DOI:
10.1039/D0SC05638C
(Edge Article)
Chem. Sci., 2020,
11, 13143-13151
Atomistic fibrillar architectures of polar prion-inspired heptapeptides†
Received
12th October 2020
, Accepted 20th October 2020
First published on 2nd November 2020
Abstract
This article provides the computational prediction of the atomistic architectures resulting from self-assembly of the polar heptapeptide sequences NYNYNYN, SYSYSYS and GYGYGYG. Using a combination of molecular dynamics and a newly developed tool for non-covalent interaction analysis, we uncover the properties of a new class of bionanomaterials, including hydrogen-bonded polar zippers, and the relationship between peptide composition, fibril geometry and weak interaction networks. Our results, corroborated by experimental observations, provide the basis for the rational design of prion-inspired nanomaterials.
1 Introduction
The cross-β spine is a common motif of amyloid fibrils; it is composed of two sandwiched β-sheets, each resulting from the lateral stacking of peptide segments. At the contact region between the β-sheets, side chains form a tight, solvent-excluded interface called a steric zipper.1–3 This regular repeat of stacked strands arranged perpendicular to the fibril growth axis represents the structural basis of amyloid fibrils. Furthermore, this apparently simple organization contains the key to the polymorphism of amyloid fibrils, i.e. the ability of the same polypeptide sequence to yield a variety of different three-dimensional architectures.2,4 Indeed, this heterogeneity arises from a multiplicity of possible polypeptide arrangements and conformations, all lying in a narrow energy range. Also short peptides have the ability to self-assemble in various dispositions with distinct probabilities, giving rise to fibrils with different morphologies; these morphologies, in turn, can translate into different mechanical properties, highlighting the importance of having accurate knowledge of the atomistic structure for revealing the experimental results and designing new systems.5,6
Formation of fibrils with hydrophobic steric zippers as a consequence of protein misfolding and aggregation is a fundamental aspect of pathogenic amyloids involved in neurodegenerative diseases (e.g. amyloid-β in Alzheimer's disease and α-synuclein in Parkinson's disease).7,8
One of the key aspects of amyloids is the fact that they represent a thermodynamic well in the conformational landscape: when a protein attains an amyloid state, the aggregation process cannot be easily reversed due to a high enthalpic cost, and the aggregated state, corresponding to a specific polymorph, can recruit new monomers, providing the means for fibril propagation. The preference for a specific polymorph among all the possible supra-structures is fine-tuned by the local environment in such a way that two different Alzheimer's disease patients may show different amyloid-β polymorphs.9–11 Once a certain fibrillar length is attained, structural interconversion is no longer observed.12
A different behavior is observed in functional prions: yeast prions are capable of switching between a soluble and an amyloid state, where globular domains remain unmodified and self-assembly takes place at the level of the so-called prion domains (PrDs), intrinsically disordered sequences rich in polar residues such as asparagine and glutamine and deficient in hydrophobic amino acids. This reversibility, which sets these systems apart from classic amyloid fibrils, makes them excellent candidates for the design of nanomaterials that can be activated by aggregation while maintaining a specific function encoded in the untouched globular domain. To understand and develop the full potential of PrDs in nanotechnology, it is necessary to analyze, at the molecular level, the aggregation patterns of prion domains.13,14
On this point, the intrinsic disorder of amyloid and prion aggregates represents a big challenge for computational techniques,15–17 as they need to describe accurately both covalent bonding and the more elusive non-covalent interactions.18,19 In this work, we employ our recently developed non-covalent interaction integral technique, which allows fast estimation of non-covalent interaction strengths in biomolecular systems to address the relative stability of prion-inspired aggregates.20,21 In a recent study, we designed a series of minimalist prion-inspired polar heptapeptides that, despite their small size, recapitulate the properties of full-length PrDs, spontaneously self-assembling into highly ordered non-toxic amyloid fibrils under physiological conditions.22 Here, we address the atomistic structure of their cross-β spines.
2 Methodology
2.1 Computational methods
MD simulations were performed with the Amber suite, using the ff14SB force field.23,24 A slow equilibration protocol was set up to relax the initial fibrillar architecture to a temperature of 300 K. Since the initial inter-sheet distance is set to a large value to avoid steric clashes, equilibration involves short low temperature unrestrained dynamics bursts to allow the fibril model to pack side chains at the steric zipper (see the ESI†). Production was run as 100 ns NPT dynamics, at a constant temperature of 300 K and removing all restraints, with the Langevin thermostat and Monte Carlo barostat.25,26 A rectangular solvent box was built around each fibril model with TIP3P waters27 with a minimum 12 Å buffer between the solvent and the simulation box. As all fibril models are neutral, no ion was added. Calculation of the relative energy of fibril architectures was performed by extracting 100 snapshots from the 100 ns production trajectory, stripping water molecules and running a 500 step geometry relaxation on each frame using the generalized Born implicit solvent model to attenuate steric clashes.28,29 NCIPLOT analysis to obtain semiquantitative information on the relative stability of lateral and inter-sheet contacts was performed on the snapshots extracted from the molecular dynamics simulations as discussed in ref. 20, 21 and 30. This information is given in the form of non-covalent interaction (NCI) integrals, which have been shown to provide a semiquantitative measure of the interaction strength.30 NCI integrals are presented for the so-called van der Waals region, which covers most of the NCIs, and is comparatively weak and the so-called attractive region, which accounts for the contribution of the strongest NCIs, involving high electron densities (e.g. strong hydrogen bonds). These integrals provide for each frame a descriptor of the collective strength of intra-sheet and inter-sheet NCIs and can be used to compare the relative stabilization of different fibril models of the same system.
NCIs can be identified by analyzing the electron density, ρ(r), and its reduced density gradient (eqn (1))
| 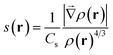 | (1) |
with
Cs = 2(3π
2)
1/3. Non-covalent interactions in molecular systems are regions characterized by low values of both
s(
r) and
ρ(
r) (they are critical points of
ρ(
r)), to distinguish them from bond critical points.
20 By calculating
ρ(
r) and
s(
r) on a three-dimensional grid centered on the molecule of interest, NCIs can be identified as the grid points in which
s(
r) and
ρ(
r) fall under a given threshold. Integration of the electron density in the NCI regions provides an estimation of the entity of non-covalent interactions in the given system. Moreover, the sign of the second eigenvalue of the Hessian of the electron density (
λ2) allows differentiation between van der Waals interactions, hydrogen bonds and steric clashes. In practice, by integrating the electron density in user-defined sign(
λ2)
ρ ranges, it is possible to separate the contribution to attractive non-covalent contacts into stronger ones (NCI
a) and weaker ones (NCI
vdw), which in our systems roughly correspond to hydrogen bonds and dispersion interactions, respectively. In this work, we computed NCI
a and NCI
vdw along the molecular dynamics trajectories of our fibril models using the computational protocol described in
ref. 21.
2.2 Experimental techniques
Preparation of fibrils for TEM visualization.
10 μL of mature isolated heptapeptide fibrils were deposited onto carbon-coated copper grids for 10 min, and the excess liquid was removed with a filter paper. 5 μL of a uranyl acetate (2% w/v) solution were applied to the grid to negatively stain the samples for 1 min and the excess was removed with a filter paper. Grids were allowed to dry on a bench to completely evaporate any residual water.
3 Results and discussion
3.1 Model generation
Initial geometry of the heptapeptide strand.
Using the NY7, SY7 and GY7 sequences, we built the fibrillar models corresponding to all possible steric zipper organizations and analyzed how the distribution of polar residues determines the preferred architecture. Since charges at the N- and C-terminus are expected to play an important role in determining the steric zipper architecture,31 we acetylated the N-terminus and amidated the C-terminus to ensure charge neutrality (see the ESI† for the shared initial geometry of the three heptapeptides). These modifications were also present in the synthetic peptides we used for experiments, since they mimic the sequence context within a PrD.
Generation of steric zipper structures.
The problem of determining the steric zipper architectures arising from a given amino acid sequence has been discussed in the classic article by Eisenberg and coworkers, which lists all the possible structures arising from self-organization.32 These architectures are classified according to: (i) intra-sheet organization (parallel or antiparallel), (ii) how side chains are packed at the interface (face-to-back, face-to-face or face = back) and (iii) the relative orientation of the β-sheets (up–up, up–down or up = down). For our polar heptapeptides, eleven non-equivalent architectures are possible, which are represented in Fig. 1. Six of these models have a parallel organization and five an antiparallel one. Each fibril model is composed of two sandwiched β-sheets defining an interface region (the steric zipper where side chains are packed in a solvent-excluded organization) and two external regions where side chains are exposed to the solvent. Since our heptapeptides present an alternation of residues on either side of the extended β-filament, each strand packs one type of residue at the interface and exposes the other to the solvent.
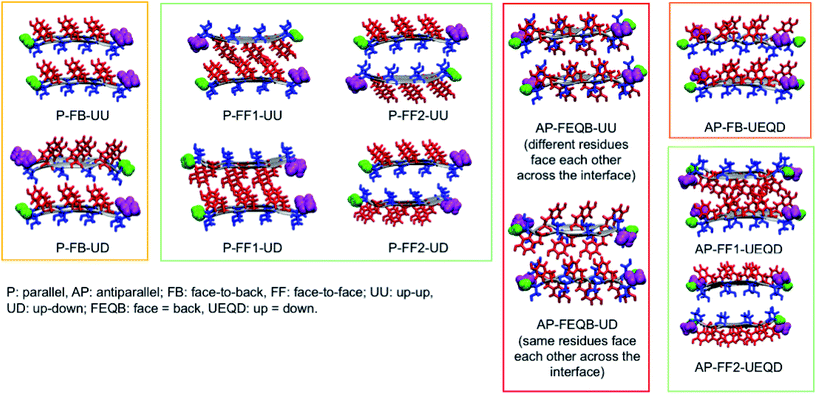 |
| Fig. 1 The eleven non-equivalent steric zipper architectures that can arise from the self-assembly of SY7. Tyrosines are represented in red and serines in blue. The corresponding architectures for NY7 and GY7 can be obtained by replacing serines with asparagines or glycines. | |
To generate all possible fibrillar architectures we used an in-house code that computes the Cartesian coordinates of steric zippers of any length applying rigid rotations and translations to the coordinates of an individual strand. The length of each β-sheet was set to 20 strands, so that each fibril model is composed of 40 strands. For each heptapeptide, we ran molecular dynamics simulations on the eleven non-equivalent possible fibrillar architectures and computed their relative energies on 100 frames sampled with a constant stride.
Of these models, we analyzed the relative stability, non-covalent interaction networks and geometrical features. As far as the latter are concerned, we analyzed the twist angle, which measures the rotation of subsequent strands around the fibril's main axis, and the tilt angle, which measures the linearity of the main axis. The formal definition of these angles is given in the ESI.†
3.2 Relative stabilities
Preferred architectures.
The relative energies of the eleven fibril models of NY7, SY7 and GY7 are shown in Table 1. Their temporal evolution is reported in the ESI.† For structure labels refer to Fig. 1. The most stable model of both NY7 and SY7 is P-FF2-UU, shown in Fig. 2a and b, respectively. These architectures are parallel, with tyrosines exposed to the solvent and asparagines (NY7) or serines (SY7) packed at the interface, forming a polar zipper. However, the most stable model of GY is AP-FF2-UEQD, an antiparallel architecture where tyrosines are exposed to the solvent and glycines are packed at the interface (Fig. 2c).
Table 1 Average relative energies and standard deviation (in parentheses) in kcal per mol per strand of the eleven possible steric zippers of NY7, SY7 and GY7. Energies are averaged over 100 frames extracted from the 100 ns MD trajectory. For structure labels refer to Fig. 1
Model |
NY7 (sd) |
SY7 (sd) |
GY7 (sd) |
AP-FEQB-UD |
10.50 (0.57) |
13.59 (0.60) |
9.23 (0.34) |
AP-FEQB-UU |
9.46 (0.56) |
12.40 (0.65) |
8.05 (0.47) |
AP-FB-UEQD |
8.31 (0.70) |
12.59 (0.74) |
13.40 (0.65) |
AP-FF1-UEQD |
11.96 (0.54) |
16.04 (0.39) |
17.97 (1.16) |
AP-FF2-UEQD |
9.67 (0.61) |
2.28 (0.43) |
0.00 (0.57) |
P-FB-UU |
3.14 (0.69) |
12.41 (0.50) |
12.37 (0.29) |
P-FB-UD |
2.00 (0.77) |
9.72 (0.49) |
15.02 (0.44) |
P-FF1-UD |
11.16 (1.48) |
19.52 (0.78) |
21.24 (1.24) |
P-FF1-UU |
8.23 (0.91) |
18.23 (0.71) |
22.28 (0.55) |
P-FF2-UD |
0.79 (0.33) |
0.87 (0.30) |
6.64 (0.74) |
P-FF2-UU |
0.00 (0.26) |
0.00 (0.57) |
9.73 (0.49) |
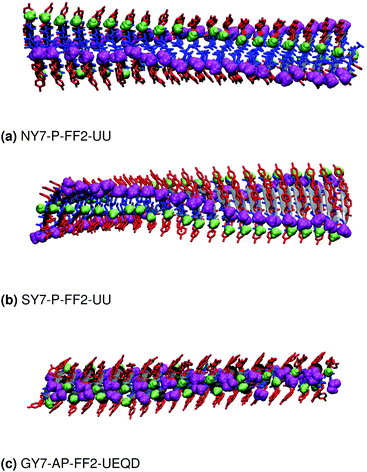 |
| Fig. 2 Structure of the most stable steric zipper of (a) NY7, (b) SY7 and (c) GY7 after 100 ns of MD. Tyrosine is represented in red and asparagine, serine and glycine in blue; the N-terminus is represented in magenta and the C-terminus in green. | |
These results indicate that despite the common features of the three heptapeptides (length, residue alternation and presence of three tyrosines), the composition affects the preferred steric zipper architecture. Also, they show that if an amino acid capable of forming strong hydrogen bonds such as serine, asparagine, or glycine is present alongside tyrosine residues, tyrosines will remain exposed to the solvent and the other residue will be packed at the interface. The evolution of the twist angles of NY7, SY7, and GY7 and along the MD trajectories is shown in Fig. S7–S9.† The results indicate a large variability both among different heptapeptides and among fibril models. In general, our models become flatter in the order GY7 < SY7 < NY7, with NY7 yielding the flattest fibrils of the set.
3.3 NY7 fibrils
The NY7 polar zipper.
The most favorable architecture of NY7 is the P-FF2-UU polar zipper, where asparagine side chains provide a strong network of both intra-sheet (Fig. 3a) and inter-sheet (Fig. 3b) hydrogen bonds, while tyrosine residues remain exposed to the solvent. Relative energies (Table 1) indicate that the related structure P-FF2-UD is essentially energetically equivalent (<1 kcal per mol per strand, Fig. 1). The difference between the two models lies in the relative orientation of the parallel β-sheets, which can be visualized considering the arrangement of the terminal groups: while in P-FF2-UU the C-termini of one sheet face the N-termini of the other, in P-FF2-UD terminal groups of the same type face each other across the interface (Fig. 1). The intersheet distance of P-FF2-UU and P-FF2-UD is ∼8 Å, which prevents direct inter-sheet contact between termini and explains the negligible energy difference between the two fibrils. Both models are comparably flat, with twist angles of 15–20° and tilt angles below 2°.
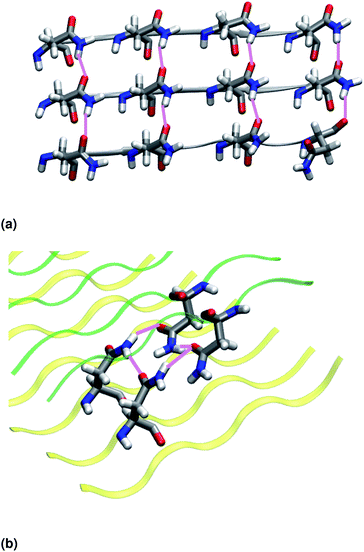 |
| Fig. 3 (a) Intra-sheet hydrogen bonds involving asparagine side chains. (b) Inter-sheet hydrogen bonds involving asparagine side chains (polar clasps). Upper and lower β-sheets are represented in green and yellow, respectively. | |
Hydrogen bonds stabilize the polar zipper.
On the opposite side of the spectrum we find the fibril model P-FF1-UD, which is unstable and disaggregates along the MD trajectory. This system has opposite residue exposure to P-FF2-UU and P-FF2-UD, packing tyrosine at the interface and leaving aspargines exposed to the solvent. This indicates that packing a residue capable of strong hydrogen bonding such as asparagine at the steric zipper is crucial for fibril stability. Interestingly, inter-sheet interactions of P-FF2-UU and P-FF2-UD through asparagine residues take the form of polar clasps (Fig. 3b), which have been recently detected in a prion protofibril.33
Parallel fibrils optimize the hydrogen bond network.
In antiparallel organizations, asparagine residues are no longer perfectly aligned along the fibril growth axis (Fig. S2†). Analysis of the intra-sheet hydrogen bond patterns and NCI integrals, shown in Table S1,† indicates that parallel fibrils have a larger number of stable asparagine side chain hydrogen bonds than antiparallel ones, resulting in a stronger stabilization. Inter-sheet stable hydrogen bonds and NCI integrals are presented in Table 2 and indicate that on average, parallel fibrils also have more favorable inter-sheet contacts than antiparallel ones. Overall, both intra- and inter-sheet side chain contacts are favored in parallel organizations over antiparallel ones. As far as backbone hydrogen bonds are concerned, the trend is opposite (Table S1†), with stronger backbone intra-sheet contacts in antiparallel fibrils over parallel ones, as expected.34 This effect, however, is not strong enough to shift structural preference to an antiparallel organization. This can be appreciated looking at the values of van der Waals and attractive NCI integrals of intra-sheet contacts (Table S1†), which are larger for parallel architectures.
Table 2 Properties of the eleven fibril models for NY7, SY7 and GY7. i-BB-SC: inter-sheet backbone-side chain H bonds; i-SC HB: inter-sheet (i) side chain H bonds. Criterion for hydrogen bond counting: presence in more than 50% of the trajectory. Information: number of contacts and average distance in Å. Twist angle and tilt angle in degrees, see the ESI for angle definitions. i-NCIvdw/NCIa: inter-sheet (i) van der Waals (vdw) and attractive (a) integrals. The most stable structure(s) are shown in bold, while those in italics do not maintain the steric zipper organization along the molecular dynamics trajectory and are excluded from further analysis
Name |
i-BB-SC |
Avgd |
i-SC HB |
Avgd |
i-NCIvdw |
i-NCIa |
Twist |
Tilt |
NY7-AP-FEQB-UD |
0 |
— |
17 |
2.74 |
56.08 |
7.67 |
161.82 |
4.75 |
NY7-AP-FEQB-UU |
0 |
— |
4 |
2.79 |
59.03 |
6.99 |
161.91 |
3.08 |
NY7-AP-FB-UEQD |
0 |
— |
25 |
2.74 |
63.12 |
11.41 |
164.25 |
4.31 |
NY7-AP-FF1-UEQD |
0 |
— |
13 |
2.83 |
57.70 |
7.33 |
166.12 |
1.70 |
NY7-AP-FF2-UEQD |
5 |
2.84 |
36 |
2.84 |
69.32 |
14.91 |
159.06 |
2.18 |
NY7-P-FB-UU |
4 |
2.79 |
20 |
2.73 |
59.89 |
9.95 |
16.63 |
1.39 |
NY7-P-FB-UD |
0 |
— |
23 |
2.85 |
64.33 |
7.90 |
10.11 |
5.79 |
NY7-P-FF1-UD
|
— |
— |
— |
— |
— |
— |
— |
— |
NY7-P-FF1-UU |
0 |
— |
72 |
2.81 |
84.18 |
12.29 |
32.46 |
1.63 |
NY7-P-FF2-UD
|
0
|
— |
59
|
2.86
|
64.45
|
16.35
|
20.56
|
1.27
|
NY7-P-FF2-UU
|
0
|
— |
31
|
2.87
|
83.25
|
17.54
|
15.94
|
1.16
|
SY7-AP-FEQB-UD |
3 |
2.77 |
20 |
2.80 |
72.31 |
9.11 |
162.87 |
4.75 |
SY7-AP-FEQB-UU |
0 |
— |
3 |
2.81 |
56.03 |
4.68 |
109.84 |
6.62 |
SY7-AP-FB-UEQD |
8 |
2.77 |
25 |
2.80 |
76.47 |
11.79 |
166.20 |
6.99 |
SY7-AP-FF1-UEQD |
0 |
— |
12 |
2.83 |
57.84 |
7.72 |
171.13 |
1.78 |
SY7-AP-FF2-UEQD |
19 |
2.72 |
13 |
2.79 |
109.10 |
16.15 |
172.43 |
2.51 |
SY7-P-FB-UU |
36 |
2.77 |
30 |
2.77 |
93.69 |
17.16 |
12.71 |
8.15 |
SY7-P-FB-UD |
0 |
— |
74 |
2.75 |
81.36 |
16.70 |
11.63 |
2.54 |
SY7-P-FF1-UD
|
— |
— |
— |
— |
— |
— |
— |
— |
SY7-P-FF1-UU |
0 |
— |
71 |
2.81 |
83.13 |
12.79 |
24.19 |
1.51 |
SY7-P-FF2-UD
|
121
|
2.70
|
17
|
2.77
|
134.89
|
21.57
|
63.66
|
4.27
|
SY7-P-FF2-UU
|
38
|
2.76
|
69
|
2.72
|
114.81
|
21.09
|
37.80
|
1.94
|
GY7-AP-FEQB-UD |
26 |
2.77 |
0 |
— |
90.04 |
10.50 |
131.59 |
4.65 |
GY7-AP-FEQB-UU |
16 |
2.78 |
0 |
— |
99.80 |
10.81 |
169.98 |
6.02 |
GY7-AP-FB-UEQD |
22 |
2.76 |
0 |
— |
78.82 |
8.58 |
128.48 |
7.49 |
GY7-AP-FF1-UEQD
|
— |
— |
— |
— |
— |
— |
— |
— |
GY7-AP-FF2-UEQD
|
0
|
— |
0
|
— |
104.69
|
7.65
|
169.40
|
1.97
|
GY7-P-FB-UU |
57 |
2.72 |
0 |
— |
82.45 |
10.59 |
19.41 |
4.57 |
GY7-P-FB-UD |
7 |
2.75 |
0 |
— |
76.92 |
8.61 |
54.12 |
4.86 |
GY7-P-FF1-UD
|
— |
— |
— |
— |
— |
— |
— |
— |
GY7-P-FF1-UU
|
— |
— |
— |
— |
— |
— |
— |
— |
GY7-P-FF2-UD |
0 |
— |
0 |
— |
102.37 |
5.80 |
31.33 |
5.99 |
GY7-P-FF2-UU |
0 |
— |
0 |
— |
90.84 |
5.51 |
60.35 |
3.87 |
3.4 SY7 fibrils
Backbone involvement in the SY7 polar zipper.
Similar to NY7, the most favorable architecture of SY7 is P-FF2-UU, with the related P-FF2-UD model at less than 1 kcal per mol per strand.
Similar to NY7, the P-FF1-UD model that packs tyrosines at the interface is unstable and disaggregates along the MD trajectory. In the preferred models, P-FF2-UU and P-FF2-UD, tyrosine residues remain exposed to the solvent, and serine residues are packed at the polar zipper, forming a strong network of intra-sheet (Fig. 4a) and inter-sheet (Fig. 4b and c) hydrogen bonds, as already observed for NY7. However, there is a significant difference between NY7 and SY7, which originates from the distinct steric hindrance of asparagine and serine: while inter-sheet hydrogen bonds in NY7-P-FF2-UU and NY7-P-FF2-UD exclusively involve asparagine side chains, in SY7 models they can involve not only serine side chains (Fig. 4b), but also backbone carbonyl groups (Fig. 4c). These contacts (named i-BB-SC in Table 2) are established across the steric zipper involving on one side the hydroxyl group of a serine side chain and on the other a backbone carbonyl group.
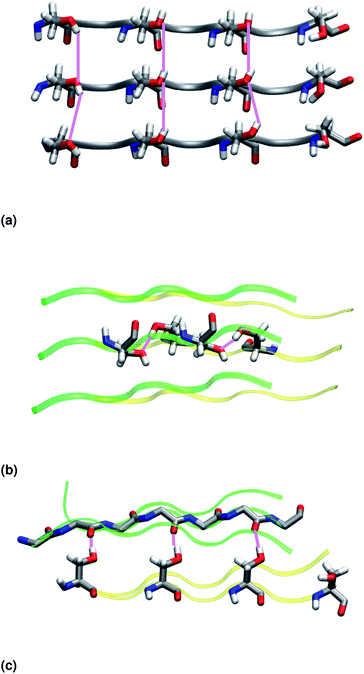 |
| Fig. 4 (a) Intra-sheet hydrogen bonds involving serine side chains. (b) Inter-sheet hydrogen bonds involving serine side chains. (c) Inter-sheet hydrogen bonds involving serine side chains and backbone carbonyl groups. Upper and lower β-sheets are represented in green and yellow, respectively. | |
Backbone involvement increases the twist angle.
The number of side chain-backbone inter-sheet hydrogen bonds is shown in Table 2, and while it is low for all NY7 fibril models, it grows significantly for SY7-P-FF2-UU and SY7-P-FF2-UD. Analyzing the inter-sheet hydrogen bond distribution (Table 2), we observed 38 BB-SC i hydrogen bonds for SY7-P-FF2-UU present in more than 50% of the trajectory and 69 involving serine side chains only. For SY7-P-FF2-UD, the relative abundance of these two contact types is inverted, with 121 BB-SC i hydrogen bonds and 17 involving side chains only. Although the total number of across-sheet hydrogen bonds remains roughly the same (107 vs. 138), as do the relative energy and NCI integrals, this different distribution strongly affects the fibril twist angle. A larger involvement of backbone groups in inter-sheet interactions enhances the twist angle, with the value for SY7-P-FF2-UD approximately double that for SY7-P-FF2-UU. We believe that direct involvement of the backbone in inter-sheet stabilizing interactions is made possible by the short inter-sheet distance (∼6 Å) of SY7, which is a consequence of the small size of serine.
Non-covalent interaction flexibility enhances stabilization.
The ability of serine side chains to form hydrogen bonds with both other serine side chains and backbone carbonyl groups across the steric zipper confers on SY7 fibrils a larger structural flexibility over NY7 ones; the latter can only form stable hydrogen bonds with other asparagine side chains. SY7 and NY7 parallel face–face architectures with the polar residues at the interface show comparable intra-sheet NCI integrals, but larger inter-sheet NCI integrals for SY7 over NY7. This points to a superior stability of serine-based fibrils over asparagine-based ones and has been confirmed experimentally by means of electron microscopy (see section Comparison with experiment).
Furthermore, we find it interesting that the energetically equivalent SY7-P-FF2-UU and SY7-P-FF2-UD steric zippers present such different twist angles. While the mechanical properties of the nano-fibrils cannot be extrapolated from our simulations on the bilayer – they depend on the overall morphology of the assembly of several bilayer units – we expect SY7-P-FF2-UU and SY7-P-FF2-UD to coexist in the nanofibril yielding a flexible morphology35 modulated by the extent of inter-sheet side chain-backbone hydrogen bonds. Similar considerations can be drawn for NY7, where the P-FF2-UU and P-FF2-UD arrangements are essentially energetically equivalent but less marked differences are observed between the two organizations.
3.5 GY7 fibrils
The GY7 steric zipper.
The most favorable architecture of GY7 is the AP-FF2-UEQD steric zipper, where glycine residues are packed at the interface and tyrosines remain exposed to the solvent (Fig. 5). As the side chains of glycines are hydrogen atoms, their packing yields an extremely short inter-sheet distance (∼4 Å). All models that pack tyrosine at the interface and leave glycines exposed to the solvent, P-FF1-UD, P-FF1-UU and AP-FF1-UEQD, are not stable and disaggregate along the MD trajectory.
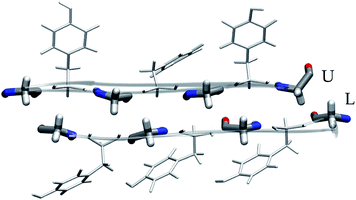 |
| Fig. 5 Residue packing at the glycine steric zipper. | |
An antiparallel organization maximizes intra- and inter-sheet contacts.
While residue organization at the zipper is the same as in NY7 and SY7, GY7 prefers an antiparallel organization over the parallel one of NY and SY. This can be explained in terms of the lack of lateral stabilizing interactions that can be formed by glycine side chains. As shown in Table S1,† for GY7 there is no significant difference in terms of side chain contacts between parallel and antiparallel architectures, while both SY7 and NY7 form more side chain hydrogen bonds in parallel organizations. When intra-sheet side chain interactions fail, β-sheet organization is dominated by backbone contacts, which are the strongest and most abundant in the model AP-FF2-UEQD. Looking now at inter-sheet NCIs, shown in Table 2, we observe that AP-FF2-UEQD, despite the absence of inter-sheet hydrogen bonds, still provides the best packing, with the largest value of the van der Waals NCI integral of all models. These results indicate that AP-FF2-UEQD is the most favorable fibril model for GY as it maximized both intra- and inter-sheet contacts.
3.6 Comparison with experiment
The design, synthesis and characterization of NY7, SY7 and GY7 fibrils were published in ref. 22. In this section we provide new TEM images of the three types of fibrils and reassess their interpretation in light of the computational results. TEM analysis reveals the presence of fibrils for NY7, SY7 and GY7 peptides (Fig. 6a–c). NY7 and SY7 fibrils differ significantly in length, with SY7 fibrils being longer. This fact might correspond to the higher structural flexibility of SY7 fibrils, as calculated, which would decrease their brittleness, relative to the likely more rigid NY7 fibrils. GY7 (Fig. 6c) fibrils appear thinner and less electrodense than those of NY7 and SY7 peptides, which will be consistent with a shorter inter β-sheet distance in GY7 fibrils, once they are assembled.
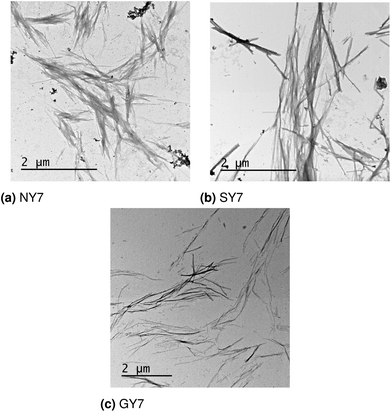 |
| Fig. 6 Electron microscopy images of (a) NY7, (b) SY7 and (c) GY7 fibrils.22 | |
3.7 Validation
NY7, SY7 and GY7 fibrils present unusually tight steric zippers.
The most striking feature of the most favorable architectures detected in NY7, SY7 and GY7 fibrils is the short inter-sheet distance compared with classic steric zippers formed by hydrophobic residues, where the distance is around 10 Å. Our attempts at aligning these fibrils for diffraction to confirm our findings have been unsuccessful so far. Due to the lack of other options, we resorted to computational validation by searching the literature for similar systems whose structure has been resolved, to assess whether our molecular dynamics protocol yields faithful steric zipper geometries.
Validation set.
Our validation set is composed of three steric zippers: a segment of the bank vole prion protein (residues 168–176, sequence QYNNQNNFV, PDB 6BTK33), a segment from the human immunoglobulin light-chain variable domain (residues 96–100, sequence YTFGQ, PDB 6DIY36) and a segment from the human prion protein (residues 113–118, sequence AGAAAA, PDB 6PQ5 (ref. 37)). The first system was chosen because it presents the same polar clasps that we observed in our simulation of NY7; the second because it packs threonine residues at the steric zipper, which resemble serines in size, and the third because it packs a small hydrophobic residue (alanine) at the interface. The intersheet distances of the three fibrils are ∼10, 8 and 5 Å, respectively, spanning from the classic amyloid distance of 6BTK to a shorter distance for 6DIY to the extraordinarily short one of 6PQ5. Thus, the intersheet distance decreases with the decreasing size of residues at the interface, the lowest being ∼5 Å for 6PQ5 where the steric zipper is composed of alanine residues. As glycine is even less bulky than alanine, we find our calculated results on GY7 to fit in this trend.
Calculated inter-sheet distances match the crystallographic values.
Starting from the crystallographic structure of the three systems, we extracted a strand and generated the corresponding 40-strand steric zippers matching the experimental organization with the same procedure employed for NY7, SY7 and GY7. For 6BTK, we left the terminals uncapped to match the experimental conditions, while for the other two, where the protein chain extends further than the resolved region, we employed the same neutral capping groups used for NY7, SY7 and GY7. Then, we ran molecular dynamics simulations with the same setup to assess whether our simulation protocol preserves the experimental architecture. Fibril geometries after 100 ns are shown in the ESI† and do not differ significantly from the experimental structures, confirming the quality of our simulation protocol. With the aim of quantitatively comparing the geometrical features of the validation set and our steric zippers, we computed the average inter-sheet distance along the molecular dynamics simulations of all systems and compared it with the available crystallographic information. Computing a single inter-sheet distance value representative of a MD simulation of a fibril is no trivial task owing to its pronounced flexibility. To guarantee a robust result, we resorted to the following protocol operating on 100 snapshots of samples with an even stride from the MD trajectory: for each frame, we iterated over each α-carbon (CA) of one β-sheet searching for the closest CA located on the opposite β-sheet. In this way, for each frame we obtained a complete set of pairwise distances between the two sheets, from which we extracted the minimum and maximum values and a mean. The distribution of these three values (minimum, maximum and mean) along the MD trajectories is represented in Fig. 7. These plots show that while the maximum and minimum values are distributed over a ∼1 Å range owing to structural flexibility, mean inter-sheet distances appear as sharp peaks, providing a robust descriptor of inter-sheet distances (see the ESI† for further details).
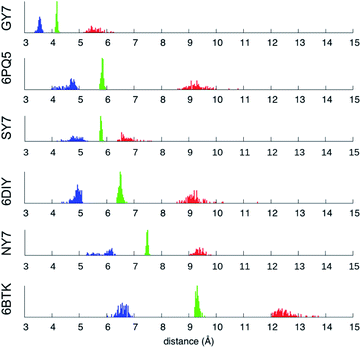 |
| Fig. 7 Minimum (blue), mean (green) and maximum (red) inter-sheet distance distribution along the MD trajectories of NY7-P-FF2-UU, SY7-P-FF2-UU, GY7-AP-FF2-UEQD and the validation set. | |
Averaging over the 100 frames yields a single value for each MD trajectory, presented in Table 3 and compared with the crystallographic distance for the validation set. To ensure comparability between calculated and crystallographic data, crystallographic inter-sheet distances were computed with the same protocol operating on the biological assembly as downloaded from the Protein Data Bank. The results shown in Table 3 show an excellent agreement between the calculated and crystallographic data for the validation set, confirming the quality of our MD protocol. Noticeably, GY7's inter-sheet distance is comparable with that of a prion structure. Indeed, the recently solved partial prion sequence of PDB 6PQ5 (ref. 37) shows an average inter-sheet distance of 5.8 Å, corresponding to a steric zipper of alanine residues. The predicted average inter-sheet distance of GY7-AP-FF2-UEQD is 4.2 Å, less than 2 Å shorter than that of 6PQ5, which is consistent with the smaller size of glycine over alanine side chains. Thus, steric zippers with a short inter-sheet distance exist, but resolution of their structure has started to become possible only in the last few years due to methodological advances (cryo-EM).38
Table 3 Minimum, mean and maximum inter-sheet distances (Å) averaged over the MD trajectories of the most stable fibrils of GY7, SY7 and NY7 and the validation set; validation set crystallographic inter-sheet distances (Å)
System |
Min. |
Mean |
Max. |
Crystallographic |
GY7-AP-FF2-UEQD |
3.5 |
4.2 |
5.6 |
— |
6PQ5
|
4.6 |
5.8 |
9.3 |
6.0 |
SY7-P-FF2-UU |
4.9 |
5.8 |
6.7 |
— |
6DIY
|
4.9 |
6.5 |
9.2 |
6.5 |
NY7-P-FF2-UU |
5.9 |
7.5 |
9.4 |
— |
6BTK
|
6.6 |
9.3 |
12.5 |
9.1 |
4 Conclusions
Using the newly developed non-covalent interaction integral method in combination with classical molecular dynamics, we have identified the most favorable polar zipper architectures resulting from the self-assembly of three heptapeptides containing tyrosine and (i) asparagine, (ii) serine and (iii) glycine. We have shown that polar residues (asparagine and serine) are packed at the interface between β-sheets, yielding a strong network of hydrogen bonds and stabilizing the resulting architectures over those that pack tyrosine at the interface. Furthermore, while asparagine polar zippers are stabilized by hydrogen bonds involving side chains only, serine polar zippers can form hydrogen bonds between backbone and side chain groups on opposite β-sheets. In general, fibril stability correlates with large intra- and inter-sheet van der Waals integrals, and for SY7 with the number of stable inter-sheet side chain–side chain and side chain–backbone hydrogen bonds. In SY7, larger twist angles correlate with a higher side chain–backbone/side chain–side chain ratio of stable inter-sheet hydrogen bonds. We have also shown that glycines, known as secondary structure breakers, can form a tight steric zipper (∼4 Å), yielding stable nanofibrils. Overall, we have demonstrated that computational techniques can predict the atomistic structure of novel prion-like assemblies with the peptide sequence as the only initial input. These predicted structures match experimental information even in highly atypical cases: we predict the first glycine steric zipper, which, like the recently resolved alanine zipper,37 is unthinkable within the framework of classic amyloid hydrophobic zippers. In this way, we show that our approach, which does not rely on structural databases, is able to tackle such new classes of non-classical steric zippers. We believe that these results will encourage the application of computational techniques, and in particular non-covalent interaction analysis, to interpret experimental results and provide the necessary physico-chemical insight to aid the rational design of related nanomaterials.
Conflicts of interest
There are no conflicts to declare.
Acknowledgements
FP acknowledges the financial support from CALSIMLAB under the public grant ANR-11-LABX-0037-01 overseen by the French National Research Agency (ANR) as part of the Investissements d'Avenir program (reference: ANR-11-IDEX-0004-02). MS and LRS gratefully acknowledge the financial support from the MINECO (CTQ2017-89132-P) and the Generalitat de Catalunya (2017SGR1323). SV gratefully acknowledges the financial support from the MINECO (BIO2016-78310-R) and ICREA (ICREA-Academia 2015 to S. V.). MDC gratefully acknowledges the financial support from the Spanish Ministry of Science and Innovation via a doctoral grant (FPU14/05786). SV, MDC and SN gratefully acknowledge the Servei de Microscopia from the Universitat Autònoma de Barcelona for their technical support.
Notes and references
- R. Nelson, M. R. Sawaya, M. Balbirnie, A. O. Madsen, C. Riekel, R. Grothe and D. Eisenberg, Nature, 2005, 435, 773–778 CrossRef CAS.
- J.-P. Colletier, A. Laganowsky, M. Landau, M. Zhao, A. B. Soriaga, L. Goldschmidt, D. Flot, D. Cascio, M. R. Sawaya and D. Eisenberg, Proc. Natl. Acad. Sci. U. S. A., 2011, 108, 16938–16943 CrossRef CAS.
- D. S. Eisenberg and M. R. Sawaya, Annu. Rev. Biochem., 2017, 86, 69–95 CrossRef CAS.
- M. Fändrich, J. Meinhardt and N. Grigorieff, Prion, 2009, 3, 89–93 CrossRef.
- Y. Raz, B. Rubinov, M. Matmor, H. Rapaport, G. Ashkenasy and Y. Miller, Chem. Commun., 2013, 49, 6561–6563 RSC.
- Y. Miller, B. Ma and R. Nussinov, J. Phys. Chem. B, 2015, 119, 482–490 CrossRef CAS.
- E. M. Rocha, B. D. Miranda and L. H. Sanders, Neurobiol. Dis., 2018, 109, 249–257 CrossRef CAS.
- M. Castello and S. Soriano, Ageing Res. Rev., 2014, 13, 10–12 CrossRef CAS.
- A. T. Petkova, W.-M. Yau and R. Tycko, Biochemistry, 2006, 45, 498–512 CrossRef CAS.
- A. K. Paravastu, R. D. Leapman, W.-M. Yau and R. Tycko, Proc. Natl. Acad. Sci. U. S. A., 2008, 105, 18349–18354 CrossRef CAS.
- J.-X. Lu, W. Qiang, W.-M. Yau, C. D. Schwieters, S. C. Meredith and R. Tycko, Cell, 2013, 154, 1257–1268 CrossRef CAS.
- R. Tycko, Protein Sci., 2014, 23, 1528–1539 CrossRef CAS.
- W. Wang, S. Navarro, R. A. Azizyan, M. Baño Polo, S. A. Esperante, A. V. Kajava and S. Ventura, Nanoscale, 2019, 11, 12680–12694 RSC.
- X.-M. Zhou, A. Entwistle, H. Zhang, A. P. Jackson, T. O. Mason, U. Shimanovich, T. P. J. Knowles, A. T. Smith, E. B. Sawyer and S. Perrett, ChemCatChem, 2014, 6, 1961–1968 CrossRef CAS.
- I. M. Ilie and A. Caflisch, Chem. Rev., 2019, 119, 6956–6993 CrossRef CAS.
-
F. Musiani and A. Giorgetti, Early Stage Protein Misfolding and Amyloid Aggregation, Academic Press, 2017, vol. 329, pp. 49–77 Search PubMed.
-
N. V. Dovidchenko and O. V. Galzitskaya, in Computational Approaches to Identification of Aggregation Sites and the Mechanism of Amyloid Growth, ed. O. Gursky, Springer International Publishing, Cham, 2015, pp. 213–239 Search PubMed.
- J. Černý and P. Hobza, Phys. Chem. Chem. Phys., 2007, 9, 5291–5303 RSC.
- K. E. Riley and P. Hobza, Wiley Interdiscip. Rev.: Comput. Mol. Sci., 2011, 1, 3–17 CAS.
- R. A. Boto, F. Peccati, R. Laplaza, C. Quan, A. Carbone, J.-P. Piquemal, Y. Maday and J. Contreras-García, J. Chem. Theory Comput., 2020, 16, 4150–4158 CrossRef CAS.
- F. Peccati, J. Chem. Inf. Model., 2020, 60, 6–10 CrossRef CAS.
- M. Díaz-Caballero, S. Navarro, I. Fuentes, F. Teixidor and S. Ventura, ACS Nano, 2018, 12, 5394–5407 CrossRef.
-
D. A. Case, R. M. Betz, W. Botello-Smith, D. S. Cerutti, T. E. Cheatham, T. Darden, R. E. Duke, T. J. Giese, H. Gohlke and A. W. Goetz, et al., AMBER16, 2016 Search PubMed.
- J. A. Maier, C. Martinez, K. Kasavajhala, L. Wickstrom, K. E. Hauser and C. Simmerling, J. Chem. Theory Comput., 2015, 11, 3696–3713 CrossRef CAS.
- R. J. Loncharich, B. R. Brooks and R. W. Pastor, Biopolymers, 1992, 32, 523–535 CrossRef CAS.
- J. Åqvist, P. Wennerström, M. Nervall, S. Bjelic and B. O. Brandsdal, Chem. Phys. Lett., 2004, 384, 288–294 CrossRef.
- W. L. Jorgensen, J. Chandrasekhar, J. D. Madura, R. W. Impey and M. L. Klein, J. Chem. Phys., 1983, 79, 926–935 CrossRef CAS.
- A. Onufriev, D. Bashford and D. A. Case, Proteins: Struct., Funct., Bioinf., 2004, 55, 383–394 CrossRef CAS.
- A. Onufriev, D. Bashford and D. A. Case, J. Phys. Chem. B, 2000, 104, 3712–3720 CrossRef CAS.
- F. Peccati, E. Desmedt and J. Contreras-García, Comput. Theor. Chem., 2019, 1159, 23–26 CrossRef CAS.
- X. Periole, T. Huber, A. Bonito-Oliva, K. C. Aberg, P. C. A. van der Wel, T. P. Sakmar and S. J. Marrink, J. Phys. Chem. B, 2018, 122, 1081–1091 CrossRef CAS.
- M. R. Sawaya, S. Sambashivan, R. Nelson, M. I. Ivanova, S. A. Sievers, M. I. Apostol, M. J. Thompson, M. Balbirnie, J. J. W. Wiltzius, H. T. McFarlane, A. O. Madsen, C. Riekel and D. Eisenberg, Nature, 2007, 447, 453–457 CrossRef CAS.
- M. Gallagher-Jones, C. Glynn, D. R. Boyer, M. W. Martynowycz, E. Hernandez, J. Miao, C.-T. Zee, I. V. Novikova, L. Goldschmidt, H. T. McFarlane, G. F. Helguera, J. E. Evans, M. R. Sawaya, D. Cascio, D. S. Eisenberg, T. Gonen and J. A. Rodriguez, Nat. Struct. Mol. Biol., 2018, 25, 131–134 CrossRef CAS.
-
Y. Maréchal, The Hydrogen Bond and the Water Molecule, Elsevier, 2007, pp. 25–47 Search PubMed.
- R. Song, X. Wu, B. Xue, Y. Yang, W. Huang, G. Zeng, J. Wang, W. Li, Y. Cao, W. Wang, J. Lu and H. Dong, J. Am. Chem. Soc., 2019, 141, 223–231 CrossRef CAS.
- B. Brumshtein, S. R. Esswein, M. R. Sawaya, G. Rosenberg, A. T. Ly, M. Landau and D. S. Eisenberg, J. Biol. Chem., 2018, 293, 19659–19671 CrossRef CAS.
- C. Glynn, M. R. Sawaya, P. Ge, M. Gallagher-Jones, C. W. Short, R. Bowman, M. Apostol, Z. H. Zhou, D. S. Eisenberg and J. A. Rodriguez, Nat. Struct. Mol. Biol., 2020, 27, 417–423 CrossRef CAS.
- E. Nwanochie and V. Uversky, Int. J. Mol. Sci., 2019, 20, 4186 CrossRef.
Footnote |
† Electronic supplementary information (ESI) available: Structural analysis of NY7, SY7 and GY7 fibrils. See DOI: 10.1039/d0sc05638c |
|
This journal is © The Royal Society of Chemistry 2020 |
Click here to see how this site uses Cookies. View our privacy policy here.