DOI:
10.1039/D0AN01769H
(Communication)
Analyst, 2021,
146, 1157-1162
Single cell biomass tracking allows identification and isolation of rare targeted therapy-resistant DLBCL cells within a mixed population†
Received
3rd September 2020
, Accepted 3rd January 2021
First published on 4th January 2021
Abstract
Adaptive resistance is a major limitation in the use of targeted therapies for cancer. Using real time biomass tracking, we demonstrate the isolation and identification of rare (1% fraction) diffuse large B cell lymphoma cells resistant to the PI3K inhibitor idelalisib, from an otherwise sensitive population. This technique allows direct study of these rare, drug tolerant cells.
Introduction
Targeted therapies use novel agents that specifically target one or more proteins in a cell and are administered based on the genetic make-up of the patients’ cancer. As these drugs are personalized to the patients’ specific cancer, they can be very effective and are considerably less toxic to normal cells than are chemotherapies. While targeted therapies continue to change the landscape of cancer therapeutics and lead to tumor shrinkage in many cases, the major drawback is patients’ cancers eventually grow back in the presence of the drug, a phenomenon known as “acquired resistance”. Unfortunately, this usually occurs within one year, and afterwards there are limited treatment options.1 In some cases, secondary mutations that confer resistance to targeted inhibitors can exist—usually at hard-to detect frequencies—prior to initiation of treatment,2 or alternatively, appear de novo;3 similarly, other non-genetic-based resistant mechanisms can also appear de novo, in response to the selection pressure of the targeted inhibitor.4
In the case of non-pre-existing resistant clones (whether mutation based or otherwise), it remains largely unknown how some cancer cells survive initial treatment, allowing them to eventually acquire resistance, usually by multiple, heterogeneous mechanisms. An increasingly-recognized reason for treatment failure involves this subpopulation of cells possessing immediate drug tolerance, at least for some period of time.5–7 These drug-tolerant cells survive long enough during initial treatment to spontaneously acquire genetic or non-genetic changes that confer long-term (stable) resistance. There remains no good way to study these drug-tolerant subpopulations because transient growth and survival at the single cell level during initial treatment is very difficult to measure.
In this work we overcome this hurdle and quickly identify and isolate rare diffuse large B cell lymphoma (DLBCL) cells tolerant of the targeted therapeutic agent, idelalisib, using a novel single cell biomass tracking approach. Idelalisib is an inhibitor of phosphatidylinositol 3-kinase (PI3K) that is sometimes used for salvage therapy in treatment refractory DLBCL patients. While DLBCL provides a specific scenario where characterizing drug-resistant subpopulations before relapse becomes clinically relevant, cancer relapse is a problem affecting nearly all cancers and isolating and studying drug-resistant subpopulations at an early stage could inform clinical decision making in nearly all of them.
Results and discussion
DLBCL is the most common subtype of non-Hodgkin's lymphoma. Current frontline therapy for DLBCL is an immunochemotherapy and chemotherapy combination of rituximab, cyclophosphamide, doxorubicin, vincristine, and prednisone (R-CHOP) with or without radiation depending on cancer stage. This combination has improved five-year survival rates up to 60–70%.8 Despite these gains in survival, up to 50% of patients become refractory to or relapse after treatment. Only about 30–40% will respond to salvage therapy and 50% will relapse after autologous stem cell transplant (ASCT), and the overall survival of patients with refractory DLBCL, defined as no response to last chemotherapy or relapse <12 months post ASCT, is only around 20%.8
Idelalisib is a potential second line therapy in DLBCL that is currently being tested in clinical trials (ClinicalTrials.gov Identifier: NCT03576443). Idelalisib induces apoptosis in malignant B-cells by inhibiting the PI3K/AKT/mTOR growth signaling pathway. We created an ex vivo, drug-resistant-clone-containing tumor using a 1
:
100 mixture of two DLBCL cell lines, SU-DHL-10 and SU-DHL-6,9 which have differential sensitivity to idelalisib. We then identified idelalisib-tolerant cells in real time by their substantial and distinct biomass growth in the presence of drug using High Speed Live Cell Interferometry (HSLCI), a multi-well biomass accumulation assay depicted in Fig. 1.10–12 Fast growing cells were isolated via an automated micropipette system and re-cultured to confirm their idelalisib resistance. Both cell lines have IGH-BCL2 (t14:18) fusions with SU-DHL-6 having a breakpoint in the major breakpoint region (MBR) and SU-DHL-10 having a different breakpoint, in intermediate cluster region (ICR), of chromosome 18. This difference in translocation breakpoints between the two cell lines enables the identification of the two lines through PCR.13
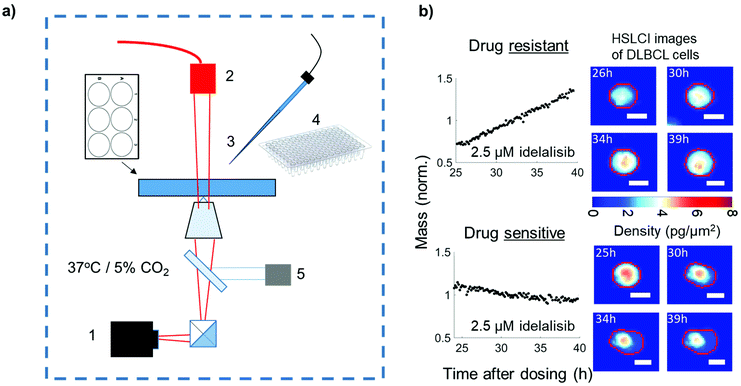 |
| Fig. 1 (a) Schema of HSLCI. (1) Wide field phase detection camera, (2) illumination source, (3) micropipette system, (4) tubes for cell collection. (5) Dynamic focus control (b) examples of drug-tolerant and -sensitive DLBCL cells present in same culture. Left shows cell mass vs. time. Right shows HSLCI cellular mass density images of the two cells. Scale bars 10 μm. | |
While previous work with HSLCI has identified rare drug resistant cells,11,12,14 these studies are the first to isolate these cells. This step forward is owed to the development of an automated micropipette system and improvements in real-time data processing.
SU-DHL 6 and 10 differential sensitivity to idelalisib measured with HSLCI
To measure the sensitivity of both SU-DHL-6 and -10 to idelalisib, cells were dosed at a range of concentrations from 0 μM to 50 μM for 24 hours, followed by monitoring biomass growth by HSLCI for 12 hours (Fig. 2). We found that SU-DHL-6 had a half maximal effective concentration (EC50) of 46 ± 13 nM (n = 3), while SU-DHL-10 had an EC50 of 7703 ± 452 nM (n = 4). Therefore, SU-DHL-10 requires greater than two orders of magnitude more idelalisib to experience a similar effect on biomass growth as SU-DHL-6. For reference, a patient taking the recommended dose of idelalisib and the dose used in the clinical trial, 150 mg, will have an maximum concentration of idelalisib of approximately 2–3 μM in their blood.15 At 2.5 μM, SU-DHL-10 growth remains close to untreated cell growth (97.8% ± 4.9% of the normalized response), while the median growth of SU-DHL-6 is negative even at 1 μM (p < 0.001 vs. untreated cells). Of note, while SU-DHL-6 untreated cells appear to have an even spread of hourly growth rates from −2%–5%, SU-DHL-10 untreated or low dosed cells appear to fall into one of two categories: growing at ∼4% or dying or dormant at ∼0%.
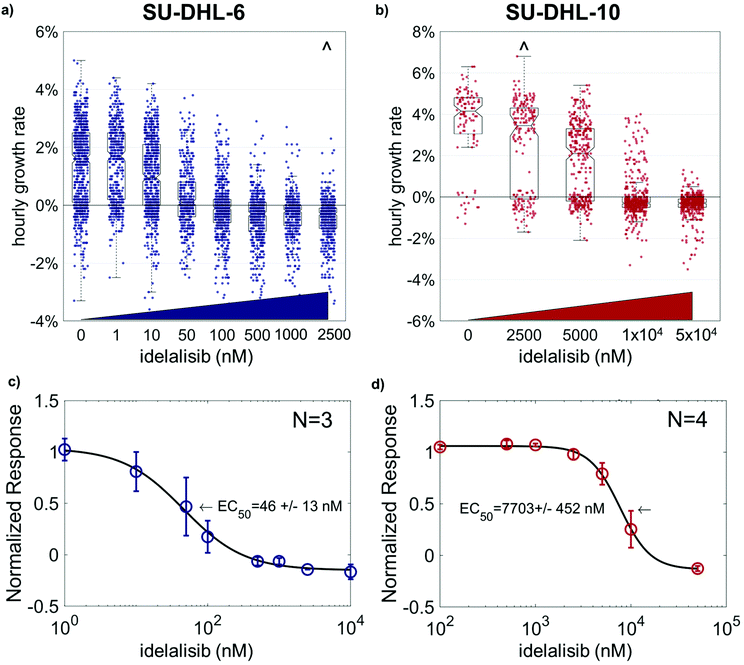 |
| Fig. 2 (a & b) Representative box-plots of SU-DHL-6 and SU-DHL-10 cells treated with idelalisib. Individual dots in the overlaying box plot represent the mass accumulation rates of single cells measured over the interval 24–36 h post-dosing. The carrot “^” indicates the maximum dose reached in the blood of human patients given the prescribed dose. (c & d) The EC50 is calculated by sigmoidal fit to the median mass accumulation rates for SU-DHL-6 (N = 3) and SU-DHL-10 (N = 4). These responses indicate SU-DHL-6 is sensitive and SU-DHL-10 is resistant to idelalisib. | |
Isolating idelalisib-tolerant cells and re-culturing to confirm resistance
Individual cultures of SU-DHL-10 and -6 cells were collected and counted, and then mixed at a 1
:
100 ratio on a six well plate. Cells were then treated with either 0 or 2.5 μM of idelalisib, which is a dose commensurate with the highest concentration seen in the blood of patients. After 24 hours the biomass growth of single cells or cell clusters was measured every 8 minutes by HSLCI for the next 12–16 h. At the end of the observation period, single fast growing cells or clusters were located and automatically collected into a micropipette (ESI Fig. 1†). This process was repeated until the desired number of cells were collected. These isolated cells were then re-cultured for two to three weeks and then re-screened in 2.5 μM idelalisib to confirm that they were indeed resistant to the drug.
Three drug resistant cell isolations were performed with a representative example shown in Fig. 3 (see also ESI Fig. 2†). The median hourly growth rates of the untreated 1
:
100 cell mixtures ranged from 1.5% ± 0.1% to 1.9% ± 0.1% in the three trials. In contrast, the median hourly growth rates of the cells treated with 2.5 μM idelalisib ranged from −0.3% ± 0.02% to 0% ± 0.07%, indicating idelalisib had significant growth inhibition effects at 2.5 μM. When evaluated with two sample t-test, the comparison of corresponding treated and untreated population for each trial yields p < 0.0001 for all three trials.
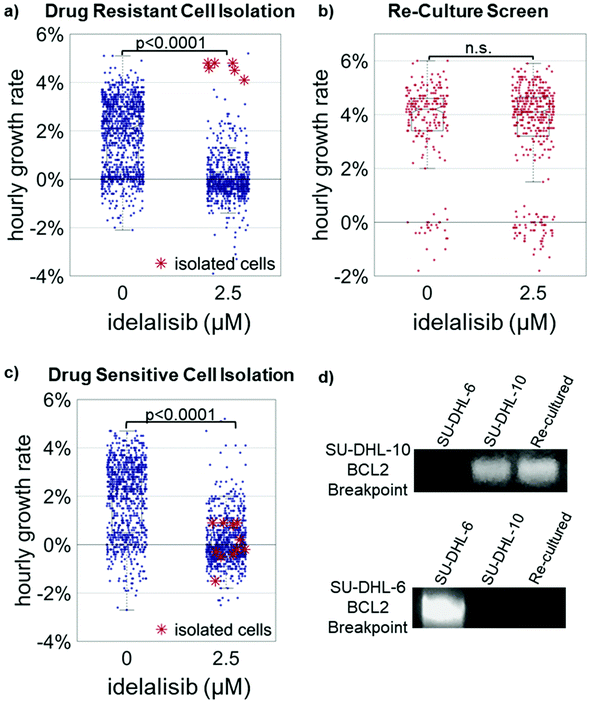 |
| Fig. 3 (a) Representative example (n = 3) of the isolation of the top 1% of growing cells from 1 : 100 SU-DHL-10/SU-DHL-6 mixture. Cells were treated with 2.5 μM idelalisib for 24 hours and mass accumulation rates were measured from 24–40 hours post dosing at which point the top 1% of growing cells were isolated by micropipette. (b) Cells isolated in (a) were cultured for 20 days then rescreened at 2.5 μM idelalisib. The median growth rate at 2.5 μM idelalisib increased from −0.2% ± 0.02% per hour in the isolation to 4.1% ± 0.12% per hour after re-culture. (c). In an experiment identical to (a), cells in the bottom <95th percentiles were isolated and cultured but no growth occurred. (d) PCR at the IGH-BCL2 loci for the cell line-specific breakpoints was performed on cells from stock SU-DHL-6 and -10 lines, and re-cultured cells indicating SU-DHL-10 cells were isolated from the 1 : 100 mixture. | |
The fastest growing 1% of cells were isolated from each of the treated population as indicated by the red asterisk “*”. These cells were then re-cultured for approximately two to three weeks. Median growth rates of untreated populations ranged from 4.0% ± 0.1% to 4.4 ± 0.1% while median growth rates of treated populations ranged from 3.8% ± 0.1% to 4.4 ± 0.07% in the three trials. When evaluated with two sample t-test, the comparison of corresponding treated and untreated population for each trial yields p > 0.30 for all three trials. The identity of the re-cultured resistant cells as SU-DHL-10 was further confirmed through PCR (Fig. 3d and ESI Fig. 3†).13 These results indicate the successful isolation of the 1% idelalisib tolerant sub-population. As a further control, cultures of media from micropipettes that were exposed in the media but collected no cells resulted in no cell growth. Additionally, collection of approximately 10 random cells in the bottom 95% of growth rates resulted in no cell growth (Fig. 3c and ESI Fig. 4†).
Between 800–1300 cells were measured in the treatment condition for each experiment, indicating a theoretical detection limit for the system as around ∼0.1% for the isolation of a single cell. While re-culturing a single cell may be more difficult, isolation, cell lysis, and DNA or RNA analysis are eminently achievable. The current bottleneck for the system is real-time data processing which could be improved by increased CPU and GPU power. Increases in processing speed could lead to 3× the amount of data processed until being limited by the camera's maximum framerate.
Future developments of this technique will include adaptation to primary cells extracted from tissue, which typically have a shorter viability window, versus cell lines which grow robustly in culture for extended periods. HSLCI has been used successfully with primary tissue from triple negative breast cancer patient-derived xenografts, including very limited quantity samples obtained from a fine needle biopsy.12,14 However, it is likely that additional optimization of media conditions would be needed for some types of primary samples. These PDX cells remain non-adherent.12 Any work with fully-adherent cells may require use of engineered surface coatings to allow the cells to be aspirated into the pipette without damage, or the careful application of proteases to reduce adhesion during collection. If this is not feasible, one would be limited to cell lysis and DNA and RNA analysis as cell damage due to collection would prevent re-culture.
Traditional dye exclusion cell viability assays conducted via microscopy at a single point in time could differentiate live cells from apoptotic or necrotic cells for isolation. However, this method would not discriminate slow growing or quiescent cells from vigorously growing cells, as does biomass tracking. Biologically, the cells growing vigorously in the presence of drug are likely to be the most interesting candidates for mechanistic studies. Furthermore, in contrast to the snapshot nature of dye exclusion assays, the kinetic of single cell responses captured by biomass tracking may prove to be particularly informative in fragile primary cultures which remain viable for only short periods after isolation.
In addition to biomass tracking, other single cell analytical methodologies can explore the heterogeneity of the drug response such as fluorescence lifetime assays (FLT) or Raman spectroscopy.16,17 While both techniques provide insights into metabolic responses to drugs, they have not been used to isolate low abundance drug-tolerant cells from mixed samples Higher throughput FLT assays are prone to photo bleaching, can have trouble resolving multi exponential decays, and data processing is not yet real-time.17 High throughput Raman based methods have only been able to provide of a snapshot in time of drug response unlike the time dynamic measurements acquired by HSLCI.18
The ability to identify and isolate live resistant subpopulations via HSLCI can be a valuable tool for both basic research and clinical decision making in solid and liquid tumors alike. Identification and re-culturing of resistant subpopulations in cell lines or primary samples could facilitate the study of mechanisms of drug-resistance and even reversion back to a drug sensitive state to help develop new therapeutics. In the clinical setting, HSLCI could be used to identify and characterize drug resistant clones, before disease relapse becomes evident in the patient. HSLCI could potentially develop into a tool to help direct evolutionary guided strategies of adaptive therapy.19–21
Materials & methods
Cells and cell culture
SU-DHL-6 and -10 cells were obtained from American Type Culture Collection (ATCC) and maintained with RMPI 1640 supplemented with 10% FBS also obtained from ATCC. Cells isolated from HSLCI screenings were at first maintained in 200 μL of RMPI 1640 in a 96-well plate until they had grown out to about 100
000 cells at which point cells were maintained in a 24 well plate, and then finally in T25s.
HSLCI screening experiments
The HSLCI platform is a custom-built inverted optical microscope coupled to an off-axis quadriwave lateral shearing interferometric camera (SID4BIO, Phasics, Inc.). Cells are imaged in single, standard-footprint (128 × 85 mm), glass-bottomed, 6 or 24 well plates (Cellvis). Acquired images are processed by a downstream PC using NVIDIA K2000 GPU and a MATLAB pipeline. All of the platform's hardware and software components are available commercially. A 40× objective (Nikon, NA 0.75) was used for all the studies. The imaging platform is installed within a standard cell culture incubator.
Between 50
000–100
000 cells per well were plated in either 24 or 6 well plates. Cells were then treated with the proper doses of idelalisib (ApeXBio). After 24 hours for treatment to take effect, cells were monitored for 12–16 hours.
To ensure the quality of hourly growth rates recorded, data was filtered such that only biomass tracks (mass vs. time) exhibiting linear fit standard errors less than 0.002 normalized mass units per hour were included. This excludes tracks were noise introduced by cell debris or drifting interrupts the tracks of otherwise stable cells. Only cells greater than 300 pg were included, as objects smaller than that never grew and appeared to be just debris.
HSLCI isolation experiments
100
000 cells per well were plated in 6 well plates. Cells were treated with either 0 or 2.5 μM of idelalisib. After 24 hours, cells were monitored for 12–16 hours. Data from 2.5 μM condition was processed in real-time, and the 0 μM data processed after the experiment. Images are then tracked frame to frame and hourly growth rates determined. Cells were then isolated with a micropipette (0.5 mm, borosilicate glass, Sutter Instruments) that was pulled using P-2000 micropipette puller (heat = 290, pull = 25, delay = 150, velocty = 20, Sutter Instruments).
The locations of cells with growth rates in top ∼1% are then sent from a processing computer to a control computer. HSLCI machine then automatically goes to each location, the user then indicates whether the tracked cell or cell cluster is present and should be isolated. Then the micropipette automatically comes down and grabs cell or cell cluster using capillary pressure. The micropipette then goes back above the surface of the media, and machine moves to the next location. After completion of collection, all the liquid in the micropipette is deposited into 100 μL of RPMI 1640 media for re-culture together in a single well of a 96-well plate.
PCR
PCR was performed on the re-cultured samples from Trials #1, 2, and 3 for detection of distinct breakpoints in BCL2 due IGH-BCL2 fusions in SU-DHL-6 and SU-DHL-10. Primer sequences and genomic coordinates are available in ESI Table #1.† Genomic DNA was extracted with Qiagen's MagAttract HMW DNA kit. DNA concentration was then measured with ThermoFisher's Quibit dsDNA HS Assay kit. Next the PCR solution composed of primers identified by Bouamar et al.13 for identifying SU-DHL-6 and SU-DHL-10 cells, millipure water and New England Biotechnologies Long AMP PCR Master Mix, was mixed with the samples. The solution was then heated to 95C for 1 minute and cycled 45 times at 95C for 15 seconds, 57C for 15 seconds and then 60C for 1 minute. 5 μL aliquots were run on a 2% agarose gel.
Author contributions
Developed the HSLCI instrumentation: G. F. M., D. G., and J. R. Designed experiments: G. F. M., and J. R. Performed experiments: G. F. M., A. M. Analyzed data: G. F. M., A. T., and J. R. Wrote the manuscript: G. F. M., A. T., and J. R. Discussed data and edited the manuscript: all authors.
Conflicts of interest
There are no conflicts of interest to declare.
Acknowledgements
We would like to thank Dr Joseph Reiner at VCU for use of his P-2000 micropipette puller. Funding was provided by National Institutes of Health grant R01CA185189 to JR, and in part, by the NCI Cancer Center Support Grant P30CA016059 to the VCU Massey Cancer Center.
References
- S. Boumahdi and F. J. de Sauvage, Nat. Rev. Drug Discovery, 2020, 19, 39–56 CrossRef CAS.
- R. A. Beckman and L. A. Loeb, Clin. Pharmacol. Ther., 2020, 108, 437–439 CrossRef.
- A. N. Hata, M. J. Niederst, H. L. Archibald, M. Gomez-Caraballo, F. M. Siddiqui, H. E. Mulvey, Y. E. Maruvka, F. Ji, H.-E. C. Bhang, V. Krishnamurthy Radhakrishna, G. Siravegna, H. Hu, S. Raoof, E. Lockerman, A. Kalsy, D. Lee, C. L. Keating, D. A. Ruddy, L. J. Damon, A. S. Crystal, C. Costa, Z. Piotrowska, A. Bardelli, A. J. Iafrate, R. I. Sadreyev, F. Stegmeier, G. Getz, L. V. Sequist, A. C. Faber and J. A. Engelman, Nat. Med., 2016, 22, 262–269 CrossRef CAS.
- K.-A. Song, Y. Hosono, C. Turner, S. Jacob, T. L. Lochmann, Y. Murakami, N. U. Patel, J. Ham, B. Hu, K. M. Powell, C. M. Coon, B. E. Windle, Y. Oya, J. E. Koblinski, H. Harada, J. D. Leverson, A. J. Souers, A. N. Hata, S. Boikos, Y. Yatabe, H. Ebi and A. C. Faber, Clin. Cancer Res., 2018, 24, 5658 CrossRef CAS.
- M. J. Hangauer, V. S. Viswanathan, M. J. Ryan, D. Bole, J. K. Eaton, A. Matov, J. Galeas, H. D. Dhruv, M. E. Berens, S. L. Schreiber, F. McCormick and M. T. McManus, Nature, 2017, 551, 247–250 CrossRef CAS.
- H. Terai, S. Kitajima, D. S. Potter, Y. Matsui, L. G. Quiceno, T. Chen, T. J. Kim, M. Rusan, T. C. Thai, F. Piccioni, K. A. Donovan, N. Kwiatkowski, K. Hinohara, G. Wei, N. S. Gray, E. S. Fischer, K. K. Wong, T. Shimamura, A. Letai, P. S. Hammerman and D. A. Barbie, Cancer Res., 2018, 78, 1044–1057 CrossRef CAS.
- A. C. Obenauf, Y. Zou, A. L. Ji, S. Vanharanta, W. Shu, H. Shi, X. Kong, M. C. Bosenberg, T. Wiesner, N. Rosen, R. S. Lo and J. Massague, Nature, 2015, 520, 368–372 CrossRef CAS.
- M. Crump, S. S. Neelapu, U. Farooq, E. Van Den Neste, J. Kuruvilla, J. Westin, B. K. Link, A. Hay, J. R. Cerhan, L. Zhu, S. Boussetta, L. Feng, M. J. Maurer, L. Navale, J. Wiezorek, W. Y. Go and C. Gisselbrecht, Blood, 2017, 130, 1800–1808 CrossRef CAS.
- A. L. Epstein, R. Levy, H. Kim, W. Henle, G. Henle and H. S. Kaplan, Cancer, 1978, 42, 2379–2391 CrossRef CAS.
- J. Reed, J. Chun, T. A. Zangle, S. Kalim, J. S. Hong, S. E. Pefley, X. Zheng, J. K. Gimzewski and M. A. Teitell, Biophys. J., 2011, 101, 1025–1031 CrossRef CAS.
- D. Huang, K. A. Leslie, D. Guest, O. Yeshcheulova, I. J. Roy, M. Piva, G. Moriceau, T. A. Zangle, R. S. Lo, M. A. Teitell and J. Reed, Anal. Chem., 2018, 90, 3299–3306 CrossRef CAS.
- G. F. Murray, T. H. Turner, K. A. Leslie, M. A. Alzubi, D. Guest, S. S. Sohal, M. A. Teitell, J. C. Harrell and J. Reed, ACS Omega, 2018, 3, 17687–17692 CrossRef CAS.
- H. Bouamar, S. Abbas, A. P. Lin, L. Wang, D. Jiang, K. N. Holder, M. C. Kinney, S. Hunicke-Smith and R. C. Aguiar, Blood, 2013, 122, 726–733 CrossRef CAS.
- G. F. Murray, T. H. Turner, D. Guest, K. A. Leslie, M. A. Alzubi, S. K. Radhakrishnan, J. C. Harrell and J. Reed, Front. Phys., 2019, 7, 158 CrossRef.
- S. Ramanathan, F. Jin, S. Sharma and B. P. Kearney, Clin. Pharmacokinet., 2016, 55, 33–45 CrossRef CAS.
- F. J. Meyer-Almes, Methods Appl. Fluoresc., 2017, 5, 042002 CrossRef.
- C. Poudel, I. Mela and C. F. Kaminski, Methods Appl. Fluoresc., 2020, 8, 024005 CrossRef CAS.
- Q. Zhang, P. Zhang, H. Gou, C. Mou, W. E. Huang, M. Yang, J. Xu and B. Ma, Analyst, 2015, 140, 6163–6174 RSC.
- R. A. Beckman, G. S. Schemmann and C. H. Yeang, Proc. Natl. Acad. Sci. U. S. A., 2012, 109, 14586–14591 CrossRef CAS.
- R. A. Gatenby, A. S. Silva, R. J. Gillies and B. R. Frieden, Cancer Res., 2009, 69, 4894–4903 CrossRef CAS.
- J. Chmielecki, J. Foo, G. R. Oxnard, K. Hutchinson, K. Ohashi, R. Somwar, L. Wang, K. R. Amato, M. Arcila, M. L. Sos, N. D. Socci, A. Viale, E. de Stanchina, M. S. Ginsberg, R. K. Thomas, M. G. Kris, A. Inoue, M. Ladanyi, V. A. Miller, F. Michor and W. Pao, Sci. Transl. Med., 2011, 3, 90ra59 CAS.
Footnote |
† Electronic supplementary information (ESI) available. See DOI: 10.1039/d0an01769h |
|
This journal is © The Royal Society of Chemistry 2021 |
Click here to see how this site uses Cookies. View our privacy policy here.