Kah, S. Beulke, K. Tiede and T. Hofmann, Nanopesticides: State of Knowledge, Environmental Fate, and Exposure Modeling, Crit. Rev. Environ. Sci. Technol., 2013, 43(16), 1823–1867 CrossRef CAS.
- W.-X. Zhang, Nanoscale iron particles for environmental remediation: An overview, J. Nanopart. Res., 2003, 5(3/4), 323–332 CrossRef CAS.
- J. Urra, I. Alkorta, I. Mijangos, L. Epelde and C. Garbisu, Application of sewage sludge to agricultural soil increases the abundance of antibiotic resistance genes without altering the composition of prokaryotic communities, Sci. Total Environ., 2019, 647, 1410–1420 CrossRef CAS PubMed.
- S. C. Bolyard, D. R. Reinhart and S. Santra, Behavior of Engineered Nanoparticles in Landfill Leachate, Environ. Sci. Technol., 2013, 47(15), 8114–8122 CAS.
- J. Wang, M. M. Nabi, S. K. Mohanty, A. R. M. N. Afrooz, E. Cantando and N. Aich,
et al. Detection and quantification of engineered particles in urban runoff, Chemosphere, 2020, 248, 126070 CrossRef CAS PubMed.
- J. R. Lead, G. E. Batley, P. J. J. Alvarez, M. N. Croteau, R. D. Handy and M. J. McLaughlin,
et al. Nanomaterials in the environment: Behavior, fate, bioavailability, and effects-An updated review, Environ. Toxicol. Chem., 2018, 37(8), 2029–2063 CrossRef CAS PubMed.
- R. R. Hurley and L. Nizzetto, Fate and occurrence of micro(nano)plastics in soils: Knowledge gaps and possible risks, Curr. Opin. Environ. Sci. Health, 2018, 1, 6–11 CrossRef.
- T. M. Straub, I. L. Pepper and C. P. Gerba, Persistence of viruses in desert soils amended with anaerobically digested sewage sludge, Appl. Environ. Microbiol., 1992, 58(2), 636–641 CrossRef CAS PubMed.
- I. Chowdhury, Y. Hong, R. J. Honda and S. L. Walker, Mechanisms of TiO2 nanoparticle transport in porous media: Role of solution chemistry, nanoparticle concentration, and flowrate, J. Colloid Interface Sci., 2011, 360(2), 548–555 CrossRef CAS PubMed.
- G. Cornelis, K. Hund-Rinke, T. Kuhlbusch, N. Van Den Brink and C. Nickel, Fate and Bioavailability of Engineered Nanoparticles in Soils: A Review, Crit. Rev. Environ. Sci. Technol., 2014, 44(24), 2720–2764 CrossRef CAS.
- A. Franchi and C. R. O'Melia, Effects of Natural Organic Matter and Solution Chemistry on the Deposition and Reentrainment of Colloids in Porous Media, Environ. Sci. Technol., 2003, 37(6), 1122–1129 CrossRef CAS PubMed.
- I. G. Godinez and C. J. G. Darnault, Aggregation and transport of nano-TiO2 in saturated porous media: Effects of pH, surfactants and flow velocity, Water Res., 2011, 45(2), 839–851 CrossRef CAS PubMed.
- J. He, D. Wang and D. Zhou, Transport and retention of silver nanoparticles in soil: Effects of input concentration, particle size and surface coating, Sci. Total Environ., 2019, 648, 102–108 CrossRef CAS PubMed.
- B. Jung, D. O'Carroll and B. Sleep, The influence of humic acid and clay content on the transport of polymer-coated iron nanoparticles through sand, Sci. Total Environ., 2014, 496, 155–164 CrossRef CAS PubMed.
- R. S. Kookana, A. B. A. Boxall, P. T. Reeves, R. Ashauer, S. Beulke and Q. Chaudhry,
et al. Nanopesticides: Guiding Principles for Regulatory Evaluation of Environmental Risks, J. Agric. Food Chem., 2014, 62(19), 4227–4240 CrossRef CAS PubMed.
- N. C. Mueller, J. Braun, J. Bruns, M. Černík, P. Rissing and D. Rickerby,
et al., Application of nanoscale zero valent iron (NZVI) for groundwater remediation in Europe, Environ. Sci. Pollut. Res., 2012, 19(2), 550–558 CrossRef CAS PubMed.
- S. Laumann, V. Micić, G. V. Lowry and T. Hofmann, Carbonate minerals in porous media decrease mobility of polyacrylic acid modified zero-valent iron nanoparticles used for groundwater remediation, Environ. Pollut., 2013, 179, 53–60 CrossRef CAS PubMed.
- D. O'Carroll, B. Sleep, M. Krol, H. Boparai and C. Kocur, Nanoscale zero valent iron and bimetallic particles for contaminated site remediation, Adv. Water Resour., 2013, 51, 104–122 CrossRef.
- R. J. B. Peters, G. van Bemmel, N. B. L. Milani, G. C. T. den Hertog, A. K. Undas and M. van der Lee,
et al. Detection of nanoparticles in Dutch surface waters, Sci. Total Environ., 2018, 621, 210–218 CrossRef CAS PubMed.
- V. S. Sousa and M. Ribau Teixeira, Metal-based engineered nanoparticles in the drinking water treatment systems: A critical review, Sci. Total Environ., 2020, 707, 136077 CrossRef CAS PubMed.
- R. J. Honda, V. Keene, L. Daniels and S. L. Walker, Removal of TiO2 Nanoparticles During Primary Water Treatment: Role of Coagulant Type, Dose, and Nanoparticle Concentration, Environ. Eng. Sci., 2014, 31(3), 127–134 CrossRef CAS PubMed.
- Q. Sun, Y. Li, T. Tang and Z. Yuan, Yu C-P. Removal of silver nanoparticles by coagulation processes, J. Hazard. Mater., 2013, 261, 414–420 CrossRef CAS PubMed.
- Y. Zhang, Y. Chen, P. Westerhoff, K. Hristovski and J. C. Crittenden, Stability of commercial metal oxide nanoparticles in water, Water Res., 2008, 42(8), 2204–2212 CrossRef CAS PubMed.
- K.-M. Yao, M. T. Habibian and C. R. O'Melia, Water and waste water filtration, Concepts and applications, Environ. Sci. Technol., 1971, 5(11), 1105–1112 CrossRef CAS.
- A. Praetorius, E. Badetti, A. Brunelli, A. Clavier, J. A. Gallego-Urrea and A. Gondikas,
et al. Strategies for determining heteroaggregation attachment efficiencies of engineered nanoparticles in aquatic environments, Environ. Sci.: Nano, 2020, 7(2), 351–367 RSC.
- M. Elimelech and C. R. O'Melia, Kinetics of deposition of colloidal particles in porous media, Environ. Sci. Technol., 1990, 24(10), 1528–1536 CrossRef CAS.
- R. Bai and C. Tien, A New Correlation for the Initial Filter Coefficient under Unfavorable Surface Interactions, J. Colloid Interface Sci., 1996, 179(2), 631–634 CrossRef CAS.
- M. Elimelech, Predicting collision efficiencies of colloidal particles in porous media, Water Res., 1992, 26(1), 1–8 CrossRef CAS.
- M. W. Hahn and C. R. O'Melia, Deposition and Reentrainment of Brownian Particles in Porous Media under Unfavorable Chemical Conditions: Some Concepts and Applications, Environ. Sci. Technol., 2004, 38(1), 210–220 CrossRef CAS PubMed.
- N. Tufenkji and M. Elimelech, Correlation Equation for Predicting Single-Collector Efficiency in Physicochemical Filtration in Saturated Porous Media, Environ. Sci. Technol., 2004, 38(2), 529–536 CrossRef CAS PubMed.
- J. Drelich and Y. U. Wang, Charge heterogeneity of surfaces: mapping and effects on surface forces, Adv. Colloid Interface Sci., 2011, 165(2), 91–101 CrossRef CAS PubMed.
- M. Tong and W. P. Johnson, Excess Colloid Retention in Porous Media as a Function of Colloid Size, Fluid Velocity, and Grain Angularity, Environ. Sci. Technol., 2006, 40(24), 7725–7731 CrossRef CAS PubMed.
- S. A. Bradford, M. Bettahar, J. Simunek and M. T. Van Genuchten, Straining and Attachment of Colloids in Physically Heterogeneous Porous Media, Vadose Zone J., 2004, 3(2), 384–394 CrossRef CAS.
- A. L. Dale, G. V. Lowry and E. A. Casman, Much ado about α: reframing the debate over appropriate fate descriptors in nanoparticle environmental risk modeling, Environ. Sci.: Nano, 2015, 2(1), 27–32 RSC.
- I. L. Molnar, P. C. Sanematsu, J. I. Gerhard, C. S. Willson and D. M. O'Carroll, Quantified Pore-Scale Nanoparticle Transport in Porous Media and the Implications for Colloid Filtration Theory, Langmuir, 2016, 32(31), 7841–7853 CrossRef CAS PubMed.
- Y. Li, Y. Wang, K. D. Pennell and L. M. Abriola, Investigation of the Transport and Deposition of Fullerene (C60) Nanoparticles in Quartz Sands under Varying Flow Conditions, Environ. Sci. Technol., 2008, 42(19), 7174–7180 CrossRef CAS PubMed.
-
S. W. Ahlstrom, H. P. Foote, R. C. Arnett, C. R. Cole and R. Serne, Multicomponent Mass Transport Model: Theory and Numerical Implementation (Discrete-Parcel–Random-Walk Version), 1977 Search PubMed.
- A. Taghavy, A. Mittelman, Y. Wang, K. D. Pennell and L. M. Abriola, Mathematical Modeling of the Transport and Dissolution of Citrate-Stabilized Silver Nanoparticles in Porous Media, Environ. Sci. Technol., 2013, 47(15), 8499–8507 CAS.
-
D. Frenkel and B. Smit, Understanding Molecular Simulation, Academic Press, San Diego, 2nd edn, 2002, 2002/01/01/, p. 664 Search PubMed.
-
A. Leach, Molecular Modelling: Principles and Applications, Pearson Education, 2nd edn, 2001 Search PubMed.
-
D. C. Rapaport, The Art of Molecular Dynamics Simulation, Cambridge University Press, Cambridge, 2nd edn, 2004 Search PubMed.
- J. W. Evans, Random and cooperative sequential adsorption, Rev. Mod. Phys., 1993, 65(4), 1281–1329 CrossRef CAS.
- L. E. Barton, M. Auffan, M. Durenkamp, S. McGrath, J.-Y. Bottero and M. R. Wiesner, Monte Carlo simulations of the transformation and removal of Ag, TiO2, and ZnO nanoparticles in wastewater treatment and land application of biosolids, Sci. Total Environ., 2015, 511, 535–543 CrossRef CAS.
- C. O. Hendren, A. R. Badireddy, E. Casman and M. R. Wiesner, Modeling nanomaterial fate in wastewater treatment: Monte Carlo simulation of silver nanoparticles (nano-Ag), Sci. Total Environ., 2013, 449, 418–425 CrossRef CAS PubMed.
- J. A. J. Meesters, W. J. G. M. Peijnenburg, A. J. Hendriks, D. Van de Meent and Quik J. T. K., A model sensitivity analysis to determine the most important physicochemical properties driving environmental fate and exposure of engineered nanoparticles, Environ. Sci.: Nano, 2019, 6(7), 2049–2060 RSC.
- J. A. J. Meesters, J. T. K. Quik, A. A. Koelmans, A. J. Hendriks and D. van de Meent, Multimedia environmental fate and speciation of engineered nanoparticles: a probabilistic modeling approach, Environ. Sci.: Nano, 2016, 3(4), 715–727 RSC.
- S. A. Bradford, J. Simunek, M. Bettahar, M. T. van Genuchten and S. R. Yates, Modeling Colloid Attachment, Straining, and Exclusion in Saturated Porous Media, Environ. Sci. Technol., 2003, 37(10), 2242–2250 CrossRef CAS PubMed.
- N. Solovitch, J. Labille, J. Rose, P. Chaurand, D. Borschneck and M. R. Wiesner,
et al. Concurrent Aggregation and Deposition of TiO2 Nanoparticles in a Sandy Porous Media, Environ. Sci. Technol., 2010, 44(13), 4897–4902 CrossRef CAS PubMed.
- S. A. Bradford, S. R. Yates, M. Bettahar and J. Simunek, Physical factors affecting the transport and fate of colloids in saturated porous media, Water Resour. Res., 2002, 38(12), 63 CrossRef.
- T. K. Darlington, A. M. Neigh, M. T. Spencer, O. T. N. Guyen and S. J. Oldenburg, Nanoparticle characteristics affecting environmental fate and transport through soil, Environ. Toxicol. Chem., 2009, 28(6), 1191–1199 CrossRef CAS PubMed.
- D. Wang, L. Ge, J. He, W. Zhang, D. P. Jaisi and D. Zhou, Hyperexponential and nonmonotonic retention of polyvinylpyrrolidone-coated
silver nanoparticles in an Ultisol, J. Contam. Hydrol., 2014, 164, 35–48 CrossRef CAS PubMed.
- E. Goldberg, M. Scheringer, T. D. Bucheli and K. Hungerbühler, Critical Assessment of Models for Transport of Engineered Nanoparticles in Saturated Porous Media, Environ. Sci. Technol., 2014, 48(21), 12732–12741 CrossRef CAS PubMed.
- J. N. Ryan and M. Elimelech, Colloid mobilization and transport in groundwater, Colloids Surf., A, 1996, 107, 1–56 CrossRef CAS.
- P. Babakhani and J. Bridge, Doong R-a, Phenrat T. Continuum-based models and concepts for the transport of nanoparticles in saturated porous media: A state-of-the-science review, Adv. Colloid Interface Sci., 2017, 246, 75–104 CrossRef CAS PubMed.
Footnote |
† Electronic supplementary information (ESI) available. See DOI: 10.1039/d1en00034a |
|
This journal is © The Royal Society of Chemistry 2021 |