A critical evaluation of short columns for estimating the attachment efficiency of engineered nanomaterials in natural soils†
Received
2nd November 2020
, Accepted 14th May 2021
First published on 24th May 2021
Abstract
Short, saturated packed columns are used frequently to estimate the attachment efficiency (α) of engineered nanomaterials (ENMs) in relatively homogeneous porous media, but a combined experimental and theoretical approach to obtain α-values for heterogeneous natural soils has not yet been agreed upon. Accurately determined α-values that can be used to study and predict ENM transport in natural soils should vary with ENM and soil properties, but not with experimental settings. We investigated the effect of experimental conditions, and used different methods to obtain soil parameters, essential to calculate α. We applied 150 different approaches to determine α-values from 52 transport experiments using short columns with 5 different natural soils and 20 and 80 nm gold- or 27 nm silver sulphide ENMs. The choice of column end-filter material and pore size appeared critical to avoid overestimating α owing to filter – ENM interactions and/or incomplete saturation of the column. Using a low ionic strength (4.4 × 10–5 mol L−1) artificial rain water as an aqueous medium avoided ENM homo- or heteroaggregation in all soils, as confirmed by single-particle inductively coupled plasma – time of flight mass spectrometry. ENM breakthrough curves could be modelled using colloid filtration theory assuming irreversible attachment only. α-Values calculated from this model, having the grain size represented by a single average size, accounting for dispersivity and effective porosity based on a prior inert tracer test, explained up to 42% of the variance in α as revealed by partial least squares analysis. However, column length and dispersivity remained as important experimental parameters, which calls for further standardisation efforts of column tests with ENMs in natural soils, preferably cross-validated with batch tests.
Environmental significance
With initial research focus on effects, calculating the exposure of engineered nanomaterials (ENM) in diverse environmental matrices is in most cases not yet possible. A major reason is the lack of agreed upon fate descriptors, i.e. quantifiable parameters that can be used routinely in exposure predictions. The attachment efficiency (α) has frequently been named as the descriptor of choice to describe fate and eventually exposure of ENMs in porous media such as soils, but a validated, generally agreed upon method to determine α for a given ENM – soil combination does not exist. Short column methods are named as the method of choice, but our paper shows that experimental settings of column experiments with heterogeneous natural soils and the chosen theory required to calculate α from the breakthrough curves has a major effect on the final value of α and/or whether there are experimental artefacts. We thus conclude that column tests need to be optimized to reduce experimental artefacts and have formulated several recommendations how strong experimental artefacts can be avoided and which theory is optimal to calculate α. The paper aims to aid the effort of finding a standard column method to determine α-values of natural soils that with some confidence have predictive value beyond the functional column test.
|
Introduction
While the last decade has seen much progress in characterizing the possible ecotoxic effects of engineered nanomaterials (ENMs), predicting their fate in different environments often proves elusive. One reason is that many environmental compartments lack generally agreed-upon ENM-specific fate descriptors. Fate descriptors are parameters quantified during functional tests that characterize the behavior of an ENM or other materials in a particular water, soil or other medium. Such parameters can then be used to predict the fate of these materials in a setting other than the functional test, e.g. in the field.1,2
There is an intimate interaction between ENMs and surfaces of solids in porous media such as soils, important to quantify for risk assessment, because it determines both bioavailability of the ENMs to soil organisms and potential transport to aquifers sometimes used for drinking water production. Transport prediction of ENMs in soils is also important for ENMs intentionally added to porous media, such as nanopesticides or nanozerovalent iron used in groundwater remediation.
Existing fate descriptors for transport of chemicals in soils such as partitioning coefficients (Kd-values) are deemed inappropriate as ENM fate predictors, because partitioning coefficients are conceptually based on an equilibrium paradigm. Particles are invariantly present in the environment as thermodynamically unstable suspensions and their behavior thus requires kinetic fate descriptors.3,4 First-order interaction rate constants are generally determined by injecting ENM suspensions into packed saturated columns of porous media and applying models to the measured breakthrough curve (BTC) and/or depth profile. These models have to a large extent been built on colloid filtration theory (CFT) where interaction of particles in porous media is seen as a two-stage process, i.e. collision of particles with the surface followed by attachment, a process that is assumed irreversible. The collision frequency of particles with pore surfaces is thought to be determined by measurable, operational parameters such as flow rate, porosity and column dimensions. The attachment efficiency (α), i.e. the chance of a particle attaching following collision with pore walls, is sometimes seen as the best alternative to Kd values.3,4 This parameter is in theory only function of properties of the specific particle and porous medium being studied. In this way, α can serve as a fate descriptor for a particular particle – medium combination and potentially be used beyond the functional (column) test in which it was determined.3
Applying CFT to column tests correctly requires rather precise knowledge of the hydrodynamic conditions in the studied porous medium. The clean filter bed is approximated as grains having a uniform spherical size, to which mass transport of ENMs and their collision frequency is described by the single-collector contact efficiency (η0). While several column experiments with ENMs have been conducted in the last decades, they were mostly done on homogeneous substrates (e.g. standardized sand or glass beads) that indeed have a more or less uniform spherical grain size. The focus of these tests was on changing the chemistry of the elution solution asserting that CFT and general colloidal theories such as the effect of pH and ionic strength are indeed applicable to these substrates.
Fewer column experiments were done with natural soils often showing much more complex interactions that can rarely be described solely on the basis of irreversible attachment. Correct interpretation of breakthrough curves from soils usually requires consideration of additional processes such as detachment, straining.5–8 Natural soils are also non-uniform and it is not clear whether the single-collector contact efficiency accurately describes the hydrodynamic conditions leading to collision within non-uniform packed columns. If not, the calculated α cannot be expected independent of the experimental conditions of the column test.2
Packed column tests as described by OECD Test no. 312 (ref. 9) have been advised as the functional test of choice to quantify the interaction of ENMs with, amongst others, natural soils during an OECD expert meeting albeit without a recommendation of how these column tests should be performed and how the BTCs should be interpreted.10 Test nr 312 has been developed primarily for predicting transport of dissolved chemicals in soils and effects of experimental design have not yet been investigated when applying this protocol to ENMs.11–18 It is insufficiently known which artefacts may be incurred during ENM column tests and exactly how CFT should be applied to calculate α. Consequently, there is no consensus on how scientifically or regulatory sound estimates of ENM fate in solids can be obtained.2
Previous meta-analyses6,19 collected large amounts of column data from the literature to investigate soil parameters affecting ENM transport. However, experimental column protocols vary widely, not only in terms of column test design, but also how soil parameters essential for calculation of α are determined. Moreover, protocols are sometimes incompletely described and the calculation methods for α vary. There is thus a large amount of hidden variation amongst reported column tests20 with ENM complicating meta-analysis to find trends in ENM behavior in soils.
In this study, experimental artefacts of short column tests are explored and different approaches to calculate α-values are evaluated on the basis of 52 such tests. The columns used in this study are considered short because the length-to-diameter ratio of all soil columns was below 3. This ratio is known to be important and should not be too high to avoid scaling effects. Large length-to-diameter ratios often give rise to a proportionally too large fraction of the total flow occurring preferentially along the boundary between soil grains and column interior. Moreover, transversal dispersivity may become relevant if the diameter is too large. A diameter-to-length ratio of four is therefore often recommended.21,22 ENMs in particular are generally retained strongly during soil column tests. Zero breakthrough is therefore often found in columns as short as 10 cm (e.g. ref. 5). We therefore chose to work with even shorter columns of 6 to 6.5 cm.
The final goal of the current study was not to suggest a standard protocol for α-value determination, but to provide the scientific basis for reaching such a protocol in the future and to offer guidance for a more accurate estimation of α-values for a specific combination of ENM and soil. Applying a uniform, experimentally artefact-free column protocol allows comparing α-values between different soils to rank these in terms of risk following ENM exposure. Accurate α-values allow predicting ENM transport and possibly even bioavailability in the field. Terrestrial nanotechnology, e.g. nano zerovalent iron for decontamination of soils or nanopesticides, could thus be screened more efficiently, better exposing the specific formulation that has the highest mobility in a given soil.
Calculations of attachment rate using CFT are most sensitive to the assumed average grain (also called collector) diameter and assumed porosity of the packed soil column.23 When using homogeneous porous media such as standardized sands, the grain size is relatively monodisperse, which this is not the case for natural soils. Different ways of determining and accounting for grain size and porosity were therefore compared. Most formulae for calculating α include these parameters and should thus in principle remove their effect on α variation. Surface charge has often been mentioned as the single most important parameter in soils to determine interactions with ENMs.19,24 It was therefore investigated whether different measures of the surface potential indeed explained variation in α-values. In addition to grain size and surface charge, parameters such as soil type, column length, flow rate, and input ENM concentration were varied. 150 different approaches were subsequently used to estimate α-values. α-Values should not vary with experimental settings nor with measurable incidental column properties such as porosity, dispersivity or grain size, because these parameters are in principle taken into account by the theoretical approach to calculate α. Combined experimental and theoretical approaches were therefore evaluated positively in this study if the α-values were influenced strongly by ENM and soil properties, and evaluated negatively if α-values are strongly affected by experimental variation.
Material and methods
Overview
Table 1 shows an overview of the experimental variables of the 52 column experiments. Full experimental details of all experiments can be found in (ESI† Table S6). Four agricultural top soils and one reference soil (Lufa 2.2) were used in saturated column tests. The column length was varied, while keeping the column diameter constant. The soils differed in texture, organic carbon content and mineralogy (see ESI† Table S2). The total concentration of injected suspensions of 20 or 80 nm Au or 27 nm Ag2S ENMs and flow rate were varied to investigate the effect of these parameters on α-values.
Table 1 Overview of experimental parameters. All soil properties can be found in ESI† Table S2 and column settings in ESI† Table S6
Parameter |
Min |
Max |
Two different methods were used to determine d10 and d50 (see text).
|
Column properties |
Column diameter (cm) |
2.5 |
2.5 |
Column length (cm) |
2.7 |
6.3 |
Column filter pore size (μm) |
10 |
70 |
Pump flow rate (mL min−1) |
0.16 |
0.62 |
Total porosity (%) |
36 |
69 |
Bulk density (g cm−3) |
1.03 |
1.68 |
NM properties |
NP core |
Au or Ag2S |
NP primary TEM diameter (nm) |
20, 27 or 80 |
NP stock concentration (mg L−1) |
0.05 |
120 |
Soil properties |
Soils |
5 different soils |
pH |
4.08 |
7.63 |
Sand (%) |
29.5 |
91.8 |
Silt (%) |
4.7 |
47 |
Clay (%) |
3.5 |
14.6 |
d
10
(μm) |
8.7 |
47.2 |
d
50
(μm) |
61 |
603 |
Total carbon (%) |
0.61 |
9.63 |
CEC (cmol kg−1) |
7.8 |
33.3 |
ζ-Potential of dispersible soil fraction calculated from the electrophoretic mobility (mV) |
−44 |
−24 |
ζ-Potential of soil fraction >25 μm calculated from the streaming potential (mV) |
−24 |
−3 |
Oxalate Fe (mg kg−1) |
208 |
2707 |
Oxalate Al (mg kg−1) |
145 |
3005 |
Practically, the effective or total porosity were used, two single collector diameters (d10 or d50) or an approach using the whole grain size distribution were used, five different correlation equations to calculate the single collector contact efficiency (η0), and finally, five different models to calculate α from experimental or modelled data (Table 3). 150 different approaches were thus compared in their efficiency to account for operational parameters and explain α-variance.
Materials
Nanomaterials.
Suspensions of (1) well-characterized, monodisperse, spherical, citrate-coated gold ENMs (gold nanoparticles, Au NPs) were used having a primary (transmission electron microscopy-determined) diameter of 20 nm or 80 nm (high optical density gold nanoparticles, BBI solutions) and (2) previously characterized26 polyvinylpyrrolidone (PVP)-coated Ag2S ENMs synthesized by applied nanoparticles (Spain) having a nominal diameter of 27 nm.
Both Au NPs and Ag2S NPs were selected since Au and Ag backgrounds in natural soils are low and these ENMs are sparingly soluble. Any total gold or silver measurement will thus conveniently reflect occurrence of ENMs. The stock suspensions were diluted in artificial rainwater (Table 2) immediately before injection of ENMs into the soil column. The initial concentration of Au or Ag in diluted stock suspensions was measured after dilution in 3% HCl (Merck, GR for analysis) followed by inductively coupled plasma-mass spectrometry (ICP-MS) measurements. Preliminary experiments verified that the difference between Au concentrations measured in Au ENM suspensions diluted using 3% HCl or Au concentrations measured after total digestion using aqua regia (and further dilution using 3% HCl) was insignificant.
Table 2 Composition of the artificial rainwater used as eluent
Salt |
Concentration (M) |
NaCl |
1.0 × 10−5 |
(NH4)2SO4 |
5.3 × 10−6 |
NaNO3 |
5.9 × 10−6 |
CaCl2 |
3.9 × 10−6 |
Eluent solution.
The OECD 312 test9 recommends using 0.01 mol L−1 CaCl2 as an eluent solution, but preliminary experiments showed that the NPs were aggregating in this eluent. An artificial rainwater representative for Swedish conditions was used instead (Table 2). The pH was 5.1 prior to addition of soil and total ionic strength was much lower (4.4 × 10−5 mol L−1) than the CaCl2 solution. The eluent solution composition was thus chosen to limit the mobilization of clay particles occurring at low ionic strength and at the same time, limit ENM homo-aggregation occurring at high ionic strength.
Soils.
5 different soils were used in the current study (ESI† Table S2). Lufa 2.2 (LUFA Speyer, Germany) is a standard soil with well-known properties. Moreover, Lufa 2.2 has been used extensively in studies of ENMs and soil contaminants and represents a textural class common among agricultural soils. This soil has therefore been proposed as a standard material to test ENM behavior in terrestrial media.27 The other four soils were sampled from agricultural fields in the southern United Kingdom. All soils were air-dried and sieved <2 mm prior to use.
Soil characterization.
Beyond common soil characteristics, the grain size and ζ-potential were characterized using multiple methods, because attachment efficiency is very sensitive to these paramaters.19,23,25 Soil mineralogy was quantified as well, because it is rarely reported in ENM transport studies but could explain variations in α-values.
Soil solution.
Solutions of the different soils were obtained and used for ζ-potential measurements. Dry, 2 mm sieved soil was dispersed in artificial rainwater at a liquid-to-solid ratio (L/S) of 5 and agitated for 24 hours. This suspension was then filtered using a 0.45 μm membrane filter. Natural colloids were removed by filtering using 3 kDa cellulose acetate ultrafiltration centrifugation tubes prior to measuring the ζ-potential. The ultrafilters were washed twice with artificial rainwater for 20 min at 3000 rpm. Thereafter, the soil solutions were centrifuged during 45 min at 3000 rpm.
Columns.
Glass columns (2.5 cm diameter × 6 cm length, Omnifit® labware) containing filters on both sides of the column were packed with artificial rainwater and soil. The columns were adjustable in size so different column lengths were obtained. The ENM recovery in artificial rain water filled columns with 30 μm PTFE Frit (Omnifit® labware), 10 μm PE Frit (polyethylene, Omnifit® labware) or 70 μm Nylon mesh 30 × 30 cm sheets (Spectrum® Laboratories, Inc.) as end-filters was measured.
Methods
Soil zeta potentials.
The ζ-potential of the water-dispersible soil fraction (ζdispersable) was determined by measuring soil solutions that were transferred in a cuvette used for electrophoretic mobility measurements (Zetasizer Nano ZS, Malvern Instruments, UK), which was converted into apparent ζ-potential by applying the Smoluchowski relationship.28 The Z-average hydrodynamic diameter was also determined in these suspensions using a Zetasizer after 1 hour and after 24 hours confirming that ENM homoaggregation in the soil solutions did not occur.
The ζ-potential of the non-dispersible soil fraction (ζn.d.) was calculated from the streaming potential measured with a SurPASS electrokinetic analyzer (Anton Paar, Austria). For each soil sample, the measuring cylinder was rinsed with the respective soil solution and the fraction <25 μm was removed. Soil samples were then mixed with their respective soil solution and wet-packed into the measuring cylinder equipped with a filter membrane of 25 μm mesh size. The streaming potential measurements were subsequently carried out in the respective soil solution as a background electrolyte. The reported ζn.d. values were calculated based on the Fairbrother–Mastin equation29 and are mean results of at least three individual values.
Soil grain size distribution.
There is no standard method for measuring the grain size of natural soils and therefore different methods are used during column studies with natural soils. The comparability of two different methods for determination of the grain size distribution of soils were therefore investigated: using dry-sieving or laser diffraction on soil suspended in artificial rain water. 500 g soil was dry-sieved through 44, 74, 105, 149, 250, 354, 590 and 1000 μm stacked sieves for 90 minutes. It was found that longer sieving times did not result in significant changes in the determined grain size distribution. Prior to laser diffraction measurements, 10 g dry soil was immersed in 100 mL artificial rainwater and this suspension was shaken for 10 minutes. The grain size was then measured without sonication while circulating the suspension through a Horiba laser scattering particle size distribution analyzer LA-950, where the particle size distribution was measured using static light scattering.
Soil mineralogy.
The <2 mm soil fraction was wet ground for 12 minutes (in ethanol or water) in a McCrone mill and spray dried to produce random powder specimens (Hillier 1999). X-ray powder diffraction (XRPD) patterns are recorded from 4–70° 2θ using copper Kα radiation. Quantitative analysis is made by a normalised full pattern reference intensity ratio (RIR) method as described in Omotoso et al.30 The expanded uncertainty on the concentration in weight% using a 95% confidence, is given by ±0.35.31 Clay fractions were obtained by timed sedimentation, prepared as oriented mounts using the filter peel transfer technique and scanned using copper Kα radiation from 3–45° 2θ in the air-dried state, after glycolation, and after heating to 300 °C for one hour. Clay minerals identified were quantified using a mineral intensity factor approach based on calculated XRPD patterns. Unless otherwise stated, for clay minerals present in amounts >10%, the uncertainty was estimated as ±5 wt%.
Heteroaggregation in soil solution.
The occurrence of heteroaggregation of ENMs was investigated using 0.45 μm filtered soil solutions that were mixed with ENM suspensions at different volumetric ratios (1
:
10; 1
:
100 and 1
:
1000) and were agitated for 1 hour. The resulting suspensions were analyzed using single particle ICP-time of flight-MS (sp-ICP-TOF-MS, TOFWERK, Switzerland)32 using an integration time of 3 ms. These ENM concentrations were much higher than those used during the column tests (Table 1) and the natural colloid concentration in saturated soil solution was higher than in column eluates. However, using these more concentrated particle suspensions reduced the probability of detecting false positives. Reducing this probability below 10% requires detection of at least 1538 particles.33 Particle signals were defined based on a threshold that was calculated as average + 3.29 × σ + 2.72 as explained in detail in ref. 34. Heteroaggregates were assumed where Au particle signals co-occurred with Al or Fe particle signals within a single integration time of 3 ms.
Column packing.
Column packing was done as recommended by Oliveira et al.35 to achieve 100% water saturation of the columns. First, artificial rainwater was added to the empty column achieving a water layer of ca. 1–2 mm above the bottom end-piece in the column. Dry soil was subsequently added in such an amount that all soil was moistened. Thereafter, rainwater and soil were alternately added in similarly low amounts until the whole column was filled. At each turn, the column was gently tapped to allow entrapped air to escape. Beakers containing rainwater and soil were weighed before and after the complete packing of the column, as well as a waste beaker placed under the column, to be able to calculate the total pore volume (including effective and occluded pore volume), and total (bulk) density of the column. The length of the column filled with soil was measured to obtain the column volume. To confirm that the columns were saturated, i.e., packed with an absence of air, selected columns were analysed using X-ray tomography (50 μm resolution, GE Phoenix v|tome|x m XRT). The 3D structure of the column was constructed using an ImageJ plugin36 visualizing air-filled pores.
Elution.
Packed columns were connected on the bottom to a peristaltic pump and on the top to an on-line conductivity meter (isoPod, eDAQ) to monitor electrical conductivity and breakthrough of the inert tracer as well as a flow meter (TruFlo™ sample monitor, glass expansion) to verify flux at the column inlet. Injection of eluent (artificial rain water) occurred from the bottom of the column up with a constant pump rate until steady-state, i.e. the change in turbidity and electrical conductivity was no longer significant. This guaranteed relatively constant conditions during inert tracer and ENM elution. The used pump rates (Table 1) correspond to rainfall intensities of 24 mm h−1 to 73 mm h−1, respectively. Upon reaching steady-state, the eluent was switched to a 10 mM NaNO3 solution as inert tracer. It was found that this concentration provided enough conductivity above the background during all column experiments. A total of two total pore volumes of the inert tracer solution was added after which artificial rainwater was added again to reobtain the steady-state background (in term of conductivity). Subsequently, two pore volumes of an ENM suspension having a known concentration C0 (Table 1) were added to the column. A pulse addition rather than a step input of ENMs was used to avoid, as much as possible, ENM processes occurring at relatively high concentrations in the soils such as blocking and ripening or even pore clogging. Such processes would unrealistically complicate the processes occurring in soils thus preventing an accurate estimation of α. CFT builds on the assumption that irreversible attachment is the only process limiting ENM transport. The total ENM concentrations (Table 1) were still lower than concentrations for which blocking and ripening of ENMs have been hypothesized.8 Directly after adding the ENM suspension to the column, the column leachate was collected in 3 to 5 mL-batches using a fraction collector (Gilson instruments). After 2 pore volumes of ENM suspension, the eluent was again switched to artificial rain water and eluent was collected for at least 4 additional pore volumes.
Effect of different porosity parameters.
Both the models used to interpret BTCs in Table 3 and the different correlation equations to calculate η0 (found in ESI†) have porosity and grain size as parameters. Some column studies have used the total porosity (θtotal) for calculating α. θtotal is the fraction of total saturated column volume occupied by water and is obtained from the known volume of water added to the column during packing. The effective porosity (θeffective) is found during fitting a transport model to the inert tracer BTC data as described further. These two porosity values are never equal, because θtotal also includes pore space not accessible to ENM transport. We have used both throughout a calculation to compare the effect of porosity parameters on the final α value.
Effect of different gran size parameters.
Grain size is similarly taken into account differently during column studies with ENMs. These studies have predominantly occurred using media with a relatively uniform grain size, justifying the use of a single diameter as a characteristic size of the porous medium. Soils do not have a uniform grain size, but the size distribution is nevertheless nearly always represented by a single representative diameter when calculating α-values. In many cases this value is d50, the diameter for which 50% of the total soil mass has lower diameter. d10, the diameter for which 10% of the soil mass has lower diameter, has, however, been shown to better represent the grain size distribution for the purpose of colloid retention calculations.53,54 We therefore used d10 or d50 during α calculation to investigate which diameter is more representative for modelling ENM behavior. We also sought to investigate whether the higher heterogeneity of natural soils could be taken into account based on the measured grain size heterogeneity. The full distribution of the grain size was therefore used in a third approach. α was calculated for each measured diameter and the final value of α was an average weighed by the relative volume of each diameter.
Models.
Table 3 shows the models used to interpret column BTCs. These are essentially solutions of different convection–dispersion equations (CDE) that vary mainly in the way ENM attachment is calculated, reflecting different assumptions on the dominating retention mechanisms. The continuous, pulse and attachment models assume that attachment is irreversible only, i.e. the most used assumption.2 The straining model assumes only irreversible straining occurs. Even though the ENM diameter
:
collector diameter ratio is much smaller than 0.005,37 ENMs might still be strained on rough surfaces and/or because of the occurrence of ENMs homo- or heteroaggregates which increases the colloid
:
collector diameter ratio.37–39 Finally, the 2-sites model assumes both irreversible and reversible attachment that very often fits BTCs of ENMs well (e.g. ref. 5). Colloids can detach from soil surfaces soil columns when these particles were previously attached in secondary energy minima40 and the probability of detachment increases with particle size because of hydrodynamic torque.41 Previously homo- or heteroaggregated ENMs could thus also experience similar torques. No blocking of sorption sites was assumed while modelling transport of ENMs in the soil columns given that the ENM concentrations were kept purposely low to avoid blocking phenomena. The models in Table 3 have in common that they are relatively simple and only one or in the case of the 2-sites model, three parameters have to be fitted to the BTC.
Calculation from ENM recovery.
α is calculated from the integrated recovery of the ENM mass after column elution (R) in the continuous and pulse models as: | 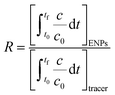 | (1) |
where C0 and C are the concentration of Au or Ag, respectively tracer in the spiking solution (C0) and column outflow (C) at the time t. t0 and tf are the beginning and end-times of the BTC. The continuous model assumes a step ENM input is used, whereas the pulse model assumes a pulse input using the formula of Valocchi42 to calculate α (Table 3). Column studies use either a pulse or a step input, but the minimum number of pore volumes required to achieve a step input instead of a pulse input is not generally agreed upon. We therefore investigated whether using either approach affects the final value of α. The pulse model requires the dispersivity, which was obtained from modelling the inert tracer BTC using a zero interaction CDE using Hydrus 1D.43
Model fitting.
α is calculated from different attachment rate constants in the attachment, straining and 2-sites models. These constants were obtained by fitting solutions of the CDEs in Table 3 to ENM BTCs using Hydrus 1D.43 Hydrus 1D calculates an objective function from the experimental and modelled data and minimizes this function using the Levenberg–Marquardt nonlinear minimization method.44D and θeff are the only variable parameters that are found by fitting a non-reactive solution transport function to the inert tracer BTC. These two parameters were fixed in subsequent fitting of the attachment, straining or 2-sites models to the ENM BTCs where the attachment rate constants were variable parameters. A constant water flux boundary condition was set both at the column inlet and outlet during all models including those for the inert tracer, because the flux at the column inlet was enforced by the peristaltic pump and the outlet flux was measured continuously and found to be relatively constant and equal to the inlet flux. Moreover, a saturated flow was always assumed (and verified, see results). The specific discharge (q) (expressed in cm s−1) was calculated from the flow rate Q according to q = Q/A where A is the column cross sectional area, thus assuming full saturation.
A concentration flux (i.e. Cauchy) boundary condition was set for both column inlet and outlet, both for models for inert tracer and ENM transport, because such conditions are more likely to preserve mass.45 The infiltrating concentration at the column inlet (z = 0) was set at the known concentration of inert tracer or ENM for the duration of the pulse injection (dead time added) and zero at other times, whereas the infiltrating concentration at the column outlet was set at zero.
The depth dependent factor ψ in the “straining” model was calculated as:
| 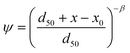 | (2) |
where
x0 is the coordinate of the location where the straining process starts,
x is the depth in the column and
β is an empirical factor set to 0.43 in this work, as proposed by Bradford
et al.37d50 was used in
eqn (2) regardless of whether
d10,
d50 or the whole grain size distribution were used in other calculations, as described further.
Correlation equations.
α is finally calculated from the calculated or fitted first-order interaction rate constants shown in Table 3. Table 3 shows that these calculations require the single-collector contact efficiency (η0) that can be calculated in different ways. Five different correlation equations were used toη0: Tufenkji and Elimelech46 (symbol “TE”), Long and Hilpert47 (symbol “LH”), Ma et al. (2010)48 (symbol “MA2010”), Nelson and Ginn49 (symbol “NG”) and Ma et al. (2013)50 (symbol “MA2013”). The relevant equations are given in ESI.† The TE correlation equation is used most, but we investigated whether other, more recently published, correlation equations would perform better in explaining the variance in α. η0 calculation requires the Hamaker constant which was set to 1.72 × 10−19 J and 9.23 10−20 J for Au (ref. 51) and Ag2S (ref. 52) respectively, assuming a predominant SiO2–ENM interaction.
Statistical analysis
Principal component analysis (PCA) was applied to explore covariance between predictor variables and observe clustering of experimental data. Predictor variables were logarithmically transformed if this reduced skewness and, based on a Kolmogorov–Smirnov test, would produce a normally distributed variable. PCA was done on z-scores of transformed predictor variables (see ESI†) using Minitab (version 18). Partial least squares analyses (PLS1) of α-values produced by the different methods was done using Matlab (v2020), which uses the SIMPLS algorithm.55 The ESI† provides background and terminology of PLS1. All α-values were logarithmically (base 10) transformed to reduce skewness and z-scores were calculated. The same transformed z-scores of the predictor variables used for PCA were also used for PLS1. The optimum number of latent variables was found by cross-validation (one-observation out, see ESI†) retaining the number of latent variables that resulted in the lowest squared mean error of prediction. A high number of predictor values tends to spoil PLS analyses reducing their consistency and introducing unnecessary variance.56 The number of predictor variables was therefore reduced by removing intercorrelating variables followed by calculation of the variable importance in projection (VIP) of each variable (see ESI†).
Results and discussion
Effect of filters and air-entrapment
Filters.
Using a PE filter with 10 μm pore size or a PTFE filter with 30 μm pore size in the column end pieces resulted in very low mass recoveries of resp. 26.4% and 0.2% of the 80 nm Au ENMs in Lufa 2.2 columns. Parallel experiments with the same filters and ENMs, but using columns filled with only artificial rainwater resulted in resp. 21.7 and 6.2% recovery, whereas in the case of the Nylon filters with 70 μm pore size, close to 100% of the ENMs was recovered during the experimental time whether or not soil was present in the columns. The choice of filters used in end pieces of soil columns are rarely reported, but was found here to significantly affect the recovery of ENMs, and thereby the estimated α-values. Poor recoveries of particles passing through filters having much larger pores owes to a polydisperse membrane pore size distribution where ENMs are strained in small pores or to particle-membrane interactions.57,58 End-filter recovery must therefore be tested at all times before starting column experiments to avoid overestimating ENM attachment in the column due to ENM-filter interactions.
Air entrapment.
Fig. 1 shows a 3D reconstruction of the X-ray tomography analysis. Fig. 1(left) shows that a significant amount of air is present in soil columns packed using a PTFE filter having a pore size ≤30 μm. The air also follows a spiral pattern. Torsion is applied to the soil column when the top end-piece is screwed on after column packing and this process may create preferential pores where entrapped air bubbles are known to accumulate.59 The amount of air is clearly reduced and the spiral patter disappeared when 70 μm Nylon of filters were used instead (Fig. 1-right). Air bubbles pass membranes with larger pores comparably easier.60 The presence of air interfaces drastically enhances ENMs retention in porous media61,62 and may thus also explain the large difference in recovery during column tests. The presence of air in columns was moreover unreproducible and can thus not be considered when calculating α-values. Air removal from columns intended to be saturated may also occur by using CO2 filled columns or degassed water,35 but these measures are rarely taken during column tests involving ENMs and absence of air in the soil has never been confirmed as in this study. Even though full air removal was not fully obtained in this study, the larger pore sized Nylon filter was still used further in column experiments. Near 100% recovery of ENMs was obtained in many cases (ESI† Table S6), which suggests further artefacts caused by air–water interfaces seldom occurred.
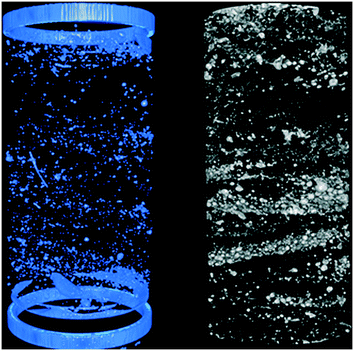 |
| Fig. 1 Figures obtained from X-ray tomography presenting the distribution of air in packed Lufa 2.2 soil columns using a 30 μm PTFE (left) or a 70 μm Nylon (right) membrane followed by 2 pore volumes of rainwater. The presence of air is shown in white or blue. The blue rings in the right figure correspond to air trapped in the end-pieces. | |
Soil properties
Surface potentials and mineralogy.
Fig. 2 shows the loading and score plots relative to the first two principal components of the PCA analysis of the predictors. The first component explained 44% of the variation and is leveraged mostly by the granulometric sand and clay contents and ζn.d.. The loading plot shows that the sand and clay content are correlated and both are inversely correlated with ζn.d.. The sand content appears closely correlated with the quartz content, the charge density of which tends to be relatively low, explaining poor correlation with ζn.d.. Conversely, log10(oxalate extractable Al) was very strongly correlated with ζn.d. (r2 = 0.93) (ESI† Fig. S2a). Oxalate extracts predominantly amorphous aluminum minerals that form coatings on other minerals such as quartz grains.63 Aluminum coatings have a relatively high point of zero charge and thus introduce positive charge in otherwise negatively charged mineral surfaces. Given that the ENMs were negatively charged, they would preferentially interact with positively charged patches on the pore surfaces.64ζn.d. had the highest PCA score and was retained for PLS1 analysis, whereas the covarying predictors sand, quartz, CEC, oxalate extractable aluminum and clay content were not included in subsequent PLS models. ζdispersable was more negative than ζn.d. and correlated strongly with the 2
:
1 clay content. Mobile soil colloids often consist of 2
:
1 clays because these minerals have a relatively high surface charge lending them a high stability, especially in the conditions of this study where ionic strength was relatively low.65
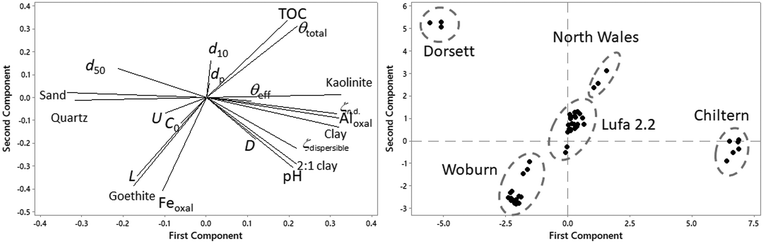 |
| Fig. 2 Loading and score plot of the principal component analysis of predictors. The core plot indicates also the soils for which the data was obtained. Symbols used in the load plot can be found in ESI† Table S1. Abbreviations: sand: granulometric sand % of the soil; clay: granulometric clay% of the soil; Feoxal: oxalate extractable iron; Aloxal: oxalate extractable aluminum; ζdispersible: ζ-potential of the dispersible fraction; ζn.d.: ζ-potential of the non-dispersible fraction; TOC: total organic carbon. | |
Mostly oxalate extractable Fe and TOC leverage the second principal component that explains 23% of the total variation. Oxalate extractable Fe and TOC appear not to correlate strongly with the ζ-potentials, possibly because iron oxides are less efficient than amorphous aluminum minerals in increasing the point of zero charge of silica63 and do not affect ζn.d to the same extent than amorphous aluminium minerals. The apparent opposite relation between oxalate extractable Fe and TOC could not be explained. Both parameters were therefore retained for PLS analysis. The goethite concentration was not retained, because oxalate partly extracts goethite.
Grain size.
d
50 appears to be somewhat correlated to the sand content, but is uncorrelated with d10. The grain size distributions measured using light scattering or dry sieving method provide very similar grain size distributions and d10 and d50 values except for the Woburn and Chiltern soils (ESI† Fig. S3). Light scattering was intense for the smallest particle sizes of the Woburn soil resulting in a much lower d10 value than calculated from the dry sieving results (ESI† Fig. S2) where such small particles were not detected (ESI† Fig. S3). Given that texture analysis did not detect an unusually high concentration of clay-sized particles in Woburn soil (ESI† Table S2), the small particles detected in Woburn suspensions using light scattering were assumed to be mostly noise. The d10-value of dry sieving of this soil was therefore much lower than the one obtained using dry sieving. Chiltern soil contains a very high calcite concentration (ESI† Table S2). Possibly a significant number of large calcite aggregates resuspended in the liquid medium used during light scattering analysis resulting in comparably lower d50-values measured using light scattering. The grain size has a strong influence on attachment,23,25 but both d10 and d50 have been used to represent the grain size of soils. Both parameters were therefore used during PLS1 analysis and the dc-values obtained by light scattering were used during PLS analysis, because soil grains size as suspended in an aqueous medium was deemed more relevant for packed saturated columns than dry sieved grains. The dry-sieving d10 value was used for the Woburn soil.
Porosity.
The variation of the total porosity (θtotal) seems to be unrelated to the effective porosity (θeff) (see also ESI† Fig. S2). θeff quantifies only the accessible pore space because it is modelled based on the inert tracer BTC and should thus be systematically lower than θtotal. θeff appears in many cases to be higher than θtotal, however, mostly during column experiments with Woburn soil (ESI† Fig. S1). This soil may not have been sufficiently dry in many cases thus underestimating θtotal. Moreover, the possible inaccuracy of θtotal measurements is reflected by unrealistic values as high as 69% obtained in some cases.
Heteroaggregation
Heteroaggregation of ENMs with naturally occurring clays or oxides was limited or inexistent. It is often stressed that heteroaggregation is an important mechanism in environmental media, including soils,2,67 but for the natural soils studied here and the relatively low ionic strength of the aqueous medium used, homo- or heteroaggregation did not occur. sp-ICP-TOF-MS analysis of saturated soil solutions mixed with ENM suspensions resulted in most cases in less than 10% co-occurrences of Au or Ag particle signals with those of Al, Fe or Si (ESI† Table S3). Mixing ENM suspensions with more diluted saturated soil solution did not consistently increase the number of co-occurrences and the fraction of observed co-occurrences was in most cases lower than the probability of random co-occurrences of these particle events (ESI† Fig. S4).
The ζ-potential of ENMs in ultrafiltered soil solution varied between −22.8 and −48.1 mV. As discussed earlier, all soils contain a significant concentration of positively charged surface sites with which the negatively charged ENMs interact. However, dispersible natural colloids are most likely 2
:
1 clays in these soils as discussed earlier. The highly negative surface potential of these colloids is reflected by ζdispersed that is significantly more negative than ζn.d. that reflects the surface potential of pore walls (ESI† Table S2). The overall electrostatic repulsion of ENMs from dispersed clay particles is thus stronger than from soil pore walls. This explains why ENMs were found to attach to soils as reflected by significant attachment rates, but did not form heteroaggregates with natural colloids. Lack of interaction of gold ENMs with stable natural soil colloids has been observed before66 and contradicts the common assumption that heteroaggregation dominates the fate of ENMs in all environmental media.2,67 Absence of heteroaggregation also confounds support for the straining model (Table 3) given that the individual ENMs are too small to experience significant straining.
Method comparison to calculate α
Porosity parameters.
Fig. 3 shows that α-values calculated using either total or effective porosity differed less than an order of magnitude. The α-values were as a whole somewhat higher when calculated based on total porosity, but there were also relative differences between samples.
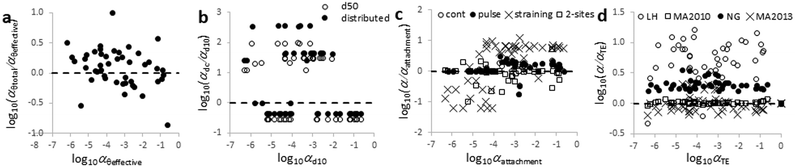 |
| Fig. 3 Selected comparisons of different approaches to calculate α. a) Using different correlation equations compared to using the TE equation while using the katt model, effective porosity and a single d50 b) using different models compared to using the attachment model while using the TE equation, effective porosity and a single d50 c) using the total versus effective porosity while using the TE equation, the attachment model and a single d50 d) using a single d50versus the whole grain size distribution while using an attachment model, the TE equation and effective porosity. | |
Grain size parameters.
Using d10 or d50 did not result in systematically different α values. There is indeed no reason why these two different grain size parameters should correlate strongly. α-Values calculated using the full grain size distribution, however, are systematically higher than those calculated with d50. Sensitivity analysis of α calculated using CFT suggests that these values should increase particularly with increasing grain size.23 Grain sizes larger than d50 thus have a proportionally larger weight on α, leading to somewhat higher values when the calculation is based on the whole distribution.
Models.
Fig. 3c compares α values obtained using different models, but many values were omitted for the continuous and pulse models given that their negative α-values could not be log-transformed. Moreover, the 2-sites model provided α values lower than 10−3 that are not visible in Fig. 3 where the scale was limited to make other differences clear.
The continuous, and pulse models provided similar α values, because relatively low dispersivity values were obtained likely owing to the relatively short columns that were used in this study.69 Moreover, the two-pore volume ENM injections were in many cases long enough to reach plateau values for the BTCs explaining why the continuous or pulse model generated similar results. At the same time, relatively low values calculated with the continuous or pulse models were often negative when recovery was not determined accurately.
Low α-values of the attachment model varied with the subjectively chosen starting katt value of iterative fitting explaining the large differences at relatively low α-values.
α-Values calculated using the straining model were linearly related to those of the attachment model as log10(αstraining) = 1.23 log10(αattachment) + 0.96 (r2 = 0.92). This relation can likely be related mathematically to eqn (2). Straining is an unlikely mechanism in these soil columns as argued before.
The 2-sites model often generated α values similar to the continuous, pulse and attachment models, but kirrev values 10−10 s−1 were sometimes fitted. The 2-sites model requires simultaneous fitting of three parameters, a procedure that may inflate parameters reducing their accuracy. Detachment was negligible in most experiments except for the Woburn soil where BTCs showed significant tailing and the 2-sites model fitted much better (ESI† Fig. S5–S8). However, even in the case of Woburn soil, irreversible attachment rate constants (katt,irrev) were orders of magnitude higher than the detachment rates, which is often the case for ENMs.19
Correlation equations.
Fig. 3d shows that α values calculated using different correlation equations decrease systematically in the order LH > NG > MA2010∼TE > MA2013. η0 values should therefore decrease in the reverse order following the formulae in Table 3. Molnar et al.68 indeed found that the η0 of nanoparticles attaching in favorable conditions (α = 1) decreased as MA2010 > TE > NG > LH, i.e. the reverse order. Nelson and Ginn49 predicted the order as TE∼MA2010 > LH > NG. The LH correlation equation appeared to cause non-systematic changes between samples compared to other correlation equations.
PLS1 analysis
General observations.
Simple linear regression between different α-values and parameters yielded only poor relations, highlighting the complex interrelation of the many factors that determine α. This justifies the PLS1 as a method to investigate the factors influencing α-values. Fig. 4 summarizes the fraction of total variance in α calculated using different approaches that could be explained by different PLS1 models. Only one latent variable was optimal or only an additional maximum 10% of the variation was explained by adding more latent variables across approaches. The weights relative to the first latent variable summarized in Fig. 5 thus express the relative importance of different variables to explain variation of α. The variable importance in projection was also used to express the weight of different variables, but this analysis resulted in the same conclusions. ESI† Table S5 shows the full PLS1 results.
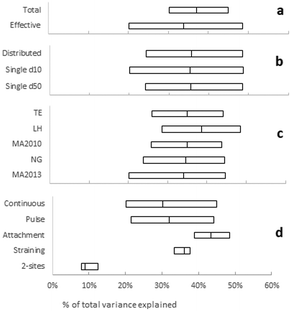 |
| Fig. 4 Averages and ranges of the total % of variance explained by PLS1 models comparing a) using θtotal or θeffective over all except the 2-site models; b) using different collector diameters over all except the 2-sites approaches c) using different correlation equations over all except the 2-sites models d) using different models. | |
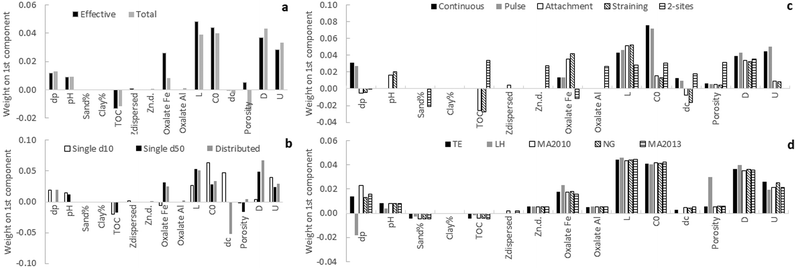 |
| Fig. 5 Average weights of different parameters on the first latent variable of PLS models for α values calculated in different ways: a. using either effective or total porosity, across all different dc, across all models except 2-sites and across all correlation equations. b. Using only effective porosity, d10, d50 or the whole distribution, across all models except 2-sites and across all correlation equations. c. Using only effective porosity, across all different dc, one of five calculation models and across all correlation equations. d. Using effective porosity, all different dc, across all calculation approaches and across all correlation equations. | |
In the ideal case, variations in α should only depend on variations in ENM- and soil properties (dp, pH, sand or clay content, TOC, ζdispersed, ζn.d., oxalate extractable Fe and Al) and the formulae in Table 3 (and ESI†) should remove any residual effect of operational parameters (L, C0, dc, θ, D, U). The methods were therefore evaluated qualitatively in terms of how much variance could be explained by soil or ENM properties and the lack of influence of operational parameters.
Fig. 4 shows that the fraction of variation explained varies between 8% for most approaches that are based on a 2-sites model and maximum 49% for an approach using an effective porosity, a single d10, the LH correlation equation in combination with the attachment model.
2-sites models were in many cases only marginally influenced by operational parameters (Fig. 5), but approaches using this model consistently explained a low fraction of the total variance (Fig. 4). This model fitted BTCs often best (ESI† Fig. S5–S8), but the simultaneous fitting of three parameters may reduce accuracy of the fitted parameters relatively to more simple models with only one parameter. 2-Sites models were therefore no longer considered in further analyses.
Effect of porosity parameters.
Using either total or effective porosity did not have a significant effect on the average fraction of total variance that was explained (Fig. 4). α-Values based on effective porosity were somewhat more influenced by ENM and soil properties and somewhat less by operational parameters, but the difference is too small to assert that using effective porosity is a superior approach (Fig. 5). Porosity appears only in some approaches as a parameter determining α-variation (ESI† Table S5). It did not play a role whether this was effective or total porosity. This is unexpected, because these parameters are not correlated (Fig. 2 and ESI† Fig. S2) and Fig. 3 shows a non-systematic difference in the α-values calculated with either porosity.
Effect of grain size parameters.
α-Values based on d50 were preferred over approaches using d10 for the purpose of this study. At first sight, using different ways of accounting for grain size do not seem to have a significant effect on the fraction of explained variance (Fig. 4). However, operational parameters leverage α values differently upon using different grain size parameters (Fig. 5). Column length and dispersivity seem to be influential operational parameters in most approaches using a d50 or the full distribution, but not in approaches using d10 in combination with the continuous or pulse models. Column length is known to affect dispersivity,70 but these variables were orthogonal in this study (Fig. 2). d10 possibly represents hydraulic properties of heterogeneous media better as suggested before,53,54 but all approaches using d10 were instead influenced by C0, the value of d10 and the approach velocity. In fact, operational parameters generally leveraged approaches based on d50 less. These α-values were moreover not leveraged by the value of grain size itself.
Effect of models.
The attachment model performed best in terms of producing α-values that correlate mostly with soil characteristics. Increasing C0 increases the calculated α-values of the continuous and pulse models (Fig. 4). These methods rely on calculating the recovery of NMs and thus require an accurate C0 determination. We often obtained relatively high recoveries. Measurement inaccuracies on C0 were thus more likely to lead to significant errors in the value of recovery making the α-values still dependent on this value. Other models are based on rate constants that are not obtained from recovery but via iterative fitting. These rate constants are thus sensitive to the shapes of the BTCs but not to recoveries. C0 therefore had lower (d10-based approaches) or zero weights on the α-variation for the attachment and straining models compared to the continuous and pulse models. Attachment and straining models performed similarly well in terms of the effect of operational parameters (Fig. 5), possibly because kstraining rate constants were linearly related to katt rate constants. The attachment model, however, explained a higher fraction of the α variation (Fig. 4) and was therefore preferred. Moreover, there is no experimental support for straining as a generally occurring mechanism for retention of small particles such as ENMs. When comparing only approaches using an attachment model in combination with a d50 to represent grain size, it appears that using an effective porosity during the calculation removes the additional effect of porosity on α-variation. Approaches using effective porosity were therefore preferred.
Effect of correlation equations.
The different correlation equations performed similarly in terms of the% of variance that could be explained (Fig. 4) and the number of operational parameters that were retained as influential (Fig. 5). The LH correlation equation performed somewhat poorer as the α-values calculation using this equation were additionally influenced by porosity in some cases. When effective porosity was used, d50 represented grain size, and an attachment model was used the LH correlation equation explained the highest fraction of total variation (49%), of any approach, but these α-values were also influenced by porosity. Using other correlation equations explained between 42 and 43% of total α-variation and ENM, soil and operational parameters similarly leveraged this variation. The accuracy of correlation equations is best for experimental conditions in which they were developed.68 The LH equation was derived based on experimental data of particles larger than 100 nm,47 whereas the other equations were developed using particles having diameters as small as 10 nm. We therefore suspect the LH equation is somewhat less applicable for calculating α-values of ENMs in natural soils.
Effect of soil characteristics.
pH, TOC, oxalate extractable Fe similarly leverage α-values calculated using effective porosity, d50 to represent grain size, using an attachment model and any correlation other than LH. While this relation should not be over-interpreted as it is only valid for the 5 soils studied, it may be argued that amorphous iron oxide coatings extracted by oxalate in particular form small patches of local positive charge that significantly increase the attachment efficiency.71 These local charges are not measurable using the ζn.d., because the latter parameter varies with the overall grain charge, which is predominantly negative. ζn.d. may therefore not have leveraged α-values, even though a previous meta-analysis found this parameter highly influential on katt values obtained from ENM saturated column tests.19 A higher TOC potentially also leads to a higher concentration of dissolved organic acids that may coat positively charged patches as well as ENMs thus reducing their mutual affinity resulting in a lower α-value as also found here. A positive trend of α-values with pH is observed but was not expected, because the charge of local positively charged patches decreases with pH, a process that should rather decrease α-values.
Conclusions
Our study can contribute to developing a standard protocol to obtain fate descriptors of ENMs for natural soils, because we identified several potential artefacts and how to avoid these. A standard column assay should always verify whether saturated conditions have been attained and whether the filter material at the column inlet and outlet does not retain ENMs. We also recommend to represent the soil grain size by its average diameter (d50) during α calculations. This value should be obtained from the grain size distribution measured in aqueous suspensions of the soil. Using a low ionic strength medium during column tests minimizes homo- and heteroaggregation. This is important to support the assumptions of relatively simple models to calculate α, models that are based on CFT where attachment to pore walls is the only assumed mechanism.
We were not able to recommend a final experimental and theoretical approach to calculate α-values that are not influenced by operational parameters. An approach that used effective porosity (rather than total porosity), d50 to represent grain size, and the attachment model produced, in the context of this study, α-values that were least affected by operational parameters. This approach requires determination of the effective porosity and dispersivity as well as numerical fitting of a katt reaction rate constant. α determinations using effective porosity and dispersivity require a prior inert tracer test to ascertain the hydrodynamic properties of the saturated column test. Adopting these recommendations when exploring systematic effects of natural soils and ENM properties will minimize experimental artefacts thus increasing the likelihood that significant trends between α-values and soil characteristics are observed. However, the low fraction of total variance explained and the residual effect of column length and dispersivity calls for further standardization efforts, preferably cross-validating α-values with a recently developed batch method for determining α-values in natural soils.72
Conflicts of interest
There are no conflicts of interest to declare.
Acknowledgements
This research was funded by the EU Horizon2020 project NanoFASE (Nanomaterial Fate and Speciation in the Environment; grant no. 646002) and the EU's 7th Framework Programme project GuideNANO (FP7/2007–2013; grant no. 604387), as well as The Swedish Research Council (grant no. 2012-03937). The authors thank Dr. Johannes Koestel for the X-ray tomography measurements and corresponding image processing.
References
- V. Micić, N. Bossa, D. Schmid, M. R. Wiesner and T. Hofmann, Environ. Sci. Technol., 2020, 54, 1250–1257 CrossRef PubMed.
- C. Svendsen, L. A. Walker, M. Matzke, E. Lahive, S. Harrison, A. Crossley, B. Park, S. Lofts, I. Lynch, S. Vázquez-Campos, R. Kaegi, A. Gogos, C. Asbach, G. Cornelis, F. von der Kammer, N. W. van den Brink, C. Mays and D. J. Spurgeon, Nat. Nanotechnol., 2020, 15, 731–742 CrossRef CAS PubMed.
- A. Praetorius, N. Tufenkji, K.-U. Goss, M. Scheringer and F. Von der Kammer, Environ. Sci.: Nano, 2014, 1, 317–323 RSC.
- G. Cornelis, Environ. Sci.: Nano, 2015, 2, 19–26 RSC.
- G. Cornelis, L. Pang, C. Doolette, J. K. Kirby and M. J. McLaughlin, Sci. Total Environ., 2013, 463–464, 120–130 CrossRef CAS PubMed.
- E. Goldberg, M. Scheringer, T. D. Bucheli and K. Hungerbuhler, Environ. Sci. Technol., 2014, 48, 12732–12741 CrossRef CAS PubMed.
- M. Baalousha, G. Cornelis, T. Kuhlbusch, I. Lynch, C. Nickel, W. Peijnenburg and N. van den Brink, Environ. Sci.: Nano, 2016, 3, 323–345 RSC.
- P. Babakhani, J. Bridge, R.-A. Doong and T. Phenrat, Adv. Colloid Interface Sci., 2017, 246, 75–104 CrossRef CAS PubMed.
-
OECD, Test No. 312: Leaching in Soil Columns, OECD Publishing, 2021 Search PubMed.
- D. Kühnel and C. Nickel, Sci. Total Environ., 2014, 472, 347–353 CrossRef PubMed.
- N. Saleh, H. J. Kim, T. Phenrat, K. Matyjaszewski, R. D. Tilton and G. V. Lowry, Environ. Sci. Technol., 2008, 42, 3349–3355 CrossRef CAS PubMed.
- X. Liu, M. Wazne, C. Christodoulatos and K. L. Jasinkiewicz, J. Colloid Interface Sci., 2009, 330, 90–96 CrossRef CAS PubMed.
- Y. G. Wang, Y. S. Li and K. D. Pennell, Environ. Toxicol. Chem., 2008, 27, 1860–1867 CrossRef CAS PubMed.
- X. Liu, D. M. Carroll, E. J. Petersen, Q. Huang and C. L. Anderson, Environ. Sci. Technol., 2009, 43, 8153–8158 CrossRef CAS PubMed.
- R. L. Johnson, G. O. B. Johnson, J. T. Nurmi and P. G. Tratnyek, Environ. Sci. Technol., 2009, 43, 5455–5460 CrossRef CAS PubMed.
- D. P. Jaisi and M. Elimelech, Environ. Sci. Technol., 2009, 43, 9161–9166 CrossRef CAS PubMed.
- R. Doshi, W. Braida, C. Christodoulatos, M. Wazne and G. O'Connor, Environ. Res., 2008, 106, 296–303 CrossRef CAS PubMed.
- A. J. Pelley and N. Tufenkji, J. Colloid Interface Sci., 2008, 321, 74–83 CrossRef CAS PubMed.
- P. Babakhani, J. Bridge, R.-A. Doong and T. Phenrat, Water Resour. Res., 2017, 53, 4564–4585 CrossRef.
- J. Lewis and J. Sjöstrom, J. Contam. Hydrol., 2010, 115, 1–13 CrossRef CAS PubMed.
- J. Lewis and J. Sjöstrom, J. Contam. Hydrol., 2010, 115, 1–13 CrossRef CAS PubMed.
- S. Banzhaf and K. H. Hebig, Hydrol. Earth Syst. Sci., 2016, 20, 3719–3737 CrossRef CAS.
-
S. Loureiro, P. S. Tourinho, G. Cornelis, N. W. Van Den Brink, M. Díez-Ortiz, S. Vázquez-Campos, V. Pomar-Portillo, C. Svendsen and C. A. Van Gestel, in Soil Pollution, Academic Press, 2018, pp. 161–190 Search PubMed.
- J. Fang, X. Q. Shan, B. Wen, J. M. Lin and G. Owens, Environ. Pollut., 2009, 157, 1101–1109 CrossRef CAS PubMed.
- J. Ren, A. I. Packman and C. Welty, Colloids Surf., A, 2001, 191, 133–144 CrossRef CAS.
- M. Baccaro, S. Harrison, H. van den Berg, L. Sloot, D. Hermans, G. Cornelis, C. A. M. van Gestel and N. W. van den Brink, Environ. Pollut., 2019, 252, 155–162 CrossRef CAS PubMed.
- N. Geitner, C. Hendren, G. Cornelis, R. Kaegi, J. Lead, G. Lowry, I. Lynch, B. Nowack, E. Petersen, E. Bernhardt, S. Brown, W. Chen, C. de Garidel-Thoron, J. Hanson, S. Harper, K. Jones, F. von der Kammer, A. Kennedy, J. Kidd, C. Matson, C. Metcalfe, J. Pedersen, W. Peijnenburg, J. Quik, S. M. Rodrigues, J. Rose, P. Sayre, M. Simonin, C. Svendsen, R. Tanguay, N. Tufenkji, T. van Teunenbroek, G. Thies, Y. Tian, J. Rice, A. Turner, J. Liu, J. Unrine, M. Vance, J. White and M. Wiesner, Environ. Sci.: Nano, 2019, 7, 13–36 RSC.
-
R. J. Hunter, Zeta Potential in Colloid Science, Principles and Applications, Academic Press, London, 1988 Search PubMed.
- F. Fairbrother and H. Mastin, J. Chem. Soc., Trans., 1924, 125, 2319–2330 RSC.
- O. Omotoso, D. K. McCarty, S. Hillier and R. Kleeberg, Clays Clay Miner., 2006, 54, 748–760 CrossRef CAS.
-
S. Hillier, in Clay Mineral Cements in Sandstones, ed. R. H. Worden and S. Morad, International Association of Sedimentologists, 2003, Special Publication, vol. 34, pp. 213–251 Search PubMed.
- O. Borovinskaya, B. Hattendorf, M. Tanner, S. Gschwind and D. Günther, J. Anal. At. Spectrom., 2013, 28, 226–233 RSC.
- J. Tuoriniemi, G. Cornelis and M. Hassellöv, Anal. Chem., 2012, 29, 743–752 Search PubMed.
- F. Loosli, J. Wang, S. Rothenberg, M. Bizimis, C. Winkler, O. Borovinskaya, L. Flamigni and M. Baalousha, Environ. Sci.: Nano, 2019, 6, 763–777 RSC.
- I. B. Oliveira, A. H. Demond and A. Salehzadeh, Soil Sci. Soc. Am. J., 1996, 60, 49–53 CrossRef.
- J. Koestel, Vadose Zone J., 2018, 17, 170062 CrossRef.
- S. A. Bradford, J. Simunek, M. Bettahar, M. T. Van Genuchten and S. R. Yates, Environ. Sci. Technol., 2003, 37, 2242–2250 CrossRef CAS PubMed.
- Y. Fujita and M. Kobayashi, Chemosphere, 2016, 154, 179–186 CrossRef CAS PubMed.
- N. Tufenkji, G. F. Miller, J. N. Ryan, R. W. Harvey and M. Elimelech, Environ. Sci. Technol., 2004, 38, 5932–5938 CrossRef CAS PubMed.
- M. W. Hahn and C. R. O'Melia, Environ. Sci. Technol., 2004, 38, 210–220 CrossRef CAS PubMed.
- J. Bergendahl and D. Grasso, Chem. Eng. Sci., 2000, 55, 1523–1532 CrossRef CAS.
- A. J. Valocchi, Water Resour. Res., 1985, 21, 808–820 CrossRef CAS.
- J. Šimůnek, M. T. Van Genuchten and M. Šejna, Vadose Zone J., 2016, 15, 1–25 CrossRef.
-
J. Šimůnek and J. W. Hopmans, in Methods of Soil Analysis, 2002, pp. 139–157, DOI:10.2136/sssabookser5.4.c7.
- F. J. Leij, T. H. Skaggs and M. T. Van Genuchten, Water Resour. Res., 1991, 27, 2719–2733 CrossRef CAS.
- N. Tufenkji and M. Elimelech, Environ. Sci. Technol., 2004, 38, 529–536 CrossRef CAS PubMed.
- W. Long and M. Hilpert, Environ. Sci. Technol., 2009, 43, 4419–4424 CrossRef CAS PubMed.
- H. Ma, J. Pedel, P. Fife and W. P. Johnson, Environ. Sci. Technol., 2010, 44, 4383 CrossRef CAS.
- K. E. Nelson and T. R. Ginn, Water Resour. Res., 2011, 47(5), 1–17 CrossRef.
- H. Ma, M. Hradisky and W. P. Johnson, Environ. Sci. Technol., 2013, 47, 2272–2278 CrossRef CAS PubMed.
- M. Götzinger and W. Peukert, Langmuir, 2004, 20, 5298–5303 CrossRef PubMed.
- J. M. Bennett, J. L. Stanford and E. J. Ashley, J. Opt. Soc. Am., 1970, 60, 224–232 CrossRef CAS.
- M. J. Martin, B. E. Logan, W. P. Johnson, D. G. Jewett and R. G. Arnold, J. Environ. Eng., 1996, 122, 407–415 CrossRef CAS.
- A. A. Porubcan and S. Xu, Water Res., 2011, 45, 1796–1806 CrossRef CAS PubMed.
- S. de Jong, Chemom. Intell. Lab. Syst., 1993, 18, 251–263 CrossRef CAS.
- A. Hoskuldsson, Chemom. Intell. Lab. Syst., 2001, 55, 23–38 CrossRef CAS.
- G. Cornelis, A. M. Forsberg-Grivogiannis, N. P. Skold, S. Rauch and J. Perez-Holmberg, Environ. Sci.: Nano, 2017, 4, 2225–2234 RSC.
- L. J. Gimbert, P. M. Haygarth, R. Beckett and P. J. Worsfold, Environ. Sci. Technol., 2005, 39, 1731–1735 CrossRef CAS PubMed.
- M. Snehota, V. Jelinkova, M. Sobotkova, J. Sacha, P. Vontobel and J. Hovind, Water Resour. Res., 2015, 51, 1359–1371 CrossRef.
-
W. Ho and K. Sirkar, Membrane Handbook, Springer, US, 2012 Search PubMed.
- S. K. Kumahor, P. Hron, G. Metreveli, G. E. Schaumann and H.-J. Vogel, Sci. Total Environ., 2015, 535, 113–121 CrossRef CAS PubMed.
- N. M. DeNovio, J. E. Saiers and J. N. Ryan, Vadose Zone J., 2004, 3, 338–351 CrossRef CAS.
- M. Arias, M. T. Barral and F. Diaz-Fierros, Clays Clay Miner., 1995, 43, 406–416 CrossRef CAS.
- G. Cornelis, C. Doolette, M. Thomas, M. J. McLaughlin, J. K. Kirby, D. Beak and D. Chittleborough, Soil Sci. Soc. Am. J., 2012, 76, 891–902 CrossRef CAS.
- J. N. Ryan and M. Elimelech, Colloids Surf., A, 1996, 107, 1–56 CrossRef CAS.
- B. Meisterjahn, E. Neubauer, F. Von der Kammer, D. Hennecke and T. Hofmann, J. Chromatogr. A, 2014, 1372, 204–211 CrossRef CAS PubMed.
- G. Cornelis, K. M. Hund-Rinke, T. Kuhlbusch, N. Van den Brink and C. Nickel, Crit. Rev. Environ. Sci. Technol., 2014, 44, 2720–2764 CrossRef CAS.
- I. L. Molnar, W. P. Johnson, J. I. Gerhard, C. S. Willson and D. M. O'Carroll, Water Resour. Res., 2015, 51, 6804–6845 CrossRef.
- A. U.-H. Khan and W. A. Jury, J. Contam. Hydrol., 1990, 5, 119–131 CrossRef CAS.
- M. Bromly, C. Hinz and L. A. G. Aylmore, Eur. J. Soil Sci., 2007, 58, 293–301 CrossRef.
- J. N. Ryan, M. Elimelech, R. A. Ard, R. W. Harvey and P. R. Johnson, Environ. Sci. Technol., 1999, 33, 63–73 CrossRef CAS.
- A. A. Turner, N. M. K. Rogers, N. K. Geitner and M. R. Wiesner, Environ. Sci.: Nano, 2020, 7, 1719–1729 RSC.
Footnote |
† Electronic supplementary information (ESI) available. See DOI: 10.1039/d0en01089h |
|
This journal is © The Royal Society of Chemistry 2021 |