Suspect and non-target screening of ovarian follicular fluid and serum – identification of anthropogenic chemicals and investigation of their association to fertility†
Received
3rd June 2021
, Accepted 17th September 2021
First published on 24th September 2021
Abstract
In this work, ultra-high performance liquid chromatography-high resolution (Orbitrap) mass spectrometry-based suspect and non-target screening was applied to follicular fluid (n = 161) and serum (n = 116) from women undergoing in vitro fertilization in order to identify substances that may be associated with decreased fertility. Detected features were prioritized for identification based on (i) hazard/exposure scores in a database of chemicals on the Swedish market and an in-house database on per- and polyfluoroalkyl substances (PFAS); (ii) enrichment in follicular fluid relative to serum; and (iii) association with treatment outcomes. Non-target screening detected 20
644 features in follicular fluid and 13
740 in serum. Two hundred and sixty-two features accumulated in follicular fluid (follicular fluid: serum ratio >20) and another 252 features were associated with embryo quality. Standards were used to confirm the identities of 21 compounds, including 11 PFAS. 6-Hydroxyindole was associated with lower embryo quality and 4-aminophenol was associated with higher embryo quality. Overall, we show the complexity of follicular fluid and the applicability of suspect and non-target screening for discovering both anthropogenic and endogenous substances, which may play a role in fertility in women.
Environmental significance
Millions of couples worldwide suffer from involuntary childlessness. The role of anthropogenic chemicals in ovarian follicular fluid is important to assess as they may affect the maturing oocyte and its competence to form a viable embryo. In this work, suspect and non-target screening of follicular fluid and serum together with a 3-tiered prioritization strategy were used to identify substances that may be associated with decreased fertility in women undergoing IVF treatment. These data show the applicability of suspect and non-target screening for discovering both anthropogenic and endogenous substances, which may play a role in fertility in women.
|
Introduction
Human infertility is defined as the inability to conceive within 12 months of actively trying, and is estimated to affect up to one out of six couples,1–6 comprising approximately 25 million citizens in the European Union alone.7 In 25–30% of fully investigated couples, the reason for infertility remains unknown.5,6 The quality, or developmental competence, of the oocyte affects the early survival of the embryo as well as the establishment of pregnancy and subsequent fetal development.8 Oocyte quality is in turn dependent on follicle growth and oocyte maturation, which is achieved during folliculogenesis, a process that takes at least half a year in humans from primordial to ovulatory stage. Primordial follicles develop through primary and secondary stages and further to antral follicles which contain follicular fluid. The follicular fluid (FF) surrounding the maturing oocyte consists of secretions from granulosa and theca cells as well as transudates from the circulation, including for example growth factors and sex steroids, but also exogenous substances.1,9–12
Growing evidence indicates that exposure to endocrine disrupting chemicals (EDCs) may negatively impact human fertility by affecting the oocyte and early embryo development,13–17 which is supported by animal studies and findings in vitro.18–24 Chemicals including bisphenol A (BPA), polychlorinated biphenyls (PCBs), dichlorodiphenyltrichloroethane (DDT), per- and polyfluoroalkyl substances (PFAS), and polybrominated diphenyl ethers (PBDEs) have been found in ovarian FF exposing the oocyte,13,25–31 with the possibility of interfering with oocyte maturation. PFAS are of particular concern due to their widespread occurrence in human blood. However, there are conflicting results regarding the effects of PFAS on fertility in epidemiological studies,25,27,32–37 which stresses the need for further investigations, especially since PFAS seem to target the ovary in different ways.38 For example, experimental in vitro studies suggest adverse effects of PFAS on oocyte maturation.39,40
According to recent estimates, over 300
000 chemicals are or have been in global chemical commerce and now constitute potential environmental pollutants.41 Traditionally, highly specific and targeted analytical approaches are applied to characterize human exposure to known substances. As a result, numerous chemicals and their transformation products are overlooked. To fill this knowledge gap, suspect- and non-target screening (NTS) approaches have emerged over the last decade as promising techniques for capturing novel anthropogenic substances. Prioritization strategies, which reduce the quantity of data from tens of thousands of features, are critical to the success of these methods.42 For example, time trends have been used to prioritize anthropogenic chemicals over endogenous substances in human serum43 and whole blood44,45 while case/control strategies have been employed for elucidating chemicals specifically associated with occupational exposure.46,47 Other studies have employed suspect screening approaches, focusing on matching features to mass spectral databases of anthropogenic substances.43,48
In this work, we applied an NTS approach to human ovarian FF and serum in order to identify substances that could potentially affect fertility in women. A 3-tiered feature prioritization approach was utilized, involving (i) matching to substances with high exposure and unknown- or moderate-high hazard scores in the Swedish Chemicals Agency Market List and further an in-house PFAS database; (ii) enrichment in FF relative to serum; and finally, (iii) association with in vitro fertilization outcomes with a focus on embryo quality. To the best of our knowledge, this is among the first studies to use NTS to investigate ovarian FF. In addition to providing insight into associations between chemicals and reproductive outcomes, the acquisition of non-target data offers the opportunity for retrospective mining, as new chemicals become available.
Material and methods
Patient recruitment
This study was approved by the Swedish Ethical Review Authority (Dnr 2015/798-31/2, 2016/360-32 and 2016/1523-32). Patients that visited the Carl von Linné clinic in Uppsala, Sweden, for assisted reproductive technology (ART) procedure consisting of ovum pick-up (OPU) for in vitro fertilization (IVF) were invited to participate in the study. Participation was voluntary; the patients could withdraw their consent at any point and they were informed that their participation would not affect their IVF-treatment. Patients received written and oral information about the study from a nurse and those agreeing to participate signed a written consent form in accordance to the Declaration of Helsinki.
From April 23 to June 16, 2016, 244 patients visited the clinic for OPU. Fifty-four patients were not invited to participate due to lack of time (n = 36), non-Swedish speaking patients (n = 14), freezing of oocytes for storage and later use (n = 1), and avoiding pressure on emotionally stressed patients (n = 3). This resulted in 190 invited patients, of whom five declined and 185 accepted to participate in the study. All patients enrolled in the study were assigned a random 3-digit code for pseudonymization of the samples. Information regarding reproductive history, cause of infertility as well as other health parameters were collected from the patients' records. Data was handled in compliance with relevant laws and institutional guidelines (the Swedish data protection law, PUL, and the general data protection regulation, GDPR), and the biological samples were registered at Uppsala Biobank (IVO 627) following the Swedish law on biobanking in health care. In the final analysis, we included patients who were non-smokers with body mass indices (BMIs) of <30 and ongoing treatment with OPU during the recruitment period. Nine patients were excluded due to smoking during the last 12 months and 14 were excluded due to BMIs ≥30 resulting in 162 patients for sample processing (Fig. 1).
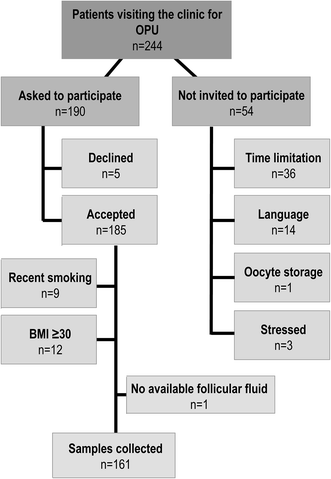 |
| Fig. 1 Flow chart of patients visiting the clinic for ovum pick-up from April 23 to June 16, 2016. Excluded patients and reason for exclusion are presented in the chart. Sampling from one patient resulted in no available follicular fluid because of blood contamination, resulting in 161 samples analyzed. | |
Sample collection
Clear FF with no visible blood contamination (n = 161) was freshly collected after OPU in 50 mL test-tubes (559
001, Sarstedt, Nümbrecht, Germany) on ice, discarding the first aliquot due to possible contamination with wash-fluid used in the OPU-tubing system. All FF aliquots from a single patient were pooled together into one sample. The samples were centrifuged at 500×g to separate cells from the supernatant FF, and the supernatant was subsequently aliquoted and stored at −80 °C until analysis. Serum collection was carried out as follows: prior to OPU, patients were given an intravenous catheter and blood was drawn into two tubes (GREI456089, VWR, Stockholm, Sweden). The first tube was discarded in order to avoid contamination from the catheter and the second was kept for processing. The blood was centrifuged within 30 minutes at 1400×g (5 minute duration) and serum was separated and stored at −80 °C until analysis. For NTS, >1.5 mL was needed for analysis, resulting in 116 samples.
Standards and reagents
A full list of 28 authentic- and 11 isotopically labelled substances can be found in Table S1 and 2 of the ESI.† The internal standard mixture was ready available in the laboratory and included substances with a wide range of physiochemical properties (e.g. Kow ranging from ∼ −1 [sucralose] to 5.7 [tonalide]).
Extraction
The extraction procedure was originally developed for human whole blood samples and is described in detail elsewhere.44,49 Prior to extraction, samples (1.5 mL of serum or 2 mL of follicular fluid) were thawed at room temperature and then spiked with 10 μL of internal standard mixture (50–125 ng of each substance; see Table S1 in the ESI† for a full list). Two mL of acetonitrile, 200 mg NaCl and 800 mg MgSO4 were added to 2 mL of FF while 2 mL of acetonitrile, 150 mg NaCl and 600 mg MgSO4 to 1.5 mL of serum. After addition of steel beads, a bead-blender (1600 Mini-G, SPEX Sample Prep, Metuchen, NJ) was used to homogenize the samples for 5 min at 1500 rpm. The mixture was centrifuged for 10 min at 2200×g and the supernatant was removed. This extraction was repeated with another volume of acetonitrile and the combined extracts were concentrated under nitrogen to 150 μL. After freezing overnight, the samples were centrifuged (5 min at 8000×g), and 100 μL of extract was transferred to a LC-vial containing 100 μL LC-MS grade water.
Instrumental analysis
Instrumental analysis was carried out using a previously developed method.44 Briefly, chromatographic separation of analytes was carried out with a Dionex UltiMate 3000 ultra-high performance liquid chromatograph equipped with a Hypersil GOLD aQ analytical column (2.1 mm × 100 mm, 1.9 μm I.D.) and a prefilter (2.1 mm, 0.2 μm) (Thermo Scientific, USA). The mobile phases consisted of LC-MS grade water with 0.1% formic acid (A) and acetonitrile with 0.1% formic acid (B). The gradient started at 5% B with a linear increase over 10 min to 99% B, followed by a 5.5 min hold and re-equilibration at 5% B for 2 min. The injection volume was set to 5 μL and the column temperature was held at 40 °C. Detection was carried out on a Q Exactive HF Orbitrap (Thermo Scientific, USA), equipped with a heated electrospray ionization (HESI) source. The capillary temperature was set to 350 °C with a spray voltage of 4.5 kV (positive mode) and 3.7 kV (negative mode), sheath gas (nitrogen) at 30/45 arbitrary units (pos/neg mode), auxiliary gas at 10/5 au (pos/neg) and auxiliary gas heater at 350 °C. A full scan was combined with a data-dependent MS2 (ddMS2) fragmentation on the top five. The full scan was run with a resolution of 120
000 Full Width at Half Maximum (FWHM) at 200 m/z and a scan range of 100–1000 Da. ddMS2 scans were run with a resolution of 15
000 FWHM at 200 m/z, normalized collision energy of 30%, an intensity threshold of 1 × 105, a dynamic exclusion for 10 seconds and an apex search between 1–10 seconds. Samples, quality controls (QCs) and blanks were run in random order with one internal standard repeated every 15 samples. Samples were run in four sequences: FF-positive mode, FF-negative mode, serum-positive mode, and serum-negative mode. A mass calibration of the Orbitrap was performed before each sequence of samples.
Data processing
Alignment, peak picking and feature determination were carried out using Compound Discoverer, versions 2.0 and 3.1 (Thermo Scientific, USA). Aggregation of peaks and adducts was implemented to define unique features. All Compound Discoverer parameters are listed in Table S3 and 4 in the ESI.† The four sequences (FF pos/neg, serum pos/neg) were processed separately in Compound Discoverer 2.0 (parameters listed in Table S3, ESI†), resulting in four separate feature lists (containing peak areas in all samples for each feature). For tier 2 (see below) we wanted to directly compare feature peak areas in FF to those in serum, which was done by reprocessing with Compound Discoverer 3.1 (this new version just became available when starting to work on this part; parameters are listed in Table S4, ESI†). This time only two feature lists were generated, one for positive and one for negative mode, each including both FF and serum samples from 116 patients (including procedural blanks for blank subtraction). For these combined feature lists the intensity thresholds during peak picking had to be raised compared to the first processing, due to the larger number of samples that needed to be processed (and by extension, the amount of time this would take). All calculated ratios between FF and serum for detected suspects were taken from these combined feature lists.
Following blank subtraction (max area sample/area blank > 5), feature prioritization was carried out using a three-tier approach (Fig. 2) which is described in detail below. Substances identified through this workflow were assigned a confidence level using the Schymanski scale.50 In brief, suspects identified through databases based on exact mass (±5 ppm) but with insufficient information for one exact structure were defined as a tentative identification and assigned a confidence level [CL] from 3–5 depending on the available data. With further information such as MS2 matches to a library spectrum the suspect was defined as a probable identification with CL 2a or as 2b when there was additional experimental evidence like homologue series. Suspects confirmed with an authentic standard were considered CL 1. Further details can be found in the ESI, Table S5.†
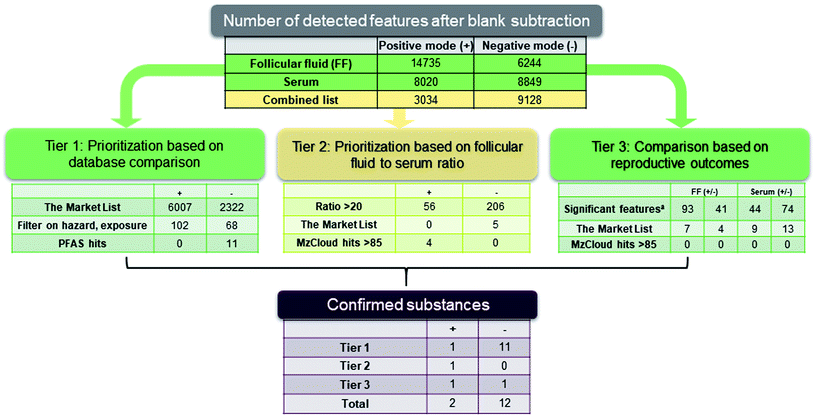 |
| Fig. 2 Overview of the tiered prioritization strategy including numbers of features and tentatively identified suspects identified using the databases the Market List and MzCloud, and confirmed substances measured in positive (+) and negative (−) ionization mode presented for all tiers. Features are defined as the combination of all ions (i.e. adducts, parent ion, in-source fragments, etc.) at a given retention time. Some duplication may exist for substances ionizing in both positive and negative mode. The combined list (yellow box) was generated re-processing the data using different thresholds (see Data processing for details) and applied in Tier 2. The Market List matches were filtered on both high exposure and unknown-to-high hazard score (see Data processing for details). asignificantly (p < 0.05) different (fold change > 1.5) suspects in the two groups. | |
Quality control
In order to avoid false-positives, both sampling blanks and procedural blanks were processed together with samples. Sampling blanks (n = 5) were prepared immediately prior to follicular fluid collection by rinsing the sample collection tubing with buffer using enough volume to fill the system with fluid and collect a volume of ∼0.5 mL after the rinse (G-RINSE, Vitrolife, Göteborg, Sweden), which was then saved for analysis. Serum was collected using vene-catheters (Venflon PVK 20 G, BD Medical Surgical Systems, Stockholm, Sweden) and sampling blanks prepared and processed the same as for follicular fluid, by rinsing vene-catheters (n = 3) prior to patient sampling. Each extraction batch of FF (n = 16 samples/batch) and serum (n = 19 samples/batch) included one procedural blank consisting of 1 mL of MilliQ water (in total n = 10 procedural blanks for FF samples and n = 6 for serum samples). During the initial stage of data processing, features with signal intensities within 5-fold of the average procedural blank intensity were removed from the dataset and were not considered thereafter. This removed 13–54% of the features in the datasets (see Table S6, ESI† for exact numbers). Moreover, once a feature was identified using an authentic standard, we re-confirmed its absence in both the sampling and procedural blanks.
In order to account for procedural losses and confirm that the extraction procedure was suitable across a wide range of substances, a suite of 11 internal standards (Table S1, ESI†) were spiked into all samples prior to extraction. Internal standard recoveries in both FF and serum samples were acceptable, ranging from 61 to 107% for all substances (relative standard deviations (RSDs) ranging from 13–30% in serum and 13–23% in FF for all samples; see Fig. S1 in the ESI†). Each extraction batch of FF or serum contained a pooled QC sample prepared from n = 3 samples of FF or 150 μL portions of all serum samples which were extracted the same way as patient samples. Internal standard recoveries in both FF and serum QCs ranged from 45–119% with RSDs of 5.4–15.9% in serum QCs and 4.5–14.2% in FF QCs (Fig. S1 and Table S8, ESI†). Finally, during instrumental analysis, internal standard solutions prepared in acetonitrile were analysed every 15 samples to monitor instrumental drift over the course of the run. RSDs for internal standard peak areas in these solutions ranged from 1.8 to 21% and showed an absence of signal drift over the course of the run (Table S8, ESI†).
Tier 1: prioritization based on database comparisons
Applying the four separate feature lists, features detected in FF or serum samples were compared to two different databases: the Swedish Chemicals Agency Market List (KEMI Market List, referred to herein as the “Market List”) and an in-house exact mass list of PFAS containing 279 PFAS previously detected in house or the literature. The Market List is available on the NORMAN Suspect List Exchange website51 and contains 30
000 substances (industrial chemicals, pharmaceuticals, pesticides, etc.) from different national/regional inventory lists with a focus on the EU market. The Market List prioritizes substances based on both human hazard and exposure scores, which are based on confidential data from the importer/manufacturer supplied to the Swedish Chemicals Agency. The exposure score is calculated based on risk of environmental contamination, such as the quantity and degree of uncontrolled release during use and the extent of use on the market (range 0–27, where 27 represent the greatest exposure). The hazard score is calculated based on hazard classifications described by the EU's Classification, Labelling and Packaging (CLP) regulation, using toxicological information regarding carcinogenic, mutagenic and reproductive toxicity (range 0–9 where 9 represent the greatest hazard) (for details, see Market List documentation).52 The entire feature list (i.e. exact masses with 5 ppm tolerance) in serum and FF was compared to the Market List and resulted in 18
462 matches. Tentative assignments were filtered based on high exposure score (≥15) and also unknown or moderate- to high hazard scores (unknown or ≥3) in FF. Filtered features occurring in >30% of the FF (n = 170) were prioritized for further investigation to limit the search to more common exposures.
Additionally, an in-house database containing PFAS exact masses were matched with the feature lists of FF and serum. Afterwards reference standards were used to confirm the detected suspects of 11 PFAS (see ESI Table S2†).
Tier 2: prioritization based on follicular fluid to serum ratio
This prioritization strategy was based on the premise that features enriched in FF may have a greater impact on the maturing oocyte. To prioritize these features, we used the combined FF/serum datasets (see data processing section) to determine the ratio of the peak area of a given feature in FF to its corresponding peak area in serum from the same individual. The features with a ratio >20 in at least 110 out of 116 patients were then searched against the full Market List and the mass spectral library mzCloud. In order to assess the impact of matrix-induced ionization effects on the calculated ratios, we compared internal standard responses between FF and serum. The ratios of IS areas in FF to serum ranged from 0.015 to 0.89 (see Table S7 in the ESI†), with a median ratio in negative mode of 0.73 and in positive mode of 0.22 suggesting more matrix suppression in FF compared to serum in positive mode. Generally, all median ratios were below 1, which results in an underreporting of enrichment factors when using peak areas in FF relative to serum. We note, however, that matrix effects could only be assessed for internal standards; we cannot rule out the possibility of higher or lower matrix-induced ionization effects for non-targets.
Tier 3: prioritization based on reproductive outcomes
Tier 3 suspects were prioritized using statistical comparisons between features in FF and serum depending on assisted reproductive technology (ART) outcomes based on (i) the live birth of an offspring, (ii) a positive pregnancy determined by a home urine hCG-test executed by the patient, and (iii) embryo quality (at least one top quality embryo ≥9.1, range 1–10) assessed on day 2 by a well-established method.53 In brief, embryo score incorporates cleavage stage with the information of embryo variables associated with higher implantation-rates at day two, i.e. variation in blastomere size and the number of mononucleated blastomeres.
Features associated with differences in reproductive outcomes were identified using MetaboAnalyst.54,55 Prior to processing, missing values (i.e. below detection limit) were replaced by a small value and the data were filtered by variance to remove excessive noise56 before normalization of the data by mean centering. The difference between the ART outcomes was investigated using multivariate statistic approaches. However, we found that orthogonal partial least squares discriminant analysis (OPLS-DA) resulted in poor separation between the groups (top embryo quality: R2 = 0.14, pregnancy test R2 = 0.10, live birth R2 = 0.07), possibly due to the massive amount of data and influence of other parameters, so that the variation in FF and serum composition alone was not sufficient to describe differences in ART outcome. Further analyses were therefore focused on embryo quality, which had the highest R2 of the three ART outcome models and, more importantly, allowed a more focused assessment of the direct effect of chemicals in the follicular fluid on the maturing oocyte and embryo development. A fold change threshold between peak area in the two ART groups of ≥1.5 (in >75% of pairs/variable) combined with t-test significance threshold of p < 0.05 provided significantly different features in FF and serum of patients with top quality embryos compared to those with lower quality. Since we are using this approach as a prioritization strategy, we were willing to accept some false positives which may arise when not adjusting for multiple testing. The significantly different features were compared to the databases mzCloud and the Market List for further prioritization. Logistic regression (glm model of CRAN package, R 3.6.1) was used assess if the groups (high vs. low embryo quality) differed in age or BMI as these are possible confounding factors. Difference in ovarian reserve estimated by the biomarker anti-müllerian hormone (AMH) in serum was investigated using the same method. P-values < 0.05 were considered significant. After prioritization, the association between the identified exogenous substances and high/low embryo quality was investigated using a logistic regression model with age, BMI and AMH as explanatory variables. AMH and features were log-transformed to reduce skewness. The variables “fertility cause” (male/female origin) and “parity” were considered for inclusion in the model but removed based on goodness of fit of the model and significance.
Results and discussion
Recruited patients
Baseline statistics of patients and the fresh cycles included in the study are presented in Table 1. The reasons behind the patients' infertility as stated in the medical records was unknown (43.8% of the cases), male infertility (24.8%), tubal factor (6.5%), endometriosis (3.9%), ovarian factor (10.4%), anovulation (9.8%) or sterilized (0.5%). The distribution of the diagnoses is similar to larger cohorts from the same clinic.57 Oocytes were fertilized either with IVF (48.5%), intraplasmatic sperm injection (ICSI, 43.5%) or a combination thereof (8%). For treatment resulting in developing embryos, 49% resulted in at least one top quality embryo. The average score of the top embryo was 8.6 ranging from 0.3–10 in all patients.
Table 1 Characteristics of patients enrolled (n = 161)
Parameter |
Value |
Ovarian reserve estimated by the biomarker anti-müllerian hormone (AMH), measured in μg L−1.
Results from fresh IVF cycles where 85% of the cycles resulted in an embryo transfer.
|
Age in years, mean (SD) |
34.6 (4.6) |
AMHa, μg L−1 (SD) |
3.25 (2.9) |
Previous IVF-treatments, n (%) |
No previous |
85 (52.8) |
One previous |
33 (20.5) |
Two or more previous |
43 (26.7) |
Body mass index (BMI), mean (SD) |
23.5 (3.04) |
Eggs for insemination, mean (range) |
10.2 (1–36) |
Parity, n (%) |
Nulliparous |
109 (68) |
Primiparous |
44 (27) |
Multiparous |
8 (5) |
Pregnancy rateb, % |
37.9 |
Live birth rateb, % |
29.6 |
Number of detected features
In the individual feature lists for FF, a total of 20
644 features were detected (14
474 in positive and 6170 in negative mode; features ionizing in both modes would be counted twice), with an average of 3141 (standard deviation (SD) ±380) unique features per patient. Of these features, 745 features were present in >90% of the patients and 2349 in >50%. In addition, 6385 features were patient-specific, i.e. detected in only one (but not necessarily the same) patient. In serum, we detected 13
740 features (6493 in positive and 7247 in negative mode), with an average of 2508 (SD ± 228) unique features per patient. A total of 2049 features occurred in >50% of patients, of which less than half (885 features) were observable in >90% of patients. In serum, 4254 features were detected in no more than one (but not necessarily the same) patient.
The combined lists (including FF and serum) comprised 3034 features in positive mode and 9128 features in negative mode (166 was detected in >50% of patients in both follicular fluid and serum and 41 in >90%). The inconsistency in the number of features between the individual and combined feature lists is due to (a) differences in the number of samples used for data processing (individual feature lists included all 161 patients for FF, while the combined feature lists only included data from 116 patients where both FF and serum data was collected); (b) the version of Compound Discoverer used; and (c) differences in settings in Compound Discoverer used for data processing (more specifically, different thresholds during peak picking and alignment, see materials and methods for more details).
Tier 1: prioritization based on database comparison
Of the 3306 features occurring in ≥30% of the FF samples, 170 matched substances with high exposure scores and unknown- or moderate-to-high hazard scores in the Market List (CL 3–5). Of these 170 tentatively identified substances, analysis of authentic standards for 14 chemicals confirmed the identities of five substances (and at the same time ruled out nine identities). Substances identified at CL 1–2 are presented in Table 2 and the remaining tentative identifications (CL 3–5) can be found in Table S9 and 10 in the ESI.† 3-pyridinecarboxamide (CL = 1; also known as nicotinamide or vitamin B3) was confirmed. Two additional substances, tris(2-butoxyethyl) phosphate (TBEP, CL = 1) and dibutylamine (CL = 1; also known as N-butyl-1-butanamine) were observed frequently in follicular fluid but was also observable at similar intensities in the sampling blanks; consequently, these substances were not considered further.
Table 2 Summary of substances identified using the various prioritization strategies
|
|
Compound |
Massa |
CAS no |
Ratiob |
RSDc |
Conf. Leveld |
Neutral monoisotopic mass (Da).
Median ratios were determined by calculating the peak area in FF/peak area in serum from the same individual.
RSD, Relative standard deviation of the ratios.
Confidence level of confirmation, see section Data processing, Materials and methods for description.50 For aall substances at CL 1 and 2b chromatograms (and, where high enough intensities were present in the samples, also MS2 spectra) are listed in Fig. S2 A–R in the ESI.
Hydroxyoctadecanoic acid found through both tier 1 & 3.
|
Tier 1 |
Identified based on database identifications |
12-Hydroxyoctadecanoic acide |
300.2664 |
106-14-9 |
0.55 (0.016–7.61) |
215.60 |
1 |
3-Pyridinecarboxamide |
122.0480 |
98-92-0 |
1.13 (0.01–71.94) |
590.54 |
1 |
Dodecanedioic acid |
230.1518 |
693-23-2 |
9.06 (1.64–152.72) |
243.40 |
1 |
Perfluoroheptanoic acid (PFHpA) |
363.9769 |
375-85-9 |
0.65 (0.37–1.00) |
20.60 |
1 |
Perfluorooctanoic acid (PFOA) |
413.9737 |
335-67-1 |
0.72 (0.33–1.18) |
17.00 |
1 |
Perfluorononanoic acid (PFNA) |
463.9705 |
375-95-1 |
0.79 (0.31–1.26) |
18.80 |
1 |
Perfluorodecanoic acid (PFDA) |
513.9673 |
335-76-2 |
0.69 (0.21–1.29) |
25.94 |
1 |
Perfluoroundecanoic acid (PFUnDA) |
563.9641 |
2058-94-8 |
0.71 (0.05–1.39) |
35.34 |
1 |
Perfluoropentane sulfonate (PFPeS) |
349.9470 |
2706-91-4 |
0.88 (0.23–1.73) |
23.70 |
2b |
Perfluorohexane sulfonate (PFHxS) |
399.9439 |
355-46-4 |
1.04 (0.01–1.65) |
19.54 |
1 |
Perfluoroheptane sulfonate (PFHpS) |
449.9407 |
375-92-8 |
0.79 (0.04–1.49) |
31.00 |
2b |
Perfluorooctane sulfonate (PFOS) |
499.9375 |
1763-23-1 |
0.64 (0.001–1.51) |
82.94 |
1 |
9-Chlorohexadecafluoro-oxanonane sulfonate (9Cl-PF3ONS) |
531.9029 |
756 426-58-1 |
0.94 (0.24–2.15) |
41.60 |
1 |
Perfluoro-ethylcyclohexane sulfonate (PFECHS) |
461.9407 |
646-83-3 |
0.88 (0.10–2.66) |
32.20 |
1 |
Tier 2 |
Accumulation in follicular fluid |
Progesterone |
314.2246 |
57-83-0 |
255.8 (78.77–646.78) |
47.48 |
2a |
Lidocaine |
234.1732 |
137-58-6 |
4559 (0.27–55 483) |
202.87 |
1 |
Epitestosterone |
288.2089 |
481-30-1 |
50.29 (9.24–163.86) |
52.14 |
2a |
17α-Hydroxyprogesterone |
330.2195 |
68-96-2 |
100.18 (9.59–2302.09) |
236.9 |
2a |
20α-Hydroxyprogesterone |
316.2402 |
145-14-2 |
25.47 (2.40–75.55) |
49.27 |
2a |
Tier 3 |
Associated with embryo quality |
4-Aminophenol |
109.0528 |
123-30-8 |
0.18 (0.02–2.51) |
171.76 |
1 |
6-Hydroxyindole |
133.0528 |
2380-86-1 |
0.88 (0.01–2.73) |
45.07 |
2a |
Although our focus was on anthropogenic chemicals, several endogenous compounds were identified, including 12-hydroxyoctadecanoic acid (CL = 1, detected in 57% of the patients) and dodecanedioic acid (CL = 1, detected in 67% of the patients). In addition to their natural occurrence, these substances are manufactured commercially and were prioritized in Tier I due to their presence on the Market List and unknown or unrecorded hazards.
Eleven PFAS were tentatively identified by matching the combined FF and serum feature list with the in-house database on PFAS; their identities were subsequently confirmed using reference standards (Table 2). The median FF:serum ratio ranged from 0.64 (PFOS) to 1.04 (PFHxS). These values are comparable to previously reported data,58 a comparison of which can be found in Table S11 in the ESI.† Some PFAS are known to cause developmental toxicity in experimental animals59 and disturb lipid metabolism in vitro39,60 and in humans.61 Some PFAS are also associated with affected ART outcomes according to previous studies.25,34 However, despite clear connections with effects reported in the peer-reviewed literature, PFAS did not appear using the other prioritization strategies in this study (i.e. enrichment in FF and connection to reproductive outcomes). In addition, several EDCs commonly investigated in relation to human health outcomes were not prioritized for identification using the approach in tier 1. Some of these compounds are not included as they do not fulfill the criteria of ubiquitous exposure even though mentioned in the Market list (some phthalates for example) and other were given low hazard score in the Market List and thus not included in the prioritization used in this study (some PFAS and parabens).
Tier 2: prioritization based on follicular fluid to serum ratio
The identification of substances enriched in FF (Tier 2) is particularly important for facilitating reproductive risk assessment based on exposure of the oocyte during maturation – a specific and sensitive period during development.62 In Tier 2, twenty-two percent of all features in the combined feature lists (2709 of 12
162 as sum of pos and neg mode) were found in both FF and serum. A total of 262 features with FF:serum ratios of ≥20 in 110 patients were compared to the full Market List and mzCloud. Suspects including identification level can be found in Table S9 and 10 (ESI).† One compound, lidocaine, was confirmed with a reference standard (CL = 1); however, this substance is used as a local anesthetic during OPU and the accumulation in FF compared to serum can be assumed from the local injection close to the sampling site. An additional four hormones and hormone derivatives (progesterone, epitestosterone, 17α-hydroxyprogesterone, and 20α-hydroxyprogesterone) could be confirmed using library spectrum data (mzCloud hit >85, CL = 2a). This result is not surprising considering that steroids such as progesterone, as well as androgens and estrogens are produced in the follicle and their concentrations are therefore higher in FF compared to plasma or serum.63–65
The ratio that was chosen as a prioritization limit in this study (>20) reflects a substantial enrichment in follicular fluid. Enrichment of anthropogenic chemicals at lower ratios are also of importance to investigate, but were not included here in an effort to reduce the number of features to a reasonable amount for identification.
Tier 3: prioritization based on reproductive outcomes
Multivariate statistical models for ART outcomes (positive pregnancy test, live birth) resulted in poor group separation. Further analysis was therefore only implemented for embryo quality. The patients with top quality embryos did not significantly differ from those with lower quality with respect to age (intercept 35.6, estimate −1.07, p = 0.07) or BMI (23.27, 0.32, p = 0.55). However, the patients with high embryo quality also had higher AMH levels (2.58, 1.17, p = 0.027). NTS detected 252 significantly different features in patients with top quality embryos compared to those with lower quality (134 in FF and 118 in serum). Features associated with patients with different embryo quality may represent substances that play a role in oocyte developmental competence. The significantly different features were further analysed and compared to The Market List and mzCloud. The Market List matched 11 suspects in FF and 22 in serum, while hits in mzCloud all scored <85% which indicate insufficient data for a probable confirmation (CL 3–5, presented in Table S9 and 10 in the ESI†). Authentic standards resulted in three confirmations: 12-hydroxydodecanoid acid (CL = 1; endogenous, discussed in Tier 1), 4-aminophenol (or isomers, see below; CL = 1) and 6-hydroxyindole (CL = 2a). The aggregated list of identified compounds is presented in Table 2.
When using a fold change threshold of ≥1.5 between peak areas of high/low embryo quality patients combined with t-test significance threshold (p < 0.05), 4-aminophenol was associated with higher embryo quality. However, this could not be confirmed with logistic regression that included the confounding factors age, BMI and AMH (Table S12 in the ESI†). 4-Aminophenol was observed in 83% of the patients but we cannot rule out that this assignment may correspond to aminophenol isomers. This chemical is found in consumer products such as personal care products and cosmetics and is used in the industry for staining fur, leather and textiles as well as in the manufacturing of pharmaceuticals such as paracetamol. In humans, the chemical aniline is metabolized into paracetamol/acetaminophen and 4-aminophenol is in turn a minor metabolite of paracetamol that is nephrotoxic.66 In this study, 4-aminophenol was present in lower concentration in serum of women with lower quality embryos and it was also detected in FF. This suggests that 4-aminophenol might have effects on oocyte maturation. An epidemiological study found no association between acetaminophen or 4-aminophenol urine concentrations in females and time to pregnancy, but a significant association for males.67
Both the tier 3 prioritization method and a logistic regression model that included confounding factors (age, BMI and AMH) found an association between 6-hydroxyindole and low embryo quality (p = 0.02, Table S12 in the ESI†). 6-Hydroxyindole was detected in serum in 34% of the patients and was found in FF. 6-Hydroxyindole is commonly used in hair dyes. Gut bacterial degradation of certain amino-acids produces indole that is absorbed from the gut and further metabolized into 6-hydroxyindole by CYP11A1.68 CYP11A1 regulates androgens and has been shown to be important in the etiology of polycystic ovary syndrome.69
Compounds previously associated with IVF outcomes such as PCBs, phthalates and bisphenols were not identified using this approach. This should not be interpreted as a negative result but rather a function of the analytical technique (these substances are analyzed by GC-MS rather than LC-MS) and/or prioritization method or outcome (embryo quality).
Conclusion
The results from this study show that FF contains a complex mixture of endogenous and anthropogenic substances. It is possible that the occurrence of anthropogenic substances inside the follicle could disrupt the composition of the FF, which in turn could lead to difficulties in conceiving. For example, studies using omics-approaches to define FF composition and the effect on fertility parameters have shown that endogenous substances differ between high or low oocyte-yielding cows70 and there are differences in protein patterns in FF that determine if the oocyte could be fertilized or not.71 By using in vitro models, exposure effects on oocyte maturation and the pre-implantation embryo can be further explored, for example in the bovine or porcine model.39,62 Only a fraction of the detected features in FF and serum were prioritized for identification in our study (Tiers 1–3), but further identifications using data from this study can be used retrospectively as new concerns arises or new compounds are discovered.72
Author contributions
IH: Methodology, Formal Analysis, Investigation, Visualization, Writing – Original Draft, Review & Editing, MP: Methodology, Validation, Formal Analysis, Investigation, Supervision, Writing – Original Draft, Review & Editing, SP: Conceptualization, Methodology, Supervision, Writing – Original Draft, Review & Editing, JB: Methodology, Resources, Validation, Supervision, Writing – Original Draft, Review & Editing, YS: Conceptualization, Funding acquisition, Methodology, Supervision, Writing – Review & Editing, PD: Resources, Methodology, Supervision, Writing – Review & Editing, MO: Supervision, Writing – Review & Editing, JH: Resources, Writing – Review & Editing. All authors reviewed and approved the manuscript before submission.
Conflicts of interest
There are no conflicts to declare.
Acknowledgements
This work was funded by FORMAS (Swedish Research Council for Environment, Agricultural Sciences and Spatial Planning, Grant No. 942-2015-476 and 2015-00623). The authors sincerely thank all women participating in the study as well as the nurses and embryologists at the Carl von Linné Clinic (Uppsala, Sweden) contributing to the sample collection. We would also wish to thank Stellan Fischer (Swedish Chemicals Agency) for his expertise regarding the incorporation of market data in non-target screening and finally we would like to thank Anton Ribbenstedt (SU, Sweden) for great support during the lab-work and analyses, Richelle Björnvang (KI, Sweden) for assistance with the data collection and Joëlle Rüegg (UU, Sweden) for fruitful discussions during this work.
References
- J. Datta, M. J. Palmer, C. Tanton, L. J. Gibson, K. G. Jones, W. Macdowall, A. Glasier, P. Sonnenberg, N. Field, C. H. Mercer, A. M. Johnson and K. Wellings, Prevalence of infertility and help seeking among 15 000 women and men, Hum. Reprod., 2016, 31, 2108–2118 CrossRef CAS PubMed.
- J. Boivin, L. Bunting, J. A. Collins and K. G. Nygren, International estimates of infertility prevalence and treatment-seeking: potential need and demand for infertility medical care, Hum. Reprod., 2007, 22, 1506–1512 CrossRef PubMed.
- M. N. Mascarenhas, S. R. Flaxman, T. Boerma, S. Vanderpoel and G. A. Stevens, National, regional, and global trends in infertility prevalence since 1990: a systematic analysis of 277 health surveys, PLoS Med., 2012, 9, e1001356 CrossRef PubMed.
- M. E. Thoma, A. C. McLain, J. F. Louis, R. B. King, A. C. Trumble, R. Sundaram and G. M. Buck Louis, Prevalence of infertility in the United States as estimated by the current duration approach and a traditional constructed approach, Fertil. Steril., 2013, 99, 1324–1331 CrossRef PubMed.
- J. L. H. Evers, Female subfertility, Lancet, 2002, 360, 151–159 CrossRef.
- M. Hull, C. Glazener, N. Kelly, D. Conway, P. Foster, R. Hinton, C. Coulson, P. Lambert, E. Watt and K. Desai, Population study of causes, treatment, and outcome of infertility, Br. Med. J., 1985, 291 Search PubMed.
-
F. Europe and ESHRE, A Policy Audit on Fertility - Analysis of 9 EU Countries, 2017 Search PubMed.
- R. L. Krisher, The effect of oocyte quality on development, J. Anim. Sci., 2004, 82, E14–E23 Search PubMed.
- A. Revelli, L. Delle Piane, S. Casano, E. Molinari, M. Massobrio and P. Rinaudo, Follicular fluid content and oocyte quality: from single biochemical markers to metabolomics, Reprod. Biol. Endocrinol., 2009, 7, 40 CrossRef PubMed.
- J. E. Fortune, Ovarian Follicular Growth and Development in Mammals, Biol. Reprod., 1994, 50, 225–232 CrossRef CAS PubMed.
- M. M. Emori and R. Drapkin, The hormonal composition of follicular fluid and its implications for ovarian cancer pathogenesis, Reprod. Biol. Endocrinol., 2014, 12 CAS.
-
G. C. L. Lachelin, in Introduction to Clinical Reproductive Endocrinology, Butterwort-Heinemann Ltd, 1991, 1991, ch. 3, pp. 20-35 Search PubMed.
- E. M. Petro, J. L. Leroy, A. Covaci, E. Fransen, D. De Neubourg, A. C. Dirtu, I. De Pauw and P. E. Bols, Endocrine-disrupting chemicals in human follicular fluid impair in vitro oocyte developmental competence, Hum. Reprod., 2012, 27, 1025–1033 CrossRef CAS PubMed.
- S. Ehrlich, P. L. Williams, S. A. Missmer, J. A. Flaws, X. Ye, A. M. Calafat, J. C. Petrozza, D. Wright and R. Hauser, Urinary bisphenol A concentrations and early reproductive health outcomes among women undergoing IVF, Hum. Reprod., 2012, 27, 3583–3592 CrossRef CAS PubMed.
- R. Machtinger, A. J. Gaskins, C. Racowsky, A. Mansur, M. Adir, A. A. Baccarelli, A. M. Calafat and R. Hauser, Urinary concentrations of biomarkers of phthalates and phthalate alternatives and IVF outcomes, Environ. Int., 2018, 111, 23–31 CrossRef CAS PubMed.
- R. Hua, Y. Zhou, B. Wu, Z. Huang, Y. Zhu, Y. Song, Y. Yu, H. Li and S. Quan, Urinary triclosan concentrations and early outcomes of in vitro fertilization-embryo transfer, Reproduction, 2017, 153, 319–325 CAS.
- R. D. Björvang and P. Damdimopoulou, Persistent environmental endocrine-disrupting chemicals in ovarian follicular fluid and in vitro fertilization treatment outcome in
women, Upsala J. Med. Sci., 2020, 125(2), 85–94 CrossRef PubMed.
- K. J. Hutt, Z. Shi, D. F. Albertini and B. K. Petroff, The environmental toxicant 2,3,7,8-tetrachlorodibenzo-p-dioxin disrupts morphogenesis of the rat pre-implantation embryo, BMC Dev. Biol., 2008, 8, 1 CrossRef PubMed.
- A. Kuchenhoff, R. Eckard, K. Buff and B. Fischer, Stage-Specific Effects of Defined Mixtures of Polychlorinated Biphenyls on In Vitro Development of Rabbit Preimplantation Embryos, Molecular Reproduction and Development, 1999, 54, 126–134 CrossRef CAS.
-
A. K. Krogenaes, I. Nafstad, J. U. Skåre, W. Farstad and A. Hafne, In vitro reproductive toxcicity of polychlorinated biphenyl congeners 153 and 126, vol. 12, 1998, 6, pp. 575–580 Search PubMed.
- A. Lindenau and B. Fischer, Embryotoxicity of polychlorinated biphenyls (PBSs) for preimplantation embryos, Reprod. Toxicol., 1996, 10, 227–230 CrossRef CAS PubMed.
- P. Seiler, B. Fischer, A. Lindenau and H. M. Beier, Effects of persistent chlorinated hydrocarbons on fertility and embryonic development in the rabbit, Hum. Reprod., 1994, 9, 1920–1926 CrossRef CAS PubMed.
- J. Ferris, K. Mahboubi, N. MacLusky, W. A. King and L. A. Favetta, BPA exposure during in vitro oocyte maturation results in dose-dependent alterations to embryo development rates, apoptosis rate, sex ratio and gene expression, Reprod. Toxicol., 2016, 59, 128–138 CrossRef CAS PubMed.
- G. Ptak, F. Zacchini, M. Czernik, A. Fidanza, C. Palmieri, L. Della Salda, P. A. Scapolo and P. Loi, A short exposure to polychlorinated biphenyls deregulates cellular autophagy in mammalian blastocyst in vitro, Hum. Reprod., 2012, 27, 1034–1042 CrossRef CAS PubMed.
- M. L. E. Petro, W. D'Hollander, A. Covaci, L. Bervoets, E. Fransen, D. De Neubourg, I. De Pauw, J. L. M. R. Leroy, E. P. A. Jorssen and P. E. J. Bols, Perfluoroalkyl acid contamination of follicular fluid and its consequence for in vitro oocyte developmental competence, Sci. Total Environ., 2014, 496, 282–288 CrossRef PubMed.
- P. I. Johnson, L. Altshul, D. W. Cramer, S. A. Missmer, R. Hauser and J. D. Meeker, Serum and follicular fluid concentrations of polybrominated diphenyl ethers and in vitro fertilization outcome, Environ. Int., 2012, 45, 9–14 CrossRef CAS PubMed.
- J. A. McCoy, J. T. Bangma, J. L. Reiner, J. A. Bowden, J. Schnorr, M. Slowey, T. O'Leary, L. J. Guillette, Jr. and B. B. Parrott, Associations between perfluorinated alkyl acids in blood and ovarian follicular fluid and ovarian function in women undergoing assisted reproductive treatment, Sci. Total Environ., 2017, 605–606, 9–17 CrossRef CAS PubMed.
- S. Jirsova, J. Masata, L. Jech and J. Zvarova, Effect of polychlorinated biphenyls (PCBs) and 1,1,1-trichloro-2,2,-bis (4-chlorophenyl)-ethane (DDT) in follicular fluid on the results of in vitro fertilization-embryo transfer (IVF-ET) programs, Fertil. Steril., 2010, 93, 1831–1836 CrossRef CAS PubMed.
- J. D. Meeker, S. A. Missmer, L. Altshul, A. F. Vitonis, L. Ryan, D. W. Cramer and R. Hauser, Serum and follicular fluid organochlorine concentrations among women undergoing assisted reproduction technologies, Environ. Health, 2009, 8, 32 CrossRef PubMed.
- Y. Zhu, B. Huang, Q. X. Li and J. Wang, Organochlorine pesticides in follicular fluid of women undergoing assisted reproductive technologies from central China, Environ. Pollut., 2015, 207, 266–272 CrossRef CAS PubMed.
- Y. Ikezuki, O. Tsutsumi, Y. Takai, Y. Kamei and Y. Taketani, Determination of bisphenol A concentrations in human biological fluids reveals significant early prenatal exposure, Hum. Reprod., 2002, 17, 2839–2841 CrossRef CAS PubMed.
- C. Fei, J. K. McLaughlin, L. Lipworth and J. Olsen, Maternal levels of perfluorinated chemicals and subfecundity, Hum. Reprod., 2009, 24, 1200–1205 CrossRef CAS PubMed.
- M. P. Velez, T. E. Arbuckle and W. D. Fraser, Maternal exposure to perfluorinated chemicals and reduced fecundity: the MIREC study, Hum. Reprod., 2015, 30, 701–709 CrossRef CAS PubMed.
- L. Governini, R. Orvieto, C. Guerranti, L. Gambera, V. De Leo and P. Piomboni, The impact of environmental exposure to perfluorinated compounds on oocyte fertilization capacity, J. Assist. Reprod. Genet., 2011, 28, 415–418 CrossRef PubMed.
- A. L. Heffernan, T. K. Cunningham, D. S. Drage, L. L. Aylward, K. Thompson, S. Vijayasarathy, J. F. Mueller, S. L. Atkin and T. Sathyapalan, Perfluorinated alkyl acids in the serum and follicular fluid of UK women with and without polycystic ovarian syndrome undergoing fertility treatment and associations with hormonal and metabolic parameters, Int. J. Hyg. Environ. Health, 2018, 221, 1068–1075 CrossRef CAS PubMed.
- Y. R. Kim, N. White, J. Braunig, S. Vijayasarathy, J. F. Mueller, C. L. Knox, F. A. Harden, R. Pacella and L. L. Toms, Per- and poly-fluoroalkyl substances (PFASs) in follicular fluid from women experiencing infertility in Australia, Environ. Res., 2020, 190, 109963 CrossRef CAS PubMed.
- G. Waterfield, M. Rogers, P. Grandjean, M. Auffhammer and D. Sunding, Reducing exposure to high levels of perfluorinated compounds in drinking water improves reproductive outcomes: evidence from an intervention in Minnesota, Environ. Health, 2020, 19, 42 CrossRef CAS PubMed.
- N. Ding, S. D. Harlow, J. F. Randolph Jr., R. Loch-Caruso and S. K. Park, Perfluoroalkyl and polyfluoroalkyl substances (PFAS) and their effects on the ovary, Hum. Reprod. Update, 2020, 26, 724–752 CrossRef CAS PubMed.
- I. Hallberg, J. Kjellgren, S. Persson, S. Orn and Y. Sjunnesson, Perfluorononanoic acid (PFNA) alters lipid accumulation in bovine blastocysts after oocyte exposure during in vitro maturation, Reprod. Toxicol., 2019, 84, 1–8 CrossRef CAS PubMed.
- A. Dominguez, Z. Salazar, E. Arenas, M. Betancourt, Y. Ducolomb, H. Gonzalez-Marquez, E. Casas, M. Teteltitla and E. Bonilla, Effect of perfluorooctane sulfonate on viability, maturation and gap junctional intercellular communication of porcine oocytes in vitro, Toxicol. In Vitro, 2016, 35, 93–99 CrossRef CAS PubMed.
- Z. Wang, G. W. Walker, D. C. G. Muir and K. Nagatani-Yoshida, Toward a Global Understanding of Chemical Pollution: A First Comprehensive Analysis of National and Regional Chemical Inventories, Environ. Sci. Technol., 2020, 54(5), 2575–2584 CrossRef CAS PubMed.
- J. Hollender, E. L. Schymanski, H. P. Singer and P. L. Ferguson, Nontarget Screening with High Resolution Mass Spectrometry in the Environment: Ready to Go?, Environ. Sci. Technol., 2017, 51, 11505–11512 CrossRef CAS PubMed.
- L. T. Miaz, M. M. Plassmann, I. Gyllenhammar, A. Bignert, O. Sandblom, S. Lignell, A. Glynn and J. P. Benskin, Temporal trends of suspect- and target-per/polyfluoroalkyl substances (PFAS), extractable organic fluorine (EOF) and total fluorine (TF) in pooled serum from first-time mothers in Uppsala, Sweden, 1996-2017, Environ. Sci.: Processes Impacts, 2020, 22, 1071–1083 RSC.
- M. M. Plassmann, S. Fischer and J. P. Benskin, Nontarget Time Trend Screening in Human Blood, Environ. Sci. Technol. Lett., 2018, 5, 335–340 CrossRef CAS.
- M. M. Plassmann, E. Tengstrand, K. M. Aberg and J. P. Benskin, Non-target time trend screening: a data reduction strategy for detecting emerging contaminants in biological samples, Anal. Bioanal. Chem., 2016, 408, 4203–4208 CrossRef CAS PubMed.
- A. Rotander, A. Kärrman, L. M. Toms, M. Kay, J. F. Mueller and M. J. Gomez Ramos, Novel fluorinated surfactants tentatively identified in firefighters using liquid chromatography quadrupole time-of-flight tandem mass spectrometry and a case-control approach, Environ. Sci. Technol., 2015, 49, 2434–2442 CrossRef CAS PubMed.
- R. Grashow, V. Bessonneau, R. R. Gerona, A. Wang, J. Trowbridge, T. Lin, H. Buren, R. A. Rudel and R. Morello-Frosch, Integrating Exposure Knowledge and Serum Suspect Screening as a New Approach to Biomonitoring: An Application in Firefighters and Office Workers, Environ. Sci. Technol., 2020, 54, 4344–4355 CrossRef CAS PubMed.
- R. R. Gerona, J. M. Schwartz, J. Pan, M. M. Friesen, T. Lin and T. J. Woodruff, Suspect screening of maternal serum to identify new environmental chemical biomonitoring targets using liquid chromatography-quadrupole time-of-flight mass spectrometry, J. Exposure Sci. Environ. Epidemiol., 2018, 28, 101–108 CrossRef CAS PubMed.
- M. Plassmann, M. Schmidt, W. Brack and M. Krauss, Detecting a wide range of environmental contaminants in human blood samples—combining QuEChERS with LC-MS and GC-MS methods, Anal. Bioanal. Chem., 2015, 407, 7047–7054 CrossRef CAS PubMed.
- E. L. Schymanski, J. Jeon, R. Gulde, K. Fenner, M. Ruff, H. P. Singer and J. Hollender, Identifying small molecules via high resolution mass spectrometry: communicating confidence, Environ. Sci. Technol., 2014, 48, 2097–2098 CrossRef CAS PubMed.
-
S. Fischer, S17 | KEMIMARKET | KEMI Market List (Version NORMAN-SLU-S17.0.1.0) KEMI_MarketList_03072017.xlsx, 2017, https://zenodo.org/record/2628787#.YUymXYgzY2w, DOI:10.5281/zenodo.2628786.
-
S. Fischer, Market List documentation, Zenodo, https://zenodo.org/record/3549665#.Xe4dJuhKhaQ, accessed 2017-07-03, 2017 Search PubMed.
- J. Holte, L. Berglund, K. Milton, C. Garello, G. Gennarelli, A. Revelli and T. Bergh, Construction of an evidence-based integrated morphology cleavage embryo score for implantation potential of embryos scored and transferred on day 2 after oocyte retrieval, Hum. Reprod., 2007, 22, 548–557 CrossRef CAS PubMed.
- J. Chong, M. Yamamoto and J. Xia, MetaboAnalystR 2.0: From Raw Spectra to Biological Insights, Metabolites, 2019, 9 CrossRef CAS PubMed.
- J. Chong, O. Soufan, C. Li, I. Caraus, S. Li, G. Bourque, D. S. Wishart and J. Xia, MetaboAnalyst 4.0: towards more transparent and integrative metabolomics analysis, Nucleic Acids Res., 2018, 46, W486–W494 CrossRef CAS PubMed.
- A. J. Hackstadt and A. M. Hess, Filtering for increased power for microarray data analysis, BMC Bioinf., 2009, 10, 11 CrossRef PubMed.
- A. Rhenman, L. Berglund, T. Brodin, M. Olovsson, K. Milton, N. Hadziosmanovic and J. Holte, Which set of embryo variables is most predictive for live birth? A prospective study in 6252 single embryo transfers to construct an embryo score for the ranking and selection of embryos, Hum. Reprod., 2015, 30, 28–36 CrossRef CAS PubMed.
- Q. Kang, F. Gao, X. Zhang, L. Wang, J. Liu, M. Fu, S. Zhang, Y. Wan, H. Shen and J. Hu, Nontargeted identification of per- and polyfluoroalkyl substances in human follicular fluid and their blood-follicle transfer, Environ. Int., 2020, 139, 105686 CrossRef CAS PubMed.
- W. M. Henderson and M. A. Smith, Perfluorooctanoic acid and perfluorononanoic acid in fetal and neonatal mice following in utero exposure to 8-2 fluorotelomer alcohol, Toxicol. Sci., 2007, 95, 452–461 CrossRef CAS PubMed.
- A. M. Watkins, C. R. Wood, M. T. Lin and B. D. Abbott, The effects of perfluorinated chemicals on adipocyte differentiation in vitro, Mol. Cell. Endocrinol., 2015, 400, 90–101 CrossRef CAS PubMed.
- T. I. Halldorsson, D. Rytter, L. S. Haug, B. H. Bech, I. Danielsen, G. Becher, T. B. Henriksen and S. F. Olsen, Prenatal exposure to perfluorooctanoate and risk of overweight at 20 years of age: a prospective cohort study, Environ. Health Perspect., 2012, 120, 668–673 CrossRef CAS PubMed.
- R. R. Santos, E. J. Schoevers and B. A. Roelen, Usefulness of bovine and porcine IVM/IVF models for reproductive toxicology, Reprod. Biol. Endocrinol., 2014, 12 CAS.
- E. A. Libersky and D. E. Boatman, Progesterone Concentrations in Serum, Follicular Fluid, and Oviductal Fluid of the Golden Hamster during the Periovulatory Period, Biol. Reprod., 1995, 53, 477–482 CrossRef CAS PubMed.
- W. Xuesong, L. Dong, J. T. Amanda, M. D. Suzanne and K. I. Ray, Estradiol, progesterone, testosterone profiles in human follicular fluid and cultured granulosa cells from luteinized pre-ovulatory follicles, Reprod. Biol. Endocrinol., 2010, 117 Search PubMed.
-
J. R. Pasqualini and F. A. Kincl, Hormones and the fetus, Chapter 1: Hormonal Mechanisms in Reproduction, Pergamon Press, Oxford, 1985 Search PubMed.
- A. Yang, D. Trajkovic, O. Illanes and F. Ramiro-Ibanez, Clinicopathological and tissue indicators of para-aminophenol nephrotoxicity in sprague-dawley rats, Toxicol. Pathol., 2007, 35, 521–532 CrossRef CAS PubMed.
- M. M. Smarr, K. L. Grantz, R. Sundaram, J. M. Maisog, M. Honda, K. Kannan and G. M. Buck Louis, Urinary paracetamol and time-to-pregnancy, Hum. Reprod., 2016, 31, 2119–2127 CrossRef CAS PubMed.
- A. Mosa, A. Gerber, J. Neunzig and R. Bernhardt, Products of gut-microbial tryptophan metabolism inhibit the steroid hormone-synthesizing cytochrome P450 11A1, Endocrine, 2016, 53, 610–614 CrossRef CAS PubMed.
- N. Gharani, D. M. Waterworth, S. Batty, D. White, C. Gilling-Smith, G. S. Conway, M. McCarthy, S. Franks and R. Williamson, Association of the steroid synthesis gene CYP11a with polycystic ovary syndrome and hyperandrogenism, Hum. Mol. Genet., 1997, 6(3), 397–402 CrossRef CAS PubMed.
- T. M. Guerreiro, R. F. Goncalves, C. Melo, D. N. de Oliveira, E. O. Lima, J. A. Visintin, M. A. de Achilles and R. R. Catharino, A Metabolomic Overview of Follicular Fluid in Cows, Front. Vet. Sci., 2018, 5, 10 CrossRef PubMed.
- Bayasula, A. Iwase, H. Kobayashi, M. Goto, T. Nakahara, T. Nakamura, M. Kondo, Y. Nagatomo, T. Kotani and F. Kikkawa, A proteomic analysis of human follicular fluid: comparison between fertilized oocytes and non-fertilized oocytes in the same patient, J. Assist. Reprod. Genet., 2013, 30, 1231–1238 CrossRef CAS PubMed.
-
J. Hollender, B. van Bavel, V. Dulio, E. Farmen, K. Furtmann, J. Koschorreck, U. Kunkel, M. Krauss, J. Munthe, M. Schlabach, J. Slobodnik, G. Stroomberg, T. Ternes, N. S. Thomaidis, A. Togola and V. Tornero, High resolution mass spectrometry-based non-target screening can support regulatory environmental monitoring and chemicals management, Environmental Sciences Europe, 2019, p. 31 Search PubMed.
Footnote |
† Electronic supplementary information (ESI) available. See DOI: 10.1039/d1em00211b |
|
This journal is © The Royal Society of Chemistry 2021 |