DOI:
10.1039/D0RA06379G
(Paper)
RSC Adv., 2020,
10, 37707-37720
Ilimaquinone (marine sponge metabolite) as a novel inhibitor of SARS-CoV-2 key target proteins in comparison with suggested COVID-19 drugs: designing, docking and molecular dynamics simulation study
Received
22nd July 2020
, Accepted 4th October 2020
First published on 13th October 2020
Abstract
The outbreak of novel coronavirus, SARS-CoV-2, has infected more than 36 million people and caused approximately 1 million deaths around the globe as of 9 October 2020. The escalating outspread of the virus and rapid rise in the number of cases require the instantaneous development of effectual drugs and vaccines. Presently, there are no approved drugs or vaccine available to treat the infection. In such scenario, one of the propitious therapeutic approaches against viral infection is to explore enzyme inhibitors amidst natural compounds, utilizing computational approaches aiming to get products with negligible side effects. In the present study, the inhibitory prospects of ilimaquinone (marine sponge metabolite) were assessed in comparison with hydroxychloroquine, azithromycin, favipiravir, ivermectin and remdesivir at the active binding pockets of nine different vital SARS-CoV-2 target proteins (spike receptor binding domain, RNA-dependent RNA polymerase, Nsp10, Nsp13, Nsp14, Nsp15, Nsp16, main protease, and papain-like-protease), employing an in silico molecular interaction based approach. In addition, molecular dynamics (MD) simulations of the SARS-CoV-2 papain-like protease (PLpro)–ilimaquinone complex were also carried out to calculate various structural parameters including root mean square fluctuation (RMSF), root mean square deviation (RMSD), radius of gyration (Rg) and hydrogen bond interactions. PLpro is a promising drug target, due to its imperative role in viral replication and additional function of stripping ubiquitin and interferon-stimulated gene 15 (ISG15) from host-cell proteins. In light of the possible inhibition of all vital SARS-CoV-2 target proteins, our study has emphasized the importance to study in depth ilimaquinone actions in vivo.
1. Introduction
In December 2019, SARS-CoV-2 (severe acute respiratory syndrome coronavirus 2) commenced and briskly spread around the world (about 213 countries and territories), constituting a pandemic of respiratory diseases designated COVID-19 (coronavirus disease 2019).1 As per the WHO (World Health Organization) situation report-181 on 9th October 2020, the virus has infected 36755449 persons and caused 1066861 deaths around the globe. Therefore, there is a grave need to find effectual drugs towards this prodigious pandemic disease. Yet, no precise treatment has been endorsed against COVID-19 except a few tested drugs like chloroquine derivatives, remdesivir, azithromycin, favipiravir, ivermectin, etc. One of the propitious targets of treatment is COVID-19 papain like protease (PLpro), as it is an obligatory enzyme required for the replication of the virus.2 Although the primary function of main protease 3CLpro and PLpro are to process the viral polyprotein in a coordination, PLpro has the auxillary role of stripping ubiquitin and ISG15 from host cell proteins to aid coronaviruses in their circumvention of the host innate immune responses.2 Consequently, this special quality of PLpro makes it as an interesting drug target for COVID-19, because it will inhibit the dysregulation of signaling cascades in virus infected cells, ultimately inhibiting the viral replication.
Nature has always been considered as the prime source for the discovery of unique and new bioactive compounds, which helps in combatting various diseases and infections.3,4 Marine sponges are known to produce innumerable metabolic substances with different pharmacological properties. Ilimaquinone is a marine sponge metabolite which was firstly identified and isolated from the bristly yellow, orange, or brown sponge named Hippospongia metachromia in 1979.5 The chemical structure of this compound (C22H30O4) contains a benzoquinone moiety, combined with trans-decalin ring system bearing an exocyclic double bond as a rearranged drimane-type sesquiterpene unit.6 It has been reported for its promising pharmacological activities such as inhibition of chemically induced inflammation, antimicrobial activity, anti-cancer activity and anti-viral activity (Rangel et al., 1997; Popov et al., 1999; Lu et al., 2007).7–9 Interestingly, it was delineated to possess antiviral activity against HIV (human immunodeficiency virus).10,11 No study till date has been reported about its possible effective role against SARS-CoV-2, as per our knowledge.
Thus, the present study was commenced to evaluate the potency of illimaquinone against SARS-CoV-2 using in silico approach broadly. We have performed molecular docking analysis of ilimaquinone in comparision with hydroxychloroquine, azithromycin, favipiravir, ivermectin and remdesivir against nine potential SARS-CoV-2 target proteins. Moreover, MD simulation studies of PLpro–ilimaquinone complex was also carried out to calculate various structural parameters including RMSD, RMSF, Rg and hydrogen bond interactions to authenticate our data.
2. Materials and methods
2.1 Protein structures
To study the interactions of different compounds with various protein structures of SARS-CoV-2, molecular docking was performed. Crystal structures of SARS proteins, SARS-CoV-2 spike receptor binding domain from SARS-CoV-2 (PDB 6M0J.pdb),12 SARS-CoV-2 RNA-dependent RNA polymerase from SARS-CoV-2 (PDB 7BTF.pdb),13 SARS-CoV-2 3CL protease (3CLpro) from SARS-CoV-2 (PDB 6M2N.pdb),14 MERS CoV nsp10 from Hyphomicrobium sp. MC1 (PDB 5NYJ.pdb),15 nsp13 from SARS-related coronavirus (PDB 6JYT.pdb),16 SARS-CoV nsp14 from SARS coronavirus Frankfurt 1 (PDB 5NFY.pdb),17 nsp15 endoribonuclease from SARS-CoV-2 (PDB 6VWW.pdb),18 SARS-CoV-2 nsp16 from SARS-CoV-2 (PDB 6YZ1.pdb)19 and SARS-CoV papain-like protease PLpro from SARS-related coronavirus (PDB 4MM3.pdb)20 were retrieved from RCSB PDB. Three dimensional structures of six compounds ilimaquinone, hydroxychloroquine, azithromycin, favipiravir, ivermectin, and remdesivir from well-known organic compound database PubChem Compound in SDF format. These compounds were then converted to PDB format using Open Babel.21
2.2 Ligand preparation
For preparation of input files for docking, PyRx virtual screening tool was used.22 The structures were minimized with Universal Force Field (UFF).23 Conjugate Gradient algorithm was used for optimization with a total of 200 steps.24 During minimization, the structure was updated every 1 step and minimization was terminated when the energy difference is less than 0.1. Energy minimized structures were saved in *.pdbqt format.
2.3 Prediction of binding site
The binding sites of the SARS-CoV-2 protein structures were predicted using Fpocket.25 The pocket with the highest Score and druggability score was considered as the most probable binding site of the proteins.
2.4 Molecular docking
Three-dimensional structures of proteins derived from RCSB PDB were was prepared for molecular docking using AutoDock Tools.26 Water molecules were removed from the initial structures and structures were optimized by adding missing hydrogen atoms. Structure files (pdb-format) of eight peptides and twenty-one compounds were docked separately against the receptor structures using molecular docking software Autodock 4.2.6.26 All the parameters used for docking of six compounds with the receptors were kept same except the grid centre differed for both the study as per the accommodation of active site of nine receptors (6M0J, 7BTF, 3CLpro, nsp10, nsp13, nsp14, nsp15, nsp16 and PLpro) inside the grid box.
Auto Grid was used for the preparation of the grid map using a grid box.26 The grid size was set to 50 × 42 × 50xyz points for 6M0J receptor. The grid size for 7BTF was set to 40 × 40 × 54xyz points, 42 × 42 × 42 for 6M2N, 60 × 50 × 44 for nsp10, 72 × 78 × 72 for nsp13, 48 × 48 × 48 for nsp14, 44 × 56 × 40 for nsp15, 40 × 50 × 40 for nsp16, and 48 × 48 × 40 for PLpro. Grid spacing was kept to 0.375 Å for all the receptors. The grid centre for 6M0J was designated at dimensions (x, y and z): −36.258, 40.262 and 13.154, for 7BTF at (x, y and z): 123.323, 112.449 and 116.933, for 6M2N at (x, y and z): −46.903, −0.209 and 49.423, for nsp10 at (x, y and z): 40.254, 31.974 and −8.761, for nsp13 at (x, y and z): 397.648, 28.494 and 66.356, for nsp14 at (x, y and z): −20.155, −32.767 and −4.232, for nsp15 at (x, y and z): −97.084, 88.202 and −2.863, for nsp16 at (x, y and z): 52.137, 71.609 and −15.111, and for PLpro at (x, y and z): 2.603, 36.146 and −23.347. The grid box is cantered in such a way that it encloses the entire binding site of both the receptors and provides enough space for translation and rotation of ligands. The generated docked conformation was ranked by predicted binding energy and topmost binding energy docked conformation was analysed using BIOVIA Discovery Studio27 for intermolecular hydrogen bonding of active site amino acid residues from the receptors with docked ligands.
2.5 Molecular dynamics simulations
Molecular Dynamics (MD) simulations were carried out for the PLpro–ilimaquinone complex for a period of 50 ns with GROMACS 2020 using the CHARMM36m force field.28 PLpro–ilimaquinone complex structure were placed in a cubic box of size 97 Å × 97 Å × 97 Å and solvated with TIP3P water molecules and ions were added. Simulation systems were neutralized with counterions and a salt concentration of 0.15 M KCl was maintained. An initial energy minimization was performed using steepest descent algorithm until the system converged to Fmax < 1000 kJ (mol nm)−1. System equilibration was performed which was followed by production simulation. System equilibration was performed at a constant number of particles, volume, and temperature (NVT), whereas production simulations were performed for duration of 50 ns using constant particles, pressure, and temperature (NPT) ensemble. The temperature of the system was kept at 303.15 K. For the simulations, the isotropic Monte Carlo (MC) barostat and the Nose–Hoover thermostat were used to maintain the pressure at 1 atm. With a time step of 2 fs, coordinates were saved at every 10 ps during the production run. The 2D plots representing the intrinsic dynamical stabilities of the complex such as root mean-square deviation (RMSD), root mean-square fluctuations (RMSF), radius of gyration (Rg), and hydrogen bond interactions between the protein and compound were generated.
3. Results and discussion
3.1 Analysis of the binding pockets of SARS-CoV-2 proteins
To perform the molecular docking analysis active site residues within SARS-CoV-2 receptor proteins were determined using Fpocket server.25 Several amino acids lining the binding pocket have been identified using Fpocket server (Table 1). The amino acid residues lining the binding site of PLpro were Pro77, Asp76, Leu178, Ala176, His175, Asn128, Thr75, His73, Asp179, Asn128, Phe173, Gly201, Val202, Pro129, and Gln174. From Fpocket analysis, several pockets were generated with associated Fpocket score and druggability score. Only the top scoring pockets were selected for molecular docking analysis and generation of grid box. During molecular docking analysis, complete binding pocket was selected for the generation of the grid box.
Table 1 Binding pocket properties of SARS-CoV-2 receptor proteins predicted by Fpocket server
Receptors |
Fpocket score |
Fpocket druggability score |
Amino acids lining the binding site |
6M0J |
0.343 |
0.029 |
Arg457, Asp467, Lys458, Arg454, Phe456, Tyr473, Pro491, Glu471, Ile472, Ser459 |
3CLpro |
0.094 |
0.004 |
Ser254, Ala255, Gly251, Val261, Ala260, Val247, Leu262 |
PLpro |
0.053 |
0.002 |
Pro77, Asp76, Leu178, Ala176, His175, Asn128, Thr75, His73, Asp179, Asn128, Phe173, Gly201, Val202, Pro129, Gln174 |
7BTF |
0.155 |
0.002 |
Arg467, Arg735, Leu731, Asn734, Arg305, Asp736, Val737, Leu470, Cys730, Asp738, Phe471, Glu474 |
Nsp10 |
0.271 |
0.606 |
Leu31, Gly109, Lys113, Lys28, Asp22, Lys25, Gly109, Asp106, Leu112, Ala24, Tyr27 |
Nsp13 |
0.086 |
0.024 |
Asn562, Pro284, Asp458, Asn562, Pro284, Thr566, Leu461, Cys441, Pro283, Cys444, Val452, Ile448, Val449 |
Nsp14 |
0.217 |
0.001 |
Phe367, Ser369, Asp390, Ala371, Tyr370, Arg391, Asn388, Pro429, Arg289, Ser434, Val389, Tyr368, Val287, Asp432, His427, Arg289, Tyr260, Leu259, His283, Asp415, Ala430, Gly417, Val290, Phe286, Lys288, Cys414 |
Nsp15 |
0.219 |
0.663 |
Ile295, His249, Leu250, Phe329, Pro343, Ser293, Phe302, Leu297, Ile305, Val320, Phe341, Tyr342, Ile322, Ile235, Leu250 |
Nsp16 |
0.157 |
0.002 |
Phe156, Tyr152, Gln159, Phe156, Val118, His119, Thr120, Ser291, Lys160, Ala121, Gly155, Thr151, Val289 |
3.2 Molecular docking analysis
Novel inhibitor identification against the protein targets consists of evaluation of binding mode of interaction between inhibitor and receptor by performing molecular docking which assesses the effect of solvent on the protein–ligand complex stability (Fig. 1–9). Molecular docking of ilimaquinone, hydroxychloroquine, azithromycin, favipiravir, ivermectin and remdesivir with nine potential SARS-CoV-2 target proteins was performed. Binding energies were calculated of the ligands against the protein targets (Table 2). From the docking analysis, ivermectin showed the highest docking score with an average energy of −8.5 kcal mol−1 among all the compounds. Remdesivir showed the lowest binding energy and highest docking score of −9.9 kcal mol−1 which was followed by ilimaquinone having the second highest binding energy of −8.1 kcal mol−1. When compared with other protein targets, ilimaquinone showed second highest binding energy against 6M0J, PLpro, Nsp10 and Nsp14 targets with docking scores of −6.9, −8.1, −7.6 and −8.1 kcal mol−1. For 3CLpro, ilimaquinone exhibited similar binding energy of −7.1 kcal mol−1 as that of remdesivir and azithromycin. Whereas, for Nsp16, binding energies of ilimaquinone showed similarity with ivermectin and remdesivir. Ilimaquinone demonstrated lowest binding energy of −8.2 kcal mol−1 with Nsp13 among other protein targets.
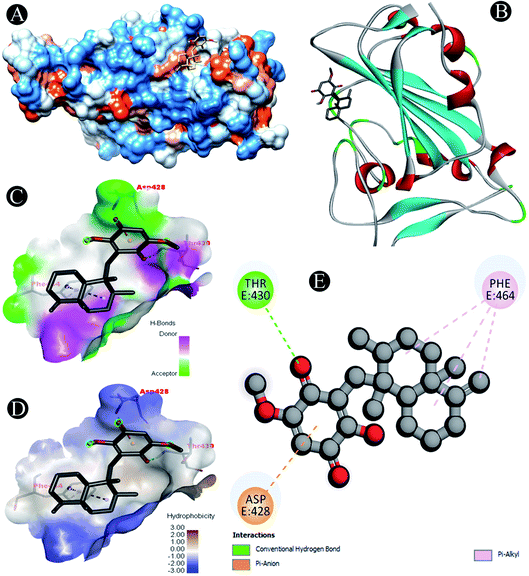 |
| Fig. 1 Visualization of docking analysis of ilimaquinone binding with 6M0J (A) hydrophobicity surface 3D representation (B) interaction of ilimaquinone with 6M0J (C) visualization of hydrogen bond (D) visualization of hydrophobic interaction (E) 2D representation describing bindings of ilimaquinone with active site of 6M0J. | |
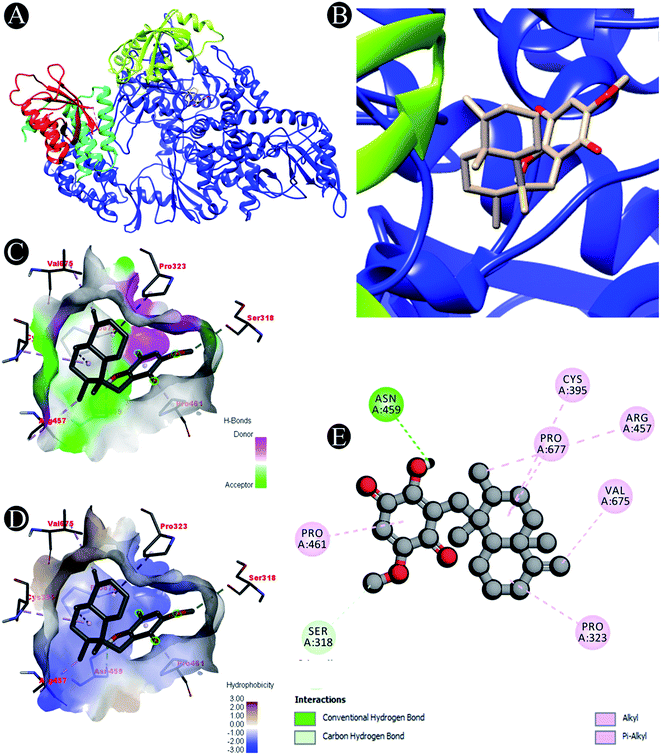 |
| Fig. 2 Visualization of docking analysis of ilimaquinone binding with 7BTF (A) interaction of ilimaquinone with 7BTF (B) close up of interaction of ilimaquinone with 7BTF (C) visualization of hydrogen bond (D) visualization of hydrophobic interaction (E) 2D representation describing bindings of ilimaquinone with active site of 7BTF. | |
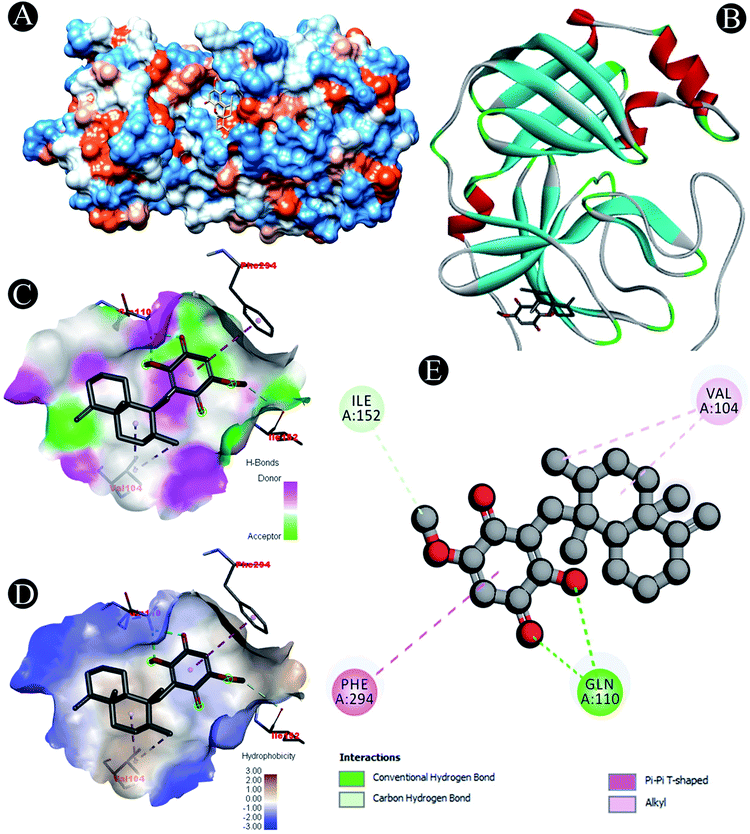 |
| Fig. 3 Visualization of docking analysis of ilimaquinone binding with 3CLpro (A) hydrophobicity surface 3D representation (B) interaction of ilimaquinone with 3CLpro (C) visualization of hydrogen bond (D) visualization of hydrophobic interaction (E) 2D representation describing bindings of ilimaquinone with active site of 3CLpro. | |
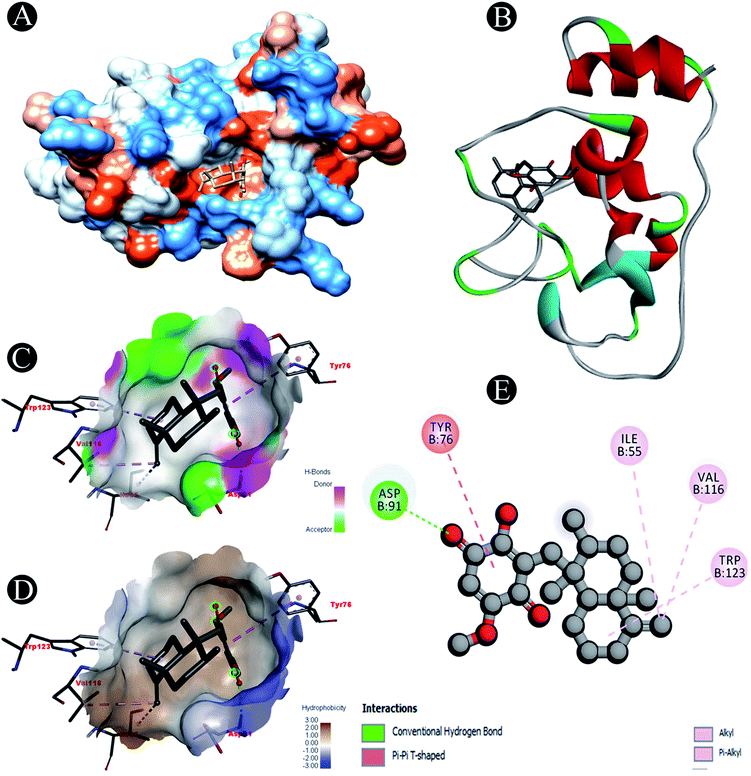 |
| Fig. 4 Visualization of docking analysis of ilimaquinone binding with Nsp10 (A) hydrophobicity surface 3D representation (B) interaction of ilimaquinone with Nsp10 (C) visualization of hydrogen bond (D) visualization of hydrophobic interaction (E) 2D representation describing bindings of ilimaquinone with active site of Nsp10. | |
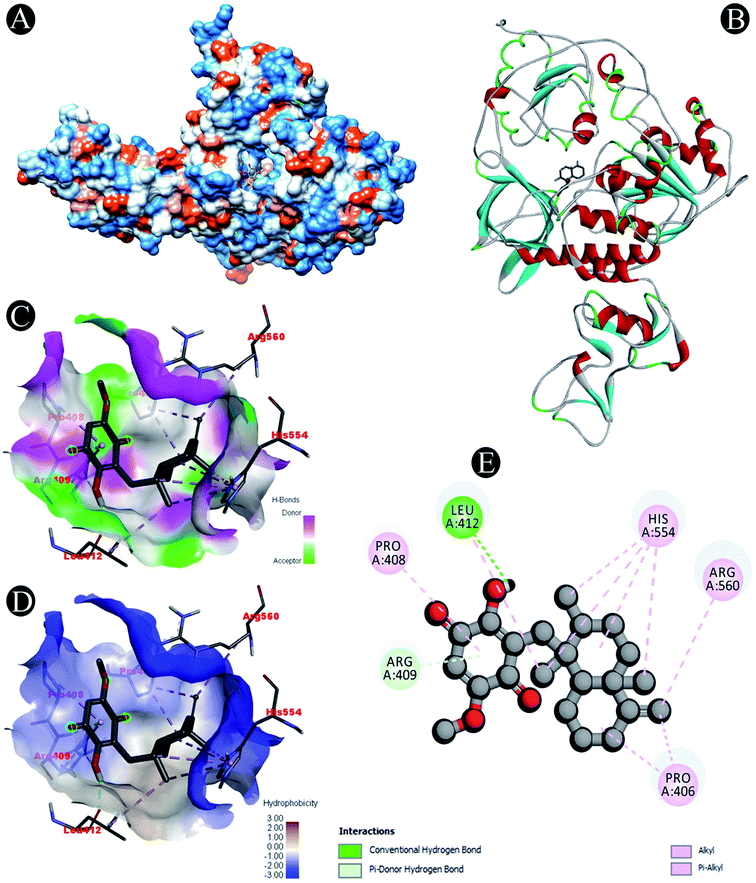 |
| Fig. 5 Visualization of docking analysis of ilimaquinone binding with Nsp13 (A) hydrophobicity surface 3D representation (B) interaction of ilimaquinone with Nsp13 (C) visualization of hydrogen bond (D) visualization of hydrophobic interaction (E) 2D representation describing bindings of ilimaquinone with active site of Nsp13. | |
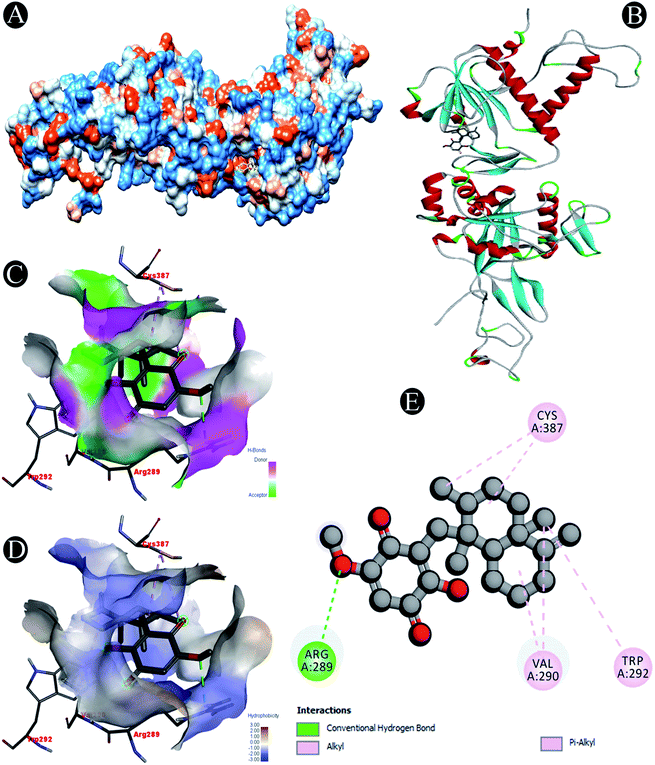 |
| Fig. 6 Visualization of docking analysis of ilimaquinone binding with Nsp14 (A) hydrophobicity surface 3D representation (B) interaction of ilimaquinone with Nsp14 (C) visualization of hydrogen bond (D) visualization of hydrophobic interaction (E) 2D representation describing bindings of ilimaquinone with active site of Nsp14. | |
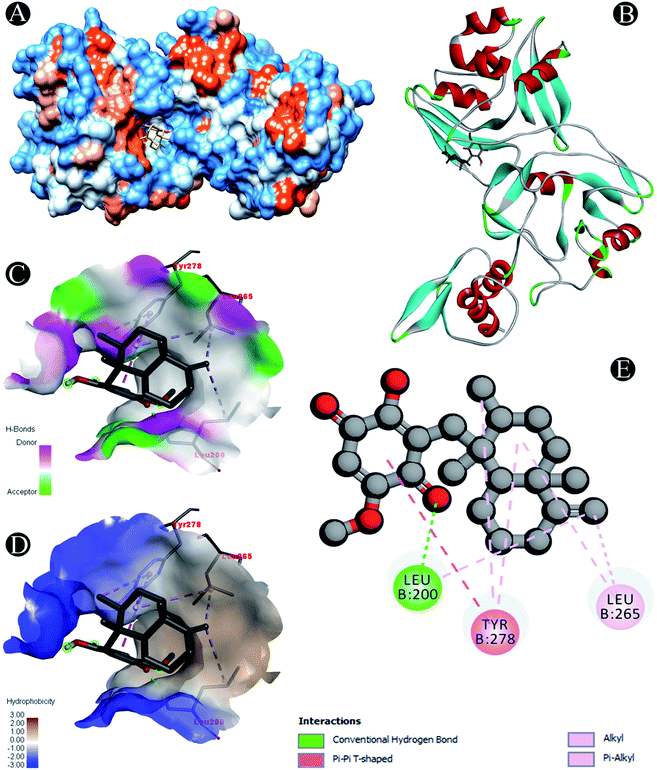 |
| Fig. 7 Visualization of docking analysis of ilimaquinone binding with Nsp15 (A) hydrophobicity surface 3D representation (B) interaction of ilimaquinone with Nsp15 (C) visualization of hydrogen bond (D) visualization of hydrophobic interaction (E) 2D representation describing bindings of ilimaquinone with active site of Nsp15. | |
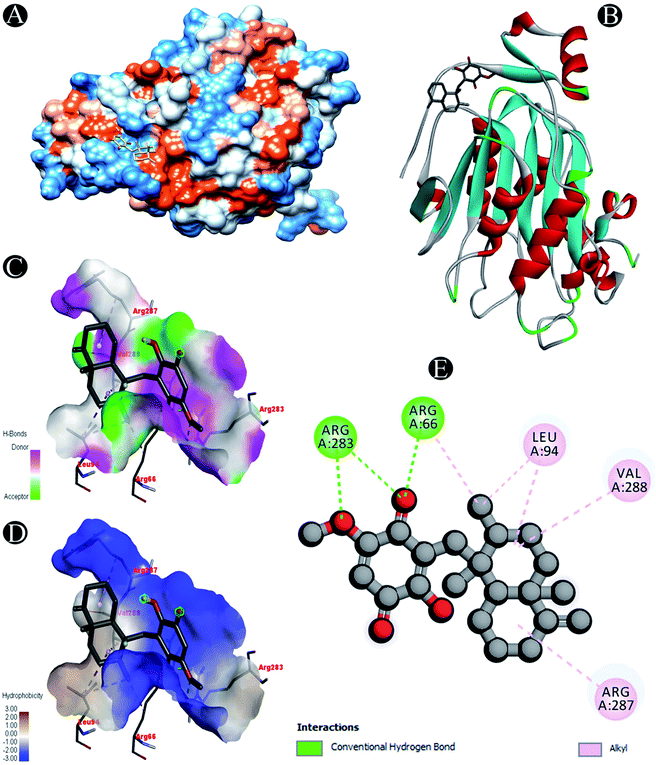 |
| Fig. 8 Visualization of docking analysis of ilimaquinone binding with Nsp16 (A) hydrophobicity surface 3D representation (B) interaction of ilimaquinone with Nsp16 (C) visualization of hydrogen bond (D) visualization of hydrophobic interaction (E) 2D representation describing bindings of ilimaquinone with active site of Nsp16. | |
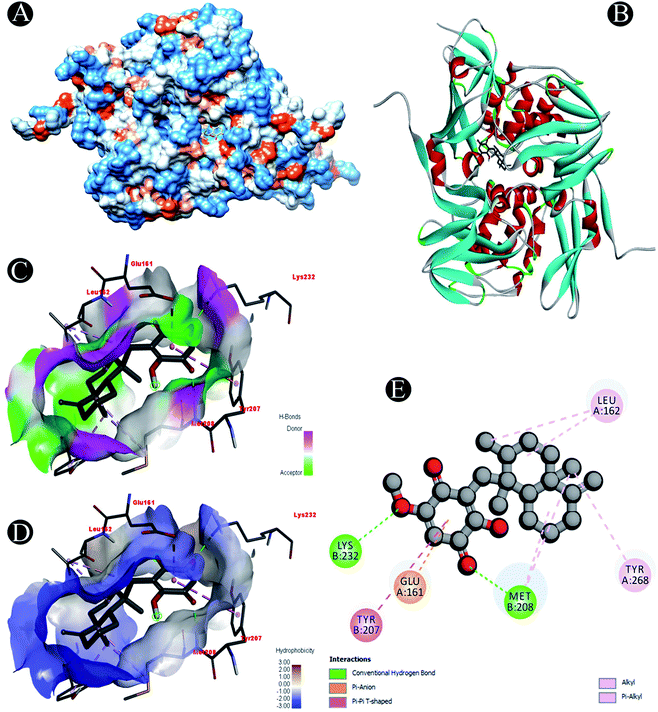 |
| Fig. 9 Visualization of docking analysis of ilimaquinone binding with Plpro (A) hydrophobicity surface 3D representation (B) interaction of ilimaquinone with Plpro (C) visualization of hydrogen bond (D) visualization of hydrophobic interaction (E) 2D representation describing bindings of ilimaquinone with active site of Plpro. | |
Table 2 Binding energies (in kcal mol−1) of compound/drug against various SARS-CoV-2 targets
Compound/drug |
6M0J |
3CLpro |
PLpro |
7BTF |
Nsp10 |
Nsp13 |
Nsp14 |
Nsp15 |
Nsp16 |
Ilimaquinone |
−6.9 |
−7.1 |
−8.1 |
−6.9 |
−7.6 |
−8.2 |
−8.1 |
−7.5 |
−7.1 |
Hydroxychloroquine |
−5.8 |
−5.3 |
−8 |
−6.1 |
−5.9 |
−6.2 |
−7.2 |
−7 |
−6.3 |
Azithromycin |
−6.7 |
−7.2 |
−6.8 |
−7.5 |
−7.3 |
−8.4 |
−8 |
−7.7 |
−6.7 |
Favipiravir |
−5.2 |
−5.4 |
−6.2 |
−5.9 |
−5.3 |
−5.2 |
−6.4 |
−5.9 |
−5.1 |
Ivermectin |
−8.5 |
−7.7 |
−7.9 |
−9.2 |
−8.6 |
−9 |
−9.4 |
−9 |
−7.2 |
Remdesivir |
−6.4 |
−7.1 |
−9.9 |
−8.2 |
−7.2 |
−7.4 |
−9.4 |
−8.7 |
−7.2 |
Receptor–ligand interactions of ilimaquinone shows that the compound is primarily involved in conventional hydrogen bond, alkyl, pi–alkyl, pi–pi T-shaped and pi–anion interactions (Table 3). Ilimaquinone was involved in 10 interactions with PLpro in the following order: hydrogen bond interactions with Met208 and Lys232 with a distance of 2 Å and 2.34 Å, 5 alkyl interactions with Met208 (3 contacts) and Leu162 (2 contacts) with a distance of 4.04 Å, 4.61 Å and 4.63 Å, 1 pi–alkyl interaction with Tyr268 (4.54 Å), 1 pi–anion interaction with Glu161 (3.89 Å), and 1 pi–pi T shaped interaction with Tyr207 (5.15 Å). When compared with Nsp13, ilimaquinone produced 12 interactions: 1 hydrogen bond with Leu412 (2.01 Å), 4 alkyl interaction with Pro406 (2 contacts with a distance of 4.28 Å and 4.35 Å), Leu412 (4.47 Å) and Arg560 (4.58 Å), 6 pi–alkyl interactions with Pro408, Arg409 and His554 (4 contacts) with distances of 5.47 Å, 5.25 Å, 4.50 Å, 5.01 Å, 5.09 Å and 5.27 Å, respectively.
Table 3 Interactive active site residues top-rated pose of ilimaquinone with the SARS-CoV-2 proteins
Receptor |
Receptor–ligand interactions |
6M0J |
Pi–anion: Asp428 |
Conventional hydrogen bond: Thr430 |
Pi–alkyl: Phe464 |
7BTF |
Carbon hydrogen bond: Ser318 |
Conventional hydrogen bond: Asn459 |
Alkyl/pi–alkyl: Pro323, Cys395, Arg457, Pro461, Val675, Pro677 |
3CLpro |
Carbon hydrogen bond: Ile152 |
Conventional hydrogen bond: Gln110 |
Alkyl: Val104 |
Pi–pi T shaped: Phe294 |
Plpro |
Conventional hydrogen bond: Met208, Lys232 |
Alkyl/pi–alkyl: Met208, Leu162, Tyr268 |
Pi–anion: Glu161 |
Pi–pi T shaped: Tyr207 |
Nsp10 |
Conventional hydrogen bond: Asp91 |
Alkyl/pi–alkyl: Ile55, Val116, Trp123 |
Pi–pi T shaped: Tyr75 |
Nsp13 |
Conventional hydrogen bond: Leu412 |
Alkyl/pi–alkyl: Pro406, Pro408, Arg409, Leu412, His554, Arg560 |
Pi–donor: Arg409 |
Nsp14 |
Conventional hydrogen bond: Arg289 |
Alkyl/pi–alkyl: Arg289, Val290, Trp292, Cys387 |
Nsp15 |
Conventional hydrogen bond: Leu200 |
Alkyl/pi–alkyl: Leu200, Leu265, Tyr278 |
Pi–pi T shaped: Tyr278 |
Nsp16 |
Conventional hydrogen bond: Arg66, Arg283 |
Alkyl: Leu94,Arg287, Val288 |
3.3 MD simulation analysis
The dynamic nature of the protein–ligand complex has been widely studied by MD simulations for scrutinizing the conformational stability, conformational changes, internal motions and protein–ligand interactions. In this study, the MD simulations was performed for PLpro–ilimaquinone complex over 50 ns under physiological conditions to monitor any changes in the conformation, its stability and interactions of ilimaquinone with amino acid residues over time. Using the generated MD trajectories, RMSD, RMSF, Rg and hydrogen bond interactions were computed as a function of time.
3.3.1 RMSD analysis. RMSD analysis is crucial for quantifying the structural stability of the protein or protein–ligand complexes within a regular time frame. RMSD analysis portrayed that the unbound PLpro started stabilising after 12 ns and maintained its stability until 50 ns (Fig. 10A). An average RMSD value of 0.263 nm was obtained for the PLpro–ilimaquinone complex from 12 ns to 50 ns. A minor bump was observed between 43 and 47 ns. The RMSD value declined again after 47 ns and continues to remain constant over the remaining time frame. A loess smoothing function applied over the RMSD trajectory shows a constant curve over the entire RMSD trajectory.29 The loess method applies Local Polynomial Regression Fitting over the polynomial surface using RMSD value as predictor (Fig. 10B). Overall, the results shows that the ilimaquinone bound PLpro complex did not influence the structural stability of the protein and retained its structural integrity.
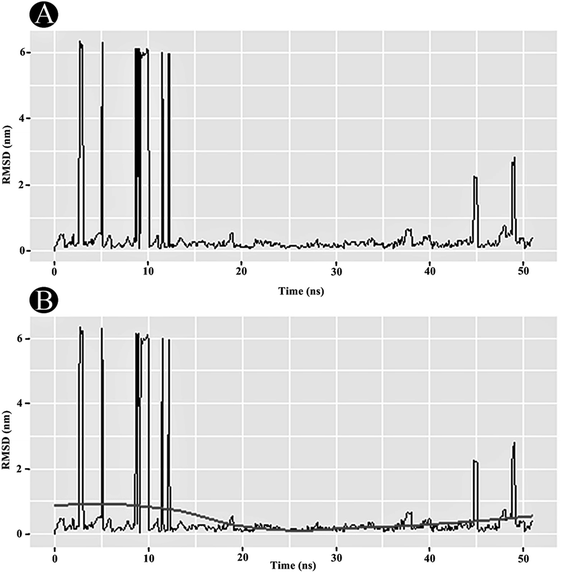 |
| Fig. 10 Plots of (A) RMSD in nm, and (B) RMSD with loess smoothing curve fitted as a function of time. | |
3.3.2 RMSF analysis. RMSF analysis is another essential parameter for identifying the rigid and flexible regions of the protein structure. It is a standard measure of the deviation of the particle from its original position. Furthermore, it can also be used to assess the flexibility of the backbone elements of the protein structure as well as the ligand. The RMSF was analysed for the entire protein structure and ligand combined demonstrating each atom's mean displacement. In the case of PLpro, highest fluctuations between 0.5 nm and 0.8 nm were observed in chain A and B residues. For chain B the residues lining 56 until 70, 186 to 198, 221 to 231, and from 313 to 319, whereas for chain A, 1 to 6, 186 to 197, from 218 to 233, and from 312 to 319 showed fluctuations as illustrated in Fig. 11A. The average RMSF value of 0.44 nm was found to be observed for the complex indicating potential interactions of ilimaquinone with the receptor protein.
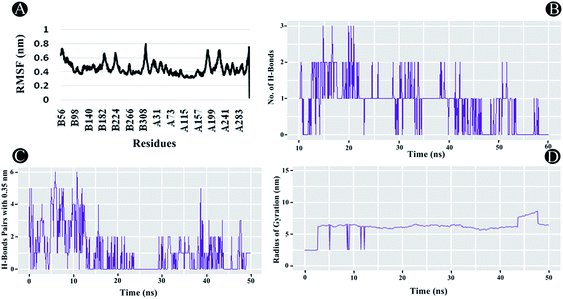 |
| Fig. 11 Molecular dynamics of ilimaquinone bound to Plpro (A) root mean square fluctuations (RMSF) (B) number of hydrogen bonds formed (C) hydrogen bond pairs formed within 0.35 nm and (D) radius of gyration (Rg) as function of simulation time. | |
3.3.3 Radius of gyration (Rg). The Rg is a constructive tool for understanding compactness and folding properties of the protein and protein–ligand complexes. In a protein structure, it can also be used to demonstrate the influence of a drug molecule, exerting conformational changes in it. Higher Rg value specifies protein molecule with loose packing, whereas, lower Rg value specifies tight packing of the protein structure. In the case of ilimaquinone bound to PLpro protein, an average Rg value of 6.104 nm was observed (Fig. 11D), which could be attributed to conformational shifts resulting in changes in the secondary structure of the protein during the MD simulations.
3.3.4 Intermolecular H-bonding. Intermolecular H-bonding is also one of the most crucial parameters for understanding the binding affinity of ligand with the protein molecule. Formation or distortion of hydrogen bonds is a principal component during the MD simulation. Large number of H-bonds present between the protein and ligand signifies a stronger binding affinity. In the case of PLpro–ilimaquinone complex, an average of 1.17 bonds were formed in the first 25 ns, whereas, an average of 0.53 bonds were formed from 25 to 50 ns (Fig. 11B and C). Overall, an average of 0.85 bonds were formed in the 50 ns time frame. A similar analysis revealed that the complex formed greater number of H-bond pairs within 0.35 nm during the MD simulation run. An average of 1.06 H-bond pairs were formed during 50 ns simulation whereas an average of 1.72 pairs were observed in the first 22 ns. These results indicate that ilimaquinone interacted effectively towards the binding site of PLpro with a significant number of hydrogen bonds.
4. Conclusion
Currently, COVID-19 is quickly outspreading around the world and looking difficult to manage its outbreak without drugs and vaccines available. Therefore, there is a hasty need for the development of novel drugs which can inhibit the causative virus SARS-CoV-2. Development of novel drugs demands lot of time, which cannot be afforded, presently. It is sensible to come across with natural products and pre-existing drugs. The primacy of computational approaches such as structural based virtual screening, molecular docking and molecular dynamics is that few important steps of the drug development process like toxicity assays, clinical trials, efficacy studies and side effect studies, which can be eliminated completely. Efforts in present research aimed at exploring the inhibitory prospects of a bioactive sponge metabolite ilimaquinone against nine potential SARS-CoV-2 target proteins, that have been considered as promising drug targets. Our analysis revealed that the ilimaquinone exhibited promising inhibitory potential against all the SARS-CoV-2 target proteins, as evident from the binding energies. Moreover, subsequent analysis of MD simulations, RMSD, RMSF, Rg and hydrogen bond interactions established ilimaquinone as the most promising inhibitory candidate against the SARS-CoV-2 papain like protease. Therefore, present findings promise to establish foundations to further validate the inhibitory potential of the ilimaquinone in both in vitro and in vivo and deploy it as an effective inhibitor of SARS-CoV-2 papain like protease in the quest for effective drug development against COVID-19.
Conflicts of interest
Authors declare no conflict of interests.
Acknowledgements
Malvi Surti is supported by a SHODH-Scheme of Developing High Quality Research (No. 635) from Education Department, Gujarat state, India.
References
- A. I. Owis, M. S. El-Hawary, D. El Amir, O. M. Aly, U. R. Abdelmohsen and M. S. Kamel, RSC Adv., 2020, 10, 19570–19575 RSC.
- Y. Baez-Santos, S. S. John and A. D. Mesecar, Antivir. Res., 2014, 115, 21–38 CrossRef.
- M. Adnan, M. Patel, M. N. Reddy and E. Alshammari, Sci. Rep., 2018, 8, 1740 CrossRef.
- M. Patel, M. S. Ashraf, A. J. Siddiqui, S. A. Ashraf, M. Sachidanandan, M. Snoussi, M. Adnan and S. Hadi, Biomolecules, 2020, 10, 920 CrossRef CAS.
- R. T. Luibrand, T. R. Erdman, J. J. Vollmer and P. J. Scheuer, Tetrahedron, 1979, 35, 609–612 CrossRef CAS.
- M. L. Bourguet-Kondracki, A. Longeon, E. Morel and M. Guyot, Int. J. Immunopharmacol., 1991, 13, 393–399 CrossRef CAS.
- H. R. Rangel, F. Dagger and R. S. Compagnone, Cell Biol. Int., 1997, 21, 337–339 CrossRef CAS.
- P. H. Lu, S. C. Chueh, F. L. Kung, S. L. Pan, Y. C. Shen and J. H. Guh, Eur. J. Pharmacol., 2007, 556, 45–54 CrossRef CAS.
- A. M. Popov, S. I. Stekhova, N. K. Utkina and N. M. Rebachuk, Pharm. Chem. J., 1999, 33, 71–73 CrossRef CAS.
- S. Loya and A. Hizi, J. Biol. Chem., 1993, 268, 9323–9328 CAS.
- L. A. Tziveleka, C. Vagias and V. Roussis, Curr. Top. Med. Chem., 2003, 3, 1512–1535 CrossRef CAS.
- J. Lan, J. Ge, J. Yu, S. Shan, H. Zhou, S. Fan, Q. Zhang, X. Shi, Q. Wang, L. Zhang and X. Wang, Nature, 2020, 581, 215–220 CrossRef CAS.
- Y. Gao, L. Yan, Y. Huang, F. Liu, Y. Zhao, L. Cao, T. Wang, Q. Sun, Z. Ming, L. Zhang, J. Ge, L. Zheng, Y. Zhang, H. Wang, Y. Zhu, C. Zhu, T. Hu, T. Hua, B. Zhang and Z. Rao, Science, 2020, 368, 779–782 CrossRef CAS.
- H. X. Su, W. F. Zhao, M. J. Li, H. Xie and Y. C. Xu, Worldwide Protein Data Bank, 2020, DOI:10.2210/pdb6m2n/pdb.
- S. M. Wei, L. Yang, Z. H. Ke, Y. Chen, D. Y. Guo and C. P. Fan, Worldwide Protein Data Bank, 2018, DOI:10.2210/pdb5ynj/pdb.
- J. Zhihui, Y. Liming, R. Zhilin, W. Lijie, J. Wang, J. Guo, L. Zheng, Z. Ming, L. Zhang, Z. Lou and Z. Rao, Nucleic Acids Res., 2019, 47, 6538–6550 CrossRef.
- F. Ferron, L. Gluais, C. Vonrhein, G. Bricogne, B. Canard and I. Imbert, Worldwide Protein Data Bank, 2018, DOI:10.2210/pdb5nfy/pdb.
- Y. Kim, R. Jedrzejczak, N. I. Maltseva, M. Wilamowski, M. Endres, A. Godzik, K. Michalska and A. Joachimiak, Protein Sci., 2020, 29, 1596–1605 CrossRef CAS.
- P. Krafcikova, J. Silhan, R. Nencka and E. Boura, Worldwide Protein Data Bank, 2020, DOI:10.2210/pdb6yz1/pdb.
- K. Ratia, A. Kilianski, Y. M. Baez-Santos, S. C. Baker and A. Mesecar, PLoS Pathog., 2014, 10, e1004113 CrossRef.
- N. M. O'Boyle, M. Banck, C. A. James, C. Morley, T. Vandermeersch and G. R. Hutchison, J. Cheminf., 2011, 3, 33, DOI:10.1186/1758-2946-3-33.
- S. Dallakyan and A. J. Olson, Methods Mol. Biol., 2015, 1263, 243–250 CrossRef CAS.
- A. K. Rappe, C. J. Casewit, K. S. Colwell, W. A. Goddard and W. M. Skiff, J. Am. Chem. Soc., 1992, 114, 10024–10035 CrossRef CAS.
- S. J. Watowich, E. S. Meyer, R. Hagstrom and R. Josephs, J. Comput. Chem., 1988, 9, 650–661 CrossRef.
- V. Le Guilloux, P. Schmidtke and P. Tuffery, BMC Bioinf., 2009, 10, 168 CrossRef.
- G. M. Morris, R. Huey, W. Lindstrom, M. F. Sanner, R. K. Belew, D. S. Goodsell and A. J. Olson, J. Comput. Chem., 2009, 16, 2785–2791 CrossRef.
- D. S. BIOVIA, Discovery studio modeling environment. Dassault Systemes, Release – 4, San Diego, 2015 Search PubMed.
- H. Bekker, H. J. C. Berendsen, E. J. Dijkstra, S. Achterop, R. van Drunen, D. van der Spoel, A. Sijbers, H. Keegstra and M. K. R. Renardus, Gromacs: A parallel computer for molecular dynamics simulations in Physics computing 92, ed. R. A. de Groot and J. Nadrchal, World Scientific Publishing, Singapore, 1993, pp. 252–256 Search PubMed.
- W. S. Cleveland, E. Grosse and W. M. Shyu, Local regression models in Statistical Models in S, ed. J. M. Chambers and T. J. Hastie, Wadsworth & Brooks/Cole, 1992 Search PubMed.
Footnote |
† Both authors have contributed equally and should be considered as first authors. |
|
This journal is © The Royal Society of Chemistry 2020 |
Click here to see how this site uses Cookies. View our privacy policy here.