Peroxy radical kinetics and new particle formation
Received
25th November 2020
, Accepted 14th January 2021
First published on 22nd January 2021
Environmental significance
New particle formation is a major contributor to atmospheric aerosol number and is enhanced by low volatility organics. Understanding the conditions under which these organics are formed is crucial to our understanding of new particle formation. These processes are often studied in laboratory smog chamber experiments, which simulate atmospheric conditions without the complication of the many species present in the actual atmosphere. In addition, parameterizations derived from those experiments may have limited applicability if they lack molecular detail; understanding this chemistry at the molecular level allows for a broader extension of experimental results. In this work we look at how species present in abundance in the atmosphere, but not in smog chambers, affect the chemistry that leads to these low volatility products. This can cause a high bias in new-particle formation rates observed in smog chamber experiments. Thus we stress the importance of knowing the exact conditions of smog chamber experiments and building molecular level understanding before extending results to atmospheric conditions.
|
1 Introduction
Our understanding of organic oxidation in the atmosphere has been transformed by recent smog-chamber1,2 and flow-reactor experiments,3–5 enabled by extraordinary advances in mass spectrometry6,7 and particle detection.8 Especially interesting is a process known as “autoxidation”, the self oxidation of organic compounds in the presence of only molecular oxygen.9–11 Within seconds after an oxidant (OH, O3, Cl, NO3, etc.) reacts with a stable volatile organic compound (VOC), autoxidation can generate highly oxygenated organic molecules (HOMs) with multiple oxygen containing functional groups. The HOMs in turn can have very low vapor pressures and thus condense to existing particles as secondary organic aerosol, even driving growth of sub-10 nm particles.12,13 In some cases the HOMs can even nucleate new particles, with14 or without15 sulfuric acid.
New-particle formation in the atmosphere contributes up to half of the number concentration of global cloud condensation nuclei and there are huge uncertainties associated with the mechanisms of nucleation and growth and their sensitivities to different conditions.16–18 Because of its contribution to cloud condensation nuclei, accurately representing new-particle formation in models is critical for understanding aerosol cloud interactions and thus aerosol climate forcing.16,19–21 One of the largest sources of uncertainty in anthropogenic aerosol forcing is the pre-industrial baseline;22 this is because the relationship between new-particle formation and the concentration of cloud condensation nuclei is highly non-linear and so the forcing – the difference between the present day and the pre-industrial – depends strongly on the pre-industrial initial condition.
Our understanding of pre-industrial aerosol in turn depends on our understanding of the contribution of biogenic vapors to aerosols. Biogenic volatile organic compounds (VOCs) dominate global VOC concentrations, especially in clean environments.23,24 Monoterpenes especially have been widely studied because of their role in secondary aerosol formation, both through growth of primary aerosol and their contribution to new particle formation. Monoterpenes, including α-pinene, are a large source of atmospheric precursor VOCs; they can be oxidized to form low-volatility organics and have been shown to contribute to growth of new particles.12,15 Organic peroxy radicals (RO2) formed after an initial step of monoterpene oxidation can autoxidize rapidly and potentially multiple times to form HOMs.
However, it appears that HOMs are always relatively minor products of VOC oxidation, with total molar yields ≲0.1 in most cases.25,26 Furthermore, HOMs do not necessarily have “low volatility” in a sense that is meaningful in the atmosphere. We define volatility classes based on saturation mass concentrations (C*, in μg m−3)27,28 according to the behavior of vapors in those classes, and there are a succession of low volatility classes with C* ≤ 0.1 μg m−3 (p° ∼ 10−6 Pa). Ultra Low Volatility Organic Compounds (ULVOC) can nucleate – they will often stick to anything, including other ULVOCs. Extremely Low Volatility Organic Compounds (ELVOC) will stick to any existing particle, no matter how small, but are not efficient nucleators. Low Volatility Organic Compounds (LVOC) stick to most particles but are sensitive to curvature and the Kelvin effect, so may not stick to particles smaller than 5–10 nm diameter. Semi-Volatile Organic Compounds (SVOC) exist in significant quantities in both the gas phase and in particles at equilibrium under typical atmospheric conditions. Even SVOC have very low vapor pressures no greater than 10−3 Pa.
While many HOMs have saturation concentrations in the LVOC, ELVOC, and ULVOC ranges due to their high oxygen content, some HOMs are in the SVOC range. This brings in an important distinction between HOMs and the volatility classes. HOMs have at least 6 oxygen atoms and are formed in the gas phase via autoxidation, but the definition places no restrictions on volatility.11 The volatility classes describe saturation concentration ranges, but do not restrict the chemistry that formed the species.
Further, substantial evidence suggests that “pure biogenic” nucleation is driven by covalently bound “dimers” (ROOR) formed when two autoxidized peroxy radicals react with each other.28–32 These dimers are formed via reaction of two peroxy radical species and result in large molecules that may be ULVOCs and thus contribute to nucleation. However, these dimers do not necessarily have to be HOMs, as two peroxy radicals that have not undergone any autoxidation may form a dimer, but most dimers are either ULVOCs or LVOCs and thus contribute to nucleation and growth of new particles. This raises a concern that applying smog-chamber or flow-reactor data to the atmosphere may not be straightforward. These are complex reaction systems, and radical termination (especially dimer formation) is a non-linear (even explicitly quadratic) process.
One specific concern is that RO2 to HO2 ratios (RO2
:
HO2) in chambers may be artificially high due to low concentrations of oxygenated carbon-containing molecules like CO, CH2O, etc. (OVOCs for shorthand). Small OVOCs promote the direct conversion of OH to HO2. In the atmosphere, where these OVOCs are typically abundant, RO2
:
HO2 ≲1,33,34 but when no OVOCs are present (which is typical of many laboratory experiments), RO2
:
HO2 can be very large. This means that chambers with relatively low OVOC levels likely will not have atmospherically relevant RO2
:
HO2. If this alters the radical termination and thus stable product formation (including HOMs and especially dimers), direct projections to atmospheric conditions will not be straightforward. Furthermore, the absolute radical concentrations (and thus overall termination rates) may also compete with autoxidation, which may again affect projections to atmospheric conditions.
We show a simplified chemical topology of this system in Fig. 1. This emphasizes the HOx–ROx radical cycle, progressing from the reaction of a VOC with an oxidant (OH here) to RO2, then an alkoxy radical (RO), then HO2, and finally back to OH. We omit many important termination steps as well as the specific conversion reactions to focus on the key dependencies. The central issue is RO2 termination, which depends on RO2
:
HO2 as well as the associated radical–radical kinetics. At sufficiently high RO2
:
HO2, RO2 cross reactions will dominate termination, either via dimer formation as shown or via the so-called “molecular” pathway.35 At sufficiently low RO2
:
HO2, RO2 + HO2 may dominate termination via hydroperoxide (ROOH) formation. VOC oxidation will immediately yield RO2, as shown, but OVOC oxidation (using CO as a surrogate in the figure as well as the rest of this study) will often bypass RO2 formation and lead straight to HO2.36 Because atmospheric VOC reaction mechanisms inevitably involve multiple oxidation steps and multiple intermediate OVOC products, the atmosphere is relatively rich in OVOCs (with fast OVOC reaction rates) compared to VOCs (and VOC reaction rates) and so tends to have RO2
:
HO2 ≲ 1.
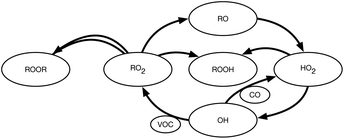 |
| Fig. 1 Phenomenology for HOx–ROx radical cycling chemistry and volatile organic hydrocarbon (VOC) oxidation (without NOx). VOC oxidation leads to organo-peroxy radicals (RO2). If the RO2 do not terminate, they produce RO radicals, some of which rapidly convert to hydroperoxy radicals (HO2). HO2 can be converted back to OH, completing the cycle. For hydrocarbons, RO2 is almost inevitably produced before HO2 and termination, etc., can make RO2 : HO2 ≫ 1. However, oxygenated volatile organic carbon (OVOCs), or most simply CO, will convert OH to HO2 directly. Because the atmosphere is rich in CO and OVOCs, RO2 : HO2 ≲ 1; however, smog chambers often have very low OVOCs and so can have RO2 : HO2 ≫ 1. This can bias “chamber” chemistry versus chemistry typical of the atmosphere. | |
In broad terms, HO2 and RO2 react with each other roughly 1 in every 10–30 collisions (k ≃ 10−11 cm3 mol−1 s−1),37 depending only weakly on the identity of the R group. A further simplification is that the HO2 + NO reaction and all RO2 + NO reactions (not shown) also react with about this frequency. Thus “high NOx” (really high NO) conditions occur when NO
:
HO2 ≫ 1 and “low-NOx” conditions when NO
:
HO2 ≪ 1.38 Here we focus on low-NO conditions. A huge complication is that RO2 + RO2 cross reaction kinetics are rich and variable, ranging from extremely slow to extremely fast.35,39,40
Many RO2 species are formed even during oxidation of a single VOC, and so there are many squared cross reactions; the real atmosphere is quadratically richer still. The rich and variable cross-reaction kinetics and relative paucity of kinetics data require parameterization of most RO2 cross reactions. Evidence suggests that electron-donating groups near the peroxy moiety (i.e. multiple CH3 groups such as t-butyl peroxy radicals) tend to raise the cross reaction barrier and reduce the rate coefficient precipitously, while electron-withdrawing groups (i.e. containing oxygen, such as peroxy acyl) tend to lower the barrier and raise the rate coefficient. Autoxidation involves successive internal H-atom transfers and oxygen addition in RO2, and so the autoxidized RO2 (we shall refer to these as “OxnRO2” with n corresponding to the number of autoxidation steps) have multiple oxygenated functional groups and thus may have high cross reaction rate coefficients. There is still significant uncertainty as to just how fast the cross reactions get for relevant OxnRO2. The peroxy acyl self reaction is fast, occurring in 1 out of 10 collisions. Some direct measurements and model simulations indicate that some OxnRO2 can react with each other on every collision,4,30 while other studies suggest reaction probabilities closer to 1 in 1003. This may be modeling or measurement error, or it may simply reflect the enormous diversity of RO2 species and their cross reactions. In light of this, we follow Schervish and Donahue28 using the general scheme first proposed by Madronich and Calvert.39 We define “anchor” self-reaction rate coefficients for classes starting with tertiary-RO2 (t-butyl RO2), extending through primary RO2 and then progressively more oxidized RO2. The rate coefficients increase dramatically through this range. The cross-reaction rate coefficients are then given by the geometric mean, including a symmetry factor
.28,39
Schervish and Donahue28 showed that the RO2 + HO2 reaction was not competitive under any of the conditions of temperature or NOx when simulating a chamber experiment without added OVOCs (and thus very high RO2
:
HO2, as we will show). While under low NOx conditions the RO2 + HO2 reaction does occur, under the conditions studied in that work, it never built up appreciable yields. However, oxidation of CO to CO2 (as a surrogate for all OVOCs) converts OH directly to HO2; this reaction can become increasingly important, potentially dominating the yields. Therefore just as NOx has been shown to suppress dimer yields, increasing HO2 is likely to have a similar effect.
Prior studies have shown that dimerization can be important under chamber conditions, but as the RO2 rate coefficients (and dimerization branching ratios) are a huge source of uncertainty in this chemistry, it is difficult to say with certainty that dimerization is important in the real atmosphere.28,30 Zhao et al.3 suggest a minimum rate coefficient of 10−12 cm3 mol−1 s−1 for relevant RO2 cross reactions while Berndt et al.4 report some rate coefficients close to the kinetic limit of 10−10 cm3 mol−1 s−1 but also find rate coefficients that span orders of magnitude for different dimers. Thus likely some dimers form very quickly while others form much more slowly. Those that form quickly may form under any relevant RO2
:
HO2 conditions in α-pinene ozonolysis experiments (both in the chamber and in the atmosphere), while those that form slowly may appear when RO2
:
HO2 is high (in the chamber, especially in dark ozonolysis experiments), but not when RO2
:
HO2 is low (in the atmosphere).
Relatively low RO2
:
HO2 values in the atmosphere are due to high concentrations of OVOCs that promote direct conversion of OH to HO2. In general, RO2
:
HO2 ≲ 1 in the atmosphere, with higher values observed in low-NOx conditions where radical cycling and radical chain lengths tend to be lower. For example, Tan et al. reported RO2
:
HO2 = 0.66 in Beijing.33 Stevens et al. report RO2
:
HO2 > 1 higher (around 15 for pristine conditions and 4 for slightly polluted conditions) at a remote site in Colorado, but still much lower than what we see in the model when no OVOCs are present.41 Modeling done under low NOx conditions for the marine atmosphere40 and in rural Alabama42 has also reported 1 < RO2
:
HO2 < 5, also much lower than our modeled RO2
:
HO2 without OVOCs.
Under chamber conditions with no or very low OVOC concentrations, OH reactivity is low because its main reaction is with the hydrocarbon of interest (for example α-pinene). Since the chamber experiments we will consider here are designed to mimic the remote atmosphere, we are especially interested in atmospheric OH reactivity measurements under those conditions. Ferracci et al.43 report measured OH reactivity from a suite of field campaigns across the world including many remote areas that span a range from 4 s−1 to almost 50 s−1, the average being around 15 s−1. Measurements of OH reactivity from the boreal forest site in Hyytiälä, Finland are also consistent with these values.44,45 In a remote forest in the Great Lakes Region in northern Michigan, di Carlo et al.46 estimate that only about 2% of a total OH reactivity of approximately 13 s−1 is attributable to monoterpenes.
A further complication is that in ozonolysis chamber experiments, the alkene + ozone reaction governs the overall radical production. This is rarely if ever true during daytime in the real atmosphere, where photolytic sources of HOx are substantial.47 Most alkenes also react with OH on almost every collision, whereas the background atmosphere has a richer distribution of OH reactivity. For all of these reasons, adding an OVOC surrogate such as CO to a chamber experiment is a potentially viable method to mimic the influence of OVOCs on the radical balance, but measures such as OH reactivity (and branching) as well as the actual RO2
:
HO2 and not simply reproducing ambient CO may better meet the experimental design objective.
This has significance when considering nucleation. Current knowledge suggests that ULVOC dimers are the species responsible for pure biogenic nucleation and lower atmospheric RO2 to HO2 ratios may help explain why pure biogenic nucleation occurs in chamber experiments, but not always under seemingly similar atmospheric conditions.
Additionally, because the autoxidation pathway (and thus HOM production) is competitive with bimolecular termination reactions, it is strongly dependent on the conditions of the experiment or the atmosphere, including both the absolute radical concentrations as well as the radical ratios. Here we focus on the termination pathway of peroxy radicals with HO2 in order to show conditions under which this pathway becomes critical that are common in the atmosphere, but may not be represented in chamber experiments. However, the actual atmosphere rarely fits into what we here consider our more atmospherically relevant regime. This is because most often all of the potential peroxy-radical reactant pathways are available and competitive in the actual atmosphere (including reaction of acyl peroxy radicals with NO2 to for peroxy acyl nitrates48 – which we omit for simplicity here). This speaks to how important it is for mechanistic representations of the atmosphere to be built based on experiments under which conditions are well-known so their extrapolation to more atmospheric conditions can be evaluated.
The major issue is this. If RO2 cross reactions are relatively slow (slower than RO2 + HO2), then chambers with unrealistically high RO2
:
HO2 will misrepresent the atmosphere and possibly overproduce dimers and other ELVOC products. However, if RO2 cross reactions are fast (potentially 30 times faster than RO2 + HO2), then they can still dominate termination for RO2
:
HO2 ≃ 1. Under those conditions, RO2
:
HO2 is less of a concern and chamber ULVOC yields, as well as nucleation and growth rates, are more likely to be directly relevant to the atmosphere. As it happens, a diversity of cross reactions may span these conditions, and reality likely lies somewhere in the middle. If this is so, it is especially important to gain a molecular level understanding to accurately predict atmospheric behavior.
2 The model
Our objective is to model the effect of OVOCs on RO2
:
HO2 and the associated oxidation products of a VOC such as α-pinene. We are specifically interested in whether typical chamber conditions result in unrealistic net yields of the lowest volatility products such as the ROOR dimers (some of which are “Ultra Low Volatility Organic Compounds”, ULVOCs, in the volatility basis set classification28). High RO2
:
HO2 might even promote an unrealistic extent of autoxidation due to insufficient HO2 termination and lead to elevated yields of “Extremely Low Volatility Organic Compounds”, ELVOCs (which also include many less oxidized dimers). This could cause chamber experiments to show either higher nucleation rates (driven by ULVOCs) or higher sub 3 nm growth rates (driven by ELVOCs)13 than would occur in the atmosphere, leading to an overall high bias in new-particle formation rates.
Any model sensitivity to chamber conditions (specifically RO2
:
HO2 and RO2 kinetics) would also suggest a corresponding sensitivity in the real atmosphere. It is possible that the real world (especially under low-NOx conditions) is very sensitive to these kinetics, making this an important topic for future research.
To simulate OVOCs in the atmosphere we will simulate adding CO (also a potentially effective experimental technique) and explore the sensitivity of RO2
:
HO2 and thus the ULVOC and ELVOC yields to CO levels. Because both the autoxidation and RO2 (cross) termination kinetics are uncertain, we shall explore a phase space spanning potential rate coefficients.
2.1 RO2 cross reactions and dimerization
We represent variability in RO2 cross reaction kinetics following the scheme first suggested by Madronich and Calvert.39 The rate coefficient for any RO2 cross reaction is parameterized as the geometric mean of the self-reaction rate coefficients (multiplied by 2 because of the difference in symmetry) defined for a sequence of RO2 ranging from slow tertiary-RO2 to fast hydroxy-RO2. We augment this by defining self-reaction rate coefficients for OxnRO2 representing 1, 2, and 3 steps of autoxidation, assuming that this will generally introduce electron withdrawing functional groups near the peroxy radical moiety. Here we investigate different scenarios of rate coefficients by changing the rate coefficient associated with Ox1RO2, Ox2RO2 and Ox3RO2, but leaving the RO2 and Ox0RO2 the same in every scenario. The RO2 and the Ox1RO2 rate coefficients are slow so that in every scenario, enough autoxidation occurs to allow the opportunity for dimers from the Ox2RO2 and the Ox3RO2 to form (consistent with observations).
We simulate 3 cases for the Ox1,2,3RO2 cross reactions: a “fast” case, “middle” case, and a “slow” case that are described in Table 1. The rate coefficients range from 10−13 cm3 mol−1 s−1 to 10−10 cm3 mol−1 s−1, which reflects the range of measured rate coefficients as well as the rate coefficients used in the other modeling studies.3,4,30 The fast case here is the same case used in Schervish and Donahue.28 Even in the fast case, the rate coefficients associated with RO2, Ox0RO2, and Ox1RO2 are kept slow so that they don't dominate over autoxidation preventing any Ox2RO2 and Ox3RO2 from forming.
Table 1 Self reaction rate coefficients (cm3 mol−1 s−1) for the three RO2 reactivity cases investigated here
Reaction |
Fast k |
Middle k |
Slow k |
k(RO2) |
10−13 |
10−13 |
10−13 |
k(Ox0RO2) |
10−13 |
10−13 |
10−13 |
k(Ox1RO2) |
10−12 |
10−13 |
10−13 |
k(Ox2RO2) |
10−11 |
10−12 |
10−13 |
k(Ox3RO2) |
10−10 |
10−12 |
10−13 |
2.2 Differences from previous implementation
Our model is described in detail in Schervish and Donahue,28 however, we provide a brief description here and discuss some changes in the following sections. The model simulates the gas-phase chemistry, culminating in formation (and loss) of RO2 species, with RO2 termination mapping into the 2D-VBS via a succession of kernels specific to each termination step. The gas-phase chemical mechanism includes small-molecule HOx and α-pinene chemistry. The reactions begin with oxidation of a precursor, in this case α-pinene via dark ozonolysis, producing peroxy radicals which then undergo two types of reactions: autoxidation or radical termination. The rate-limiting step in autoxidation is an internal H-atom transfer, so it is represented as a unimolecular process with a relatively large activation energy and thus a strong temperature dependence, immediately followed by O2 addition. We allow peroxy radicals (OxnRO2) to undergo up to three stages of autoxidation with n being the number of times the peroxy radical has autoxidized from 0 to 3. Each of these peroxy radicals is represented as a point in the 2D-VBS with more autoxidized peroxy radicals being progressively lower in volatility and higher in O
:
C. Here we will look at only cases with no NOx present so the radical termination options available to each peroxy radical are unimolecular termination, reaction with HO2 to form a hydroperoxide, and dimerization.
Once a simulation has reached steady state with a stable production distribution, these products are mapped into the 2D-VBS using kernels anchored to the peroxy radical surrogate (C* and O
:
C) that produced them. These kernels allow us to represent a wide variety of stabilization pathways producing a wide variety of different products through one surrogate species; the variety of species that the surrogate represents are instead mapped to a distribution of products within the 2D-VBS defined by a transformation relative to the surrogate RO2 volatility and O
:
C. Overall, the chemical mechanism will tell us how much, for example, Ox2ROOH (termination with HO2 of a peroxy radical that has gone through two steps of autoxidation) is produced in the simulation and the kernels tell us where in the 2D-VBS to distribute all of that product.
2.2.1 RO2 kinetics.
As in Schervish and Donahue,28 we represent the RO2 cross reactions as a branching between dimer formation and monomer formation following an RO2 association reaction. In that work we modeled the branching ratio based on the RO2 volatility. Here we assume any reaction between two autoxidized peroxy radicals (OxnRO2) will form a dimer 100% of the time. We also assume a dimer branching ratio for reactions between peroxy radicals that have not autoxidized (RO2) to be 0.05. For cross reactions between OxnRO2 and RO2, we assume a branching ratio of 0.5. There is some evidence that the dimerization formation rate (the association rate coefficient times the branching ratio toward dimers) is representing average behavior that is slower than what we see in chamber experiments. This can be seen through measured rate coefficients for dimerization being around 10−13 cm3 mol−1 s−1 at the slowest,49 while we use that as the association rate coefficient, the actually dimerization rate coefficient is slowed down quite a bit for the least autoxidized peroxy radicals by the volatility dependence of the branching ratio. There is also evidence for higher branching toward dimers based on higher dimer yields in experiments under high NOx50 and low temperature31 conditions than we see in the version of the model used in Schervish and Donahue.28
2.2.2 Inclusion of CO.
When none of the OVOCs that promote conversion of OH to HO2 are present, the main mechanism for HOx cycling is through the reactions of HOx with ozone. Under these conditions the conversion of OH to HO2 is slow leading to HO2
:
OH ≃ 2 and an RO2
:
HO2 ≃ 1000. When OVOCs are present we expect to see higher HO2 concentrations and a lower RO2
:
HO2. We add CO as a proxy for all of these OVOCs and add the following reaction allowing another pathway for conversion of OH to HO2. | CO + OH(+O2) → CO2 + HO2 | (1) |
2.2.3 α-Pinene oxidation by OH.
Reflective of more recent work on the products of OH oxidation of α-pinene, we have increased the fraction of peroxy radicals formed from OH reactions that are able to autoxidize to 0.2 from our previous value of 0.1.51,52
2.3 CLOUD CSTR simulation
In our previous study we simulated a chamber as a batch reactor and explored the time evolution of radicals and reaction products for different temperatures and NOx concentrations. Here we simulate a Continuously Stirred Tank Reactor (CSTR, or steady-state chamber) with conditions that closely mimic those of the CERN CLOUD chamber. Specifically, we simulate a CSTR with a flushing timescale of 3 h and a steady inflow of VOC (α-pinene), and ozone. All simulations are at 298 K. The CO concentration is fixed at concentrations between 0 and 10
000 ppb. For all of the results discussed below we allow the system to run to a steady state (≃5 h after starting reagent inflow) and discuss only those steady-state values. The model parameters are in Table 2.
Table 2 Model parameters to simulate typical α-pinene + ozone CLOUD experiment
Parameter |
Value |
ϕ
α-pinene
|
0.0018 ppb s−1 |
ϕ
O3
|
0.072 ppb s−1 |
k
flush
|
9.26 × 10−5 s−1 (τ = 3 h) |
k
wall
|
1.70 × 10−3 s−1 (τ = 10 min) |
In chambers with fairly low aerosol loading (such as the CLOUD chamber), the major loss of condensible vapors is (presumably irreversible) deposition to the chamber walls. Under typical fan conditions in CLOUD, the H2SO4 deposition timescale is roughly 4 minutes.53 The organic vapors are heavier, with correspondingly lower diffusion constants, and so have deposition timescales of order 10 min.54,55 This correspondence of vapor deposition timescales between CLOUD and remote ambient conditions is a design feature, though caution is required because turbulent deposition to the chamber walls scales as
whereas laminar condensation to particles scales as D (or molecular speed in the kinetic regime).56,57 In order to compare modeled ULVOC collision frequencies and modeled size-dependent growth rates with chamber data, we therefore add a wall-loss term to our simulation of the CSTR. Here that is 0.0017 s−1 (a 10 min lifetime).
Here we simulate a dark ozonolysis experiment driven by the α-pinene + ozone reaction. Unlike the atmosphere, where photochemical HOx production is a major radical source during the day, in chamber experiments such as this the “heartbeat” of the chemistry – the intrinsic rate – is controlled by the ozonolysis rate. Alkene ozonolysis produces OH, and here we assume an OH yield of 0.8.58,59 The critical OH branching shown in Fig. 1 reduces to a competition between α-pinene (kOH ≃ 5.4 × 10−11 cm3 mol−1 s−1) and CO (kOH ≃ 2.4 × 10−13 cm3 mol−1 s−1). Thus we expect that CO will need to exceed α-pinene by a factor of approximately 500 for RO2
:
HO2 to approach 1.
3 Results
3.1 CO concentration and OH reactivity
Fig. 2 shows radical levels and RO2
:
HO2vs. CO concentration, which are essentially identical for the three different RO2 reactivity scenarios (the figure shows the middle case). RO2
:
HO2 is inversely proportional to CO for much of the range (log–log slope = −1) and ultimately approaches a value near 2. For low CO it exceeds 100; these very large values are partly a consequence of the simplified α-pinene mechanism that resolves RO2 products into the 2D-VBS instead of treating subsequent RO chemistry explicitly. The ratio is slightly lower when RO2 reactions are faster because the sink for RO2via the cross reactions increases, leading to much lower steady-state RO2 concentrations, but only slightly lower HO2 concentrations. The model shows that very high CO concentrations are required to reach atmospherically relevant RO2
:
HO2, but as CO is acting as a proxy for any compound whose oxidation would promote the conversion of OH to HO2, these high concentrations merely represent what is necessary to reach that conversion rate. Also, at these high CO levels, the absolute RO2 and HO2 concentrations of 2–5 × 108 mole cm−3 are reasonable for low-NO atmospheric conditions, suggesting that the termination chemistry will be appropriate. However, the OH has been almost completely scavenged by CO, and so multi-generation oxidation (aging) by OH will be negligible. This suggests that experiments conducted under these (high CO) conditions would likely provide a good representation of first-generation atmospheric chemistry but miss any multi-generation chemistry important to the atmosphere. This is both a feature and a limitation.
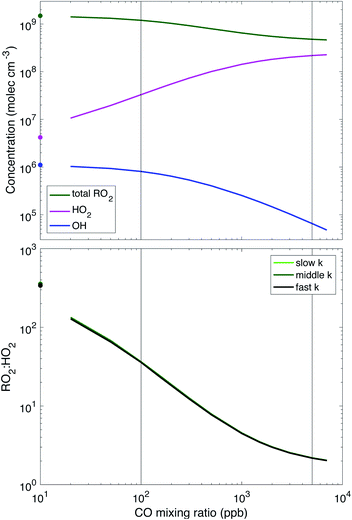 |
| Fig. 2 Key radical levels vs. CO concentration in dark α-pinene ozonolysis. Top: concentrations of total RO2, HO2, and OH. Bottom: RO2 : HO2. All RO2 cross reaction scenarios give nearly identical results. Symbols on the y-axis indicate the values without CO. Added CO suppresses OH and enhances HO2 while slightly reducing RO2 (because HO2 decreases the RO2 lifetime). The vertical lines are at CO concentrations of 100 ppbv and 5000 ppbv. Values of RO2 : HO2 below 10 (roughly the largest RO2 : HO2 observed in the atmosphere) require CO ≳ 1000 ppb. | |
Fig. 3 shows both the total OH reactivity and the fraction of OH reacting with the parent hydrocarbon (α-pinene) vs. added CO in this simulated chamber experiment. Looking at OH reactivity we can see that when CO is absent, the OH reactivity is very low (around 0.5 s−1), much lower than reported OH reactivity in the remote atmosphere. About 1000–3000 ppb of CO is required to reproduce an OH reactivity of 10–15 s−1 typical of measurements in the remote atmosphere. In this range, the fraction of this reactivity that is due to α-pinene, the only primary hydrocarbon in the model, is less than 10%. While primary hydrocarbons can account for up to 50% of the total OH reactivity in the atmosphere, according to di Carlo et al.46 much of this is due to more abundant hydrocarbons like isoprene and a much lower fraction when only α-pinene is present at a concentration of approximately 800 ppt, a lower percentage is completely reasonable. In this simulated experiment, especially because the governing α-pinene + ozone reaction automatically produces RO2 with a stoichiometry of 1, only a low fraction of OH reactivity can go to the α-pinene to mimic the reaction topology shown in Fig. 1. This again indicates added CO of several ppm.
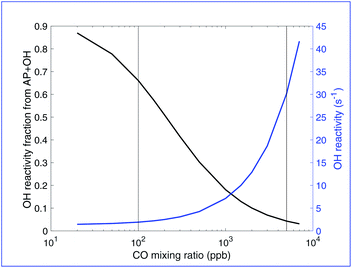 |
| Fig. 3 OH reactivity vs. CO. The left y-axis and black curve show the fraction due to the α-pinene + OH reaction and the right y-axis and blue curve show the absolute reactivity. At low CO, OH reactivity is low and over 80% is attributable to the α-pinene + OH reaction. As CO increases, the OH reactivity increases and the fraction due to α-pinene decreases. OH reactivity in the remote atmosphere can range from 4–50 s−1, but 10–15 s−1 is typical, with about 2% of the reactivity attributable to α-pinene. In this plot that occurs in the range of 1000–3000 ppb of CO. The vertical lines at 100 ppb and 5000 ppb are the conditions of the steady-state VBS distributions in Fig. 4 and 5. | |
The vertical lines in Fig. 2 and 3 for CO = 100 and 5000 ppbv represent two cases we explore in greater depth below, along with the 0 CO case indicated with symbols on the y-axes. Relatively low CO between 0 and 100 ppbv is likely to represent typical conditions in chamber experiments without intentionally added CO (but instead just what is present in the balance air). The higher CO case, as shown, leads to OH reactivity and RO2
:
HO2 that is more consistent with typical atmospheric values.
3.2 Dimers and ULVOCs
3.2.1 Volatility basis set distributions.
Fig. 4 shows distributions of products for the three RO2 rate coefficient cases at three different CO mixing ratios as a 1-dimensional volatility basis set. The various volatility classes described above are shown in the color-coded horizontal bar across the top of the figure. The vertical bars are color coded by the RO2 termination pathway, as indicated in the legend. Each row shows a different RO2 reactivity case – fast on top, middle in the middle, and slow on the bottom – and each column is a different steady-state CO concentration. The two non-zero CO concentrations are shown as vertical dashed lines in Fig. 2; as such the two left-hand columns represent a potential range for “unmodified” chambers with no-to-low CO in the carrier gas, while the right-hand column represents conditions with a more atmospheric RO2
:
HO2. Looking across any row of shows the change in product distributions with increasing CO, once again as a proxy for any OVOC that will promote direct conversion of OH to HO2. For zero CO (and thus low HO2), most of the RO2 that does not undergo autoxidation terminates to molecules via a simplified pathway shown in light green labeled “non-HOM products”. In every case, the product distributions are different at different CO concentrations. Most notably, as CO increases for every case there is a marked increase in the products from the RO2 + HO2 reaction, shown in pink, and a decrease in the dimers, shown in crimson. When we look down a column, we see the effect of slowing down the RO2 kinetics has on the product distributions. Unsurprisingly we see a decrease in dimers as those reactions are slowed.
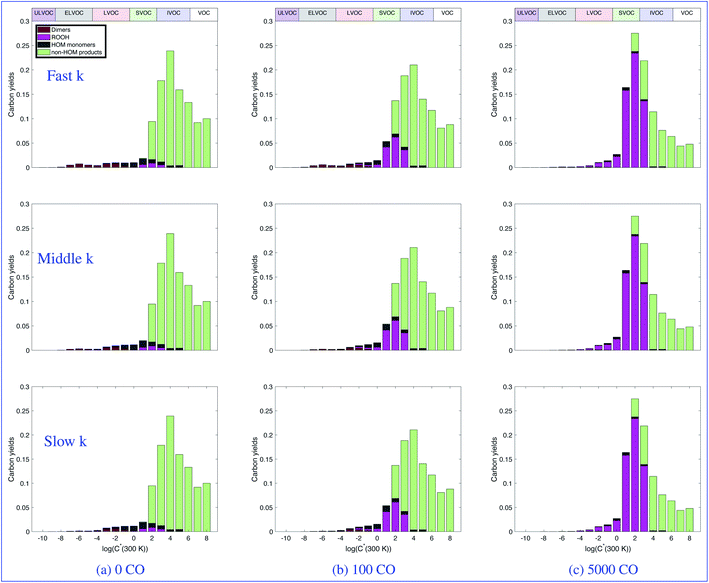 |
| Fig. 4 Volatility basis set distributions of the products from α-pinene ozonolysis (including autoxidation and dimerization) at different CO mixing ratios for three different peroxy-radical reactivity scenarios. Each row shows a different reactivity case: the top row is the fast case, the middle row is the middle case, and the bottom row is the slow case. Each column shows a different CO mixing ratio in ppb, as indicated. Bar colors show the RO2 termination pathway, while different volatility classes are indicated via the horizontal bars along the top. CO enhances HO2 and tends to replace dimers (crimson) with hydroperoxides (magenta), reducing the yields of the lowest volatility products. | |
Notably, the “traditional” α-pinene oxidation products (light green) predominate in all cases. Modifying RO2
:
HO2 changes the distribution across the board, but the yield changes in the SVOC region and above are modest, with some redistribution of products toward hydroperoxides (dark magenta) overall. Consequently, chamber experiments focused on mass yields in the 1–1000 μg m−3 would see modest changes in overall mass yields with increased hydroperoxide content as RO2
:
HO2 decreases. This is consistent with observations.60,61 Further, the very low yields of the entire “xLVOC” ensemble is notable and the variability with RO2
:
HO2 serves to emphasize that this tail is highly sensitive to conditions. This serves as a cautionary tale on the one hand but also suggests that corresponding yields in the real atmosphere are likely also quite sensitive to conditions, and that this variability might well be important to atmospheric behavior.
Fig. 5 focuses on the lower volatility products. Here we can more clearly see the suppression of dimers both by increasing CO as well as by decreasing the RO2 rate coefficient. We can also very clearly see the effect on the ULVOCs. The only products that fall in the ULVOC range are the dimers formed from the self reaction of two Ox3RO2 radicals and a small fraction of the cross reaction between an Ox3RO2 and an Ox2RO2. Therefore it makes sense that the ULVOCs follow the same trend as the dimers in general. Looking across the top row (the fast case), we see low, but visible yields of ULVOCs with very low CO, but at the highest CO mixing ratio shown (representing more ambient RO2
:
HO2), the yield is approximately halved. In the middle case, the RO2 kinetics are still fast enough to sustain visible ULVOC yields at low CO, but the influence of CO is much more pronounced at 100 ppb of CO and there are almost no ULVOCs present at 5000 ppb of CO. In the slow case, while the dimer trend is still noticeable, the ULVOC yields are notably lower even at low CO, so there is little visible influence of CO on the ULVOC yields. As nucleation is dependent on the amount of ULVOCs, we expect to see different nucleation rates at each of the different CO mixing ratios and for each of the RO2 reactivity scenarios.
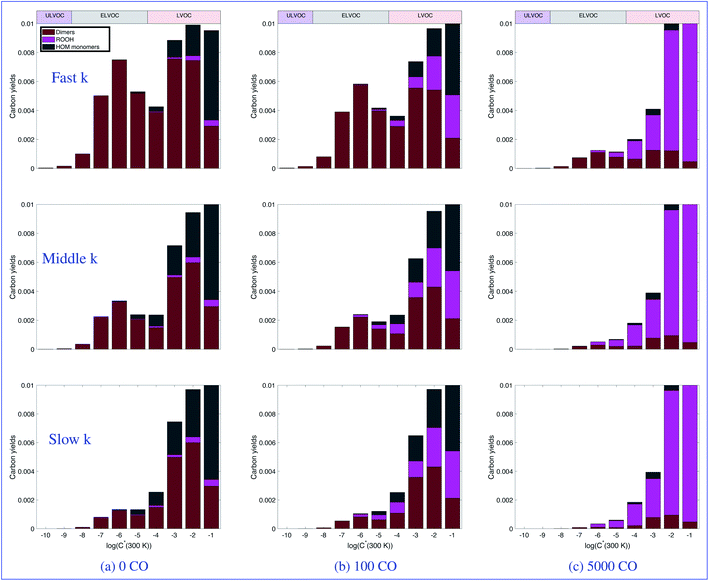 |
| Fig. 5 Volatility basis set distributions of the products from α-pinene ozonolysis (including autoxidation and dimerization) at different CO mixing ratios for three different peroxy-radical reactivity scenarios, focusing on the lowest volatility products. Each row shows a different reactivity case: the top row is the fast case, the middle row is the middle case, and the bottom row is the slow case. Each column shows a different CO mixing ratio in ppb, as indicated. Bar colors show the RO2 termination pathway, while different volatility classes are indicated via the horizontal bars along the top. The suppression of dimer formation by HO2 (promoted by CO) is clearly evident. | |
3.2.2 Overall yield sensitivity.
Fig. 6 shows the total dimers for each of the RO2 reactivity cases as well as the total oxidized dimers (total dimers minus the non-HOM dimers, formed from the reaction of peroxy radicals that do not undergo autoxidation) over a wide range of CO mixing ratios. The total dimer formation for the fast case is a factor of 7–8 lower at very high CO compared to low CO; most of the dimers in this case are HOM dimers. In the other cases, the dimers are reduced by about an order of magnitude with added CO. Broadly, adding CO and thus decreasing RO2
:
HO2 from an unrealistic to a more atmospherically relevant value can decrease the total dimer concentrations (yields) by almost an order of magnitude, regardless of the RO2 reactivity case considered. The total dimer concentrations are however also sensitive to the RO2 reactivity as well.
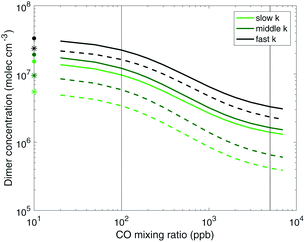 |
| Fig. 6 Total steady-state dimer concentrations vs. CO from α-pinene ozonolysis simulation. The dashed lines show dimers formed from RO2 that have undergone at least one step of autoxidation. Adding CO to match atmospheric RO2 : HO2 (the second vertical line) sharply reduces dimer concentrations in all cases. This shows that dimer yields are likely to be highly sensitive to RO2 : HO2 over the range of values found in chambers and the real atmosphere, and that chamber dimer yields should be applied to atmospheric simulations with caution. | |
Total dimers are not a sufficiently precise measure of the actual situation; Fig. 5 shows that the dimers are distributed over almost 10 orders of magnitude in volatility. Thus, in Fig. 7 we show the relative change of each dimer class normalized by the zero CO case, where the dimer class is the total autoxidation number (i.e. Ox = 6 is a dimer produced from Ox3RO2 + Ox3RO2). We use a linear y-axis scale to emphasize the strength of these effects. Looking at the suppression of dimers due to CO (actually HO2), we see in the fast case, the oxidized dimers are suppressed far less at low CO mixing ratios when their cross reactions are fast. The dimers in the middle and slow cases are also consistently suppressed by CO, with the most oxidized dimers being suppressed slightly more aggressively by CO when their rate coefficient is slower. The concentration of the most oxidized dimers in the slow and middle cases is comparable to the fast case when no CO is present, but they are suppressed more aggressively by HO2 in the slow and middle cases, creating situations where we might measure appreciable dimers under low CO chamber conditions, but not under atmospheric conditions with lower RO2
:
HO2.
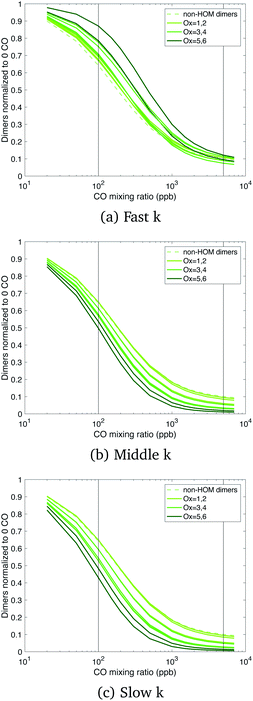 |
| Fig. 7 Dimer levels vs. CO (normalized to zero CO) from α-pinene ozonolysis for different RO2 reactivity cases. CO suppresses dimer formation by enhancing HO2 concentrations and thus lowering RO2 : HO2. The effect of increasing CO is greatest on the slowest forming dimers and is very different (and less extreme) in the fast RO2 reactivity case, when the peroxy radical reactions with each other are faster than their reactions with HO2. | |
3.2.3 Nucleation.
Dimers are critical to nucleation, but not all dimers can contribute to nucleation as even some HOM dimers only reach the LVOC or ELVOC classes. Schervish and Donahue28 proposed the ULVOC volatility class (log
C* < −8.5) based on evidence that compounds in this range are highly associated with observed “pure organic” nucleation. Cluster formation must be related to the collision rate of these ULVOC species, and so we can compare modeled ULVOC collision rates with measured nucleation rates. To calculate the ULVOC collision rate we assume collision rate coefficient of kcoll = 3 × 10−10 cm3 mol−1 s−1, which we then multiply by the ULVOC concentration squared. In Fig. 8 we show the collision frequency of ULVOCs for the representative RO2 kinetic cases as a function of added CO (thus RO2
:
HO2).
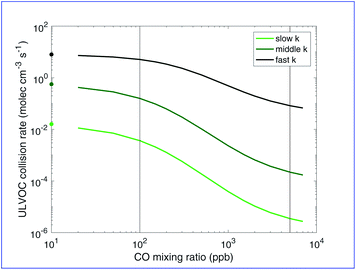 |
| Fig. 8 ULVOC collision rates vs. CO for different peroxy radical reactivity cases following α-pinene ozonolysis in a steady-state chamber such as the CLOUD chamber. This is the maximum likely nucleation rate. Symbols on the y-axis show the ULVOC collision rate at zero CO. For rapid RO2 reactions the ULVOC collision rate (and thus nucleation) is only weakly sensitive to CO (and thus RO2 : HO2). However, for the slower RO2 reactivity cases the nucleation rate is highly sensitive to CO, suggesting that control of RO2 branching is important in chamber experiments. | |
4 Discussion
It is not news that low-NOx chamber experiments can deviate from atmospheric conditions by favoring RO2 cross reactions over HO2 reactions. However, the recent emergence of autoxidation and the potential for very rapid RO2 cross reactions involving highly oxidized RO2 radicals substantially complicates this issue. Further, new-particle formation appears to be especially sensitive to the covalently bound dimers formed from those cross reactions, because the high molecular weight combined with significant polar functionality can drive the products into the Ultra Low Volatility Organic Carbon (ULVOC) class. Our simulations show that yields of these dimers are sensitive to RO2
:
HO2 over the range of conditions likely to be found in chambers and in the atmosphere, and also that they are sensitive to a plausible range of RO2 cross reaction rate coefficients, which are currently not well constrained. The simulations also suggest that manipulating (and measuring) RO2
:
HO2 is chamber experiments is important. However, these dimers are also clearly visible in ambient measurements,62 and so complete elimination of dimer formation under ambient conditions is unlikely.
Our results are generally consistent with the results from McFiggans et al.63 McFiggans et al.63 show that both monomer and dimers are drastically reduced in the presence of small molecules that compete for RO2, including due to the increase in HO2 caused by increasing CO. However, our simulation and the experiments presented in McFiggans et al.63 differ significantly, making a direct comparison difficult. Specifically, we simulate dark ozonolysis with some OH chemistry at low CO, whereas OH is the primary oxidant in McFiggans et al.;63 this different chemistry could cause the products to be different enough to lead to different volatility distributions when compared with our dark ozonolysis simulation. Notably, McFiggans et al.63 see a decrease in SOA yields (with organic aerosol concentrations of 1–10 μg m−3) in the α-pinene + CO experiments while our simulation shows an increase in the ROOH yields in the SVOC range.
We have maintained the same reaction kernels from the original work in Schervish and Donahue;28 we are focused on the dimer and ULVOC products. The major take-home message of our work is that we must consider the radical termination reactions carefully before jumping to extend results to the atmosphere, and so we elect not to “tune” the termination kernels to observed yields here. As all of the chemistry is resolved before the kernels are implemented to distribute the products in the VBS, the RO2 + HO2 kernel does not affect any other products. One attempt to “tune” radical-VBS dimer yields to match both chamber and flow-tube dimer yields has been applied to a chemical transport model simulation of nucleation over the Amazon; there Zhao et al.64 required a mixture of “high” and “low” autoxidation barrier OxRO2 in order to match experimental dimer yields. McFiggans et al.63 also provide gas-phase mass spectrometry data that show large decreases in the α-pinene produced monomers and dimers. Our dimer results are consistent with this, but we see little to no change in the LVOC region where HOM monomers dominate. This discrepancy may be due to the limitations (specificity) of the nitrate chemical ionization source, which clusters less efficiently with less oxygenated and thus higher volatility products.12,13 However, our focus here is on nucleation and especially dimer formation. In this our model is consistent with the results of McFiggans et al.63 where in a mixture including α-pinene and CO, dimers were reduced by a factor of 2 compared to a pure α-pinene case.
We are especially interested in how these parameters affect nucleation, and how this might vary between chambers and the real atmosphere. Fig. 8 shows our modeled ULVOC collision rates are indeed highly sensitive to both RO2
:
HO2 and RO2 reactivity. The conditions simulated here are comparable to conditions in CLOUD where nucleation rates are observed between 0.1 and 10 s−1.15 Our modeled ULVOC collision frequencies at low CO are consistent with ULVOC nucleation efficiency for all RO2 reactivity cases considered, indicating that any of these association rate scenarios would be consistent with experimental findings at low CO mixing ratios. This is sensible as RO2
:
HO2 is high and HO2 termination is minor. However, extending to more atmospheric conditions, the system is very sensitive to added CO. When association is slowed down for the less oxidized peroxy radicals, high concentrations of Ox2RO2 and Ox3RO2 can be achieved and produce ULVOC dimers. With about 7000 ppb of CO present, the ULVOC collision rate is about 3 orders of magnitude lower than for zero CO in the middle case. The slow case shows similar sensitivity. Overall, Fig. 8 shows that nucleation rates measured in chambers may extend to the real atmosphere, but that this is highly sensitive to the (uncertain) RO2 cross reaction kinetics.
The actual nucleation (stable cluster formation) rate is likely to depend on volatility within the ULVOC class (and also temperature, as the class itself moves with temperature-dependent volatility28). Observations also show that pure biogenic nucleation is highly sensitive to charge, with ions increasing observed new-particle formation rates by up to two orders of magnitude.15 Just as lowered temperature stabilizes clusters, ions can as well; consequently it is plausible that the compounds at the lower end of the ELVOC class in Fig. 5 are more effective in nucleation within charged clusters. Broadly, we expect the nucleation efficiency to be a function of the xLVOC volatility distribution (predominantly ULVOC and ELVOC), with efficiency near 1 for collisions of the lowest volatility species and falling off toward higher volatility, modulated by effects such as charge. Implementation of schemes based on this phenomenology suggest that biogenic nucleation (especially ion induced) may be very important in the present-day tropical atmosphere over the Amazon.64
5 Conclusion
Chamber experiments are often run under conditions leading to very low HO2 concentrations and thus high RO2 to HO2 ratios (RO2
:
HO2) due to the low concentrations of oxidized volatile organic compounds that can convert OH directly to HO2. High RO2
:
HO2 in turn can lead to high concentrations of dimers due to the lack of competition for RO2 from HO2. Depending on the association rate coefficient for forming these dimers, they may or may not form when HO2 concentrations are higher in the real atmosphere. When RO2 + RO2 rate coefficients are fast, dimer concentrations, and the resulting ULVOC dimer yields, stay high at all the CO concentrations (used as a proxy for all oxidized volatile organic compounds), but when RO2 + RO2 rate coefficients are slow, dimers are formed in high concentrations when CO concentrations are low, but fall off quickly as CO increases. Therefore some dimers may be forming under high RO2
:
HO2 in chamber experiments, contributing to nucleation and/or growth that will exceed values in the real atmosphere. However, we also conclude that the system is likely sensitive to these same elements (RO2
:
HO2 as well as the RO2 cross reaction kinetics) in the real atmosphere, thus real-world new-particle formation is likely to be sensitive to these same factors. Thus it is crucial to constrain RO2
:
HO2 and RO2 branching in order to accurately extrapolate their results to the atmosphere. At a minimum it is highly advisable to manipulate RO2
:
HO2 by adding a reagent such as CO (potentially to quite high levels) in order to experimentally explore the chamber sensitivity to these conditions.
Author contributions
MS and NMD conceived the project and designed the overall scope. MS implemented and executed the radical VBS model. MS and NMD wrote the manuscript.
Conflicts of interest
The authors declare no conflicts.
Acknowledgements
This work was supported by Grant AGS1801897 from the U.S. National Science Foundation.
Notes and references
- M. Shrivastava, C. D. Cappa, J. Fan, A. H. Goldstein, A. B. Guenther, J. L. Jimenez, C. Kuang, A. Laskin, S. T. Martin, N. L. Ng, T. Petaja, J. R. Pierce, P. J. Rasch, P. Roldin, J. H. Seinfeld, J. Shilling, J. N. Smith, J. A. Thornton, R. Volkamer, J. Wang, D. R. Worsnop, R. A. Zaveri, A. Zelenyuk and Q. Zhang, Rev. Geophys., 2017, 55, 509–559 CrossRef.
- D. Pagonis and P. J. Ziemann, Aerosol Sci. Technol., 2018, 52, 1178–1193 CrossRef CAS.
- Y. Zhao, J. A. Thornton and H. O. T. Pye, Proc. Natl. Acad. Sci. U. S. A., 2018, 115, 12142–12147 CrossRef CAS.
- T. Berndt, W. Scholz, B. Mentler, L. Fischer, H. Herrmann, M. Kulmala and A. Hansel, Angew. Chem., Int. Ed., 2018, 57, 3820–3824 CrossRef CAS.
- Z. Peng and J. L. Jimenez, Chem. Soc. Rev., 2020, 49, 2570–2616 RSC.
- F. D. Lopez-Hilfiker, C. Mohr, M. Ehn, F. Rubach, E. Kleist, J. Wildt, T. F. Mentel, A. Lutz, M. Hallquist, D. Worsnop and J. A. Thornton, Atmos. Meas. Tech., 2014, 7, 983–1001 CrossRef.
- T. Jokinen, M. Sipilä, H. Junninen, M. Ehn, G. Lönn, J. Hakala, T. Petäjä, R. L. Mauldin III, M. Kulmala and D. R. Worsnop, Atmos. Chem. Phys., 2012, 12, 4117–4125 CrossRef CAS.
- J. Kontkanen, K. Lehtipalo, L. Ahonen, J. Kangasluoma, H. E. Manninen, J. Hakala, C. Rose, K. Sellegri, S. Xiao, L. Wang, X. Qi, W. Nie, A. Ding, H. Yu, S. Lee, V.-M. Kerminen, T. Petäjä and M. Kulmala, Atmos. Chem. Phys., 2017, 17, 2163–2187 CrossRef CAS.
- J. D. Crounse, L. B. Nielsen, S. Jørgensen, H. G. Kjaergaard and P. O. Wennberg, J. Phys. Chem. Lett., 2013, 4, 3513–3520 CrossRef CAS.
- M. Ehn, J. A. Thornton, E. Kleist, M. Sipila, H. Junninen, I. Pullinen, M. Springer, F. Rubach, R. Tillmann, B. Lee, F. Lopez-Hilfiker, S. Andres, I.-H. Acir, M. Rissanen, T. Jokinen, S. Schobesberger, J. Kangasluoma, J. Kontkanen, T. Nieminen, T. Kurten, L. B. Nielsen, S. Jorgensen, H. G. Kjaergaard, M. Canagaratna, M. D. Maso, T. Berndt, T. Petaja, A. Wahner, V.-M. Kerminen, M. Kulmala, D. R. Worsnop, J. Wildt and T. F. Mentel, Nature, 2014, 506, 476–479 CrossRef CAS.
- F. Bianchi, T. Kurtén, M. Riva, C. Mohr, M. Rissanen, R. Pontus, T. Berndt, J. Crounse, P. Wennberg, T. F. Mentel, J. Wildt, H. Junninen, T. Jokinen, M. Kulmala, D. Worsnop, J. Thornton, N. M. Donahue, H. G. Kjaergaard and M. Ehn, Chem. Rev., 2019, 119, 3472–3509 CrossRef CAS.
- J. Tröstl, W. K. Chuang, M. Heinritzi, C. Yan, U. Molteni, L. Ahlm, C. Frege, F. Bianchi, R. Wagner, M. Simon, K. Lehtipalo, C. Williamson, J. S. Craven, J. Duplissy, A. Adamov, J. Almeida, A.-K. Bernhammer, M. Breitenlechner, S. Brilke, A. Dias, S. Ehrhart, R. C. Flagan, A. Franchin, C. Fuchs, H. Gordon, R. Guida, M. Gysel, A. Hansel, C. R. Hoyle, T. Jokinen, H. Junninen, J. Kangasluoma, H. Keskinen, J. Kim, M. Krapf, A. Kürten, A. Laaksonen, M. Lawler, M. Leiminger, S. Mathot, O. Möhler, T. Nieminen, A. Onnela, T. Petäjä, F. M. Piel, P. Miettinen, M. P. Rissanen, L. Rondo, N. Sarnela, S. Schobesberger, K. Sengupta, M. Sipilä, J. N. Smith, G. Steiner, A. Tomè, A. Virtanen, A. C. Wagner, E. Weingartner, D. Wimmer, P. M. Winkler, P. Ye, K. S. Carslaw, J. Curtius, J. Dommen, J. Kirkby, M. Kulmala, I. Riipinen, D. R. Worsnop, N. M. Donahue and U. Baltensperger, Nature, 2016, 530, 527–531 CrossRef.
- D. Stolzenburg, L. Fischer, A. L. Vogel, M. Heinritzi, M. Schervish, M. Simon, A. C. Wagner, L. Dada, L. R. Ahonen, A. Amorim, A. Baccarini, P. S. Bauer, B. Baumgartner, A. Bergen, F. Bianchi, M. Breitenlechner, S. Brilke, S. Buenrostro Mazon, D. Chen, A. Dias, D. C. Draper, J. Duplissy, I. El Haddad, H. Finkenzeller, C. Frege, C. Fuchs, O. Garmash, H. Gordon, X. He, J. Helm, V. Hofbauer, C. R. Hoyle, C. Kim, J. Kirkby, J. Kontkanen, A. Kürten, J. Lampilahti, M. Lawler, K. Lehtipalo, M. Leiminger, H. Mai, S. Mathot, B. Mentler, U. Molteni, W. Nie, T. Nieminen, J. B. Nowak, A. Ojdanic, A. Onnela, M. Passananti, T. Petäjä, L. L. J. Quéléver, M. P. Rissanen, N. Sarnela, S. Schallhart, C. Tauber, A. Tomé, R. Wagner, M. Wang, L. Weitz, D. Wimmer, M. Xiao, C. Yan, P. Ye, Q. Zha, U. Baltensperger, J. Curtius, J. Dommen, R. C. Flagan, M. Kulmala, J. N. Smith, D. R. Worsnop, A. Hansel, N. M. Donahue and P. M. Winkler, Proc. Natl. Acad. Sci. U. S. A., 2018, 115, 9122–9127 CrossRef CAS.
- F. Riccobono, S. Schobesberger, C. E. Scott, J. Dommen, I. K. Ortega, L. Rondo, J. Almeida, A. Amorim, F. Bianchi, M. Breitenlechner, A. David, A. Downard, E. Dunne, J. Duplissy, S. Ehrhart, R. C. Flagan, A. Franchin, A. Hansel, H. Junninen, M. Kajos, H. Keskinen, A. Kupc, O. Kupiainen, A. Kürten, T. Kurtén, A. N. Kvashin, A. Laaksonen, K. Lehtipalo, V. Makhmutov, S. Mathot, T. Nieminen, T. Olenius, A. Onnela, T. Petäjä, A. P. Praplan, F. D. Santos, S. Schallhart, J. H. Seinfeld, M. Sipilä, D. V. Spracklen, Y. Stozhkov, F. Stratmann, A. Tomé, G. Tsagkogeorgas, P. Vaattovaara, H. Vehkamäki, Y. Viisanen, A. Vrtala, P. E. Wagner, E. Weingartner, H. Wex, D. Wimmer, K. S. Carslaw, J. Curtius, N. M. Donahue, J. Kirkby, M. Kulmala, D. R. Worsnop and U. Baltensperger, Science, 2014, 344, 717–721 CrossRef CAS.
- J. Kirkby, J. Duplissy, K. Sengupta, C. Frege, H. Gordon, C. Williamson, M. Heinritzi, M. Simon, C. Yan, J. Almeida, J. Tröstl, T. Nieminen, I. K. Ortega, R. Wagner, A. Adamov, A. Amorim, A.-K. Bernhammer, F. Bianchi, M. Breitenlechner, S. Brilke, X. Chen, J. Craven, A. Dias, S. Ehrhart, R. C. Flagan, A. Franchin, C. Fuchs, R. Guida, J. Hakala, C. R. Hoyle, T. Jokinen, H. Junninen, J. Kangasluoma, J. Kim, M. Krapf, A. Kürten, A. Laaksonen, K. Lehtipalo, V. Makhmutov, S. Mathot, U. Molteni, A. Onnela, O. Peräkylä, F. Piel, T. Petäjä, A. P. Praplan, K. Pringle, A. Rap, N. A. Richards, I. Riipinen, M. P. Rissanen, L. Rondo, N. Sarnela, S. Schobesberger, C. E. Scott, J. H. Seinfeld, M. Sipilä, G. Steiner, Y. Stozhkov, F. Stratmann, A. Tomé, A. Virtanen, A. L. Vogel, A. Wagner, P. E. Wagner, E. Weingartner, D. Wimmer, P. M. Winkler, P. Ye, X. Zhang, A. Hansel, J. Dommen, N. M. Donahue, D. R. Worsnop, U. Baltensperger, M. Kulmala, K. S. Carslaw and J. Curtius, Nature, 2016, 530, 521–526 CrossRef.
- J. R. Pierce and P. J. Adams, Atmos. Chem. Phys., 2009, 9, 1339–1356 CrossRef CAS.
- J. Merikanto, D. V. Spracklen, G. W. Mann, S. J. Pickering and K. S. Carslaw, Atmos. Chem. Phys., 2009, 9, 8601–8616 CrossRef CAS.
- D. V. Spracklen, K. S. Carslaw, M. Kulmala, V.-M. Kerminen, S.-L. Sihto, I. Riipinen, J. Merikanto, G. W. Mann, M. P. Chipperfield, A. Wiedensohler, W. Birmili and H. Lihavainen, Geophys. Res. Lett., 2008, 35, L06808 Search PubMed.
- M. Wang and J. E. Penner, Atmos. Chem. Phys., 2009, 9, 239–260 CrossRef CAS.
- J. Kazil, P. Stier, K. Zhang, J. Quaas, S. Kinne, D. O'Donnell, S. Rast, M. Esch, S. Ferrachat, U. Lohmann and J. Feichter, Atmos. Chem. Phys., 2010, 10, 10733–10752 CrossRef CAS.
- H. Gordon, K. Sengupta, A. Rap, J. Duplissy, C. Frege, C. Williamson, M. Heinritzi, M. Simon, C. Yan, J. Almeida, J. Tröstl, T. Nieminen, I. K. Ortega, R. Wagner, E. M. Dunne, A. Adamov, A. Amorim, A.-K. Bernhammer, F. Bianchi, M. Breitenlechner, S. Brilke, X. Chen, J. S. Craven, A. Dias, S. Ehrhart, L. Fischer, R. C. Flagan, A. Franchin, C. Fuchs, R. Guida, J. Hakala, C. R. Hoyle, T. Jokinen, H. Junninen, J. Kangasluoma, J. Kim, J. Kirkby, M. Krapf, A. Kürten, A. Laaksonen, K. Lehtipalo, V. Makhmutov, S. Mathot, U. Molteni, S. A. Monks, A. Onnela, O. Peräkylä, F. Piel, T. Petäjä, A. P. Praplan, K. J. Pringle, N. A. D. Richards, M. P. Rissanen, L. Rondo, N. Sarnela, S. Schobesberger, C. E. Scott, J. H. Seinfeld, S. Sharma, M. Sipilä, G. Steiner, Y. Stozhkov, F. Stratmann, A. Tomé, A. Virtanen, A. L. Vogel, A. C. Wagner, P. E. Wagner, E. Weingartner, D. Wimmer, P. M. Winkler, P. Ye, X. Zhang, A. Hansel, J. Dommen, N. M. Donahue, D. R. Worsnop, U. Baltensperger, M. Kulmala, J. Curtius and K. S. Carslaw, Proc. Natl. Acad. Sci. U. S. A., 2016, 113, 12053–12058 CrossRef CAS.
- K. S. Carslaw, H. Gordon, D. S. Hamilton, J. S. Johnson, L. A. Regayre, M. Yoshioka and K. J. Pringle, Curr. Clim. Change Rep., 2017, 3, 1–15 CrossRef.
- A. Guenther, T. Karl, P. Harley, C. Wiedinmyer, P. I. Palmer and C. Geron, Atmos. Chem. Phys., 2006, 6, 3181–3210 CrossRef CAS.
- S. H. Chung and J. H. Seinfeld, J. Geophys. Res.: Atmos., 2002, 107, 4407 CrossRef.
- T. Jokinen, M. Sipilä, S. Richters, V.-M. Kerminen, P. Paasonen, F. Stratmann, D. Worsnop, M. Kulmala, M. Ehn, H. Herrmann and T. Berndt, Angew. Chem., Int. Ed., 2014, 53, 14596–14600 CrossRef CAS.
- M. P. Rissanen, T. Kurtén, M. Sipilä, J. A. Thornton, J. Kangasluoma, N. Sarnela, H. Junninen, S. Jørgensen, S. Schallhart, M. K. Kajos, R. Taipale, M. Springer, T. F. Mentel, T. Ruuskanen, T. Petäjä, D. R. Worsnop, H. G. Kjaergaard and M. Ehn, J. Am. Chem. Soc., 2014, 136, 15596–15606 CrossRef CAS.
- N. M. Donahue, S. A. Epstein, S. N. Pandis and A. L. Robinson, Atmos. Chem. Phys., 2011, 11, 3303–3318 CrossRef CAS.
- M. Schervish and N. M. Donahue, Atmos. Chem. Phys., 2020, 20, 1183–1199 CrossRef CAS.
- C. Frege, I. K. Ortega, M. P. Rissanen, A. P. Praplan, G. Steiner, M. Heinritzi, L. Ahonen, A. Amorim, A.-K. Bernhammer, F. Bianchi, S. Brilke, M. Breitenlechner, L. Dada, A. Dias, J. Duplissy, S. Ehrhart, I. El-Haddad, L. Fischer, C. Fuchs, O. Garmash, M. Gonin, A. Hansel, C. R. Hoyle, T. Jokinen, H. Junninen, J. Kirkby, A. Kürten, K. Lehtipalo, M. Leiminger, R. L. Mauldin, U. Molteni, L. Nichman, T. Petäjä, N. Sarnela, S. Schobesberger, M. Simon, M. Sipilä, D. Stolzenburg, A. Tomé, A. L. Vogel, A. C. Wagner, R. Wagner, M. Xiao, C. Yan, P. Ye, J. Curtius, N. M. Donahue, R. C. Flagan, M. Kulmala, D. R. Worsnop, P. M. Winkler, J. Dommen and U. Baltensperger, Atmos. Chem. Phys., 2018, 18, 65–79 CrossRef CAS.
- U. Molteni, M. Simon, M. Heinritzi, C. R. Hoyle, A.-K. Bernhammer, F. Bianchi, M. Breitenlechner, S. Brilke, A. Dias, J. Duplissy, C. Frege, H. Gordon, C. Heyn, T. Jokinen, A. Kürten, K. Lehtipalo, V. Makhmutov, T. Petäjä, S. M. Pieber, A. P. Praplan, S. Schobesberger, G. Steiner, Y. Stozhkov, A. Tomé, J. Tröstl, A. C. Wagner, R. Wagner, C. Williamson, C. Yan, U. Baltensperger, J. Curtius, N. M. Donahue, A. Hansel, J. Kirkby, M. Kulmala, D. R. Worsnop and J. Dommen, Earth Space Chem., 2019, 3, 873–883 CrossRef CAS.
- Q. Ye, M. Wang, V. Hofbauer, D. Stolzenburg, D. Chen, M. Schervish, A. Vogel, R. L. Mauldin, R. Baalbaki, S. Brilke, L. Dada, A. Dias, J. Duplissy, I. El Haddad, H. Finkenzeller, L. Fischer, X. He, C. Kim, A. Kürten, H. Lamkaddam, C. P. Lee, K. Lehtipalo, M. Leiminger, H. E. Manninen, R. Marten, B. Mentler, E. Partoll, T. Petäjä, M. Rissanen, S. Schobesberger, S. Schuchmann, M. Simon, Y. J. Tham, M. Vazquez-Pufleau, A. C. Wagner, Y. Wang, Y. Wu, M. Xiao, U. Baltensperger, J. Curtius, R. Flagan, J. Kirkby, M. Kulmala, R. Volkamer, P. M. Winkler, D. Worsnop and N. M. Donahue, Environ. Sci. Technol., 2019, 53, 12357–12365 CrossRef CAS.
- M. Simon, L. Dada, M. Heinritzi, W. Scholz, D. Stolzenburg, L. Fischer, A. C. Wagner, A. Kürten, B. Rörup, X.-C. He, J. Almeida, R. Baalbaki, A. Baccarini, P. S. Bauer, L. Beck, A. Bergen, F. Bianchi, S. Bräkling, S. Brilke, L. Caudillo, D. Chen, B. Chu, A. Dias, D. C. Draper, J. Duplissy, I. El Haddad, H. Finkenzeller, C. Frege, L. Gonzalez-Carracedo, H. Gordon, M. Granzin, J. Hakala, V. Hofbauer, C. R. Hoyle, C. Kim, W. Kong, H. Lamkaddam, C. P. Lee, K. Lehtipalo, M. Leiminger, H. Mai, H. E. Manninen, G. Marie, R. Marten, B. Mentler, U. Molteni, L. Nichman, W. Nie, A. Ojdanic, A. Onnela, E. Partoll, T. Petäjä, J. Pfeifer, M. Philippov, L. L. J. Quéléver, A. Ranjithkumar, M. Rissanen, S. Schallhart, S. Schobesberger, S. Schuchmann, J. Shen, M. Sipilä, G. Steiner, Y. Stozhkov, C. Tauber, Y. J. Tham, A. R. Tomé, M. Vazquez-Pufleau, A. Vogel, R. Wagner, M. Wang, D. S. Wang, Y. Wang, S. K. Weber, Y. Wu, M. Xiao, C. Yan, P. Ye, Q. Ye, M. Zauner-Wieczorek, X. Zhou, U. Baltensperger, J. Dommen, R. C. Flagan, A. Hansel, M. Kulmala, R. Volkamer, P. M. Winkler, D. R. Worsnop, N. M. Donahue, J. Kirkby and J. Curtius, Atmos. Chem. Phys., 2020, 20, 9183–9207 CrossRef CAS.
- Z. Tan, K. Lu, A. Hofzumahaus, H. Fuchs, B. Bohn, F. Holland, Y. Liu, F. Rohrer, M. Shao, K. Sun, Y. Wu, L. Zeng, Y. Zhang, Q. Zou, A. Kiendler-Scharr, A. Wahner and Y. Zhang, Atmos. Chem. Phys., 2019, 19, 7129–7150 CrossRef CAS.
- Z. Tan, F. Rohrer, K. Lu, X. Ma, B. Bohn, S. Broch, H. Dong, H. Fuchs, G. I. Gkatzelis, A. Hofzumahaus, F. Holland, X. Li, Y. Liu, Y. Liu, A. Novelli, M. Shao, H. Wang, Y. Wu, L. Zeng, M. Hu, A. Kiendler-Scharr, A. Wahner and Y. Zhang, Atmos. Chem. Phys., 2018, 18, 12391–12411 CrossRef CAS.
- G. S. Tyndall, R. A. Cox, C. Granier, R. Lesclaux, G. K. Moortgat, M. J. Pilling, A. R. Ravishankara and T. J. Wallington, J. Geophys. Res., 2001, 106, 12157 CrossRef CAS.
- R. Atkinson, D. L. Baulch, R. A. Cox, J. N. Crowley, R. F. Hampson, R. G. Hynes, M. E. Jenkin, M. J. Rossi, J. Troe and T. J. Wallington, Atmos. Chem. Phys., 2008, 8, 4141–4496 CrossRef CAS.
- R. Atkinson, D. L. Baulch, R. A. Cox, J. N. Crowley, R. F. Hampson, R. G. Hynes, M. E. Jenkin, M. J. Rossi, J. Troe and I. Subcommittee, Atmos. Chem. Phys., 2006, 6, 3625–4055 CrossRef CAS.
-
P. O. Wennberg, IGAC Newsletter No. 50 - July 2013, 2013, https://issuu.com/igacnews/docs/igac/3 Search PubMed.
- S. Madronich and J. G. Calvert, J. Geophys. Res.: Atmos., 1990, 95, 5697 CrossRef CAS.
- N. M. Donahue and R. G. Prinn, J. Geophys. Res.: Atmos., 1990, 95, 18387–18411 CrossRef CAS.
- P. S. Stevens, J. H. Mather, W. H. Brune, F. Eisele, D. Tanner, A. Jefferson, C. Cantrell, R. Shetter, S. Sewall, A. Fried, B. Henry, E. Williams, K. Baumann, P. Goldan and W. Kuster, J. Geophys. Res.: Atmos., 1997, 102, 6379–6391 CrossRef CAS.
- C. A. Cantrell, J. A. Lind, R. E. Shetter, J. G. Calvert, P. D. Goldan, W. Kuster, F. C. Fehsenfeld, S. A. Montzka, D. D. Parrish, E. J. Williams, M. P. Buhr, H. H. Westberg, G. Allwine and R. Martin, J. Geophys. Res.: Atmos., 1992, 97, 20671–20686 CrossRef.
- V. Ferracci, I. Heimann, N. L. Abraham, J. A. Pyle and A. T. Archibald, Atmos. Chem. Phys., 2018, 18, 7109–7129 CrossRef CAS.
- V. Sinha, J. Williams, J. Lelieveld, T. Ruuskanen, M. Kajos, J. Patokoski, H. Hellen, H. Hakola, D. Mogensen, M. Boy, J. Rinne and M. Kulmala, Environ. Sci. Technol., 2010, 44, 6614–6620 CrossRef CAS.
- A. C. Nölscher, J. Williams, V. Sinha, T. Custer, W. Song, A. M. Johnson, R. Axinte, H. Bozem, H. Fischer, N. Pouvesle, G. Phillips, J. N. Crowley, P. Rantala, J. Rinne, M. Kulmala, D. Gonzales, J. Valverde-Canossa, A. Vogel, T. Hoffmann, H. G. Ouwersloot, J. Vilà-Guerau de Arellano and J. Lelieveld, Atmos. Chem. Phys., 2012, 12, 8257–8270 CrossRef.
- P. di Carlo, W. H. Brune, M. Martinez, H. Harder, R. Lesher, X. Ren, T. Thornberry, M. A. Carroll, V. Young, P. B. Shepson, D. Riemer, E. Apel and C. Campbell, Science, 2004, 304, 722–725 CrossRef CAS.
- J. A. Logan, M. J. Prather, S. C. Wofsy and M. B. McElroy, J. Geophys. Res.: Atmos., 1981, 86, 7210–7254 CrossRef CAS.
- H. L. Singh, L. J. Salas, B. A. Ridley, J. D. Shetter, N. M. Donahue, F. C. Fehsenfeld, D. W. Fahey, D. D. Parish, E. J. Williams, S. C. Liu, G. Hübler and P. C. Murphy, Nature, 1985, 318, 347–349 CrossRef CAS.
- H. O. T. Pye, E. L. D'Ambro, B. H. Lee, S. Schobesberger, M. Takeuchi, Y. Zhao, F. Lopez-Hilfiker, J. Liu, J. E. Shilling, J. Xing, R. Mathur, A. M. Middlebrook, J. Liao, A. Welti, M. Graus, C. Warneke, J. A. de Gouw, J. S. Holloway, T. B. Ryerson, I. B. Pollack and J. A. Thornton, Proc. Natl. Acad. Sci. U. S. A., 2019, 116, 6641–6646 CrossRef CAS.
- C. Yan, W. Nie, A. L. Vogel, L. Dada, K. Lehtipalo, D. Stolzenburg, R. Wagner, M. P. Rissanen, M. Xiao, L. Ahonen, L. Fischer, C. Rose, F. Bianchi, H. Gordon, M. Simon, M. Heinritzi, O. Garmash, P. Roldin, A. Dias, P. Ye, V. Hofbauer, A. Amorim, P. S. Bauer, A. Bergen, A.-K. Bernhammer, M. Breitenlechner, S. Brilke, A. Buchholz, S. B. Mazon, M. R. Canagaratna, X. Chen, A. Ding, J. Dommen, D. C. Draper, J. Duplissy, C. Frege, C. Heyn, R. Guida, J. Hakala, L. Heikkinen, C. R. Hoyle, T. Jokinen, J. Kangasluoma, J. Kirkby, J. Kontkanen, A. Kürten, M. J. Lawler, H. Mai, S. Mathot, R. L. Mauldin, U. Molteni, L. Nichman, T. Nieminen, J. Nowak, A. Ojdanic, A. Onnela, A. Pajunoja, T. Petäjä, F. Piel, L. L. J. Quéléver, N. Sarnela, S. Schallhart, K. Sengupta, M. Sipilä, A. Tomé, J. Tröstl, O. Väisänen, A. C. Wagner, A. Ylisirniö, Q. Zha, U. Baltensperger, K. S. Carslaw, J. Curtius, R. C. Flagan, A. Hansel, I. Riipinen, J. N. Smith, A. Virtanen, P. M. Winkler, N. M. Donahue, V.-M. Kerminen, M. Kulmala, M. Ehn and D. R. Worsnop, Sci. Adv., 2020, 6, eaay4945 CrossRef CAS.
- T. Berndt, S. Richters, T. Jokinen, N. Hyttinen, T. Kurtén, R. V. Otkjær, H. G. Kjaergaard, F. Stratmann, H. Herrmann, M. Sipilä, M. Kulmala and M. Ehn, Nat. Commun., 2016, 7, 13677 CrossRef CAS.
- T. Berndt, S. Richters, R. Kaethner, J. Voigtländer, F. Stratmann, M. Sipilä, M. Kulmala and H. Herrmann, J. Phys. Chem. A, 2015, 119, 10336–10348 CrossRef CAS.
- J. Duplissy, J. Merikanto, A. Franchin, G. Tsagkogeorgas, J. Kangasluoma, D. Wimmer, H. Vuollekoski, S. Schobesberger, K. Lehtipalo, R. C. Flagan, D. Brus, N. M. Donahue, H. Vehkamäki, J. Almeida, A. Amorim, P. Barmet, F. Bianchi, M. Breitenlechner, E. M. Dunne, R. Guida, H. Henschel, H. Junninen, J. Kirkby, A. Kürten, A. Kupc, A. Määttänen, V. Makhmutov, S. Mathot, T. Nieminen, A. Onnela, A. P. Praplan, F. Riccobono, L. Rondo, G. Steiner, A. Tome, H. Walther, U. Baltensperger, K. S. Carslaw, J. Dommen, A. Hansel, T. Petäjä, M. Sipilä, F. Stratmann, A. Vrtala, P. E. Wagner, D. R. Worsnop, J. Curtius and M. Kulmala, J. Geophys. Res.: Atmos., 2016, 212, 1752–1775 Search PubMed.
- J. E. Krechmer, D. Pagonis, P. J. Ziemann and J. L. Jimenez, Environ. Sci. Technol., 2016, 50, 5757–5765 CrossRef CAS.
- P. Ye, X. Ding, J. Hakala, V. Hofbauer, E. S. Robinson and N. M. Donahue, Aerosol Sci. Technol., 2016, 50, 822–834 CrossRef CAS.
- A. Kürten, C. Williamson, J. Almeida, J. Kirkby and J. Curtius, Atmos. Chem. Phys., 2015, 15, 4063–4075 CrossRef.
-
N. Donahue, W. Chuang and M. Schervish, Advances in Atmospheric Chemistry: Organic Oxidation and Multiphase Chemistry, World Scientific, 2019, ch. 4, vol. 2, pp. 199–317 Search PubMed.
- A. A. Presto and N. M. Donahue, J. Phys. Chem. A, 2004, 108, 9096–9104 CrossRef CAS.
- S. E. Paulson, M. Chung, A. D. Sen and G. Orzechowska, J. Geophys. Res.: Atmos., 1998, 103, 25533–25539 CrossRef CAS.
- K. M. Henry and N. M. Donahue, Aerosol Sci. Technol., 2011, 45, 686–690 CAS.
- M. Krapf, I. E. Haddad, E. A. Bruns, U. Molteni, K. R. Daellenbach, A. S. Prévôt, U. Baltensperger and J. Dommen, Chem, 2016, 1, 603–616 CAS.
- T. Jokinen, J. Kontkanen, K. Lehtipalo, H. E. Manninen, J. Aalto, A. Porcar-Castell, O. Garmash, T. Nieminen, M. Ehn, J. Kangasluoma, H. Junninen, J. Levula, J. Duplissy, L. R. Ahonen, P. Rantala, L. Heikkinen, C. Yan, M. Sipilä, D. R. Worsnop, J. Bäck, T. Petäjä, V.-M. Kerminen and M. Kulmala, Sci. Rep., 2017, 7, 45707 CrossRef CAS.
- G. McFiggans, T. F. Mentel, J. Wildt, I. Pullinen, S. Kang, E. Kleist, S. Schmitt, M. Springer, R. Tillmann, C. Wu, D. Zhao, M. Hallquist, C. Faxon, M. Le Breton, Å. M. Hallquist, D. Simpson, R. Bergström, M. E. Jenkin, M. Ehn, J. A. Thornton, M. R. Alfarra, T. J. Bannan, C. J. Percival, M. Priestley, D. Topping and A. Kiendler-Scharr, Nature, 2019, 565, 587–593 CrossRef CAS.
- B. Zhao, M. Shrivastava, N. M. Donahue, H. Gordon, M. Schervish, J. E. Shilling, R. A. Zaveri, J. Wang, M. O. Andreae, C. Zhao, B. Gaudet, Y. Liu, J. Fan and J. D. Fast, Proc. Natl. Acad. Sci. U. S. A., 2020, 117, 25344–25351 CrossRef CAS.
|
This journal is © The Royal Society of Chemistry 2021 |