Viscosity and liquid–liquid phase separation in healthy and stressed plant SOA†
Received
10th December 2020
, Accepted 2nd March 2021
First published on 17th March 2021
Abstract
Molecular composition, viscosity, and liquid–liquid phase separation (LLPS) were investigated for secondary organic aerosol (SOA) derived from synthetic mixtures of volatile organic compounds (VOCs) representing emission profiles for Scots pine trees under healthy and aphid-herbivory stress conditions. Model “healthy plant SOA” and “stressed plant SOA” were generated in a 5 m3 environmental smog chamber by photooxidation of the mixtures at 50% relative humidity (RH). SOA from photooxidation of α-pinene was also prepared for comparison. Molecular composition was determined with high resolution mass spectrometry, viscosity was determined with the poke-flow technique, and liquid–liquid phase separation was investigated with optical microscopy. The stressed plant SOA had increased abundance of higher molecular weight species, reflecting a greater fraction of sesquiterpenes in the stressed VOC mixture compared to the healthy plant VOC mixture. LLPS occurred in both the healthy and stressed plant SOA; however, stressed plant SOA exhibited phase separation over a broader humidity range than healthy plant SOA, with LLPS persisting down to 23 ± 11% RH. At RH ≤25%, both stressed and healthy plant SOA viscosity exceeded 108 Pa s, a value similar to that of tar pitch. At 40% and 50% RH, stressed plant SOA had the highest viscosity, followed by healthy plant SOA and then α-pinene SOA in descending order. The observed peak abundances in the mass spectra were also used to estimate the SOA viscosity as a function of RH and volatility. The predicted viscosity of the healthy plant SOA was lower than that of the stressed plant SOA driven by both the higher glass transition temperatures and lower hygroscopicity of the organic molecules making up stressed plant SOA. These findings suggest that plant stress influences the physicochemical properties of biogenic SOA. Furthermore, a complex mixture of VOCs resulted in a higher SOA viscosity compared to SOA generated from α-pinene alone at ≥25% RH, highlighting the importance of studying properties of SOA generated from more realistic multi-component VOC mixtures.
Environmental significance
Plants stressed by herbivore attacks emit a very different bouquet of volatile organic compounds compared to healthy plants. Atmospheric oxidation of volatiles from stressed plants results in organic haze with very different properties compared to that from healthy plants. The stressed plant haze has particles that are more viscous and more prone to phase separation into aqueous and organic parts. As a result of high viscosity, the mixing of molecules in particles emitted by stressed trees takes hours even, under conditions of ambient relative humidity. The long mixing time affects the dynamics of particle growth and various important properties of the resulting particles including their ability to act as cloud and ice nuclei.
|
Introduction
Secondary organic aerosol (SOA) dominates the total fine particle mass in the atmosphere.1,2 SOA is formed from the condensation of oxidation products of volatile organic compounds (VOCs). Approximately 100
000 different organic compounds have been measured in the atmosphere,3 resulting in the highly complex composition of SOA and a wide range of volatility, hygroscopicity, and reactivity.1 Of the total amount of atmospheric VOCs, roughly 90% come from biogenic sources.4 Because of the chemical complexity of ambient SOA, only 10% of the total mass of SOA has been speciated in terms of molecular composition.1 A comprehensive understanding of the formation, properties, and transformation of SOA is essential to understand their impact on atmospheric processes, climate, and human health.1
The most abundant biogenic VOCs are isoprene (C5H8), monoterpenes (C10H16), and sesquiterpenes (C15H24).4,5 Because α-pinene and limonene typically dominate the monoterpene emission profile in forested areas,6,7 they have commonly been used as representative monoterpenes in laboratory and modeling studies investigating biogenic SOA. In laboratory studies,8,9 SOA generated from a single VOC is commonly used because these simpler systems allow for the investigation of their fundamental physical and chemical properties. These single VOC experimental studies provide parameterizations of SOA formation mechanisms for air quality and climate models. However, ambient SOA is produced from a more complex mixture of VOCs. Using complex VOC mixtures can impact the oxidant reactivity and subsequent products formed when compared to selected single VOCs.10 The discrepancy between laboratory-generated SOA and real atmospheric SOA could lead to errors in the estimation of their impact on climate and air quality, as well as their impact on human health. Therefore, it is pertinent to conduct experiments on SOA formed from realistically complex mixtures of VOCs to better represent ambient scenarios.
Plant health needs to be considered when deciding which VOCs to use to replicate biogenic SOA in a laboratory setting. Plants become stressed due to changing environmental conditions, including biotic and abiotic factors.11 Some examples of abiotic stress include increased temperature, drought, or low soil nutrient levels.11 Examples of biotic stress in plants include insect feeding (including defoliators, bark borers, or sap-sucking aphids) known as herbivory, insect egg laying known as oviposition, or pathogen attack.11–14 When plants become stressed, biochemical defense pathways are initiated, which alters their VOC emissions in both quantity and types of compounds being emitted.15,16 Aphid-herbivory typically increases sesquiterpene emissions from pine trees.17 During stress events, different types of compounds such as acyclic sesquiterpenes are produced.15 The function of these stress VOC emissions includes increased membrane thermotolerance, antioxidant properties, tri-trophic signaling (e.g., attracting natural enemies of herbivores), and inter- and intra-plant communication.18,19 In addition, these VOCs can protect plants from further destruction by priming them for the next stress event they may experience, which can lead to quicker recovery times.20,21 Importantly, changes in healthy and stressed biogenic VOC emissions can alter SOA composition, as well as the distribution of volatilities of SOA compounds, with the potential to alter climate-relevant SOA properties.
Studying how the change in the emission profile of plants impacts SOA properties is important because plant stress is common in ecosystems, and is rapidly increasing in many locations due to a changing climate.22 Moreover, the SOA formed from stressed plants could impact climate differently than SOA formed from healthy plants due to differences in composition and physical properties. Recent studies have shown that herbivore stress has the potential to decrease or increase SOA mass yields,16,23 increase SOA mass and cloud condensation nuclei (CCN) number in the boreal forest,24 decrease hygroscopicity of the resulting particles,25 and increase the relative proportion of fragmentation vs. functionalization reactions in the gas phase.15 Therefore, the chemical and physical properties of healthy versus stressed plant SOA should be investigated to parse out if there is a significant difference between the two systems, with implications for changes in aerosol properties in an evolving environment.
SOA particles were commonly assumed to be liquid-like, permitting fast diffusion of molecules through the particle. However, studies of SOA material generated from the oxidation of single biogenic VOCs, including isoprene and various terpenes, have shown that SOA becomes highly viscous semi-solids or glassy solids under certain environmental conditions such as low temperature and low RH.8,26–38 Increases in viscosity lead to much slower diffusion rates within the SOA, impacting particle growth and evaporation, gas-particle partitioning, size distributions, multiphase chemistry, and the ability of SOA to serve as nuclei for liquid cloud droplets or ice particles.35,39–51
The viscosity of SOA material depends on the molecular weight and the degree of oxidation of its chemical constituents.28,30,52,53 Moreover, the viscosity of SOA is strongly affected by temperature and RH. Water acts as a plasticizer, therefore an increase in RH leads to a decrease in viscosity of amorphous organic materials, allowing for higher diffusion rates of molecules within the SOA.30,39,54 However, an increase in RH may not lead to a decrease in viscosity of organic aerosol with very low hygroscopicity, as seen in previous studies investigating cooking organic aerosol.55 Viscosity and diffusion are interrelated and it is practical to estimate diffusion rates of large organic molecules within SOA from measured viscosity and the Stokes–Einstein equation.56,57 For example, diffusion rates of small organic molecules, water, and oxidants within SOA can be estimated from SOA viscosity using the fractional Stokes–Einstein equation.58
Recently, a parameterization was developed for predicting the viscosity of SOA based on its chemical composition; which can be used with chemical transport models to predict viscosity of SOA in the atmosphere.52,59,60 This parameterization, together with high resolution mass spectrometry measurements of chemical composition, has been used successfully to predict the viscosity of several types of SOA formed from isoprene, α-pinene, toluene, and diesel fuel vapors.8,34,61,62 However, the accuracy of the parameterization for predicting the viscosity of SOA from a mixture of VOCs has not been evaluated.
As an added layer of complexity, individual SOA particles can undergo liquid–liquid phase separation (LLPS) and form two distinct liquid phases, an inner aqueous-rich phase and an outer organic-rich phase. LLPS in SOA particles was initially observed in mixed particles containing organic and inorganic species.63–65 This LLPS phenomena was thought to be, at least partially, driven by the presence of the inorganic salts, which can cause salting out of organics.63 More recently, it has been shown that LLPS also occurs in SOA particles in the absence of inorganic salts.62,66–69 The LLPS events in SOA particles can impact the extent to which gas-particle partitioning of semivolatile compounds may occur,70–72 the reactive uptake of gasses,73–75 as well as their ability to act as nuclei for cloud droplets and ice particles,67,76–78 making it an important process to study.
In this work, we investigate chemical composition, viscosity, and LLPS in SOA produced from complex mixtures of VOCs representative of emissions from healthy plant (abbreviated as hp-SOA from here on) and stressed plant (sp-SOA) pine trees. We compare the results to SOA generated from α-pinene to test whether the physicochemical properties of particles made from a single VOC are comparable to more representative SOA formed from complex mixtures of VOCs. The VOC mixtures used in this work are based on the emission profile of healthy and aphid-stressed Scots pine saplings (Pinus sylvestris L.) previously reported by Faiola et al.15 Pine trees (Pinaceae) have a wide spatial distribution79 and are typically found in boreal forests which cover one-third of the global forested area80 making them a good representative plant for studying biogenic SOA over boreal forests. In addition, we investigate the accuracy of the parameterization from DeRieux et al. (2018)59 by comparing the predicted viscosities of the SOA to the experimentally determined viscosities. Volatility and viscosity are closely related and it was recently reported that SOA with low volatility showed an increased viscosity.29,32,81 Therefore, we also report the volatility distribution of compounds within the hp-SOA and sp-SOA using the parameterization from Li et al. (2016).60
We find that SOA produced from the VOC mixtures is more viscous compared to the more commonly studied α-pinene SOA. Increased farnesene and other sesquiterpene mixing ratios used in the generation of sp-SOA significantly increased the viscosity of the resulting sp-SOA when compared to hp-SOA. In addition, two liquid phases are persistent in sp-SOA over a wider RH range compared to hp-SOA. These findings suggest that consideration of complex plant VOC mixtures and effects of plant stress are important in determining the physicochemical properties of biogenic SOA.
Experimental
VOC mixtures
Two VOC mixtures were created from commercially available compounds listed in Table S1.† The compounds were mixed in molar fractions chosen to represent the VOC profile of compounds emitted from healthy and stressed 8 year old Scots pine (Pinus sylvestris L) trees, as reported in Faiola et al. (2019).15 Valencene was used as a representative cyclic sesquiterpene and a farnesene isomer mixture was used as a proxy for acyclic sesquiterpenes. Ylisirniö et al. (2020) analyzed a similar farnesene isomer mixture to the one used in this study (Sigma Aldrich) and found a significant fractional contribution by bisabolene isomers (40%) and other unidentified sesquiterpenes (20%) in addition to the farnesene isomers.82 The mixing ratios of monoterpenes and sesquiterpenes that were evaporated into the chamber were calculated assuming total desorption into the chamber and are shown in Fig. S1.† The farnesene isomers bar in Fig. S1† contains the bisabolene and other sesquiterpenes observed by Ylisirniö et al. (2020) – we have not performed an explicit analysis of the injected mixture to further classify them. The total VOC mixing ratio was approximately the same across all experiments (200 ppb). The dominant VOC in the healthy mixture was 3-carene with a mixing ratio of 128 ppb. In the stressed mixture α-pinene was the most abundant monoterpene and, compared to the healthy mixture, had three sesquiterpenes with farnesene having the highest mixing ratio (28 ppb) of the three.
SOA generation and collection
SOA was generated by OH-initiated photooxidation of VOCs in air under low-NOx conditions in a 5 m3 Teflon environmental chamber. The chamber was operated in the batch mode at 50% RH and room temperature (21–26 °C) for all trials. Seed particles were not used to avoid interference with experimental viscosity and LLPS measurements. Evaporation of 45 μl of 30 wt% H2O2 into the chamber via a heated inlet (45 °C) produced approximately 2 ppm of H2O2 vapor serving as photochemical OH precursor. Following oxidant injection, 8 μl of either healthy or stressed VOC mixtures were evaporated into the chamber via the same heated inlet, and a bank of UV-B lights was turned on (the UV-B lamp spectral flux density in the chamber is shown in Fig. S2†). To verify completeness of injection of the VOCs into the chamber, gas-phase abundance of monoterpenes (m/z 137.1330) and sesquiterpenes (m/z 205.1956) were monitored using a proton-transfer-reaction time-of flight mass spectrometer (PTR-ToF-MS; Ionicon model 8000) with H3O+ as the reagent ion. The particle size and number concentration were monitored using a scanning mobility particle sizer (SMPS; TSI differential mobility analyzer model 3080 and CPC model 3775). The ozone and NO/NOy concentrations in the chamber were monitored using an O3 analyzer (Thermo Scientific, Model 49i) and a NO-DIF-NOy analyzer (Thermo Scientific, Model 42I-Y), respectively. After three hours of photooxidation, when the particle mass concentration in the chamber reached approximately 200 μg m−3, the UV-B lights were turned off. The steady-state OH concentration was 1.4 × 106 cm−3 calculated from the rate of decay of limonene under similar experimental conditions used in this study. At this OH concentration, the VOC lifetimes were 0.6–3.8 h (Table S1†), at least 50% of each VOC was consumed by the end of the irradiation period. The PTR-ToF-MS data suggested that 40–50% of total monoterpenes and sesquiterpenes were consumed.
For the viscosity and LLPS measurements, SOA particles were collected at 30 L min−1 onto hydrophobic glass substrates placed in stage 9 of a non-rotating microorifice uniform deposit impactor (MOUDI) with all the remaining MOUDI stages removed. Under normal operating conditions, stage 9 collects particles with diameters above 100 nm,83 however, its operation without other MOUDI stages increases the pressure drop across the stage, thus increasing the jet velocity and decreasing the minimal particle size, but potentially increasing particle bounce. The 50% RH in the chamber should help reduce the bouncing effect compared to what would happen in a dry air case.84 This method of collection resulted in discrete spots of aggregated SOA particles. By the time of the viscosity or LLPS measurement, the individual submicron SOA particles in some of the spots merged into “macroparticles” with spherical cap geometry and diameters of 30–100 μm. Hydrophobic glass slides were generated by coating plain glass slides (Hampton Research) with trichloro(1H,1H,2H,2H-perfluorooctyl)silane or FluoroPel 800 (Cytonix USA). Simultaneously, SOA was collected on aluminum foils for high resolution mass spectrometry analysis using the same sampling method. Samples were collected for 2–3 hours. Samples were then placed in protective plastic enclosures, sealed with a vacuum food sealer, and stored in a freezer at −20 °C until analysis (except for 24 h transit time when the samples had to be shipped to other participating laboratories on ice at 0 °C).
High-resolution mass spectrometry
The high-resolution mass spectrometry data were obtained using a high-resolution Q Exactive HF-X Orbitrap mass spectrometer (Thermo Scientific) with a mass resolving power of 2.4 × 105 (at m/z 200) outfitted with a nano-desorption electrospray ionization source (nano-DESI-HRMS).85 Nano-DESI-HRMS was performed in negative and positive modes for the collected SOA samples, similar to previous work.86 The spray voltage was set to 3.5 kV, the capillary temperature was 250 °C, and the S-lens ion funnel RF level was 80. The solvent consisted of a 1
:
1 (v/v) mixture of LC-MS grade acetonitrile and water. The sample was line-scanned starting from the area free of the analyte. Then, all peaks that rose above a signal to noise ratio of 3 were considered as analyte related.85 In addition to the samples, a solvent blank was prepared following the same procedure above, but using a control substrate without analyte. Molecular formulas were assigned similarly to previous work.86,87 Briefly, the peaks were extracted from the mass spectra, and peaks containing 13C isotopes were removed. All peaks were assigned to the formulas CxHyOz with an accuracy of ±0.001 m/z units while containing the assignments to closed-shell ions with even nominal masses and constraining H/C to 0.30–2.25 and O/C to 0.00–2.30. The assigned ion formulas were corrected for the ionization mechanism, and all the HRMS results below are reported as formulas of neutral SOA compounds. The assumed ionization mechanisms were formation of adducts with H+ or Na+ for positive ions and deprotonation for negative ions.
Liquid–liquid phase separation
Events of LLPS in substrate deposited samples of SOA material were measured for all samples using an optical microscope equipped with a CCD (charge-coupled device) camera. The procedure for LLPS has been described by Song et al. (2019).62 In short, the hydrophobic glass slides containing the SOA samples were placed in a RH and temperature-controlled flow cell. The RH was monitored using a chilled-mirror dew point hygrometer (General Eastern M4/E4 dew point monitor, Canada). The SOA particles were initially conditioned at 98% RH in the flow cell for 10 minutes. At this RH, particles already exhibited LLPS. The RH was decreased at a rate of 1% RH min−1 until LLPS was no longer visible. A picture was taken every 10 s throughout the duration of the experiment via the CCD. Afterwards, the RH was quickly dropped down to 0% to confirm LLPS did not reappear at lower RH. Three trials were performed for each SOA generation condition.
Viscosity measurements
Viscosities of SOA were determined using the poke-flow technique described elsewhere.8,26 This method relies on observing the flow of material under an optical microscope after deforming it with a blunt object.88 A schematic of the poke-flow apparatus is shown in Fig. S3.† After collecting SOA on the hydrophobic slides, the slides were placed into a flow-cell mounted onto a polarizing metallurgical inverted microscope (AMScope). Dry or humidified nitrogen passed through the cell and the RH of the outgoing gas flow was measured continuously with an optical chilled mirror hygrometer (1311DR, Chilled Mirror Hygrometer, General Eastern). The hygrometer RH was calibrated with respect to the deliquescence relative humidity (DRH) of potassium carbonate (43% DRH). The temperature was continuously monitored with a thermocouple (Omega HH200A) attached to the top of the flow cell. All experiments were performed at 291–295 K. Before any experiments were performed, the slides with SOA were conditioned at the desired RH (approximately 0%, 10%, 25%, 40% or 50%) for 1.5 h. Longer conditioning times of up to 24 h were later investigated for each SOA type to see if the measured viscosity was sensitive to the conditioning time; however, there was no significant change as shown in Fig. S4.† For the poke-flow experiments, a hydrophobically coated (OilSlip 110, Cytonix USA) needle was mounted to a micromanipulator arm that could move it in XYZ directions. The needle was placed directly above an SOA “macroparticle” (diameter of 30–50 μm), and then moved downwards vertically to penetrate the particle surface and contact the surface of the hydrophobic glass slide. Upon removal of the needle, a visible hole was left behind resulting in the formation of a particle with half-torus geometry. The poked particle was allowed to flow until the area of the hole (A) had recovered to one quarter of the original area of the poke hole (¼A). If allowed long enough, particles would recover to their original, energetically favorable state of spherically capped geometry. The time of the ¼A recovery will be referred to as the experimental flow time (τexp,flow).
Viscosity of the SOA was determined from τexp,flow and fluid dynamics simulations, performed using the Microfluidics module within COMSOL Multiphysics.8,9 The initial configuration in the simulations was half-torus geometry with dimensions consistent with the dimensions in the experiment. The simulations required surface tension, slip length, density, and contact angle. Conservative upper and lower limits were used in the simulations (Table S2†), resulting in conservative upper and lower limits for the simulated viscosities. In the simulations, the material flowed and the hole in the half-torus geometry decreased to minimize the surface energy of the system, consistent with the experiments. From the simulations, we determined the time for the diameter of the hole in the half-torus geometry to decrease to ¼A referred to as τmodel,flow. To determine viscosity from τexp,flow, the viscosity in the simulations was adjusted until τmodel,flow was within 1% of τexp,flow.
Under some conditions, for example at 0% RH, the poked particles did not visibly flow over the course of the observation (6 h for hp-SOA and 19 hours for sp-SOA). In these cases, lower limits to viscosity were obtained from the simulations by assuming the fluid flowed by ≤0.5 μm (the spatial resolution of the microscope) within the observation time in the experiments.
In the poke flow experiments, SOA “macroparticles” were exposed to flow of N2 gas for several hours (1.5–24 h). During this time, semivolatile compounds could potentially evaporate from the SOA, resulting in a change in composition and possibly in viscosity.89 To determine if evaporation of semivolatile compounds was significant in the poke-flow experiments, new SOA samples were exposed to a dry (<0.5% RH) flow of N2 gas in the poke-flow apparatus for 24 h, while monitoring the cross-sectional area of the “macroparticles” (Fig. S5†). The flow rate of the dry N2 gas was the same as the rate used for the measurements because this could affect the evaporation of the SOA particles. These tests showed that the area of the particles changed by less than 2%. Hence, evaporation of semivolatile compounds was regarded as a minor effect in our experiments, at least for dry conditions (<0.5% RH). Even though semivolatile compounds did not evaporate under dry conditions, they could still have evaporated at higher RH values when the viscosity was lower and diffusion within the particles was faster.89–92 The loss of semivolatile compounds should lead to an increase in viscosity.89,90,93 The fact that the viscosity did not significantly change using conditioning times ranging from 1.5 h to 24 h in the poke-flow experiments, even at 50% RH (Fig. S4†), suggests that any loss of semivolatile compounds in our experiment did not significantly affect the viscosity of the particles, even at the highest RH values used in our study.
The mixing times for water in our hp-SOA and sp-SOA “macroparticles” were calculated using the fractional Stokes–Einstein equation at a given RH and temperature (additional details are provided in the ESI†). For the hp-SOA and sp-SOA at ≤25% RH, we cannot be sure that equilibrium was established with the surroundings at 1.5 hours. Therefore, these particles may have had a higher water content than expected based on equilibrium with gas-phase water at ≤25% RH, and hence may have higher viscosity, consistent with the lower limit we report. At 40 and 50% RH, equilibrium established in 1.5 hours is within the uncertainty of our calculations (Fig. S6†).
To investigate mixing times of organic molecules within the SOA by molecular diffusion, we first converted the measured viscosity into a diffusion coefficient (D) using the Stokes–Einstein equation:
|  | (1) |
where
kB is the Boltzmann constant,
T is temperature in Kelvin,
η is the viscosity in Pa s, and
r is the hydrodynamic radius. When calculating diffusion coefficients, we assumed a hydrodynamic radius of 0.38 nm for diffusing SOA molecules.
94
Next, D was converted into the characteristic mixing time within the particle using the following equation:
|  | (2) |
For the purposes of this estimation, the particle diameter was set to dp = 200 nm, which is roughly the median diameter in the volume distribution of ambient SOA-containing particles.95–98 For reference, accumulation mode particles typically range in diameters from 100 to 1000 nm.
Volatility predictions
The assigned molecular formulas from the HRMS data were used to determine the volatility distribution of compounds found within the hp-SOA and sp-SOA. The volatility was calculated based on parameterizations of pure compound saturation mass concentration (C0) by elemental composition reported in Li et al. (2016) for CHO compounds using eqn (3), |  | (3) |
where n0C is the reference carbon number, and nC and nO represent the number of carbon and oxygen atoms, respectively. The values for parameters n0C, bC, bO, and bCO were 22.6, 0.4481, 1.656, and −0.779, respectively, as determined for reference CHO compounds by Li et al. (2016).60
Viscosity predictions
Viscosity was predicted using the method described by DeRieux et al. (2018).59 Briefly, the glass transition temperature (Tg,i), at which a phase transition between amorphous solid and semi-solid states occurs,30 can be predicted as a function of molecular composition for a single compound:59 |  | (4) |
nC, nH, and nO are the number of carbon, hydrogen, and oxygen atoms, respectively. Values of the coefficients [n0C, bC, bH, bCH, bO, and bCO] are [12.13, 10.95, −41.82, 21.61, 118.96, −24.38] for CHO compounds.59 The glass transition temperature of the SOA under dry conditions (Tg,org) was estimated using the Gordon–Taylor equation, assuming the Gordon–Taylor constant (kGT) of 1 for each organic mixture component:53 |  | (5) |
where wi is the mass fraction of an organic compound i.53 We estimated wi using two different methods. In the first method, wi values were assumed to be proportional to the observed peak abundances in the mass spectra. In the second method, the relative abundance of each compound [Ai] was converted from the peak abundance (Ii) considering the effect of molecular weight (Mi) and the degree of unsaturation (represented by the [H/C]i ratio) on ionization efficiency following the method in Nguyen et al. (2013):99 | 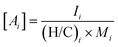 | (6) |
where (H/C)i × Mi is known as adjusted mass (AM).99 Note that we assumed the effective limit of detection (LOD) used in Nguyen et al. (2013) to be zero. This is a reasonable approximation based on the results in Nguyen et al. (2013) that LOD decreased quickly at high AM. In our study, more than 90% of the detected compounds have an AM value higher than 200 Da, resulting in a small LOD.
Under humid conditions, the water content in SOA was estimated using the effective hygroscopicity parameter (κ).100 The κ values derived from cloud condensation nuclei measurements by Zhao et al. (2017)25 of 0.15 and 0.07 were used for hp-SOA and sp-SOA, respectively. This was based on the assumption that hp-SOA were formed from monoterpene-dominated emissions and sp-SOA were formed from sesquiterpene-dominated emissions, with a high proportion of farnesenes and bisabolene, which is consistent with the synthetic profiles studied in this work.25 These κ values are likely upper limits for these types of SOA. The true water content at 50% RH may be lower but likely will still follow the same trend as reported here. Tg of organic–water mixtures (Tg,mix) was then calculated by the Gordon–Taylor equation using a Gordon–Taylor constant (kGT) of 2.5:30,101
| 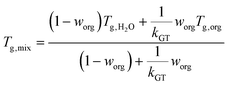 | (7) |
where
Tg,H2O and
Tg,org are the glass transition temperatures of water and SOA organics, and
worg is the mass fraction of the organic compounds in the particle. The mass concentration of water (
mH2O) and SOA (
mSOA) can be used to estimate
worg as
worg =
mSOA/(
mSOA +
mH2O), and
mH2O can be estimated using the effective hygroscopicity factor (
κ):
100 | 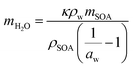 | (8) |
where the density of water (
ρw) is 1 g cm
−3, the density of SOA particles (
ρSOA) is assumed to be 1.4 g cm
−3, and
aw is the water activity calculated as
aw =
RH/100.
102
Using the calculated Tg,mix, the temperature-dependence of viscosity was calculated by applying the Vogel–Tammann–Fulcher (VTF) equation:
| 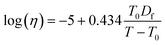 | (9) |
where
T0 is the Vogel temperature calculated as
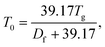
which is deduced from an assumed viscosity of 10
12 Pa s at the glass transition temperature,
103 and
T is the temperature at which the viscosity measurements were conducted (291 K). The fragility parameter (
Df), which characterizes deviation of the temperature dependence of viscosity from the Arrhenius behavior
59,104 was assumed to be 10 based on our previous study.
59 The viscosity is predicted assuming one phase, despite the LLPS observations at higher RH as we do not know composition in the different phases.
Results and discussion
Molecular composition of SOA particles
The high-resolution mass spectra obtained for the hp-SOA and sp-SOA in positive and negative modes are shown in Fig. 1. While the mass spectra indicate similar features, the mass spectrum of the sp-SOA has a larger summed peak abundance for species with molecular weight (MW) above 250 Da (43% for negative mode and 73% for positive mode) than that of the hp-SOA (37% for negative mode and 69% for positive mode). We define high molecular mass as >250 Da because this usually designates a transition region between monomer and dimer products in monoterpene SOA.90,105 This difference between the two mass spectra is expected because the stressed plant VOC mixture contained more sesquiterpene species compared to the healthy plant VOC mixture. The average O
:
C ratios (average ± 1SD) calculated from the high-resolution mass spectrometry data of the hp-SOA and sp-SOA were 0.51 ± 0.20 and 0.41 ± 0.18, respectively. The H
:
C ratios were 1.52 ± 0.19 and 1.52 ± 0.18 for the hp-SOA and sp-SOA, respectively. The lower value of O
:
C in the sp-SOA means that the compounds are on average less oxidized and supports our choice of lower κ to model hygroscopicity of sp-SOA. The most abundant peak in the negative mode mass spectrum for sp-SOA corresponded to C9H14O4, whereas in the hp-SOA the largest peak was C7H12O5. The top 4 peaks in each ionization mode and their reported formulas, names, and plausible structures based on previous studies are listed in Table S3.† It should be noted the exact m/z reported in Table S3† represent various isomers for each sum formula and the exact dominant structures corresponding to each peak have not been confirmed. These compounds include previously reported photooxidation products for both monoterpenes and sesquiterpenes.106–109
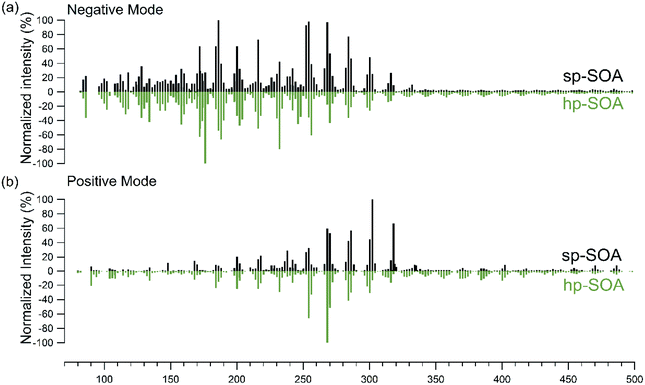 |
| Fig. 1 Nano-DESI mass spectra in (a) negative mode and (b) positive mode for healthy (green) and stressed (black) plant SOA as a function of the molecular weight of the detected neutral compound. | |
The total organic mass concentration for each system was approximately 200 μg m−3 and there were several molecules with predicted volatilities in excess of that value, as seen in the Intermediate Volatility Organic (IVOC; 300 < C0 < 3 × 106 μg m−3) region in Fig. S7.† The abundance of these low molecular weight, intermediate volatility molecules, particularly in the negative ion mode mass spectra, is surprising because they should be too volatile to partition into the condensed phase and contribute significantly to the particle mass under our experimental conditions. There are several possible explanations for the presence of these higher volatility species. First, although ESI-based methods are typically regarded as “soft”, some in-source fragmentation may be occurring. Second, it is possible that these volatile species could be physically entrapped in the particles during the particle growth phase. For example, Vander Wall et al. (2019) identified a burying mechanism in which semivolatile compounds became trapped in viscous SOA, preventing them from escaping back into the gas phase.110 Third, these compounds could be formed after the particles are collected by spontaneous decomposition of larger organic compounds such as peroxides,111,112 with the decomposition products still remaining in the particles due to the high viscosity. At this time, we cannot rule out any of these explanations.
LLPS
LLPS occurred in both the healthy and stressed plant SOA samples as shown in Fig. 2. The light-colored circle in the center of the particle is an optical effect of light scattering from a hemispherical uniform particle.113 For the hp-SOA, the particle was phase separated at 98% RH. As the relative humidity decreased, the inner aqueous-rich phase started to shrink until it reached a critical RH, called the separation relative humidity (SRH), at 91 ± 5% RH where LLPS completely disappeared. Upon further RH reduction, only a single organic-rich phase remained. The sp-SOA particle exhibited phase separation over a broader humidity range than the hp-SOA, with LLPS persisting down to 23 ± 11% RH.
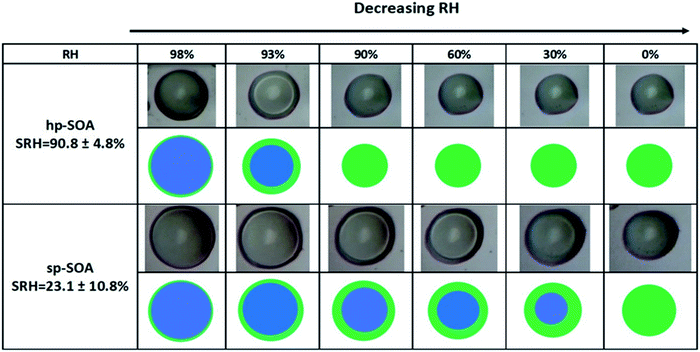 |
| Fig. 2 Optical images and illustrations of stressed and healthy plant SOA particles taken while decreasing relative humidity. The green represents the organic-rich phase and blue represents the aqueous-rich phase. The relative error of the separation relative humidity (SRH) was obtained by using twice the standard deviation from the four measurement results plus an uncertainty of the hydrometer (∼2.5%). The diameter of the particles was between 50–80 μm. | |
Several previous studies have investigated LLPS in SOA from the ozonolysis of single biogenic VOC precursors. In these cases, LLPS was observed only at RH values above 90% RH.66–68 The existence of LLPS in a purely organic SOA particle below 90% RH has only been observed previously for SOA generated by the photooxidation of diesel fuel vapors.62 The presence of LLPS down to as low as 20% RH for sp-SOA, suggests that LLPS could be more common in organic SOA systems than previously thought.
Experimental viscosity and mixing times
Fig. 3 and S8† show images of particles during the poke flow experiments for sp-SOA and hp-SOA, respectively. At 50% RH the holes formed by the needle closed on a time scale of 100–5000 s. In contrast, the “macroparticles” shattered under dry conditions and the particle fragments remained largely unchanged on the timescale of the experiment.
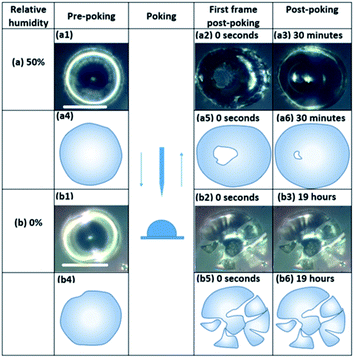 |
| Fig. 3 Optical images of SOA particles produced from photooxidation with VOCs from stressed trees during a poke-flow experiment at (a) 50%, and (b) 0% RH. Images (a1 and b1) are pre-poking images. Images (a4 and b4) are demonstrative diagrams of pre-poking. Images (a2 and b2) are the first frame post-poking. Images (a5 and b5) are demonstrative diagrams of the first frame post-poking. Images (a3 and b3) are the post-poking images. Images (a6 and b6) are demonstrative diagrams of post-poking. The white scale bars correspond to 50 μm. | |
The experimental flow time (τexp,flow) of hp-SOA, sp-SOA, and α-pinene SOA are shown in Fig. 4a. At RH ≤10%, τexp,flow was ≥104 seconds for all three SOA. At RH = 25%, τexp,flow was ≥104 seconds for hp-SOA and sp-SOA, but 1–6 × 103 s for α-pinene SOA. As RH increased the τexp,flow decreased, leading to lower viscosity at higher RH. Over the RHs investigated, the τexp,flow values had the following order: sp-SOA > hp-SOA > α-pinene SOA. This order suggests that stressed plant SOA had the highest viscosity, followed by healthy plant SOA and then α-pinene SOA in descending order. We attribute this difference, at least in part, to the relative amount of sesquiterpenes used to generate the SOA, since sesquiterpenes result in SOA compounds with higher molecular weights, and therefore, higher glass transition temperatures and lower hygroscopicity. Fig. 4b shows viscosity values calculated from τexp,flow measurements. At RH ≤10%, in all cases the viscosity values were ≥108 Pa s (for reference the viscosity of tar pitch is similar to 108 Pa s). At RH >25%, the viscosity decreases as the RH increased. This decrease is expected because the viscosity of water is much less than the viscosity of the condensed organic material and as RH increases the water content of SOA also increases. At 40 and 50% RH, the sp-SOA contain two phases: an aqueous-rich and organic-rich phase. When calculating the viscosity of these particles we did not take into account the presence of two phases in the particles. Hence, the measured viscosity is due to a combination of both phases. The aqueous-rich phase likely has a lower viscosity than the measured viscosity due to the plasticizing effect of water, and conversely the organic-rich phase likely has a higher viscosity than the measured viscosity. At 50% RH, the viscosity of hp-SOA and sp-SOA was ≥104 Pa s (for reference, the viscosity of peanut butter is roughly 103 Pa s). This high viscosity at 50% RH can be explained, in part, by the low hygroscopicity of the SOA samples generated from sesquiterpenes. For example, Varutbangkul et al. (2006) reported a growth factor of only ∼1.01 for sesquiterpene high-NOx SOA at 50% RH,114 resulting in a volume fraction of water of only a few percent, probably insufficient for making the SOA material softer. For comparison, the viscosity values of the hp-SOA and sp-SOA are higher than toluene SOA (anthropogenic SOA proxy) at 40% and 50% RH (Fig. S9†). At 0% RH, the viscosity of α-pinene SOA, hp-SOA, and sp-SOA were in all cases in excess of 108 Pa s – lower limit afforded by the measurement uncertainty. However, at 40% and 50% RH, sp-SOA had the highest viscosity, followed by hp-SOA and then α-pinene SOA in descending order. The elevated viscosity observed in the plant SOA over α-pinene SOA is likely due to the influence of the sesquiterpenes on the MW of the SOA products. The results from this study are consistent with previous studies of rebound of SOA particles generated from Scots pine tree emissions.115
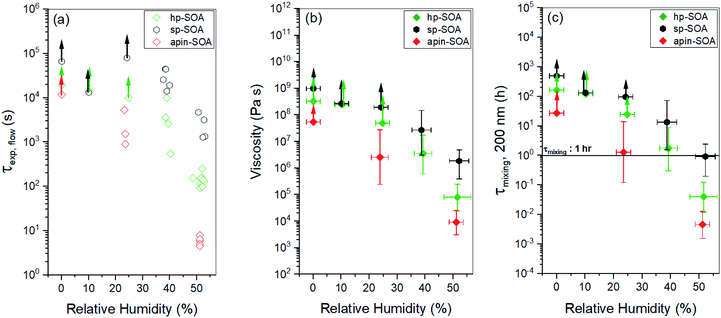 |
| Fig. 4 Results from the poke-flow experiments for hp-SOA (green diamonds), sp-SOA (black hexagons), and α-pinene-SOA (red diamonds): (a) experimental flow time, τexp,flow; (b) viscosity; (c) mixing times of organic molecules within a 200 nm sized particle (τmixing, 200 nm). In panel (a), error in the RH measurement was ±2.5%. In panel (c), horizontal τmixing bars correspond to the 1 h mixing band, which is roughly the mixing time assumed in chemical transport models. Upward arrows indicate lower limits. Vertical bars represent the calculated lower and upper limits of viscosity and τmixing, 200 nm (83% confidence interval) based on COMSOL simulations (see Table S2† for input parameters). | |
Current chemical transport models assume mixing times of organic molecules by molecular diffusion within SOA is less than 1 h, meaning they are well mixed. Mixing times calculated from diffusion based on the viscosity measurements and the Stokes–Einstein equation are shown in Fig. 4c. From the mixing times inferred from the experiments for hp-SOA and sp-SOA, it was found that samples at 0–25% RH had mixing times greater than 10 h, suggesting strong diffusive limitations for condensed-phase transport of particle components. At 40% RH, the median mixing times were still >10 h and 1 h for sp-SOA and hp-SOA, respectively. The hp-SOA samples were observed to have mixing times of <1 h at 50% RH, meaning they can be regarded as well mixed. The mixing times for α-pinene photooxidation SOA had lower mixing time compared to hp-SOA or sp-SOA. Our results indicate that particles produced from realistic mixtures of VOCs have longer mixing time scales in comparison to the 1 h well-mixed assumption at <40% RH. The well-mixed assumption for biogenic SOA, produced under healthy and stressed conditions, is therefore incorrect below 40% RH.
Viscosity predictions
The viscosity predictions for the hp-SOA and sp-SOA are shown in Fig. 5 along with the experimental viscosity values determined via poke-flow method. The predicted viscosity values were calculated using relative peak abundances in a combined mass spectrum that included compounds observed in both positive and negative ion modes. Specifically, peak abundances were normalized to 1 in both positive and negative mass spectra, and the normalized relative peak abundances for the same formula from the two ionization modes were added. The predictions were done after scaling the peak abundances by the adjusted mass based on eqn (3), as well as with unscaled peak abundances similar to our previous studies.59,62Fig. 5 shows that the predicted viscosity values from unscaled peak abundances are approximately two orders of magnitude higher than the predictions for the scaled intensities at ∼50% RH, confirming the expectation that predicted viscosities depend strongly on the assumed relative abundance of detected compounds. The experimentally determined viscosities correlate better with the prediction from the unscaled peak abundances. In theory, the mass scaled approach should correlate better with the experimentally determined viscosities because the ionization efficiency for electrospray is greater for higher molecular weight species, which could lead to an overestimation of SOA viscosity determined from molecular formula assignments.116 However, possible in-source fragmentation (see molecular composition of SOA section) could balance out this effect leading to the unscaled approach correlating with the data better.
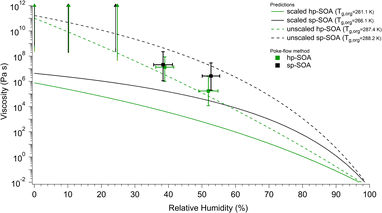 |
| Fig. 5 Predicted viscosity as a function of RH compared to the experimental values determined by the poke-flow method. Vertical bars represent the calculated lower and upper limits of viscosity using the COMSOL simulations (see Table S2† for input parameters). Horizontal bars represent the uncertainty in the relative humidity measurement. The upward arrows indicate lower limits of the viscosity. Predicted viscosities were calculated for healthy and stressed plant SOA using either scaled (solid lines) or unscaled (dashed lines) peak abundances in the mass spectra that combined both positive and negative ion mode peaks. | |
Regardless of the peak abundance scaling method, the sp-SOA is predicted to have higher viscosity than the hp-SOA. The predicted Tg,org values are higher for the sp-SOA, resulting in differences in viscosities of a factor of 2–10 for the predicted viscosity under dry conditions (Fig. 5). The difference between the viscosity predictions of hp-SOA and sp-SOA becomes larger around 50% RH which we suspected was due to the higher assumed hygroscopicity parameter used for the hp-SOA. To test this hypothesis, a sensitivity test was done to investigate the impact the assumed hygroscopicity parameter has on the predicted viscosity. Fig. S7a† shows the viscosity values that individual SOA compounds would have at 50% RH and 291 K, calculated with κ = 0.15 for the healthy and κ = 0.07 for the stressed plant SOA. Shown in Fig. S7b† are the predicted viscosities at 50% RH assuming the same hygroscopicity parameter (κ = 0.1) for both hp-SOA and sp-SOA. A comparison of Fig. S7a and b† indicates the viscosity prediction is dependent on the hygroscopicity parameter used and is the main factor controlling the increased difference in the predicted viscosity between hp-SOA and sp-SOA as RH increases. Fig. S10† shows the predicted viscosity for both data sets assuming κ = 0.1. Compared to the predictions shown in Fig. 5 and S10† shows a smaller difference in overall particle viscosity (approximately an order of magnitude) between the two data sets over all RHs instead of several orders of magnitude as shown in Fig. 5. The overall predicted particle viscosity at >50% RH was larger for hp-SOA when κ = 0.1 compared to when κ = 0.15 and was lower for sp-SOA when κ = 0.1 compared to when κ = 0.07. We suggest that hygroscopicity of SOA produced by mixtures should be explicitly measured to minimize this source of uncertainty in the viscosity predictions. We also should note that factors other than hygroscopicity could play a role in controlling the predicted viscosity values. The predictions are also sensitive to the fragility parameter Df, which is assumed to be the same for hp-SOA and sp-SOA in the absence of more reliable information. Future work should focus on explicit measurements of Df for realistic SOA models.
To gain additional insight into the factors controlling the SOA viscosity, Fig. 6 shows predicted Tg,org and viscosity for individual SOA compounds in the hp-SOA and sp-SOA samples under dry conditions, plotted as a function of compound-specific saturation mass concentration (C0) calculated from eqn (3). Fig. 6 shows the regions representing extremely low volatility organic compounds (ELVOC; C0 < 3 × 10−4 μg m−3), low-volatility organic compounds (LVOC; 3 × 10−4 < C0 < 0.3 μg m−3), semivolatile organic compounds (SVOC; 0.3 < C0 < 300 μg m−3), and intermediate volatility organic compounds (IVOC; 300 < C0 < 3 × 106 μg m−3).81 Because smaller MW compounds are more volatile117 and less viscous,118,119Tg,org steadily decreases as C0 increases. The Tg,org values for the observed healthy and stressed compounds are similar under dry conditions. However, there are individual species identified within the SVOC regime that are larger in abundance in the sp-SOA. The larger abundance of lower volatility species in the sp-SOA with large Tg,org values could drive the difference in particle viscosity between the sp-SOA and hp-SOA. The results of this work indicated the addition of SOA products from sesquiterpenes generally increases SOA particle viscosity. This work also revealed that physicochemical properties of SOA produced from realistic mixtures of biogenic VOCs cannot always be accurately modeled by SOA formed from a single VOC.
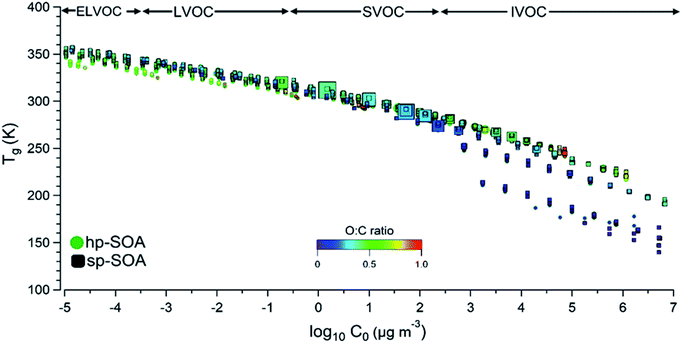 |
| Fig. 6 Glass transition temperature (Tg) of hp-SOA and sp-SOA under dry conditions as a function of saturation mass concentration of individual compounds (C0). Tg is predicted according to DeRieux et al., (2018) and C0 is estimated according to Li et al., (2016). The warmer the color, the higher the O/C ratio. The larger the circle or square marker size, the larger the relative abundance of the species based on the HRMS analysis. | |
We recently reported similar viscosity and LLPS measurements for SOA prepared by the ozonolysis of β-caryophyllene,120 which had 1–2 orders of magnitude lower viscosity than both hp-SOA and sp-SOA, even at 50% RH. Since β-caryophyllene is a cyclic compound, its ozonolysis does not lead to an extensive fragmentation of the molecular backbone,121 thus resulting in compounds with similar molecular weights as from oxidation with OH.122 Therefore, higher viscosity of sp-SOA and hp-SOA compared to that of β-caryophyllene SOA points to additional, presently unknown, factors that affect viscosity of SOA produced from complex mixtures. It is possible a simpler combination of a monoterpene and sesquiterpene could replicate real plant photooxidation SOA, but comparable measurements of this kind have not been reported.
Conclusions
This work demonstrates that products from sesquiterpene oxidation increase viscosity of SOA prepared from simulated mixtures of plant volatiles. It follows that physical properties of biogenic SOA cannot be modeled by parameters developed for SOA generated from a single-component VOC. Guenther et al., (2012) estimated the sesquiterpene to monoterpene emission ratio from boreal needleleaf trees to be ∼16% even for healthy plants4 and this ratio is expected to be even higher for stressed plants. These values are comparable across the different “emission types” previously investigated, therefore, sesquiterpenes could be playing a larger role in SOA properties than is currently assumed. In this study, molar fractions of sesquiterpenes were ∼5% and 25% for healthy and stressed mixtures, respectively, which are reasonable mixtures for a boreal forest environment. In addition, the boreal forest covers an extensive area of land that is particularly sensitive to climate, so real-world sp-SOA could have even higher viscosity than that investigated in this study. The characteristic mixing times for a typical 200 nm diameter particle of the hp-SOA and sp-SOA were greater than 1 h for RH ≤25%, and considerably longer than the corresponding mixing times for α-pinene photooxidation SOA. The results indicate that these healthy and stressed pine tree SOA at low relative humidities may be misrepresented within air quality/climate models that treat biogenic SOA as a mixture of SOA formed from isoprene, monoterpenes, and sesquiterpenes yet neglect viscosity and LLPS as parameters.123
Author contributions
CLF, AL, MS, AKB, and SAN designed research; NRS, GC, YH, APSH, and KS performed experiments; NRS, GC, YH, YL performed data analysis; NRS wrote the paper. All authors contributed to editing the manuscript.
Conflicts of interest
There are no conflicts to declare.
Acknowledgements
The experimental portion of this work was supported by the US National Science Foundation (grant AGS-1853639) and the Natural Science and Engineering Research Council of Canada (grant RGPIN/04315-2014). The viscosity prediction portion of the work was supported by the U.S. Department of Energy, Office of Science, Office of Biological & Environmental Research (BER), Atmospheric Science program (ASR) under award number DOE DE-SC0018349. NRS thanks the University of California, Irvine Department of Chemistry for support with Rowland Graduate Research Fellowship.
References
- M. Hallquist, J. C. Wenger, U. Baltensperger, Y. Rudich, D. Simpson, M. Claeys, J. Dommen, N. M. Donahue, C. George, A. H. Goldstein, J. F. Hamilton, H. Herrmann, T. Hoffmann, Y. Iinuma, M. Jang, M. E. Jenkin, J. L. Jimenez, A. Kiendler-Scharr, W. Maenhaut, G. McFiggans, T. F. Mentel, A. Monod, A. S. H. Prévôt, J. H. Seinfeld, J. D. Surratt, R. Szmigielski and J. Wildt, Atmos. Chem. Phys., 2009, 9, 5155–5236 CrossRef CAS.
- M. Shrivastava, C. D. Cappa, J. Fan, A. H. Goldstein, A. B. Guenther, J. L. Jimenez, C. Kuang, A. Laskin, S. T. Martin, N. L. Ng, T. Petaja, J. R. Pierce, P. J. Rasch, P. Roldin, J. H. Seinfeld, J. Shilling, J. N. Smith, J. A. Thornton, R. Volkamer, J. Wang, D. R. Worsnop, R. A. Zaveri, A. Zelenyuk and Q. Zhang, Rev. Geophys., 2017, 55, 509–559 CrossRef.
- A. H. Goldstein and I. E. Galbally, Environ. Sci. Technol., 2007, 41, 1514–1521 CrossRef CAS PubMed.
- A. B. Guenther, X. Jiang, C. L. Heald, T. Sakulyanontvittaya, T. Duhl, L. K. Emmons and X. Wang, Geosci. Model Dev., 2012, 5, 1471–1492 CrossRef.
- E. A. Marais, D. J. Jacob, J. R. Turner and L. J. Mickley, Environ. Res. Lett., 2017, 12, 054018 CrossRef.
- A. Guenther, C. N. Hewitt, D. Erickson, R. Fall, C. Geron, T. Graedel, P. Harley, L. Klinger, M. Lerdau, W. A. Mckay, T. Pierce, B. Scholes, R. Steinbrecher, R. Tallamraju, J. Taylor and P. Zimmerman, J. Geophys. Res., 1995, 100, 8873–8892 CrossRef CAS.
- A. María Yáñez-Serrano, C. Nölscher, E. Bourtsoukidis, E. Gomes Alves, L. Ganzeveld, B. Bonn, S. Wolff, M. Sa, M. Yamasoe, J. Williams, M. O. Andreae and J. Kesselmeier, Atmos. Chem. Phys., 2018, 18, 3403–3418 CrossRef.
- L. Renbaum-Wolff, J. W. Grayson, A. P. Bateman, M. Kuwata, M. Sellier, B. J. Murray, J. E. Shilling, S. T. Martin and A. K. Bertram, Proc. Natl. Acad. Sci. U. S. A., 2013, 110, 8014–8019 CrossRef CAS PubMed.
- J. W. Grayson, M. Song, M. Sellier and A. K. Bertram, Atmos. Meas. Tech., 2015, 8, 2463–2472 CrossRef.
- G. McFiggans, T. F. Mentel, J. Wildt, I. Pullinen, S. Kang, E. Kleist, S. Schmitt, M. Springer, R. Tillmann, C. Wu, D. Zhao, M. Hallquist, C. Faxon, M. Le Breton, Å. M. Hallquist, D. Simpson, R. Bergström, M. E. Jenkin, M. Ehn, J. A. Thornton, M. R. Alfarra, T. J. Bannan, C. J. Percival, M. Priestley, D. Topping and A. Kiendler-Scharr, Nature, 2019, 565, 587–593 CrossRef CAS PubMed.
- J. K. Holopainen and J. Gershenzon, Trends Plant Sci., 2010, 15, 176–184 CrossRef CAS PubMed.
- Ü. Niinemets, A. Kännaste and L. Copolovici, Front. Plant Sci., 2013, 4, 1–15 Search PubMed.
- Ü. Niinemets, A. Arneth, U. Kuhn, R. K. Monson, J. Peñuelas and M. Staudt, Biogeosciences, 2010, 7, 2203–2223 CrossRef.
- F. Loreto and J. P. Schnitzler, Trends Plant Sci., 2010, 15, 154–166 CrossRef CAS PubMed.
- C. L. Faiola, I. Pullinen, A. Buchholz, F. Khalaj, A. Ylisirniö, E. Kari, P. Miettinen, J. K. Holopainen, M. Kivimäenpää, S. Schobesberger, T. Yli-Juuti and A. Virtanen, ACS Earth Space Chem., 2019, 3, 1756–1772 CrossRef CAS PubMed.
- C. L. Faiola, A. Buchholz, E. Kari, P. Yli-Pirilä, J. K. Holopainen, M. Kivimäenpää, P. Miettinen, D. R. Worsnop, K. E. J. Lehtinen, A. B. Guenther and A. Virtanen, Sci. Rep., 2018, 8, 3053 CrossRef CAS PubMed.
- M. Kivimäenpää, A. B. Babalola, J. Joutsensaari and J. K. Holopainen, Forests, 2020, 11, 573 CrossRef.
- J. K. Holopainen, Trends Plant Sci., 2004, 9, 529–533 CrossRef CAS PubMed.
- C. E. Vickers, J. Gershenzon, M. T. Lerdau and F. Loreto, Nat. Chem. Biol., 2009, 5, 283–291 CrossRef CAS PubMed.
- J. Engelberth, H. T. Alborn, E. A. Schmelz and J. H. Tumlinson, Proc. Natl. Acad. Sci. U. S. A., 2004, 101, 1781–1785 CrossRef CAS PubMed.
- C. J. Frost, H. M. Appel, J. E. Carlson, C. M. De Moraes, M. C. Mescher and J. C. Schultz, Ecol. Lett., 2007, 10, 490–498 CrossRef PubMed.
- F. Loreto, M. Dicke, J. P. Schnitzler and T. C. J. Turlings, Plant, Cell Environ., 2014, 37, 1905–1908 CrossRef PubMed.
- T. F. Mentel, E. Kleist, S. Andres, M. Dal Maso, T. Hohaus, A. Kiendler-Scharr, Y. Rudich, M. Springer, R. Tillmann, R. Uerlings, A. Wahner and J. Wildt, Atmos. Chem. Phys., 2013, 13, 8755–8770 CrossRef.
- J. Joutsensaari, P. Yli-Pirilä, H. Korhonen, A. Arola, J. D. Blande, J. Heijari, M. Kivimäenpää, S. Mikkonen, L. Hao, P. Miettinen, P. Lyytikäinen-Saarenmaa, C. L. Faiola, A. Laaksonen and J. K. Holopainen, Atmos. Chem. Phys., 2015, 15, 12139–12157 CrossRef CAS.
- D. F. Zhao, A. Buchholz, R. Tillmann, E. Kleist, C. Wu, F. Rubach, A. Kiendler-Scharr, Y. Rudich, J. Wildt and T. F. Mentel, Nat. Commun., 2017, 8, 14067 CrossRef CAS PubMed.
- J. W. Grayson, Y. Zhang, A. Mutzel, L. Renbaum-Wolff, O. Böge, S. Kamal, H. Herrmann, S. T. Martin and A. K. Bertram, Atmos. Chem. Phys., 2016, 16, 6027–6040 CrossRef CAS.
- A. Pajunoja, J. Malila, L. Hao, J. Joutsensaari, K. E. J. Lehtinen and A. Virtanen, Aerosol Sci. Technol., 2014, 48, i–iv CrossRef CAS.
- E. Saukko, A. T. Lambe, P. Massoli, T. Koop, J. P. Wright, D. R. Croasdale, D. A. Pedernera, T. B. Onasch, A. Laaksonen, P. Davidovits, D. R. Worsnop and A. Virtanen, Atmos. Chem. Phys., 2012, 12, 7517–7529 CrossRef CAS.
- Y. Zhang, L. Nichman, P. Spencer, J. I. Jung, A. Lee, B. K. Heffernan, A. Gold, Z. Zhang, Y. Chen, M. R. Canagaratna, J. T. Jayne, D. R. Worsnop, T. B. Onasch, J. D. Surratt, D. Chandler, P. Davidovits and C. E. Kolb, Environ. Sci. Technol., 2019, 53, 12366–12378 CrossRef CAS PubMed.
- T. Koop, J. Bookhold, M. Shiraiwa and U. Pöschl, Phys. Chem. Chem. Phys., 2011, 13, 19238–19255 RSC.
- D. A. Ullmann, M. L. Hinks, A. M. MacLean, C. L. Butenhoff, J. W. Grayson, K. Barsanti, J. L. Jimenez, S. A. Nizkorodov, S. Kamal and A. K. Bertram, Atmos. Chem. Phys., 2019, 19, 1491–1503 CrossRef CAS.
- W. M. Champion, N. E. Rothfuss, M. D. Petters and A. P. Grieshop, Environ. Sci. Technol. Lett., 2019, 6, 513–519 CrossRef CAS.
- E. Järvinen, K. Ignatius, L. Nichman, T. B. Kristensen, C. Fuchs, C. R. Hoyle, N. Höppel, J. C. Corbin, J. Craven, J. Duplissy, S. Ehrhart, I. El Haddad, C. Frege, H. Gordon, T. Jokinen, P. Kallinger, J. Kirkby, A. Kiselev, K. H. Naumann, T. Petäjä, T. Pinterich, A. S. H. Prevot, H. Saathoff, T. Schiebel, K. Sengupta, M. Simon, J. G. Slowik, J. Tröstl, A. Virtanen, P. Vochezer, S. Vogt, A. C. Wagner, R. Wagner, C. Williamson, P. M. Winkler, C. Yan, U. Baltensperger, N. M. Donahue, R. C. Flagan, M. Gallagher, A. Hansel, M. Kulmala, F. Stratmann, D. R. Worsnop, O. Möhler, T. Leisner and M. Schnaiter, Atmos. Chem. Phys., 2016, 16, 4423–4438 CrossRef.
- M. Song, P. F. Liu, S. J. Hanna, Y. J. Li, S. T. Martin and A. K. Bertram, Atmos. Chem. Phys., 2015, 15, 5145–5159 CrossRef CAS.
- Y. J. Li, P. Liu, Z. Gong, Y. Wang, A. P. Bateman, C. Bergoend, A. K. Bertram and S. T. Martin, Environ. Sci. Technol., 2015, 49, 13264–13274 CrossRef CAS PubMed.
- Y. Li and M. Shiraiwa, Atmos. Chem. Phys., 2019, 19, 5959–5971 CrossRef CAS.
- Q. Ye, M. A. Upshur, E. S. Robinson, F. M. Geiger, R. C. Sullivan, R. J. Thomson and N. M. Donahue, Chem, 2018, 4, 318–333 CAS.
- A. Pajunoja, A. T. Lambe, J. Hakala, N. Rastak, M. J. Cummings, J. F. Brogan, L. Hao, M. Paramonov, J. Hong, N. L. Prisle, J. Malila, S. Romakkaniemi, K. E. J. Lehtinen, A. Laaksonen, M. Kulmala, P. Massoli, T. B. Onasch, N. M. Donahue, I. Riipinen, P. Davidovits, D. R. Worsnop, T. Petäjä and A. Virtanen, Geophys. Res. Lett., 2015, 42, 3063–3068 CrossRef CAS.
- J. P. Reid, A. K. Bertram, D. O. Topping, A. Laskin, S. T. Martin, M. D. Petters, F. D. Pope and G. Rovelli, Nat. Commun., 2018, 9, 956 CrossRef PubMed.
- M. L. Hinks, M. V. Brady, H. Lignell, M. Song, J. W. Grayson, A. K. Bertram, P. Lin, A. Laskin, J. Laskin and S. A. Nizkorodov, Phys. Chem. Chem. Phys., 2016, 18, 8785–8793 RSC.
- T. Berkemeier, S. S. Steimer, U. K. Krieger, T. Peter, U. Pöschl, M. Ammann and M. Shiraiwa, Phys. Chem. Chem. Phys., 2016, 18, 12662–12674 RSC.
- P. Liu, Y. J. Li, Y. Wang, A. P. Bateman, Y. Zhang, Z. Gong, A. K. Bertram, S. T. Martin, J. A. Paulson and T. H. Chan, ACS Cent. Sci., 2018, 4, 207–215 CrossRef CAS PubMed.
- R. Schmedding, Q. Z. Rasool, Y. Zhang, H. O. T. Pye, H. Zhang, Y. Chen, J. D. Surratt, F. D. Lopez-Hilfiker, J. A. Thornton, A. H. Goldstein and W. Vizuete, Atmos. Chem. Phys., 2020, 20, 8201–8225 CrossRef CAS PubMed.
- R. A. Zaveri, J. E. Shilling, A. Zelenyuk, J. Liu, D. M. Bell, E. L. D'Ambro, C. J. Gaston, J. A. Thornton, A. Laskin, P. Lin, J. Wilson, R. C. Easter, J. Wang, A. K. Bertram, S. T. Martin, J. H. Seinfeld and D. R. Worsnop, Environ. Sci. Technol., 2018, 52, 1191–1199 CrossRef CAS PubMed.
- Y. Zhang, Y. Chen, Z. Lei, N. E. Olson, M. Riva, A. R. Koss, Z. Zhang, A. Gold, J. T. Jayne, D. R. Worsnop, T. B. Onasch, J. H. Kroll, B. J. Turpin, A. P. Ault and J. D. Surratt, ACS Earth Space Chem., 2019, 3, 2646–2658 CrossRef CAS.
- A. Zelenyuk, D. Imre, J. Beránek, E. Abramson, J. Wilson and M. Shrivastava, Environ. Sci. Technol., 2012, 46, 12459–12466 CrossRef CAS PubMed.
- Q. Mu, M. Shiraiwa, M. Octaviani, N. Ma, A. Ding, H. Su, G. Lammel, U. Pöschl and Y. Cheng, Sci. Adv., 2018, 4, eaap7314 CrossRef PubMed.
- M. Shrivastava, S. Lou, A. Zelenyuk, R. C. Easter, R. A. Corley, B. D. Thrall, P. J. Rasch, J. D. Fast, S. L. M. Simonich, H. Shen and S. Tao, Proc. Natl. Acad. Sci. U. S. A., 2017, 114, 1246–1251 CrossRef CAS PubMed.
- S. S. Steimer, M. Lampimäki, E. Coz, G. Grzinic and M. Ammann, Atmos. Chem. Phys., 2014, 14, 10761–10772 CrossRef.
- F. A. Houle, W. D. Hinsberg and K. R. Wilson, Phys. Chem. Chem. Phys., 2015, 17, 4412–4423 RSC.
- Z. Li, K. A. Smith and C. D. Cappa, Atmos. Chem. Phys., 2018, 18, 14585–14608 CrossRef CAS.
- M. Shiraiwa, Y. Li, A. P. Tsimpidi, V. A. Karydis, T. Berkemeier, S. N. Pandis, J. Lelieveld, T. Koop and U. Pöschl, Nat. Commun., 2017, 8, 15002 CrossRef PubMed.
- H. P. Dette, M. Qi, D. C. Schröder, A. Godt and T. Koop, J. Phys. Chem. A, 2014, 118, 7024–7033 CrossRef CAS PubMed.
- E. Mikhailov, S. Vlasenko, S. T. Martin, T. Koop and U. Pöschl, Atmos. Chem. Phys., 2009, 9, 9491–9522 CrossRef CAS.
- Y. Li, A. Tasoglou, A. Liangou, K. P. Cain, L. Jahn, P. Gu, E. Kostenidou and S. N. Pandis, Atmos. Environ., 2018, 176, 103–109 CrossRef CAS.
- E. Evoy, A. M. Maclean, G. Rovelli, Y. Li, A. P. Tsimpidi, V. A. Karydis, S. Kamal, J. Lelieveld, M. Shiraiwa, J. P. Reid and A. K. Bertram, Atmos. Chem. Phys., 2019, 19, 10073–10085 CrossRef CAS.
- H. C. Price, J. Mattsson and B. J. Murray, Phys. Chem. Chem. Phys., 2016, 18, 19207–19216 RSC.
- E. Evoy, S. Kamal, G. N. Patey, S. T. Martin and A. K. Bertram, J. Phys. Chem. A, 2020, 124, 2301–2308 CrossRef CAS PubMed.
- W.-S. Wong Derieux, Y. Li, P. Lin, J. Laskin, A. Laskin, A. K. Bertram, S. A. Nizkorodov and M. Shiraiwa, Atmos. Chem. Phys., 2018, 18, 6331–6351 CrossRef CAS.
- Y. Li, U. Pöschl and M. Shiraiwa, Atmos. Chem. Phys., 2016, 16, 3327–3344 CrossRef CAS.
- M. Song, P. F. Liu, S. J. Hanna, R. A. Zaveri, K. Potter, Y. You, S. T. Martin and A. K. Bertram, Atmos. Chem. Phys., 2016, 16, 8817–8830 CrossRef CAS.
- M. Song, A. M. Maclean, Y. Huang, N. R. Smith, S. L. Blair, J. Laskin, A. Laskin, W.-S. W. DeRieux, Y. Li, M. Shiraiwa, S. A. Nizkorodov and A. K. Bertram, Atmos. Chem. Phys., 2019, 19, 12515–12529 CrossRef CAS.
- Y. You, M. L. Smith, M. Song, S. T. Martin and A. K. Bertram, Int. Rev. Phys. Chem., 2014, 33, 43–77 Search PubMed.
- Y. You, L. Renbaum-Wolff, M. Carreras-Sospedra, S. J. Hanna, N. Hiranuma, S. Kamal, M. L. Smith, X. Zhang, R. J. Weber, J. E. Shilling, D. Dabdub, S. T. Martin and A. K. Bertram, Proc. Natl. Acad. Sci. U. S. A., 2012, 109, 13188–13193 CrossRef CAS PubMed.
- M. A. Freedman, Chem. Soc. Rev., 2017, 46, 7694–7705 RSC.
- L. Renbaum-Wolff, M. Song, C. Marcolli, Y. Zhang, P. F. Liu, J. W. Grayson, F. M. Geiger, S. T. Martin and A. K. Bertram, Atmos. Chem. Phys., 2016, 16, 7969–7979 CrossRef CAS.
- P. Liu, M. Song, T. Zhao, S. S. Gunthe, S. Ham, Y. He, Y. M. Qin, Z. Gong, J. C. Amorim, A. K. Bertram and S. T. Martin, Nat. Commun., 2018, 9, 4076 CrossRef PubMed.
- M. Song, P. Liu, S. T. Martin and A. K. Bertram, Atmos. Chem. Phys., 2017, 17, 11261–11271 CrossRef CAS.
- N. Rastak, A. Pajunoja, J. C. Acosta Navarro, J. Ma, M. Song, D. G. Partridge, A. Kirkevåg, Y. Leong, W. W. Hu, N. F. Taylor, A. Lambe, K. Cerully, A. Bougiatioti, P. Liu, R. Krejci, T. Petäjä, C. Percival, P. Davidovits, D. R. Worsnop, A. M. L. Ekman, A. Nenes, S. Martin, J. L. Jimenez, D. R. Collins, D. O. Topping, A. K. Bertram, A. Zuend, A. Virtanen and I. Riipinen, Geophys. Res. Lett., 2017, 44, 5167–5177 CrossRef CAS.
- J. H. Seinfeld, G. B. Erdakos, W. E. Asher and J. F. Pankow, Environ. Sci. Technol., 2001, 35, 1806–1817 CrossRef CAS.
- A. Zuend, C. Marcolli, T. Peter and J. H. Seinfeld, Atmos. Chem. Phys., 2010, 10, 7795–7820 CrossRef CAS.
- E. I. Chang and J. F. Pankow, Atmos. Environ., 2006, 40, 6422–6436 CrossRef CAS.
- J. A. Thornton, C. F. Braban and J. P. D. Abbatt, Phys. Chem. Chem. Phys., 2003, 5, 4593–4603 RSC.
- V. F. McNeill, J. Patterson, G. M. Wolfe and J. A. Thornton, Atmos. Chem. Phys., 2006, 6, 1635–1644 CrossRef CAS.
- R. Schmedding, M. Ma, Y. Zhang, S. Farrell, H. O. T. Pye, Y. Chen, C. Wang, Q. Z. Rasool, S. H. Budisulistiorini, A. P. Ault, J. D. Surratt and W. Vizuete, Atmos. Environ., 2019, 213, 456–462 CrossRef CAS PubMed.
- G. P. Schill and M. A. Tolbert, Atmos. Chem. Phys., 2013, 13, 4681–4695 CrossRef.
- J. F. Davies, A. Zuend and K. R. Wilson, Atmos. Chem. Phys., 2019, 19, 2933–2946 CrossRef CAS.
- J. Ovadnevaite, A. Zuend, A. Laaksonen, K. J. Sanchez, G. Roberts, D. Ceburnis, S. Decesari, M. Rinaldi, N. Hodas, M. C. Facchini, J. H. Seinfeld and C. O'Dowd, Nature, 2017, 546, 637–641 CrossRef CAS.
-
L. Hämet-Ahti, A. Palmén; P. Alanko, P. M. A. Tigerstedt, Woody Flora of Finland, University Press, Helsinki, 1992 Search PubMed.
- S. Gauthier, P. Bernier, T. Kuuluvainen, A. Z. Shvidenko and D. G. Schepaschenko, Science, 2015, 349, 819–822 CrossRef CAS PubMed.
- Y. Li, D. A. Day, H. Stark, J. L. Jimenez and M. Shiraiwa, Atmos. Chem. Phys., 2020, 20, 8103–8122 CrossRef CAS.
- A. Ylisirniö, A. Buchholz, C. Mohr, Z. Li, L. Barreira, A. Lambe, C. Faiola, E. Kari, T. Yli-Juuti, S. A. Nizkorodov, D. R. Worsnop, A. Virtanen and S. Schobesberger, Atmos. Chem. Phys., 2020, 20, 5629–5644 CrossRef.
- V. A. Marple, K. L. Rubow and S. M. Behm, Aerosol Sci. Technol., 1991, 14, 434–436 CrossRef CAS.
- A. P. Bateman, A. K. Bertram and S. T. Martin, J. Phys. Chem. A, 2015, 119, 4386–4395 CrossRef CAS PubMed.
- P. J. Roach, J. Laskin and A. Laskin, Anal. Chem., 2010, 82, 7979–7986 CrossRef CAS PubMed.
- L. T. Fleming, P. Lin, A. Laskin, J. Laskin, R. Weltman, R. D. Edwards, N. K. Arora, A. Yadav, S. Meinardi, D. R. Blake, A. Pillarisetti, K. R. Smith and S. A. Nizkorodov, Atmos. Chem. Phys., 2018, 18, 2461–2480 CrossRef CAS.
- S. A. Nizkorodov, J. Laskin and A. Laskin, Phys. Chem. Chem. Phys., 2011, 13, 3612–3629 RSC.
- B. J. Murray, A. E. Haddrell, S. Peppe, J. F. Davies, J. P. Reid, D. O'Sullivan, H. C. Price, R. Kumar, R. W. Saunders, J. M. C. Plane, N. S. Umo and T. W. Wilson, Atmos. Chem. Phys., 2012, 12, 8575–8587 CrossRef CAS.
- T. Yli-Juuti, A. Pajunoja, O. P. Tikkanen, A. Buchholz, C. Faiola, O. Väisänen, L. Hao, E. Kari, O. Peräkylä, O. Garmash, M. Shiraiwa, M. Ehn, K. Lehtinen and A. Virtanen, Geophys. Res. Lett., 2017, 44, 2562–2570 CrossRef CAS PubMed.
- A. Buchholz, A. T. Lambe, A. Ylisirniö, Z. Li, O.-P. Tikkanen, C. Faiola, E. Kari, L. Hao, O. Luoma, W. Huang, C. Mohr, D. R. Worsnop, S. A. Nizkorodov, T. Yli-Juuti, S. Schobesberger and A. Virtanen, Atmos. Chem. Phys., 2019, 19, 4061–4073 CrossRef CAS.
- R. A. Zaveri, J. E. Shilling, A. Zelenyuk, M. A. Zawadowicz, K. Suski, S. China, D. M. Bell, D. Veghte and A. Laskin, Environ. Sci. Technol., 2020, 54, 2595–2605 CrossRef CAS PubMed.
- Z. Li, O. P. Tikkanen, A. Buchholz, L. Hao, E. Kari, T. Yli-Juuti and A. Virtanen, ACS Earth Space Chem., 2019, 3, 2775–2785 CrossRef CAS.
- J. Wilson, D. Imre, J. Beránek, M. Shrivastava and A. Zelenyuk, Environ. Sci. Technol., 2015, 49, 243–249 CrossRef CAS PubMed.
- K. E. Huff Hartz, T. Rosenørn, S. R. Ferchak, T. M. Raymond, M. Bilde, N. M. Donahue and S. N. Pandis, J. Geophys. Res.: Atmos., 2005, 110, D14208 CrossRef.
- A. M. Maclean, C. L. Butenhoff, J. W. Grayson, K. Barsanti, J. L. Jimenez and A. K. Bertram, Atmos. Chem. Phys., 2017, 17, 13037–13048 CrossRef CAS.
- U. Pöschl, S. T. Martin, B. Sinha, Q. Chen, S. S. Gunthe, J. A. Huffman, S. Borrmann, D. K. Farmer, R. M. Garland, G. Helas, J. L. Jimenez, S. M. King, A. Manzi, E. Mikhailov, T. Pauliquevis, M. D. Petters, A. J. Prenni, P. Roldin, D. Rose, J. Schneider, H. Su, S. R. Zorn, P. Artaxo and M. O. Andreae, Science, 2010, 329, 1513–1516 CrossRef PubMed.
- S. T. Martin, M. O. Andreae, D. Althausen, P. Artaxo, H. Baars, S. Borrmann, Q. Chen, D. K. Farmer, A. Guenther, S. S. Gunthe, J. L. Jimenez, T. Karl, K. Longo, A. Manzi, T. Müller, T. Pauliquevis, M. D. Petters, A. J. Prenni, U. Pöschl, L. V. Rizzo, J. Schneider, J. N. Smith, E. Swietlicki, J. Tota, J. Wang, A. Wiedensohler and S. R. Zorn, Atmos. Chem. Phys., 2010, 10, 11415–11438 CrossRef CAS.
- I. Riipinen, J. R. Pierce, T. Yli-Juuti, T. Nieminen, S. Häkkinen, M. Ehn, H. Junninen, K. Lehtipalo, T. Petäjä, J. Slowik, R. Chang, N. C. Shantz, J. Abbatt, W. R. Leaitch, V.-M. Kerminen, D. R. Worsnop, S. N. Pandis, N. M. Donahue and M. Kulmala, Atmos. Chem. Phys., 2011, 11, 3865–3878 CrossRef CAS.
- T. B. Nguyen, S. A. Nizkorodov, A. Laskin and J. Laskin, Anal. Methods, 2013, 5, 72–80 RSC.
- M. D. Petters and S. M. Kreidenweis, Atmos. Chem. Phys., 2007, 7, 1961–1971 CrossRef CAS.
- B. Zobrist, C. Marcolli, D. A. Pedernera and T. Koop, Atmos. Chem. Phys., 2008, 8, 5221–5244 CrossRef CAS.
- M. Kuwata and S. T. Martin, Proc. Natl. Acad. Sci. U. S. A., 2012, 109, 17354–17359 CrossRef CAS PubMed.
- C. A. Angell, Chem. Rev., 2002, 102, 2627–2650 CrossRef CAS PubMed.
- C. A. Angell, J. Non-Cryst. Solids, 1991, 131–133, 13–31 CrossRef CAS.
- D. E. Romonosky, A. Laskin, J. Laskin and S. A. Nizkorodov, J. Phys. Chem. A, 2015, 119, 2594–2606 CrossRef CAS PubMed.
- I. El Haddad, N. Marchand, B. Temime-Roussel, H. Wortham, C. Piot, J.-L. Besombes, C. Baduel, D. Voisin, A. Armengaud and J.-L. Jaffrezo, Atmos. Chem. Phys., 2011, 11, 2059–2079 CrossRef CAS.
- M. Jaoui, E. Corse, T. E. Kleindienst, J. H. Offenberg, M. Lewandowski and E. O. Edney, Environ. Sci. Technol., 2006, 40, 3819–3828 CrossRef CAS PubMed.
- W. Fang, L. Gong and L. Sheng, Environ. Chem., 2017, 14, 75–90 CrossRef CAS.
- L. D. Yee, G. Isaacman-Vanwertz, R. A. Wernis, M. Meng, V. Rivera, N. M. Kreisberg, S. V Hering, M. S. Bering, M. Glasius, M. A. Upshur, A. G. Bé, R. J. Thomson, F. M. Geiger, J. H. Offenberg, M. Lewandowski, I. Kourtchev, M. Kalberer, S. De Sá, S. T. Martin, M. L. Alexander, B. B. Palm, W. Hu, P. Campuzano-Jost, D. A. Day, J. L. Jimenez, Y. Liu, K. A. Mckinney, P. Artaxo, J. Viegas, A. Manzi, M. B. Oliveira, R. De Souza, L. A. T. Machado, K. Longo and A. H. Goldstein, Atmos. Chem. Phys., 2018, 18, 10433–10457 CrossRef CAS.
- A. C. Vander Wall, V. Perraud, L. M. Wingen and B. J. Finlayson-Pitts, Environ. Sci.: Processes Impacts, 2020, 22, 66–83 RSC.
- M. Krapf, I. El Haddad, E. A. Bruns, U. Molteni, K. R. Daellenbach, A. S. H. Prévôt, U. Baltensperger and J. Dommen, Chem, 2016, 1, 603–616 CAS.
- H. Li, Z. Chen, L. Huang and D. Huang, Atmos. Chem. Phys., 2016, 16, 1837–1848 CrossRef CAS.
- A. K. Bertram, S. T. Martin, S. J. Hanna, M. L. Smith, A. Bodsworth, Q. Chen, M. Kuwata, A. Liu, Y. You and S. R. Zorn, Atmos. Chem. Phys., 2011, 11, 10995–11006 CrossRef CAS.
- V. Varutbangkul, F. J. Brechtel, R. Bahreini, N. L. Ng, M. D. Keywood, J. H. Kroll, R. C. Flagan, J. H. Seinfeld, A. Lee and A. H. Goldstein, Atmos. Chem. Phys., 2006, 6, 2367–2388 CrossRef CAS.
- A. Virtanen, J. Kannosto, H. Kuuluvainen, A. Arffman, J. Joutsensaari, E. Saukko, L. Hao, P. Yli-Pirilä, P. Tiitta, J. K. Holopainen, J. Keskinen, D. R. Worsnop, J. N. Smith and A. Laaksonen, Atmos. Chem. Phys., 2011, 11, 8759–8766 CrossRef CAS.
- C. M. Kenseth, N. J. Hafeman, Y. Huang, N. F. Dalleska, B. M. Stoltz and J. H. Seinfeld, Environ. Sci. Technol., 2020, 54, 12829–12839 CrossRef CAS.
- M. Shiraiwa, T. Berkemeier, K. A. Schilling-Fahnestock, J. H. Seinfeld and U. Pöschl, Atmos. Chem. Phys., 2014, 14, 8323–8341 CrossRef.
- L. H. Thomas, R. Meatyard, H. Smith and G. H. Davis, J. Chem. Eng. Data, 1979, 24, 161–164 CrossRef CAS.
- N. E. Rothfuss and M. D. Petters, Environ. Sci. Technol., 2017, 51, 271–279 CrossRef CAS PubMed.
- A. M. Maclean, N. R. Smith, Y. Li, Y. Huang, A. P. S. Hettiyadura, G. V. Crescenzo, M. Shiraiwa, A. Laskin, S. A. Nizkorodov and A. K. Bertram, ACS Earth Space Chem., 2021, 5, 305–318 CrossRef CAS.
- M. Jaoui, S. Leungsakul and R. M. Kamens, J. Atmos. Chem., 2003, 45, 261–287 CrossRef CAS.
- A. Lee, A. H. Goldstein, J. H. Kroll, N. L. Ng, V. Varutbangkul, R. C. Flagan and J. H. Seinfeld, J. Geophys. Res., 2006, 111, D17305 CrossRef.
- M. Shrivastava, M. O. Andreae, P. Artaxo, H. M. J. Barbosa, L. K. Berg, J. Brito, J. Ching, R. C. Easter, J. Fan, J. D. Fast, Z. Feng, J. D. Fuentes, M. Glasius, A. H. Goldstein, E. G. Alves, H. Gomes, D. Gu, A. Guenther, S. H. Jathar, S. Kim, Y. Liu, S. Lou, S. T. Martin, V. F. McNeill, A. Medeiros, S. S. de Sá, J. E. Shilling, S. R. Springston, R. A. F. Souza, J. A. Thornton, G. Isaacman-VanWertz, L. D. Yee, R. Ynoue, R. A. Zaveri, A. Zelenyuk and C. Zhao, Nat. Commun., 2019, 10, 1046 CrossRef PubMed.
Footnote |
† Electronic supplementary information (ESI) available. See DOI: 10.1039/d0ea00020e |
|
This journal is © The Royal Society of Chemistry 2021 |