DOI:
10.1039/D0AN01459A
(Tutorial Review)
Analyst, 2021,
146, 429-443
Application of lectin-based biosensor technology in the detection of foodborne pathogenic bacteria: a review
Received
23rd July 2020
, Accepted 27th October 2020
First published on 27th October 2020
Abstract
Foodborne diseases caused by pathogenic bacteria pose a serious threat to human health. Early and rapid detection of foodborne pathogens is urgently needed. The use of biosensors to identify and detect pathogenic bacteria has attracted ample attention because of their high sensitivity, near real-time quantification without enrichment, on-site detection, simple operation, and so on. As a promising alternative recognition element in biosensors, lectins have been widely studied in bacterial detection because of their high stability and low cost. In this review, we highlight the progress of lectin-based pathogen detection methods, including various electrochemical methods, optical methods and quartz crystal microbalance methods, as well as lectin based microfluidic methods. The interaction mechanism between lectins and bacterial recognition site-sugars is also studied. Finally, the future prospects and challenges in the development of lectin-based biosensors are discussed.
1. Introduction
According to the World Health Organization (WHO) survey results, foodborne diseases cause millions of infections every year, leading to high rates of morbidity and mortality.1 Food safety incidents not only pose a serious threat to the health of people, but also cause huge economic losses to consumers and food-related industries, and adversely affect the social, economic and political stability of a country. Many different diseases, including those caused by bacteria, viruses, parasites, chemicals and prions, can be transmitted to humans through contaminated food, water and the environment.2 Outbreaks of foodborne diseases and sporadic cases frequently occur in countries all over the world. Foodborne disease outbreaks have adversely affected trade and food security.3 Due to the seriousness and harmfulness of foodborne diseases, it is important to pay attention to and solve this problem. A sensitive and efficient detection technology plays a vital role in ensuring food safety, especially in the control of foodborne diseases caused by pathogenic bacteria; unfortunately, existing detection technologies still have flaws in practical application, including but not limited to low sensitivity and poor accuracy. Therefore, it is necessary to develop a fast, accurate and efficient modern food safety detection technology to quickly detect hazard factors in food. Quickly evaluating the hygienic quality of food can prevent the occurrence of food poisoning, effectively controlling foodborne diseases.4,5 In the past few decades, various methods have been developed to detect foodborne pathogens. Common detection methods of various foodborne pathogens are listed in detail in Table 1.
Table 1 Comparison of commonly used bacterial detection methods
Method type |
Method name |
Advantages |
Disadvantages |
Detection samples and limit of detection (LOD) |
Ref. |
Traditional methods |
Traditional microbial culture method |
Simple, high sensitivity, low false positive, mature technology |
Time consuming, expensive instruments, many subjective factors, and high activity requirements |
Hot water systems, 50 cfu L−1, 500 cfu L−1 |
6–9
|
Detection method based on immunology |
Enzyme-linked immunosorbent assay (ELISA) |
Simple, specific, rapid, and low demand for equipment |
Many influencing factors, unstable results, high requirements for operation and high false positive |
Salmonella enteritidis, 105 cfu mL−1; Campylobacter jejuni subsp.jejuni O:23, 50 cfu μL−1 |
10–16
|
Immunomagnetic separation technique |
High sensitivity, high specificity and fast separation speed |
Low purity, large sample demand, and false positive |
Escherichia coli (E. coli) O157:H7, 102 cfu mL−1 |
17–20
|
Immunofluorescence labeling technique |
High sensitivity; high specificity; and high speed |
False positive and subjective |
Vibrio cholerae, 104 cells per 400 ml |
21–23
|
Nucleic acid-based detection method |
Polymerase chain reaction (PCR) |
Mature technology and commercialized |
Expensive equipment, easily contaminated, and high equipment requirements |
E. coli O157:H7, 5 × 102 cells per mL−1 |
24–27
|
Isothermal nucleic acid rapid detection technology |
Simple, fast and specific |
Poor repeatability, easy cross contamination, and high false positives |
Salmonella, S. aureus and Listeria monocytogenes, 10 fg μL−1 |
28–30
|
PCR-DHPLC |
Simple equipment, fast analysis and high sensitivity |
Low stability and accuracy |
Enterobacter sakazakii, 181 cfu mL−1 |
31–35
|
In summary, many existing detection techniques have great limitations, including high costs, experimentation, inflexible conditions, and so on.36,37 In the pursuit of quantification and characterization of pathogens, the biggest analytical challenges are the time-consuming process and lack of sensitivity of the common methods. Fast response time and high sensitivity of biosensors address and solve the analytical challenges of the common methods.38
Electrochemical biosensors,39–41 surface plasmon resonance (SPR) biosensors,42–44 fluorescence biosensors,45 colorimetric biosensors,46 surface enhanced Raman scattering (SERS) biosensors47 and quartz crystal microbalance (QCM) biosensors48,49 are widely used in the detection of foodborne pathogens. In the present, 68 types of recognition elements have been used for the detection of bacteria,50 and lectins are one of the most promising substitutes. By using the sugar-binding physiological function of plant lectins to specifically identify cells, bacteria or glycoproteins, lectin biosensors with high speeds, sensitivity, stability and low costs have been developed.51 Lectins have unique sugar specificity and can bind non-covalently and reversibly with sugars. Because of the wide variety of lectins, they have been used in many fields such as molecular biology, pharmaceutical and clinical medicine and have gradually been applied for the isolation and detection of foodborne pathogenic bacteria due to their specific binding to bacterial surface sugar in eggs and their low cost and high stability compared to antibodies. As the most important recognition method of bacterial detection, bacteria can be used as antigens and their corresponding antibodies as recognition elements. The specific binding between antigens and antibodies is used to develop biosensors to detect bacteria. Antibodies show that the change of charge heterogeneity will affect the spatial conformation of antibodies and even cause changes in biological activity.52 By fixing lectins, a sensor was built to analyze the target, the construction strategy and analysis process of which are shown in Fig. 1, and the lectin sensor can detect bacteria, glycoproteins or whole cells, and analyze the sugar type of the sugar chain, including the screening of tumor markers.53
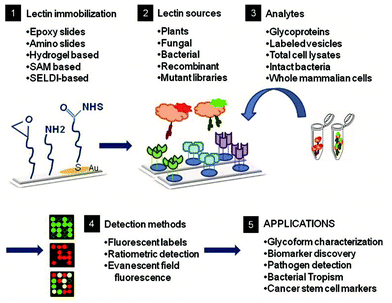 |
| Fig. 1 Construction and application of a lectin sensor.53 | |
Lectins can be used with different detection methods and materials to rapidly detect foodborne pathogens. Concanavalin A (conA) and wheat germ agglutinin (WGA) are commonly used in the establishment of rapid detection methods for foodborne pathogenic bacteria. Other characteristics of lectins have also been used with other bacterial detection techniques and other innovative materials.54,55 Xu et al. established a rapid detection method for E. coli O157:H7 in milk samples based on the observation that the structure of conA changes under different pH conditions, along with immunomagnetic separation and the colloidal gold colouration method. The limit of detection is 41 cfu mL−1.55 Mikaelyan et al. immobilized WGA and lens culinaris agglutinin (LCA) on the surface of silver nanoparticles for the detection of bacteria. The results showed that the detection limits of WGA to S. aureus and E. coli were 103 cells per mL and 3 × 103 cells per mL, respectively. The detection limits of lentil lectin to S.aureus and E. coli were 5 × 103 cells per mL and 5 × 104 cells per mL, respectively.54
There has been a continuous development of a wide variety of nano-materials and detection methods in recent years. As a recognition molecule, lectins can be used with various materials and methods, and have their characteristics be fully utilized. The application prospects in the field of foodborne pathogen detection will become much broader. The recent development of lectin-based detection technologies calls for a systematic and comprehensive review. Herein, we review the applications of lectin-based EIS, amperometric, electrochemical displacement, colorimetric, fluorescence, SPR, SERS and QCM detection techniques in the detection of foodborne pathogens. The combination of these kinds of biosensors with microarray chips and microfluidic chips is the frontier and focus of this field.
2. Interaction mechanism between lectins and specific recognition of pathogenic bacteria
Lectins are a group of proteins extracted from plants or animals, which can agglutinate cells and precipitated glycoconjugates.56 Some contain as much as 55% sugar (such as potato lectins), composed of mainly mannose, glucose and galactose. There are also sugar-free lectins, such as conA, WGA and peanut lectin (PNA). The structures of individual lectins are different. Most of them are rich in aspartic acid, serine and threonine, but low in sulfur-containing amino acids.57 Some lectins contain metal ions needed for their biological activity; for example, each subunit of ConA contains a Mn2+ and a Ca2+. The monomer of ConA (which specifically recognises a murine D-mannose and α-glucose) contains a Mn2+ binding site S1, a Ca2+ binding site S2, and a sugar-binding site.58 The presence of these two metals is necessary for the specific combination of ConA and sugar (Fig. 2).59
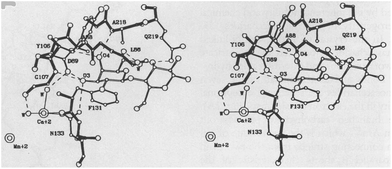 |
| Fig. 2 The combining site of lectin.59 | |
Most lectins are polymerised by subunits, and the specificity of sugar binding to lectins is related to the conformation of the sugar recognition domain of lectins. The interactions between sugars and lectins include hydrophobic accumulation, hydrogen bonds and coordination bonds formed between different sugar ligands and meridian groups; each of the interacting metal ions plays a very important role. Hydrogen bonds are an important force in the recognition for sugar–lectin. There is a strong hydrogen bond between the light group on oligosaccharides and the polar amino acid residue on the protein.60–62
David A. R. Sanders et al. studied the binding of sugar–lectin in ConA and α1–2 dimannose complex with 1.2 Å resolution by using an electron density map63 (Fig. 3).
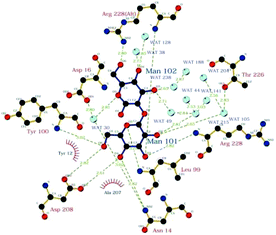 |
| Fig. 3 Schematic of interaction between Man-(αl-2) Man and ConA.63 | |
The theory of lectins as recognition molecules emerged in the 1980s.64 Almost all biological cells carry sugars on the surface. Acting like the antennae of cells, theses monosaccharides or oligosaccharides bind to cellular proteins or phospholipids in a certain way, recognising all kinds of foreign information. The relationship between lectins and related sugars is like the relationship between keys and locks.65
The reason why lectins can bind to specific bacteria is that the cell walls of the bacteria exist in a structure that corresponds to the specific sugars of the lectin. For example, the cell wall of Bacillus anthracis contains galactose-containing polysaccharides, which can be specifically combined with all galactose-specific lectins (such as soybean agglutinin (SBA)). Lipopolysaccharides (LPS) of Salmonella typhimurium (S. typhimurium) and related S. typhimurium contain terminal D glucose residues, so ConA with this sugar specificity can specifically bind to it.57
3. Current lectin-based biosensing methods for foodborne pathogen detection
In the application of biosensors, the biological interactions between lectins and polysaccharides are transformed into signals that are easy to quantify, analyse and present in a user-friendly manner. These biosensors are simple, fast and cheap analytical equipment, and are expected to be a useful tool for bacterial detection.58,60–62,64–66 In recent years, lectin-based biosensors using different detection techniques such as electrochemistry methods67–73 and optical methods42,74–77 have been proposed and widely studied. Among them, electrochemical technology, especially electrochemical impedance spectroscopy (EIS), has been more and more widely used in various bacterial biosensors because of its simplicity and high sensitivity.
3.1 Lectin-based electrochemical biosensing methods
Electrochemical biosensors are portable, small in size and low cost, and use electrodes (solid electrodes, ion-selective electrodes or gas sensing electrodes) as signal conversion elements and fixed carriers, and biologically sensitive substances (such as enzymes, antigens, antibodies, hormones, and lectins) or organisms themselves (cells, organelles, and tissues) as sensing elements. Through the specific recognition between biomolecules and the target molecules, their reaction signals are converted into measurable electrical signals, such as capacitance, current, potential and conductivity to realize the quantitative or qualitative detection of target analytes (Fig. 4).78–80 The electrochemical biosensor is a kind of biosensor widely used in the detection of foodborne pathogenic bacteria. Electrodes act as electron donors or receivers, which can simulate the electron transfer mechanism and metabolic process of biological systems, and determine thermodynamic and kinetic parameters. The specificity of the biological reaction, sensitivity and real-time detection of electrical analysis methods are used to provide a powerful means for the detection of biological substances.81,82 There are several electrochemical techniques that can be applied to biosensors.83,84
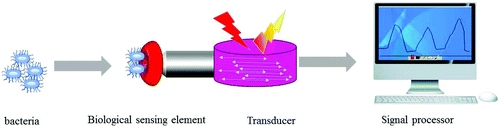 |
| Fig. 4 A schematic of a biosensor with an electrochemical transducer. | |
The first application of electrochemical detection in sugar biology was reported by Joshi and Wang in 2006. PNA was immobilized on a gold substrate coated with a mixed self-assembled monolayer (SAM). The competitive binding degree between nanocrystalline labeled sugars and analytes was monitored by sensitive amperometric stripping voltammetry. The use of nanoparticle tracing for monitoring lectin–sugar interaction and a bioassay of disease-related sugar chain markers was demonstrated for the first time.85
3.1.1 EIS biosensing methods.
The lectin molecule is smaller than the antibody, so it is easy to form a denser biofilm on the electrode surface, giving the sensitivity of detection. LaBelle et al. developed a compact biosensor without labeling for rapid (<80 s) AC impedance detection of lectin interactions for the first time.86 EIS can detect analytes at the single molecular level in an unlabeled manner and the change of the resistance of the layer on the electrode surface to the redox probe in solution. This is due to the fact that the higher the amount of protein/DNA on the surface of the biosensor, the higher the resistance of this layer, and this can be used for the quantification of analytes.87 An unlabeled impedance biosensor based on lectins has been developed to detect E. coli. ConA and E. coli are immobilized on the surface of the screen-printed gold electrode through the formation of a lectin–bacteria complex. The detection range of the biosensor was 5.0 × 103–5.0 × 107 cfu mL−1. This EIS biosensor shows high selectivity and high sensitivity.88 By optimizing the working conditions of the impedance sensor, such as the pH value of the buffer and the incubation time of lectins, ConA was immobilized on the surface of a gold electrode assembled with 1-hydrophobic undecanoic acid to detect sulfate-reducing bacteria (SRB). The detection range of SRB concentration is 1.8–1.8 × 107 cfu mL−1.89 The method has the following advantages: it is simple, low cost and time saving. Making use of the rich lectin binding sites on the surface of bacteria, WGA was further used to enhance the signal. Therefore, an impedance biosensor using a novel screen-printed cross microelectrode (SPIMS) and WGA for signal amplification was proposed for the detection of E. coli O157:H7. The detection limit of the electrochemical impedance immunosensor is 102 cfu mL−1, and the linear range is 102–107 cfu mL−1. The total detection time is less than 1 hour, and it has considerable sensitivity and a fast response speed (Fig. 5).90
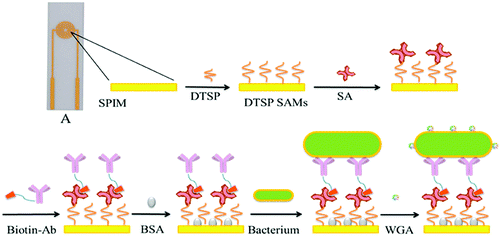 |
| Fig. 5 An electrochemical impedance immunosensor based on self-assembled monolayers was proposed for the rapid detection of E. coli O157:H7 with signal amplification using WGA.90 | |
It is also interesting to study the charge transfer resistance and anodic current peak of an endotoxin contaminated biosensor by electrochemical impedance spectroscopy and cyclic voltammetry. An electrode interface was constructed using an L-cysteine-gold nanoparticle (AuNpCys) composite to be immobilized by electrostatic interaction in the network of a poly(vinyl chloride-vinyl acetate maleic acid) (PVM) layer on a bare gold electrode.
CramoLL lectin was self-assembled onto a PVM-AuNpCys modified gold electrode, and an impedance biosensor was prepared by electrostatic interaction (Fig. 6).91
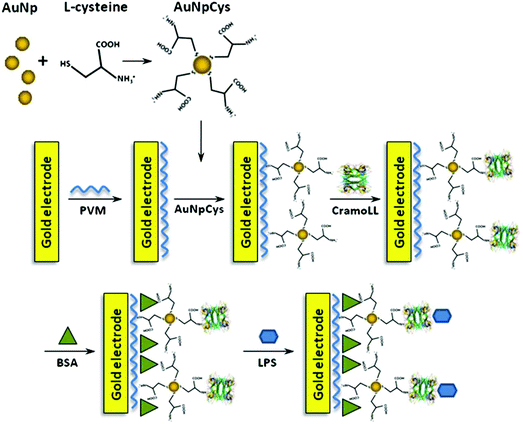 |
| Fig. 6 Mechanism for the reaction of Cys with AuNp and schematic representation of the PVM–AuNpCys–CramoLL–BSA–LPS biosensor system.91 | |
The group developed an unlabeled lectin biosensor, which can quantitatively determine the binding of lectins immobilized on the surface of carbohydrate sensors to LPSs on Gram-negative bacteria for an antibiotic sensitivity test. The sensor uses the quinone group on the polythiophene interface to form a carbohydrate platform for immobilization of ConA. It can measure LPS binding by orthogonal quartz crystal microbalance and electrochemical readouts (EQCM). This orthogonal conversion provides cross-validation, better sensor sensitivity and a dynamic range of measurements.92 The assembly process on the modified electrode was characterized by EIS and scanning electron microscopy (SEM). EIS measurements showed that the resistance charge transfer (RCT) at the electrode/electrolyte interface increased significantly after the interaction of ConA lectins with specific carbohydrates in bacterial toxin molecules. Our results show that ConA lectin still retains its activity after being immobilized on the surface of electrosynthesized polyaniline (PANI), and there is an electrochemical impedance response of the bioelectrode, which is linearly related to the degree of lectin–toxin interaction, and the RCT value for E. coli is the maximum (14.40 Ω k) and that for S. aureus is 17.80 Ω k. The electrosynthesised PANI thin film is a good supporting layer for the covalent binding of lectins on the electrode surface. Therefore, the recognition system provides a suitable bionic interface for the detection of specific components of Gram-positive and Gram-negative bacteria.93 Using ferrocene (FC) as an electrochemical redox marker is a promising way to develop amperometric and voltammetric biosensors with high sensitivity.94
3.1.2 Amperometric biosensing methods.
An enzymatic glucose electrochemical biosensor is a kind of electrochemical glucose biosensor based on glucose oxidase modified by various substrates. Tangbo et al. prepared a novel amperometric biosensor for nonenzymatic glucose. Based on the biospecific binding of ConA to D-glucose on the methionine (TH) modified electrode, ConA was immobilized on the methionine modified electrode, and the sensor surface was established for the specific recognition of glucose. The ConA biosensor has a good linear relationship in the concentration range of 1.0 × 10−6–1.0 × 10−4 M, and the detection limit of D-glucose is 7.5 × 10−7 M. Due to the special adhesion mechanism of polydopamine (pDA) to TH and ConA amino groups through Michael addition and Schiff base reactions, the biosensor platform shows high stability, which shows that the TH signal is almost not weakened during multiple scans, and the biosensor has a good long-term stability speed (Fig. 7).95 The team also prepared a new polythiophene interface, which contains the fused quinone part, and then glycosylated it to form a carbohydrate platform for bacterial detection. Importantly, the interface can be detected by electrochemical and QCM sensors and by direct fimbria-mannose binding and LPSs mediated by ConA. The sensor can work in either electrochemical mode or weight mode. In the electrochemical measurement, the peak current of the sensor by square wave voltammetry decreases with the increase of concentration, which is due to the interference of the electron transfer of quinone-viscous thiophene. In the QCM detection mode, on the other hand, the resonance frequency of the sensor varies with the concentration of E. coli. The detection limit of the electrochemical sensor is 25 cells per mL, the detection limit of the QCM sensor is 50 cells per mL (Fig. 7 and Fig. 8).96 ConA acts as a recognition element for bacterial toxins (Fig. 9).
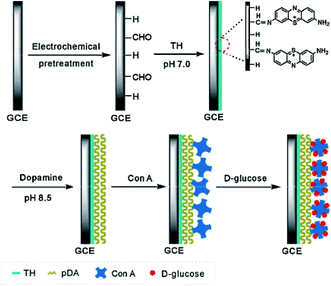 |
| Fig. 7 Schematic representation of the fabricated glucose amperometric biosensor.95 | |
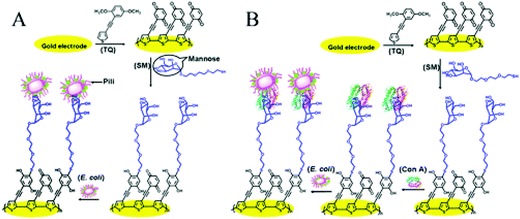 |
| Fig. 8 Schematic diagrams of different E. coli detection modes: (A) direct detection of E. coli using fimbria–mannose binding and (B) detection of E. coli mediated by LPS-mannose-bound ConA.96 | |
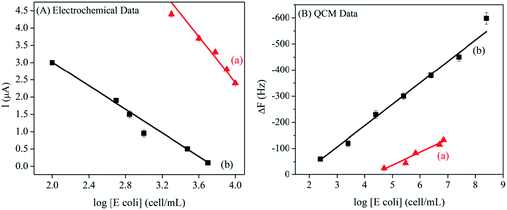 |
| Fig. 9 The calibration curve of the sensor signal is drawn according to the logarithm of E. coli concentration, in which (A) represents the SWV electrochemical signal and (B) represents the QCM frequency signal. In addition, (A) describes the fimbria–mannose binding through the SM/TQ interface, and (B) describes the ConA-mediated binding through the ConA/SM/TQ interface.96 | |
3.1.3 Electrochemical displacement biosensing methods.
An electrochemical displacement sensor does not require a step after the addition of the target analyte. It is considered to be reagent-less and practical for on-site applications. Firstly, the gold electrode was modified with thio-mannose/oligoethylene glycol (OEG) conjugated self-assembled monolayers, and then FC boroxyl alcohol derivatives were pre-assembled on the surface of mannose as a reporter molecule. The sensor has also been successfully applied for the detection of E. coli, and the detection limit is about 6 × 102 cells per ml. The fast response time is 15 min. It can still be further developed into a portable sensor device (Fig. 10).97 Proteins and polymers modified with synthetic lectins such as boroxyalcohol and boric acid are also interesting.98–102
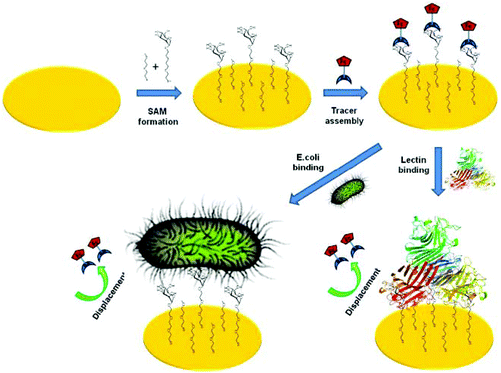 |
| Fig. 10 Schematic representation of electrochemical displacement assay for carbohydrate binding protein and E. coli detection.103 | |
3.1.4 Electrochemical biosensors combined with lectin microarrays.
Currently, electrochemical sensors based on lectin arrays are also being developed. Ertl and Mikkelsen developed a lectin-based sensor array to qualitatively distinguish bacterial strains. The lectin modified membrane was fixed on the surface of a platinum electrode to obtain the electrochemical signal of respiratory and circulatory activity. The cells captured on the lectin modified Immunodyne ABC membrane and physically immobilized on the Pt working electrode were measured by the chronovolumetric method. The lectin-based sensor array was exposed to live cells of Gram-negative and Gram-positive bacteria and yeast, and chronocoulometry was performed to produce a response pattern of each organism to each lectin. Principal component analysis was used to classify the chronocoulometric results for the different microbial strains. Through this new method, six microbial species (Bacillus cereus, Staphylococcus aureus (S. aureus), Proteus vulgaris, E. coli, Enterobacter aerogenes, and Saccharomyces cerevisiae) can be easily identified.104 This strategy has the advantages of simple and low-cost manufacturing of a controllable and reproducible lectin interface. This has potential to develop new platforms for the detection of pathogenic bacteria.
3.2 Optical biosensing methods
Optical biosensors are very attractive because they can directly carry out untagged real-time detection of bacteria. They are often used for medical purposes and are promising for assessing many diseases, which improves people's quality of life. Optical sensors have several important advantages. The performance of the optical sensor is not sensitive to electromagnetic interference. In addition, optical sensors do not need electrical signals in the sensing area, so they can operate in the hazardous environment of industrial factories. Several types of optical biosensors have been demonstrated for the detection of chemical contaminants, bacterial pathogens and toxins in food.105,106 Many of the optical biosensors we introduce here are based on the combination of lectins and magnetic nanoparticles and the specific recognition of lectins to detect pathogens (Fig. 11).
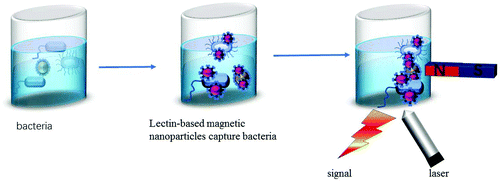 |
| Fig. 11 A schematic of an optical biosensor. | |
3.2.1 Colorimetric biosensing methods.
The latest development of the colorimetric biosensor shows great potential; it is highly sensitive, fast, portable, cost-effective, and can meet the actual needs of rapid detection.107 Xu et al. proposed a new colorimetric biosensor that uses antibody-modified magnetic nanoparticles to capture and concentrate E. coli O157:H7, then label it with ConA, adjust the pH value to dissociate the ConA tetramer to release the dimer, collect it and re-form the tetramer after changing the pH value to cause the aggregation of dextran modified H7. Because of ConA's interesting pH-dependent conformation-transformation behavior, ConA can be creatively released from the bacterial surface and then reconstructed based on pH regulation for the rapid detection of E. coli O157:H7 in milk samples based on the pH regulation of dimers/tetramers with ConA and ConA-glycosyl groups. The biosensor showed a detection limit as low as 41 cfu mL−1, a short detection time (less than 95 minutes) and satisfactory specificity. The biosensor can also be used for the analysis of milk samples.108 The pH dependent conformation conversion of ConA can also be used in the design of other intelligent response devices. Moreover, mannose derivatives have been self-assembled onto preformed citrate-capped water-soluble gold nanoparticles and developed as a stable colloidal system for the detection of carbohydrate-binding proteins by colorimetry. By using a short (C2) hydrocarbon chain between the gold surface and the mannose recognition center, a selective, quantitative and important colorimetric method for analyzing carbohydrate binding proteins with ConA has been developed (Fig. 12).109
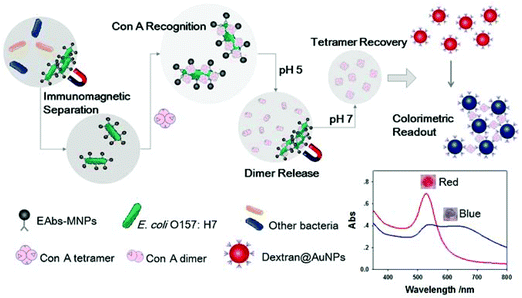 |
| Fig. 12 Schematic diagram of a colorimetric biosensor.109 | |
3.2.2 Fluorescent biosensing methods.
Fluorescence-based sensors have high sensitivity, but the use of tags requires multi-step detection protocols to extend the detection time, and the finely balanced affinity of interacting biomolecules for displacement analysis leads to the cross-sensitivity of the sensor to non-target analytes.105 Hovhannisyan et al. used an eggshell lectin-sensitized quantum dot–silver nanoparticle hybrid system as the recognition ligand to measure the optical changes caused by the interaction of sensitized nanoparticles with bacteria to detect S. aureus. The fluorescence change caused by the resonance energy transfer between AgNPs and bacteria during the interaction between quantum dots (QDs) and bacteria was detected. The application of hybrids provides high sensitivity analysis and allows the detection limit of S. aureus to be 6 × 103 cells per mL−1.110 A lectin-functionalized ZnO nanorod (ZnO-NR) array has been used as a three-dimensional nano-biological interface. E. coli was detected by fluorescence microscopic imaging through the multivalent binding of ConA to E. coli cell surface polysaccharides. The ZnO-NR array detection method based on three-dimensional lectin functionalization has a reasonable detection limit and good linear range (1.0 × 103–1.0 × 107cfu mL−1) (Fig. 13).111
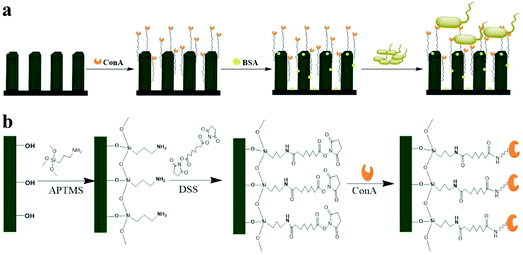 |
| Fig. 13 The schematic of a ConA-functionalized 3D ZnO-NR array is used for bacterial capture (a), and the immobilization of bacterial binding molecules on the surface of 3D ZnO-NR (b).111 | |
3.2.3 SPR biosensing methods.
SPR is a powerful optical biosensor technique for non-labeled biological affinity interaction analysis (antigen–antibody, lectin–sugar and receptor hormone). This is a phenomenon that occurs in the process of optical illumination on the metal surface, which can be used to analyze the interaction of biomolecules.112 SPR allows real-time, continuous monitoring of interaction processes, allowing for the use of highly automated equipment for routine analysis without training. A novel SPR biosensor using lectins as biological receptors for the rapid detection of E. coli O157:H7 was prepared. The lectins were detected by the recognition principle of selective interaction between lectins and carbohydrate components on the surface of bacterial cells. When Triticum Vulgaris lectin was used as a binding molecule, the detection limit of E. coli O157:H7 was 3 × 103 cfu mL−1. The results show that the lectin-based SPR biosensor is sensitive, reliable and effective for the detection of E. coli O157:H7, which has a broad scope in food safety analysis (Fig. 14).113
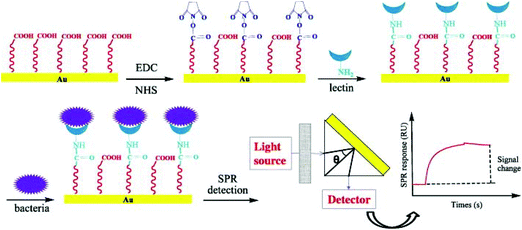 |
| Fig. 14 Schematic of a surface plasmon resonance biosensor based on lectin for the detection of E. coli O157:H7.113 | |
3.2.4 SERS biosensing methods.
SERS is an ultra-sensitive selective analytical technique, which can be used to detect target molecules attached to or adjacent to the surface of precious metal nanoparticles.114 The enhancement effect observed is several orders of magnitude larger than that reported by traditional Raman scattering. However, the enhancement effect varies greatly according to the difference between the analyte and substrate.114,115 The lectins were detected by the recognition principle of selective interaction between lectins and carbohydrate components on the surface of bacterial cells. When Triticum Vulgaris lectin was used as a binding molecule, the detection limit of E. coli O157:H7 was 3 × 103 cfu mL−1. The results show that the lectin-based SPR biosensor is sensitive, reliable and effective for the detection of E. coli O157:H7, which has a broad prospect in food safety analysis.116–128 The SERS of a variety of bacterial strains have been obtained on the SiO2 substrate excited at 785 nm on gold nanoparticles. On these SERS active substrates, the Raman cross-sections of both Gram-positive and Gram-negative bacteria were enhanced by more than 104. The surface enhancement makes it possible to observe the Raman spectrum of a single bacterial cell excited by low incident power and short data acquisition time. The SERS spectrum of Bifidobacterium anthracis shows the ability to analyze at the single cell level. These results demonstrate the potential of SERS to detect and identify species-and strain-specific bacterial pathogens on these vitreous substrates covered with gold particles.129 SERS has also been used to study carbohydrate–lectin interactions on the surface of mammalian cells. Lectin-functionalized silver nanoparticles were used to detect the expression of carbohydrates at the cell interface.130 The research group derived this method for the detection of bacterial pathogens, using lectin-functionalized magnetic nanoparticles to capture and isolate bacteria from the sample matrix, combined with SERS technology to develop a new type of biosensor for the isolation and detection of a variety of bacterial pathogens. This new detection method includes the use of SERS-active nanoparticles with strain-specific antibodies to specifically detect bacterial pathogens. Therefore, the sample is captured by “magnetic beads” and detected by Raman spectroscopy, achieving simple and fast optical detection. Three kinds of bacteria (E. coli, S. typhimurium and methicillin-resistant S. aureus) were isolated and detected successfully with this method. The lowest detection concentration of each strain was only 101 cfu mL−1. In addition to the detection of single pathogens, the mixture of three strains of bacteria was isolated and identified by SERS in the same sample matrix, and the triple detection was confirmed by principal component analysis. The multi-channel biosensor has the advantages of a fast detection speed and high sensitivity, and can be used to detect bacterial pathogens in single and multiple channels76 (Fig. 15). SERS sandwiched determination of lectins can detect fungal cell wall polysaccharides with a sensitivity less than 200 pg ml−1.131
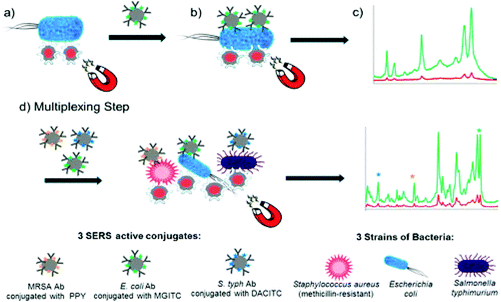 |
| Fig. 15 Schematic illustration of single-plex and multiplex SERS methods for the detection of pathogenic bacteria.76 | |
3.3 Lectin-based quartz crystal microbalance methods
QCM equipment is a kind of mass sensor, which is very suitable for the detection of high molecular weight analytes.132 The main advantage of QCM in biological measurement is that it can measure very small quality changes and monitor quality deposition in real time. In addition, such measurements can be made using natural molecules without labeling.133 The use of QCM is based on the change of quartz crystal resonance frequency caused by the mass accumulation resulting from carbohydrate–lectin interaction. Lectins were used to bridge specific carbohydrate structures and bacteria with complementary carbohydrate pockets. Functional mannose self-assembled monolayers combined with ConA were used as molecular recognition elements. Finally QCM was used as a sensor to detect E. coli W1485. The multivalent binding of ConA to O antigen on the surface of Escherichia coli promotes the strong adhesion of Escherichia coli to the mannose-modified QCM surface by forming bridge bonds between them. The results showed that the sensitivity and specificity of the carbohydrate QCM biosensor were significantly improved and the detection limit was hundreds of bacterial cells, 7.5 × 102–7.5 × 107cells per mL−1 (Fig. 16 and 17).134 Serra et al. developed a method of using ConA and E. coli to evaluate lectin immobilization methods and biosensors and to detect and quantify microorganisms by immobilizing lectins on the surface of gold-plated quartz crystals. The detection limit of E. coli is 1.0 × 104 cfu mL−1, when using free bacteria binding to lectins. Introducing a filter into the measurement cell can significantly change the frequency of E. coli at a concentration of 1.0 × 103cfu mL−1, further improving the sensitivity, and distinguish between living cells and non-living cells.135
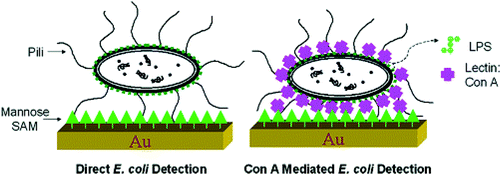 |
| Fig. 16 Schematic representation of direct E. coli detection and Con A-mediated E. coli detection.134 | |
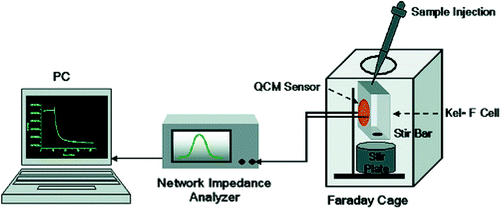 |
| Fig. 17 QCM sensor setup.134 | |
A new method for identifying Campylobacter jejuni strains based on lectin–bacteria interaction characterized seven strains of Campylobacter jejuni by lectin typing. Each strain had different dissipation patterns measured by QCM sensors. Four kinds of lectins (WGA, Maackia amurensis lectin, Lens culinaris lectin and con A) were used to select the recognized bacteria according to their different carbohydrate specificity. The gold surface of a quartz crystal was modified with 11-mercaptoundecanoic acid. Bacterial cells can be used for lectin typing without preliminary treatment.136 The advantage of this method is that the analysis results are reliable and repeatable, and it avoids the necessity of bacterial sample preparation. This method can also be further developed to be suitable for the identification of other bacterial species, especially pathogens. Lectins can also be immobilized on the surface of 11-mercaptoundecanoic acid modified crystals by amine coupling, which is a new method for flow injection detection of pathogenic enterobacteria based on the lectin QCM biosensor. Biosensors can identify the presence of different bacteria using lectins immobilized on the surface of QCM crystals, which specifically bind to some oligosaccharides injected into the bacterial cell wall. The biosensor has a detection limit of 103 cells. The determination of bacterial cells by flow injection takes about 30 minutes. This method can achieve rapid and sensitive quantitative analysis, and it does not lose the activity of lectins when used many times.137 The QCM biosensor can be used for the selective detection of marine pathogenic SRB, based on vancomycin-functionalized magnetic nanoparticles (Van-mNPs) in an external magnetic field with bacteria–mNP conjugates attached to the gold electrode surface. The QCM biosensor showed obvious response to vancomycin-sensitive S. aureus, but not to vancomycin-resistant Vibrio anguillarum. There is a good linear relationship between QCM response and the logarithm of bacterial concentration in the range of 1.8 × 104–1.8 × 107cfu mL−1. The sensor system has a broad application prospect and provides a simple and convenient method for the detection of pathogenic bacteria.138
3.4 Lectin-based microfluidic methods
The field detection technology of microfluidic chip is a rapid detection technology developed in the past decade. Because the microfluidic technology has the characteristics of sample flow control, chip closure, small pipeline and strong controllability, the detection sensitivity and repeatability of microfluidic chip field detection technology are improved in varying degrees compared with the traditional detection technology. Therefore, the field detection technology of microfluidic chip is favoured more and more by the market. The combination of microfluidic chip technology and lectins as a means of bacterial detection is also the focus of many researchers’ attention.139,140 Nguyen et al. developed a highly sensitive nano-biosensor based on isothermal amplification, which combines microfluidic enrichment with conA functionalized microchannels and asymmetric herringbone groove arrays for the rapid detection of pathogens. The experimental results show that this unlabeled method can real-time detect the serum concentration of S. typhimurium in urine samples (10 mL) as low as 5 cfu mL−1. In addition, the use of microfluidic enrichment increased the sensitivity by 1.76 orders of magnitude. The whole experiment was completed in 100 minutes141 (Fig. 18). This work has demonstrated that an integrated microfluidic system can automate the entire process to detect Salmonella or other pathogenic bacteria causing urinary tract infection. The whole process, including bacterial isolation, cell lysis, nucleic acid amplification and optical detection, can be performed on a single chip.
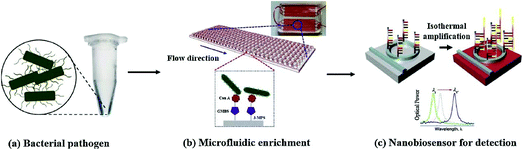 |
| Fig. 18 The principle of rapid and efficient detection of bacteria by the combination of the microfluidic enrichment method and DNA nano-biosensor.141 | |
4 Conclusion and prospects
Lectins have unique sugar specificity and can bind non-covalently and reversibly with sugars. Because of their wide variety, lectins have been used in many fields such as molecular biology, pharmacology, clinical medicine and so on. Lectins have been gradually applied for the isolation and detection of foodborne pathogenic bacteria because of their specific binding ability to bacterial surface sugar in eggs and their low cost and high stability compared with antibodies. Currently, the application of lectins in the isolation and detection of foodborne pathogens is gradually maturing, and it is not uncommon for concanavalin A and wheat germ lectins to be used in the rapid detection of foodborne pathogens. There are more reports that were not discussed.142 Further application of other characteristics of lectins, combined with other bacterial detection techniques, led to the innovative development of rapid detection methods. Despite these developments, some key issues still need to be solved in future research, and we speculate on some of these directions. The main issues are as follows:
(1) The selectivity of lectin-based methods is one of the biggest challenges in the detection of some target bacteria. Lectins are specific for bacterial recognition, but they are not as specific as antibodies. Moreover, it is difficult to achieve specific recognition in multiple and complex real environments. To understand the interaction between bacterial surfaces and lectins immobilized in biosensors and to improve the recognition system of lectins are important. Lectin microarrays, combined with immunomagnetic separation and PCR technology, can help to improve the selectivity.
(2) The sensitivity of the new method based on lectin is sufficient, and the biosensor can replace the traditional methods and meet the needs of food safety. According to the review of the literature, the LOD value of most lectin-based methods is 103 cfu mL−1, but that of microfluidic methods can be as low as 5 cfu mL−l, so work is still needed to enhance the signal, for example, the use of excellent nanomaterials combined with microfluidic methods.
(3) The existing reports on the application of lectins for the detection of foodborne pathogens still have great limitations. The types of lectins used are very limited, and the development and utilization of various lectins is not thorough enough, especially in practical application. With the continuous development of related fields of biology, people have obtained a deeper understanding of lectins. We need to constantly develop new lectins for the detection of bacteria. It is believed that lectins will be able to play a greater role in the field of rapid detection of foodborne pathogens. There is an urgent need to develop an easy-to-use, cost-effective, rapid and sensitive field detection method for foodborne pathogens. With the development of nanomaterials and emerging microfluidic technologies, many new lectin-based detection systems integrated with these emerging technologies are expected to successfully be able to perform on-site real-time detection of foodborne pathogens.
In conclusion, with the rapid development of nanotechnology, biotechnology and microfluidic technology, many new lectin-based detection systems combined with these emerging technologies are expected to be successful in the detection of foodborne pathogens in the future.
Abbreviations
WHO | World Health Organization |
ELISA | Enzyme-linked immunosorbent assay |
PCR | Polymerase chain reaction |
SPR | Surface plasmon resonance |
SERS | Surface enhance Raman scattering |
QCM | Quartz crystal microbalance |
ConA | Concanavalin A |
WGA | Wheat germ agglutinin |
E. coli
|
Escherichia coli
|
PNA | Peanut lectin |
SBA | Soybean agglutinin |
LPS | Lipopolysaccharide |
EIS | Electrochemical impedance spectroscopy |
SAM | Self-assembled monolayer |
SRB | Sulfate-reducing bacteria |
SPIMS | Screen-printed cross microelectrode |
AuNpCys | Cysteine–gold nanoparticle |
PVM | Polyvinyl chloride-vinylacetate maleic acid |
EQCM | Quartz crystal microbalance and electrochemical readouts |
SEM | Scanning electron microscope |
RCT | Resistance charge transfer |
PANI | Electrosynthesized polyaniline |
FC | Ferrocene |
OEG | Oligoethylene glycol |
TH | Methionine |
pDA | Polydopamine |
S. typhimurium
|
Salmonella typhimurium
|
S. aureus
|
Staphylococcus aureus
|
QDs | Quantum dots |
ZnO-NR | ZnO nanorod array |
Van-mNPs | Vancomycin-functionalized magnetic nanoparticles |
Conflicts of interest
The authors declare no competing financial interest.
Acknowledgements
This work was supported by Xinjiang Uygur Autonomous region Natural Science Foundation Project (2019D01A69), Xinjiang Uygur Autonomous region University Scientific Research Program Key Project (XJEDU20191019), the Scientific Research and Development Project of Xinjiang Normal University (XJNUZX202003), the Doctoral Research and Innovation Program of Xinjiang Normal University (XJ107622007), and the National Natural Science Foundation of China (22064016).
References
- C. Stein, T. Kuchenmuller, S. Hendrickx, A. Pruss-Ustun, L. Wolfson, D. Engels and J. Schlundt, PLoS Neglected Trop. Dis., 2007, 1, e161 CrossRef.
- E. Scallan, R. M. Hoekstra, F. J. Angulo, R. V. Tauxe, M. A. Widdowson, S. L. Roy, J. L. Jones and P. M. Griffin, Emerging Infect. Dis., 2011, 17, 7–15 CrossRef.
- C. Frank, D. Werber, J. P. Cramer, M. Askar and G. Krause, N. Engl. J. Med., 2011, 365, 1771–1780 CrossRef CAS.
- C. Frank, D. Werber, J. P. Cramer, M. Askar and G. Krause, N. Engl. J. Med., 2011, 365, 1771–1780 CrossRef CAS.
- S. Vigneshvar, C. C. Sudhakumari, B. Senthilkumaran and H. Prakash, Front. Bioeng. Biotechnol., 2016, 4, 11 CAS.
- A. K. Bhunia, Future Microbiol., 2014, 9, 935–946 CrossRef CAS.
- K. J. Lee, S. L. Wang, A. Hwang, J. Moon, T. Kang, K. Park and J. Jeong, Analyst, 2017, 143, 332–338 RSC.
- M. A. Abdou, Das Medizinische Laboratorium, 1982, 35, 123 CAS.
- O. Lazcka, F. J. D. Campo and F. X. Muñoz, Biosens. Bioelectron., 2007, 22, 1205–1217 CrossRef CAS.
- E. P. Krysinski and R. C. Heimsch, Appl. Environ. Microb., 1977, 33, 947–954 CrossRef CAS.
- S. A. Minnich, P. A. Hartman and R. C. Heimsch, Appl. Environ. Microb., 1982, 43, 877–893 CrossRef CAS.
- Q. Wen, X. Jiao, X. Liu, R. Zhang and Y. Jing, Chin. J. Vet. Sci., 1995, 2, 105–111 Search PubMed.
- R. L. Brigmon, S. G. Zam, G. Bitton and S. R. Farrah, J. Immunol. Methods, 1992, 152, 135–142 CrossRef CAS.
- I. Hochel, D. Viochna, J. Škvor and M. Musil, Folia Microbiol., 2004, 49, 579–586 CrossRef CAS.
- M. Vaz-Velho, G. Duarte and P. Gibbs, J. Microbiol. Methods, 2000, 40, 151 CrossRef.
- J. L. Ferreira, J. AOAC Int., 2019, 1 CAS.
- K. S. Cudjoe, T. Hagtvedt and R. Dainty, Int. J. Food Microbiol., 1995, 27, 11–25 CrossRef CAS.
- B. M. Taban, U. Ben and S. A. Aytac, J. Dairy Sci., 2009, 92, 2382–2388 CrossRef CAS.
- X. Wang and M. F. Slavik, J. Food Prot., 1999, 62, 717 CrossRef CAS.
- Q. Q. Tang, J. P. Wang, Z. Z. Ye, L. Gai and Y. B. Ying, Spectrosc. Spect. Anal., 2009, 29, 2614–2618 CAS.
- B. Yan, A. C. Cheng, M. S. Wang, S. X. Deng, Z. H. Zhang, N. C. Yin, P. Cao and S. Y. Cao, World J. Gastroenterol., 2008, 14, 776–781 CrossRef.
- B. Yan, A. C. Cheng, M. S. Wang, S. X. Deng, Z. H. Zhang, N. C. Yin, P. Cao and S. Y. Cao, World J. Gastroenterol., 2008, 14, 776–781 CrossRef.
- X. Q. Li, M. Wang, H. Yi, Y. F. Liu, Z. Y. Mo, B. Huang, L. Y. Jiang, Y. Liu, J. W. Fan and J. H. Li, China Public Health, 2005, 12, 1437–1438 Search PubMed.
- Y. H. Fan, Q. P. Wu, R. Q. Wu, X. X. Kou, J. M. Zhang and H. Q. Wu, Microbiology, 2005, 32, 102–107 Search PubMed.
- D. N. Fredricks, T. L. Fiedler, K. K. Thomas, B. B. Oakley and J. M. Marrazzo, J. Clin. Microbiol., 2007, 45, 3270–3276 CrossRef CAS.
- J. A. Higgins, S. Nasarabadi, J. S. Karns, D. R. Shelton, M. Cooper, A. Gbakima and R. P. Koopman, Biosens. Bioelectron., 2003, 18, 1115–1123 CrossRef CAS.
- F. Zhu, S. Rogelj and T. L. Kieft, Int. J. Food Microbiol., 2005, 99, 47–57 CrossRef.
- N. Tsugunori, O. Hiroto, M. Harumi, Y. Toshihiro, W. Keiko, A. Nobuyuki and H. Tetsu, Nucleic Acids Res., 2000, 12 Search PubMed.
- K. Jiang, Q. F. Lv, X. Wang, L. Xia, Z. Zhang and X. Z. Chen, Food Sci., 2013, 34, 192–197 Search PubMed.
- K. Jiang, H. Zhang, X. Wang, P. P. Liu, J. F. Huang and Z. N. Zhou, Food Mach., 2015, 31, 92–97 Search PubMed.
- D. F. Zhang, F. Yuan, K. Wang, H. R. Yang, Y. Hu, Y. S. Zhao, Y. Chen and Y. Q. Ge, China Public Health, 2012, 28, 1515–1518 CAS.
- Y. K. Qian, J. Yuan, F. Gao, H. Y. Wang, Y. X. Xiao and J. J. Zhang, Chin. J. Health Lab. Technol., 2014, 24, 2014–2016 CAS.
- F. J. Yang, Y. P. Wang, Y. N. Wu and J. Z. Shen, Chin. J. Food Hyg., 2010, 22, 389–392 Search PubMed.
- C. S. Kodama, S. Cuadros-Orellana, C. H. M. M. Bandeira, D. A. Graças, A. S. Santos and A. Silva, Genet. Mol. Res., 2014, 13, 1304–1313 CrossRef CAS.
- Y. ChunHu, C. JiJuan, G. JiaXiang and Z. Yi, Microbiology, 2008, 35, 1845–1849 Search PubMed.
- V. Velusamy, K. Arshak, O. Korostynska, K. Oliwa and C. Adley, Biotechnol. Adv., 2010, 28, 232–254 CrossRef CAS.
- O. Lazcka, F. J. D. Campo and F. X. Muñoz, Biosens. Bioelectron., 2007, 22, 1205–1217 CrossRef CAS.
- R. Pourakbari, N. Shadjou, H. Yousefi, I. Isildak, M. Yousefi, M. R. Rashidi and B. Khalilzadeh, Mikrochim. Acta, 2019, 186, 820 CrossRef CAS.
- E. Cesewski and B. N. Johnson, Biosens. Bioelectron., 2020, 159, 112214 CrossRef CAS.
- Z. Zhang, J. Zhou and X. Du, Micromachines, 2019, 10, 2–16 Search PubMed.
- S. Muniandy, S. J. Teh, K. L. Thong, A. Thiha, I. J. Dinshaw, C. W. Lai, F. Ibrahim and B. F. Leo, Crit. Rev. Anal. Chem., 2019, 1–24 Search PubMed.
- A. A. Bergwerff and V. K. Frans, J. Aoac Int., 2019, 3 Search PubMed.
- J. Zhou, Q. Qi, C. Wang, Y. Qian and L. Fu, Biosens. Bioelectron., 2019, 142, 111449 CrossRef CAS.
- F. C. Dudak and S. H. B. Dr, Biotechnol. J., 2009, 4, 1003–1011 CrossRef CAS.
- N. Elahi, M. Kamali, M. H. Baghersad and B. Amini, Mater. Sci. Eng., C, 2019, 105, 110113 CrossRef CAS.
- Y. Zhang, W. Tan, Y. Zhang, H. Mao and J. Yu, Biosens. Bioelectron., 2019, 142, 111570 CrossRef CAS.
- A. Kowalczyk, J. Krajczewski, A. Kowalik, J. L. Weyher, I. Dzięcielewski, M. Chłopek, S. Góźdź, A. M. Nowicka and A. Kudelski, Biosens. Bioelectron., 2019, 132, 326–332 CrossRef CAS.
- K. A. Marx, T. Zhou, A. Montrone, D. Mcintosh and S. J. Braunhut, Anal. Biochem., 2007, 361, 77–92 CrossRef CAS.
- K. Noi, A. Iwata, F. Kato and H. Ogi, Anal. Chem., 2019, 91, 9398–9402 CrossRef CAS.
- V. Templier, A. Roux, Y. Roupioz and T. Livache, TrAC, Trends Anal. Chem., 2015, 79, 71–79 CrossRef.
- H. Q. Tan, C. C. Zhang, X. Qu and C. S. Liu, Polym. Bull., 2016, 5, 91–102 Search PubMed.
- M. C. Manning, D. K. Chou, B. M. Murphy, R. W. Payne and D. S. Katayama, Pharm. Res., 2010, 27, 544–575 CrossRef.
- G. Gupta, A. Surolia and S. G. Sampathkumar, Omics, 2010, 14, 419–436 CrossRef CAS.
- M. V. Mikaelyan, G. G. Poghosyan, O. D. Hendrickson, B. B. Dzantiev and V. K. Gasparyan, Anal. Chim. Acta, 2017, 981, 80–85 CrossRef CAS.
- X. Xu, Y. Yuan, G. Hu, X. Wang, P. Qi, Z. Wang, Q. Wang, X. Wang, Y. Fu, Y. Li and H. Yang, Sci. Rep., 2017, 7, 1452 CrossRef.
- I. J. Goldstein, R. C. Hughes, M. Monsigny, T. Osawa and N. Sharon, Nature, 1980, 285, 66 CrossRef.
- W. Y. Han, J. Vet. Univ., 1986, 4, 444–449 Search PubMed.
- X. Q. Zeng, C. A. S Andrade, M. D. L. Oliveira and X. L. Sun, Anal. Bioanal. Chem., 2012, 402, 3161–3176 CrossRef CAS.
- B. Shaanan, H. Lis and N. Sharon, ence, 1991, 254, 862–866 CrossRef CAS.
- X. L. Mao, Y. Luo, Z. P. Dai, K. Y. Wang and B. C. Lin, Anal. Chem., 2004, 76, 6941–6947 CrossRef CAS.
- M. D. Fernandez, F. J. Canada, J. Jimenez-Barbero and G. Cuevas, J. Am. Chem. Soc., 2005, 127, 7379–7386 CrossRef.
- C. P. Swaminathan, N. Surolia and A. Surolia, J. Am. Chem. Soc., 1998, 120, 5153–5159 CrossRef CAS.
- D. A. R. Sanders, D. N. Moothoo, J. Raftery, A. J. Howard, J. R. Helliwell and J. H. Naismith, J. Mol. Biol., 2001, 310, 875–884 CrossRef CAS.
- X. Dan, W. Liu and T. B. Ng, Med. Res. Rev., 2016, 36, 221–247 CrossRef CAS.
- I. Ofek and N. Sharon, Infect. Immun., 1988, 56, 539–547 CrossRef CAS.
- Y. X. Wang, Z. Z. Ye and Y. B. Ying, Sensors, 2012, 12, 3449–3471 CrossRef.
- O. Laczka, J.-M. Maesa, N. Godino, J. del Campo, M. Fougt-Hansen, J. P. Kutter, D. Snakenborg, F.-X. Muñoz-Pascual and E. Baldrich, Biosens. Bioelectron., 2011, 26, 3633–3640 CrossRef CAS.
- Y. K. Ye, W. W. Yan, Y. Q. Liu, S. D. He, X. D. Cao, X. Xu, H. S. Zheng and S. Gunasekaran, Anal. Chim. Acta, 2019, 1074, 80–88 CrossRef CAS.
- C. K. Joung, H. N. Kim, M. C. Lim, T. J. Jeon, H. Y. Kim and Y. R. Kim, Biosens. Bioelectron., 2013, 44, 210–215 CrossRef CAS.
- M. S. Cheng, J. S. Ho, S. H. Lau, V. T. K. Chow and C. S. Toh, Biosens. Bioelectron., 2013, 47, 340–344 CrossRef CAS.
- L. Lu, G. Chee, K. Yamada and S. Jun, Biosens. Bioelectron., 2012, 42C, 492–495 Search PubMed.
- A. Bonanni, M. Pumera and Y. Miyahara, Phys. Chem. Chem. Phys., 2011, 13, 4980–4986 RSC.
- H. Yang, Y. Wang, H. Qi, Q. Gao and C. Zhang, Biosens. Bioelectron., 2012, 35, 376–381 CrossRef CAS.
- G. Acharya, C. L. Chang and C. Savran, J. Am. Chem. Soc., 2006, 128, 3862–3863 CrossRef CAS.
- M. A. Seia, S. V. Pereira, C. A. Fontán, I. E. D. Vito, G. A. Messina and J. Raba, Sens. Actuators, B, 2012, 168, 297–302 CrossRef CAS.
- H. Kearns, R. Goodacre, L. E. Jamieson, D. Graham and K. Faulds, Anal. Chem., 2017, 89, 12666–12673 CrossRef CAS.
- G. Safina, M. V. Lier and B. Danielsson, Talanta, 2009, 77, 468–47214 CrossRef.
- J. E. Frew and H. A. Hill, Anal. Chem., 2010, 39, 1747–1763 Search PubMed.
- E. Cesewski and B. N. Johnson, Biosens. Bioelectron., 2020, 159, 112214 CrossRef CAS.
- F. Xi, J. Gao, J. Wang and Z. Wang, J. Electroanal. Chem., 2011, 656, 252–257 CrossRef CAS.
- H. Y. Lau, H. Q. Wu, E. J. H. Wee, M. Trau and Y. L. Wang, Sci. Rep., 2017, 7, 38896 CrossRef CAS.
-
C. M. Pandey and B. D. Malhotra, Nanomaterials for Biosensors, 2019 Search PubMed.
- A. Hushegyi and J. Tkac, Anal. Methods, 2014, 6, 6610 RSC.
- L. Klukova, T. Bertok, P. Kasak and J. Tkac, Anal. Methods, 2014, 6, 4922–4931 RSC.
- Z. Dai, A. N. Kawde, Y. Xiang, J. T. La Belle, J. Gerlach, V. P. Bhavanandan, L. Joshi and J. Wang, J. Am. Chem. Soc., 2006, 128, 10018–10019 CrossRef CAS.
- J. T. La Belle, J. Q. Gerlach, S. Svarovsky and L. Joshi, Anal. Chem., 2007, 79, 6959–6964 CrossRef CAS.
- E. Pale Ek, J. Tká, M. Barto Ík, T. Bertók, V. Ostatná and J. Pale Ek, Chem. Rev., 2015, 115, 2045–2108 CrossRef.
- M. Gamella, S. Campuzano, C. Parrado, A. J. Reviejo and J. M. Pingarrón, Talanta, 2009, 78, 1309 CrossRef.
- Y. Wan, D. Zhang and B. Hou, Talanta, 2009, 80, 218–223 CrossRef CAS.
- Z. Li, Y. Fu, W. Fang and Y. Li, Sensors, 2015, 15, 19212–19224 CrossRef CAS.
- M. D. Oliveira, C. A. Andrade, M. T. Correia, L. C. Coelho, P. R. Singh and X. Zeng, J. Colloid Interface Sci., 2011, 362, 194–201 CrossRef CAS.
- F. Ma, A. Rehman, M. Sims and X. Zeng, Anal. Chem., 2015, 87, 4385–4393 CrossRef CAS.
- J. S. L. Da Silva, M. D. L. Oliveira, C. P. de Melo and C. A. S. Andrade, Colloids Surf., B, 2014, 117, 549–554 CrossRef CAS.
- P. Qi, D. Zhang, Y. Wan and D. Lv, Talanta, 2014, 118, 333–338 CrossRef CAS.
- F. Li, Y. Feng, L. Yang, L. Li, C. Tang and B. Tang, Biosens. Bioelectron., 2011, 26, 2489–2494 CrossRef CAS.
- F. Ma, A. Rehman, H. Liu, J. Zhang, S. Zhu and X. Zeng, Anal. Chem., 2015, 87, 1560–1568 CrossRef CAS.
- D. Dechtrirat, N. Gajovic-Eichelmann, F. Wojcik, L. Hartmann, F. F. Bier and F. W. Scheller, Biosens. Bioelectron., 2014, 58, 1–8 CrossRef CAS.
- A. Mahalingam, A. R. Geonnotti, J. Balzarini and P. F. Kiser, Mol. Pharmaceutics, 2011, 8, 2465–2475 CrossRef CAS.
- S. N. Narla, P. Pinnamaneni, H. Nie, Y. Li and X. L. Sun, Biochem. Biophys. Res. Commun., 2014, 443, 562–567 CrossRef CAS.
- S. D. Bull, M. G. Davidson, J. M. H. Van den Elsen, J. S. Fossey, A. T. A. Jenkins, Y. B. Jiang, Y. Kubo, F. Marken, K. Sakurai and J. Zhao, Acc. Chem. Res., 2013, 46, 312–326 CrossRef CAS.
- R. Watahiki, K. Sato, K. Suwa, S. Niina, Y. Egawa, T. Seki and J. I. Anzai, J. Mater. Chem. B, 2014, 2, 5809 RSC.
- K. Sato, T. Mao, M. Ito, E. Abe and J. I. Anzai, Langmuir, 2014, 30, 9247–9250 CrossRef CAS.
- D. Dechtrirat, N. Gajovic-Eichelmann, F. Wojcik, L. Hartmann, F. F. Bier and F. W. Scheller, Biosens. Bioelectron., 2014, 58, 1–8 CrossRef CAS.
- P. Ertl and S. R. Mikkelsen, Anal. Chem., 2001, 73, 4241–4248 CrossRef CAS.
- J. Homola, M. Piliarik and P. Kvasnicka, Methods Mol. Biol., 2007, 276, 63 Search PubMed.
- K. M. Byun, J. Opt. Soc. Korea, 2010, 14, 65–76 CrossRef CAS.
- S. M. Yoo and Y. L. Sang, Trends Biotechnol., 2015, 34, 7–25 CrossRef.
- X. Xu, Y. Yuan, G. Hu, X. Wang, P. Qi, Z. Wang, Q. Wang, X. Wang, Y. Fu, Y. Li and H. Yang, Sci. Rep., 2017, 7, 1452 CrossRef.
- D. C. Hone, A. H. Haines and D. A. Russell, Langmuir, 2003, 19, 7141–7144 CrossRef CAS.
- F. Ma, A. Rehman, M. Sims and X. Zeng, Anal. Chem., 2015, 87, 4385–4393 CrossRef CAS.
- L. Zheng, Y. Wan, P. Qi, Y. Sun, D. Zhang and L. Yu, Talanta, 2017, 167, 600–606 CrossRef CAS.
- B. Liedberg, C. Nylander and I. Lundström, Biosens. Bioelectron., 1995, 10, i–ix CrossRef CAS.
- Y. Wang, Z. Ye, C. Si and Y. Ying, Food Chem., 2013, 136, 1303–1308 CrossRef CAS.
- S. Laing, K. Gracie and K. Faulds, Chem. Soc. Rev., 2016, 45, 1901–1918 RSC.
- Y. Wang, M. Salehi, M. Schuetz and S. Schluecker, Chem. Commun., 2014, 50, 2711–2714 RSC.
- R. M. Jarvis and R. Goodacre, Chem. Soc. Rev., 2008, 37, 931 RSC.
- D. I. Ellis, V. L. Brewster, W. B. Dunn, J. W. Allwood, A. P. Golovanov and R. Goodacre, Chem. Soc. Rev., 2012, 41, 5706–5727 RSC.
- S. Laing, K. Gracie and K. Faulds, Chem. Soc. Rev., 2016, 45, 1901–1918 RSC.
- Y. Wang, M. Salehi, M. Schuetz and S. Schluecker, Chem. Commun., 2014, 50, 2711–2714 RSC.
- S. W. Bishnoi, Y. J. Lin, M. Tibudan, Y. Huang, M. Nakaema, V. Swarup and T. A. Keiderling, Anal. Chem., 2011, 83, 4053–4060 CrossRef CAS.
- M. M. Harper, K. S. Mckeating and K. Faulds, Phys. Chem. Chem. Phys., 2013, 15, 5312–5328 RSC.
- W. Q. Wang, V. Hynninen, L. Qiu, A. W. Zhang, T. Lemma, N. N. Zhang, H. H. Ge, J. J. Toppari, V. P. Hytoenen and J. Wang, Sens. Actuators, B, 2017, 239, 515–525 CrossRef CAS.
- J. A. Dougan and K. Faulds, Analyst, 2012, 137, 545 RSC.
- L. Lan, Y. Yao, J. Ping and Y. Ying, Biosens. Bioelectron., 2017, 91, 504–514 CrossRef CAS.
- H. Li, Q. Chen, M. Mehedi Hassan, X. Chen, Q. Ouyang, Z. Guo and J. Zhao, Biosens. Bioelectron., 2017, 92, 192–199 CrossRef CAS.
- J. Simpson, D. Craig, K. Faulds and D. Graham, Nanoscale Horiz., 2015, 1, 60–63 RSC.
- S. P. Ravindranath, Y. Wang and J. Irudayaraj, Sens. Actuators, B, 2011, 152, 183–190 CrossRef CAS.
- K. E. Stephen, D. Homrighausen, G. Depalma, C. H. Nakatsu and J. Irudayaraj, Analyst, 2012, 137, 4280 RSC.
- W. R. Premasiri, D. T. Moir, M. S. Klempner, N. Krieger, G. Jones and L. D. Ziegler, J. Phys. Chem. B, 2005, 109, 312–320 CrossRef CAS.
- D. Craig, S. McAughtrie, J. Simpson, C. McCraw, K. Faulds and D. Graham, Anal. Chem., 2014, 86, 4775–4782 CrossRef CAS.
- A. Olszewska, E. Armstrong, M. Olszewski, M. Batenjany and A. Ostafin, J. Nanomed. Nanotechnol., 2016, 7, 1–5 Search PubMed.
- Z. Shen, M. Huang, C. Xiao, Y. Zhang, X. Zeng and P. G. Wang, Anal. Chem., 2007, 79, 2312–2319 CrossRef CAS.
- K. Lebed, A. J. Kulik, L. Forró and M. Lekka, J. Colloid Interface Sci., 2006, 299, 41–48 CrossRef CAS.
- Z. Shen, M. Huang, C. Xiao, Y. Zhang, X. Zeng and P. G. Wang, Anal. Chem., 2007, 79, 2312–2319 CrossRef CAS.
- B. Serra, M. Gamella, A. J. Reviejo and J. M. Pingarrón, Anal. Bioanal. Chem., 2008, 391, 1853–1860 CrossRef CAS.
- M. E. Yakovleva, A. P. Moran, G. R. Safina, T. Wadström and B. Danielsson, Anal. Chim. Acta, 2011, 694, 1–5 CrossRef CAS.
- G. Safina, M. Vanlier and B. Danielsson, Talanta, 2008, 77, 468–472 CrossRef CAS.
- Y. Wan, D. Zhang and B. Hou, Biosens. Bioelectron., 2010, 25, 1847–1850 CrossRef CAS.
-
B. D. Malhotra and M. A. Ali, Nanomaterials for Biosensors, 2018, pp. 263–293 Search PubMed.
- S. Kwakye and A. Baeumner, Anal. Bioanal. Chem., 2003, 376, 1062–1068 CrossRef CAS.
- T. N. T. Dao, J. Yoon, C. E. Jin, B. Koo, K. Han, Y. Shin and T. Y. Lee, Sens. Actuators, B, 2018, 262, 588–594 CrossRef CAS.
- H. Yang, H. Zhou, H. Hao, Q. Gong and N. Kai, Sens. Actuators, B, 2016, b229, 297–304 CrossRef.
|
This journal is © The Royal Society of Chemistry 2021 |