Linking a hierarchy of attitude effect to student engagement and chemistry achievement
Received
2nd August 2019
, Accepted 1st November 2019
First published on 6th November 2019
Abstract
There are numerous influential factors contributing to students’ successful learning in introductory chemistry courses. One popularized factor is mathematical preparedness as gauged by a prerequisite mathematics grade or a standardized exam score. Less well known factors are students’ attitude toward the subject of chemistry or students’ involvement with chemistry. In this replication and extension study, students’ attitude in introductory chemistry courses, including a chemistry course designed for nursing and allied health students, was measured using the Attitude toward the Subject of Chemistry Inventory. Results show that a low-involvement hierarchy of attitude effect for students’ attitude toward chemistry, previously observed after 5 weeks, persists throughout a semester, and is likely widespread in classes across our department. Results also suggest a measurable difference between students’ emotional satisfaction with chemistry and their emotional attachment to chemistry, the latter of which is connected with their engagement with chemistry rather than their achievement in chemistry. Students’ low-involvement influence on chemistry achievement is being mediated predominantly by the affective subcomponent of their attitude. Attitudes are learned and a low-involvement hierarchy of attitude effect is susceptible to positive change. The results presented herein cement the reliability and generality of previous findings and extend those findings. The hierarchy of attitude effect can serve as a bifocal lens to capture evidence of students’ engagement with chemistry learning (learning process) as well as their chemistry achievement (learning product).
Introduction
Students have a choice in their education, and like any other consumer group, students expect value for money as paying customers of educational products (Arambewela et al., 2005; Maringe, 2005). Congruently, institutions of higher education recognize the importance of marketing directly to students as consumers of their educational products (Lauer, 2008). Therefore, courses such as chemistry are being marketed to students by institutions of higher education as educational products. Numerous marketing models exist that claim to explain the customers’ (students’) decision-making process, and many of these hierarchy of effect marketing models are not only applicable to marketing in higher education but can influence outcomes (Hemsley-Brown et al., 2009).
Chemistry achievement
The ultimate outcome in science, technology, engineering, and math (STEM) courses is students’ achievement. Achievement in STEM courses is a top priority for students as customers in these courses. Students expect and trust that the educators and institutions offering these courses are disseminating research-based best practices for maximizing their achievement. The currency of achievement in education is typically a course grade, and in the US this contributes to students’ grade point average (GPA). Thus, a course grade and its interpretation by students is how chemistry achievement or achievement in other STEM courses is typically measured. Chemistry achievement can also be assessed using standardized American Chemical Society (ACS) exams offered through the ACS Examinations Institute (ACS-EI). The latter exams have an arguable advantage of being norm-referenced and offering the chance to compare students’ performance against national samples (Lewis and Lewis, 2007; Murphy et al., 2012).
There are many avenues of research currently connecting with achievement in STEM. Active learning has been demonstrated to outcompete traditional lecture-based instruction in a wide variety of STEM courses (Freeman et al., 2014). The flipped classroom has documented advantages for exam performances and reductions in failure rates (Mooring et al., 2016; Ryan and Reid, 2016). Within chemistry education research, students’ prior success in middle school can positively influence achievement in high school (Kahveci, 2015) and similar experiences in high school chemistry can influence later achievement in college chemistry (Tai et al., 2005). In addition to findings that students’ mathematical ability can influence their chemistry achievement (Scott, 2012; Xu et al., 2013), students’ affective domain has been extensively investigated and has been linked to their chemistry achievement (Cukrowska et al., 1999; Barbera et al., 2008; Bauer, 2008; Lewis et al., 2009; Uzuntiryaki and Aydin, 2009; Else-Quest et al., 2013; Xu et al., 2013; Ferrell et al., 2016; Ross et al., 2018; Villafañe and Lewis, 2016).
Attitudes toward chemistry
Attitude has been defined as, “…a psychological tendency that is expressed by evaluating a particular entity with some degree of favor or disfavor” (Eagly and Chaiken, 1993). Attitude is a tripartite composite structure consisting of affective (A), behavioral (B), and cognitive (C) constructs (Rosenberg and Hovland, 1960; Wagner and Sherwood, 1969; Aiken, 1980). A popular way to measure students’ attitude toward chemistry is using the Attitude toward the Subject of Chemistry Inventory (ASCI) developed by Bauer (2008) or the shortened version (ASCIv2) modified by Xu and Lewis (2011). These instruments are introduced and described in our previous publication (Ross et al., 2018). Students’ attitude toward chemistry can be monitored and influenced during a course. In undergraduate college-level chemistry, a positive change in students’ attitude toward chemistry has been linked to more motivated student behavior (Berg, 2005; Richter-Egger et al., 2010; Chan and Bauer, 2014). A similar connection between students’ attitude and chemistry achievement has been observed at the secondary school level (Osborne et al., 2003). Students’ attitude toward chemistry has been observed to decline as they advance through high school (Montes et al., 2018).
The influence of students’ attitude toward science has been shown to explain 48% of the variance in students’ achievement in chemistry (Villafañe and Lewis, 2016). Students’ experiences in the classroom and their influence on attitude can be further impacted by new teaching approaches. Research of the interplay between curriculum and pedagogy changes, students’ attitude and their grades is current and ongoing, and the readership is directed to our earlier publication for further information (Ross et al., 2018).
Mathematical ability
A significant positive correlation exists between mathematical ability and achievement in chemistry (Bunce and Hutchinson, 1993; Spencer, 1996; Wagner et al., 2002; Ewing et al., 2005; Lewis and Lewis, 2007). Interestingly, Chan and Bauer (2014) demonstrated that students’ math SAT scores provided redundant information toward their affective profiling. In accordance, the capacity to overestimate the importance of students’ mathematical ability on their chemistry achievement has been shown when other factors like prior conceptual chemistry knowledge are ignored (Seery, 2009; Xu et al., 2013). Whereas mathematical ability and attitude together were shown to account for as much as 57% of the variance in chemistry achievement, the explained variance increased to 69% when students’ prior conceptual knowledge was also considered (Xu et al., 2013). Other factors would account for the remaining 31% variance in chemistry achievement. A likely candidate factor capable of influencing chemistry achievement is students’ involvement, which will be introduced shortly.
Hierarchy of attitude effect
There exist orders of influence among the sub-components of attitude (C, A, and B) which give insights into the degree of investment an individual has regarding an attitudinal object. Out of numerous theoretical hierarchy of attitude effects, two of them likely transfer over to the chemistry classroom, namely the low- and high-involvement hierarchy models (Ross et al., 2018). Here, involvement is, “a person's perceived relevance of the object based on their inherent needs, values, and interests” (Zaichkowsky, 1985). For example, the low-involvement hierarchy of attitude effect (C → B → A) model is observed when an individual has little invested in a decision-making process toward an attitudinal object, has no emotional attachment to the attitudinal object, and only develops feelings concerning the attitudinal object after making a decision with respect to the object. On the other hand, the high-involvement hierarchy of attitude effect (C → A → B) model is present when an individual is highly invested in a decision making process and values the attitudinal object (Krugman, 1965; Vakratsas and Ambler, 1999; Solomon, 2015; Eisend and Tarrahi, 2016).
Involvement
The concept of involvement is likely to be unfamiliar to many chemistry education researchers and educators since it has its origins in the marketing research of television advertisements, where researchers were interested to discover how the placement of advertisements engaged (involved) the viewing customers (Krugman, 1965; Rothschild, 1978; Vakratsas and Ambler, 1999). Krugman identified low involvement with a low frequency of “bridging experiences” or connections between the product being advertised and the customer. The concept of involvement has also been used in reference to the relationship between a consumer and a product (Clarke and Belk, 1978).
The modern chemistry student shares many characteristics with a typical consumer who is influenced by product placement and marketing (Hemsley-Brown et al., 2009). For example, chemistry students are subjected to marketing by college counselors, college catalogues, and career centers, not to mention the possible subjugation from personal, familial, and societal expectations. Therefore, from the students’ perspective, it is entirely likely and reasonable that a hierarchy of attitude effect would exist toward the subject of chemistry.
There are three antecedent factors of involvement that concern the person, the product, and the circumstance, respectively (Zaichkowsky, 1986). For example, inherent values, individual experiences, cultural norms and expectations can influence the ‘person’ component of involvement. The characteristics and qualities of the product, its tangibility or abstractness, its differentiable qualities or perceived value and utility, are contributors to the ‘product’ component of involvement. The timing and circumstance of the person and product encounter makes up the ‘circumstance’ portion of involvement. For example, a student who only needs a passing grade in a final chemistry course would probably experience the chemistry course differently (lower involvement) than a chemistry major embarking on a path toward graduate level chemistry courses (higher involvement).
Although low involvement toward a product does not necessarily signify a low perception of a product, it is synonymous with low engagement with a product (Lastovicka and Gardner, 1978). Therefore, students’ low involvement toward the subject of chemistry (the product in chemistry education) could be argued as undesirable for effective chemistry learning due to the likelihood of low student engagement. Fortunately, students’ low involvement toward chemistry is fluid and it should be possible to shift it to a high involvement toward chemistry, which could signal that students are engaging more with chemistry (Petty and Cacioppo, 1979).
Student involvement in our chemistry department
Recently, evidence has been presented showing the potential role of involvement to serve as a lens through which to view students’ attitude toward the subject of chemistry (Ross et al., 2018). Therein, the authors provided evidence of students’ low involvement toward the subject of chemistry. Although this work did introduce the hierarchy of attitude effect to explore students’ attitude toward chemistry, it did so in a 5 week intersession chemistry class and with only one cohort of students from one class (introductory CHEM 65 students). Since students’ attitude changes with time exposure to an attitudinal object, the results from a 5 week study do not necessarily extrapolate to a 15 week semester class (Brandriet et al., 2013). For example, it is uncertain whether a low-involvement hierarchy of attitude effect present after 5 weeks would remain unaltered for the additional 10 weeks of a regular 15 week semester; a switch to a high-involvement hierarchy of attitude effect by the end of a 15 week class could occur; this possibility was not explored by Ross et al. (2018). Furthermore, the work by Ross et al. (2018) did not consider extraneous interactions with the tripartite structure of students’ attitudes, such as math prerequisite performance or the influence of attitude on chemistry achievement.
Research looking at factors that influence the learning outcomes of students in predominantly minority student serving institutions is lacking in the chemistry education research literature (Cole and Espinoza, 2008; Basile and Lopez, 2015). East Los Angeles College (ELAC) is the largest Hispanic serving 2 year college in California, with a Fall 2018 enrollment of 41
875 students, which breaks down to an 80.7% Hispanic/LatinX, a 12.0% Asian/Pacific Islander, and a 7.3% other ethnicity population. No other ethnicity out of the remaining 7.3% of students during Fall 2018 exceeded 2.5%, and these proportions are typical at ELAC. Therefore, ELAC contains an ideal student population with whom to conduct chemistry education research towards addressing the aforementioned deficit of such research in predominantly minority student serving institutions. For the reasons indicated above, and to minimize adventitious independent variables, the authors chose the same institution (ELAC) to conduct their follow-up research.
In this follow up study to Ross et al. (2018), students’ involvement with their chemistry course is evaluated toward the end of a regular semester length course, and also expanded to more than one cohort (other than only CHEM 65 students). The authors contend that an investigation of the generality and potential applicability of the results obtained previously (Ross et al., 2018), has merit and has teaching implications for other chemistry departments. The scarcity of structural equation modeling (SEM) analysis in the chemistry education research literature provides an opportunity for studies using SEM to address chemistry education questions, such as students’ involvement, that would be difficult to investigate using other methods (Xu et al., 2013).
Present study
Influencers of chemistry achievement
The role of students’ ability in math and their attitude toward the subject of chemistry on their achievement in chemistry has previously been studied (Bunce and Hutchinson, 1993; Cooper and Pearson, 2012; Xu et al., 2013; Korpershoek et al., 2015). However, to the authors’ knowledge at the time of writing this paper, neither the influence of math on students’ hierarchy of attitude effect toward chemistry nor students’ hierarchy of attitude effect on their chemistry achievement has previously been studied. Therefore, this work seeks answers to as yet unanswered, novel questions, in addition to extending previously asked questions. The following research questions were specifically considered:
(1) Does the low-involvement hierarchy of attitude effect observed in a 5 week intersession introductory chemistry class (CHEM 65) repeat with a new cohort of students, and if so, does it persist for the duration of a regular 15 week semester class?
(2) Does the low-involvement hierarchy of attitude effect exist beyond CHEM 65 students in our department?
(3) How does a hierarchy of attitude effect connect with students’ prerequisite math ability and eventual chemistry achievement?
Methods
Attitudes
As described in Ross et al. (2018), the original 20-item ASCI survey instrument developed by Bauer (2008), rather than the shorter ASCIv2 modified by Xu and Lewis (2011), was used to investigate students’ attitude data and to detect the hierarchy of attitude effect. The ASCI survey is a semantic differential tool that was originally designed to probe 5 subscales of students’ attitude toward the subject of chemistry, namely interest and utility, anxiety, intellectual accessibility, fear, and emotional satisfaction. The ASCI survey instrument was available to participants in an online format since it has been shown to work well either online or when administered as a paper-and-pencil instrument (Brandriet et al., 2011). When completing the original ASCI survey, participants are asked to best match their own opinion on a 7-point scale to the two polar objectives offered in each item statement. The ASCI survey was originally planned to be administered during week 11 of the 15 week Fall 2018 semester at ELAC. However, owing to a lack of participation during week 11, the ASCI survey remained available to voluntary participants between weeks 11 and 15. Whereas attitudes can potentially change during an extended sampling period as used here, inspection of students’ survey responses separately from weeks 11 and 15 reveals no apparent differences. This is consistent with our previously reported findings that students’ attitudes do not change during a 5 week intersession course (Ross et al., 2018).
Measuring chemistry achievement
We sought to connect students’ hierarchy of attitude effect with their eventual chemistry grade. Using students’ final course grade to represent their chemistry achievement has previously been employed (Brandriet et al., 2011). To do so reliably here, we accessed the students’ eventual chemistry grades from the academic records department in our institution. Final course grades consisted of both lecture and laboratory grades: 90–100%, A; 80–89%, B; 70–79%, C; 50–69%, D; <50%, F. The chemistry grades returned were letter grades (A, B, C, D, F, W). These letter grades were converted to GPA scores (A = 4, B = 3, C = 2, D = 1, F = 0) ready for analysis. Students receiving a withdrawal (W) were not considered in the study.
Measuring mathematical ability
In their 2013 paper, Xu et al. demonstrated that the importance of students’ mathematical ability toward their eventual achievement in a chemistry class can be over-inflated if the influence of prior conceptual knowledge in chemistry on their chemistry achievement is not also considered. Owing to the anticipated importance of mathematical ability on students’ chemistry achievement, we obtained students’ prerequisite math grades from our academic records department. Final course grades were based on the same percentages outlined earlier for chemistry course grades and were converted to GPA scores as outlined above for chemistry grades. Students receiving a withdrawal (W) were not considered in the study.
Intermediate algebra (MATH 125) is the common math prerequisite for both CHEM 51 and CHEM 65 courses in our college. CHEM 65 and CHEM 51 can both serve as introductory chemistry classes for students wanting to enter allied health courses or nursing courses. Both of these chemistry courses consist of mandatory lecture and laboratory components and equally satisfy the requirement of a chemistry class with laboratory experience at ELAC. Also, these two classes are representative members of the two unique chemistry tracks available to students in our department. CHEM 65 introduces students to most topics found in a first semester general chemistry course (chemistry major track). CHEM 51 introduces students to topics found in general chemistry, organic chemistry, and biochemistry (allied health and nursing track). Thus, these two classes represent a relatively broad sampling of students within our department and their results should be broadly applicable to our department rather than niche and relevant only to students involved in the study.
Participants
Student participants were invited from all sections of CHEM 65 and CHEM 51 classes in our institution. CHEM 65 students were chosen to address the replication requirements of research question 1. CHEM 51 students were selected to broaden the scope of our earlier study and to extend our probe of students’ involvement in chemistry within our department (research question 2). Students’ math prerequisite grades and eventual chemistry grades were requested for student participants in this study to address research question 3.
The lead professor for each class section was informed in writing about the nature of the research study, its broad goals, and the reasons for requesting student participation. Each lead professor was given a pack of student consent forms that had to be read and signed by participating student volunteers prior to their voluntary completion of the ASCI survey. The student consent form indicated to student volunteers that their participation was being requested and not demanded, and that their identity would be kept confidential in any publications or presentations stemming from the research data collected.
This research project and the student consent forms were approved by the Office of Institutional Effectiveness and Advancement at ELAC prior to the collection of students’ data. Student participants completed the ASCI survey instrument one time only and self-identified in each chemistry class as shown in Table 1: N = 77, 74.0% female and 26.0% male, where 75.3%, 16.9% and 7.8% self-identified as Hispanic/LatinX, Asian/Pacific Islander, and other, respectively. Despite low voluntary student participation (12.1% of all CHEM 51 and 65 students participated), the race composition of the student sample used in this research was representative of the enrolled population in our chemistry department during the Fall 2018 semester (Table 1). Furthermore, the grade distribution of student volunteers in this study was representative of the full cohort of students in the department (Table 2).
Table 1 Demographics of student participants
Demographic |
%a |
%b |
CHEM 51c |
CHEM 65c |
All students enrolled in our chemistry department, Fall 2018, N = 1111.
Student volunteers in this research, N = 77.
Number of students from CHEM 51 and CHEM 65 classes.
Race.
|
Female |
58.1 |
74.0 |
34 |
23 |
Male |
41.9 |
26.0 |
13 |
7 |
Hispanic/LatinX |
71.6 |
75.3 |
35 |
23 |
Asian/Pacific Islander |
21.9 |
16.9 |
8 |
5 |
Otherd |
6.5 |
7.8 |
4 |
2 |
Table 2 Grade distributions of student participants
|
% CHEM 51 |
% CHEM 65 |
Gradea |
This studyb |
All studentsc |
This studyd |
All studentse |
Withdrawals were not considered.
N = 47.
N = 163.
N = 30.
N = 348.
|
A |
34.0 |
32.5 |
16.7 |
21.0 |
B |
31.9 |
28.2 |
20.0 |
24.4 |
C |
27.7 |
25.8 |
23.3 |
25.6 |
D |
6.4 |
4.9 |
26.7 |
10.0 |
F |
0.0 |
8.6 |
13.3 |
19.0 |
Data collection and analysis
Students were provided with a uniform resource locator (URL) through which to access an electronic version of the ASCI survey. Students’ completed survey data was downloaded and pasted into a spreadsheet provided in the supplemental section of the original publication (Bauer, 2008). Structural modeling of students’ attitude data was performed using XLSTAT 2019.1 software as previously described in our earlier work (Ross et al., 2018).
Partial least squares structural equation modeling (PLS-SEM) was used to model students’ attitude data and its connections to math prerequisite grade and eventual chemistry achievement grade. The operation of PLS-SEM along with our reasons for choosing it have previously been discussed and the readership is directed to our earlier publication for further information (Ross et al., 2018). To reiterate briefly here, PLS-SEM is able to return meaningful results from non-parametric data, including data containing single-item constructs, and from relatively small sample sizes, none of which would be possible using CB-SEM. A model's complexity has little influence over the sample size requirements using PLS-SEM due to the operation of the algorithm employed (Hair et al., 2016, p. 22). A power estimate on our sample size of N = 77 shows that it is adequate, and even for a smaller sample size of N = 53 would be adequate to measure R2 values as low as 0.25 at a 1% significance level (Hair et al., 2016, p. 26).
Results
Descriptive statistics
The test-retest consistency of the data retrieved from the ASCI survey instrument from introductory chemistry students in our institution has previously been investigated, obviating the need for doing so again here (Ross et al., 2018). Good distribution normality was indicated in the ASCI data retrieved. Skewness and kurtosis values for all items used in this study were all less than ±1.0 except for items 2 and 6, which were less than ±1.5 (Appendix 1). Average student scores for the ASCI items ranged between 2.935 and 5.987 (1–7 range, 4-midpoint, where score value is proportional to the positivity of the attitude) (Appendix 1).
Data from the pooled CHEM 51 and CHEM 65 students returned average scores for each latent construct that increased from C < Anx < A < B (Anx = anxiety). Average item scores were 3.643 (C), 3.939 (Anx), 4.623 (A), and 5.584 (B). In CHEM 51 and CHEM 65, students on average displayed low-to-moderate anxiety toward chemistry, as evidenced by values close to but below the 4-midpoint value. The approximate average item scores were consistent with our previous findings. Items from the cognition construct averaged below the 4-midpoint value in CHEM 51 and CHEM 65, suggesting that these students find chemistry to be intellectually inaccessible. Items from the affective construct scored above the 4-midpoint value, suggesting that students find chemistry to be emotionally satisfying. Items from the behavioral intention construct scored highest of all and encouragingly suggest that students find chemistry to be interesting and useful (Ross et al., 2018).
Reliability and validity of structural models
Only the low-involvement (model 1) and high-involvement (model 2) hierarchies were explored based on their theoretical likelihoods and our previous work (Beatty and Kahle, 1988; Solomon, 2015; Ross et al., 2018) (Fig. 1). Latent attitude constructs are represented by ovals, where those displaying adjusted R2 values are endogenous constructs. Standardized path coefficient values (β) are shown midpoint along connections between latent constructs (*, p < 0.05; **, p < 0.001). The reliability of the structural models presented here is noted by Cronbach's alpha and composite reliability values greater than 0.70 (Appendix 2) (Sijtsma, 2009; Arjoon et al., 2013; Hair et al., 2016, p. 111; Taber, 2018). The validity of the structural models presented here is evidenced by average variance extracted (AVE) values greater than 0.50 and heterotrait–monotrait (HTMT) values less than ±0.90 (Appendix 3) (Fornell and Larcker, 1981; Hair et al., 2019). Model validity was further supported by positive Q2 values for the endogenous constructs (Appendix 4) (Sharma and Kim, 2012; Sarstedt et al., 2014; Hair et al., 2016, p. 202; Sharma et al., 2019). Math and affect both returned small q2 effect sizes on chemistry achievement (Appendix 4) (Seery, 2009; Xu et al., 2013; Hair et al., 2016, p. 207).
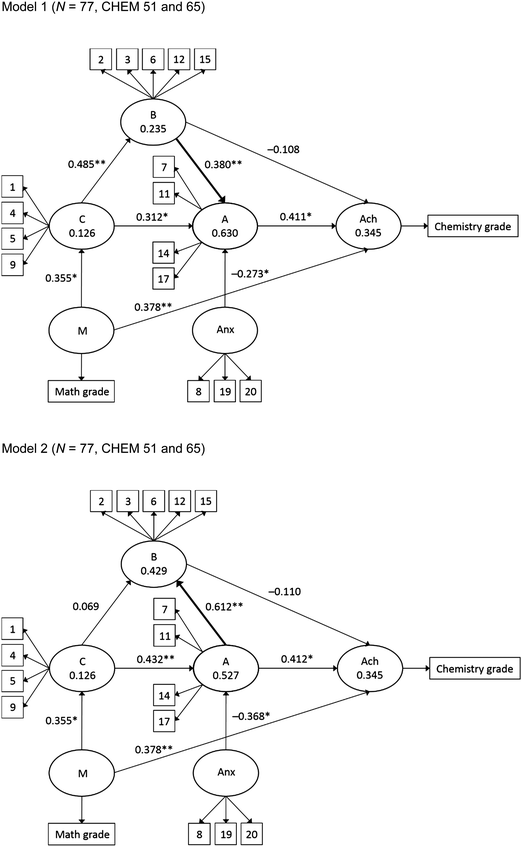 |
| Fig. 1 Structural models from ASCI data, math prerequisite course grade and chemistry final course grade. A, affect; B, behavioral intention; C, cognition; Anx, anxiety; M, math; Ach, chemistry achievement. Numbers in ovals are adjusted R2 values. Numbers on arrows are path coefficient β values. *p < 0.05; **p < 0.001. | |
Interpretation of structural models
Cohen's path analysis.
Cohen's path analysis was performed on models 1 and 2 to decide which model was best supported by the students’ data. Cohen's path analysis argues that a causal direction is supported when it results in a reduction in the total squared errors (TSE) between estimated and actual path coefficients in a structural model (Cohen et al., 1993) as previously described by us (Ross et al., 2018).
Evidence in support of a low-involvement hierarchy of attitude effect in students’ data (low student engagement with chemistry) was found following application of Cohen's path analysis (Cohen et al., 1993; Sun and Zhang, 2006; Wilson et al., 2007; Sattler et al., 2010). Cohen's path analysis returned TSE values of 0.0845 and 0.1565 for low-involvement (C → B → A) and high-involvement (C → A → B) hierarchy models 1 and 2, respectively (Appendix 5). Therefore, reversing the causal path from A → B to B → A resulted in a TSE change of (0.0845–0.1565)/0.1565 = −46.0%. The negative percentage is interpreted as a reduction in TSE, so low-involvement hierarchy model 1 is favored by the data rather than high-involvement hierarchy model 2. This value is very similar to the value of −49.0% we reported previously and speaks of this method's reliability (Ross et al., 2018). Independent analysis of CHEM 51 (N = 111) and CHEM 65 (N = 77) data separately, without any connections to math prerequisite grades or chemistry grades, revealed the presence of a low-involvement hierarchy of attitude effect in both sets of data. This supports our interpretation of models 1 and 2 and argues against over interpretation of the data. Sample sizes were reduced to a combined N = 77 since not all students took their math prerequisite classes at ELAC and therefore could not contribute to models 1 or 2. Collectively, these results answer research question 1 in the affirmative.
The complexity of the models in this study allowed us to calculate the d effect size. Cohen's effect size (d = TSE2 − TSE1/s, where s is the pooled standard deviation for the TSE values) is used to compare the effect of changing the model. The large d effect size calculated (2.84) indicates that model 1 is strongly favored over model 2 by the students’ data (Cohen, 1988). While this result is consistent with our previous findings (Ross et al., 2018), these results are extended beyond only CHEM 65 students to a mixture of CHEM 65 and CHEM 51 students, hence these results provide reliability to our earlier work and generalize the findings, offering broader applicability to our department. These results answer research question 2 in the affirmative.
Low-involvement hierarchy model 1 accounts for approximately 35% of the variance in our students’ overall chemistry course grade by way of influences from mathematics and attitude (adj. R2 (Ach) = 0.345, Table 3). The f2 effect size for the math construct on chemistry achievement (0.190, medium) was greater than the f2 effect size for the affective construct on chemistry achievement (0.128, small). Bootstrapping assessment of the path coefficients in model 1 indicates that all paths are significant at the p < 0.01 level except for the path from B → Ach, which is not significant (Appendix 6) (Kline, 2015, p. 250; Hair et al., 2016, p. 149).
Table 3 In-sample predictive relevance of models 1 and (2)a
Construct |
Adjusted R2 |
f
2
|
Affect |
Behavioral intention |
Cognition |
Chemistry achievement |
N = 77. Larger adjusted R2 values suggests the model has greater in-sample predictive relevance for an endogenous construct (0.75, 0.50 and 0.25 are considered substantial, moderate and weak, respectively). f2 is the effect size of adjusted R2. Values for f2 of 0.02, 0.15 and 0.35 are considered small, medium and large effects, respectively.
Single item construct.
|
Affect |
0.630 (0.527) |
|
(0.361) |
|
0.128 (0.128) |
Behavioral intention |
0.235 (0.429) |
0.292 |
|
|
0.010 (0.010) |
Cognition |
0.126 (0.126) |
0.141 (0.224) |
0.307 (0.005) |
|
|
Anxiety |
|
0.110 (0.163) |
|
|
|
Mathb |
|
|
|
0.144 (0.144) |
0.190 (0.191) |
Chemistry achievementb |
0.345 (0.345) |
|
|
|
|
Discussion
Student involvement with chemistry
Neither model 1 nor model 2 are different in how they represent the 35% explained variance of students’ chemistry achievement. While this is not too surprising since students’ attitudes are thought to be more than mere metrics of their academic ability (Bauer, 2005), it highlights the advantage that measuring students’ involvement (engagement) with chemistry, a precondition of chemistry learning, can provide to indicate students’ learning in class that might be underrepresented when measuring only their chemistry achievement. Students’ active engagement in class has been shown to be proportional to their chemistry achievement (Chan and Bauer, 2016).
Linking student involvement with chemistry to engagement
Low student involvement has been linked to low levels of student engagement (Petty and Cacioppo, 1979). A plausible explanation for such a low-involvement hierarchy is due to students harboring an a priori emotional prejudice toward the subject of chemistry, which has not yet been challenged during their limited experience in entry-level chemistry class (Ajzen, 1985). Were any students in our study to exhibit the supposedly desirable high-involvement hierarchy effect, suggesting a high level of student engagement and its links to greater chemistry achievement (Chan and Bauer, 2016), it would imply that these students have an emotional attachment to the subject of chemistry, perhaps cultivated by former experiences in chemistry classes (Ajzen, 1985). Interestingly, our students’ ASCI scores indicate their emotional satisfaction with chemistry as evidenced by midpoint values above 4. It is surprising that students’ emotional satisfaction with chemistry is apparently independent of their emotional attachment to chemistry, which is low as evidenced by their low involvement (vide supra). It is possibly naïve to expect introductory chemistry students to establish an emotional attachment to the subject of chemistry, yet if actualized it could facilitate their chemistry learning, and therefore is a goal worthy of targeting by chemistry teaching practice.
The non-differentiating substitutability of attitudinal objects has been linked with low involvement (Korgaonkar and Moschis, 1982). CHEM 65 and CHEM 51 possess non-differentiating substitutability since either course could serve as a gateway course for students wanting to pursue vocational courses. Thus, our students’ low-involvement hierarchy of attitude effect toward CHEM 65 and CHEM 51 could stem from their awareness and correct interpretation that neither CHEM 65 nor CHEM 51 are terminal chemistry courses but are instead substitutable gateway chemistry courses to further study. Alternatively, perhaps students’ low involvement with CHEM 65 and CHEM 51 is due to their lack of engagement with chemistry at this introductory and non-committal level.
Comparison of the influence of affect on chemistry achievement, and vice versa obtained here, with literature reports is complicated by the nuanced results reported therein. For example, math rather than attitude has been shown to greater influence chemistry achievement (Xu et al., 2013), whereas math SAT scores have demonstrated redundancy toward students’ affective profiling (Chan and Bauer, 2014). Since the connection between the affective construct and chemistry achievement in model 1 is channeling the entire hierarchy of attitude effect (other possible connections were less favorable), this result implies that math is more important than attitude to our students’ chemistry achievement, although their attitude is also significantly important. However, it is possible that model 1 could exhibit the same over-inflated influence of math ability on chemistry achievement found by others (Seery, 2009; Xu et al., 2013).
Emotional satisfaction and emotional attachment with chemistry
Others have identified how students’ attitude positively correlates with their chemistry achievement (Cukrowska et al., 1999; Bauer, 2008; Xu et al., 2013). Our work suggests that these positive correlations are likely hiding involvement effects, which if low versus high, could be negatively impacting students’ chemistry learning if not also their chemistry achievement (e.g., emotional attachment influence).
Differences between hierarchies of attitude effects are linked to students’ perceived benefits of the attitude object (Korgaonkar and Moschis, 1982). Students who view the attitude object ‘chemistry’ as being useful to their future career plans would regard chemistry as an extrinsic benefit to their career goals, and this should be captured by their cognitive construct. In contrast, students who have previously experienced the attitude object ‘chemistry’, and with whom chemistry has previously created an emotional attachment, would additionally regard chemistry as an intrinsic benefit to their career goals, and this should be captured by their affective construct (Park and Yoo, 2018). Based on evidence presented herein, CHEM 51 and CHEM 65 students could be exhibiting low involvement with chemistry due to the reasonable assertion that students are unlikely to show an emotional attachment to the subject of chemistry. However, it must be remembered that students did show evidence of their emotional satisfaction with the subject of chemistry (ASCI scores above the 4-midpoint value). Alternatively, students’ disengagement from chemistry could originate from their lack of the necessary familiarity with the subject to develop any emotional connection to chemistry.
Altering student involvement with chemistry
Fortunately, there is a favored directionality to change of involvement. Students’ low involvement is likely more susceptible to change to high involvement compared with the reverse change from a high to low involvement (Swinyard and Coney, 1978). Ways in which students’ low involvement might be flipped to high involvement and hence greater engagement with chemistry learning might include switching from a traditional lecture-based class to a more interactive class setup, or switching from traditional physical textbooks to more interactive electronic textbooks that include interactive simulations and videos embedded within them.
There is justification for pursuing ways to increase students’ emotional attachment to chemistry. Ways to pursue students’ increased emotional attachment to chemistry could include increasing students’ self-expression during chemistry (Vlachos et al., 2010). A practical example of this could be to afford students the opportunity to select and pursue a relevant topic in chemistry that they care about and to which they are capable of becoming emotionally attached. Chemistry topics that impact the environment and human health are likely to be favored by students and are congruent with augmenting their self-expression in chemistry.
Cognitive and affective determinants of emotional attachment that could be of interest in chemistry education include self-congruence, responsiveness, quality, reputation and trust (Japutra et al., 2014). To support students’ emotional attachment, chemistry educators could offer timely feedback regarding student progress and provide ungraded formative assessments to foster students’ self-congruence and their psychological needs of autonomy and competence (Thomson, 2006).
The subtle differences between students’ emotional satisfaction and their emotional attachment reported in other disciplines, when applied to our chemistry attitude data, suggests that our students’ low involvement is probably being directed toward the subject of chemistry, whereas their lack of emotional attachment is arguably regarding their chemistry achievement rather than the subject of chemistry (Ball and Tasaki, 1992).
Conclusions
Implications for chemistry teaching
Model 1 should have broad applicability to our chemistry department since it represents the two separate chemistry tracks offered at ELAC. Therefore, model 1 should have more value for our chemistry department in terms of planning and improving learning outcomes for students than it would had it only incorporated one cohort of students as was the case in our original study (Ross et al., 2018).
The work presented herein has implications and potential benefits to other chemistry departments. Model 1 provides an example of a roadmap by which broadly applicable and nuanced hierarchy of attitude effect data can be retrieved for any cohort of students, including predominantly minority student serving institutions.
Simply noting, as other studies have done, that positive student attitudes correlate with chemistry achievement considers only the product of chemistry achievement and ignores the process of learning chemistry, and overlooks the extra component of student involvement with chemistry. Herein, both low-involvement and high-involvement interpretations of our students’ data return positive correlations between attitudes and chemistry achievement and account for the same explained variance in achievement (Fig. 1), yet the students are actually displaying a low engagement with their learning (Appendix 5). Thus it is possible that only monitoring the correlation between students’ attitude and their chemistry achievement could lead to an incorrect conclusion about chemistry learning, or at least an unnecessarily incomplete conclusion about chemistry learning.
While authors of affective domain research often caution their readers about the granularity of their results, which likely pertain only to specific students within specific institutions, and are less broadly applicable to others, the results presented herein demonstrate the potential for the broad applicability of attitude data to an entire chemistry department. The ASCI instrument, rather than the shortened ASCIv2 instrument, is suited to researchers questioning their students’ involvement in chemistry courses in their departments.
Whereas others have highlighted the opportunity to refine structural models in order to increase explained variance, we consider the explained variance reported here as an insight into chemistry learning occurring at a point in time for a given cohort of students, and suggest that the gradient between two temporally distinct explained variance measurements is more useful than the absolute value of an explained variance measurement (Xu et al., 2013; Boddey and de Berg, 2018). Therefore, we believe that the benefit of measuring explained variance in chemistry achievement lies in its application as a tool for others to monitor engagement and learning in their institutions rather than as an absolute value to compare with other student cohorts.
Whereas low involvement from students toward chemistry does not necessarily translate to students’ low valuation of their chemistry education, it does point to students’ low level of engagement with their chemistry education (Petty and Cacioppo, 1979). Thus, as part of their effective practice, chemistry educators would likely be interested in measuring their students’ involvement level toward chemistry, monitoring the gradient of its change over time, and its response to changes in curriculum and pedagogy.
Efforts to positively influence students’ attitude toward chemistry continues to be an active area of chemistry education research and practice (Abdullah et al., 2009; Koponen et al., 2012; Vishnumolakala et al., 2017). Chemistry educators could incorporate metacognitive conversations with their students about the influence of involvement hierarchy on their achievement in chemistry. This would be a valuable component of chemistry education practice if framed perhaps as a summer bridge program, a prerequisite, or as a corequisite addition to a chemistry course (Cook et al., 2013).
Limitations of study
This research and the conclusions drawn have numerous limitations that should be made transparent. The results and conclusions made in this research were from students’ self-assessment attitude data taken during one semester from one survey instrument in one institution, and from less than 100% of available students (not all students volunteered their data and time). That being said, the student sample used in this research is reasonably representative of the enrolled population in our chemistry department during the Fall 2018 semester (Table 1).
In situations where the attitudinal object is not physically present and self-assessment surveys are used to garner attitudinal data, correlations between the components of attitude can be overestimated (Greenwald, 1982; Breckler, 1984). This points to the choice of measuring device featuring as a variable in the results obtained; although this point is arguably inescapable, and we feel benign in this case, it should be acknowledged.
The work performed here and its findings stemmed from analyses by PLS-SEM, for reasons already discussed. Therefore, by not performing modeling analyses using covariance-based structural equation modeling (CB-SEM), we forwent the opportunity to measure the fit of the proposed structural models. While we encourage other researchers with the happenstance and good fortune of sufficiently large student numbers to perform hierarchy of attitude effect studies using the covariance-based modeling approach, we believe that this research approach appeals most to other large institutions and serves less the more abundant institutions lacking lecture halls containing hundreds of students from whom to acquire chemistry education research data. The broad readership of this journal should benefit from an equally broad approach to attitude research as it relates to chemistry education research, and we feel that our methodology complements the more common approach taken by researchers who use CB-SEM.
The department in which this research was conducted serves predominantly Hispanic students, and so the results obtained herein are most likely to generalize to other chemistry departments with a similar student constituency. Furthermore, we assumed that all students were encountering chemistry with a comparable prior attitude bias. Out of the 77 student samples, 6 were from students repeating their class. Removal of these 6 data sets did not significantly alter the results of the analyses so they were included in the values reported herein. We did not account for students’ prior exposure to high school chemistry (Tai et al., 2005).
Notwithstanding the limitations highlighted here, and those not apparent to the authors at the time of writing, we assert that this work will be a valuable contribution to current efforts in chemistry education research, which exists to advance the practice of chemistry education and subsequently the learning of chemistry students. The capacity to measure students’ involvement with their chemistry education and assess their responses to curriculum and pedagogy changes is clearly a desirable and necessary goal in chemistry education. The measurement of a hierarchy of attitude effect with PLS-SEM and its link to chemistry achievement can contribute towards achieving this goal, and thus provides an added lens through which chemistry educators can guide authentic and meaningful chemistry learning.
Conflicts of interest
There are no conflicts to declare.
Appendices
Appendix 1
Table 4 Descriptive statistics of select ASCI data and grade data used in model analysis (N = 77)
Construct |
Item number |
Item |
Mean |
SD |
Skew |
Kurt |
Item reversed here to aid interpretation; negatively stated item is reversed before averaging.
0–4 score range.
|
Cognition |
1a |
Easy |
Hard |
2.935 |
1.471 |
0.638 |
0.071 |
4 |
Complicated |
Simple |
3.169 |
1.524 |
0.450 |
−0.382 |
5 |
Confusing |
Clear |
3.714 |
1.485 |
−0.076 |
−0.384 |
9a |
Comprehensible |
Incomprehensible |
4.753 |
1.369 |
−0.191 |
−0.281 |
Behavioral intention |
2 |
Worthless |
Beneficial |
5.987 |
1.145 |
−1.244 |
1.310 |
3a |
Exciting |
Boring |
4.844 |
1.546 |
−0.357 |
−0.471 |
6a |
Good |
Bad |
5.805 |
1.405 |
−1.048 |
0.410 |
12a |
Interesting |
Dull |
5.740 |
1.232 |
−0.848 |
0.483 |
15a |
Worthwhile |
Useless |
5.545 |
1.363 |
−0.976 |
0.875 |
Affect |
7a |
Satisfying |
Frustrating |
4.429 |
1.855 |
−0.203 |
−0.918 |
11a |
Pleasant |
Unpleasant |
4.688 |
1.379 |
−0.114 |
−0.166 |
14a |
Comfortable |
Uncomfortable |
4.805 |
1.442 |
−0.260 |
−0.283 |
17 |
Chaotic |
Organized |
4.571 |
1.798 |
−0.303 |
−0.814 |
Anxiety |
8a |
Scary |
Fun |
3.481 |
1.600 |
0.434 |
−0.222 |
19a |
Tense |
Relaxed |
4.935 |
1.622 |
−0.489 |
−0.549 |
20a |
Insecure |
Secure |
3.403 |
1.341 |
0.114 |
0.243 |
Mathb |
|
|
|
2.532 |
1.252 |
−0.619 |
−0.394 |
Chemistry achievementb |
|
|
|
2.571 |
1.178 |
−0.418 |
−0.729 |
Appendix 2
Table 5 Internal consistency reliability: composite reliability (ρ) and Cronbach's alpha (α) for models 1 and 2a
Latent construct |
Internal consistency reliabilityb |
Composite reliability (ρ) |
Cronbach's alpha (α) |
N = 77.
Values greater than 0.70 are generally considered to indicate construct reliability.
|
Affect |
0.853 |
0.762 |
Behavioral intention |
0.850 |
0.777 |
Cognition |
0.858 |
0.773 |
Anxiety |
0.841 |
0.708 |
Appendix 3
Table 6 Discriminant validity: Fornell–Larcker criteriona and (HTMT)b for models 1 and 2c
|
Affect |
Behavioral intention |
Cognition |
Anxiety |
AVE |
Fornell–Larcker criterion indicates discriminant validity when AVE value for each construct is greater than cross-correlations squared with other constructs.
Heterotrait–monotrait ratio (HTMT) indicates discriminant validity when less than ±0.90.
N = 77.
|
Affect |
1.000 |
|
|
|
0.583 |
Behavioral intention |
0.430 (0.815) |
1.000 |
|
|
0.534 |
Cognition |
0.458 (0.811) |
0.234 (0.546) |
1.000 |
|
0.590 |
Anxiety |
0.429 (−0.863) |
0.214 (−0.579) |
0.439 (−0.863) |
1.000 |
0.631 |
Appendix 4
Table 7 Out-of-sample predictive relevance of structural model 1a
Latent construct |
Q
2
|
q
2
|
Affect |
Behavioral intention |
Cognition |
Chemistry achievement |
N = 77. Q2 > 0 indicates that a model has out-of-sample predictive relevance for an endogenous construct; Q2 < 0 indicates a lack of out-of-sample predictive relevance for an endogenous construct. q2 is the effect size of Q2. Values for q2 of 0.02, 0.15 and 0.35 are considered to be small, medium and large effects, respectively.
|
Affect |
0.373 |
|
−0.001 |
−0.000 |
0.060 |
Behavioral intention |
0.125 |
0.053 |
|
−0.001 |
0.007 |
Cognition |
0.074 |
0.022 |
|
|
−0.002 |
Anxiety |
|
0.019 |
0.000 |
0.000 |
0.000 |
Math |
|
−0.001 |
−0.002 |
|
0.084 |
Chemistry achievement |
0.362 |
−0.001 |
0.000 |
0.000 |
|
Appendix 5
Table 8 Cohen's path analysis of models 1 and 2a
|
Direct path |
Indirect path |
Estimated |
Actual |
SE |
N = 77.
Model 1 represents the low-involvement (C → B → A) hierarchy. Model 2 represents the high-involvement (C → A → B) hierarchy. A, affect; B, behavioral intention; C, cognition; Anx, anxiety; M, math; Ach, chemistry achievement.
Total squared error is minimized in a superior model.
|
Path, model 1b |
Anx → Ach |
|
Anx → A → Ach |
−0.1122 |
−0.2613 |
0.0222 |
M → Ach |
M → Ach |
M → C → B → Ach; M → C → A → Ach; M → C → B → A → Ach |
0.4318 |
0.5060 |
0.0055 |
C → Ach |
|
C → B → A → Ach; C → B → Ach; C → A → Ach |
0.1515 |
0.3410 |
0.0359 |
B → Ach |
B → Ach |
B → A → Ach |
0.0482 |
0.1831 |
0.0182 |
A → Ach |
A → Ach |
|
0.4111 |
0.4635 |
0.0027 |
|
|
|
|
|
0.0845 (TSE)
|
|
Path, model 2b |
Anx → Ach |
|
Anx → A → Ach |
−0.1516 |
−0.2613 |
0.0120 |
M → Ach |
M → Ach |
M → C → B → Ach; M → C → A → Ach |
0.4385 |
0.5060 |
0.0046 |
C → Ach |
|
C → B → Ach; C → A → Ach; C → A → B → Ach |
0.1413 |
0.3410 |
0.0399 |
B → Ach |
B → Ach |
|
−0.1100 |
0.1831 |
0.0859 |
A → Ach |
A → Ach |
A → B → Ach |
0.3447 |
0.4635 |
0.0141 |
|
|
|
|
|
0.1565 (TSE)
|
Appendix 6
Table 9 Standardized path coefficient values (β) and their significance for models 1 and 2a
Model |
Path |
β
|
95% CI |
t-Value |
p-Value |
N = 77. Critical t-values for a two-tailed test: <1.96 (p > 0.05), 1.96 (p = 0.05), >2.58 (p < 0.001). Paths with a 95% confidence interval (CI) including zero should be rejected even with p < 0.05. A, affect; B, behavioral intention; C, cognition; Anx, anxiety; M, math; Ach, chemistry achievement.
|
1 |
C → A |
0.312 |
[0.129; 0.490] |
3.204 |
0.002 |
C → B |
0.485 |
[0.317; 0.635] |
4.797 |
0.000 |
B → A |
0.380 |
[0.218; 0.540] |
4.613 |
0.000 |
B → Ach |
−0.108 |
[−0.368; 0.116] |
−0.851 |
0.397 |
Anx → A |
−0.273 |
[−0.432; −0.112] |
−2.836 |
0.006 |
A → Ach |
0.411 |
[0.130; 0.694] |
3.059 |
0.003 |
M → C |
0.355 |
[0.118; 0.549] |
3.286 |
0.002 |
M → Ach |
0.378 |
[0.185; 0.576] |
3.720 |
0.000 |
|
2 |
C → A |
0.432 |
[0.224; 0.628] |
4.073 |
0.000 |
C → B |
0.069 |
[−0.168; 0.297] |
0.581 |
0.563 |
A → B |
0.612 |
[0.406; 0.829] |
5.168 |
0.000 |
B → Ach |
−0.110 |
[−0.379; 0.125] |
−0.861 |
0.392 |
Anx → A |
−0.368 |
[−0.565; −0.167] |
−3.472 |
0.001 |
A → Ach |
0.412 |
[0.137; 0.700] |
3.063 |
0.003 |
M → C |
0.355 |
[0.130; 0.546] |
3.285 |
0.002 |
M → Ach |
0.378 |
[0.186; 0.572] |
3.731 |
0.000 |
Acknowledgements
We gratefully acknowledge the numerous chemistry professors and students who volunteered their time and data in support of this research.
References
- Abdullah M., Mohamed N. and Ismail Z. H., (2009), The effect of an individualized laboratory approach through microscale chemistry experimentation on students’ understanding of chemistry concepts, motivation, and attitudes, Chem. Educ. Res. Pract., 10, 53–61.
- Aiken L. R., (1980), Attitude measurement and research, New Dir. Test. Meas., 7, 1–24.
- Ajzen I., (1985), From intentions to actions: a theory of planned behavior, in Kuhl J. and Beckmann J., (ed.), Action-control: from cognition to behavior, Heidelberg: Springer, pp. 11–39.
- Arambewela R., Hall J. and Zuhair S., (2005), Postgraduate international students from Asia: factors influencing satisfaction, J. Market. High. Educ., 15(2), 105–127.
- Arjoon J. A., Xu X. and Lewis J. E., (2013), Understanding the state of the art for measurement in chemistry education research: examining the psychometric evidence, J. Chem. Educ., 90(5), 536–545.
- Ball A. D. and Tasaki L. H., (1992), The role and measurement of attachment in consumer behavior, J. Consum. Psychol., 1(2), 155–172.
- Barbera J., Adams W. K., Wieman C. E. and Perkins K. K., (2008), Modifying and validating the Colorado Learning Attitudes about Science Survey for use in chemistry, J. Chem. Educ., 85(10), 1435–1439.
- Basile V. and Lopez E., (2015), And still I see no changes: enduring views of students of color in science and mathematics education policy reports, Sci. Educ., 99(3), 519–548.
- Bauer C. F., (2005), Beyond “student attitudes”: chemistry self-concept inventory for assessment of the affective component of student learning, J. Chem. Educ., 82(12), 1864–1870.
- Bauer C. F., (2008), Attitude towards chemistry: a semantic differential instrument for assessing curriculum impacts, J. Chem. Educ., 85(10), 1440–1445.
- Beatty S. E. and Kahle L. R., (1988), Alternative hierarchies of the attitude-behavior relationship: the impact of brand commitment and habit, J. Acad. Market. Sci., 16(2), 1–10.
- Berg C. A. R., (2005), Factors related to observed attitude change toward learning chemistry among university students, Chem. Educ. Res. Pract., 6(1), 1–18.
- Boddey K. and de Berg K., (2018), A framework for understanding student nurses’ experience of chemistry as part of a health science course, Chem. Educ. Res. Pract., 19, 597–616.
- Brandriet A. R., Xu X., Bretz S. L. and Lewis J. E., (2011), Diagnosing changes in attitude in first-year college chemistry students with a shortened version of Bauer's semantic differential, Chem. Educ. Res. Pract., 12, 271–278.
- Brandriet A. R., Ward R. M. and Bretz S. L., (2013), Modeling meaningful learning in chemistry using structural equation modeling, Chem. Educ. Res. Pract., 14, 421–430.
- Breckler S. J., (1984), Empirical validation of effect, behavior, and cognition as distinct components of attitude, J. Pers. Soc. Psychol., 47(6), 1191–1205.
- Bunce D. M. and Hutchinson K. D., (1993), The use of the GALT (group assessment of logical thinking) as a predictor of academic success in college chemistry, J. Chem. Educ., 70(3), 183–187.
- Chan J. Y. K. and Bauer C. F., (2014), Identifying at-risk students in general chemistry via cluster analysis of affective characteristics, J. Chem. Educ., 91(9), 1417–1425.
- Chan J. Y. K. and Bauer C. F., (2016), Learning and studying strategies used by general chemistry students with different affective characteristics, Chem. Educ. Res. Pract., 17, 675–684.
- Clarke K. and Belk R., (1978), The effects of product involvement and task definition on anticipated consumer effort, in Hunt H. K., (ed.), Advances in consumer research, Ann Arbor: Association for Consumer Research, pp. 313–318.
- Cohen J., (1988), Statistical power analysis for the behavioral sciences, Hillsdale, NJ: Lawrence Erlbaum.
- Cohen P. R., Carlsson A., Ballesteros L. and Amant R. S., (1993), Automating path analysis for building causal models from data: first results and open problems, Eleventh National Conference on Artificial Intelligence, Washington, DC.
- Cole D. and Espinoza A., (2008), Examining the academic success of Latino students in science technology engineering and mathematics (STEM) majors, J. Coll. Stud. Dev., 49(4), 285–300.
- Cook E., Kennedy E. and McGuire S. Y., (2013), Effect of teaching metacognitive learning strategies on performance in general chemistry courses, J. Chem. Educ., 90(8), 961–967.
- Cooper C. and Pearson P., (2012), A genetically optimized predictive system for success in general chemistry using a diagnostic algebra test, J. Sci. Educ. Technol., 21(1), 197–205.
- Cukrowska E., Staskun M. G. and Schoeman H. S., (1999), Attitudes towards chemistry and their relationship to student achievement in introductory chemistry courses, South Afr. J. Chem.-Suid-Afr. Tydskr. Chem., 52(1), 8–14.
- Eagly A. H. and Chaiken S., (1993), The psychology of attitudes, Fort Worth, TX: Harcourt Brace Jovanovich.
- Eisend M. and Tarrahi F., (2016), The effectiveness of advertising: a meta-meta-analysis of advertising inputs and outcomes, J. Advert., 45(4), 519–531.
- Else-Quest N. M., Mineo C. C. and Higgins A., (2013), Math and science attitudes and achievement at the intersection of gender and ethnicity, Psychol. Women. Q., 37(3), 293–309.
- Ewing M., Huff K., Andrews M. and King K., (2005), Assessing the reliability of skills measured by the SAT® (Office of Research and Analysis, Trans.), The College Board.
- Ferrell B., Phillips M. M. and Barbera J., (2016), Connecting achievement motivation to performance in general chemistry, Chem. Educ. Res. Pract., 17, 1054–1066.
- Fornell C. and Larcker D. F., (1981), Evaluating structural equation models with unobservable variables and measurement error, J. Mark. Res., 18(1), 39–50.
- Freeman S., Eddy S. L., McDonough M., Smith M. K., Okoroafor N., Jordt H. and Wenderoth M. P., (2014), Active learning increases student performance in science, engineering, and mathematics, Proc. Natl. Acad. Sci. U. S. A., 111(23), 8410–8415.
- Greenwald A. G., (1982), Is anyone in charge? Personalysis versus the principle of personal unity, in Suis J., (ed.), Psychological perspectives on the self, Hillsdale, NJ: Erlbaum, vol. 1, pp. 151–181.
- Hair J. F., Hult G. T. M., Ringle C. M. and Sarstedt M., (2016), A primer on partial least squares structural equation modeling (PLS-SEM), 2nd edn, Los Angeles: Sage Publications, Inc.
- Hair J. F., Risher J. J., Sarstedt M. and Ringle C. M., (2019), When to use and how to report the results of PLS-SEM, Eur. Bus. Rev., 31(1), 2–24.
- Hemsley-Brown J., Yaakop A. and Gilbert D., (2009), Hierarchy-of-effects (HOE) models and higher education, in Maringe F. and Guedes M. G., (ed.), Global Competitiveness in Higher Education: New Marketing Challenges for Research and Practice – Proceedings of Fourth International Conference on Higher Education Marketing, Guimarães, Portugal: Universidade do Minho, pp. 241–255.
- Japutra A., Ekinci Y. and Simkin L., (2014), Exploring brand attachment, its determinants and outcomes, J. Strat. Market., 22(7), 616–630.
- Kahveci A., (2015), Assessing high school students’ attitudes toward chemistry with a shortened semantic differential, Chem. Educ. Res. Pract., 16, 283–292.
- Kline R. B., (2015), Principles and practice of structural equation modeling, 4th edn, New York: The Guilford Press.
- Koponen J., Pyörälä E. and Isotalus P., (2012), Comparing three experiential learning methods and their effect on medical students’ attitudes to learning communication skills, Med. Teach., 34, e198–e207.
- Korgaonkar P. K. and Moschis G. P., (1982), An experimental study of cognitive dissonance, product involvement, expectations, performance and consumer judgment of product performance, J. Advert., 11, 32–44.
- Korpershoek H., Kuyper H. and Van der Werf G., (2015), The relation between students’ math and reading ability and their mathematics, physics, and chemistry examination grades in secondary education, Int. J. Sci. Math. Educ., 13, 1013–1037.
- Krugman H. E., (1965), The impact of television advertising: learning without involvement, Public Opin. Q., 29, 349–356.
- Lastovicka J. L. and Gardner D. M., (1978), Components of involvement, in Maloney J. C. and Silverman B., (ed.), Attitude research plays for high stakes, Chicago: American Marketing Association, pp. 53–73.
- Lauer L. D., (2008), Advertising can be an effective integrated marketing tool, J. Market. High. Educ., 17(1), 13–15.
- Lewis S. E. and Lewis J. E., (2007), Predicting at-risk students in general chemistry: comparing formal thought to a general achievement measure, Chem. Educ. Res. Pract., 8(1), 32–51.
- Lewis S. E., Shaw J. L., Heitz J. O. and Webster G. H., (2009), Attitude counts: self-concept and success in general chemistry, J. Chem. Educ., 86(6), 744–749.
- Maringe F., (2005), University marketing: perceptions, practices and prospects in the less developed world, J. Market. High. Educ., 15(2), 129–153.
- Montes L. H., Ferreira R. A. and Rodriguez C., (2018), Explaining secondary school students’ attitudes towards chemistry in Chile, Chem. Educ. Res. Pract., 19, 533–542.
- Mooring S. R., Mitchell C. E. and Burrows N. L., (2016), Evaluation of a flipped, large-enrollment organic chemistry course on student attitude and achievement, J. Chem. Educ., 93, 1972–1983.
- Murphy K., Holme T., Zenisky A., Caruthers H. and Knaus K., (2012), Building the ACS exams anchoring concept content map for undergraduate chemistry, J. Chem. Educ., 89, 715–720.
- Osborne J., Simon S. and Collins S., (2003), Attitudes towards science: a review of the literature and its implications, Int. J. Sci. Educ., 25(9), 1049–1079.
- Park M. and Yoo J., (2018), Benefits of mass customized products: moderating role of product involvement and fashion innovativeness, Heliyon, 4(2), DOI:10.1016/j.heliyon.2018.e00537.
- Petty R. E. and Cacioppo J. T., (1979), Issue involvement can increase or decrease persuasion by enhancing message-relevant cognitive responses, J. Pers. Soc. Psychol., 37(10), 1915–1926.
- Richter-Egger D. L., Hagen J. P., Laquer F. C., Grandgenett N. F. and Shuster R. D., (2010), Improving student attitudes about science by integrating research into the introductory chemistry laboratory: interdisciplinary drinking water analysis, J. Chem. Educ., 87(8), 862–868.
- Rosenberg M. J. and Hovland C. I., (1960), in Hovland C. I. and Rosenberg M. J. (ed.), Attitude organization and change: an analysis of consistency among attitude components, New Haven, CT: Yale University Press.
- Ross J., Nuñez L. and Lai C. C., (2018), Partial least squares structural equation modeling of chemistry attitude in introductory college chemistry, Chem. Educ. Res. Pract., 19, 1270–1286.
- Rothschild M. L., (1978), Advertising strategies for high and low involvement situations, in Maloney J. C. and Silverman B., (ed.), Attitude research plays for high stakes, Chicago: American Marketing Association, pp. 53–73.
- Ryan M. D. and Reid S. A., (2016), Impact of the flipped classroom on student performance and retention: a parallel controlled study in general chemistry, J. Chem. Educ., 93, 13–23.
- Sarstedt M., Ringle C. M., Henseler J. and Hair J. F., (2014), On the emancipation of PLS-SEM: a commentary on Rigdon (2012), Long Range Plann., 47(3), 154–160.
- Sattler H., Völckner F., Riediger C. and Ringle C. M., (2010), The impact of brand extension success drivers on brand extension price premiums, Int. J. Res. Mark., 27, 319–328.
- Scott F. J., (2012), Is mathematics to blame? An investigation into high school students’ difficulty in performing calculations in chemistry, Chem. Educ. Res. Pract., 13(3), 330–336.
- Seery M. K., (2009), The role of prior knowledge and student aptitude in undergraduate performance in chemistry: a correlation-prediction study, Chem. Educ. Res. Pract., 10, 227–232.
- Sharma P. N., and Kim K. H., (2012), Model selection in information systems research using partial least squares based structural equation modeling, Proceedings of the international conference on information systems, Orlando, Florida.
- Sharma P. N., Sarstedt M., Shmueli G., Kim K. H. and Thiele K. O., (2019), PLS-based model selection: the role of alternative explanations in information systems research, J. Assoc. Inf. Syst., 20(4), 346–397.
- Sijtsma K., (2009), On the use, the misuse, and the very limited usefulness of Cronbach's alpha, Psychometrika, 74(1), 107–120.
- Solomon M., (2015), Consumer behavior: buying, having and being, 11th edn, Upper Saddle River, NJ: Pearson Prentice Hall.
- Spencer H. E., (1996), Mathematical SAT test scores and college chemistry grades, J. Chem. Educ., 73(12), 1150–1153.
- Sun H. and Zhang P., (2006), Causal relationships between perceived enjoyment and perceived enjoyment and perceived ease of use: an alternative approach, J. Assoc. Inf. Syst., 7(9), 618–645.
- Swinyard W. R. and Coney K. A., (1978), Promotional effects on a high versus low-involvement electorate, J. Consum. Res., 5, 41–48.
- Taber K. S., (2018), The use of Cronbach's alpha when developing and reporting research instruments in science education, Res. Sci. Educ., 48, 1273–1296.
- Tai R. H., Sadler P. M. and Loehr J. F., (2005), Factors influencing success in introductory college chemistry, J. Res. Sci. Teach., 42(9), 987–1012.
- Thomson M., (2006), Human brands: investigating antecedents to consumers’ strong attachments to celebrities, J. Market., 70, 104–119.
- Uzuntiryaki E. and Aydin Y. C., (2009), Development and validation of chemistry self-efficacy scale for college students, Res. Sci. Educ., 39(4), 539–551.
- Vakratsas D. and Ambler T., (1999), How advertising works: what do we really know? J. Market., 63, 26–43.
- Villafañe S. M. and Lewis J. E., (2016), Exploring a measure of science attitude for different groups of students enrolled in introductory college chemistry, Chem. Educ. Res. Pract., 17, 731–742.
- Vishnumolakala V. R., Southam D. C., Treagust D. F., Mocerino M. and Qureshi S., (2017), Students’ attitudes, self-efficacy and experiences in a modified process-oriented guided inquiry learning undergraduate chemistry classroom, Chem. Educ. Res. Pract., 18, 340–352.
- Vlachos P. A., Theotokis A., Pramatari K. and Vrechopoulos A., (2010), Consumer-retailer emotional attachment: some antecedents and the moderating role of attachment anxiety, Eur. J. Market., 44(9), 1478–1499.
- Wagner R. V. and Sherwood J. J., (1969), The study of attitude change, Brooks/Cole Publishing Company.
- Wagner E. P., Sasser H. and DiBiase W. J., (2002), Predicting students at risk in general chemistry using pre-semester assessments and demographic information, J. Chem. Educ., 79(6), 749–755.
- Wilson B., Callaghan W., Ringle C. M. and Henseler J., (2007), Exploring causal path directionality for a marketing model using Cohen's path method, in PLS'07: 5th international symposium on PLS and related methods, Aas, Norway, pp. 57–61.
- Xu X. and Lewis J. E., (2011), Refinement of a chemistry attitude measure for college students, J. Chem. Educ., 19(5), 561–568.
- Xu X., Villafane S. M. and Lewis J. E., (2013), College students’ attitudes toward chemistry, conceptual knowledge and achievement: structural equation model analysis, Chem. Educ. Res. Pract., 14, 188–200.
- Zaichkowsky J. L., (1985), Measuring the involvement construct in marketing, J. Consum. Res., 12(3), 341–352.
- Zaichkowsky J. L., (1986), Conceptualizing involvement, J. Advert., 15(2), 4–14.
|
This journal is © The Royal Society of Chemistry 2020 |
Click here to see how this site uses Cookies. View our privacy policy here.