DOI:
10.1039/D1AN00726B
(Paper)
Analyst, 2021,
146, 4495-4505
Rapid ultra-sensitive diagnosis of clostridium difficile infection using a SERS-based lateral flow assay†
Received
26th April 2021
, Accepted 19th June 2021
First published on 21st June 2021
Abstract
Clostridium difficile (C. diff) infection is one of the most contagious diseases associated with high morbidity and mortality rates in hospitalised patients. Accurate diagnosis can slow its spread by determining the most effective treatment. Herein, we report a novel testing platform as a proof-of-concept for the selective, sensitive, rapid and cost-effective diagnosis of C. diff infection (CDI) based on a duplex measurement. This was achieved by detecting two specific biomarkers, surface layer protein A (SlpA) and toxin B (ToxB), using a surface enhanced Raman scattering-based lateral flow assay (SERS-based LFA). The simultaneous duplex detection of SlpA with ToxB has not been described for the clinical diagnosis of CDI previously. The SlpA biomarker “AKDGSTKEDQLVDALA” was first reported by our group in 2018 as a species-specific identification tool. The second biomarker, ToxB, is the essential virulence biomarker of C. diff pathogenic strains and is required to confirm true infection pathogenicity. The proposed SERS-based LFA platform enabled rapid duplex detection of SlpA and ToxB on separate test lines using a duplex LF test strip within 20 minutes. The use of a handheld Raman spectrometer to scan test lines allowed for the highly sensitive quantitative detection of both biomarkers with a lowest observable concentration of 0.01 pg μL−1. The use of a handheld device in this SERS-based LFA instead of benchtop machine paves the way for rapid, selective, sensitive and cheap clinical evaluation of CDI at the point of care (POC) with minimal sample backlog.
1. Introduction
Clostridium difficile (C. diff) is a Gram-positive species of spore-forming bacteria, that is considered the main cause of infectious diarrhoea in hospitalised patients.1C. diff infection (CDI) can also cause a more serious bowel inflammation leading to fatal pseudomembranous colitis.1 The CDI can be easily transmitted from person-to-person by direct contact with contaminated objects and asymptomatic carriers. During the CDI, the glutamate dehydrogenase (GDH) enzyme level is elevated in stool samples by all C. diff strains, which may or may not be pathogenic, and can be used as an indicator for the presence of the infection.2 The main virulence factors associated with C. diff are toxins A/B which are secreted by pathogenic strains leading to cell rounding and ultimately cell death.3 Most pathogenic strains of C. diff produce both toxins (A+/B +) which are encoded by the two genes tcdA and tcdB, respectively. However, there have been numerous reports of the clinical importance of A-negative and B-positive (A−/B +) isolates, so toxin B must be detected to cover all pathogenic isolates.3–5 The detection of the toxins level during CDI to evaluate the infection severity remains controversial.6 Some preliminary studies suggested that the ability to quantify toxin levels in stool could potentially be clinically valuable to manage the infection and implement effective treatment protocols.6–9 As quantitative toxin assays become available, correlating toxins concentration with infection severity could improve the CDI assessment and treatment.6 In the last two decades, the prevalence of epidemic and antibiotic-resistant pathogenic strains of C. diff, that produce higher amounts of toxins than normal, has been correlated to the increased mortality rate that associated with severe CDI in Europe and North America.10–12 Despite the availability of therapies, treatment failure and recurrence are common.13 Therefore, the early, rapid and accurate diagnosis of CDI is essential for appropriate medical intervention and to initiate infectious control measures, such as cleaning and quarantine.5
The optimal method for CDI diagnosis remains debatable.13 The two classic methods used for C. diff diagnosis were stool analysis via toxigenic culture and cell culture cytotoxicity assay. While the toxigenic culture identifies only the toxigenic organism and not the toxin itself, the cell culture cytotoxicity assay can primarily detect the more potent toxin B.6 However, due to their complexity and delayed turnaround time, both methods are unsuitable for the clinical testing of CDI at POC.6 In 2013, a large UK-based study was carried out on 12
420 faecal samples to validate these two methods. The study considered both methods as having inadequate specificity, insufficient sensitivity and noted that most UK diagnostic laboratories do not use these tests.14 The study also indicated that the toxin detection is the most crucial step in the diagnosis of CDI, and concluded that the deficiencies of existing tests should drive further assay development.14 In the UK, the current testing and diagnosis protocol for CDI is a two-step testing algorithm, that consists of a GDH enzyme immunoassay (EIA) or molecular assay to screen samples, followed by toxins A/B EIA if the first test in the algorithm is positive.15 Accordingly, simple and user-friendly membrane EIA16 and commercially available lateral flow (LF)17 tests have been introduced to the diagnostic market for the simultaneous testing of GDH and toxins A/B in a single system (3 in 1). These tests allow clinical diagnosis of CDI in a short time, as well as determine if the infectious strain is secreting toxins or not. Despite the ease of use and the reasonable cost of these tests, they suffer from limitations, in particular, they can only indicate the presence of GDH and toxins A/B qualitatively as the results cannot be interpreted quantitatively. Therefore, they cannot be used to determine the extent of infection severity. Additionally, some isolates of Clostridium sordellii may react in the test due to the production of immunologically related toxins which can interfere with the test results.18 Furthermore, the antiserum against C. diff GDH has the potential to cross-react with GDH from other anaerobes.19 Therefore, the quantification capacity and selectivity of these tests remain controversial. In a previous study, we have reported SlpA “AKDGSTKEDQLVDALA” as a new specific biomarker for C. diff that can be used for centralised laboratory analysis using conventional enzyme-linked immunosorbent assay (ELISA) formats.12 We have successfully demonstrated that SlpA is considered as a unique biomarker for C. diff species only which presents in all C. diff strains sequenced to-date. We have also reported the successful generation of affinity reagents (antibodies), that displayed negligible cross-reactivity to other species which have closely related biomarker signatures as SlpA.12 Therefore, this species-specific recognition is an ideal candidate for C. diff diagnosis. We concluded that study with a future work scope of applying SlpA and its antibodies to a new assay for CDI that is compatible with POC testing in a lateral flow assay (LFA) format.
Conventional LFAs have many advantages such as visual interpretation of the results in a short time, long term stability and ease of use. However, they possess major limitations in terms of quantification capability and detection sensitivity.20,21 The LF strips are often used as qualitative screening tests where the test result is interpreted via monitoring the colour change of the test lines by the naked eye. Therefore, the sensitivity of the test can be inadequate for the early diagnosis of target analytes especially in POC application.20 To overcome these limitations, surface enhanced Raman scattering (SERS) can be integrated with LFAs in a single platform. SERS is a powerful analytical technique because of its specific fingerprint spectrum and sensitivity.22 It can be used for the ultra-sensitive quantification of different bacterial biomarkers to evaluate the bacterial infection severity.23 Besides its eminent sensitivity, SERS offers good specificity and strong multiplexing ability.24,25 Therefore, the integration of SERS with LFAs can improve the detection sensitivity and quantification capability of the conventional POC-based LFA strips.23 The approach of integrating SERS with a LFA in one platform has been extensively reported for on-site and facile diagnosis of bacterial infections and disease biomarkers to enhance the detection limits.23,26–29
Herein we present for the first time, a proof-of-concept novel duplex SERS-based LFA for CDI diagnosis through the sensitive detection of SlpA and ToxB. The simultaneous detection of ToxB and SlpA instead of GDH in this duplex test can indicate the presence of CDI and toxigenicity without any cross-reactivity from other species, which is a potential risk with GDH assays.19 For this purpose, we developed selective SERS nanotags for SlpA and ToxB by functionalising Raman reporter-labelled gold nanoparticles with highly selective antibodies for the biomarkers. The SERS nanotags were then mixed and used as detection probes for SlpA and ToxB in buffer solution and synthetic stool matrix. By using duplex LF test strips, SlpA and ToxB were visually detected within 20 minutes via a colour change in the test line zones. The SERS scan for these test lines using a handheld Raman spectrometer enabled sensitive quantitative detection for both biomarkers with a lowest observable concentration of 0.01 pg μL−1. Thus, this method enabled selective and ultra-sensitive quantitative detection of SlpA and ToxB in a short time using a stable, simple, cost-effective and user-friendly platform.
To the best of our knowledge, the duplex diagnosis of CDI by monitoring SlpA and ToxB has not been described before. Apart from our previously reported method using sandwich ELISA,12 no analytical method has been reported for the detection of SlpA. Moreover, SERS has not previously been used for the detection of ToxB. In addition, the use of a handheld Raman spectrometer with this novel duplex LFA, paves the way to move the C. diff diagnostic test from localised laboratories to POC application.30–32 This would allow hospital staff to implement infectious disease control measures in a timely manner, which could lead to more cost-effective systems.33
2. Experimental
2.1. Chemicals and materials
Sodium tetrachloroaurate(III) dihydrate, sodium citrate tribasic dihydrate, sodium tetraborate, boric acid, bovine serum albumin (BSA), polyvinylpyrrolidone (PVP), tris(hydroxymethyl)aminomethane (Tris), skim milk powder, Empigen detergent and anti-Mouse IgG (whole molecule) antibody produced in rabbit were purchased from Sigma Aldrich (UK). Malachite green isothiocyanate (MGITC) was purchased from Thermo fisher scientific (UK). Native C. diff toxin B protein was purchased from Abcam (UK). C. diff toxin B mouse monoclonal detection antibody (mAbToxB1) and capture antibody (mAbToxB2) were sourced from BBI solutions (UK). They are a matched pair with product numbers BM347-N4A8 and BM347-T4G1, respectively. SlpA, SlpA mouse monoclonal detection antibody (mAb521) and IgG polyclonal SlpA capture antibody (pAbSlpA) were harvested and purified by the research team as described previously.12 Based on the identified biomarker amino acid sequence, a linear peptide was synthesized to produce mAb521 through the hybridoma technique (Abmart). Human-based synthetic stool matrix of item number 01.380.05 was purchased from ClaremontBio (USA). The stool matrix was developed for research purposes to mimic human stool. The basic components are a collection of key possible PCR-inhibitory compounds at relevant amounts in actual stool based on literature. Protein LoBind tubes were sourced from Eppendorf (UK). UniSart nitrocellulose membrane CN95 was purchased from Sartorius (UK). Lateral flow backing card 60 mm × 300 mm was obtained from Kenosha C.V. (The Netherlands). Whatman CF6 absorbent pad was purchased from GE Healthcare (UK).
2.2. Preparation of lateral flow test strips
To prepare SlpA LF test strips, 1 mg mL−1 of pAbSlpA (pH 9.6) and 1 mg ml−1 of anti-mouse IgG antibody (pH 9.6) were sprayed onto a the CN95 nitrocellulose membrane to give a test and control lines, respectively, using an Imagene IsoFlow flatbed dispenser (USA). The membrane was then air dried for 1 hour at room temperature. Next, the membrane was blocked with a mixture of 2% milk protein and 0.1% Empigen (pH 9.6) and allowed to dry at room temperature, then kept at 4 °C overnight. The membrane was then assembled with LF backing card and absorbent pad. Finally, the membrane was cut into strips of 4 mm width and stored at 4 °C. To prepare ToxB LF test strips, the same procedure that was used to prepare SlpA test strips was repeated again, except spraying 1 mg mL−1 of mAbToxB2 (pH 9.6) onto the test line.
To prepare the duplex LF test strips, the same procedure was repeated again and both SlpA and ToxB capture antibodies were sprayed over the membrane as two separate test lines for both biomarkers, alongside the control line.
2.3. Preparation of SERS nanotags
To prepare SlpA SERS nanotag, gold nanoparticles (AuNPs) of ∼47 nm size were first prepared using a citrate reduction method.34 Briefly, all glassware was cleaned first with aqua regia (HCl, HNO3 3
:
1, v/v) then washed thoroughly with distilled water. 55.5 g of sodium tetrachloroaurate(III) dihydrate was added to 500 mL of distilled water in a 3 necked round bottom flask and heated until boiling. Then, a solution of 66.5 g of sodium citrate tribasic dihydrate in 7.5 mL distilled water was added to the flask. The mixture was kept boiling for 15 minutes with continuous stirring, then allowed to cool to room temperature. The AuNPs were then characterised using extinction spectroscopy (Cary 60 UV-Vis, spectral bandwidth = 1.5 nm, Agilent Technologies), dynamic light scattering (DLS) measurement, zeta potential analysis (Zetasizer, Malvern) and scanning electron microscope (SEM) (Fig. S1, S2 and S3, respectively, ESI†). SlpA SERS nanotag was prepared by shaking 1 mL of AuNPs with 15 μL of 5 μM Raman reporter malachite green isothiocyanate (MGITC) for 15 minutes in a protein LoBind tube using a compact shaker at speed 200 rpm. Next, 100 μL of 20 mM borate buffer (pH 9), 25 μL of 1.5 μM mAb521 and 5 μL of 10% PVP were added to the solution with continuous shaking for 1 hour to attach the antibody onto the surface of the AuNPs by physical adsorption. To avoid any non-specific binding, 100 μL of 1% BSA was added to the mixture with continuous shaking for 30 minutes to block the bare sites on the AuNPs surface. The mixture was then centrifuged at 5000 rpm for 30 minutes to remove excess unattached reagents. After removing the supernatant layer, the pellet was briefly vortexed and re-dispersed into 20 mM borate buffer (pH 9). Finally, 10× concentrated SERS nanotag solution was obtained and kept at 4 °C ready for use. ToxB SERS nanotag solution was prepared following the same preparation protocol of SlpA SERS nanotag using 10 μL of 2 μM mAbToxB1. The prepared SERS nanotags were then characterised using extinction spectroscopy, DLS measurement and zeta potential analysis.
2.4. SERS calibration curves for SlpA and ToxB
To construct SERS calibration curves for SlpA and ToxB, serial dilutions of each biomarker in 0.01 M Tris buffer (pH 8.4) were mixed with their corresponding SERS nanotags in separate LoBind tubes for 5 minutes, leading to the formation of immuno-complexes between the targets and their corresponding SERS nanotags. The resulting complex solutions were then placed into a 96 well-plate and the LF test strips of SlpA and ToxB were dipped into the assigned wells for 10 minutes. Accordingly, the test lines became visible to the naked eye. Finally, the excess complex solutions continued to flow over the strips forming a red colour on the control line. To avoid any Raman signal background for the test lines, the LF strips were then washed by dipping into 0.01 M Tris buffer (pH 8.4) for 5 minutes, then allowed to dry at room temperature. Strips were then fixed onto an in-house 3D printed Raman aperture adaptor that was used as a sample holder35 and the test lines were scanned using a handheld Raman spectrometer (CBEx, Snowy Range Instruments, USA). All the SERS measurements were carried out using orbital raster scanning (ORS) mode over the range 600–1800 cm−1 using the Peak 1.3.68 software. A 638 nm laser excitation source with 5 mW of laser power was used with an acquisition time of 0.5 s. The collected spectra were then baseline corrected using MatLab software. Finally, the average intensity of the SERS signal of the MGITC Raman peak at 1620 cm−1 was plotted against SlpA concentration (0.01–200 pg μL−1) and ToxB concentration (0.01–400 pg μL−1).
2.5. Control study
To confirm the specificity of the LF strips towards their targets, the strips were tested against different targets. 200 pg μL−1 of SlpA and 400 pg μL−1 of ToxB were used as negative controls for ToxB and SlpA strips, respectively. In addition, 0.01 M Tris buffer (pH 8.4) was also tested as a blank control for both biomarkers’ strips. The same procedure described in the previous section was repeated and the test lines were scanned using a handheld Raman spectrometer to monitor the SERS signal.
2.6. Duplex detection and clinical application
In order to perform duplex detection of both biomarkers, 8 different samples (samples 1–8 in Table 1) were prepared in 0.01 M Tris buffer (pH 8.4) by blending different concentrations of SlpA and ToxB together. Each prepared sample was then added to a mixture of SlpA and ToxB SERS nanotags in a separate LoBind tubes for 5 minutes to allow for the formation of the immuno-complexes. The mixtures were then transferred to a 96 well-plate and the duplex LF test strips were dipped into the wells for 10 minutes. A red colour started to appear first on the test lines of SlpA then ToxB and finally on the control line. The strips were then washed by dipping into 0.01 M Tris buffer (pH 8.4) for 5 minutes, air dried and scanned using a handheld Raman spectrometer for their test lines.
Table 1 Concentrations of the samples used for duplex detection
Sample no. |
SlpA (pg μL−1) |
ToxB (pg μL−1) |
Denotes the samples spiked in synthetic stool matrix equivalent to clinical sample.
|
1 |
200 |
400 |
2 |
100 |
400 |
3 |
50 |
400 |
4 |
25 |
400 |
5 |
200 |
400 |
6 |
200 |
200 |
7 |
200 |
100 |
8 |
200 |
50 |
9a |
0.5 |
0.5 |
10a |
40 |
40 |
11a |
80 |
80 |
To demonstrate the feasibility of using the duplex LF test strip for the clinical diagnosis of C. diff in a biological specimen, a blank 1% synthetic stool matrix solution was spiked with 3 different samples (samples 9–11 in Table 1). The same procedure used to perform the duplex detection in buffer solution was repeated again and the strips were scanned by a handheld Raman spectrometer to acquire the SERS signals of the test lines. Finally, the SERS signal intensities of the MGITC Raman peak at 1620 cm−1 were used for the quantification of spiked samples in the synthetic stool matrix.
3. Results and discussion
3.1. Synthesis of SERS nanotags for SlpA and ToxB
The principle of the SERS-based LFA platform for CDI diagnosis is illustrated in Fig. 1. In this method, selective SERS nanotags were developed and used as duplex detection probes for SlpA and ToxB in buffer solution and synthetic stool samples. The SERS nanotags were used to improve the specificity and sensitivity performance of the assay in a quantitative manner. In order to obtain a reproducible enhancement for the SERS signal, the SERS nanotags were carefully designed. The SERS nanotags for both targets were prepared in a similar manner, utilising different antibodies for each target. Gold nanoparticles (AuNPs) were selected as the source of enhancement due to their high stability against oxidation, high extinction cross-section in the visible spectral range and their easy functionalisation with different biomolecules using established reactions.36,37 For the labelling of the AuNPs, malachite green isothiocyanate (MGITC) was selected as the Raman reporter because the isothiocyanate group in its structure has a strong coupling affinity towards AuNPs, allowing its anchoring onto the AuNPs surface resulting in a stable SERS signal.38 After conjugation to the AuNPs surface, the structure of MGITC is locked in its π-conjugated form and will no longer be affected by pH changes.38 In addition, the prominent characteristic Raman peak of MGITC at 1620 cm−1 (aromatic ring stretching) allows accurate SERS quantification.39
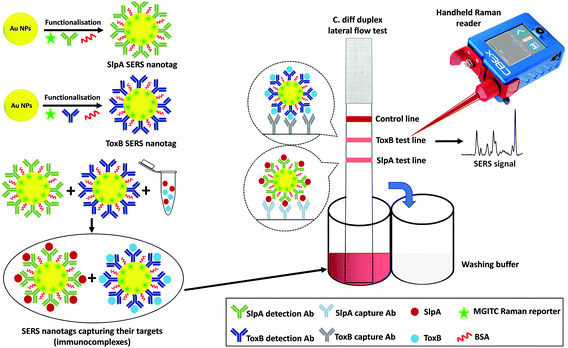 |
| Fig. 1 Schematic representation for the duplex detection of SlpA and ToxB by SERS-based LFA. | |
For the selective capture of SlpA and ToxB from buffer solution and synthetic stool matrix, the labelled-AuNPs were functionalised with highly specific antibodies for the targets via passive adsorption due to the electrostatic and hydrophobic interactions between the AuNPs and the antibodies.20,21 Polyvinylpyrrolidone (PVP) was then added to quench the nanoparticles aggregation and to stabilize the SERS nanotags, as well as to improve their flow over the LF strips.40,41 After treatment with PVP, BSA was added to backfill any bare sites on the surface of the AuNPs and avoid non-specific absorbance of any interfering molecules that may be present in the sample matrix.21 Finally, the SERS nanotags were centrifuged to remove any excess unattached reagents and the pellets were resuspended in borate buffer. The use of borate buffer was previously reported to show a remarkable performance in LFA.42–44 Its utilisation generated highly stable SERS nanotags and stronger visual intensity for the test lines compared to other tested buffers, resulting in a higher overall sensitivity for the assay. The conjugation of antibodies onto the AuNPs surface was monitored by comparing the extinction spectra, DLS and zeta potential of bare AuNPs and the SERS nanotags of both biomarkers. As shown in Fig. S1 (ESI†), a band shift was observed in the AuNPs extinction spectrum from 528 nm to 534 and to 531 nm after producing the SERS nanotags for SlpA and ToxB, respectively. These shifts were attributed to the increase in AuNPs size. The DLS measurements showed that the average size of the AuNPs increased from 47.5 nm to 85.5 nm and to 79.8 nm after the synthesis of the SERS nanotags of SlpA and ToxB, respectively (Fig. S2A, ESI†). Additionally, the average zeta potential of the AuNPs changed from −45.2 mV to nearly −12 mV after producing both SERS nanotags (Fig. S2B†). All these experimental findings can be used as an evidence for the successful attachment of the antibodies and Raman reporter onto the AuNPs surface.21,41
3.2. Quantitative SERS detection of SlpA and ToxB
The principle of this SERS-based LFA is depending on the formation of a sandwich complex forms onto the test lines of the LF strips following a dipstick format.45 Each SERS nanotag was mixed with its corresponding target for 5 minutes to enable the selective capture of the analytes forming immuno-complexes.30 Each biomarker's LF strips were then dipped into the assigned immuno-complex solutions in the 96 well-plate for 10 minutes to allow the solutions to migrate upward by capillary force. This led to formation and accumulation of sandwich complexes on the test lines due to the presence of pre-immobilised capture antibodies. Accordingly, red coloured test lines were developed and could be visually identified by the naked eye. The remaining immuno-complex solutions continued to flow and were non-specifically captured by the anti-mouse IgG antibody on the control line forming a red colour. It was found that the colour strength of the test lines increased with the increase in the biomarkers concentration. However, this colour change was observed only for high concentrations of SlpA (≥∼25 pg μL−1) and ToxB (≥∼100 pg μL−1) as shown in Fig. 2A and B insets, respectively. The control lines remained colored for all concentrations, indicating that the LF strips were working correctly.30 The LF strips were then washed out with 0.01 M Tris buffer (pH 8.4) for 5 minutes to remove the excess immuno-complex solutions from the strips surface to avoid the Raman background signal that can interfere with the test lines scan. This enabled highly sensitive quantitative detection for both biomarkers test lines using a handheld Raman spectrometer to acquire their SERS signals in 0.5 s. Each test line was scanned in orbital raster scanning (ORS) mode to acquire the average SERS signal from test line zones and to reduce the SERS signal variability between repeated scans.46–48 As shown in Fig. 2A–D, the mean SERS signal intensity of the peak at 1620 cm−1 (n = 3) was found to increase with SlpA and ToxB concentrations in the range 0.01–200 and 0.01–400 pg μL−1, respectively. The lowest observable concentration by SERS was 0.01 pg μL−1. The insets in Fig. 2C and D demonstrates the linear dynamic ranges for the quantification of both biomarkers. Theses dynamic ranges included five concentrations for each biomarker. These concentrations were 0.1, 1, 25, 50 and 100 pg μL−1 for SlpA and 0.1, 1, 50, 100 and 200 for ToxB (Fig. S4, ESI†). The relationships between the SERS signal intensity of the peak at 1620 cm−1 and the concentrations of SlpA and ToxB were found to follow the linear regression equations y = 41.44x + 1420 (R2 = 0.9907) and y = 18.78x + 2549.3 (R2 = 0.9816), respectively. The results demonstrated that the SERS-based LFA was much more sensitive than visual detection of the LFA and SERS allowed quantification beyond what was visible to the naked eye, while maintaining the simplicity and speed of the analysis.
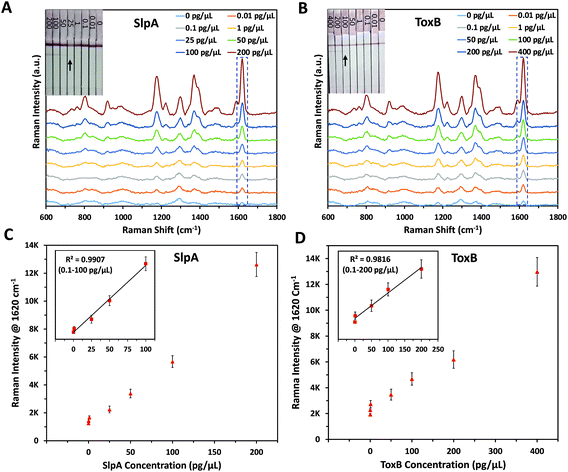 |
| Fig. 2 Raman spectra of (A) SlpA SERS nanotag mixed with different concentrations of SlpA samples (0–200 pg μL−1), (B) ToxB SERS nanotag mixed with different concentrations of ToxB samples (0–400 pg μL−1) on LF strips using handheld Raman spectrometer. The insets show photographic pictures of different biomarkers concentration on their LF strips demonstrating a visual detection limit of 25 pg μL−1 (SlpA) and 100 pg μL−1 (ToxB). Corresponding SERS calibration curves for (C) SlpA and (D) ToxB. The insets show the linear dynamic ranges for SlpA (0.1–100 pg μL−1) and ToxB (0.1–200 pg μL−1), respectively. Error bars indicate standard deviations from three measurements. All the SERS measurements were carried out using a handheld Raman spectrometer equipped with a 638 nm laser excitation source at 5 mW laser power with an acquisition time of 0.5 s at ORS mode. | |
As mentioned before, the quantitative detection of SlpA has been reported only in our previous study using ELISA with a LOD of 12.4 pg μL−1.12 By using this SERS-based LFA, the lowest detectable concentration was reduced by more than three orders of magnitude to reach 0.01 pg μL−1. Additionally, no SERS method has previously been published for the quantification of ToxB. There are some previous reports for ToxB detection, such as: ELISA,13 polymerase chain reaction,49,50 single-molecule counting technology,51 loop-mediated isothermal amplification,52 electrochemical and fluorescence assays.53–55 These methods showed a notable detection sensitivity with a high reproducibility. However, they suffer from lengthy and often complicated preparation procedures that can increase the analysis time and backlog of samples. Additionally, they require the use of costly reagents and expensive instrumentation that need to be operated by trained technicians which increases the overall cost of the analysis. As indicated by the SERS quantification results, our SERS-based LFA platform compares favourably to the other detection methods in terms of sensitivity, simplicity and speed of the analysis. In addition, the use of handheld Raman instrument for the scanning of the paper-based surface demonstrates the capacity of the developed platform to be adopted for POC testing in a more cost-effective way.
3.3. Reproducibility and selectivity of the developed SERS-based LFA
In order to confirm the reproducibility of the proposed SERS-based LFA, five different SERS scans were carried out interdaily using five independent LF strips for each of the lowest and highest concentrations of SlpA (0.1 and 100 pg μL−1) and ToxB (0.1 and 200 pg μL−1) in their linear dynamic ranges. Each strip was scanned three times during the day. A group of 15 SERS measurements were collected for each concentration of the biomarkers using ORS mode to record the mean intensity values. As indicated in Fig. 3A and B, the small variations between the SERS signal intensity of the peak at 1620 cm−1 between different strips for the same biomarker concentration indicated that the developed SERS-based LFA demonstrated good reproducibility. In addition, the calculated relative standard deviation (RSD) values between the different scans of the high and low concentrations of both biomarkers ranged between 5.74% and 8.43% (Fig. 3A and B). Therefore, the developed SERS-based LFA demonstrated good reproducibility between measurements.
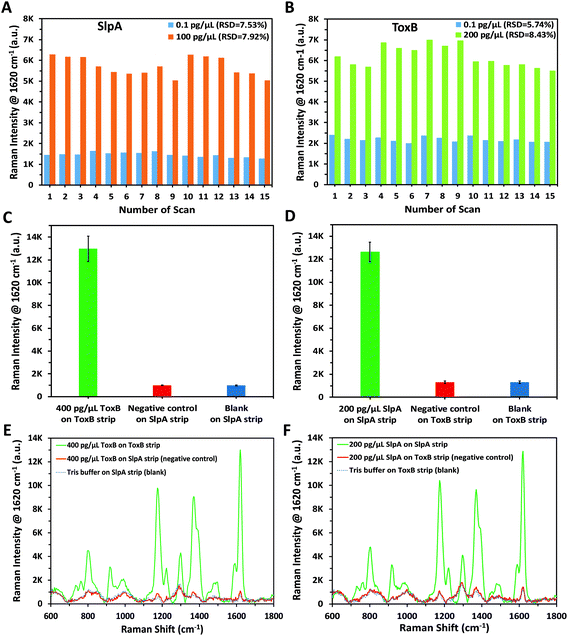 |
| Fig. 3 Reproducibility test by measuring SERS signal intensity of the peak at 1620 cm−1 for different concentrations of (A) SlpA (0.1 and 100 pg μL−1), (B) ToxB (0.1 and 200 pg μL−1). Each concentration was tested using five independent LF strips on different days and each strip was scanned three times during the day. Selectivity test was carried out by measuring the averaged SERS signal intensity (n = 3) of the peak at 1620 cm−1 for (C) SlpA strip using 400 pg μL−1 ToxB (negative control), Tris buffer (blank) and comparing the results against ToxB strip, (D) ToxB strip using 200 pg μL−1 SlpA (negative control), Tris buffer (blank) and comparing the results against SlpA strip (E) Corresponding Raman spectra for bar chart C, (F) corresponding Raman spectra for bar chart D. Error bars indicate standard deviations from three measurements. All the SERS measurements were carried out using a handheld Raman spectrometer equipped with a 638 nm laser excitation source at 5 mW laser power with an acquisition time of 0.5 s at ORS mode. | |
To demonstrate the selectivity of our methodology, negative control tests were carried out for both SlpA and ToxB strips using the opposite targets following the same procedures used for their quantification. As shown in Fig. 3C and D, these tests were performed by using SlpA and ToxB strips to detect 400 pg μL−1 of ToxB and 200 pg μL−1 of SlpA, respectively, as demonstrators for highly concentrated interfering molecules. Additionally, a blank sample of 0.01 M Tris buffer was examined using both strips. After running the mixtures on the LF strips, no red colour was observed on the test lines despite using the highest concentrations of SlpA and ToxB. The red colour developed only when using the LF strips with their dedicated targets. This was attributed to the high selectivity of the detection and capture antibodies on the SERS nanotags and LF strips towards their targets. The SERS scanning for the test lines yielded the same results. The averaged SERS signal intensity (n = 3) at the peak 1620 cm−1 for the negative control and blank samples were in agreement and were negligible when compared to that using the LF strip with the matching biomarker (Fig. 3C–F). These findings demonstrated that our developed LF strips respond only to the corresponding biomarker and thus indicate the high selectivity of the developed methodology.
3.4. Duplex detection and synthetic clinical stool matrix application
To demonstrate the capacity of our platform to perform duplex detection of SlpA and ToxB, duplex LF test strips were designed to have two separate test lines containing capture antibodies for the biomarkers beside the control line (Fig. 1). The SERS nanotags of both biomarkers were then mixed and used as a detection probe to test different mixtures of SlpA and ToxB. As shown in Table 1, a fixed concentration of ToxB (400 pg μL−1) was tested with different concentrations of SlpA ranging from 25–200 pg μL−1 to form the immuno-complexes onto the duplex LF strips. Another set of samples were prepared by mixing different concentrations of ToxB (50–400 pg μL−1) with a fixed concentration of SlpA (200 pg μL−1). Finally, these mixtures were tested similarly using the duplex LF test strips. A red colour on the SlpA test line started to appear first when the immuno-complexes solution reached the first test line due to the pulling of SlpA SERS nanotag-SlpA complex by the capture antibody pAbSlpA in a sandwich assay format. The solution continued to migrate over the strip and was captured by mAbToxB2 on ToxB test line forming a red colour due to the accumulation of ToxB SERS nanotag-ToxB-mAbToxB2 in a sandwich form. Finally, the excess immuno-complexes were captured by the anti-mouse IgG antibody on the control line forming a red colour. As shown in Fig. 4A and B, two test lines and one control line were observed on the strips within 10 minutes for the duplex detection of SlpA and ToxB. As indicated in Fig. 4A, the red colour of the SlpA test line became stronger with increasing SlpA concentration. In contrast, the red colour intensity of the ToxB test line was the same regardless of the SlpA concentration. Similarly, by testing the other sample sets, the red colour of the ToxB test line became more intense by increasing the ToxB concentration, while the red colour intensity of the SlpA test line remained unchanged (Fig. 4B). The control line colour intensity in all the duplex test strips was unaffected by the change in both biomarker concentrations, which indicates the duplex LF test strips were working correctly.56 The SERS scanning for both test lines on the duplex LF strip indicated the same results (Fig. 4C and D). In addition, the corresponding SERS signal intensity of the peak at 1620 cm−1 for the tested concentrations of SlpA and ToxB was strongly correlated with that of the same concentrations in their SERS calibration curves (Fig. 4E and F). Therefore, the cross reaction of the two biomarkers on the duplex LF test strip was negligible and the SERS-based LFA platform can be used for the simultaneous quantitative and selective detection of SlpA and ToxB.
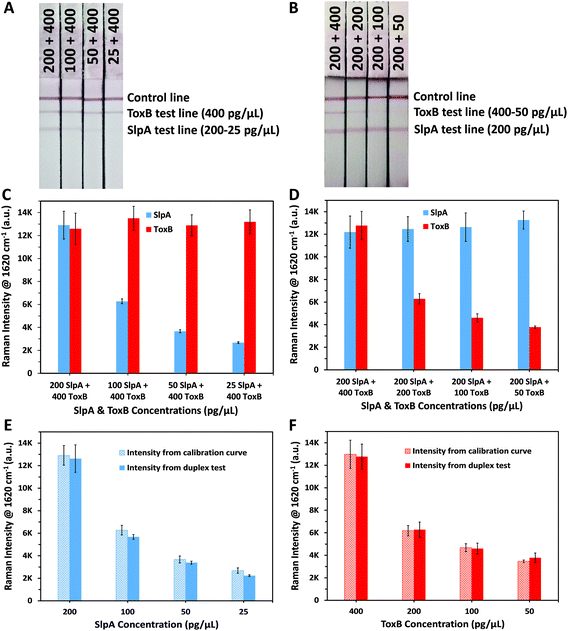 |
| Fig. 4 Duplex LF test strips of (A) fixed ToxB concentration (400 pg μL−1) with different SlpA concentrations (200–25 pg μL−1) and, (B) fixed SlpA concentration (200 pg μL−1) with different ToxB concentrations (400–50 pg μL−1). Averaged SERS signal intensity of the peak at 1620 cm−1 (n = 3) for duplex LF test strips of (C) fixed ToxB and different SlpA concentrations and, (D) fixed SlpA and different ToxB concentrations. Comparison between SERS signal intensity of the peak at 1620 cm−1 that obtained from calibration curves and duplex test for the same concentration of (E) SlpA and (F) ToxB. Error bars indicate standard deviations from three measurements. All the SERS measurements were carried out using a handheld Raman spectrometer equipped with a 638 nm laser excitation source at 5 mW laser power with an acquisition time of 0.5 s at ORS mode. | |
To evaluate the quantitative detection performance of the developed duplex SERS-based LFA in a clinical scenario, we applied this platform for the duplex quantification of 3 different concentrations of each biomarker spiked into a synthetic stool matrix. The chosen concentrations within the linear dynamic range of the calibration curves were 0.5, 40 and 80 pg μL−1 to simulate different bacterial loads with CDI in the human body. As per Table 2, different concentrations of the biomarkers were mixed and spiked into 1% of synthetic stool matrix. The use of diluted stool matrix is common due to its high viscosity. In addition, stool sample dilution minimizes the effect of the large amounts of inhibitory materials and debris that are contained in the matrix which can lead to clotting and errors in running the test.57,58 After performing the duplex detection of the spiked samples using the duplex LF test strips, the SERS signal intensity increased, as expected, with increase in SlpA and ToxB concentrations (Fig. 5A and B). The quantitative analysis of spiked SlpA and ToxB samples was carried out by monitoring the SERS signal intensity of the peak at 1620 cm−1 for the corresponding test lines. The linear regression equations derived from SlpA and ToxB calibration curves were used to calculate the recovered concentrations of the biomarkers in the spiked samples according to their SERS signal intensity. The average percentage recovery for the spiked samples concentration ranged from 96% to 104.66% (n = 3) (Table 2). Additionally, a blank sample of the synthetic stool matrix was tested using the duplex LF test strip and scanned by SERS, the resulted spectra showed a minimal signal background due to the high selectivity of our platform (Fig. 5A and B). Thus, the proposed novel SERS-based LFA platform demonstrated a high capacity for the duplex ultra-sensitive quantification and correct clinical diagnosis of the target biomarkers of CDI. This was accompanied with high selectivity and reproducibility. In addition, the use of simple point and shoot feature of the handheld Raman spectrometer to scan the LF strips instead of using benchtop Raman microscope make this platform easy to use without the need for special technical expertise.59 Moreover, the readout time of the test lines using a handheld Raman spectrometer in ORS mode is much faster than the lengthy mapping scan of the test lines using the benchtop Raman microscopes which require scanning hundreds to thousands of pixels. Accordingly, this paves the way towards an accurate, early and rapid clinical diagnosis of CDI at the POC and in hospitals wards,27,30,31,60 which will greatly assist in implementing the best treatment protocol and infectious disease control measures in a timely manner.
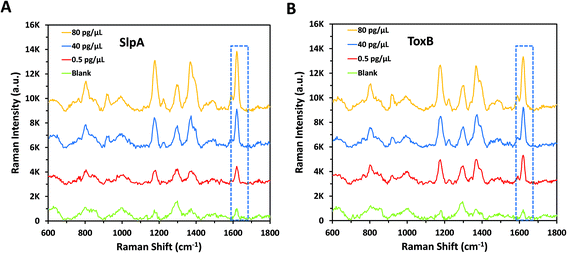 |
| Fig. 5 Raman spectra of blank and different concentrations of (A) SlpA and (B) ToxB spiked in a synthetic stool matrix. The spectra obtained by scanning the corresponding test lines onto the duplex LF test strip using a handheld Raman spectrometer. All the SERS measurements were carried out using a handheld Raman spectrometer equipped with a 638 nm laser excitation source at 5 mW laser power with an acquisition time of 0.5 s at ORS mode. | |
Table 2 Quantification of SlpA and ToxB in spiked synthetic stool samples using SERS-based LFA
Spiked concentration (pg μL−1) |
SlpA |
ToxB |
Found (pg μL−1) |
% Recoverya |
Found (pg μL−1) |
% Recoverya |
Average for three different determinations for each sample.
|
0.50 |
0.52 |
104.00 |
0.48 |
96.00 |
40.00 |
41.38 |
103.45 |
39.23 |
98.08 |
80.00 |
78.51 |
98.14 |
83.73 |
104.66 |
4. Conclusion
In this work, we presented a novel duplex SERS-based LFA platform for the selective and sensitive quantification of the C. diff biomarkers, SlpA and ToxB within 20 minutes. Highly specific SERS nanotags for SlpA and ToxB were developed by functionalising Raman reporter-labelled AuNPs with selective antibodies for both biomarkers. These SERS nanotags were used as detection probes that enabled the rapid visual duplex detection of SlpA and ToxB using one LF strip. The integrative use of SERS to scan the LF strips enabled a highly sensitive quantification of SlpA and ToxB with a lowest observable concentration of 0.01 pg μL−1. The platform showed good reproducibility and selectivity towards its targets in both buffer solution and synthetic clinical matrix. Compared to other methods reported for C. diff diagnosis, this novel quantitative platform is highly selective, sensitive, fast, cost-effective with minimal sample pre-treatment steps and simple manual operation. The proposed duplex platform has not been discussed previously or introduced in the diagnostic market for the clinical testing of CDI. The duplex test for ToxB and SlpA instead of GDH can result in a more accurate diagnosis of CDI without any cross-reactivity interference from other similar species while determining the infection pathogenicity. The main area of future work is to apply this SERS-based LFA platform for the quantitative and ultra-sensitive duplex detection of SlpA with ToxB in a larger scale study of clinical specimens to further investigate its capacity to be a potential alternative diagnostic test for CDI. We believe the continuous and future progress in the integration of handheld Raman spectrometers with LFA, instead of using benchtop Raman microscopes, could turn SERS-based LFA into an ideal platform for the accurate, early and high throughput detection of other bacterial biomarkers at POC and in low resource settings.
Conflicts of interest
There are no conflicts to declare.
Acknowledgements
This research was funded by the EPSRC IRC in Early-Warning Sensing Systems for Infectious Diseases (i-sense) EP/ K031953/1, EPSRC IRC in Agile Early Warning Sensing Systems for Infectious Diseases & Antimicrobial Resistance EP/R00529X/1 and IRC Next Steps Plus: Ultra-Sensitive Enhanced NanoSensing of Anti-Microbial Resistance (uSense) EP/R018391/1. The research data associated with this paper will become available from the University of Strathclyde at the following link: https://doi.org/10.15129/892e2c07-e848-4b87-b0e0-97d2faced701.
References
- S. Khanna and D. S. Pardi, Mayo Clin. Proc., 2012, 87, 1106–1117 CrossRef CAS PubMed.
- J. W. Cheng, M. Xiao, T. Kudinha, Z. P. Xu, L. Y. Sun, X. Hou, L. Zhang, X. Fan, F. Kong and Y. C. Xu, PLoS One, 2015, 10, e0144604 CrossRef PubMed.
- S. Di Bella, P. Ascenzi, S. Siarakas, N. Petrosillo and A. Di Masi, Toxins, 2016, 8, 134 CrossRef PubMed.
- D. Drudy, S. Fanning and L. Kyne, Int. J. Infect. Dis., 2007, 11, 5–10 CrossRef CAS PubMed.
- R. P. Vonberg, E. J. Kuijper, M. H. Wilcox, F. Barbut, P. Tüll, P. Gastmeier, P. J. van den Broek, A. Colville, B. Coignard, T. Daha, S. Debast, B. I. Duerden, S. van den Hof, T. van der Kooi, H. J. Maarleveld, E. Nagy, D. W. Notermans, J. O'Driscoll, B. Patel, S. Stone and C. Wiuff, Clin. Microbiol. Infect., 2008, 14(Suppl 5), 2–20 CrossRef PubMed.
- N. R. Pollock, J. Clin. Microbiol., 2016, 54, 259–264 CrossRef CAS PubMed.
- A. B. Ryder, Y. Huang, H. Li, M. Zheng, X. Wang, C. W. Stratton, X. Xu and Y.-W. Tang, J. Clin. Microbiol., 2010, 48, 4129–4134 CrossRef.
- T. Åkerlund, B. Svenungsson, Å. Lagergren and L. G. Burman, J. Clin. Microbiol., 2006, 44, 353–358 CrossRef PubMed.
- B. Huang, D. Jin, J. Zhang, J. Y. Sun, X. Wang, J. Stiles, X. Xu, M. Kamboj, N. E. Babady and Y.-W. Tang, J. Clin. Microbiol., 2014, 52, 1105–1111 CrossRef PubMed.
- J. R. O'Connor, S. Johnson and D. N. Gerding, Gastroenterology, 2009, 136, 1913–1924 CrossRef.
- V. G. Loo, L. Poirier, M. A. Miller, M. Oughton, M. D. Libman, S. Michaud, A. M. Bourgault, T. Nguyen, C. Frenette, M. Kelly, A. Vibien, P. Brassard, S. Fenn, K. Dewar, T. J. Hudson, R. Horn, P. Rene, Y. Monczak and A. Dascal, N. Engl. J. Med., 2005, 353, 2442–2449 CrossRef CAS PubMed.
- B. M. Lawry, C. L. Johnson, K. Flanagan, J. A. Spoors, C. J. McNeil, A. Wipat and N. Keegan, Anal. Chem., 2018, 90, 13475–13482 CrossRef CAS PubMed.
- L. Song, M. Zhao, D. C. Duffy, J. Hansen, K. Shields, M. Wungjiranirun, X. Chen, H. Xu, D. A. Leffler, S. P. Sambol, D. N. Gerding, C. P. Kelly and N. R. Pollock, J. Clin. Microbiol., 2015, 53, 3204–3212 CrossRef CAS PubMed.
- T. D. Planche, K. A. Davies, P. G. Coen, J. M. Finney, I. M. Monahan, K. A. Morris, L. O'Connor, S. J. Oakley, C. F. Pope, M. W. Wren, N. P. Shetty, D. W. Crook and M. H. Wilcox, Lancet Infect. Dis., 2013, 13, 936–945 CrossRef PubMed.
-
J. Robotham and M. Wilcox, Updated Guidance on the Diagnosis and Reporting of Clostridium Difficile, Department of Health, 2012 Search PubMed.
- H. S. Chung and M. Lee, J. Invest. Med., 2017, 65, 88–92 CrossRef.
- N. V. Dmitrieva, G. A. Klyasova, N. V. Bakulina, M. A. Sukhina, S. V. Zhuravel, E. A. Belousova, V. T. Ivashkin, S. V. Goryunov, E. A. Prokhorovich, T. R. Kameneva, A. A. Samsonov, A. V. Yakovenko and S. V. Kazakov, Infect. Dis. Ther., 2018, 7, 523–534 CrossRef PubMed.
- A. M. Larson, A. M. Fung and F. C. Fang, J. Clin. Microbiol., 2010, 48, 124–130 CrossRef CAS PubMed.
- T. D. Wilkins and D. M. Lyerly, J. Clin. Microbiol., 2003, 41, 531–534 CrossRef PubMed.
- L. Blanco-Covian, V. Montes-Garcia, A. Girard, M. T. Fernandez-Abedul, J. Perez-Juste, I. Pastoriza-Santos, K. Faulds, D. Graham and M. C. Blanco-Lopez, Nanoscale, 2017, 9, 2051–2058 RSC.
- S. Choi, J. Hwang, S. Lee, D. W. Lim, H. Joo and J. Choo, Sens. Actuators, B, 2017, 240, 358–364 CrossRef CAS.
- W. A. Hassanain, E. L. Izake, A. Sivanesan and G. A. Ayoko, J. Pharm. Biomed. Anal., 2017, 136, 38–43 CrossRef CAS.
- H. Chen, A. Das, L. Bi, N. Choi, J. I. Moon, Y. Wu, S. Park and J. Choo, Nanoscale, 2020, 12, 21560–21570 RSC.
- J. Langer, D. Jimenez de Aberasturi, J. Aizpurua, R. A. Alvarez-Puebla, B. Auguie, J. J. Baumberg, G. C. Bazan, S. E. J. Bell, A. Boisen, A. G. Brolo, J. Choo, D. Cialla-May, V. Deckert, L. Fabris, K. Faulds, F. J. Garcia de Abajo, R. Goodacre, D. Graham, A. J. Haes, C. L. Haynes, C. Huck, T. Itoh, M. Kall, J. Kneipp, N. A. Kotov, H. Kuang, E. C. Le Ru, H. K. Lee, J. F. Li, X. Y. Ling, S. A. Maier, T. Mayerhofer, M. Moskovits, K. Murakoshi, J. M. Nam, S. Nie, Y. Ozaki, I. Pastoriza-Santos, J. Perez-Juste, J. Popp, A. Pucci, S. Reich, B. Ren, G. C. Schatz, T. Shegai, S. Schlucker, L. L. Tay, K. G. Thomas, Z. Q. Tian, R. P. Van Duyne, T. Vo-Dinh, Y. Wang, K. A. Willets, C. Xu, H. Xu, Y. Xu, Y. S. Yamamoto, B. Zhao and L. M. Liz-Marzan, ACS Nano, 2020, 14, 28–117 CrossRef CAS.
- T. J. Moore, A. S. Moody, T. D. Payne, G. M. Sarabia, A. R. Daniel and B. Sharma, Biosensors, 2018, 8, 46 CrossRef PubMed.
- M. Eryilmaz, E. Acar Soykut, D. Cetin, I. H. Boyaci, Z. Suludere and U. Tamer, Analyst, 2019, 144, 3573–3580 RSC.
- S. H. Lee, J. Hwang, K. Kim, J. Jeon, S. Lee, J. Ko, J. Lee, M. Kang, D. R. Chung and J. Choo, Anal. Chem., 2019, 91, 12275–12282 CrossRef CAS PubMed.
- J. Hwang, S. Lee and J. Choo, Nanoscale, 2016, 8, 11418–11425 RSC.
- L. Shi, L. Xu, R. Xiao, Z. Zhou, C. Wang, S. Wang and B. Gu, Front. Microbiol., 2020, 11, 596005 CrossRef PubMed.
- R. Xiao, L. Lu, Z. Rong, C. Wang, Y. Peng, F. Wang, J. Wang, M. Sun, J. Dong, D. Wang, L. Wang, N. Sun and S. Wang, Biosens. Bioelectron., 2020, 168, 112524 CrossRef CAS PubMed.
- V. Tran, B. Walkenfort, M. Konig, M. Salehi and S. Schlucker, Angew. Chem., Int. Ed., 2019, 58, 442–446 CrossRef CAS PubMed.
- B. Khlebtsov and N. Khlebtsov, Nanomaterials, 2020, 10, 2228 CrossRef CAS.
- A. K. Barker, E. Scaria, N. Safdar and O. Alagoz, JAMA Network Open, 2020, 3, e2012522 CrossRef.
- J. Kimling, M. Maier, B. Okenve, V. Kotaidis, H. Ballot and A. Plech, J. Phys. Chem. B, 2006, 110, 15700–15707 CrossRef CAS.
- S. Mabbott, S. C. Fernandes, M. Schechinger, G. L. Cote, K. Faulds, C. R. Mace and D. Graham, Analyst, 2020, 145, 983–991 RSC.
- L. Dykman and N. Khlebtsov, Chem. Soc. Rev., 2012, 41, 2256–2282 RSC.
- S. Laing, L. E. Jamieson, K. Faulds and D. Graham, Nat. Rev. Chem., 2017, 1, 0060 CrossRef CAS.
- X. Qian, S. R. Emory and S. Nie, J. Am. Chem. Soc., 2012, 134, 2000–2003 CrossRef CAS PubMed.
- H. Kearns, R. Goodacre, L. E. Jamieson, D. Graham and K. Faulds, Anal. Chem., 2017, 89, 12666–12673 CrossRef CAS PubMed.
- S. McAughtrie, K. Lau, K. Faulds and D. Graham, Chem. Sci., 2013, 4, 3566–3572 RSC.
- R. Wang, K. Kim, N. Choi, X. Wang, J. Lee, J. H. Jeon, G.-E. Rhie and J. Choo, Sens. Actuators, B, 2018, 270, 72–79 CrossRef CAS.
- J. Li, D. McMillan and J. Macdonald, Sens. Mater., 2015, 27, 549–561 CAS.
- P. Noguera, G. A. Posthuma-Trumpie, M. van Tuil, F. J. van der Wal, A. de Boer, A. P. Moers and A. van Amerongen, Anal. Bioanal. Chem., 2011, 399, 831–838 CrossRef CAS.
- C. Suarez-Pantaleon, J. Wichers, A. Abad-Somovilla, A. van Amerongen and A. Abad-Fuentes, Biosens. Bioelectron., 2013, 42, 170–176 CrossRef CAS PubMed.
- M. Sanchez-Purra, B. Roig-Solvas, A. Versiani, C. Rodriguez-Quijada, H. de Puig, I. Bosch, L. Gehrke and K. Hamad-Schifferli, Mol. Syst. Des. Eng., 2017, 2, 401–409 RSC.
- W. A. Hassanain, E. L. Izake and G. A. Ayoko, Anal. Chem., 2018, 90, 10843–10850 CrossRef CAS PubMed.
- R. Agoston, E. L. Izake, A. Sivanesan, W. B. Lott, M. Sillence and R. Steel, Nanomedicine, 2016, 12, 633–641 CrossRef CAS PubMed.
- M. S. Schmidt, J. Hubner and A. Boisen, Adv. Mater., 2012, 24, OP11–OP18 CAS.
- A. Pallis, J. Jazayeri, P. Ward, K. Dimovski and S. Svobodova, J. Med. Microbiol., 2013, 62, 1350–1356 CrossRef CAS PubMed.
- S. Persson, M. Torpdahl and K. E. Olsen, Clin. Microbiol. Infect., 2008, 14, 1057–1064 CrossRef CAS PubMed.
- J. Sandlund, A. Bartolome, A. Almazan, S. Tam, S. Biscocho, S. Abusali, J. J. Bishop, N. Nolan, J. Estis, J. Todd, S. Young, F. Senchyna and N. Banaei, J. Clin. Microbiol., 2018, 56, e00908-18 CrossRef PubMed.
- H. Kato, T. Yokoyama, H. Kato and Y. Arakawa, J. Clin. Microbiol., 2005, 43, 6108–6112 CrossRef CAS PubMed.
- Y. S. Fang, S. Y. Chen, X. J. Huang, L. S. Wang, H. Y. Wang and J. F. Wang, Biosens. Bioelectron., 2014, 53, 238–244 CrossRef CAS PubMed.
- Z. Zhu, L. Shi, H. Feng and H. S. Zhou, Bioelectrochemistry, 2015, 101, 153–158 CrossRef CAS PubMed.
- Y. Zhang, L. Zhang, L. Yang, C. I. Vong, K. F. Chan, W. K. K. Wu, T. N. Y. Kwong, N. W. S. Lo, M. Ip, S. H. Wong, J. J. Y. Sung, P. W. Y. Chiu and L. Zhang, Sci. Adv., 2019, 5, eaau9650 CrossRef PubMed.
- D. Zhang, L. Huang, B. Liu, H. Ni, L. Sun, E. Su, H. Chen, Z. Gu and X. Zhao, Biosens. Bioelectron., 2018, 106, 204–211 CrossRef CAS PubMed.
- M. Lounnas, A. Diack, M. P. Nicol, S. Eyangoh, E. Wobudeya, O. Marcy, S. Godreuil and M. Bonnet, Tuberculosis, 2020, 125, 102002 CrossRef CAS PubMed.
- L. Feghoul, M. Salmona, J. Cherot, M. Fahd, J.-H. Dalle, C. Vachon, A. Perrod, P. Bourgeois, C. Scieux, A. Baruchel, F. Simon and J. LeGoff, J. Clin. Microbiol., 2016, 54, 928–933 CrossRef CAS PubMed.
- D. I. Ellis, H. Muhamadali, S. A. Haughey, C. T. Elliott and R. Goodacre, Anal. Methods, 2015, 7, 9401–9414 RSC.
- D. Zhang, L. Huang, B. Liu, Q. Ge, J. Dong and X. Zhao, Theranostics, 2019, 9, 4849–4859 CrossRef CAS PubMed.
Footnote |
† Electronic supplementary information (ESI) available. See DOI: 10.1039/d1an00726b |
|
This journal is © The Royal Society of Chemistry 2021 |