DOI:
10.1039/D0EE03757E
(Review Article)
Energy Environ. Sci., 2021,
14, 1701-1721
Life cycle assessment of carbon dioxide removal technologies: a critical review†
Received
30th November 2020
, Accepted 15th February 2021
First published on 16th February 2021
Abstract
A large number of prospective climate scenarios rely on Carbon Dioxide Removal (CDR) technologies to limit global warming below 2 °C. To date, however, a comprehensive understanding of the overall life-cycle environmental impacts of CDR technologies is missing. We present a critical review on conducted Life Cycle Assessments (LCAs) of a comprehensive set of CDR technologies: afforestation and reforestation, biochar, soil carbon sequestration, enhanced weathering, ocean fertilisation, bioenergy with carbon capture and storage, and direct air carbon capture and storage. One of the key observations is that emissions avoided due to substitution of certain processes (due to system expansion in LCA) can be easily misinterpreted as negative emissions, i.e. as carbon removal from the atmosphere. Based on the observed inconsistencies and shortcomings, we recommend to interpret available CDR LCA results with caution. To improve the understanding of environmental implications of CDR deployment, we recommend (1) to conduct LCAs with multiple environmental impact categories, (2) to consider the temporal aspect of emissions in biomass-related CDR technologies, (3) to focus on so far overlooked CDR technologies, (4) to be as transparent as possible regarding methodological choices, (5) to capture environmental side-effects, and (6) to distinguish between ‘avoided emissions’ and ‘negative emissions’ – only negative emissions correspond to permanent removal from the atmosphere. We conclude that more comprehensive and rigorous LCAs are needed to help inform the design of CDR technology portfolios and to aid in anticipatory governance.
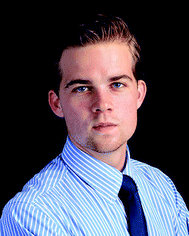
Tom Terlouw
| Tom Terlouw is a PhD candidate in the Technology Assessment group of the Paul Scherrer Institute and in the Institute of Energy and Process Engineering at ETH Zürich. He received his MSc degree in Energy Science from Utrecht University. His personal interests, and current research topics, are sustainability and life cycle assessment, smart grids, optimization and more recently carbon dioxide removal technologies. |
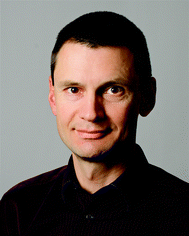
Christian Bauer
| Christian Bauer is senior scientist at the Paul Scherrer Institute in Switzerland. His research domains are environmental life cycle assessment and sustainability assessment, with a focus on mobility and energy technologies and associated fuel supply chains. Carbon dioxide removal options and their environmental impacts have only recently attracted his professional attention. He is member of the board of the ecoinvent association operating the worldwide leading LCA database. |
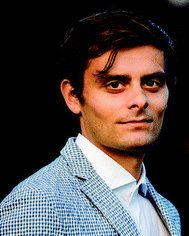
Lorenzo Rosa
| Dr Lorenzo Rosa is a Postdoctoral Research Associate in the Institute of Energy and Process Engineering at ETH Zürich. His research focuses on sustainable water-energy-food systems and the deployment of technologies for climate change adaptation and mitigation. Dr Rosa received his PhD from University of California, Berkeley in 2020. |
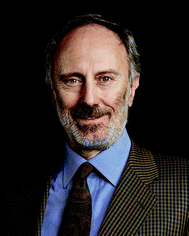
Marco Mazzotti
| Marco Mazzotti, an Italian and Swiss chemical engineer, has been professor of process engineering at ETH Zürich since May 1997. He was coordinating lead author of the IPCC Special Report on CCS (2002–2005), President of the International Adsorption Society (2010–2013), and chairman of the Board of the ETH Zürich Energy Science Center (2011–2017). He was a contributor to the 2007 Nobel Peace Prize awarded to the Intergovernmental Panel on Climate Change (IPCC). He has published more than 350 papers, cited more than 18 000 times, resulting in an H-index of 73 (Google Scholar). Forty-seven doctoral students have graduated with him. |
Broader context
Carbon Dioxide Removal (CDR) technologies are receiving increasing attention from the scientific community, policymakers, and commercial enterprises. While there is agreement that CDR technologies have significant potential to remove greenhouse gases, the understanding of the overall environmental consequences of their large-scale deployment is still very limited. Life Cycle Assessment (LCA) is a suitable methodology to evaluate these environmental impacts and has been applied to CDR schemes. However, our review on CDR LCA studies shows that LCA is often applied in inconsistent, misleading, and ambiguous ways. We provide a perspective on how to conduct future LCA studies of CDR technologies in a consistent way avoiding common mistakes, and we show LCA-related research challenges that should be addressed to aid informed decision making.
|
1 Introduction
As the global economy was affected by Covid-19, annual global fossil CO2-emissions declined from 2019 to 2020 by seven percent to approximately 33 Gt CO2.1 However, this decline is supposed to represent a temporary effect and immediate action aiming at reduction of emissions is required to limit the increase of global temperatures between a maximum of 1.5 °C and 2 °C as stated in the Paris Agreement.2 A variety of models has been developed to assess and determine future energy pathways and associated impacts on climate change.3,4 Integrated assessment models aim to model the future state of energy systems and technologies by generating energy scenarios.5–7
Based on integrated assessment model projections, the Intergovernmental Panel for Climate Change (IPCC) emphasized that the more delay in the peak of global CO2-emissions, the more the world will need to rely on Carbon Dioxide Removal (CDR) technologies (or Negative Emission Technologies (NETs)) to achieve climate goals.8 Most integrated assessment model scenarios rely on the large-scale deployment of CDR technologies to have a bigger chance than 50% to limit global temperature increases to less than 2 °C.9,10
Hence, it is expected that CDR technologies will have a crucial role to reach future climate goals.6,8,9,11–14 In this study, we define CDR technologies as intentional human efforts to remove Greenhouse Gas (GHG) emissions from the atmosphere (based on Minx et al.12).
However, there seems inconsistency in the scientific community on the definition and characteristics of negative emissions derived from CDR technologies. Based on the work of Tanzer and Ramírez,15 we emphasize that CDR technologies should meet the following requirements to result in negative GHG emissions.
First, a CDR technology permanently removes GHGs from the atmosphere. Second, all upstream and/or downstream GHG emissions are quantified and presented in the emission balance of a specific CDR technology. Third, the total removal of GHG emissions must be larger than the total GHG emissions emitted to the atmosphere, through processes required for GHG removal such as energy supply, transport, and/or land use changes.
The latter requirements demonstrate the complex characteristics of CDR technologies. Therefore, environmental assessment methodologies which exclude upstream and downstream environmental impacts are insufficient to assess CDR technology systems.15,23 Alternatively, Life Cycle Assessment (LCA) is the most widely used methodology to assess product systems (i.e. total supply chains, an example is provided in Fig. 2) on their entire life-cycle environmental performance.24–27 Further, LCA is a flexible methodology which offers various modelling choices, and as such can be used to quantify a variety of environmental impacts, such as acidification potential, ecotoxicity and water depletion.
Currently, the deployment of CDR technologies at the gigatonne scale is still debatable and real demonstration projects are scarce.8,12,28 Consequently, most CDR technologies are not sufficiently examined regarding their overall environmental performance. Relying on a gigatonne scale deployment of CDR technologies – without evaluating their performance from a full life-cycle perspective – could result in infeasible climate goals if the environmental benefits are less than expected.12,28 Further, relying on CDR technologies could result in a moral hazard, since policy-makers could use CDR technologies as a safeguard to postpone climate mitigation measures.12,29 Therefore, it is of great importance to evaluate the environmental performance of CDR technologies from a holistic point of view before a large-scale global implementation. To date, a wide body of literature reviews have been conducted on CDR technologies.
For example, Minx et al.12 and Fuss et al.13 presented an in-depth techno-economic review of a portfolio of CDR technologies. Their CDR technology portfolio included Afforestation and Reforestation (AR), biochar, Soil Carbon Sequestration (SCS), Enhanced Weathering (EW), Ocean Fertilisation (OF), Bioenergy with Carbon Capture and Storage (BECCS) and Direct Air Carbon Capture and Storage (DACCS). Technology potentials – in terms of CO2 removal capacity – were presented for 2050 with substantial uncertainties. Further, the National Academies of Sciences, Engineering, and Medicine30 reviewed the current status of different CDR technologies (i.e. AR, SCS, BECCS, DACCS, EW and other emerging CDR technologies) and concluded that a couple of land-based CDR technologies (i.e. BECCS, AR, SCS) are ready for a large scale deployment from a technical and economical point of view. However, the authors concluded that a large scale deployment of these CDR technologies does not remove sufficient CO2 to achieve the climate goals of the Paris Agreement. Moreover, Li and Wright31 presented a CDR technology review of the techno-economic and life-cycle performance of biomass production pathways used for the production of transportation fuels and power generation technologies. Besides, Goglio et al.23 presented a review of the current challenges in LCAs related to Greenhouse Gas Removal Technologies (GGRTs), and gave useful recommendations for future LCAs. The authors recommended to use a functional unit which captures the GHG removal potential of a CDR technology, to define multi-functional units in case of multiple product functions, to adopt multiple environmental impact categories, to apply consistent system boundaries, to utilize best available data, and to consider a method to include temporal aspects of carbon emissions and removals. Further, Smith et al.10 described potential environmental impacts and biophysical limits related to BECCS, DACCS, EW and AR. Their results showed that, to date, there is no CDR technology without any significant impact on land, albedo, energy, water, nutrients or cost. The authors argued to focus on emission reduction instead of relying on CDR technologies. Further, LCA literature reviews have been conducted on specific CDR technologies. For example, Goglio et al.26 focused on the integration of soil carbon changes in LCA, which is related to SCS systems. Additionally, a recent work of Matuštík et al.32 reviewed LCA studies for pyrolysis-based biochar systems with biochar as soil amendment function.
In general, above studies focused on the environmental and economic performance of CDR technologies, determined with different methodologies. The application of different methodologies and assumptions – often without a thorough discussion of their implications – resulted in a large variation of results on environmental and economic indicators per CDR technology. To our knowledge, an up-to-date and critical review on the environmental life-cycle performance and associated LCA modelling choices of a large portfolio of CDR technologies is missing. This study aims to close these research gaps and determines the current status of LCA literature on CDR technologies. We include the same CDR technology portfolio as proposed in Minx et al.12 and Fuss et al.,13 and use LCA as the most appropriate methodology to assess CDR technologies regarding their overall environmental performance. The contributions of this study can be summarized as follows:
• We include a wide variety of CDR technologies in our portfolio: AR, SCS, EW, OF, biochar, BECCS and DACCS. Additionally, we aim to capture LCAs of other promising CDR technologies.
• A comprehensive literature review is conducted to present the current status of LCA of these CDR technologies.
• A critical review is presented on the most important LCA aspects, such as the system boundaries, functional unit, multi-functionality and Life Cycle Impact Assessment (LCIA) categories applied.
• We synthesize the results, discuss current limitations and give guidelines for future (LCA) research on CDR technologies.
The scientific and social relevance of this work can be summarized as follows. First of all, our review should encourage the scientific community to assess the environmental performance of CDR technologies in a holistic and comprehensive way. Further, our recommendations represent guidelines on how to conduct LCAs of CDR technologies in a consistent way.
Table 1 gives an overview of CDR technologies and important definitions.
Table 1 Overview of key definitions and abbreviations used in this paper
Abbreviation |
Meaning |
Definition |
AR |
Afforestation and reforestation |
Planting of trees on places which have not been forested recently, while reforestation can be defined as the restocking of trees or forests on (recently depleted) land.13 |
|
Biochar |
|
Biochar is a condensed carbon rich substance that can be produced at a large scale from biomass, for example with pyrolysis. Biochar can be used as either an energy product or as soil amendment.16 |
|
BECCS |
Bioenergy with carbon capture and storage |
Capture and permanent sequestration of biogenic CO2 during an energy conversion process from biomass (e.g. to produce energy within a power plant).17 |
|
CDR |
Carbon dioxide removal |
Permanent, or temporal, removal of CO2 or GHG emissions from the atmosphere. |
|
DACCS |
Direct air carbon capture and storage |
Capturing of CO2 from the ambient air, due the binding of sorbents, and the subsequent storage of captured CO2 in a permanent way.18,19 |
|
EW |
Enhanced weathering |
Practice to stimulate the process of rock decomposition, while simultaneously increasing cation release to produce alkanity and geogenic nutrients to enhance atmospheric CO2-capture.13,20 |
|
NET |
Negative emission technology |
Technology which aims to capture GHG emissions from the atmosphere and which deployment results in negative GHG emissions, i.e. removal of GHGs from the atmosphere. |
|
OF |
Ocean fertilisation |
Practice to enhance biological processes in oceans to stimulate the uptake of atmospheric CO2.21 |
|
SCS |
Soil carbon sequestration |
Removal of CO2 from the atmosphere due to increased carbon sequestration in soil organic matter, arising from improved management practices (e.g. due to a land management change or land use change).13,22 |
2 Methodology
2.1 Life cycle assessment
LCA is required to obtain the overall environmental impacts of CDR technologies. The goal of an LCA is to determine all environmental impacts of a product or service over its lifetime.25,27,33 LCA is particularly suitable to assess product systems, which have a significant impact around all stages of their life-cycle, including the production and the end-of-life phase as is the case for most CDR technologies.15,23 LCA methodology is defined by a series of standards from the International Organization for Standardization (ISO), ISO 14040 and ISO 14044.34,35 Further, ISO 14067 offers a framework, and corresponding regulations, for the determination of the carbon footprint of products.36
2.2 Literature search
Scopus, Web of Science and Google Scholar were explored for our literature search. Search terms related to specific CDR technologies were generated, such as ‘Negative Emission Technologies’ or ‘Greenhouse Gas Removal Technologies’ or ‘Greenhouse gas Removal’ and/or ‘Carbon Dioxide Removal’. Further, we added the specific CDR technology to the search term, e.g. ‘afforestation or reforestation’ in case of AR. In addition, we added our targeted methodology ‘Life Cycle Assessment’ (or ‘Life-Cycle Analysis’ or ‘LCA’) to the search terms, to capture a specific technology assessed with LCA. We applied these search queries for all CDR technologies, by substituting ‘afforestation or reforestation’ with the name of the specific CDR technology. The literature search has initially been executed in March and April 2020 and has been updated in November 2020, without any limits in terms of publication date. Note that we only focus on LCA studies in this work, and therefore exclude other environmental studies which do not assess CDR technologies using the LCA methodology.
2.3 Structure of our review
This review will be structured along the selected portfolio of CDR technologies. Hence, we aim to review LCAs for each CDR technology by giving a critical review on essential LCA characteristics, such as the functional unit, system boundaries, multi-functionality and environmental impact categories. Additionally, overview tables are shown in Section 8 of the ESI,† where the LCA parameters are presented for selected LCA studies, including the results on Global Warming Potential (GWP) (sometimes referred as carbon footprint, GHG emissions or impacts on climate change). Further, we discuss crucial aspects and ongoing LCA-related debates for a specific CDR technology.
3 Review
In this section, we shortly present an overview of the current state and prevalence of scientific publications on CDR technologies, evaluated by means of LCA. Fig. 1 demonstrates the increasing coverage of LCA studies on CDR technologies in academic literature. The first publications were generated in 1995, more publications followed after 2005. An increasing trend, in the annual number of publications, followed after 2006. Interestingly, there was a peak in the number of publications on LCAs of CDR technologies in 2015, which was the year most countries agreed on the climate actions of the Paris Agreement. The number of citations on LCAs of CDR technologies almost grew exponentially in the last decade, which again shows the increased popularity of CDR technology related LCAs. The pie chart in Fig. 1 shows the main research fields of LCAs on CDR technologies (derived from Research Areas in Web of Science37). The main research fields are environmental sciences ecology, engineering and energy fuels, respectively.
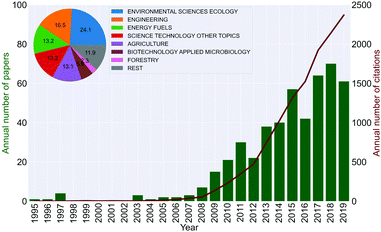 |
| Fig. 1 Overview of annual number of published articles and citations which included a form of LCA and NET in their abstract, title or keywords. Additionally, main research fields of LCA studies of CDR technologies are indicated in the pie chart. Data is obtained from Web of Science.37 For research areas, we showed the share of the number of times the research area was attributed to a paper in research areas under categories/classification. | |
In the following sections, we discuss each of the CDR technologies, which are categorized as AR, Biochar, SCS, EW, OF, BECCS, DACCS and other promising CDR technologies. With ‘other promising CDR technologies’, we refer to CDR technologies which could not be categorized in our CDR technology portfolio. We aim to give a short definition (see also Table 1) and introduction to each CDR technology and refer the readers to the most comprehensive sources for a specific CDR technology.
3.1 Afforestation and reforestation (AR)
Afforestation refers to the planting of trees on places which have not been forested recently, while reforestation is defined as the restocking of trees or forests on recently depleted land.13 In AR systems, CO2 is (temporary) removed from the atmosphere due to sequestration of C by the forest as a result of the photosynthesis process.38
3.1.1 LCAs.
We found four studies that meet our search criteria, please refer to the overview Table in the ESI.† Therefore, LCA studies on AR are relatively scarce, while LCAs on the forest production sector have been widely conducted.39 A short overview and summary of these studies is presented in the ESI.†
System boundaries.
AR LCA studies usually include two stages determining the GHG balance, namely carbon dynamics of the forest and life-cycle GHG emissions derived from forest management. The studies identified included different management activities such as seed production, planting of trees, seedling cultivation, fertilization, harvesting, thinning and transportation. Some papers used a cut-off of activities in their system boundaries. For example, the work of Gaboury et al.40 excluded the production of infrastructures since no life cycle inventory data was available at the time of assessment. Also, the work of García-Quijano et al.41 mentioned the importance of including wildfire disturbances, although this was not considered in their work.
Functional Unit.
Most AR papers used a land area-based functional unit, i.e. per hectare of forest or land. This functional unit seems reasonable, since the main performance indicator of AR LCAs is the uptake of CO2 per unit of land.
Multi-functionality.
Usually, the main – and only – function of the AR product system is plantation growth which in turn could result in carbon sequestration. Hence, no useful co- or by-products are generated and allocation was not applied in any of the AR papers.
Environmental impact categories.
In general, most studies reported annual carbon sequestration rates between 0.8 and 1.0 tC ha−1 year−1. Our selection of LCA studies revealed low carbon emissions from forest management practices, e.g. Lun et al.42 reported 1% carbon emissions of the total carbon sequestration per rotation period for a plantation forest. However, environmental impacts on other LCIA categories are not well-known. Most (n = 3) selected AR studies ignored the importance of environmental impact categories, other than carbon storage or GWP. Only the work of García-Quijano et al.41 assessed additional environmental impacts, namely land use by also addressing soil, biodiversity, water and vegetation indicators.
From the limited number of studies,40–43 we can observe that there are different modelling approaches used. This can be explained by the carbon models used (see Section 1.1 in the ESI,† for more explanation), local system boundaries (tree species), differences in LCA modelling choices (e.g. system boundaries), and the inclusion of potential disturbances during the assessment period.
3.1.2 Reversibility and (indirect) land use change ((i)LUC).
In addition, CO2 storage in forests can be reversible, since its permanency depends on many factors and potential disturbances such as wildfires, water scarcity, storms, flooding and pests infestation.40,44–48 Further, direct and indirect land use change (i.e. LUC and iLUC) can also greatly influence the GHG emission balance (please refer to Section 1.2 in the ESI,† for more explanation).49,50
3.1.3 Timing of emissions.
Another crucial and complex aspect of AR, and other biomass related CDR technologies, is the timing of GHG emissions during the assessment period of an LCA. Conventional LCA is based on static modelling of GHG emissions which thereby neglects the temporal aspects of the occurrence of GHG emissions. However, the inclusion of these temporal aspects on GHG emissions could have a significant impact on the LCA results.51–53 A more comprehensive discussion on the timing of GHG emissions is provided in Section 1.3 of the ESI.†
3.2 Biochar
Biochar can be defined as a carbon rich material derived from the thermal degradation of biomass, under limited oxygen conditions.54–56 Within a biochar system, CO2 is removed from the atmosphere due to the sequestration of carbon by photosynthetic organisms.54 This harvested biomass is subsequently used to produce biochar, derived from a thermochemical conversion process, such as slow pyrolysis,54,57 fast pyrolysis,58,59 gasification60 or torrefaction.61,62
Pyrolysis generates multiple by-products, such as bio-oil and syngas.54,55 The resulting biochar product can be used as sorbent,63 fuel,64 catalyst procursor,65 or as soil amendment to store captured carbon in soils.66,67 Soil amendment with biochar seems to offer most potential in terms of CO2 removal and storage,32 hence we will focus on soil amendment in our review. One beneficial characteristic of biochar is its stability in soil over time (reported values in the range of decades to ∼1000 years).68,69 If the carbon is stored in the soil over a long time horizon, the extracted CO2 can be considered as permanently stored.13,70 Further, biochar could increase crop productivity as a result of improved soil fertility due to increased water retention and reduced nutrient leaching.16,54,56
An overview of biochar applications and production pathways is provided in Wang et al.54 and Sun Cha et al.71 A recent review paper of Matuštík et al.32 focused on LCAs of pyrolysis based biochar systems with a soil amendment function, and exhibited hotspots (i.e. the main contributors) in life cycle inventories of biochar, such as the selection of feedstock (to produce biochar), stability of biochar in soils and the performance and conditions of the pyrolysis unit. The next sub-section builds upon the work of Matuštík et al.32 We discuss associated key issues and present the most important LCA parameters in the ESI.†
3.2.1 LCAs.
Generally speaking, there is a wide body of LCAs conducted on biochar systems. The ESI,† presents an overview of selected literature. We found 36 LCA studies on biochar, hence, a popular research topic (i.e.57–60,72–103). All selected LCAs report significant carbon abatement and/or negative GHG emission potential when the biochar is used as soil amendment. However, GWP impacts can hardly be compared, since each biochar LCA exhibits specific boundary conditions, use different functional units and parameters, such as feedstock consumption and LCA modelling choices. Three representative biochar LCAs are presented in the ESI.†
System boundaries.
Biochar studies usually include activities such as fertilizer use and production, feedstock generation, feedstock preparation or collection (e.g. storage and drying), transportation, the conversion process (e.g. fast/slow pyrolysis), and the application of biochar as soil amendment. Most biochar studies applied a cradle-to-grave approach, hence they included all environmental impacts during the entire life-cycle. We found a significant difference between the type of feedstock used for the generation of biochar. The main difference is due to the use of agricultural residues or energy crops. Residues and waste based feedstock are usually associated with lower environmental impacts, since environmental impacts in the feedstock production stage are hardly present (e.g.60,94,101), while energy crops generate additional environmental impacts due to feedstock production.72 A discussion of the utilization of waste feedstocks is presented in Section 4.2.5.
In general, the LCA community acknowledges that LCAs should be performed on a cradle-to-grave basis to capture all life-cycle environmental impacts.34 We want to emphasize the variation in terms of included side-effects between studies. With side-effects we refer to positive and negative consequences of the implementation of a CDR technology.13 We found that biochar LCA studies frequently ignored to address potential side-effects within their system boundaries – such as (i)LUC and albedo changes – although they have a great influence.
For example, Roberts et al.72 clearly emphasized the crucial role of the inclusion of iLUC in LCA, since the scenario without iLUC resulted in negative GHG emissions (−442 kg CO2-eq. t−1 switchgrass), while the scenario with iLUC (GHG penalty based on Searchinger et al.104) resulted in environmental burdens (36 kg CO2-eq. t−1 switchgrass). Moreover, the surface albedo could change considerably as a result of the dark color of biochar when amended to the top soil, which in turn affects GWP impacts.105–107 Meyer et al.107 aimed to quantify the climate change impact of albedo changes of biochar, and examined this albedo impact on GWP characterization factors and applied this in an LCA. Their results demonstrated that the consideration of albedo change in biochar systems could reduce climate mitigation benefits by 13–22%.
Further, multiple studies acknowledged that environmental impacts of biochar systems depend on site-specific conditions due to local ecosystem characteristics (e.g. albedo, soil erosion, land use type, and climate).74,86,98 Related to site-specific issues, a great variability has been found on the carbon stability of biochar in soils. Carbon stability in soils is one of the most sensitive parameters for the negative GHG emission potential of biochar.57,73,103 This site-specific parameter can vary from decades to thousands of years.68,69 Overall, the inclusion of these side-effects within the system boundaries of an LCA are crucial for the final results.
Functional unit.
In general, our selection of biochar LCA studies distinguished between two types of functional units: mass unit of feedstock used or managed (e.g.60,72,88,90,94,101,102), or mass unit of biochar produced and/or applied (e.g.76,78,91,93,95,99). Some studies generated alternative functional units to demonstrate the impacts per land area (e.g.57,79,82,97). Few studies presented extended functional units based on the by-products produced (e.g.57,80).
Multi-functionality.
An important characteristic of the production of biochar (e.g. via pyrolysis) is its multi-functionality resulting in by-products, such as bio-oil and syngas.54 These by-products can have additional economic and/or environmental value when consumed by other activities, such as the generation of hydrocarbons (e.g. from syngas) and/or electricity (e.g. from bio-oil). From an LCA point of view, co-products induce complexity: LCA practitioners can choose between different approaches regarding how to deal with multi-functionality, such as allocation of process exchanges to individual products on an economic or mass basis, or system expansion (i.e. reduce the total impact by the impact of avoided processes).
When product systems generate more than one (valuable) product, ISO recommends to use system expansion to avoid allocation procedures.35 Consequently, system expansion or substitution was used in approximately 70% of the biochar LCA studies to deal with multi-functionality of product systems. Most studies (e.g.59,87,90) assumed an avoidance of electricity and heat production due to the use of by-products of the biochar production process. Some other studies (e.g.59,89,99) also included the avoidance of fertiliser consumption, since biochar could enhance soil fertility, i.e. reduce N-fertilization.54,56 Moghaddam et al.82 compared the GWP results between physical allocation and system expansion, and demonstrated significant differences between the two approaches. For some other studies, it remained unclear how and if they handled multi-functionality in their product systems, although this modelling choice has a considerable effect on LCA results of biochar systems.82 A thorough discussion of these modelling choices is outside the scope of this review, although they are crucial for the interpretation of the results.108–111
The overview table of biochar studies – presented in the ESI,† – reveals substantial ‘negative’ GHG emissions reported by most biochar LCA studies, due to the avoidance of electricity or heat production using fossil fuels (e.g.57,72,73,101,103) or the avoidance of fertilizer production (e.g.72,102). However, these 'negative' emissions could be misinterpreted,15 hence the question is, if the results are meaningful for the determination of truly negative GHG emissions equivalent to the permanent removal of CO2 from the atmosphere. Most biochar LCA studies used a system expansion (or substitution) approach, assigning environmental credits for the avoidance of processes present in a reference system due to the use of by-products of biochar production. The avoided burdens of these substituted processes are accounted for as negative environmental impacts (with a minus sign), hence ‘negative emissions’. In most situations, when system expansion is applied, such ‘negative’ emissions show environmental impacts which can be avoided compared to a reference system. Therefore, such ‘negative’ emissions do not imply the capturing of GHGs from the atmosphere, hence cannot be categorized as negative GHG emissions according to the definition of CDR technologies (see Section 1).
An example of multi-functionality, associated modeling choices, implications of certain choices and recommendations is provided in Section 4.3.7.
Environmental impact categories.
The overview table on biochar studies in the ESI,† shows that all studies assessed the GWP impact category (as GWP, CO2-eq. or GHG emissions). Approximately 50% of the studies included additional environmental impact categories. Acidification potential and eutrophication potential were the most assessed environmental impact categories after GWP, and were covered in approximately 30% (n = 11) of the biochar LCA studies. Multiple studies revealed the importance of the inclusion of other impacts categories, such as acidification, eutrophication and human health related environmental impacts (e.g.73,74). These environmental impacts can be significant, and could compromise GHG related benefits in some situations.
3.3 Soil carbon sequestration (SCS)
SCS refers to the removal of CO2 from the atmosphere due to increased carbon sequestration in soil organic matter, arising from improved management practices (e.g. due to a land management change or LUC).13,22 If soil carbon remains in place over long time periods, the sequestered soil carbon can be considered as permanently stored.13,22 However, note that SCS could be reversible and is time limited, usually up to a couple of decades.112–114
The quantification of environmental impacts of SCS is a complex activity, due to the multi-functionality of product systems (e.g. milk and meat production due to improved agricultural practices). Earlier work emphasized that LCA is an appropriate method to assess agricultural practices due to its holistic approach, although there is no consensus on how to account for soil carbon changes and sequestration.26 Hence, many agricultural LCA studies still exclude SCS and/or soil quality in LCAs.26,115,116 Further, van der Werf et al.116 argued that LCAs on agricultural systems usually neglect essential environmental issues (e.g. land degradation, biodiversity and pesticide effects).
We refer to the review of Goglio et al.26 for an overview of the integration of soil carbon change (and sequestration) methodologies and their application in LCA. Our findings build upon the work of Goglio et al.26 and van der Werf et al.116
3.3.1 LCAs.
We found 35 studies (i.e.113,115,117–149) which met our search criteria, these are presented in the overview table of SCS studies in the ESI.† Most SCS LCA studies included SCS in their product system, although the main purpose of these studies was not the assessment of SCS as a self-standing CDR technology. A summary of a couple of such studies is presented in the ESI.†
Our selection of LCA studies demonstrated a small, but significant, offset of GWP from SCS as a result of its carbon sequestration potential. However, some SCS studies showed a loss of soil carbon instead of SCS for a variety of cropping systems (e.g.130,138,142). Further, most studies reported total carbon emissions for the entire product system, but not negative carbon emissions due to SCS as such. The SCS potential mainly depends on the methodology and goal, the function(s) of the product system, and the (local) conditions of a case study.
System boundaries.
We found that most studies on SCS excluded some life-cycle stages. Many studies focused on cradle-to-(farm)gate (e.g.120,122,127,139). Hence, these studies excluded activities of the products delivered after the (farm)-gate stage. For livestock related LCAs, medicines and machineries were usually excluded (e.g.115,135,145,147). The inclusion of all upstream GHG emissions (due to agronomic management) in a study by Gao et al.138 resulted in an offset of all negative GHG emissions and resulted in positive GHG emissions.
We observed that most SCS studies neglected the impact of side-effects. For example, iLUC was usually excluded in the system boundaries, while some of the studies could be affected by iLUC. Whether including iLUC or not can have, however, large implications on LCA results: for example, multiple studies proposed land use change for a different grazing strategy (e.g. in136,147,149). Some of these grazing strategies were more sustainable, although they produced less meat or milk. One could expect that the demand for these products will not change. Consequently, land elsewhere would be converted to agricultural land, which would result in additional environmental impacts and these could offset negative GHG emissions obtained from sustainable grazing. On the one hand, such side-effects could have a big influence on LCA results. On the other hand, they are often highly uncertain: van der Werf et al.116 emphasized that cause-effects of (i)LUC are not well known. Further, the authors argued that agricultural LCAs lack the ability to include the total environmental impact of ecosystem services due to its product based approach, especially when switching to a more sustainable agricultural strategy.116 Besides, there is still no consensus on how to include iLUC in LCA.116 Schmidt et al.,150 Sanchez et al.151 and Finkbeiner152 give possible guidelines on how to include iLUC in LCA.
Functional unit.
LCA studies on SCS showed a wide variety of functional units applied. Functional units can be categorized based on the goal of an LCA. Studies that included SCS as part of crop management or production, commonly applied a functional unit in the form of land use ‘ha per year’ (e.g.138,139,141,143,146), the amount of energy generated from these crops (e.g.137,139), or as crop product delivered ‘kg of product’ (e.g.113,117,120,133). In contrast, dairy LCAs usually presented their functional unit per liter or kg of dairy product (e.g.115,118,145). Alternatively, LCAs for the livestock industry frequently used 'kg of live weight' as functional unit (e.g.124,126,135).
Multi-functionality.
SCS was usually included as a separate process within the product system. Consequently, the impact of SCS depends on the (co-)product SCS is attributed to. Allocation was frequently applied to consider the total value of a multi-functional product system, for functions such as milk, meat and crops. For example, livestock studies frequently allocated co-products based on mass (e.g.123,124,135) or economic value (e.g.117,130). For cropping systems, allocation was usually not required with gate-to-gate system boundaries, since the only function was to deliver the feedstock to the farm gate. Few studies119,122,147 showed the influence of different allocation methodologies and demonstrated the importance of modelling choices.
Environmental impact categories.
All SCS studies assessed the product system regarding GWP. Approximately 30% of the SCS studies also included eutrophication potential (n = 11) and/or acidification potential (n = 10). However, we noticed that only 30% (n = 11) of the studies assessed impact categories other than GWP. However, Brandão et al.139 demonstrated that a good performance on GWP could result in a poor performance on other impact categories, such as acidification and eutrophication potential. Further, different research discussed the importance of ecotoxicity (e.g. to consider pesticide issues) and biodiversity as impact categories in agricultural LCAs.116,145 However, only 14% of selected SCS studies included a toxicity indicator (n = 5), and only 6% (n = 2) of our sample considered biodiversity as impact category.
3.3.2 How to account for soil carbon changes in LCA?.
Most SCS studies aimed to capture the soil organic carbon content over a certain period of time, accounting for different management practices. However, there is no agreement in the LCA community on how to account for SCS. This ongoing debate is discussed in Section 3.1 of the ESI.†
3.4 Enhanced weathering (EW)
EW is a practice to stimulate the process of rock decomposition, while simultaneously increasing cation release to produce alkanity and geogenic nutrients to enhance atmospheric CO2-capture.13,20 In EW systems, CO2 is removed from the atmosphere due to additional CO2-uptake from accelerated weathering of rocks.153 The extracted CO2 can be considered as permanently stored when the (fine grained) rock materials are dissolved in the presence of water and CO2, and the dissolution products are transported to the ocean where captured CO2 is permanently stored in deep ocean layers.154,155 One should notice that weathering of rocks is a natural process, although the natural process is rather slow (order of centuries to thousands of years, depending on the particle and its size).155 EW aims to accelerate this process considerably in order to shift the impact of weathering to human time scales.13,156
We found one comprehensive LCA study on EW.157 Hence, we identify that this CDR technology is an underexamined research direction in terms of LCA. This study is described in the ESI.†
The implementation of EW could potentially result in many side-effects. For example, EW modifies the chemical properties of ecosystems, such as soil, land and water and as such could influence ecosystems and agricultural productivity.20,156,158,159 We refer to the review of Hartmann et al.20 for potential side-effects of EW. Negative side-effects could be quantified using environmental impact categories – e.g. ecotoxicity – in LCA.
3.5 Ocean fertilisation (OF)
OF can be described as a geoengineering practice to enhance biological processes in oceans to stimulate the uptake of atmospheric CO2.21 The goal of OF is to permanently store significant amounts of atmospheric CO2 in deep oceanic layers.21,160 OF can be reached by mixing or supplying limiting nutrients, such as phosphorus (P), nitrogen (N) and iron (Fe), to enhance growth rates of marine species (e.g. algae).21,161
No OF LCAs have been found. Currently, there is a lack of long-term OF experiments.21 OF might include many undetermined side-effects, such as the possible reversibility of carbon storage.21 The work of Williamson et al.21 presents a critical review and an explanation for the immature research state of this CDR technology.
3.6 Bioenergy with carbon capture and storage (BECCS)
BECCS is the capture and permanent sequestration of biogenic CO2 during an energy conversion process from biomass (e.g. to produce energy within a power plant).17 BECCS can result in net negative GHG emissions when the amount of CO2 extracted from the atmosphere (and the permanent storage) offsets GHG emissions from the life-cycle of BECCS systems.162,163 We refer to Kemper162 for a comprehensive review on BECCS.
3.6.1 LCAs.
We found 11 BECCS LCA studies which matched our search terms,163–173 see the overview table of BECCS studies in the ESI.† Most LCA studies exhibited negative GHG emissions from BECCS systems, although there were system layouts, which resulted in positive GHG emissions, mainly due to upstream activities such as feedstock production, solvent preparation168 and also side-effects (e.g. iLUC163). In the ESI,† we describe three representative LCA studies of BECCS.
System boundaries.
BECCS systems usually include activities such as feedstock production, feedstock preparation, feedstock conversion (e.g. combustion), carbon capture from feedstock conversion, (geological) storage and transportation of CO2. We found significant differences in the application of system boundaries in BECCS LCA studies, which often leads to substantial differences in LCA results.
Using a gate-to-gate approach excludes upstream and downstream activities and therefore cannot be considered as an LCA, as these activities usually have a considerable impact. Correctly implementing an LCA approach corresponds to cradle-to-grave boundaries (i.e.Fig. 2 applied on a BECCS system, modified and adopted from Tanzer and Ramírez15). Additional environmental impacts can result from (i)LUC when dedicated crops are used as biomass source. In these cases, environmental impacts of side effects should be considered as these can be significant.104,163 However, including such side-effects does not seem to be common practice.
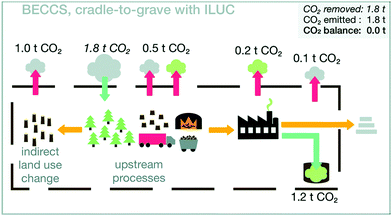 |
| Fig. 2 Illustration of the inclusion of iLUC in the system boundaries of BECCS, adapted from Tanzer and Ramírez15 with permission from The Royal Society of Chemistry. | |
Fajardy and Mac Dowell163 included different types of feedstock and (i)LUC, hence the chosen system boundaries correspond to Fig. 2. The latter study also demonstrated the crucial role of the inclusion of temporal aspects on GHG emissions, which is further discussed in Section 4.1 of the ESI.†
174 Multiple other studies excluded (i)LUC considerations since waste streams (e.g. municipal solid waste, landfill gas and/or organic residues) were used as feedstock (e.g.164–166,169). Note that the utilization of municipal solid waste as feedstock partially results in non-biogenic CO2-emissions due to the share of non-biogenic waste. Many studies use waste streams as feedstock which avoids ecological (e.g. LUC and iLUC) and social issues (e.g. competition for food), resulting from the use of energy crops.49 However, availability of future biomass waste stream needs to be considered from a system perspective – we discuss this issue in Section 4.2.5.
The above examples clearly demonstrate the differences between the chosen system boundaries of BECCS LCA studies. Sometimes it remained unclear how and why the authors have chosen their system boundaries though, also since these studies did not include complete life cycle inventories.
Functional unit.
Most studies used a functional unit based on the mass of feedstock used (e.g.164,172,173) or per unit of power generated (e.g.165,167,168,171).
Multi-functionality.
Some studies applied substitution to combined heat and power generation with credits for avoided heat and/or electricity generation (e.g.164,166,172). In general, we observe a lack in transparency in some studies, since it remained unclear if and how multi-functionality was dealt with.
Environmental impact categories.
More than 80% of BECCS LCA studies (n = 9) included multiple environmental impact categories. For example, more than 45% (n = 5) of our sample included a water footprint and/or ozone depletion indicator. Hence, it seems that there is an agreement to consider multiple environmental impact categories for the assessment of BECCS systems.
3.7 Direct air carbon capture and storage (DACCS)
DACCS can be described as the capturing of CO2 from ambient air, due to the use of sorbent materials, and the subsequent storage of captured CO2 in a permanent way.18,19 Comprehensive discussions on Direct Air Capture (DAC) systems are provided in Fasihi et al.,175 Sanz-Pérez et al.176 and Bui et al.,177 respectively.
3.7.1 LCAs.
Only three comprehensive DACCS LCA studies have been found.178–180 These studies are shortly summarized in the ESI.† In the following sections we present some key elements for future work on DACCS LCAs.
System boundaries.
A cradle-to-grave approach is required to include end-of-life considerations. End-of-life environmental impacts could originate from the injection, (re)compression, infrastructure requirements (e.g. pipelines) and transportation of CO2.180–183 All DAC(CS) LCA studies excluded at least one of these potential environmental impacts during the carbon storage stage, except a recent study of Terlouw et al.180
Functional unit.
For DACCS systems, the main objective is to remove CO2-emissions from the atmosphere. Recommendations on an appropriate functional unit for CDR technologies are presented in Section 4.3.4. All DACCS LCA studies used the amount of CO2-removal from the atmosphere as functional unit.
Environmental impact categories.
DACCS LCA showed a significant CO2 removal potential. However, this sometimes came at the expense of other environmental burdens due to increased use of background processes and fuel consumption to capture CO2 from the atmosphere.180,184 This raises questions about the environmental trade-offs coming along with removing CO2 from the atmosphere via DACCS.
3.7.2 Proprietary technology.
DACCS is an emerging – and proprietary – technology, hence DAC(CS) publications often lack transparency. Consequently, detailed life cycle inventory of DAC(CS) systems is hard to find. Therefore, we argue for more transparency in the data provision of DACCS systems (see Section 4.3.1). More discussion and examples are provided in Section 5.1 of the ESI.†
3.8 Other promising CDR technologies
This section presents LCA studies on promising CDR technologies, which could not be categorized according to our CDR technology portfolio.
The review of Fuss et al.13 mentioned the potential of methane and nitrous oxide removal technologies185–187 and blue carbon.188 To our knowledge, no LCA studies have been conducted on methane and nitrous oxide removal technologies. Further, we have excluded carbon capture and utilization as GHG removal technology, since carbon capture and utilization does not meet our definition of a CDR technology. One LCA study has been found on marine carbon sequestration.
The study of N'Yeurt et al.,189 a simplified LCA, presented ocean afforestation as a potential CDR technology. Ocean afforestation aims to increase natural populations of algae feedstocks in oceans to remove CO2 from the atmosphere. The authors argued that ocean afforestation could theoretically offset all anthropogenic CO2-emissions. Hence, more sophisticated LCA of ocean afforestation should be conducted.
Lastly, another interesting CDR technology research direction is using infrastructure to store CO2.190 For example, timber use and concrete carbonation can contribute to building materials in order to store CO2 over a long time horizon.190,191
4 Discussion
4.1 Synthesis
Table 2 summarizes our main findings for each CDR option in a comparative way and highlights the main gaps and uncertainties that need to be addressed in future research, in particular LCA-based research. Fig. 3 visualizes Table 2 and shows how each CDR technology scores on essential characteristics, the GWP indicator (equivalent to the net-removal of greenhouse gases from the atmosphere), and estimates the storage stability of CO2 for a specific CDR technology.
Table 2 Overview of CDR technologies and assessment of essential characteristics in the LCA context. Complete classifications, rubrics and explanations are provided in Section 7 of the ESI. n.a. not available (e.g. due to a lack of LCA studies), ◁ based on one LCA study only
Status Quo |
Coverage in LCA literature |
Coverage of LCIA categories |
Multi-functionality |
Correct application of ‘negative emission concept’ |
Reliability of present LCA results |
Importance of side-effects |
Main uncertainties |
Scale |
Low-high |
Low-high |
Not relevant-relevant |
Hardly-always |
Low-high |
Less important-very important |
Afforestation/reforestation (AR) |
Medium |
Low |
Not relevant |
Always |
Medium |
Important |
Temporal disturbances, Local conditions |
Biochar |
High |
Medium |
Relevant |
Hardly |
Low |
Very important |
Biochar stability in soils, Local conditions |
Soil carbon sequestration (SCS) |
High |
Medium |
Relevant |
Hardly |
Low |
Very important |
Application in LCA, Local conditions |
Enhanced weathering (EW) |
Low |
Medium |
Not relevant |
Always◁ |
High◁ |
Very important |
Limited experiments, Weathering rates |
Ocean fertilization (OF) |
None |
None |
Not relevant |
n.a. |
n.a. |
Very important |
Reversibility, Unknown side-effects |
Bio-energy with carbon capture and storage (BECCS) |
High |
High |
Relevant |
Sometimes |
Medium |
Very important |
Temporal aspects, Availability of biomass, Carbon storage sites |
Direct air carbon capture and storage (DACCS) |
Medium |
Medium |
Not relevant |
Always |
High |
Less important |
Energy consumption, Carbon storage sites |
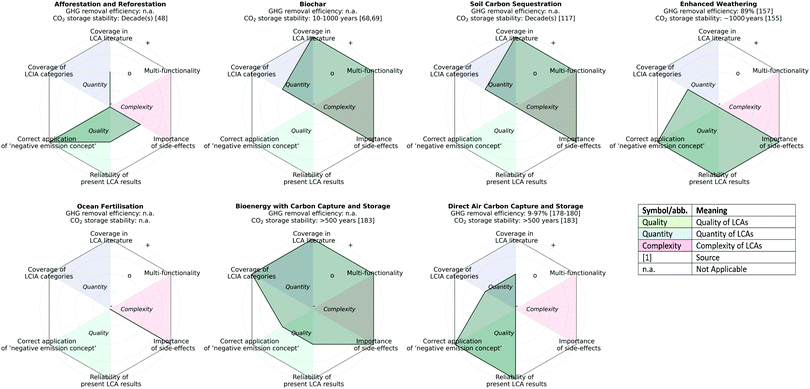 |
| Fig. 3 Spider graphs synthesizing our findings in line with Table 2. The scores in the spider graph are based on the classifications presented in Table A1 of Section 7 in the ESI.† Complete classifications, rubrics and explanation are also provided in Section 7 of the ESI.† Further, we estimate the GHG removal efficiency – i.e. percentage net GHG removed per gross CO2 captured – and the stability of CO2 storage per CDR technology, based on the available literature. | |
4.1.1 Coverage in LCA literature.
With coverage in LCA literature, we refer to the number of LCA studies per CDR technology. Many LCA studies have been performed on biochar (n = 36) and SCS (n = 35). However, SCS was included in larger (agricultural) product systems, but was never assessed as a self-standing CDR technology. Further, fewer LCA studies were conducted on BECCS (n = 11) and AR (n = 4). A limited number of LCA studies was found on DACCS (n = 3), EW (n = 1) and OF (n = 0). EW and OF are the least explored CDR technologies in terms of LCAs. Hence, we could not generate meaningful conclusions for these CDR technologies other than that it is of great importance to conduct comprehensive LCAs and experimental case studies for these CDR options.
4.1.2 Coverage of LCIA categories.
With coverage of LCIA categories, we refer to the variety of LCIA categories considered. We have excluded OF in this assessment category, due to a lack of LCA studies for this CDR technology. In general, biochar and SCS were assessed on a wider range of environmental impact categories. However, still 50% of the biochar LCA studies excluded other impact categories than impacts on climate change. SCS studies usually excluded relevant impact categories, such as biodiversity and ecotoxicity indicators.116 AR LCAs were almost entirely focused on the GWP and/or carbon sequestration impact categories. In addition to impacts on climate change, BECCS LCA studies frequently included a water footprint (or water scarcity), ozone depletion, and ecotoxicity environmental impact category, but usually excluded land use-related impacts. Especially the inclusion of a water footprint seems to be important in BECCS, as Rosa et al.192 estimated a comparably high prospective water consumption for BECCS to meet climate targets. This trade-off requires a thorough evaluation considering local boundary conditions, since water is a local resource and nowadays water scarcity is already widespread and perceived as a socio-economic risk to human activities.192,193
4.1.3 Multi-functionality.
With multi-functionality, we refer to the number of functions the product system provides. Biochar and SCS systems usually provide multiple product functions. For biochar, this could be the removal of GHG emissions from the atmosphere, improved soil fertility (from soil amendment of biochar) and energy production (from syngas and/or bio-oil as by-products of pyrolysis). For SCS, we identified multiple product system functions depending on the agricultural system evaluated. Also LCA of BECCS often includes a couple of product system functions, such as energy generation and carbon removal due to CCS. Multi-functional product systems generate additional complexity in modelling choices (see Section 4.3.7) and often impede comparison of results from different LCA studies.
In contrast, AR, EW, OF and DACCS represent targeted CDR technologies with limited, or no multi-functionality. The main purpose of these CDR technologies is to remove GHG emissions from the atmosphere. However, additional social, economic or environmental benefits and/or burdens could be generated from side effects. For example, an improved performance on biodiversity and soil stability for AR or increased crop productivity through EW.159
4.1.4 Correct application of negative emissions.
Correct application of negative emissions refers to a proper application and use of the concept of 'negative emissions' complying with the definition of negative emissions as embedded in the CDR technology definition in Section 1. A significant number of BECCS, SCS and biochar LCA studies used system expansion to avoid allocation of multi-functional processes. One implication of system expansion is that reported negative emissions are often not meaningful for CDR technologies, since no distinction is made between avoided and real negative emissions (CO2 removed from the atmosphere). Hence, one should be careful when interpreting LCA results from LCAs applying system expansion. We found an appropriate application of negative emissions in DACCS and EW (although based on only one LCA study); this can be explained by their focus on GHG removal only.
4.1.5 Reliability of present LCA results.
Reliability of present LCA results refers to the quality and reliability of the results currently presented in CDR technology LCA studies. Do the available LCA results represent the overall environmental performance of different CDR technologies and is their quality sufficient for decision support? LCA results for EW seem to be relatively reliable, although based on only one study. AR and BECCS systems were widely covered by different LCAs. However, AR LCA was limited in the consideration of LCIA categories, while (especially) BECCS LCA excluded important side-effects. LCA results for DACCS seem to be reliable, while the interpretation of LCA results for biochar and SCS systems is complex. The three DACCS studies agree on the fact that the carbon removal efficiency is largely driven by the energy supply requirements for the DAC process. However, the majority of studies excluded a detailed analysis of the carbon storage stage thereby excluding possible environmental impacts, due to the transportation of CO2, injection and compression of CO2, and their related infrastructure requirements. For biochar and SCS, the results could be easily misunderstood due to the often misleading utilization of the negative sign (i.e. ‘–‘) for both negative emission and avoided emissions. Further, SCS was never assessed as self-standing CDR technology and was usually included as process within the system boundaries of agricultural LCA studies.
4.1.6 Importance of side-effects.
Side effects could be considered within a system boundary of an LCA, but frequently they are not. Most CDR technologies (except DACCS) cause a variety of side effects. DACCS results in comparatively minor side effects due to its flexibility of when, how and where it can be implemented.194 Further, DACCS systems (potentially) cause less harmful environmental side-effects compared to other CDR technologies, since DACCS systems generally avoid (i)LUC, food competition and ecosystem implications (e.g. soil quality change), although they exhibit high energy consumption178,195 and could result in water issues in water scarce areas.192 On the contrary, all other CDR technologies can exhibit substantial side effects including (i)LUC, food and water competition,192,193 biodiversity and albedo change as well as ecosystem disturbances.49 A full understanding of these – often depending on regional, local, or even site-specific boundary conditions – is often missing, especially for EW and OF.13,21
4.1.7 Main uncertainties.
We consider temporal disturbances and variable local conditions as the most uncertain factors in LCA of AR (these factors also apply to biochar and BECCS). Temporal disturbances of forests can greatly influence the LCA results and could determine whether an AR project removes or emits GHGs to the atmosphere.40,51,196 For biochar, we identify biochar stability in soil,57,73,85,197 the performance of the biomass conversion (e.g. pyrolysis) process32,198 and local conditions79,98 as the main uncertainties. Main uncertainties of SCS are associated with the rate of SCS and the possible reversibility due to soil dynamics.112,199 Further, there is no agreement on how to account for soil carbon changes – hence SCS – in LCA.26 Uncertainty for OF is mainly related to the possible reversibility of carbon storage and the lack of real experiments assessed over long periods of time.21 Uncertainties regarding EW are also due to limited availability of experimental results, weathering rates (i.e. sequestration potential)154,157 as well as energy consumption requirements for rock grinding.159 Uncertainties of BECCS systems are mainly related to biomass availability, CO2 storage capacity and sites, and the competition between food, land and water.200 Uncertainties in DACCS LCA systems mainly concern the availability of (geological) storage sites12 and the energy consumption during CO2 capture.175
4.2 Open issues and limitations
4.2.1 Sustainability.
Our work focused on the environmental performance of CDR technologies. However, environmental performance is only one of the pillars of sustainability, besides economic and social aspects.201,202 Previous reviews identified economic and societal aspects of CDR technologies (e.g.10,12–14,203,204). Besides life-cycle environmental performance, the LCA community could contribute to the assessment of the economic and social aspects of CDR technologies by conducting life-cycle cost assessments205 and social LCAs, respectively.206–208 This could result in a better understanding of the overall sustainability of CDR technologies.
4.2.2 LCA as one of the assessment tools.
We emphasize that LCA is only one of the tools to assess CDR technologies on their (environmental) performance.209,210 Alternative assessment tools should be used in a complementary way, such as risk assessment and material flow analysis.210
4.2.3 Selection of LCA CDR technology studies.
We selected our LCA CDR technology studies based on different search queries in Web of Science, Scopus and Google Scholar. However, the studied research field is still improving in quality and is growing fast in quantity. Therefore, our review might have overlooked very recent studies. However, we believe that our sample of LCA studies reflects the current state of CDR technology LCAs in a representative and comprehensive way.
4.2.4 Comparability between LCA studies.
Our review revealed that available LCA results for most CDR technologies must not be compared without further harmonization, since consistency in terms of system boundaries, applied models, key assumptions, used background data and modeling choices is generally missing. For example, some studies extended their system boundaries to include side effects, while others neglected those. In addition, a lack of transparency impedes comparison of LCA CDR technology studies.
4.2.5 Waste streams as biomass feedstock.
Many biomass based studies (e.g. on BECCS and biochar) use waste streams and assume that the waste streams come entirely (or almost) burden-free within their LCI. However, the question arises how much future biomass waste can be effectively used as biomass feedstock for CDR technologies, when the competition for alternative uses increases.211 Theoretically, a reduction of waste streams can be expected due to the evolution towards a more circular economy in the future.177 Hence, we recommend to explore alternative biomass feedstock options.
4.2.6 Alternative system layouts and pathways.
CDR technology systems are characterized by their variety of system layouts and value chains. For example, BECCS systems include the use of novel feedstock like algae,172 different power plant configurations and blending ratios of biomass and fossil energy carriers167 as well as the production of hydrogen from biomethane with CCS.169 This large variety needs to be thoroughly addressed in further LCA.
4.2.7 Ethics.
Recent works estimated significant GHG removal potentials for CDR technologies, although with large uncertainties regarding their economic and environmental performance.13,29 Therefore, the potential of CDR technologies could be easily overestimated and policy makers could rely on this future potential and subsequently postpone climate mitigation actions.12,212 Such ethical issues will not be captured by LCA, but high-qualitative and transparent LCA studies could provide an unbiased basis for the debate about CDR technologies in society.
4.3 Recommendations and future research
4.3.1 Transparency.
Most CDR technology LCAs did not include their life cycle inventory and we consider this as mostly inacceptable. Such procedure can only be justified in case of proprietary technologies, such as DAC. Apart from such exceptions, LCAs without (disclosed) complete life cycle inventories should actually be rejected during the review process, since the results cannot be interpreted and compared in a meaningful way without complete and disclosed datasets as well as modeling assumptions. Such transparency is essential for harmonization of CDR-related LCA results.
4.3.2 System boundaries: the inclusion of side-effects.
Many CDR technology LCAs were based on a cradle-to-gate analysis, which means that the end-of-life phase is not included. However, the end-of-life phase can result in significant environmental impacts,15 hence we recommend a cradle-to-grave perspective. Further, we identified the inclusion of side effects and site-specific assumptions as being crucial for LCA results. We found that side effects, which are often case-specific, have hardly been quantified and should be considered in a proper LCA. However, we acknowledge that LCA might not be the best-suited method to address side-effects, which are currently not well understood and that a combination of LCA with other methods, such as risk assessment, might be required.
4.3.3 Temporal aspects.
Temporal aspects – i.e. timing of extraction of CO2 from the atmosphere and GHG emissions – can be important for biomass-related CDR technologies and their performance in terms of ‘net-negativity’ and should thus be investigated (see Section 3.1.3). Therefore, we recommend to consider temporal aspects in biomass related projects (e.g. with dynamic LCA53), and to be transparent about the methodology used.
4.3.4 Functional unit.
LCA studies on CDR technologies should use an appropriate functional unit, which considers the primary goal of a CDR technology (i.e. GHG removal from the atmosphere). An appropriate functional unit is one that can be used to compare different CDR technologies,23e.g. environmental impacts per tonne of CO2-eq. removed. Such a functional unit allows for a reliable comparison and the identification of CDR technologies with the best overall environmental performance. Based on this functional unit, environmental burdens specifically relevant for certain CDR options can be quantified, for example the quantity of GHG removed from the atmosphere per unit of land use in case of BECCS. Such non-GHG related burdens can, if consistently quantified, also be used for comparison of different CDR options.
4.3.5 Environmental impact categories: comprehensive approach.
A comprehensive analysis based on a variety of environmental impact categories was usually missing, most CDR technology LCAs focused on climate change impacts. However, only the quantification of a wide set of environmental impact categories allows for an identification of potential (socio)-environmental co-benefits and trade-offs. Further, we recommend to assess CDR technologies on other potentially important and emerging environmental indicators, such as soil quality,116 biodiversity,116 water consumption192 and metal scarcity/criticality.213
4.3.6 Soil carbon sequestration: general LCA approach.
We recommend to introduce a general approach to include soil carbon changes in LCA,26 for example by introducing a new environmental impact category or integrating soil carbon changes in other impact categories, in particular GWP. A general LCA approach for soil carbon changes could overcome the overwhelming number of current approaches. This general approach should be easily applicable for most LCA studies to account for soil carbon changes, including SCS. The inclusion of soil carbon changes and/or soil quality could have a significant impact on GWP and other environmental impact categories.26,116,139 A soil quality indicator could consider effects on land degradation, biodiversity and pesticide issues.116 In the short term, without a generally accepted approach in place, we recommend to deal with large uncertainties associated with carbon sequestration rates and the permanence of soil carbon storage by performing sensitivity analysis and by evaluating different scenarios (e.g. as in Antonini et al.169).
4.3.7 Accounting for negative emissions.
System expansion was frequently used to avoid allocation for multi-output processes. System expansion usually resulted in avoided emissions, which were accounted for as negative emissions, hence could be easily misunderstood. An example of system expansion with its implications and recommendations is given in the next paragraphs. We use a biochar system as an example, since biochar systems usually include multiple product outputs.
An example of such a biochar system with system expansion is visualized in Fig. 4. Note that this figure represents a simplified scheme and focuses on CO2-eq. emissions only; GWP values are indicative. Fig. 3 identifies the possible impact of system expansion on the GWP results of a biochar LCA. This specific biochar system includes environmental impacts derived from fertilizer production (used to produce feedstock), feedstock production, feedstock preparation, slow pyrolysis, biochar soil amendment, methane production (from syngas) and electricity production (from bio-oil). Besides that, we included transportation processes within our system boundaries indicated with a ‘T’. Potential side effects (e.g. iLUC and albedo changes) are excluded.
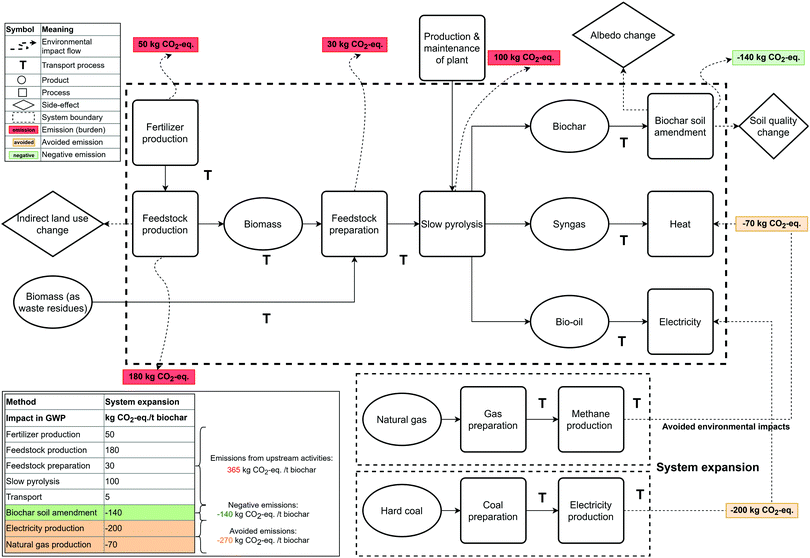 |
| Fig. 4 Example of a product system in an LCA for a biochar system, indicating the difference between ‘avoided’ emissions due to system expansion/substitution and ‘negative emissions’ due to the permanent removal of CO2 from the atmosphere. Note that for simplification, side effects such as albedo changes and iLUC are not included in the quantification of GWP results in this scheme, but should be accounted for in LCA. GWP values are indicative. | |
A reference system is proposed for the application of system expansion to avoid allocation associated with by-products (i.e. syngas and bio-oil), where we assume that electricity is generated from hard coal. The electricity supply chain includes the coal processing, transport and electricity production by coal. A similar reference system is visualized for the production of natural gas to be used for heating. When applying system expansion, environmental credits are given for avoided processes, which are ‘heat production from methane’ and ‘electricity production from coal’ in this specific case. As mentioned earlier, avoided processes are most often assigned with ‘negative’ GHG emissions in LCA literature – this is, however, misleading in the context of CDR technologies.
We indicate carbon emissions with a red color, negative GHG emissions in green and avoided GHG emissions in orange. Further, note that ‘biochar soil amendment’ results in permanent carbon storage, hence the carbon flow of this process is indicated in green.
The GWP results in Fig. 4 – according to common procedure (adding ‘avoided’ and ‘negative’ emissions) – would result in net negative GHG emissions for the biochar system (i.e. a total of −45 kg CO2-eq. t−1 biochar). However, the question arises, if these results are meaningful for the quantification of negative GHG emissions according to the CDR technology definition. We argue they are not, since avoided GHG emissions do not represent permanent removal of GHGs from the atmosphere and therefore must not be added to truly negative GHG emissions in the sense of ‘permanently removed from the atmosphere’. LCA studies must distinguish between avoided and negative emissions. In addition, the specification of the reference system is always arbitrary: in our example, electricity is produced using hard coal. If this was produced by renewables or by natural gas, it could substantially reduce the credited CO2 emissions.214
Alternatively, LCA practitioners can use an attributional approach and can allocate environmental impacts of multi-output processes, e.g. based on mass, physical characteristics or monetary value. The same biochar system is presented in Fig. 4, using mass allocation. In this specific case, 35% of the total environmental burdens of upstream activities are allocated to the biochar product.55 Note that this would not be the best allocation method per se and that the type of allocation is a subjective choice. The overall GWP results in Fig. 5, however, show real negative GHG emissions, instead of the non-meaningful ‘negative emissions’ shown in Fig. 4.
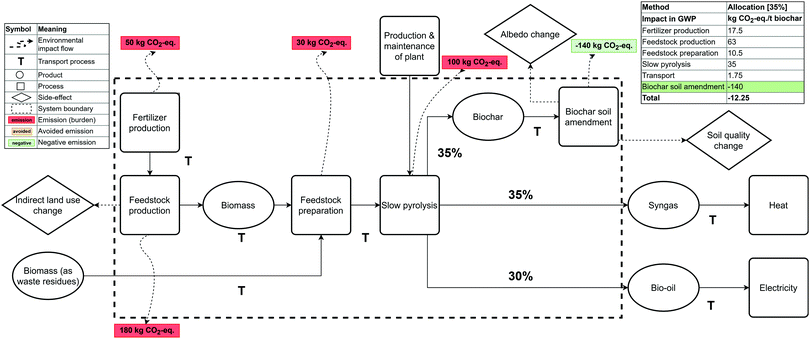 |
| Fig. 5 Example of an attributional LCA for a biochar system, applying mass allocation and quantifying real negative emissions. Note that for simplification, side effects such as albedo changes and iLUC are not included in the quantification of GWP results in this scheme, but should be accounted for in LCA. GWP values are indicative. | |
In both cases, LCA results can be quantified for our recommended functional unit – burdens per unit of CO2-eq. removed, corresponding to the real negative emissions and not to the sum of negative and avoided GHG emissions.
The implications on the LCA results – in this case only GHG emissions – of either system expansion or allocation are significant. Using system expansion led to a GHG removal of 140 kg CO2-eq., 200 kg CO2-eq. avoided GHG emissions and total upstream GHG emissions of 365 kg CO2-eq. per ton of biochar application, while using allocation resulted in total GHG emissions of −12.25 kg CO2-equivalent per ton of biochar application. As mentioned, both approaches have advantages and disadvantages and the accuracy and viability of both approaches are largely influenced by decisions of an LCA practitioner, such as the chosen substitution products (system expansion) and the type of allocation. Consequently, we argue to perform a sensitivity analysis to analyze both approaches as well as to demonstrate the most sensitive parameters driving the LCA results.
To conclude, LCA of CDR technologies must avoid ambiguity in the interpretation of ‘negative emissions’. When system expansion is chosen and the goal of an LCA is to show the environmental performance and carbon removal of CDR technologies, we argue to make a distinction between real negative emissions and avoided emissions in the LCA results. In addition, we recommend to apply both system expansion and allocation in CDR technology LCAs, in which multi-functionality plays an important role, and to compare the results obtained from the different approaches.
4.3.8 General guidelines and standardization.
General LCA modelling guidelines for each CDR technology option could increase comparability and the overall quality of CDR technology LCA studies. Due to the complexity of CDR technologies, we argue to establish such guidelines.
Fig. 6 demonstrates our recommendations which should be considered when conducting an LCA on CDR technologies. Note that we avoid the repetition of general guidelines presented in LCA handbooks. The general guidelines should always be followed, hence our recommendations should function on top of the general guidelines of the ISO.
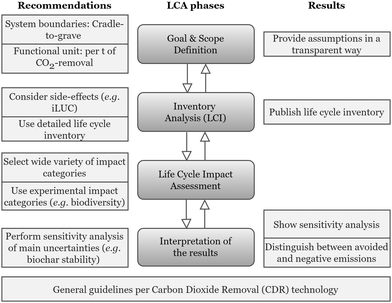 |
| Fig. 6 Additional guidelines for LCAs on CDR technologies which should be considered on top of the general guidelines of the ISO. | |
Our three most important recommendations for CDR LCAs are: (1) to distinguish between avoided and negative emissions, (2) to consider side-effects within the system boundaries of an LCA and (3) to quantify LCA results per ton of CO2-removal as functional unit in a transparent way. First, CDR potentials can only be quantified with a clear distinction between negative and avoided GHG-emissions – without such a distinction, any CDR evaluation misses its purpose. Second, the inclusion of side-effects within the system boundaries of an LCA showed to be extremely important. In fact, CDR schemes have a complex nature due to many inter-dependencies (e.g. the water, energy and food security nexus) and thus could result in severe side-effects. Some of these side-effects can be quantified with traditional life cycle impact categories and therefore we recommended to select a wide set of environmental impact categories as well. Third, the application of a common functional unit (per ton of CO2-removal) – together with transparency in terms of modeling choices and LCI data – enables a meaningful comparison between different CDR technologies and LCA studies.
5 Conclusions
Previous research and prospective energy system models assigned significant GHG removal potentials to CDR technologies, though with a limited understanding of overall environmental implications. This study aimed to determine the current state of knowledge regarding LCAs on CDR technologies. Associated methodological choices were critically reviewed from an LCA point of view. We demonstrated the immature and complex nature of LCA studies on CDR technologies, manifested by both the ambiguous quality and quantity of CDR technology LCA studies. We identified several shortcomings in the reviewed literature: especially LCAs on biochar and SCS often deal with avoided and negative (in the sense of removal from the atmosphere) emissions in an additive way, which does not result in meaningful CDR technology impacts on climate change. Further, the application of multiple environmental impact categories is limited and side effects are usually not included, which results in additional uncertainty on the overall environmental impacts. We also found substantial lack of transparency, both on modeling choices and inventory data, which hinders harmonization of LCAs and reduces usefulness of available studies in decision contexts. Based on the available body of CDR LCA literature, we conclude that the available LCA results are – for most CDR options – not representative nor reliable and should not be used in a decision making context without a very careful interpretation.
The identified shortcomings must be eliminated to fully understand the environmental benefits and potential trade-offs associated with CDR technologies before any large-scale implementation. To achieve this, more research is needed before relying on CDR technologies. Our recommendations for best-practice can serve as a guideline for future LCA studies on CDR technologies evaluated with LCA. Given all the present uncertainties, we consider strongly relying on CDR technologies to achieve climate goals as inappropriate. Hence, we see no reason to postpone climate mitigation measures.
Conflicts of interest
There are no conflicts of interest to declare.
Acknowledgements
This work has partially received financial support from the Kopernikus Project Ariadne (FKZ 03SFK5A), funded by the German Federal Ministry of Education and Research.
References
- P. Friedlingstein, M. O'Sullivan, M. W. Jones, R. M. Andrew, J. Hauck, A. Olsen, G. P. Peters, W. Peters, J. Pongratz and S. Sitch,
et al.
, Earth System Science Data, 2020, 12, 3269–3340 CrossRef.
- COP, Paris Climate Change Conference – November 2015, COP 21, 2015, p. 32.
- H.-K. Ringkjøb, P. M. Haugan and I. M. Solbrekke, Renewable Sustainable Energy Rev., 2018, 96, 440–459 CrossRef.
- S. Pauliuk, A. Arvesen, K. Stadler and E. G. Hertwich, Nat. Climate Change, 2017, 7, 13–20 CrossRef.
- J. Weyant, Rev. Environ. Economics Policy, 2017, 11, 115–137 CrossRef.
- D. van Vuuren, S. Deetman, J. van Vliet, M. van den Berg, B. van Ruijven and B. Koelbl, Clim. Change, 2013, 118, 15–27 CrossRef.
- K. Riahi, D. P. van Vuuren, E. Kriegler, J. Edmonds, B. C. O'Neill, S. Fujimori, N. Bauer, K. Calvin, R. Dellink, O. Fricko, W. Lutz, A. Popp, J. C. Cuaresma, S. K. C., M. Leimbach, L. Jiang, T. Kram, S. Rao, J. Emmerling, K. Ebi, T. Hasegawa, P. Havlik, F. Humpenöder, L. A. Da Silva, S. Smith, E. Stehfest, V. Bosetti, J. Eom, D. Gernaat, T. Masui, J. Rogelj, J. Strefler, L. Drouet, V. Krey, G. Luderer, M. Harmsen, K. Takahashi, L. Baumstark, J. C. Doelman, M. Kainuma, Z. Klimont, G. Marangoni, H. Lotze-Campen, M. Obersteiner, A. Tabeau and M. Tavoni, Global Environ. Change, 2017, 42, 153–168 CrossRef.
- J. Rogelj, D. Shindell, K. Jiang, S. Fifita, P. Forster, V. Ginzburg, C. Handa, H. Kheshgi, S. Kobayashi, E. Kriegler, L. Mundaca, R. Séférian and M. V. Vilariño, Global Warming of 1.5 °C. An IPCC Special Report on the impacts of global warming of 1.5 °C above pre-industrial levels and related global greenhouse gas emission pathways, in the context of strengthening the global response to the threat of climate change, 2018, 1–1308 Search PubMed.
- A. J. Sykes, M. Macleod, V. Eory, R. M. Rees, F. Payen, V. Myrgiotis, M. Williams, S. Sohi, J. Hillier, D. Moran, D. A. Manning, P. Goglio, M. Seghetta, A. Williams, J. Harris, M. Dondini, J. Walton, J. House and P. Smith, Global Change Biol., 2020, 26, 1085–1108 CrossRef.
- P. Smith, S. J. Davis, F. Creutzig, S. Fuss, J. Minx, B. Gabrielle, E. Kato, R. B. Jackson, A. Cowie, E. Kriegler, D. P. Van Vuuren, J. Rogelj, P. Ciais, J. Milne, J. G. Canadell, D. McCollum, G. Peters, R. Andrew, V. Krey, G. Shrestha, P. Friedlingstein, T. Gasser, A. Grübler, W. K. Heidug, M. Jonas, C. D. Jones, F. Kraxner, E. Littleton, J. Lowe, J. R. Moreira, N. Nakicenovic, M. Obersteiner, A. Patwardhan, M. Rogner, E. Rubin, A. Sharifi, A. Torvanger, Y. Yamagata, J. Edmonds and C. Yongsung, Nat. Climate Change, 2016, 6, 42–50 CrossRef CAS.
- T. Gasser, C. Guivarch, K. Tachiiri, C. D. Jones and P. Ciais, Nat. Commun., 2015, 6, 7958 CrossRef CAS.
- J. C. Minx, W. F. Lamb, M. W. Callaghan, S. Fuss, J. Hilaire, F. Creutzig, T. Amann, T. Beringer, W. De Oliveira Garcia, J. Hartmann, T. Khanna, D. Lenzi, G. Luderer, G. F. Nemet, J. Rogelj, P. Smith, J. L. Vicente Vicente, J. Wilcox and M. Del Mar Zamora Dominguez, Environ. Res. Lett., 2018, 13, 063001 CrossRef.
- S. Fuss, W. F. Lamb, M. W. Callaghan, J. Hilaire, F. Creutzig, T. Amann, T. Beringer, W. De Oliveira Garcia, J. Hartmann, T. Khanna, G. Luderer, G. F. Nemet, J. Rogelj, P. Smith, J. V. Vicente, J. Wilcox, M. Del Mar Zamora Dominguez and J. C. Minx, Environ. Res. Lett., 2018, 13, 063002 CrossRef.
- G. F. Nemet, M. W. Callaghan, F. Creutzig, S. Fuss, J. Hartmann, J. Hilaire, W. F. Lamb, J. C. Minx, S. Rogers and P. Smith, Environ. Res. Lett., 2018, 13, 063003 CrossRef.
- S. E. Tanzer and A. Ramírez, Energy Environ. Sci., 2019, 12, 1210–1218 RSC.
- J. Lehmann, Nature, 2007, 143–144 CrossRef CAS.
- D. L. Sanchez, N. Johnson, S. T. McCoy, P. A. Turner and K. J. Mach, Proc. Natl. Acad. Sci. U. S. A., 2018, 115, 4875–4880 CrossRef CAS.
- G. Realmonte, L. Drouet, A. Gambhir, J. Glynn, A. Hawkes, A. C. Köberle and M. Tavoni, Nat. Commun., 2019, 10, 3277 CrossRef CAS.
- A. Marcucci, S. Kypreos and E. Panos, Clim. Change, 2017, 144, 181–193 CrossRef.
- J. Hartmann, A. J. West, P. Renforth, P. Köhler, C. L. De La Rocha, D. A. Wolf-Gladrow, H. H. Dürr and J. Scheffran, Rev. Geophys., 2013, 51, 113–149 CrossRef.
- P. Williamson, D. W. Wallace, C. S. Law, P. W. Boyd, Y. Collos, P. Croot, K. Denman, U. Riebesell, S. Takeda and C. Vivian, Process Saf. Environ. Prot., 2012, 90, 475–488 CrossRef CAS.
- K. Paustian, E. Larson, J. Kent, E. Marx and A. Swan, Fron. Climate, 2019, 1, 8 CrossRef.
- P. Goglio, A. G. Williams, N. Balta-Ozkan, N. R. Harris, P. Williamson, D. Huisingh, Z. Zhang and M. Tavoni, J. Cleaner Prod., 2020, 244, 118896 CrossRef.
-
E. Loiseau, G. Junqua, P. Roux and V. Bellon-Maurel, Environmental assessment of a territory: An overview of existing tools and methods, 2012 Search PubMed.
- S. Hellweg and L. Milà i Canals, Science, 2014, 344, 1109–1113 CrossRef CAS.
- P. Goglio, W. N. Smith, B. B. Grant, R. L. Desjardins, B. G. McConkey, C. A. Campbell and T. Nemecek, J. Cleaner Prod., 2015, 104, 23–39 CrossRef CAS.
- C. van der Giesen, S. Cucurachi, J. Guinée, G. J. Kramer and A. Tukker, J. Cleaner Prod., 2020, 259, 120904 CrossRef.
- S. Fuss, J. Canadell, G. Peters, M. Tavoni, R. Andrew, P. Ciais, R. Jackson, C. Jones, F. Kraxner, N. Nakicenovic, C. Le Quéré, M. Raupach, A. Sharifi, P. Smith and Y. Yamagata, Nat. Climate Change, 2014, 4, 850–853 CrossRef CAS.
- H. Lawford-Smith and A. Currie, Biol. Lett., 2017, 13, 28381632 Search PubMed.
-
National Academies of Sciences, Engineering, and Medicine, Negative Emissions Technologies and Reliable Sequestration: A Research Agenda, Washington, DC, The National Academies Press, 2019 Search PubMed.
- W. Li and M. M. Wright, Energy Technol., 2019, 1900871 Search PubMed.
- J. Matuštík, T. Hnátková and V. Kočí, J. Cleaner Prod., 2020, 259, 120998 CrossRef.
- J. B. Guinée, R. Heijungs, G. Huppes, A. Zamagni, P. Masoni, R. Buonamici, T. Ekvall and T. Rydberg, Environ. Sci. Technol., 2011, 45, 90–96 CrossRef.
-
ISO, ISO 14040: Life Cycle Assessment, Principles and Framework, 2006 Search PubMed.
-
ISO, ISO 14044: Life cycle assessment – Requirements and guidelines, 2006 Search PubMed.
-
ISO, ISO 14067:2013 Greenhouse gases – Carbon footprint of products - Requirements and guidelines for quantification and communication, 2013 Search PubMed.
- Web of Science, Web of Science (online), 2020.
-
D. Baldocchi and J. Penuelas, Global Change Biol., 2019.
- D. Klein, C. Wolf, C. Schulz and G. Weber-Blaschke, Int. J. Life Cycle Assess., 2015, 20, 556–575 CrossRef CAS.
- S. Gaboury, J. F. Boucher, C. Villeneuve, D. Lord and R. Gagnon, For. Ecol. Manage., 2009, 257, 483–494 CrossRef.
- J. F. García-Quijano, J. Peters, L. Cockx, G. Van Wyk, A. Rosanov, G. Deckmyn, R. Ceulemans, S. M. Ward, N. M. Holden, J. Van Orshoven and B. Muys, Clim. Change, 2007, 83, 323–355 CrossRef.
- F. Lun, Y. Liu, L. He, L. Yang, M. Liu and W. Li, J. Cleaner Prod., 2018, 177, 178–186 CrossRef.
- A. M. E. Brunori, P. Sdringola, F. Dini, L. Ilarioni, L. Nasini, L. Regni, P. Proietti, S. Proietti, A. Vitone and F. Pelleri, J. Cleaner Prod., 2017, 144, 69–78 CrossRef.
- M. Brandão, A. Levasseur, M. U. Kirschbaum, B. P. Weidema, A. L. Cowie, S. V. Jørgensen, M. Z. Hauschild, D. W. Pennington and K. Chomkhamsri, Int. J. Life Cycle Assess., 2013, 18, 230–240 CrossRef.
- A. Albers, P. Collet, A. Benoist and A. Hélias, Int. J. Life Cycle Assess., 2019, 1242–1258 Search PubMed.
- L. Wang and P. D'Odorico, Proc. Natl. Acad. Sci. U. S. A., 2019, 116, 24925–24926 CrossRef CAS.
- G. Ceccherini, G. Duveiller, G. Grassi, G. Lemoine, V. Avitabile, R. Pilli and A. Cescatti, Nature, 2020, 583, 72–77 CrossRef CAS.
- K. Zhu, J. Zhang, S. Niu, C. Chu and Y. Luo, Nat. Commun., 2018, 9, 1–8 CrossRef.
- B. Wicke, P. Verweij, H. Van Meijl, D. P. Van Vuuren and A. P. Faaij, Biofuels, 2012, 1, 87–100 CrossRef.
- J. Fargione, J. Hill, D. Tilman, S. Polasky and P. Hawthorne, Science, 2008, 319, 1235–1238 CrossRef CAS.
- A. Levasseur, P. Lesage, M. Margni, M. Brandão and R. Samson, Clim. Change, 2012, 115, 759–776 CrossRef CAS.
- A. Levasseur, P. Lesage, M. Margni and R. Samson, J. Ind. Ecol., 2013, 17, 117–128 CrossRef CAS.
- A. Levasseur, P. Lesage, M. Margni, L. Deschênes and R. Samson, Environ. Sci. Technol., 2010, 44, 3169–3174 CrossRef CAS.
- D. Wang, P. Jiang, H. Zhang and W. Yuan, Sci. Total Environ., 2020, 137775 CrossRef CAS.
-
S. Sohi, E. Krull, E. Lopez-Capel and R. Bol, Adv. Agronomy, Academic Press, 2010, vol. 105, pp. 47–82 Search PubMed.
- J. Lehmann, M. C. Rillig, J. Thies, C. A. Masiello, W. C. Hockaday and D. Crowley, Soil Biol. Biochem., 2011, 43, 1812–1836 CrossRef CAS.
- J. Hammond, S. Shackley, S. Sohi and P. Brownsort, Energy Policy, 2011, 39, 2646–2655 CrossRef CAS.
- D. Barry, C. Barbiero, C. Briens and F. Berruti, Biomass Bioenergy, 2019, 122, 472–480 CrossRef CAS.
- Y. Cao and A. Pawłowski, Bioresour. Technol., 2013, 127, 81–91 CrossRef CAS.
- R. Ibarrola, S. Shackley and J. Hammond, Waste Manage., 2012, 32, 859–868 CrossRef CAS.
- Y.-F. Huang, F.-S. Syu, P.-T. Chiueh and S.-L. Lo, Bioresour. Technol., 2013, 131, 166–171 CrossRef CAS.
- S. K. Thengane, J. Burek, K. S. Kung, A. F. Ghoniem and D. L. Sanchez, J. Cleaner Prod., 2020, 275, 123177 CrossRef CAS.
- M. Ahmad, A. U. Rajapaksha, J. E. Lim, M. Zhang, N. Bolan, D. Mohan, M. Vithanage, S. S. Lee and Y. S. Ok, Chemosphere, 2014, 99, 19–33 CrossRef CAS.
- M. Waqas, A. Aburiazaiza, R. Miandad, M. Rehan, M. Barakat and A. Nizami, J. Cleaner Prod., 2018, 188, 477–488 CrossRef CAS.
- X. Xiong, I. K. Yu, L. Cao, D. C. Tsang, S. Zhang and Y. S. Ok, Bioresour. Technol., 2017, 246, 254–270 CrossRef CAS.
- M. Zhang and Y. S. Ok, Carbon Manage., 2014, 5, 255–257 CrossRef CAS.
- M. Patel, X. Zhang and A. Kumar, Renewable Sustainable Energy Rev., 2016, 53, 1486–1499 CrossRef CAS.
-
J. Lehmann, Biochar for Environmental Management, Routledge, 2012 Search PubMed.
- J. Wang, Z. Xiong and Y. Kuzyakov, GCB Bioenergy, 2015, 8, 512–523 CrossRef.
- P. Smith, Global Change Biol., 2016, 22, 1315–1324 CrossRef.
- J. S. Cha, S. H. Park, S.-C. Jung, C. Ryu, J.-K. Jeon, M.-C. Shin and Y.-K. Park, J. Ind. Eng. Chem., 2016, 40, 1–15 CrossRef CAS.
- K. G. Roberts, B. A. Gloy, S. Joseph, N. R. Scott and J. Lehmann, Environ. Sci. Technol., 2010, 44, 827–833 CrossRef CAS.
- J. F. Peters, D. Iribarren and J. Dufour, Environ. Sci. Technol., 2015, 49, 5195–5202 CrossRef CAS.
- M. Sparrevik, J. L. Field, V. Martinsen, G. D. Breedveld and G. Cornelissen, Environ. Sci. Technol., 2013, 101, 35–43 Search PubMed.
- S. R. Hamedani, T. Kuppens, R. Malina, E. Bocci, A. Colantoni and M. Villarini, Energies, 2019, 12, 2166 CrossRef CAS.
- A. B. Smebye, M. Sparrevik, H. P. Schmidt and G. Cornelissen, Biomass Bioenergy, 2017, 101, 35–43 CrossRef CAS.
- L. Fryda, R. Visser and J. Schmidt, Detritus, 2019, 5, 132–149 Search PubMed.
- K. Homagain, C. Shahi, N. Luckai and M. Sharma, J. For. Res., 2015, 26, 799–809 CrossRef CAS.
- T. L. Oldfield, N. Sikirica, C. Mondini, G. Lopez, P. J. Kuikman and N. M. Holden, J. Environ. Manage., 2018, 218, 465–476 CrossRef CAS.
- S. Grierson, V. Strezov and J. Bengtsson, Algal Res., 2013, 2, 299–311 CrossRef.
- H. Thers, S. N. Djomo, L. Elsgaard and M. T. Knudsen, Sci. Total Environ., 2019, 671, 180–188 CrossRef CAS.
- E. Ahmadi Moghaddam, N. Ericsson, P. A. Hansson and Å. Nordberg, Energy Sustainability Soc., 2019, 9, 6 CrossRef.
- A. Mohammadi, M. Sandberg, G. Venkatesh, S. Eskandari, T. Dalgaard, S. Joseph and K. Granström, J. Ind. Ecol., 2019, 23, 1039–1051 CrossRef CAS.
- P. Galgani, E. van der Voet and G. Korevaar, Waste Manage., 2014, 34, 2454–2465 CrossRef.
- A. Mohammadi, A. Cowie, T. L. Anh Mai, R. A. de la Rosa, P. Kristiansen, M. Brandão and S. Joseph, J. Cleaner Prod., 2016, 116, 61–70 CrossRef.
- M. Sparrevik, H. Lindhjem, V. Andria, A. M. Fet and G. Cornelissen, Environ. Sci. Technol., 2014, 48, 4664–4671 CrossRef CAS.
- J. L. Field, C. M. H. Keske, G. L. Birch, M. W. DeFoort and M. F. Cotrufo, GCB Bioenergy, 2013, 5, 177–191 CrossRef.
- V. Uusitalo and M. Leino, J. Cleaner Prod., 2019, 227, 48–57 CrossRef CAS.
- A. Mohammadi, A. L. Cowie, T. L. Anh Mai, M. Brandão, R. Anaya de la Rosa, P. Kristiansen and S. Joseph, J. Cleaner Prod., 2017, 162, 260–272 CrossRef.
- P. Bartocci, G. Bidini, P. Saputo and F. Fantozzi, Chem. Eng. Trans., 2016, 50, 217–222 Search PubMed.
- P. Brassard, S. Godbout, F. Pelletier, V. Raghavan and J. H. Palacios, Biomass Bioenergy, 2018, 116, 99–105 CrossRef CAS.
- L. Miller-Robbie, B. A. Ulrich, D. F. Ramey, K. S. Spencer, S. P. Herzog, T. Y. Cath, J. R. Stokes and C. P. Higgins, J. Cleaner Prod., 2015, 91, 118–127 CrossRef CAS.
- P. Llorach-Massana, E. Lopez-Capel, J. P. na, J. Rieradevall, J. I. Montero and N. Puy, Waste Manage., 2017, 67, 121–130 CrossRef CAS.
- B. Dutta and V. Raghavan, Int. J. Renewable Energy Environ. Eng., 2014, 5, 106 CrossRef.
- E. Muñoz, G. Curaqueo, M. Cea, L. Vera and R. Navia, J. Cleaner Prod., 2017, 158, 1–7 CrossRef.
- A. Clare, S. Shackley, S. Joseph, J. Hammond, G. Pan and A. Bloom, GCB Bioenergy, 2015, 7, 1272–1282 CrossRef CAS.
- X. Xu, K. Cheng, H. Wu, J. Sun, Q. Yue and G. Pan, GCB Bioenergy, 2019, 11, 592–605 CrossRef CAS.
- M. Owsianiak, G. Cornelissen, S. E. Hale, H. Lindhjem and M. Sparrevik, J. Cleaner Prod., 2018, 200, 259–268 CrossRef.
- S. Robb and P. Dargusch, Carbon Manage., 2018, 9, 105–114 CrossRef CAS.
- A. Mohammadi, A. Cowie, T. L. A. Mai, R. A. de la Rosa, M. Brandão, P. Kristiansen and S. Joseph, Energy Procedia, 2016, 97, 254–261 CrossRef CAS.
- T. Mattila, J. Grönroos, J. Judl and M. R. Korhonen, Process Saf. Environ. Prot., 2012, 90, 452–458 CrossRef CAS.
- H. Wu, M. A. Hanna and D. D. Jones, Biomass Bioenergy, 2013, 54, 260–266 CrossRef CAS.
- E. S. Azzi, E. Karltun and C. Sundberg, Environ. Sci. Technol., 2019, 53, 8466–8476 CrossRef CAS.
- T. Searchinger, R. Heimlich, R. A. Houghton, F. Dong, A. Elobeid, J. Fabiosa, S. Tokgoz, D. Hayes and T. H. Yu, Science, 2008, 319, 1238–1240 CrossRef CAS.
- R. M. Bright, Environ. Sci. Technol., 2015, 49, 3291–3303 CrossRef CAS.
- P. Sieber, N. Ericsson and P.-A. Hansson, Environ. Impact Assess. Rev., 2019, 77, 191–200 CrossRef.
- S. Meyer, R. M. Bright, D. Fischer, H. Schulz and B. Glaser, Environ. Sci. Technol., 2012, 46, 12726–12734 CrossRef CAS.
- B. P. Weidema, M. Pizzol, J. Schmidt and G. Thoma, J. Cleaner Prod., 2018, 174, 305–314 CrossRef.
- T. Ekvall, A. Azapagic, G. Finnveden, T. Rydberg, B. P. Weidema and A. Zamagni, Int. J. Life Cycle Assess., 2016, 21, 293–296 CrossRef.
- A. Zamagni, J. Guinée, R. Heijungs, P. Masoni and A. Raggi, Int. J. Life Cycle Assess., 2012, 17, 904–918 CrossRef.
- R. J. Plevin, M. A. Delucchi and F. Creutzig, J. Ind. Ecol., 2014, 18, 73–83 CrossRef.
-
C. M. Godde, I. J. de Boer, E. Zu Ermgassen, M. Herrero, C. E. van Middelaar, A. Muller, E. Röös, C. Schader, P. Smith, H. H. van Zanten and T. Garnett, Clim. Change, 2020.
- K. Venkat, J. Sustainable Agric., 2012, 36, 620–649 CrossRef.
- J. F. Parr and L. A. Sullivan, Soil Biol. Biochem., 2005, 117–124 CrossRef CAS.
- I. Batalla, M. T. Knudsen, L. Mogensen, Ó. D. Hierro, M. Pinto and J. E. Hermansen, J. Cleaner Prod., 2015, 104, 121–129 CrossRef CAS.
- H. M. G. van der Werf, M. T. Knudsen and C. Cederberg, Nat. Sustainability, 2020, 3, 419–425 CrossRef.
- L. G. Smith, G. J. D. Kirk, P. J. Jones and A. G. Williams, Nat. Commun., 2019, 10, 1–10 CrossRef CAS.
- E. Sabia, S. Kühl, L. Flach, C. Lambertz and M. Gauly, Sustainability, 2020, 12, 2128 CrossRef CAS.
- D. O'Brien, J. Capper, P. Garnsworthy, C. Grainger and L. Shalloo, J. Dairy Sci., 2014, 97, 1835–1851 CrossRef.
- C. Du, L. Kulay, O. Cavalett, L. Dias and F. Freire, Int. J. Life Cycle Assess., 2017, 23, 787–799 CrossRef.
- S. Bosco, N. N. o Di Nasso, N. Roncucci, M. Mazzoncini and E. Bonari, Eur. J. Agron., 2016, 78, 20–31 CrossRef.
- E. Aguilera, G. Guzmán and A. Alonso, Agron. Sustainable Dev., 2014, 35, 725–737 CrossRef.
- A. Horrillo, P. Gaspar and M. Escribano, Animals, 2020, 10, 162 CrossRef.
- A. Eldesouky, F. Mesias, A. Elghannam and M. Escribano, J. Cleaner Prod., 2018, 200, 28–38 CrossRef.
- P. Tidåker, G. Bergkvist, M. Bolinder, H. Eckersten, H. Johnsson, T. Kätterer and M. Weih, Eur. J. Agron., 2016, 80, 45–54 CrossRef.
- N. Halberg, J. E. Hermansen, I. S. Kristensen, J. Eriksen, N. Tvedegaard and B. M. Petersen, Agron. Sustainable Dev., 2010, 30, 721–731 CrossRef CAS.
- M. K. Alam, R. W. Bell and W. K. Biswas, J. Cleaner Prod., 2019, 224, 72–87 CrossRef CAS.
- J. Queirós, J. Malça and F. Freire, J. Cleaner Prod., 2015, 99, 266–274 CrossRef.
- E. Matsuura, M. Komatsuzaki and R. Hashimi, Sustainability, 2018, 10, 152 CrossRef.
- D. Liang, F. Sun, M. Wattiaux, V. Cabrera, J. Hedtcke and E. Silva, J. Dairy Sci., 2017, 100, 5957–5973 CrossRef CAS.
- C. D. Scown, W. W. Nazaroff, U. Mishra, B. Strogen, A. B. Lobscheid, E. Masanet, N. J. Santero, A. Horvath and T. E. McKone, Environ. Res. Lett., 2012, 7, 014011 CrossRef.
- K. A. Jung, S.-R. Lim, Y. Kim and J. M. Park, Environ.
Prog. Sustainable Energy, 2016, 36, 200–207 CrossRef.
- A. Zucaro, A. Forte, S. Faugno, A. Impagliazzo and A. Fierro, J. Cleaner Prod., 2018, 172, 4200–4211 CrossRef CAS.
- W. Wang and R. C. Dalal, Eur. J. Agron., 2015, 66, 74–82 CrossRef CAS.
- T. T. Siqueira and M. Duru, J. Cleaner Prod., 2016, 112, 2485–2494 CrossRef.
- T. Wang, W. Richard Teague, S. C. Park and S. Bevers, Sustainability, 2015, 7, 13500–13521 CrossRef CAS.
- M. Manouchehrinejad, K. Sahoo, N. Kaliyan, H. Singh and S. Mani, Int. J. Life Cycle Assess., 2020, 25, 89–104 CrossRef CAS.
- B. Gao, T. Huang, X. Ju, B. Gu, W. Huang, L. Xu, R. M. Rees, D. S. Powlson, P. Smith and S. Cui, Global Change Biol., 2018, 24, 5590–5606 CrossRef.
- M. Brandão, L. Milà i Canals and R. Clift, Biomass Bioenergy, 2011, 35, 2323–2336 CrossRef.
- S. Kim, B. E. Dale and R. Jenkins, Int. J. Life Cycle Assess., 2009, 14, 160–174 CrossRef CAS.
- M. V. Galdos, C. C. Cerri, R. Lal, M. Bernoux, B. Feigl and C. E. P. Cerri, GCB Bioenergy, 2010, 2, 37–44 CrossRef CAS.
- I. Gelfand, R. Sahajpal, X. Zhang, R. C. Izaurralde, K. L. Gross and G. P. Robertson, Nature, 2013, 493, 514–517 CrossRef CAS.
- U. Zaher, C. Stöckle, K. Painter and S. Higgins, Agric. Syst., 2013, 122, 73–78 CrossRef.
- R. Parajuli, M. T. Knudsen, S. N. Djomo, A. Corona, M. Birkved and T. Dalgaard, Sci. Total Environ., 2017, 586, 226–240 CrossRef CAS.
- M. T. Knudsen, T. Dorca-Preda, S. N. Djomo, N. Peña, S. Padel, L. G. Smith, W. Zollitsch, S. Hörtenhuber and J. E. Hermansen, J. Cleaner Prod., 2019, 215, 433–443 CrossRef CAS.
- P. R. Adler, S. J. Del Grosso and W. J. Parton, Ecological Applications, 2007, 17, 675–691 CrossRef.
- S. Salvador, M. Corazzin, A. Romanzin and S. Bovolenta, J. Environ. Manage., 2017, 196, 644–650 CrossRef CAS.
- P. L. Stanley, J. E. Rowntree, D. K. Beede, M. S. DeLonge and M. W. Hamm, Agric. Syst., 2018, 162, 249–258 CrossRef.
- J. E. Rowntree, R. Ryals, M. S. DeLonge, W. R. Teague, M. B. Chiavegato, P. Byck, T. Wang and S. Xu, Future of Food: Journal on Food, Agriculture and Society, 2016, 4(3), 31–38 Search PubMed.
- J. H. Schmidt, B. P. Weidema and M. Brandão, J. Cleaner Prod., 2015, 99, 230–238 CrossRef.
- S. T. Sanchez, J. Woods, M. Akhurst, M. Brander, M. O'Hare, T. P. Dawson, R. Edwards, A. J. Liska and R. Malpas, J. R. Soc., Interface, 2012, 9, 1105–1119 CrossRef.
- M. Finkbeiner, Biomass Bioenergy, 2014, 62, 218–221 CrossRef.
- L. T. Bach, S. J. Gill, R. E. M. Rickaby, S. Gore and P. Renforth, Front. Climate, 2019, 1, 7 CrossRef.
- J. Strefler, T. Amann, N. Bauer, E. Kriegler and J. Hartmann, Environ. Res. Lett., 2018, 13, 034010 CrossRef.
- J. Hartmann, A. J. West, P. Renforth, P. Köhler, C. L. De La Rocha, D. A. Wolf-Gladrow, H. H. Dürr and J. Scheffran, Rev. Geophys., 2013, 51, 113–149 CrossRef.
- L. L. Taylor, J. Quirk, R. M. Thorley, P. A. Kharecha, J. Hansen, A. Ridgwell, M. R. Lomas, S. A. Banwart and D. J. Beerling, Nat. Climate Change, 2016, 138–147 Search PubMed.
- D. Lefebvre, P. Goglio, A. Williams, D. A. Manning, A. C. de Azevedo, M. Bergmann, J. Meersmans and P. Smith, J. Cleaner Prod., 2019, 233, 468–481 CrossRef CAS.
- D. J. Beerling, J. R. Leake, S. P. Long, J. D. Scholes, J. Ton, P. N. Nelson, M. Bird, E. Kantzas, L. L. Taylor, B. Sarkar, M. Kelland, E. DeLucia, I. Kantola, C. Müller, G. Rau and J. Hansen, Nat. Plants, 2018, 138–147 CrossRef.
- D. J. Beerling, E. P. Kantzas, M. R. Lomas, P. Wade, R. M. Eufrasio, P. Renforth, B. Sarkar, M. G. Andrews, R. H. James, C. R. Pearce, J. F. Mercure, H. Pollitt, P. B. Holden, N. R. Edwards, M. Khanna, L. Koh, S. Quegan, N. F. Pidgeon, I. A. Janssens, J. Hansen and S. A. Banwart, Nature, 2020, 242–248 CrossRef CAS.
- GESAMP, High level review of a wide range of proposed marine geoengineering techniques, 2019, (Boyd, P.W. and Vivian, C.M.G., eds.). (IMO/FAO/UNESCO-IOC/UNIDO/WMO/IAEA/UN/UN Environment/UNDP/ISA Joint Group of Experts on the Scientific Aspects of Marine Environmental Protection). Rep. Stud. No. 98, 144p.
- D. P. Harrison, Environ. Res. Lett., 2017, 12, 035001 CrossRef.
- J. Kemper, Int. J. Greenhouse Gas Control, 2015, 40, 401–430 CrossRef CAS.
- M. Fajardy and N. Mac Dowell, Energy Environ. Sci., 2017, 10, 1389–1426 RSC.
- N. Pour, P. A. Webley and P. J. Cook, Energy Procedia, 2017, 6044–6056 CrossRef CAS.
- N. Pour, P. A. Webley and P. J. Cook, Appl. Energy, 2018, 224, 615–635 CrossRef.
- N. Pour, P. A. Webley and P. J. Cook, Int. J. Greenhouse Gas Control, 2018, 68, 1–15 CrossRef CAS.
- C. Cumicheo, N. Mac Dowell and N. Shah, Int. J. Greenhouse Gas Control, 2019, 90, 102798 CrossRef CAS.
- B. Yang, Y.-M. Wei, Y. Hou, H. Li and P. Wang, Appl. Energy, 2019, 252, 113483 CrossRef CAS.
- C. Antonini, K. Treyer, A. Streb, M. van der Spek, C. Bauer and M. Mazzotti, Sustainable Energy Fuels, 2020, 4(6), 2967–2986 RSC.
- X. Lu, L. Cao, H. Wang, W. Peng, J. Xing, S. Wang, S. Cai, B. Shen, Q. Yang, C. P. Nielsen and M. B. McElroy, Proc. Natl. Acad. Sci. U. S. A., 2019, 116, 8206–8213 CrossRef CAS.
- Q. Yi, Y. Zhao, Y. Huang, G. Wei, Y. Hao, J. Feng, U. Mohamed, M. Pourkashanian, W. Nimmo and W. Li, Appl. Energy, 2018, 225, 258–272 CrossRef CAS.
- C. M. Beal, I. Archibald, M. E. Huntley, C. H. Greene and Z. I. Johnson, Earth's Future, 2018, 6, 524–542 CrossRef CAS.
- F. Cheng, M. D. Porter and L. M. Colosi, Energy Convers. Manage., 2020, 203, 112252 CrossRef CAS.
-
M. Fajardy, A. Koberle, N. Mac Dowell and A. Fantuzzi, BECCS deployment: a reality check, 2019, https://www.imperial.ac.uk/media/imperial-college/grantham-institute/public/publications/briefing-papers/BECCS-deployment-a-reality-check.pdf.
- M. Fasihi, O. Efimova and C. Breyer, J. Cleaner Prod., 2019, 224, 957–980 CrossRef CAS.
- E. S. Sanz-Pérez, C. R. Murdock, S. A. Didas and C. W. Jones, Chem. Rev., 2016, 116, 11840–11876 CrossRef.
- M. Bui, C. S. Adjiman, A. Bardow, E. J. Anthony, A. Boston, S. Brown, P. S. Fennell, S. Fuss, A. Galindo, L. A. Hackett, J. P. Hallett, H. J. Herzog, G. Jackson, J. Kemper, S. Krevor, G. C. Maitland, M. Matuszewski, I. S. Metcalfe, C. Petit, G. Puxty, J. Reimer, D. M. Reiner, E. S. Rubin, S. A. Scott, N. Shah, B. Smit, J. P. Trusler, P. Webley, J. Wilcox and N. Mac Dowell, Energy Environ. Sci., 2018, 11, 1062–1176 RSC.
- S. Deutz and A. Bardow, Nat. Energy, 2021, 6, 203–213 CrossRef.
- M. M. de Jonge, J. Daemen, J. M. Loriaux, Z. J. Steinmann and M. A. Huijbregts, Int. J. Greenhouse Gas Control, 2019, 80, 25–31 CrossRef CAS.
-
T. Terlouw, K. Treyer, C. Bauer and M. Mazzotti, Life Cycle Assessment of Direct Air Carbon Capture and Storage with low-carbon energy sources [Submitted], 2021 Search PubMed.
- R. M. Cuéllar-Franca and A. Azapagic, J. CO2 Utiliz., 2015, 9, 82–102 CrossRef.
- A. Vinca, J. Emmerling and M. Tavoni, Front. Energy Res., 2018, 6, 40 CrossRef.
- Working Group III of the Intergovernmental Panel on Climate Change [Metz, B., O. Davidson, H. C. de Coninck, M. Loos, and L. A. Meyer (eds.)], IPCC, 2005: IPCC Special Report on Carbon Dioxide Capture and Storage, Cambridge technical report, 2005.
- C. van der Giesen, C. J. Meinrenken, R. Kleijn, B. Sprecher, K. S. Lackner and G. J. Kramer, Environ. Sci. Technol., 2017, 51, 1024–1034 CrossRef CAS.
- R. de Richter, T. Ming, P. Davies, W. Liu and S. Caillol, Prog. Energy Combust. Sci., 2017, 60, 68–96 CrossRef.
- S. A. Montzka, E. J. Dlugokencky and J. H. Butler, Nature, 2011, 476, 43–50 CrossRef CAS.
- R. Jackson, E. Solomon, J. Canadell, M. Cargnello and C. Field, Nat. Sustainability, 2019, 2, 436–438 CrossRef.
- N. Jiao, H. Wang, G. Xu and S. Aricò, Natl. Sci. Rev., 2018, 5, 464–468 CrossRef.
- A. de Ramon N’Yeurt, D. P. Chynoweth, M. E. Capron, J. R. Stewart and M. A. Hasan, Process Saf. Environ. Prot., 2012, 90, 467–474 CrossRef.
-
G. Churkina, A. Organschi, C. P. Reyer, A. Ruff, K. Vinke, Z. Liu, B. K. Reck, T. E. Graedel and H. J. Schellnhuber, Buildings as a global carbon sink, 2020 Search PubMed.
- F. Xi, S. J. Davis, P. Ciais, D. Crawford-Brown, D. Guan, C. Pade, T. Shi, M. Syddall, J. Lv, L. Ji, L. Bing, J. Wang, W. Wei, K. H. Yang, B. Lagerblad, I. Galan, C. Andrade, Y. Zhang and Z. Liu, Nat. Geosci., 2016, 880–883 CrossRef CAS.
- L. Rosa, D. L. Sanchez, G. Realmonte, D. Baldocchi and P. D'Odorico, Renewable Sustainable Energy Rev., 2020, 110511 Search PubMed.
- L. Rosa, J. A. Reimer, M. S. Went and P. D'Odorico, Nat. Sustainability, 2020, 658–666 CrossRef.
- C. Chen and M. Tavoni, Clim. Change, 2013, 118, 59–72 CrossRef CAS.
- C. Beuttler, L. Charles and J. Wurzbacher, Front. Climate, 2019, 1, 10 CrossRef.
- M. Gusti, Water, Air, and Soil Pollution: Focus, 2007, 7, 475–482 CrossRef CAS.
- D. Woolf, J. E. Amonette, F. A. Street-Perrott, J. Lehmann and S. Joseph, Nat. Commun., 2010, 1, 1–9 Search PubMed.
- P. Roy and G. Dias, Renewable Sustainable Energy Rev., 2017, 77, 59–69 CrossRef CAS.
- R. Jandl, M. Rodeghiero, C. Martinez, M. F. Cotrufo, F. Bampa, B. van Wesemael, R. B. Harrison, I. A. Guerrini, D. deB Richter, L. Rustad, K. Lorenz, A. Chabbi and F. Miglietta, Sci. Total Environ., 2014, 468-469, 376–383 CrossRef CAS.
- M. Fridahl and M. Lehtveer, Energy Res. Soc. Sci., 2018, 42, 155–165 CrossRef.
- A. Rajeev, R. K. Pati, S. S. Padhi and K. Govindan, J. Cleaner Prod., 2017, 162, 299–314 CrossRef.
- B. Purvis, Y. Mao and D. Robinson, Sustainability Sci., 2019, 14, 681–695 CrossRef.
- H. J. Buck, Clim. Change, 2016, 139, 155–167 CrossRef CAS.
- J. Pires, Sci. Total Environ., 2019, 672, 502–514 CrossRef CAS.
- M. Pizzol, B. Weidema, M. Brandão and P. Osset, J. Cleaner Prod., 2015, 86, 170–179 CrossRef.
- B. P. Weidema, Int. J. Life Cycle Assess., 2006, 11, 89–96 CrossRef.
-
A. Moltesen, A. Bonou, A. Wangel and K. P. Bozhilova-Kisheva, Life Cycle Assessment: Theory and Practice, Springer, Cham, 2017, pp. 401–422 Search PubMed.
- C. Benoît, G. A. Norris, S. Valdivia, A. Ciroth, A. Moberg, U. Bos, S. Prakash, C. Ugaya and T. Beck, Int. J. Life Cycle Assess., 2010, 15, 156–163 CrossRef.
- S. Ahlroth, M. Nilsson, G. Finnveden, O. Hjelm and E. Hochschorner, J. Cleaner Prod., 2011, 19, 145–156 CrossRef.
-
K. Andersson, S. Brynolf, H. Landquist and E. Svensson, Methods and Tools for Environmental Assessment. in Shipping and the Environment, ed. K. Andersson and S. Brynolf and J. Lindgren, M. Wilewska-Bien, Springer, Berlin, Heidelberg, 2016, pp. 265–293 Search PubMed.
- P. Smith, H. Haberl, A. Popp, K. H. Erb, C. Lauk, R. Harper, F. N. Tubiello, A. De Siqueira Pinto, M. Jafari, S. Sohi, O. Masera, H. Böttcher, G. Berndes, M. Bustamante, H. Ahammad, H. Clark, H. Dong, E. A. Elsiddig, C. Mbow, N. H. Ravindranath, C. W. Rice, C. Robledo Abad, A. Romanovskaya, F. Sperling, M. Herrero, J. I. House and S. Rose, Global Change Biol., 2013, 8, 2285–2302 CrossRef.
- D. Lenzi, Global Sustainability, 2018, 1, e7 CrossRef.
- T. Terlouw, X. Zhang, C. Bauer and T. Alskaif, J. Cleaner Prod., 2019, 221, 667–677 CrossRef.
- ecoinvent 3.6 – ecoinvent, 2020, https://www.ecoinvent.org/database/ecoinvent-36/ecoinvent-36.html, Accessed on Fri, May 08, 2020.
Footnote |
† Electronic supplementary information (ESI) available. See DOI: 10.1039/d0ee03757e |
|
This journal is © The Royal Society of Chemistry 2021 |