DOI:
10.1039/C9SC03711J
(Edge Article)
Chem. Sci., 2020,
11, 525-533
Serum Raman spectroscopy as a diagnostic tool in patients with Huntington's disease†
Received
27th July 2019
, Accepted 14th November 2019
First published on 14th November 2019
Abstract
Huntington's disease (HD) is an autosomal dominant neurodegenerative disorder caused by an abnormal CAG expansion in exon 1 of the huntingtin (HTT) gene. Given its genetic basis it is possible to study patients both in the pre-manifest and manifest stages of the condition. While disease onset can be modelled using CAG repeat size, there are no easily accessible biomarkers that can objectively track disease progression. Here, we employed a holistic approach using spectral profiles generated using both surface-enhanced Raman spectroscopy (SERS) and Raman Spectroscopy (RS), on the serum of healthy participants and HD patients covering a wide spectrum of disease stages. We found that there was both genotype- and gender-specific segregation on using the full range in the fingerprint region with both SERS and RS. On a more detailed interrogation using specific spectral intervals, SERS revealed significant correlations with disease progression, in particular progression from pre-manifest through to advanced HD was associated with serum molecules related to protein misfolding and nucleotide catabolism. Thus, this study shows the potential of Raman spectroscopy-based techniques for stratification of patients and, of SERS, in particular, to track disease status through provision of ‘spectral’ biomarkers in HD, with clinical applications for other diseases and trials looking at disease modifying therapies.
Introduction
Huntington's disease (HD) is an inherited autosomal dominant disorder caused by an expanded CAG gain-of-function mutation in the HTT gene. HD typically affects people in mid-life and leads to progressive motor, cognitive, and affective problems, along with weight loss and circadian rhythm disturbances. HD is currently incurable and normally runs a clinical course over 20 years in most patients. While a genetic test exists to ascertain whether patients carry the mutation, there are currently no accurate ways to mark disease onset. At the present time, diagnosis is based on the presence of motor signs in the right genetic context, although moves are afoot to try and refine this given that a number of non-motor problems can be seen ahead of any motor abnormalities in many HD patients1–3 and animal models.4 Such cognitive and psychiatric impairments are associated with structural3 and functional connectivity changes using MRI.5,6 While these measures are starting to be used in clinical trials, the cost and inconvenience associated with such imaging is not inconsequential, especially as with time chorea (which consists of dance-like involuntary movement) develops in most patients that can degrade the quality of the scans so obtained. Thus, alternative simpler disease biomarkers have been explored in HD, including those using blood. Venepuncture is a simple procedure but previous attempts looking for specific serum molecules to track HD have proven unsuccessful. For instance, the level of brain-derived neurotrophic factor (BDNF) is tightly controlled to wild-type (WT), but not mutant HTT,7 and so unsurprisingly neither the level of BDNF mRNA nor protein in the plasma reliably tracks HD progression.8 Another approach looked at the degree of fragmentation of mutant Huntingtin protein in peripheral immune cells using time-resolved Förster resonance energy transfer, but repeated measurements on a single sample produced considerable variability.9 It has been demonstrated more recently that the level of neurofilament light chain in the blood, which is elevated as neurodegeneration proceeds, is a potential biomarker to track the onset and progression of HD.10 However, this marker simply reflects cell loss and so is raised in other neurological events including ischemic stroke and Alzheimer's disease, and as such does not necessarily track HD per se.11,12
Given all this, we sought to investigate whether we could use recent advances in techniques based on Raman scattering to develop a new serum biomarker approach in patients with HD.13 Spontaneous Raman Spectroscopy (RS), in particular, is increasingly being researched for applications in medical diagnostics using biofluids, cell and tissue samples due to its high chemical specificity and capacity to reveal molecular information.14,15 RS is a sensitive, label-free, and non-destructive optical method, which measures the light inelastically scattered by intrinsic molecular vibrations in a specimen. The measurement yields a ‘spectral fingerprint’ corresponding to the molecular and biochemical composition of the sample. Practically the measurement is simple to undertake-for example a droplet of blood serum (Fig. 1A) is interrogated with a laser to give a Raman spectrum (blue spectrum, Fig. 1B). Peaks in the resulting spectra correspond to information from molecular constituents in the serum, thereby generating unique signatures for them.
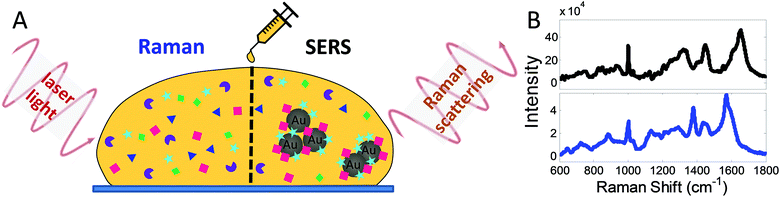 |
| Fig. 1 Methodology with RS and SERS. (A) An incident laser light excites characteristic vibrations on interaction with the molecules (coloured symbols) in the sample (here illustrated for a drop of blood serum) resulting in Raman scattering which is then detected as a spectrum (B). While all molecules in the bulk of the sample can be interrogated with RS, only molecules in the direct vicinity of gold nanoparticles (AuNPs) (grey spheres) contribute to the SERS signature. Signals in SERS (B, black spectrum) are orders of magnitude stronger than in RS (B, blue spectrum), as well as surface-selective (cyan stars and red squares in A). | |
While spontaneous Raman spectroscopy provides a characteristic ‘fingerprint’ of a sample, signals can be weak. For this reason, the optical properties of nanomaterials can be used to produce stronger signals, which is called surface-enhanced Raman spectroscopy (SERS). SERS uses the enhancing effect of nano-scale metals, typically ‘coinage’ metals and materials such as gold nanoparticles (AuNPs), as these interact with the incident light and nanostructure (see SERS in Fig. 1A). Thus, AuNPs can be added as an assay reagent before the measurement and because this enhancing effect is locally confined to molecules within proximity of the AuNPs, the obtained SERS spectrum (black spectrum, Fig. 1B) mainly reflects contributions from these molecules.16–18
Both RS and SERS have been used previously to measure serum analytes including glucose, cholesterol, triglycerides, urea and albumin.14,19 Furthermore, Raman-based techniques have been used successfully in a variety of medical conditions including asthma,20 neurodegenerative diseases,13,21,22 malaria,14 as well as head-and-neck,23 nasopharyngeal,24 breast,25,26 liver,27 and colorectal cancers.28 RS may also be a useful diagnostic tool for Alzheimer's disease using patient serum.22,29 In HD, RS has previously been used to study fibroblasts from patients,30,31 although its potential to assess disease states or to provide a biomarker has never been explored. However, it would have an obvious advantage when employed in this way as mutant huntingtin (mHTT) affects multiple pathways in multiple types of cell systems and organs,32 and as such RS/SERS could be used to identify HD-specific “signatures” rather than single biomarker molecules. This is likely to better reflect the complete range of cell autonomous and non-cell autonomous abnormalities found in this neurological condition.
We therefore acquired Raman and SERS spectra in a cross-sectional cohort of HD patients and control participants (n = 62) and applied both a principal component (PCA) and linear discriminant analysis (LDA) to interrogate our high dimensional dataset. Multivariate techniques such as PCA and LDA are widely used in biomedical RS/SERS due to the complexity of spectral data sets and have found application in cellular16,18,33 and tissue analysis including in blood/serum for diagnosis.34–37 Using PCA/LDA on acquired spectra and by carefully assessing different spectral regions for their marker abilities, we found that SERS is able to detect, with a higher specificity than RS, disease stages in line with clinical assessments. In addition, we also found that there were specific spectral features associated with the HD samples due to changes in serum molecules that are related to protein misfolding and nucleotide catabolism.
In summary, we have shown the feasibility of obtaining spectral biomarkers by Raman spectroscopy-based techniques such as SERS in HD patients and that this approach could potentially play an important role in the early diagnosis of HD and its treatment with disease-modifying therapies.
Results and discussion
While the analysis of bio-fluid samples is relatively straightforward for RS, optimization is essential for SERS analysis due to the use of aggregated AuNPs (ESI Fig. S1†). We first optimised and validated our SERS methodology using cortical homogenates and serum samples from female R6/2 mice: a well-known transgenic murine model of HD (Fig. 2).4,38,39 In R6/2 mice (CAG repeat size 141–157), neurological abnormalities are observed around 7–8 weeks of age and progressively worsen until about 12–13 weeks of age, when they are euthanised due to major weight loss.39
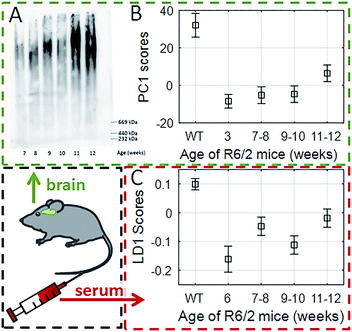 |
| Fig. 2 Tracking the progression of HD in cortical homogenates (A and B) and serum of transgenic R6/2 mice with SERS (C). (A) The AGERA shows both an increase in mutant Huntingtin aggregate size and intensity in the cortex of R6/2 mice as disease progresses. (B) Using a PCA approach we were able to separate WT littermates from transgenic littermates at 12 weeks of age; PC1 scores generated from SERS spectra of cortical homogenates of the R6/2 mice at different disease stages showed a similar progression profile to AGERA analysis. (C) Using a SERS approach on the serum, LD1 scores also correlated with the disease progression. Box and whiskers indicate estimated mean and standard deviation of multivariate ANOVA with post-hoc Bonferroni correction. | |
We first showed that there was (as expected) accumulation of mutant Huntingtin aggregates in cortical (brain) homogenates of R6/2 mice using agarose gel electrophoresis (AGERA) with the MW8 antibody (Fig. 2A). The SERS data was then compared with WT littermates using PCA and LDA (ESI Table S1 and Fig. S2†). Using this approach, we found that the first principal component (PC1) and linear discriminant scores (LD1) were sufficient for genotype classification into WT and HD, respectively. A significant (p < 0.001) segregation between PC1 scores in both groups was associated with disease progression (Fig. 2B). This signature was characterised by dominant spectral changes in those peaks assigned to proline (993 and 1070 cm−1) and β-sheet protein conformation (955, 1020 and 1086 cm−1). Furthermore, our data showed that even at 3 weeks of age when the R6/2 mice and WT littermates are clinically indistinguishable,33 SERS was already capable of genotype classification (ESI Table S2†). In contrast to brain homogenates, with serum samples PCA alone was not able to provide enough segregation (ESI Fig. S2B†) and it was only when applying both PCA-LDA that we were able to achieve a significant segregation of the LD1 scores with disease progression using serum samples (Fig. 2C) (p < 0.001). All of this thus supported the potential application of using SERS to study HD patient serum.
To study the application of RS/SERS results in clinical specimens, we began by analyzing the stability of serum samples and the reproducibility of the spectral results under different storage conditions. We found that prolonged storage at 4 °C (>2 days) affected the reproducibility of RS measurements, while SERS spectra remained consistent when the samples were stored at either 4 °C (≤15 days) or frozen and then thawed (ESI Fig. S3 and Table S3†). PC scores of RS/SERS spectra from serum samples were compared between HD patients (nMale-HD = 27 and nFemale-HD = 20) and healthy participants (nMale-ctrl = 5 and nFemale-ctrl = 10) (ESI Table S4†). For the RS data (Fig. 3A), PC1 and PC2 scores revealed a significant distinction between HD patients and healthy participants (PC1: pmale HD-ctrl = 0.003, PC2: pmale HD-ctrl = 0.001, pfemale HD-ctrl = 0.001). For SERS (Fig. 3B), there was a significant genotype effect only in male participants in the PC2 direction (PC2: pmale HD-ctrl = 0.02). While these results were generated using the full spectral range, it is better to identify specific features in the spectral intervals which are the best classification discriminators and relate to the molecular basis of HD pathology. We therefore sub-grouped the spectral data into intervals of 50 cm−1. Using RS, HD patients could be clearly distinguished from healthy participants using the spectral region between 300–500 cm−1 and 600–1650 cm−1, with the most significant distinction observed for the spectral region of 600–800 cm−1 (p < 0.01, Fig. 3C). For SERS, we found a significant distinction between all HD patients (male and female) and healthy participants in the 400–1200 cm−1 range, with the most significant distinction being found between 750–800 cm−1 (p < 0.01, Fig. 3D).
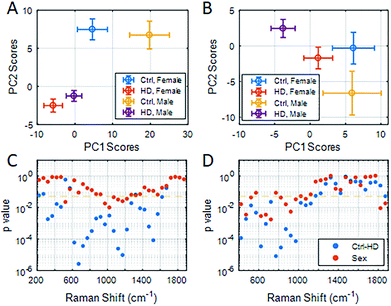 |
| Fig. 3 PC1 vs. PC2 scores represented as a scatter plot for RS (A) and SERS (B) analysis of serum from HD patients and healthy participants (ctrl) using the full spectral range (RS: 200–1900 cm−1, SERS: 400–1900 cm−1). (C and D) PC1 scores were used to compute the p value for group segregation between genotype (blue) and gender (red), to indicate spectral intervals of significant group segregation for RS (C) and SERS (D). The significance level of p = 0.05 is indicated by an orange dashed line. Results were analyzed using ANOVA with post-hoc Bonferroni correction. | |
To better characterise the molecular changes, the mean (Fig. 4A and B), standard deviation (Fig. 4C and D) and the difference spectra (Δ in Fig. 4E and F) were calculated for both RS and SERS spectra. As the intensity of the difference spectra (black line in Fig. 4E and F) was smaller than the respective standard deviation, we sought to use the more robust PCA-LDA approach to generate spectral LD loadings (rainbow-coloured line in Fig. 4E and F). The pattern of LD loadings and the difference spectra are highly consistent, although their intensities differ. The coloured line represents the explained variance of the PC1 scores (stepwise for 20 cm−1 intervals) specific to spectral peaks in the LD loadings (Fig. 4E and F, red to blue: 100% to 0% explained variance). The explained variance of RS only exceeds 90% in the range of 950–1750 cm−1, while it covers most of the spectrum (400–1800 cm−1) for SERS (Fig. 4E and F).
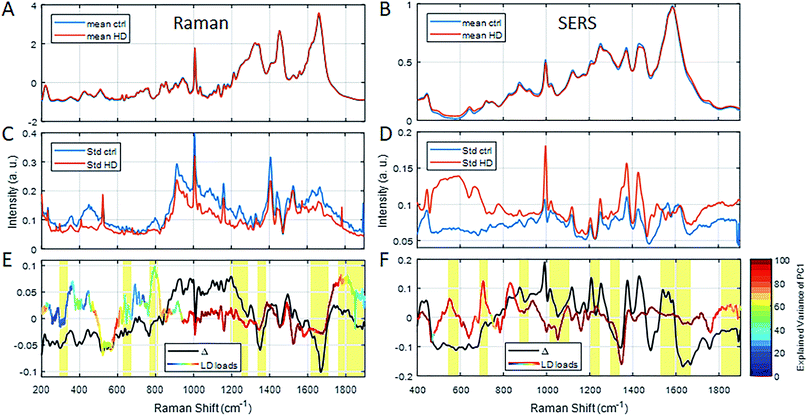 |
| Fig. 4 Average RS (A) and SERS (B) spectra of serum from healthy control subjects (blue lines) and HD patients (red line) as well as their standard deviations (C and D, respectively). The different spectra of the averages for RS (black line, E) and SERS (black line, F) are within the standard deviation (C and D) of the average spectra (A and B). Hence, LD loadings for each of the spectral methods, respectively (coloured line in E and F), are also taken into account alongside the explained variance of the underlying PC1 scores (colouration of LD loadings line, see colour bar in E). Yellow marked regions indicate important peaks. Refer to ESI† for peak assignments. | |
To determine if such spectral differences correlated with clinical measures, we next grouped correlated PC1 scores for intervals (each 50 cm−1) of the RS (Fig. 5) and SERS spectra (Fig. 6) to a range of standard clinical parameters in the HD patients including: (i) age, (ii) independence score (Indp), (iii) CAG repeat size (CAG), (iv) disease burden score (DB), (v) functional assessment score (FA), (vi) total functional capacity score (TFC), as well as (vii) total Unified Huntington's Disease Rating Scale (UHDRS) motor score (see Experimental section).40–42 Since some clinical assessment scales show linear correlation, for instance the DB is a function of age and CAG repeat size,40 linear regression of the estimated means (post-hoc ANOVA) of grouped PC1 scores from HD patients was computed and the adjusted R2 (aR2) was used to correlate spectral regions and clinical parameters (Fig. 5 and 6, and summarised in ESI Fig. S4†).
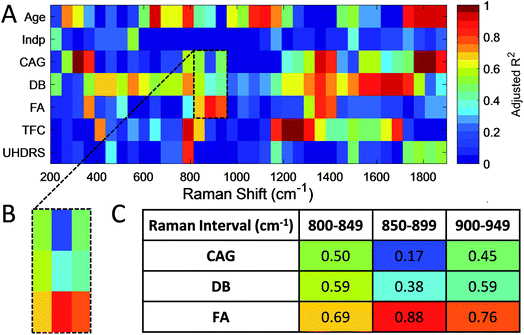 |
| Fig. 5 (A, B) The colour map shows the linear correlation of PC1 scores for spectral RS intervals (50 cm−1, x-axis) with different clinical HD assessment parameters (y-axis). High correlation is observed with patient's age (650–700 cm−1, 1750–1880 cm−1), DB (1600–1650 cm−1), CAG repeat size (300–350 cm−1, 1750–1850 cm−1) and TFC (1200–1250 cm−1). (C) The aR2 of the linear fit is colour-coded for each spectral interval and clinical parameter. Darker red colouration suggests better linear correlation. | |
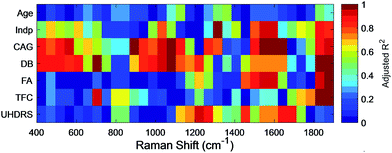 |
| Fig. 6 The colour map shows the linear correlation of PC1 scores for SERS intervals (50 cm−1, x-axis) with different clinical HD assessment parameters (y-axis). PC1 scores from SERS were highly correlated with Indp levels (1300–1350 cm−1), CAG repeat length (1600–1650 cm−1), DB scores (700–750 cm−1), FA scores (1300–1350 cm−1), TFC (1300–1350 cm−1) and UHDRS motor scores (1200–1250 cm−1). The aR2 of the linear fit is colour-coded for each spectral interval and clinical parameter. Darker red colouration suggests better linear correlation. | |
PC1 scores of serum RS showed a high correlation, that is, aR2 > 0.90 only in some spectral regions relative to the patient's age, CAG and TFC (Fig. 5). We found that the influence of patient age on the PC1 scores of RS spectral ranges are negligible, with the exception of the region of 650–700 cm−1 (aR2Age = 0.91) and 1750–1800 cm−1 (aR2Age = 0.98) with significant peaks at 655 and 692 cm−1, in line with the age-related features assigned previously to C–S stretching and twisting modes in proteins, respectively.43 This therefore provides the basis for distinguishing spectral changes specific to HD from those related to age. The CAG repeat length determines in part the age of onset and less so the rate of progression in HD44,45 and, in combination with the patient's age, the DB score can be computed. PC1 scores of RS showed a linear correlation with CAG size only between 300–350 cm−1 and 1750–1850 cm−1 with an aR2CAG of 1.00 and 0.97, respectively, and with DB in the range of 1600–1650 cm−1 (aR2DB = 0.93).
For SERS, PC1 scores showed a high correlation (aR2 > 0.95) to Indp, CAG, DB, FA, TFC and UHDRS scores (summarised in ESI Fig. S4 and Table S5†). In particular, both CAG size and DB correlated with PC1 scores in the SERS spectra (Fig. 6, ESI Fig. S4 and Table S5†) in the same regions, particularly in the region of 500–600 cm−1 (aR2CAG = 0.97 and aR2DB = 0.86) and 1000–1100 cm−1 (aR2CAG = 0.94 and aR2DB = 0.96). The overlapping SERS regions correlated to CAG repeat length and DB scores, which was not found with the RS data, suggesting that there are HD-associated molecules in the serum, which although present at a very low concentration, have an affinity for gold nanoparticles. Characteristic peaks associated with these intervals are at 640, 996, 1022 and 1120 cm−1, which are assigned to phenylalanine (996 cm−1) and uric acid, respectively (ESI Table S6†). Interestingly, serum levels of uric acid have been linked to catabolism46 that has been described as a feature of HD in some studies, including an earlier metabonomic study from our group.47,48 Additionally, DB scores correlated strongly (aR2DB > 0.99) to the PC1 scores of SERS in the region of 700–750 cm−1. This interval shows a characteristic peak at 720 cm−1, which can be assigned to DNA/RNA (adenine) and has previously been shown to be a diagnostic marker for diseases such as cancer and other systemic metabolic disorders.49
The UHDRS was developed to provide a standardised assessment of clinical features and progression of HD in patients. It includes motor and functional assessments, evaluation on the level of independence of patients, and a measure of functional capacity.41 A UHDRS motor score of ≥5 is usually considered diagnostic of disease state. We therefore correlated UHDRS motor scores to PC1 scores in manifest (UHDRS ≥ 5) and pre-manifest (UHDRS < 5) HD patients as well as healthy participants. While no correlation was observed between UHDRS scores and PC1 scores in RS, we found a linear correlation in the range of 1200–1300 cm−1 and 1600–1700 cm−1 for SERS (both aR2UHDRS = 0.91, Fig. 6) and combining the data from both regions improved the correlation (Fig. 7A, aR2UHDRS = 0.97). RS/SERS data contained samples from male and female participants as all three intervals did not show a significant segregation with gender. The effect of genotype on motor impairments, in particular the transition from pre-manifest to manifest, could only be revealed using a supervised PCA-LDA approach on SERS data classed as control vs. HD (Fig. 7B). UHDRS vs. LD1 scores showed a clear distinction (pcontrol-manifestHD < 0.001) between the control participants and manifest HD groups. The pre-manifest HD group (UHDRS motor < 5) did not show clear segregation from the controls (pcontrol-premanifestHD = 0.25) nor the manifest HD groups (ppremanifest-manifestHD > 0.92), but appeared to occupy a transitional state. These group segregations, in both the PC and the LD space, were based on significant peaks in the region of 1200–1300 cm−1 and 1600–1700 cm−1 (Fig. 6 and UHDRS in ESI Table S6†). These bands were assigned to amide III and amide I,50 respectively, giving important structural information on the protein structure. The peak at 1245 cm−1 is mainly assigned to the C–C and C–N stretching modes in β-sheet protein secondary structures.51 Complementarily, the peak at 1667 cm−1 in the amide I band originates from the C
O stretching mode in β-sheets.51,52 These two bands suggest a higher content of β-sheet protein structures present in the HD sera compared to healthy controls, in line with previous reports on the presence of mutant Huntingtin aggregates in the patient blood.53 Overall, our data suggested that PC1 scores for manifest HD patients show a linear correlation with UHDRS scores, while pre-manifest HD patients appear to be an interim group between healthy controls and manifest HD patients in the LD space, suggesting that SERS may be useful for defining disease onset.
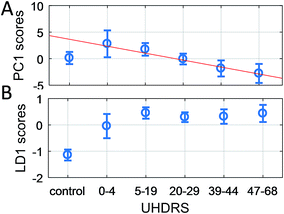 |
| Fig. 7 Grouped UHDRS motor scores vs. PC1 scores (A) and LD1 scores (B) from the combined SERS spectral region 1200–1300 cm−1 and 1600–1700 cm−1. (A) A linear curve (aR2UHDRS = 0.97) has been fitted to the PC1 scores of manifest HD groups (UHDRS ≥ 5), which also has a good correlation for the pre-manifest group (UHDRS < 5). (B) In contrast, the LD1 scores significantly distinguishes (pcontrol-HD < 0.001) the control group from all four manifest HD groups, while the pre-manifest HD group appears to occupy a transitional state between the healthy controls and manifest HD subjects. | |
Conclusion
In conclusion, using both a RS and SERS analytical approach to study the serum samples of HD patients, we have demonstrated that SERS is better suited as a novel and robust tool by which to detect molecular changes related to disease onset and progression in HD. In particular, various patient characteristics (e.g. age, genetic status and DB scores) along with clinical scores (e.g. Indp, TFC, FA and UHDRS) show linear correlations to the specific spectral intervals of RS and/or SERS spectra. While RS is capable of measuring intrinsic patient characteristics such as the age and genetic predisposition (CAG repeat size), SERS offers a higher level of specificity with respect to disease status in HD patients including clinical scores. This indicates the presence of HD associated molecules at very low concentrations in the serum with a natural affinity for gold nanoparticles, and thus providing selectivity for detection using SERS. Overall, our findings suggest that SERS correlates well with established clinical assessments, while offering an easy, patient-friendly and objective assessment of HD state and stage. It therefore has the potential to be used for the diagnosis of disease onset as well as being of value in tracking disease progress and response to disease-modifying therapies which are now starting to be trialled in the clinic.54
Experimental section
Ethics and processing of human samples
All human samples were collected with informed consent under full ethical approval in accordance with the United Kingdom's Department of Health guidelines and local ethical approval (NRES Committee East of England – Cambridge Central, reference no. 96/085). Demographic profiles for all participants can be found in ESI Table S4.† Blood was collected and serum samples were prepared by allowing the blood to clot at room temperature for 20 minutes, followed by centrifugation (2000×g) at 4 °C for 20 minutes. Serum samples were either frozen at −20 °C for storage or used fresh at 4 °C within 6 hours of collection.
Ethics and processing of animal samples
All animal procedures were performed in accordance with the UK Animals (Scientific Procedures) Act 1986 and approved by the University of Cambridge Animal Welfare Policy. R6/1 transgenic mice were purchased from the Jackson Laboratory (USA) and the colony was maintained by backcrossing to C57BL/6 females purchased from Harlan Laboratories (UK). After the mice were weaned, tissue from ear biopsies was sent to Largen Inc. (USA) for genotyping. All animals were kept in a temperature and humidity-controlled (22 °C) room on a 12 hour light/dark cycle. The mice were separately housed in single-sex cages with 3–4 mice per cage. Water and food were made freely available in the home cage. Animals were culled using Schedule 1 methods at the specified age and, after sacrifice, blood was immediately collected via a heart puncture (mice) while serum samples were prepared as described above. Brains were removed from the skull with the frontal cortex dissected and snap-frozen to −80 °C before further processing.
Agarose gel electrophoresis (AGERA)
Crude tissue homogenates were prepared using a dounce homogeniser in 1% Triton X-100 plus complete protease inhibitor cocktail (Roche). Homogenates were centrifuged (4000×g) at 4 °C for 10 minutes, and electrophoresis was performed on a gel containing 1.8% agarose (Bioline) and 0.1% SDS (Sigma) dissolved in 375 mM Tris–HCl (pH 8.8). A total of 25 μg of protein was loaded per well. The AGERA gel was run at 100 V in Tris–glycine SDS running buffer and transferred in Tris–glycine transfer buffer at 15 V at 4 °C. Immunoblotting was performed using the mouse monoclonal MW8 antibody (1/1000, DSHB) against mutant huntingtin protein.
RS and SERS experiments
All experiments were performed using a Renishaw® inVia Raman microscope with 633 and 785 nm HeNe and diode lasers, typically ≈1 mW.
For RS experiments, 10 μL of serum was placed on the surface of a gold-coated (200 nm vapour deposited; surface roughness <1 nm) silicon wafer and covered with a small glass coverslip (Ø 13 mm) to limit evaporation during the measurements. A gold-coated wafer was used to provide a smooth reflective surface to increase path length and hence, Raman signal collection. Raman spectra in the range of 200–2000 cm−1 were acquired in point scan mode using a ×50 working objective and an exposure time of 15 seconds. A grating of 1200 lines/mm was used with an appropriate edge filter. Raman spectroscopy was carried out typically with 1 mW of laser power using a HeNe 633 nm laser.
For SERS experiments, 1 ml of citrate-capped, spherical gold nanoparticles (Ø 40 nm, 9 × 10−10 particles per mL, 2.96 × 10−4 M of gold per L, BBI Solutions, UK) was centrifuged (15 min at 10
000 rpm) and 980 μL of supernatant was removed. 5 μL of the concentrated AuNPs were pre-aggregated by mixing with 1 μL of 10× concentrated phosphate buffered saline (Sigma) and then mixed with 5 μL of patient serum. After 30 min of incubation, aggregated AuNPs were spun down (30 s at 8000 rpm) and the mixture was pipetted onto a glass slide and covered by a glass coverslip. All SERS experiments were performed using a Renishaw® inVia Raman microscope with a 633 nm laser in streamline mode for map scans (pixel size: 600 nm × 600 nm). A Leica 100× (NA = 0.85) objective was used. Measurements on larger AuNPs clusters were carried out in line mapping (StreamLine©) using a collection time of 15–20 s per line over a spectral range of 400–2200 cm−1. The excitation power density was ∼2 × 104 W cm−2. Wire 3.4 software was used for data acquisition and initial processing.
Data analysis
Exported raw spectra from map scans were background subtracted using MATLAB 2017a as done previously16–18 following z-score standardization prior to PCA and PCA-LDA analysis.
The correlation of RS/SERS results with clinical measures (Fig. 5–7) was achieved by regression analysis of grouped PC1 scores of the RS and SERS spectra with disease features of HD patients. Due to the complexity of the RS/SERS as well as clinical patient data, a methodology was developed to identify correlations between these seven assessment categories and the PC1 scores, which were calculated for spectral intervals of 50 cm−1. This spectral segmentation allows for the identification of significant peaks. Following the initial PCA analysis, PC1 scores of HD patients were split evenly into four groups for each disease feature. PC1 scores of healthy participants formed a separate group, except for the patient's age, where five groups were chosen without a distinction between HD and healthy participants. ANOVA with post-hoc Bonferroni correction for multiple comparisons were generated for each group as were estimated means and standard deviations.
Author contributions
A. H., W. L. K., S. M. and R. A. B. conceived the study and designed the experiments. S. L. M. and R. A. B. consented and collected all samples. A. H. and W. L. K. performed the experiments, A. H., W. L. K., and S. M. analyzed the results. The manuscript was written through contributions of all authors. All authors have given approval to the final version of the manuscript.
Conflicts of interest
There are no conflicts to declare.
Acknowledgements
This study was funded by the Rosetrees Trust (CM619). W. L. K. is supported by the MRC (MR/S005528/1). S. M. acknowledges funding through EPSRC grant (EP/H028757/2) and an ERC grant (NanoChemBioVision 638258). R. A. B. receives support from the National Institute for Health Research award as a senior investigator (NF-SI-0616-10011), through the Biomedical Research Center at the University of Cambridge (146281), as well as support from the Wellcome/MRC Cambridge Stem Cell Institute (203151/Z/16/Z).
References
- J. S. Paulsen, D. R. Langbehn, J. C. Stout, E. Aylward, C. A. Ross, M. Nance, M. Guttman, S. Johnson, M. MacDonald and L. J. Beglinger,
et al., Detection of Huntington's Disease Decades Before Diagnosis: The Predict-HD Study, J. Neurol., Neurosurg. Psychiatry, 2008, 79, 874–880 CrossRef CAS PubMed.
- J. S. Paulsen, J. D. Long, C. A. Ross, D. L. Harrington, C. J. Erwin, J. K. Williams, H. J. Westervelt, H. J. Johnson, E. H. Aylward and Y. Zhang,
et al., Prediction of Manifest Huntington's Disease with Clinical and Imaging Measures: A Prospective Observational Study, Lancet Neurol., 2014, 13, 1193–1201 CrossRef PubMed.
- S. J. Tabrizi, R. I. Scahill, G. Owen, A. Durr, B. R. Leavitt, R. A. Roos, B. Borowsky, B. Landwehrmeyer, C. Frost and H. Johnson,
et al., Predictors of Phenotypic Progression and Disease Onset in Premanifest and Early-Stage Huntington's Disease in the Track-HH Study: Analysis of 36-Month Observational Data, Lancet Neurol., 2013, 12, 637–649 CrossRef.
- L. A. Lione, R. J. Carter, M. J. Hunt, G. P. Bates, A. J. Morton and S. B. Dunnett, Selective Discrimination Learning Impairments in Mice Expressing the Human Huntington's Disease Mutation, J. Neurosci., 1999, 19, 10428–10437 CrossRef CAS.
- D. L. Harrington, M. Rubinov, S. Durgerian, L. Mourany, C. Reece, K. Koenig, E. Bullmore, J. D. Long, J. S. Paulsen and S. M. Rao, Network Topology and Functional Connectivity Disturbances Precede the Onset of Huntington's Disease, Brain, 2015, 138, 2332–2346 CrossRef PubMed.
- S. L. Mason, J. Zhang, F. Begeti, N. V. Guzman, A. S. Lazar, J. B. Rowe, R. A. Barker and A. Hampshire, The Role of the Amygdala During Emotional Processing in Huntington's Disease: From Pre-Manifest to Late Stage Disease, Neuropsychologia, 2015, 70, 80–89 CrossRef PubMed.
- E. Cattaneo, C. Zuccato and M. Tartari, Normal Huntingtin Function: An Alternative Approach to Huntington's Disease, Nat. Rev. Neurosci., 2005, 6, 919–930 CrossRef CAS PubMed.
- C. Zuccato, M. Marullo, B. Vitali, A. Tarditi, C. Mariotti, M. Valenza, N. Lahiri, E. J. Wild, J. Sassone and A. Ciammola,
et al., Brain-Derived Neurotrophic Factor in Patients with Huntington's Disease, PLoS One, 2011, 6, e22966 CrossRef CAS.
- A. Weiss, U. Träger, E. J. Wild, S. Grueninger, R. Farmer, C. Landles, R. I. Scahill, N. Lahiri, S. Haider and D. Macdonald,
et al., Mutant Huntingtin Fragmentation in Immune Cells Tracks Huntington's Disease Progression, J. Clin. Invest., 2012, 122, 3731–3736 CrossRef CAS.
- L. M. Byrne, F. B. Rodrigues, K. Blennow, A. Durr, B. R. Leavitt, R. A. C. Roos, R. I. Scahill, S. J. Tabrizi, H. Zetterberg and D. Langbehn,
et al., Neurofilament Light Protein in Blood as a Potential Biomarker of Neurodegeneration in Huntington's Disease: A Retrospective Cohort Analysis, Lancet Neurol., 2017, 16, 601–609 CrossRef CAS PubMed.
- N. Mattsson, U. Andreasson, H. Zetterberg and K. Blennow, Association of Plasma Neurofilament Light with Neurodegeneration in Patients with Alzheimer Disease, JAMA Neurology, 2017, 74, 557–566 CrossRef PubMed.
- S. Tiedt, M. Duering, C. Barro, A. G. Kaya, J. Boeck, F. J. Bode, M. Klein, F. Dorn, B. Gesierich and L. Kellert,
et al., Serum Neurofilament Light: A Biomarker of Neuroaxonal Injury after Ischemic Stroke, Neurology, 2018, 91, e1338–e1347 CrossRef CAS PubMed.
- G. Devitt, K. Howard, A. Mudher and S. Mahajan, Raman Spectroscopy: An Emerging Tool in Neurodegenerative Disease Research and Diagnosis, ACS Chem. Neurosci., 2018, 9, 404–420 CrossRef CAS PubMed.
- K. Kong, C. Kendall, N. Stone and I. Notingher, Raman Spectroscopy for Medical Diagnostics-from in-Vitro Biofluid Assays to in-Vivo Cancer Detection, Adv. Drug Delivery Rev., 2015, 89, 121–134 CrossRef CAS PubMed.
- A. Bonifacio, S. Cervo and V. Sergo, Label-Free Surface-Enhanced Raman Spectroscopy of Biofluids: Fundamental Aspects and Diagnostic Applications, Anal. Bioanal. Chem., 2015, 407, 8265–8277 CrossRef CAS PubMed.
- A. Huefner, W. L. Kuan, K. H. Muller, J. N. Skepper, R. A. Barker and S. Mahajan, Characterization and Visualization of Vesicles in the Endo-Lysosomal Pathway with Surface-Enhanced Raman Spectroscopy and Chemometrics, ACS Nano, 2016, 10, 307–316 CrossRef CAS PubMed.
- A. Huefner, D. Septiadi, B. D. Wilts, I. I. Patel, W. L. Kuan, A. Fragniere, R. A. Barker and S. Mahajan, Gold Nanoparticles Explore Cells: Cellular Uptake and Their Use as Intracellular Probes, Methods, 2014, 68, 354–363 CrossRef CAS PubMed.
- A. Huefner, W. L. Kuan, R. A. Barker and S. Mahajan, Intracellular SERS Nanoprobes for Distinction of Different Neuronal Cell Types, Nano Lett., 2013, 13, 2463–2470 CrossRef CAS PubMed.
- A. J. Berger, T. W. Koo, I. Itzkan, G. Horowitz and M. S. Feld, Multicomponent Blood Analysis by near-Infrared Raman Spectroscopy, Appl. Opt., 1999, 38, 2916–2926 CrossRef CAS PubMed.
- A. Sahu, K. Dalal, S. Naglot, P. Aggarwal and C. Murali Krishna, Serum Based Diagnosis of Asthma Using Raman Spectroscopy: An Early Phase Pilot Study, PLoS One, 2013, 8, e78921 CrossRef CAS PubMed.
- M. Paraskevaidi, P. L. Martin-Hirsch and F. L. Martin, Progress and Challenges in the Diagnosis of Dementia: A Critical Review, ACS Chem. Neurosci., 2018, 9, 446–461 CrossRef CAS PubMed.
- M. Paraskevaidi, C. L. M. Morais, D. E. Halliwell, D. M. A. Mann, D. Allsop, P. L. Martin-Hirsch and F. L. Martin, Raman Spectroscopy to Diagnose Alzheimer's Disease and Dementia with Lewy Bodies in Blood, ACS Chem. Neurosci., 2018, 9, 2786–2794 CrossRef CAS PubMed.
- A. T. Harris, A. Lungari, C. J. Needham, S. L. Smith, M. A. Lones, S. E. Fisher, X. B. Yang, N. Cooper, J. Kirkham and D. A. Smith,
et al., Potential for Raman Spectroscopy to Provide Cancer Screening Using a Peripheral Blood Sample, Head Neck Oncol., 2009, 1, 34 CrossRef PubMed.
- S. X. Li, Q. Y. Zeng, L. F. Li, Y. J. Zhang, M. M. Wan, Z. M. Liu, H. L. Xiong, Z. Y. Guo and S. H. Liu, Study of Support Vector Machine and Serum Surface-Enhanced Raman Spectroscopy for Noninvasive Esophageal Cancer Detection, J. Biomed. Opt., 2013, 18, 27008 CrossRef PubMed.
- J. L. Pichardo-Molina, C. Frausto-Reyes, O. Barbosa-Garcia, R. Huerta-Franco, J. L. Gonzalez-Trujillo, C. A. Ramirez-Alvarado, G. Gutierrez-Juarez and C. Medina-Gutierrez, Raman Spectroscopy and Multivariate Analysis of Serum Samples from Breast Cancer Patients, Lasers Med. Sci., 2007, 22, 229–236 CrossRef CAS PubMed.
- L. Guo, Y. Zhang, J. Y. Lü, W. B. Gao, S. F. Wu and R. Y. Wang, Multivariate Statistical Analysis of Serum from Breast Cancer Patients Using Surface Enhanced Raman Spectrum, Spectrosc. Spectral Anal., 2013, 33, 1553–1556 CAS.
- L. Taleb, G. Thiefin, C. Gobinet, V. Untereiner, B. Bernard-Chabert, A. Heurgue, C. Truntzer, P. Hillon, M. Manfait and P. Ducoroy,
et al., Diagnosis of Hepatocellular Carcinoma in Cirrhotic Patients: A Proof-of-Concept Study Using Serum Micro-Raman Spectroscopy, Analyst, 2013, 138, 4006–4014 RSC.
- X. Li, T. Yang and S. Li, Discrimination of Serum Raman Spectroscopy between Normal and Colorectal Cancer Using Selected Parameters and Regression-Discriminant Analysis, Appl. Opt., 2012, 51, 5038–5043 CrossRef CAS PubMed.
- E. Ryzhikova, O. Kazakov, L. Halamkova, D. Celmins, P. Malone, E. Molho, E. A. Zimmerman and I. K. Lednev, Raman Spectroscopy of Blood Serum for Alzheimer's Disease Diagnostics: Specificity Relative to Other Types of Dementia, J. Biophotonics, 2015, 8, 584–596 CrossRef PubMed.
- M. Muratore, Raman Spectroscopy and Partial Least Squares Analysis in Discrimination of Peripheral Cells Affected by Huntington's Disease, Anal. Chim. Acta, 2013, 793, 1–10 CrossRef CAS PubMed.
- D. Tsikritsis, A. Elfick and A. Downes, Raman Spectroscopy of Fibroblast Cells from a Huntington's Disease Patient, Spectrosc. Lett., 2016, 49, 535–541 CrossRef CAS.
- D. Bano, F. Zanetti, Y. Mende and P. Nicotera, Neurodegenerative processes in Huntington's disease, Cell Death Dis., 2011, 2, e228 CrossRef CAS PubMed.
- I. Notingher, G. Jell, P. L. Notingher, I. Bisson, O. Tsigkou, J. M. Polak, M. M. Stevens and L. L. Hench, Multivariate analysis of Raman spectra for in vitro non-invasive studies of living cells, J. Mol. Struct., 2005, 744–747, 179–185 CrossRef CAS.
- S. Feng, J. Lin, Z. Huang, G. Chen, W. Chen, Y. Wang, R. Chen and H. Zeng, Esophageal cancer detection based on tissue surface-enhanced Raman spectroscopy and multivariate analysis, Appl. Phys. Lett., 2013, 102, 043702 CrossRef.
- Z. Li, C. Li, D. Lin, Z. Huang, J. Pan, G. Chen, J. Lin, N. Liu, Y. Yu, S. Feng and R. Chen, Surface-enhanced Raman spectroscopy for differentiation between benign and malignant thyroid tissues, Laser Phys. Lett., 2014, 11, 045602 CrossRef.
- K. Gajjar, L. D. Heppenstall, W. Pang, K. M. Ashton, J. Trevisan, I. I. Patel, V. Llabjani, H. F. Stringfellow, P. L. Martin-Hirsch, T. Dawson and F. L. Martin, Diagnostic segregation of human brain tumours using Fourier-transform infrared and/or Raman spectroscopy coupled with discriminant analysis, Anal. Methods, 2012, 5, 89–102 RSC.
- J. Wang, Y. Y. Zeng, J. Q. Lin, L. Lin, X. C. Wang, G. N. Chen, Z. F. Huang, B. H. Li, H. S. Zeng and R. Chen, SERS spectroscopy and multivariate analysis of globulin in human blood, Laser Phys. Lett., 2014, 24, 065602 CrossRef.
- J. Li, N. Popovic and P. Brundin, The Use of the R6 Transgenic Mouse Models of Huntington's Disease in Attempts to Develop Novel Therapeutic Strategies, Neurotherapeutics, 2005, 2, 447–464 CrossRef PubMed.
- H. G. Luesse, J. Schiefer, A. Spruenken, C. Puls, F. Block and C. M. Kosinski, Evaluation of R6/2 HD Transgenic Mice for Therapeutic Studies in Huntington's Disease: Behavioral Testing and Impact of Diabetes Mellitus, Behav. Brain Res., 2001, 126, 185–195 CrossRef CAS PubMed.
- J. B. Penney Jr, J. P. Vonsattel, M. E. MacDonald, J. F. Gusella and R. H. Myers, CAG Repeat Number Governs the Development Rate of Pathology in Huntington's Disease, Ann. Neurol., 1997, 41, 689–692 CrossRef CAS PubMed.
- Huntington Study Group, Unified Huntington's Disease Rating Scale: Reliability and Consistency. Huntington Study Group, Mov. Disord., 1996, 11, 136–142 CrossRef PubMed.
- T. A. Mestre, A. C. Bachoud-Levi, J. Marinus, J. C. Stout, J. S. Paulsen, P. Como, K. Duff, C. Sampaio, C. G. Goetz and E. Cubo,
et al., Rating Scales for Cognition in Huntington's Disease: Critique and Recommendations, Mov. Disord., 2017, 33, 187–195 CrossRef PubMed.
- T. Makhnii, O. Ilchenko, A. Reynt, Y. Pilgun, A. Kutsyk, D. Krasnenkov, M. Ivasyuk and V. Kukharskyy, Age-Related Changes in FTIR and Raman Spectra of Human Blood, Ukr. J. Phys., 2016, 61, 853–862 CrossRef.
- W. L. Kuan, A. Kasis, Y. Yuan, S. L. Mason, A. S. Lazar, R. A. Barker and J. Goncalves, Modelling the Natural History of Huntington's Disease Progression, J. Neurol., Neurosurg. Psychiatry, 2015, 86, 1143–1149 CrossRef CAS PubMed.
- D. R. Langbehn, R. R. Brinkman, D. Falush, J. S. Paulsen and M. R. Hayden, A New Model for Prediction of the Age of Onset and Penetrance for Huntington's Disease Based on CAG Length, Clin. Genet., 2004, 65, 267–277 CrossRef CAS PubMed.
- A. Bonifacio, S. Dalla Marta, R. Spizzo, S. Cervo, A. Steffan, A. Colombatti and V. Sergo, Surface-Enhanced Raman Spectroscopy of Blood Plasma and Serum Using Ag and Au Nanoparticles: A Systematic Study, Anal. Bioanal. Chem., 2014, 406, 2355–2365 CrossRef CAS PubMed.
- P. Auinger, K. Kieburtz and M. P. McDermott, The Relationship between Uric Acid Levels and Huntington's Disease Progression, Mov. Disord., 2010, 25, 224–228 CrossRef PubMed.
- B. R. Underwood, D. Broadhurst, W. B. Dunn, D. I. Ellis, A. W. Michell, C. Vacher, D. E. Mosedale, D. B. Kell, R. A. Barker and D. J. Grainger,
et al., Huntington Disease Patients and Transgenic Mice Have Similar Pro-Catabolic Serum Metabolite Profiles, Brain, 2006, 129, 877–886 CrossRef PubMed.
- D. Lin, S. Feng, J. Pan, Y. Chen, J. Lin, G. Chen, S. Xie, H. Zeng and R. Chen, Colorectal Cancer Detection by Gold Nanoparticle Based Surface-Enhanced Raman Spectroscopy of Blood Serum and Statistical Analysis, Opt. Express, 2011, 19, 13565–13577 CrossRef CAS PubMed.
- R. Petry, M. Schmitt and J. Popp, Raman Spectroscopy-a Prospective Tool in the Life Sciences, ChemPhysChem, 2003, 4, 14–30 CrossRef CAS PubMed.
- N. M. Perney, L. Braddick, M. Jurna, E. T. Garbacik, H. L. Offerhaus, L. C. Serpell, E. Blanch, L. Holden-Dye, W. S. Brocklesby and T. Melvin, Polyglutamine Aggregate Structure in Vitro and in Vivo; New Avenues for Coherent Anti-Stokes Raman Scattering Microscopy, PLoS One, 2012, 7, e40536 CrossRef CAS PubMed.
- N. C. Maiti, M. M. Apetri, M. G. Zagorski, P. R. Carey and V. E. Anderson, Raman Spectroscopic Characterization of Secondary Structure in Natively Unfolded Proteins: A-Synuclein, J. Am. Chem. Soc., 2004, 126, 2399–2408 CrossRef CAS.
- A. Weiss, D. Abramowski, M. Bibel, R. Bodner, V. Chopra, M. DiFiglia, J. Fox, K. Kegel, C. Klein and S. Grueninger,
et al., Single-Step Detection of Mutant Huntingtin in Animal and Human Tissues: A Bioassay for Huntington's Disease, Anal. Biochem., 2009, 395, 8–15 CrossRef CAS.
- S. J. Tabrizi, B. R. Leavitt, G. B. Landwehrmeyer, E. J. Wild, C. Saft, R. A. Barker, N. F. Blair, D. Craufurd, J. Priller and H. Rickards,
et al., Targeting Huntingtin Expression in Patients with Huntington's Disease, N. Engl. J. Med., 2019, 380, 2307–2316 CrossRef CAS.
Footnotes |
† Electronic supplementary information (ESI) available. See DOI: 10.1039/c9sc03711j |
‡ AH and WLK contributed equally to the work. |
|
This journal is © The Royal Society of Chemistry 2020 |
Click here to see how this site uses Cookies. View our privacy policy here.