DOI:
10.1039/D1BM00210D
(Paper)
Biomater. Sci., 2021,
9, 7420-7431
A 3D pancreatic tumor model to study T cell infiltration†
Received
5th February 2021
, Accepted 2nd September 2021
First published on 27th October 2021
Abstract
The desmoplastic nature of the pancreatic ductal adenocarcinoma (PDAC) tumor microenvironment (TME) prevents the infiltration of T cells and the penetration of chemotherapeutic drugs, posing a challenge to the validation of targeted therapies, including T cell immunotherapies. We present an in vitro 3D PDAC-TME model to observe and quantify T cell infiltration across the vasculature. In a three-channel microfluidic device, PDAC cells are cultured in a collagen matrix in the central channel surrounded, on one side, by endothelial cells (ECs) to mimic a blood vessel and, on the opposite side, by pancreatic stellate cells (PSCs) to simulate exocrine pancreas. The migration of T cells toward the tumor is quantified based on their activation state and TME composition. The presence of EC-lining drastically reduces T cell infiltration, confirming the essential role of the vasculature in controlling T cell trafficking. We show that activated T cells migrate ∼50% more than the not-activated ones toward the cancer cells. Correspondingly, in the absence of cancer cells, both activated and not-activated T cells present similar migration toward the PSCs. The proposed approach could help researchers in testing and optimizing immunotherapies for pancreatic cancer.
1. Introduction
Pancreatic cancer, in particular pancreatic ductal adenocarcinoma (PDAC), is one of the leading causes of cancer-related death worldwide, with an overall 5-year patient survival rate of less than 9%, due to the lack of early detection tools and limited response toward conventional treatments.1–3 In particular, only 10% of the patients presents a resectable tumor, as the majority of the tumors have already developed metastases or advanced lesions upon detection.1 In the last decade, despite significant advances in the development of cancer immunotherapeutic strategies,4,5 treatment response for pancreatic cancer has been limited.6
The poor prognosis of PDAC is likely to be attributed to the desmoplastic nature of its tumor microenvironment (TME), characterized by an accumulation of stromal cells and deposition of the extracellular matrix (ECM).7,8 In particular, pancreatic stellate cells (PSCs) and cancer-associated fibroblasts (CAFs) are the dominant tumor-associated stroma cells that produce excessive ECM proteins such as collagen and fibronectin, which result in the formation of a dense barrier that limits vascularization and diminishes drug delivery efficacy and T cell infiltration.9,10 In addition, the distinct presence of immunosuppressive cells, such as T regulatory cells and myeloid derived suppressive cells, and an anergic vasculature in the PDAC-TME further dampen T cell recruitment, proliferation and functions, leading to inadequate anti-tumor immune response necessary for tumor eradication.11–18
Understanding the complex and heterogeneous cellular landscape in the PDAC-TME and how it affects the immune infiltration is important to improve the current treatment interventions, specifically to develop different strategies for making the PDAC-TME more permissive to T cell infiltration as well as maintaining active T cell effector functions within the tumor. However, the present attempts to study immune infiltrates have been restricted by the unavailability of PDAC tissue biopsies and the lack of appropriate models. Animal models, such as genetically engineered mouse models and patient-derived xenograft mouse models, although expensive, time-consuming and presenting ethical issues,19,20 have provided useful information about some molecular mechanisms involved in the establishment of a PDAC-TME. However, many other mechanisms remain unknown and a careful interpretation of the results derived from these animal models is required due to interspecies differences.
3D in vitro models have recently been a promising culture method to provide in vivo-like physiologically relevant conditions and reduce the burden of animal models.21–24 Among the existing 3D culture platforms, microfluidic systems represent a promising tool to recreate the spatial tissue architecture in a highly controllable fashion to recapitulate the in vivo microenvironment and test immunotherapeutic strategies.25–27 3D microfluidic models allow a real-time analysis of cellular interactions, the possibility to mimic the main steps of the metastatic cascade and elucidate critical factors in the tumor progression.28–33 For instance, the involvement of macrophages in regulating the intravasation of breast cancer cells through an endothelial layer when co-cultured in a microfluidic device was demonstrated by Zervantonakis et al.34 In a microfluidic-based lung carcinoma model, Bai et al. investigated the contribution of tumor-associated macrophages (TAMs) in modulating the epithelial-to-mesenchymal transition (EMT) of cancer cell aggregates.35 Additionally, Penny et al. showed that TAMs promote PDAC cell extravasation through a vascular wall by co-culturing cancer cells, macrophages and endothelial cells (ECs) in a microfluidic device.36 The anti-tumor activity of engineered T cells specific for Hepatitis B virus (Hep-B) associated hepatocellular carcinoma (HCC) was evaluated by Pavesi et al. using another 3D microfluidic model,37,38 while Lee et al. elucidated the immunosuppressive role of monocytes toward engineered T cells targeting HCC cell aggregates via the immune checkpoint programmed cell death protein 1 (PD-1) and its ligand PD-L1.39 In a recent work, T cell homing to colorectal tumors was modeled in a microfluidic system that mimics the vascular and the extravascular compartments.40 Other authors have also shown that it is possible to mimic the effects of fluid flow in microfluidic systems for studying immune infiltration.41,42
To provide our contribution to the field, we designed a 3D microfluidic-based PDAC-TME model to assess the infiltration of T cells across the vasculature. In particular, ECs and PSCs were used to mimic a blood vessel and the exocrine pancreas, respectively. We monitored T cell migration toward PDAC cells embedded into a collagen type I matrix, providing a quantitative assessment of T cell efficiency in transmigrating into the TME in relation to their activation state and the presence of PDAC cells, PSCs and EC linings.
2. Results
2.1. Development of a pancreatic tumor model by triculture of tumor, stromal and endothelial cells
To model the PDAC-TME in vitro, a three-channel microfluidic device was used (Fig. 1A). The device consists of two lateral channels and a central region defined by an array of triangular pillars. Cancer cells were cultured in the central hydrogel region, while one lateral fluidic channel was seeded with ECs that, after 2 days, formed an endothelial monolayer resembling a blood vessel (the green layer in Fig. 1). The other channel was seeded with PSCs (grey cells in Fig. 1) to represent the exocrine region of the pancreas and allow us to study PSCs' contribution to T cell migration (red cells in Fig. 1). Importantly, the device tridimensional layout allows both physical and molecular interactions between the different cell types cultured in the system. Once the triculture was established, T cells were introduced into the microchannels.
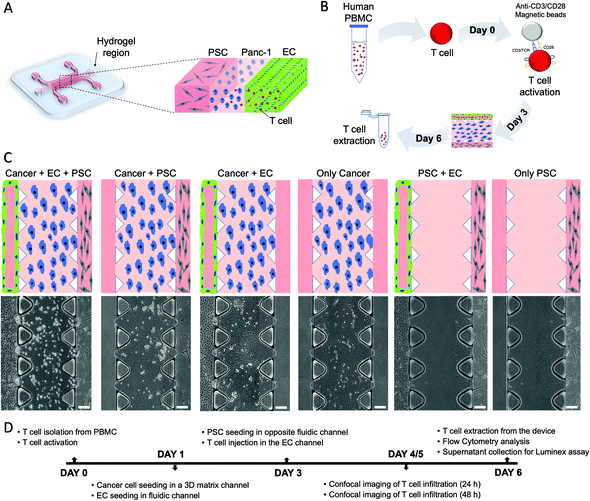 |
| Fig. 1 Experimental set-up and development of the in vitro PDAC-TME model. (A) Schematic of the three channel microfluidic device. The PDAC-TME model inside the device consists of cancer cells (PANC-1, blue) seeded in the central 3D gel region flanked by the 2 medium channels that contain endothelial cells (ECs, green) and pancreatic stellate cells (PSCs, grey), respectively. (B) Schematic of the T cell isolation, activation, seeding and extraction procedure. (C) Schematic layout (top) and brightfield images (bottom) of the different experimental conditions showing the different cell types in their respective microfluidic channels. Scale bars are 100 μm. (D) Experimental timeline. | |
After isolation from healthy peripheral blood mononuclear cells, T cells were activated via magnetic beads and, then, inserted in the endothelial channel (Fig. 1B). T cell infiltration in the PDAC region was quantified as a function of T cell activation and the presence of the endothelial barrier and stromal cells. Therefore, we considered six different experimental conditions as reported in Fig. 1C: only cancer, only PSC, cancer + PSC, cancer + EC, PSC + EC, cancer + PSC + EC.
The hydrogel region containing infiltrated T cells was then imaged at 24 h and 48 h, followed by cell extraction from the devices to analyze the T cells by flow cytometry and supernatant extraction from the devices to analyze the cytokine expression by Luminex, as reported in the experiment timeline in Fig. 1D.
2.2. Endothelial cells self-assemble to mimic a vascular barrier
Throughout the culture in the fluidic microchannel, ECs self-assembled to create an endothelial lining mimicking a blood vessel. Representative confocal images of the ECs showed the integrity of the endothelium at the interface with the collagen region, as reported in Fig. 2A and in the Movie S1, ESI.† To validate the integrity of the endothelial barrier, a permeability assay was run using fluorescent dextran with a molecular weight of 70 kDa. The dextran was introduced in the vascular channel and allowed to diffuse into the gel compartment. Images were acquired for 30 min with a fluorescent microscope to quantify the vascular permeability coefficient (P) by image analysis. The fluorescence images, as shown in Fig. 2B, depict the permeation of the fluorescent tracer in the extravascular space at 3 different time points, namely 5, 15, and 30 min post infusion. The permeability coefficient was measured in two configurations: in the presence of endothelial cells only (only EC) and in the presence of endothelial cells co-cultured with naïve T cells for 48 h (EC + T cell). Values of the permeability coefficients of 0.23 ± 0.13 μm s−1 and 0.71 ± 0.28 μm s−1 were obtained respectively for the two conditions, as shown in Fig. 2C. This statistically significant difference in permeability between the two configurations (p < 0.01) would suggest that the presence of T cells affects the integrity of the vascular barrier and, specifically, increases the vascular permeability locally by about three fold.
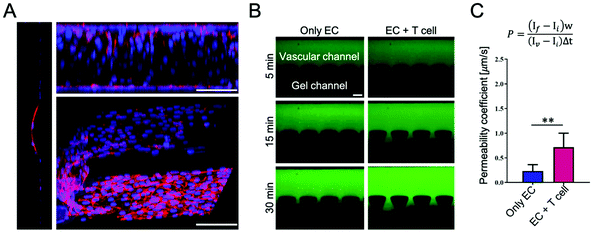 |
| Fig. 2 Microfluidic vascular model. (A) A 3D reconstruction of confocal z-stack images of endothelial cells immunostained with VE-cadherin (red) and DAPI (blue) to show the confluency of the endothelial monolayer. Cross section (left), side view (top right), 3D view (bottom right). Scale bars are 100 μm. (B) Characterization of the endothelial permeability. Representative fluorescence images of 70 kDa FITC-Dextran diffusing into the vascular channel in the presence of T cells (EC + T cell) or not (only EC). Scale bar is 150 μm. (C) Formula to calculate the permeability coefficients plotted in the graph as mean + SD, n = 8. Statistical analysis was done with Student's t-test. ** p < 0.01. | |
2.3. Impact of the endothelial barrier on T cell infiltration in the PDAC region
To perform their anti-tumor function, T cells have to migrate from the systemic circulation into the tumor tissues.43 Similarly, in our model, T cells have to transmigrate from the vascular compartment (left chamber in Fig 1C) across the endothelial barrier to reach the tumor region (central chamber in Fig. 1C) containing the PDAC cells cultured in the 3D extracellular-like matrix (Fig. 1C). T cell infiltration in the central compartment was monitored via confocal imaging at 24 h and 48 h post injection. The number of infiltrated T cells within the central region was quantified by means of the Spot function of IMARIS software that allows counting the number of point-like structures. A T cell was considered infiltrated if, and only if, the whole cell body was into the central region. The number of T cells infiltrated in the central region after 24 h is shown in Fig. 3 for activated (Fig. 3A) and not-activated (Fig. 3C) T cells, respectively. Significant differences were observed when comparing activated T cell infiltration in the presence or absence of the endothelial vessel (Fig. 3A). In particular, an average of 75.35 activated T cells infiltrated in the cancer monoculture condition (only cancer) versus 37.63 T cells in the co-culture of cancer cells with ECs (cancer + EC) (p < 0.0001). Similarly, ECs' presence drastically reduced activated T cell infiltration in the co-culture of cancer cells with PSCs (cancer + PSC) with an average of 86.73 infiltrated T cells in the cancer + PSC condition versus 41.37 infiltrated T cells in the triculture of cancer cells with PSCs and ECs (cancer + PSC + EC) (p < 0.0001). Furthermore, a reduced infiltration of activated T cells was observed when comparing the monoculture of PSCs (only PSC) with an average of 48.59 infiltrated T cells and the co-culture of PSCs with ECs (PSC + EC) with 15.02 T cells (p < 0.0001). Overall, without ECs, activated T cells were able to migrate in the collagen region about 1 time to 2 times more than those in the conditions with ECs, as shown in Fig. 3A. Representative images of activated T cell infiltration into the gel compartment for the different conditions were acquired by confocal microscopy (Fig. 3B).
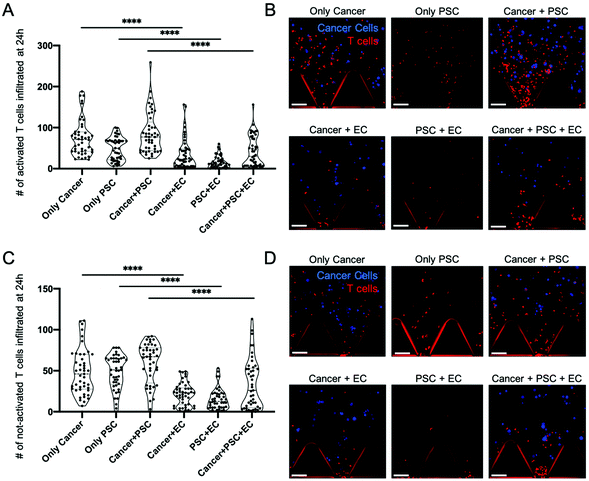 |
| Fig. 3 Effects of the endothelial barrier on T cell infiltration in the PDAC-TME model at 24 h. (A) Violin plot of the number of activated T cells infiltrated into the central region after 24 h from their injection into the device. T cells were stimulated with anti-CD3/CD28 Dynabeads for 5 days to promote activation. (B) Representative confocal images of activated T cells infiltrating into the central hydrogel channel of the microfluidic device under different experimental conditions. (C) Violin plot of the number of not-activated T cells infiltrated into the central region after 24 h of T cell injection into the device. (D) Representative confocal images of not-activated T cells infiltrating in the central hydrogel channel under different experimental conditions. T cells were labelled with CellTrace Violet and are shown in red in (B) and (D). PDAC cells were labelled with Cell Tracker Orange and are shown in blue in (B) and (D). The red trapezoidal shapes in (B) and (D) are the posts of the microfluidic device which allow to identify the gel interface during imaging and data analysis. Data in (A) and (C) are plotted with violin plots showing the probability density for each value, n = 5. Statistical analysis is done with one-way ANOVA with multiple comparisons. **** p < 0.0001. The scale bars are 100 μm. | |
Similar restriction by the ECs was observed for the not-activated T cell population. Indeed, the not-activated T cell infiltration process was reduced in the presence of the endothelial barrier (Fig. 3C). In particular, an average of 48.98 not-activated T cells infiltrated in the cancer monoculture condition (only cancer) versus 21.89 T cells in the cancer cells with ECs condition (cancer + EC) (p < 0.0001). Co-culture of cancer cells with PSCs (cancer + PSC) reported an average of 58.91 infiltrated not-activated T cells versus 33.89 under triculture conditions (cancer + PSC + EC) (p < 0.0001). Monoculture of PSCs (only PSC) presented an average of 47.82 infiltrated not-activated T cells and the co-culture of PSCs with ECs (PSC + EC) had 15.93 T cells (p < 0.0001). As done for activated T cells, representative images of not-activated T cells infiltrated into the gel compartment for the different conditions were acquired by confocal microscopy (Fig. 3D).
As shown in Fig. S2, ESI,† these results on T cell infiltration were consistent between 24 h and 48 h, demonstrating that the presence of ECs has a significant impact upon the T cell migration toward the tumor tissue in line with previous observations.44,45
2.4. Impact of T cell activation on infiltration and cytokine expression levels in the 3D system
T cell activation was performed by cell stimulation with anti-CD3/CD28 magnetic beads, which provide essential co-stimulatory signals for functional cell activation.46 Both activated and not-activated T cells were cultured in the presence of IL-2 to promote proliferation47 and both populations resulted in an increased expansion. In particular, the activated T cells had a 4-fold increase in the expansion, while the not-activated T cells reached only a 2.5-fold increase (data not shown). To assess the effect of activation on T cell infiltration, we directly compared T cell infiltration for activated and not-activated T cells without (Fig. 4A) or with (Fig. 4B) ECs at 24 h post injection. Without ECs, activated T cells displayed a significantly higher infiltration than not-activated T cells toward the 3D region filled with cancer cells either in monoculture (75.35 activated vs. 48.98 not-activated) or in co-culture with PSCs (86.73 activated vs. 58.91 not-activated) (Fig. 4A). Overall, activated T cells infiltrated ∼54% more than not-activated T cells toward pancreatic cancer cells (only cancer) and ∼47% more under the co-culture condition (cancer + PSC). The endothelial barrier diminished the T cell infiltration, but we could still observe a statistically significant higher infiltration of activated T cells toward the 3D hydrogel region filled with cancer cells in co-culture with ECs (Fig. 4B). Importantly, activated and not-activated T cells migrated similarly toward PSCs, both in the absence (Fig. 4A) or in the presence (Fig. 4B) of an endothelial barrier, suggesting that the effects of activation are evident only when T cells migrate toward cancer cells. In fact, by the direct comparison of activated T cell infiltration toward cancer cells and toward PSCs with or without an endothelial barrier, we observed a significantly higher infiltration of T cells toward cancer cells (Fig. S3A and S3B, ESI†). Instead, not-activated T cells showed the same infiltration toward cancer and PSCs with and without endothelium (Fig. S3C and S3D, ESI†). Similar considerations are applicable to the data at 48 h post injection (Fig. S4 and S5, ESI†).
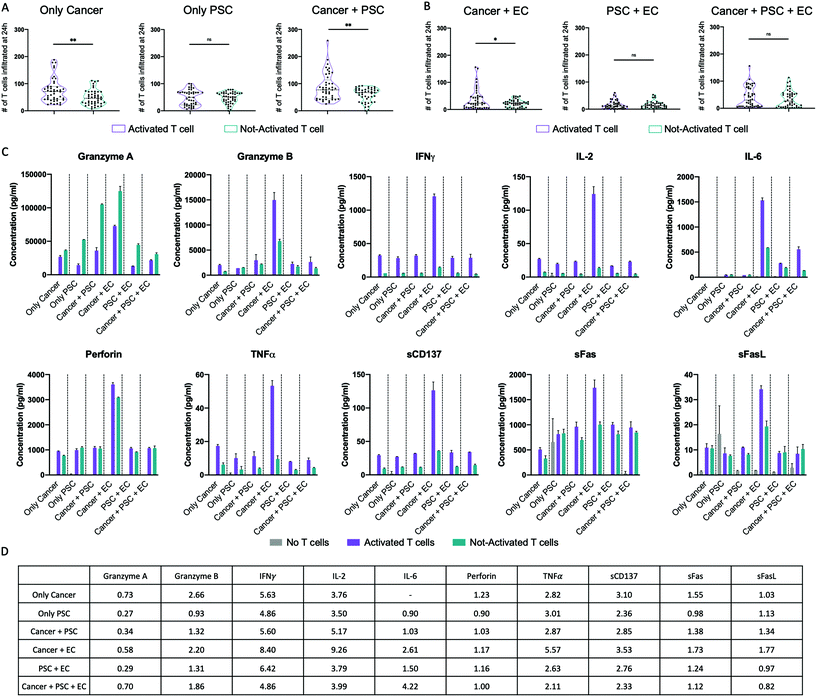 |
| Fig. 4 Effects of activation and cell culture condition on T cell infiltration and cytokine expression in the 3D PDAC system. (A and B) Violin plots showing the probability density of the number of infiltrating T cells in the 3D gel region in all tested conditions without ECs (only cancer, only PSC, cancer + PSC) (A) and with ECs (cancer + EC, PSC + EC, cancer + PSC + EC) (B). Statistical analysis done with Student's t-test. ** p < 0.01. * p < 0.05. ns: not significant. (C) Bar plots of the data obtained from Luminex multiplex cytokine analysis for each tested analyte grouped per cell culture condition. Results are reported as analyte concentration in pg ml−1. (D) Table of the foldchange in the cytokine expression between activated and not-activated T cell samples. The relative expression was calculated as the ratio between the analyte concentration in the sample with activated T cells and the analyte concentration in the sample with not-activated T cells. | |
Utilizing the Luminex multiplex technology, we measured the levels of 11 cytokines in the supernatant of the different cell culture conditions without T cells, with activated T cells, and with not-activated T cells (Fig. 4C). All the tested analytes were detectable except the IL-4 that presented values lower than the standard range. Samples with activated T cells expressed a higher level of IFNγ, IL-2, sCD137, and TNFα than samples with not-activated T cells for all the culture conditions. Granzyme B and sFas expression levels were higher in samples with activated T cells than in samples with not-activated T cells for all the culture conditions except the condition with only PSCs, while granzyme A was lower in samples with activated T cells than in samples with not-activated T cells for all the culture conditions. IL-6 was higher in samples with activated T cells than in samples with not-activated T cells only for the culture conditions with ECs. Notably, the sample with activated T cells in the cancer + EC culture condition presented the highest level of all the cytokines except the granzyme A. The fold change in cytokine expression levels between the samples with activated and not-activated T cells under different culture conditions is reported in the table in Fig. 4D.
2.5. Flow cytometry analysis on T cells before and after culture in the 3D system
Flow cytometry analysis was performed to show the possibility to assess the expression of specific T cell markers either before or after T cell culture in the 3D microfluidic system. The percentage of CD3+ T cells gated for CD4+ and CD8+ for both activated (Fig. 5A) and not-activated (Fig. 5D) T cells was analyzed before their injection into the microfluidic device. Activated T cells presented 75.8% of CD4+ T cells and 17.3% of CD8+ T cells. Not-activated T cells comprised 59.5% of CD4+ T cells and 34.9% of CD8+ T cells. Our data for not-activated T cells reflected the higher percentage of CD4+ T cells commonly found in peripheral blood where the CD4+ to CD8+ T cell ratio is usually around 2
:
1.48 Activated T cells showed a ratio CD4+
:
CD8+ T cells of about
4
:
1 that could be due to the activation of Dynabeads which has been shown to preferentially support the CD4+ T cell expansion.49 The expression of PD-1 was analyzed because PD-1 is widely recognized as an activation marker50,51 and its expression on naïve T cells is induced upon CD3/CD28 stimulation as it was confirmed by our flow cytometry data. Before injection into the device, activated CD3+ T cells expressed 40.3% of PD-1 (Fig. 5B), while not-activated T cells had a PD-1 population of 17.8% (Fig. 5E). Moreover, PD-1 expression increased with the T cell activation in both CD8+ and CD4+ T cell populations (Fig. S6, ESI†). Activated and not-activated T cells also presented a low amount of TIM3 (Fig. 5C and F) that usually is highly expressed in exhausted T cells.52 These results confirmed the efficacy of T cell stimulation, obtained via Dynabeads, with high consistency among the T cell subtypes.
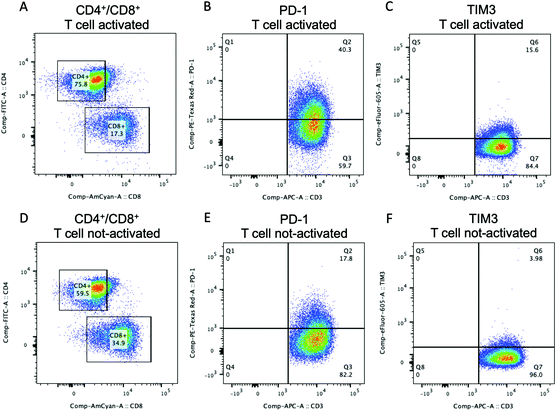 |
| Fig. 5 Flow cytometry analysis on T cells. Activated (A) or not-activated (D) CD3+ T cells were gated for CD4 and CD8+cells. Cells were labeled with FITC conjugated anti-CD4 and AmCyan conjugated anti-CD8. Flow cytometry analysis of PD-1 expression on activated (B) and not-activated (E) T cells. Cells were labeled with APC conjugated anti-CD3 and Texas Red conjugated anti-PD-1. Flow cytometry analysis of TIM3 on activated (C) and not-activated (F) T cells. Cells were labeled with APC conjugated anti-CD3 and eFluor 605 conjugated anti-TIM3. CD3+ T cells were stimulated with anti-CD3/CD28 Dynabeads for 5 days in the presence of IL-2. At least 50 000 events were acquired. | |
PD-1 expression was also quantified on CD3+ T cells after 48 h of co-culture in the device for each experimental condition. The percentage of PD-1+ activated T cells was overall lower compared to activated T cells before injections (Fig. S7, ESI†) and remained slightly higher for activated versus not-activated T cells when cancer cells were alone (Fig. S7A and B, ESI†) or in co-culture with ECs only (Fig. S7G and H, ESI†) or with ECs and PSCs (Fig. S7K and L, ESI†). The percentage of PD-1+ T cells was similar for activated versus not activated T cells when cancer cells were in co-culture with PSC (Fig. S7E and F, ESI†). Interestingly, under all the above conditions, the CD4+
:
CD8+ ratio decreased (Fig. S8, ESI†), showing an expansion in favor of the CD8+ T cell population.
3. Discussion
We report here the design, development, and validation of a 3D multiculture system to study T cell infiltration into the PDAC-TME, incorporating critical cellular and non-cellular components. PDAC cells, PSCs and ECs were co-cultured inside a microfluidic device to mimic the complex in vivo TME. The PANC-1 cell line was selected to model the malignant pancreatic cells, and PSCs were used to represent the exocrine region while human umbilical vein endothelial cells (HUVECs) formed an endothelial monolayer mimicking a blood vessel close to the tumor tissue. T cells isolated from peripheral blood were introduced into the vascular channel and allowed to migrate toward the tumor cells embedded in an extracellular-matrix-like hydrogel. This cellular layout demonstrates the ability to co-culture four different types of cells in one single platform (Fig. 1). Immunofluorescence labelling of cells and the transparency of the microfluidic device allowed the visualization of the cell position into the system at different time points by confocal microscopy. We showed how the in vitro model permits for a quantitative assessment of T cell functionality in terms of trans-endothelial migration and tumor infiltration which mimic in vivo conditions. Importantly, we demonstrated the possibility to extract T cells from the device to perform an additional functional characterization such as the expression of specific activation and exhaustion markers by flow cytometry.
Our results showed that T cell infiltration is affected by the presence of a vascular endothelium, which is in agreement with current research studies45 showing the essential role of the endothelial barrier in mediating immune cell trafficking. The endothelium, in fact, regulates a large cascade of events consisting of immune cell rolling, adhesion, intravasation, and paracellular–transcellular transmigration steps.43 Moreover, ECs can release a set of cytokines and soluble factors which can enhance or suppress the immune response.53
The presented biomimetic endothelial layer, despite lacking the contribution of smooth muscle cells and pericytes, formed a functional vascular channel as assessed by the vascular endothelial cadherin (VE-Cadherin) expression and the measurement of permeability coefficients (Fig. 2) which are in agreement with previous in vitro models.34,54,55 Of note, the permeability values were still far from the in vivo value (P = 0.0098 μm s−1),56 possibly because of the intrinsic limitations of the in vitro model and the lack of other molecular and cellular components, such as pericytes.57
Taking into consideration that both the presence of the endothelial barrier as well as other TME components are known to play key roles in regulating T cell migration, the inclusion of an endothelial barrier in a 3D model provides a better estimation of T cell infiltration in tumor, which in turn provides a better tool for testing current cell therapy strategies. Neglecting the contribution of endothelial cells in the analysis of T cell infiltration could significantly overestimate their migration and leading to failures in subsequent clinical trials. In our model, we were able to demonstrate, for the first time in a PDAC model,58 that the presence of ECs dampened T cell infiltration, hence preventing them from reaching the tumor cells, regardless of T cell activation state either after 24 h (Fig. 3) or after 48 h from injection into the system (Fig. S2, ESI†).
We activated T cells by means of anti-CD3/CD28 magnetic Dynabeads stimulation.46,59 Anti-CD3 activates the T cell receptor (TCR) complex, which is typically activated with the cognate antigen from APCs; anti-CD28 binds to the CD28 co-stimulatory receptor expressed on the T cells and prevents T cell anergy similarly to what is observed after CD28 interaction with CD80/CD86 ligands expressed by APCs.53,59 We demonstrated that anti-CD3/CD28 stimulation enhanced PD-1 expression on both CD4+ and CD8+ T cells before their injection into the microfluidic device. Expression levels of PD-1 have been directly linked to TCR signal strength.60 The role of T cell activation in T cell infiltration propensity was assessed in our 3D model and a higher activated T cell infiltration in response to tumor stimuli was observed at 24 h (Fig. 4) and 48 h (Fig. S5, ESI†) supporting other studies showing that T cell activation may result in the modification of T cell motility patterns and impact their migration rate.61 Activated T cell infiltration in the tumor region was higher than not-activated T cell infiltration even in the presence of ECs (Fig. 4), which is in line with the literature reporting that activated T cells migrate more effectively through endothelial barriers than resting T cells.62,63
In addition to the assessment of the impact of the endothelial barrier and the T cell activation state, we compared T cell infiltration in the presence of cancer cells only, PSCs only and cancer cells with PSCs, with and without ECs (Fig. S3 and S4, ESI†). Our data demonstrated that activated T cells preferred to migrate toward malignant cells either at 24 h or 48 h after injection because activated T cells presented higher infiltration toward cancer cells than toward PSCs, with or without ECs (Fig. S3A, B and S4A, B, ESI†). The observed higher infiltration of activated T cells in the above-mentioned conditions was reflected by an increased PD-1 expression compared to not-activated T cells (Fig. 5). The addition of organ-specific stromal cells (cancer + PSC) with or without ECs did not contribute to the increase in activated T cell infiltration in our system (Fig. S3A, B and S4A, B, ESI†). Not-activated T cells, instead, were hyporeactive to tumor cells and responded with a similar infiltration toward cancer cells only, toward PSCs only and the co-culture condition (cancer + PSC) without ECs at either 24 h or 48 h after injection (Fig. S3C and S4C, ESI†) in agreement with a documented random walk in response to different stimuli.64 Interestingly, we observed an increase in the infiltration of not-activated T cells only for the triculture of cancer cells, PSCs and ECs either at 24 h or 48 h after injection (Fig. S3D and S4D, ESI†), suggesting that the more complex cellular assembly in the system may promote not-activated T cell migration.
The Luminex multiplex assay gave us insights into the cytokine expression levels during T cell infiltration under different co-culture conditions. The sample with activated T cells in the cancer + EC culture condition presented about 9-fold higher expression level of IFNγ and IL-2 than the samples with not-activated T cells suggesting that the presence of EC leads to a stronger T cell activation. In the same cancer + EC culture condition with activated T cells, we also observed higher levels of perforin, granzyme B and sFas, suggesting an increased cytotoxic ability of activated T cells compared to not-activated T cells. Interestingly, the samples from the triculture of pancreatic cancer cells, PSCs, and ECs presented much lower expression levels of the same cytokines suggesting that the presence of PSC is dampening the T cell inflammatory response, possibly, to promote tumor growth. Our observations agree with previous studies showing how PDAC cells can reprogram the stromal cells to promote an immunosuppressive microenvironment and sustain their growth.65–67 These results highlight the importance of including both endothelial and stromal cells in tumor models for studying T cells infiltration and function. Although further experiments are needed to elucidate the mechanisms underlying our observations, we showed the advantage of our model in discerning key components involved in T cell infiltration into the TME using different experimental conditions, and the versatility of methods of analysis to provide a more physiological tool compared to other 2D and 3D in vitro models where the vascular region and cell–cell or cell–ECM interactions are missing.
4. Conclusion
A 3D multicellular tumor model was developed to study T cell infiltration in human pancreatic tumors through a perfusable endothelialized microvessel. Pancreatic cancer cells, organ-specific stromal cells and endothelial cells were co-cultured inside a microfluidic device to recapitulate the complex in vivo TME. T cell infiltration was quantitatively evaluated as a function of the T cell activation status and TME cellular components. Our work highlights the essential role of the vascular endothelium and TME in T cell migration.
In conclusion, this study contributes to the progress of in vitro, biologically-accurate 3D pancreatic cancer models to advance our understanding of T cell infiltration mechanisms, in the context of different TMEs, and efficiently select optimal personalized immunotherapies for individual patients.
5. Experimental section
5.1. Cell culture
HUVECs expressing the green fluorescent protein (GFP) were purchased from Angioproteomie, cultured in T75 flasks with Endothelial Growth Medium (EGM-2, Lonza) and used between passage 3 and 6. PANC-1 cells were purchased from ATCC® and cultured in Dulbecco's Modified Eagle's Medium (DMEM) supplemented with 1% Pen–Strep (Gibco), 1% GlutaMAX (Gibco) and 10% fetal bovine serum (FBS) (Gibco). PSCs were purchased from ScienCell and cultured in Stellate Cell Medium (SteCM) (ScienCell) following the manufacturer's instructions and used between passage 10 and 20. Cell lines were maintained in a humidified CO2 incubator at 37 °C and 5% CO2.
5.2. Microfluidic device
To develop the PDAC model, we adopted a commercially available microfluidic device (DAX-1, AIM Biotech Pte. Ltd.). The device is made with a cyclic olefin polymer (COC) sealed with an oxygen-permeable membrane and consists of two lateral channels, 500 μm wide, and a central region, 1.30 mm wide, divided by an array of triangular pillars placed at a distance of 100 μm (Fig. 1A). This allows the physical and molecular interaction between the different cell types in the system. The device presents a height of 250 μm. The two lateral compartments were used to simulate the vascular and stromal environment, respectively, whereas the central channel was used to host cancer cells embedded in a collagen matrix to mimic the malignant tissue.
5.3. Embedding cancer cells into the matrix
The 3 mg ml−1 collagen solution was prepared by mixing 10× phosphate-buffered saline (PBS) with phenol red (Life technologies), 0.5 M NaOH (Sigma Aldrich), deionized water (Gibco), and Collagen type I from rat tail (Corning Life Science).68 PANC-1 cells were labeled with the Cell Tracker Orange CMRA dye (Invitrogen) at 10 μM in 1× PBS (Gibco). 1 × 106 PANC-1 cells, dispersed in the collagen solution, were seeded in the central chamber of the devices. The devices were then placed into their dedicated holders and sterile water was added into the designated reservoirs to prevent dehydration of the hydrogel. The devices were incubated for about 30 min at 37 °C and 5% CO2 to allow collagen polymerization before hydration of the media channels. EGM-2 medium (120 μL) was added to each medium channel.
5.4. Endothelial and stroma cell culture in device
To promote cell adhesion and the formation of the HUVEC monolayer, 50 μg ml−1 of fibronectin (Sigma Aldrich) was injected into one of the two lateral channels and incubated for 1 h at 37 °C and 5% CO2. After washing the fibronectin in excess, GFP+ HUVEC suspension in EGM-2 (20 μl) at 3 × 106 cells per ml was introduced into the same fluidic compartment to form a uniform endothelial monolayer around the channel's wall. Similarly, PSC suspension in EGM-2 (20 μl) at 1 × 106 cells per ml was introduced into the other lateral fluidic channel. The devices were kept in the incubator at 37 °C and 5% CO2. EGM-2 medium was refreshed daily.
5.5. Permeability assay
To measure the permeability of the endothelial barrier, 70 kDa FITC-Dextran (Sigma Aldrich) was added to the endothelial microchannel at a concentration of 100 μg ml−1. The system was then imaged for 30 min using an inverted fluorescence microscope. The vascular permeability coefficient P to dextran was quantified by ImageJ software considering six regions of interest (ROIs), three into the vascular channel (intravascular), and three into the gel channel (extravascular) as described in Fig. S1, ESI.† Briefly, to calculate P, the following equation was derived from Fick's diffusion equation as previously described:28
where P is the diffusive permeability coefficient (μm s−1), If it is the mean fluorescence intensity of the extravascular ROIs at the final time, Ii is the mean fluorescence intensity of the extravascular ROIs at the initial time. IV is the mean fluorescence intensity in the vascular ROIs. Δt is the time difference between the analyzed frames, and w is the width of the ROI.
5.6. Human T cell isolation and activation
T cells were isolated from blood cones from the Singapore's Health Sciences Authority (project reference no: 201306-04). Peripheral blood mononuclear cells (PBMCs) were isolated from whole blood cones by Ficoll–Paque density gradient centrifugation (GE Healthcare), and T cells were positively isolated using anti-CD3 microbeads (Miltenyi Biotec, Auburn, CA). Cell viability was assessed by Trypan blue and the average percentage of viable cells was 88.80 ± 7.628%. Cells were kept in Roswell Park Memorial Institute (RPMI) culture medium containing 30 U ml−1 of recombinant human IL-2 (Miltenyi Biotec) until experiments were conducted.
T cells were activated with anti-CD3/CD28 magnetic beads (Dynabeads, Thermo Fisher). Dynabeads were first washed by resuspending the beads, vortexing for 30 s, and transferring the desired volume into a new tube (1.25 μl per 105 T cells) with 1 ml of buffer. Then, the tube was placed in a magnetic field for 1 min to separate the supernatant that was discarded. Washed Dynabeads were resuspended in the same volume of culture media as the initial volume of Dynabeads taken from the vial and were then added to the isolated CD3+ population of T cells (1 × 106 ml−1) for 5 days at 37 °C and 5% CO2 with 30 U ml−1 of IL-2 in RPMI medium. After 3 days, the cell density was adjusted back to 1 × 106 ml−1 in RPMI medium containing 30 U ml−1 of IL-2. After 5 days, Dynabeads were removed using the magnet and T cells were inserted into the device.
5.7. T cell seeding
T cells were collected from the culture flasks and were stained with CellTrace™ Violet (Thermo Fisher) at 5 μM in 1× PBS for 10 min, washed and resuspended in EGM-2 media containing 30 U ml−1 IL-2. 30 μl of T cell suspension in EGM-2 at 8 × 106 cell per ml were added in the vascular channel filled with HUVECs; 20 μl of medium were removed from the opposite outlet to create a pressure gradient and bring the T cells close to the interface with the gel region. The devices were maintained in a CO2 incubator at 37 °C and 5% CO2 for 24 h or 48 h until imaging. The imaging acquisition was performed using an Opera Phenix High Content Screening confocal system (PerkinElmer) and the acquired images were analyzed by IMARIS software (Bitplane).
5.8. Immunofluorescence staining and imaging
After 5 days, each compartment of the device was washed once with 1× PBS and fixed with 4% paraformaldehyde (PFA) (Sigma Aldrich) for 15 min at room temperature. After washing twice with 1× PBS, the channels were filled with 0.1% solution of Triton X-100 (Sigma Aldrich) in 1× PBS and incubated for 10 min at room temperature to allow cell membrane permeabilization. Next, samples were blocked with 3% bovine serum albumin (Life technologies) for 2 h at room temperature and then incubated with a monoclonal anti-human VE-Cadherin antibody (1
:
100, Enzo technology) overnight at 4 °C. Finally, devices were incubated with an Alexa Fluor 647 anti-mouse secondary antibody (1
:
500, Enzo technology) for 1 h at room temperature. Cell nuclei were stained with DAPI (5 mg ml−1, Invitrogen). Images were acquired using an FV1000 or FV3000RS confocal inverted microscope (Olympus).
5.9. Luminex multiplex immunoassay
The Luminex xMAP bead-based technology was used to assess the cytokine expression in the cell culture supernatant after 48 h from T cell injection. The samples were obtained from three devices for each culture condition. Each sample was used undiluted and run in duplicate. The assay was run with the Human CD8+ T cell MAG premixed panel including the following analytes: granzyme A, granzyme B, interferon γ (IFNγ), interleukin-2 (IL-2), IL-4, IL-6, perforin, sCD137, sFas, sFasL, tumor necrosis factor-α (TNF-α). Standards were included in the assay to generate a standard curve for each analyte. Results were reported as analyte concentrations in pg ml−1.
5.10. Flow cytometry
At the end of each experiment, cells cultured in the devices were retrieved to perform flow cytometry analysis. Each compartment of the microfluidic device was washed with 1× PBS and cells embedded in the gel compartment were dissociated from the matrix by an enzymatic treatment with Collagenase type I (Gibco) at 1.5 mg ml−1, incubating the devices for 5 min at 37 °C and 5% CO2. Cells were collected in Eppendorf tubes and prepared for the flow cytometry analysis.
Cell concentration was adjusted at 1 × 106 cell per ml in cold FACS buffer. Conjugated primary antibodies (1
:
500) were added to the cell suspension for 20 min at 4 °C protected from light. Then, cells were washed once by 5 min centrifugation and resuspended in 200 μl of 2% PFA for 40 min at room temperature protected from light. Cells were washed using FACS buffer and kept protected from light until the analysis was performed. Finally, cells were incubated with anti-human monoclonal antibodies for CD4 (FITC), CD8 (AmCyan), CD3 (APC), PD-1 (Texas Red), TIM3 (eFluor 605), (Thermo Fisher). Cell fluorescence was measured using a BD Fortessa LSR cell analyzer (BD Biosciences) from SIgN Flow Cytometry Platform. Data were analyzed using FlowJo software (FlowJo, LLC).
5.11. Statistical analysis
Statistical analysis of each experiment was performed using Prism 8.2 (GraphPad Software). A comparison between the different conditions was performed by Student's t-test or ANOVA followed by the Tukey-HSD post-hoc test when appropriate. Results are presented with bar plot as mean + SD or violin plots showing the probability density for each value. Each dot of the violin plot represents the number of T cells counted in the central region. The significant threshold was considered p < 0.05; ns represents not significant, * represents p ≤ 0.05, ** represents p ≤ 0.01, *** represents p ≤ 0.001 and **** represents p ≤ 0.0001.
Author contributions
H. M., Y. J. T. performed experiments, analyzed data and drafted the manuscript. A. S. M. T., D. Z. M. T., helped in performing experiments and analyzing data. A. P. and P. D. supervised the project and reviewed the manuscript. G. A. supervised the project, designed the study, contributed to analyze data and write the manuscript. All authors approved the final version of the manuscript.
Conflicts of interest
A. P. is a consultant for AIM Biotech Pte. Ltd.
Acknowledgements
This work was funded by the Biomedical Research Council (BMRC), Agency for Science, Technology and Research (A*STAR). This project used the SIgN Flow Cytometry Platform, SIgN, A*STAR Research Entities supported by the National Research Foundation, Singapore, under its Shared Infrastructure Support (SIS), Immunomonitoring Service Platform (ISP) (NRF2017-SISFP09). The project used the confocal microscope at SIgN, A*STAR Research Entities and the confocal microscope at RSC, A*STAR Microscopy Platform, Light Microscopy facility. We would like to thank Akhila Balachander and Shuping Lin for their support with the microscope trainings. The project used the SIgN Multiplex Analysis of Proteins (MAP) Facility to run the cytokine analysis and we would like to thank Wilson How, Norman Leo Fernandez and Olaf Rötzschke for their helpful suggestions.
References
- P. Rawla, T. Sunkara and V. Gaduputi, World J. Oncol., 2019, 10, 10 CrossRef PubMed.
- R. L. Siegel, K. D. Miller and A. Jemal, Cancer J. Clin., 2020, 70, 7 CrossRef PubMed.
- A. Adamska, A. Domenichini and M. Falasca, Int. J. Mol. Sci., 2017, 18, 1338 CrossRef PubMed.
- D. Sakellariou-Thompson, M.-A. Forget, C. Creasy, V. Bernard, L. Zhao, Y. U. Kim, M. W. Hurd, N. Uraoka, E. R. Parra and Y. A. Kang, Clin. Cancer Res., 2017, 23, 7263 CrossRef CAS PubMed.
- M. M. Boyiadzis, J. M. Kirkwood, J. L. Marshall, C. C. Pritchard, N. S. Azad and J. L. Gulley, J. Immunother. Cancer, 2018, 6, 1 Search PubMed.
- L. R. Brunet, T. Hagemann, A. Gaya, S. Mudan and A. Marabelle, OncoImmunology, 2016, 5, e1112942 CrossRef PubMed.
- D. Mahadevan and D. D. Von Hoff, Mol. Cancer Ther., 2007, 6, 1186 CrossRef CAS PubMed.
- S. Suklabaidya, P. Dash, B. Das, V. Suresh, P. K. Sasmal and S. Senapati, Lab. Invest., 2018, 98, 27 CrossRef CAS PubMed.
- J. Schnittert, R. Bansal and J. Prakash, Trends Cancer, 2019, 5, 128 CrossRef CAS PubMed.
- A. Cannon, C. Thompson, B. R. Hall, M. Jain, S. Kumar and S. K. Batra, Genes Cancer, 2018, 9, 78 CrossRef CAS PubMed.
- D. Klein, Front. Oncol., 2018, 8, 367 CrossRef PubMed.
- A. E. Dirkx, M. G. oude Egbrink, M. J. Kuijpers, S. T. van der Niet, V. V. Heijnen, J. C. Bouma-ter Steege, J. Wagstaff and A. W. Griffioen, Cancer Res., 2003, 63, 2322 CAS.
- K. Castermans and A. W. Griffioen, Biochim. Biophys. Acta, Rev. Cancer, 2007, 1776, 160 CrossRef CAS PubMed.
- I. M. Stromnes, P. D. Greenberg and S. R. Hingorani, Clin. Cancer Res., 2014, 20, 5157 CrossRef CAS PubMed.
- C. E. Clark, S. R. Hingorani, R. Mick, C. Combs, D. A. Tuveson and R. H. Vonderheide, Cancer Res., 2007, 67, 9518 CrossRef CAS PubMed.
- E. J. Wherry, Nat. Immunol., 2011, 12, 492 CrossRef CAS PubMed.
- A. D. Wells, J. Immunol., 2009, 182, 7331 CrossRef CAS PubMed.
- H. K. Hwang, H. I. Kim, S. H. Kim, J. Choi, C. M. Kang, K. S. Kim and W. J. Lee, Oncol. Lett., 2016, 12, 4477 CrossRef CAS PubMed.
- M. Swayden, P. Soubeyran and J. Iovanna, Front. Oncol., 2019, 9, 1443 CrossRef PubMed.
- W. Qiu and G. H. Su, Cancer Metastasis Rev., 2013, 32, 83 CrossRef CAS PubMed.
- S. N. Bhatia and D. E. Ingber, Nat. Biotechnol., 2014, 32, 760 CrossRef CAS PubMed.
- G. Mehta, A. Y. Hsiao, M. Ingram, G. D. Luker and S. Takayama, J. Controlled Release, 2012, 164, 192 CrossRef CAS PubMed.
- S. Tsai, L. McOlash, K. Palen, B. Johnson, C. Duris, Q. Yang, M. B. Dwinell, B. Hunt, D. B. Evans and J. Gershan, BMC Cancer, 2018, 18, 335 CrossRef PubMed.
- J. Drost and H. Clevers, Nat. Rev. Cancer, 2018, 18, 407 CrossRef CAS PubMed.
- G. Adriani, A. Pavesi, A. T. Tan, A. Bertoletti, J. P. Thiery and R. D. Kamm, Drug Discovery Today, 2016, 21, 1472 CrossRef CAS PubMed.
-
S. W. Lee, G. Adriani, R. D. Kamm and M. R. Gillrie, in Tumor Microenvironment, ed. A. Birbrair, Springer, Cham, 2020, vol. 1224, p. 87 Search PubMed.
- S. Maharjan, B. Cecen and Y. S. Zhang, Small Methods, 2020, 4, 2000235 CrossRef CAS PubMed.
- H. Mollica, R. Palomba, R. Primavera and P. Decuzzi, ACS Biomater. Sci. Eng., 2019, 5, 4834 CrossRef CAS PubMed.
- H. Mollica, A. Coclite, M. E. Miali, R. C. Pereira, L. Paleari, C. Manneschi, A. DeCensi and P. Decuzzi, Biomicrofluidics, 2018, 12, 042205 CrossRef PubMed.
- A. Miermont, S. W. L. Lee, G. Adriani and R. D. Kamm, Sci. Rep., 2019, 9, 1 CAS.
- A. Mercurio, L. Sharples, F. Corbo, C. Franchini, A. Vacca, A. Catalano, A. Carocci, R. D. Kamm, A. Pavesi and G. Adriani, Front. Pharmacol., 2019, 10, 349 CrossRef CAS PubMed.
- S. Bersini, A. Miermont, A. Pavesi, R. D. Kamm, J. P. Thiery, M. Moretti and G. Adriani, Oncotarget, 2018, 9, 36110 CrossRef PubMed.
- S. W. L. Lee, R. J. Seager, F. Litvak, F. Spill, J. L. Sieow, P. H. Leong, D. Kumar, A. S. M. Tan, S. C. Wong, G. Adriani, M. H. Zaman and R. D. Kamm, Integr. Biol., 2020, 12, 90 CrossRef PubMed.
- I. K. Zervantonakis, S. K. Hughes-Alford, J. L. Charest, J. S. Condeelis, F. B. Gertler and R. D. Kamm, Proc. Natl. Acad. Sci. U. S. A., 2012, 109, 13515 CrossRef PubMed.
- J. Bai, G. Adriani, T.-M. Dang, T.-Y. Tu, H.-X. L. Penny, S.-C. Wong, R. D. Kamm and J.-P. Thiery, Oncotarget, 2015, 6, 25295 CrossRef PubMed.
- H. L. Penny, J. L. Sieow, G. Adriani, W. H. Yeap, P. See Chi Ee, B. San Luis, B. Lee, T. Lee, S. Y. Mak and Y. S. Ho, OncoImmunology, 2016, 5, e1191731 CrossRef PubMed.
- A. Pavesi, A. T. Tan, S. Koh, A. Chia, M. Colombo, E. Antonecchia, C. Miccolis, E. Ceccarello, G. Adriani, M. T. Raimondi, R. D. Kamm and A. Bertoletti, JCI Insight, 2017, 2, e89762 CrossRef PubMed.
-
G. Adriani, A. Pavesi and R. D. Kamm, in Methods in Cell Biology, ed. J. Doh, D. Fletcher and M. Piel, Elsevier, 2018, vol. 146, p. 199 Search PubMed.
- S. W. L. Lee, G. Adriani, E. Ceccarello, A. Pavesi, A. T. Tan, A. Bertoletti, R. D. Kamm and S. C. Wong, Front. Immunol., 2018, 9, 416 CrossRef PubMed.
- D. Di Mascolo, S. Varesano, R. Benelli, H. Mollica, A. Salis, M. R. Zocchi, P. Decuzzi and A. Poggi, Cancers, 2020, 12, 104 CrossRef CAS PubMed.
- S. Parlato, A. De Ninno, R. Molfetta, E. Toschi, D. Salerno, A. Mencattini, G. Romagnoli, A. Fragale, L. Roccazzello and M. Buoncervello, Sci. Rep., 2017, 7, 1 CrossRef PubMed.
- E. Agliari, E. Biselli, A. De Ninno, G. Schiavoni, L. Gabriele, A. Gerardino, F. Mattei, A. Barra and L. Businaro, Sci. Rep., 2014, 4, 1 Search PubMed.
- K. Ley, C. Laudanna, M. I. Cybulsky and S. Nourshargh, Nat. Rev. Immunol., 2007, 7, 678 CrossRef CAS PubMed.
- A. Aung, V. Kumar, J. Theprungsirikul, S. K. Davey and S. Varghese, Cancer Res., 2020, 80, 263 CrossRef CAS PubMed.
- R. J. Buckanovich, A. Facciabene, S. Kim, F. Benencia, D. Sasaroli, K. Balint, D. Katsaros, A. O'Brien-Jenkins, P. A. Gimotty and G. Coukos, Nat. Med., 2008, 14, 28 CrossRef CAS PubMed.
- A. Trickett and Y. L. Kwan, J. Immunol. Methods, 2003, 275, 251 CrossRef CAS PubMed.
- J. D. Miller, S. E. Clabaugh, D. R. Smith, R. B. Stevens and L. E. Wrenshall, Immunol. Cell Biol., 2012, 90, 159 CrossRef CAS PubMed.
-
C. R. Kleiveland, in The impact of food bioactives on health, Springer, Cham, 2015, p. 161 Search PubMed.
- Y. Li and R. J. Kurlander, J. Transl. Med., 2010, 8, 104 CrossRef PubMed.
- Y. Agata, A. Kawasaki, H. Nishimura, Y. Ishida, T. Tsubat, H. Yagita and T. Honjo, Int. Immunol., 1996, 8, 765 CrossRef CAS PubMed.
- R. J. Greenwald, G. J. Freeman and A. H. Sharpe, Annu. Rev. Immunol., 2005, 23, 515 CrossRef PubMed.
- A. C. Anderson, N. Joller and V. K. Kuchroo, Immunity, 2016, 44, 989 CrossRef CAS PubMed.
- J. Mai, A. Virtue, J. Shen, H. Wang and X.-F. Yang, J. Hematol. Oncol., 2013, 6, 61 CrossRef PubMed.
- S. M. Albelda, P. M. Sampson, F. R. Haselton, J. McNiff, S. Mueller, S. Williams, A. Fishman and E. Levine, J. Appl. Physiol., 1988, 64, 308 CrossRef CAS PubMed.
- Y. T. Ho, G. Adriani, S. Beyer, P.-T. Nhan, R. D. Kamm and J. C. Y. Kah, Sci. Rep., 2017, 7, 1 CrossRef CAS PubMed.
- M. R. Dreher, W. Liu, C. R. Michelich, M. W. Dewhirst, F. Yuan and A. Chilkoti, J. Natl. Cancer Inst., 2006, 98, 335 CrossRef CAS PubMed.
- A. Armulik, G. Genové and C. Betsholtz, Dev. Cell, 2011, 21, 193 CrossRef CAS PubMed.
- E. Tomás-Bort, M. Kieler, S. Sharma, J. B. Candido and D. Loessner, Theranostics, 2020, 10, 5074 CrossRef PubMed.
- J. E. Smith-Garvin, G. A. Koretzky and M. S. Jordan, Annu. Rev. Immunol., 2009, 27, 591 CrossRef CAS PubMed.
- S. Simon and N. Labarriere, OncoImmunology, 2018, 7, e1364828 CrossRef PubMed.
- A. de la Zerda, M. J. Kratochvil, N. A. Suhar and S. C. Heilshorn, APL Bioeng., 2018, 2, 021501 CrossRef PubMed.
- N. Oppenheimer-Marks, L. S. Davis and P. E. Lipsky, J. Immunol., 1990, 145, 140 CAS.
- R. I. Brezinschek, P. E. Lipsky, P. Galea, R. Vita and N. Oppenheimer-Marks, J. Immunol., 1995, 154, 3062 CAS.
- M. F. Krummel, F. Bartumeus and A. Gérard, Nat. Rev. Immunol., 2016, 16, 193 CrossRef CAS PubMed.
- B. A. Pereira, C. Vennin, M. Papanicolaou, C. R. Chambers, D. Herrmann, J. P. Morton, T. R. Cox and P. Timpson, Trends Cancer, 2019, 5, 724–741 CrossRef PubMed.
- Y. Fu, S. Liu, S. Zeng and H. Shen, Mol. Cancer, 2018, 17, 62 CrossRef PubMed.
- J. S. Wilson, R. C. Pirola and M. V. Apte, Front. Physiol., 2014, 5, 52 Search PubMed.
- Y. Shin, S. Han, J. S. Jeon, K. Yamamoto, I. K. Zervantonakis, R. Sudo, R. D. Kamm and S. Chung, Nat. Protoc., 2012, 7, 1247 CrossRef CAS PubMed.
Footnote |
† Electronic supplementary information (ESI) available. See DOI: 10.1039/d1bm00210d |
|
This journal is © The Royal Society of Chemistry 2021 |