DOI:
10.1039/D0RA06038K
(Paper)
RSC Adv., 2020,
10, 40264-40275
Pharmacophore-based approaches in the rational repurposing technique for FDA approved drugs targeting SARS-CoV-2 Mpro†
Received
10th July 2020
, Accepted 10th October 2020
First published on 4th November 2020
Abstract
Novel coronavirus (CoV) is the primary etiological virus responsible for the pandemic that started in Wuhan in 2019–2020. This viral disease is extremely prevalent and has spread around the world. Preventive steps are restricted social contact and isolation of the sick individual to avoid person-to-person transmission. There is currently no cure available for the disease and the search for novel medications or successful therapeutics is intensive, time-consuming, and laborious. An effective approach in managing this pandemic is to develop therapeutically active drugs by repurposing or repositioning existing drugs or active molecules. In this work, we developed a feature-based pharmacophore model using reported compounds that inhibit SARS-CoV-2. This model was validated and used to screen the library of 565 FDA-approved drugs against the viral main protease (Mpro), resulting in 66 drugs interacting with Mpro with higher binding scores in docking experiments than drugs previously reported for the target diseases. The study identified drugs from many important classes, viz. D2 receptor antagonist, HMG-CoA inhibitors, HIV reverse transcriptase and protease inhibitors, anticancer agents and folate inhibitors, which can potentially interact with and inhibit the SARS-CoV-2 Mpro. This validated approach may help in finding the urgently needed drugs for the SARS-CoV-2 pandemic with infinitesimal chances of failure.
1. Introduction
Coronaviruses (CoVs) are enveloped viruses belonging to the Coronaviridae family and have single-stranded RNA.1 The CoVs reported in literature are known to infect humans as well as other mammals, but the severe acute respiratory syndrome CoV (SARS-CoV) and Middle East respiratory syndrome (MERS-CoV) from 2012 and 2020, respectively, are from zoonotic sources and were reported to have high infection and mortality rates.2 These belong to the beta CoV genus, which broadly includes both the SARS and MERS CoVs. The pandemic known as COVID-19 has caused almost 33 million infections and more than one million deaths as of 27 September 2020.3 The disease is now in a very deadly and infectious situation which has caused 7
139
553; 5
730
184; 4
627
780; 1
122
241; 784
268; 782
695; 710
049; 693
556; 665
188; 664
799 and 481
141 cases in the countries like the USA, India, Brazil, Russia, Columbia, Peru, Mexico, Spain, South Africa, Argentina, and France, respectively.4 The daily toll for infections is also high and is at peak till 20th May 2020 in these countries.
In these deadly conditions, the disease lacks approved effective drugs, which has made this condition more serious and critical when even an asymptomatic carrier of the virus can infect >2 healthy individuals. The well-documented approach of our group to rationally redefine the usage of existing drugs for an alternative use rather than the reported repurposing of drugs is a reasonable way to resolve the time constraints and clinical trial process of drug development for this pandemic. The use of antiviral drugs like oseltamivir, favinapir, ganciclovir–ritonavir, remdesivir, and lopinavir has been clinically tested against COVID-19 disease. Chloroquine, an antimalarial drug, has been recognized to be effective for COVID-19 treatment.5–7 These are examples of the strategy on a hit and trial basis. Based on these studies, several researches have taken drug discovery to a new level using computational methods to identify drug candidates for this lethal pathogen.8–13 These targets include angiotensin-converting enzyme 2 (ACE2), RNA-dependent RNA polymerase (RdRp), spike proteins, and the main protease (Mpro) of the deadly virus. These targets can be efficiently used for the identification of existing drugs or rational design of new chemical entities.10,12,13 In our works since 2010, we have reported a well-defined protocol based on computational repurposing for different diseases like osteoporosis, diabetes, filaria, malaria, Alzheimer's disease, obesity, and many others through direct and indirect drug design approaches.14–22
The main objective of our research in this manuscript is to use a well-validated protocol to repurpose drugs through state-of-the-art computational chemistry techniques using a pharmacophore model based on common features of reported SARS-CoV-2 inhibitors. This model has been validated and used in virtual screening experiments to identify the top hits or drugs that may inhibit SARS-CoV-2 main protease. These identified drugs were further validated for selectivity of the target proteins by molecular docking to find a probable mechanistic pathway for inhibition of the virus. These FDA-approved drugs may be emergent drugs for the pandemic as the safety index and all toxicity data are available, so this work fulfills the urgent need for leads for this deadly virus.
2. Materials and methods
2.1 Common feature pharmacophore model
Based on our previous studies in the repositioning of drugs through pharmacophore modeling, a well-defined protocol was implemented as reported in the literature by our group.14–22 Here, medications used in the treatment of COVID-19 have been compiled from literature studies. As quantitative data of the 3 log unit variation for the target is unavailable, we selected HipHop, or common feature pharmacophore model or qualitative modeling, for the identification of compounds, as reported by us in many previous reports. We built a common feature pharmacophore HipHop model using ten structurally diverse compounds with clinical activity in the disease (training set, Fig. 1).
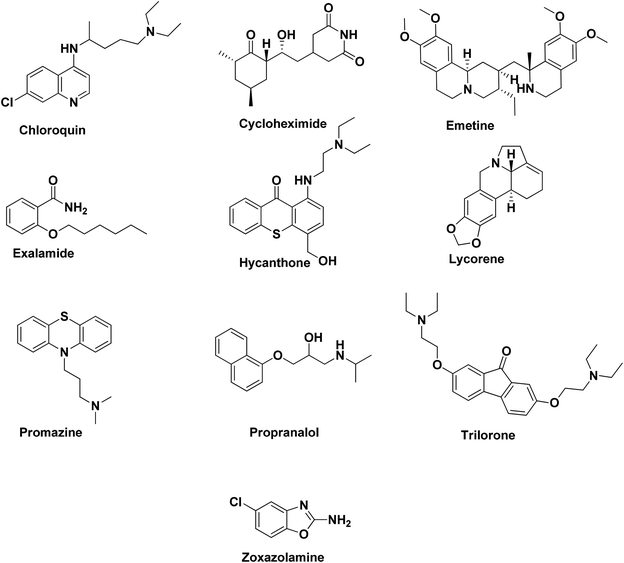 |
| Fig. 1 Structures of training set compounds. | |
2.2 Common feature pharmacophore generation
The pharmacophore generation protocol was performed using the HipHop algorithm of Catalyst as employed in Discovery Studio 2020 (DS 2020).23,24 All training set compounds were drawn/built using ISIS Draw 2.5 and imported into DS 2020 Windows. The CHARMm force field was applied to optimize the training set compounds.25 The conformations of these compounds were generated using the ‘diverse conformation generation’ protocol of DS 2020 with default parameters (principal value = 2, maximum omit feature = 0, interfeature distance 2 Å). The most active compound was assigned a score of 1 and moderately and less active compounds were assigned a score of 0. The ‘feature mapping’ protocol was run to detect common features in the training set. In this case, all the compounds were considered highly active.
2.3 Pharmacophore-based virtual screening (PBVS)
The PBVS approach was used to identify potential hits for COVID-19. The validated model of the pharmacophore (Hypo-1) was used as a query to search for compounds in the Zinc, Asinex, Drug Bank, Maybridge,26,27 and in-house virtual databases using the ‘best flexible search’ option in DS 2020. The resulting hits were screened based on fit values <2.5, followed by additional screening using physiochemical properties. In addition, these hits (565) were subjected to visual inspection for proper alignments with Hypo-1 and finally subjected to molecular docking. After completing the virtual screening process, the 66 most potent hits were retrieved from these databases. Ten of these top hits were selected based on their MolDock and rerank scores for further study.
2.4 Molecular docking studies
Molecular docking was performed using the MolDock module in Molegro Virtual Docker (MVD) software.28 The scoring function of molecular docking in MolDock is based on piecewise linear potentials (PLPs).29 PDB IDs 4YOI and 6LU7 (ref. 30 and 31) have been reported as co-crystal structures of the Mpro; we selected 6LU7 for this study. A re-ranking method was applied to the highest-ranked poses to increase the accuracy of docking. The search algorithm ‘MolDock SE’ was applied for this analysis, with a population size of 50 and a maximum number of iterations of 1500 as parameters. Other parameters were kept as defaults with the number of runs at 10. Since MVD relies on an evolutionary algorithm, repeated docking runs do not result in precisely the same poses and interactions. To address this intrinsic arbitrariness, ten successive runs were performed and the three best poses were used to visualize further interactions as previously reported by us.19–22
3. Results and discussion
3.1 Pharmacophore modeling
Ten pharmacophore models (hypotheses) were generated from the training set of compounds presented in Fig. 1 (ref. 32) with rank scores ranging from 75.397 to 64.693 (Table 1). The resulting ten hypotheses contained two common features, viz. a two hydrogen bond acceptor-lipid (2H) and two hydrophobic (Z) features. Hypo-1 (Fig. 2A) was chosen from these ten hypotheses as it mapped all the features of the most active molecule in the ten-compound training set, lycorene, which was mapped with one hydrogen bond acceptor lipid (HABL) at the 1,3-dioxolane of the benzo[d][1,3]dioxole ring with one of the oxygens. The other HABL function was mapped on the cyclohex-3-ene-1,2-diol one –OH. The aromatic ring of the benzo[d][1,3]dioxole part served one hydrophobic function while the fused pyrrolidine ring was mapped for the other hydrophobic functions. The common feature pharmacophore model was used because it is an important tool for extracting the important features of training set compounds. The other parameter which may be required for the quantitative hypotheses is the one log unit difference in the most active and least active compounds. In this dataset, with the HipHop module, a resulting pharmacophore has a good chance to map with all-inclusive features and has further validity with test set compounds that are excluded from the training set. All the features of the pharmacophore generation protocol of the module were kept at defaults. The scores support the selection of Hypo-1 as the best hypothesis represented in Table 1. The mapping of training set compounds on the developed model is presented in Fig. 2B–E.
Table 1 Results of pharmacophore runa
Hypo |
Features |
Rank |
Direct hit |
Partial hit |
Max fit |
H, hydrogen bond acceptor; Z, hydrophobic group. Direct hit; all the features of the hypothesis are mapped. Direct hit = 1 means yes and direct hit = 0 is no. Partial hit; partial mapping of the hypothesis. Partial hit = 1 means yes and partial hit = 0 means no. |
01 |
ZZHH |
75.397 |
1111111111 |
0000000000 |
4 |
02 |
ZZHH |
72.787 |
1111111111 |
0000000000 |
4 |
03 |
ZZHH |
72.473 |
1111111111 |
0000000000 |
4 |
04 |
ZZHH |
71.472 |
1111111111 |
0000000000 |
4 |
05 |
ZZHH |
70.998 |
1111111111 |
0000000000 |
4 |
06 |
ZZHH |
69.619 |
1111111111 |
0000000000 |
4 |
07 |
ZZHH |
69.251 |
1111111111 |
0000000000 |
4 |
08 |
ZZHH |
67.687 |
1111111111 |
0000000000 |
4 |
09 |
ZZHH |
64.695 |
1111111111 |
0000000000 |
4 |
10 |
ZZHH |
64.693 |
1111111111 |
0000000000 |
4 |
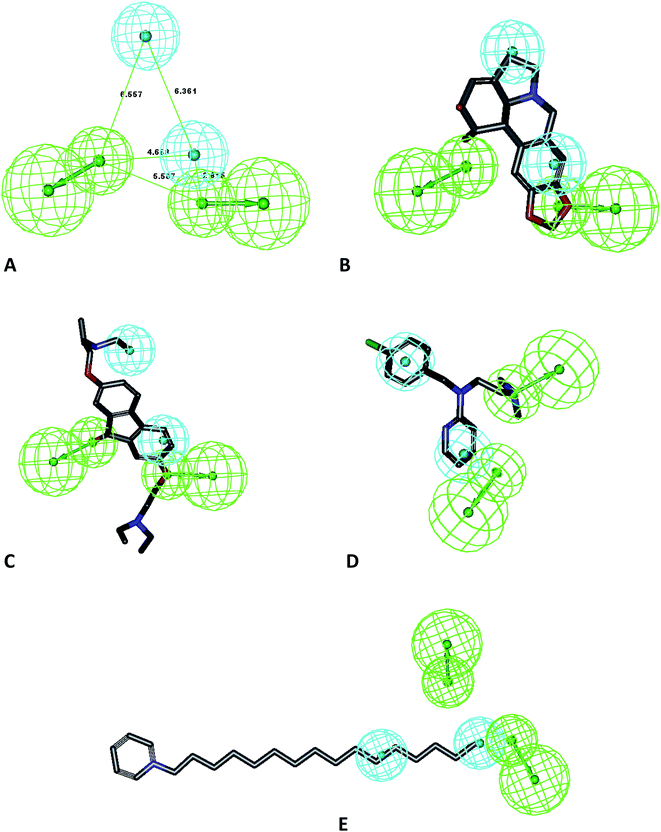 |
| Fig. 2 (A) The representative pharmacophore model Hypo-1. (B) Mapping of lycorene on Hypo-1. (C) Mapping of trilorene on Hypo-1. (D) Mapping of doxazosin on Hypo-1. (E) Mapping of cetylpyridinium on Hypo-1. | |
3.2 Pharmacophore validation
To avoid error in pharmacophore generation, as well as for further application in the screening of libraries, we validated our model using the mapping of molecules which were not included in the test set. The results from the test set prediction are included as Table 2. Furthermore, we studied the mapping in a recently published study for selective Mpro inhibitors along with the compounds used in clinical settings for the treatment of SARS-CoV-2. The results of this study confirmed the applicability of this model to predict and differentiate active from less active compounds. A total of 18 compounds was used in the external dataset and the ligand pharmacophore mapping was used to map these compounds. The mapping showed that Hypo-1 predicted these compounds in accordance with their reported activity as highly active, moderately active, and least active (Table 2) and Hypo-1 also validated the external set of compounds with good predictive values. The ligand pharmacophore mapping of the test set representative compounds is presented in Fig. 3A–E. The molecules were predicted correctly as highly active and moderately active as per their reported EC50 values. The large macromolecular structure of vanilomycin showed lower fit values due to lesser mapping compared to the structure of the molecule in Fig. 3A. The mappings of the external test set of compounds, viz. carmafour, cinanserin, hydroxychloroquine, and shikonin, are represented in Fig. 3B–E, respectively. This study confirmed the applicability of the model for pharmacophore-based virtual screening experiments (PBVS) (Table 3).
Table 2 The fit values of the test set of compounds. The reported compounds were classified based on their EC50 values as most active +++ (0–30 μM), moderately active ++ (30.1–50 μM), and least active + (50.1–150 μM)
Sr. no. |
Compound name |
Fit value |
Predicted scale |
Reported scale |
Reported EC50 |
1 |
Mycophenoic acid |
3.94703 |
+++ |
+++ |
1.95 |
2 |
Antimycin |
3.83514 |
+++ |
+++ |
1.65 |
3 |
Mycophenolate |
3.82501 |
+++ |
+++ |
1.58 |
4 |
Dihydroxy acetyl |
3.7138 |
+++ |
+++ |
1.71 |
5 |
Salinomycin sod |
3.63292 |
+++ |
+++ |
0.29 |
6 |
Monensin |
3.48821 |
+++ |
+++ |
3.81 |
7 |
Doxazosin |
2.99589 |
+++ |
+++ |
4.97 |
8 |
Chloropyramine |
2.99589 |
+++ |
+++ |
1.79 |
9 |
Vanilomycin |
2.98885 |
+++ |
+++ |
4.43 |
10 |
Berbamine |
2.98486 |
+++ |
+++ |
1.48 |
11 |
Diperidon |
2.98257 |
+++ |
+++ |
1.71 |
12 |
Pristimerin |
2.97336 |
+++ |
+++ |
1.99 |
13 |
Desipramine |
2.96494 |
+++ |
+++ |
1.67 |
14 |
Loperamide |
2.9592 |
+++ |
+++ |
1.86 |
15 |
Oligomycin |
2.95825 |
+++ |
+++ |
0.19 |
16 |
Papaverine |
2.94479 |
+++ |
+++ |
1.61 |
17 |
Alprenolol |
2.92827 |
+++ |
+++ |
1.95 |
18 |
Ticlopidine |
2.89131 |
+++ |
+++ |
1.41 |
19 |
Harmine |
2.88484 |
+++ |
+++ |
1.9 |
20 |
Terandine |
2.81998 |
+++ |
+++ |
0.29 |
21 |
Conessine |
2.64779 |
+++ |
+++ |
2.34 |
22 |
4-Hydroxy chalcone |
2.27288 |
+++ |
+++ |
1.52 |
23 |
Phenazopyridine |
2.25038 |
+++ |
+++ |
1.92 |
24 |
Phenyl mercuric acetate |
1.99957 |
+++ |
+++ |
2.17 |
25 |
Pyrvinium pamoate |
1.81371 |
+++ |
+++ |
3.21 |
26 |
Cetylpyridinium |
1.68394 |
+++ |
+++ |
4.31 |
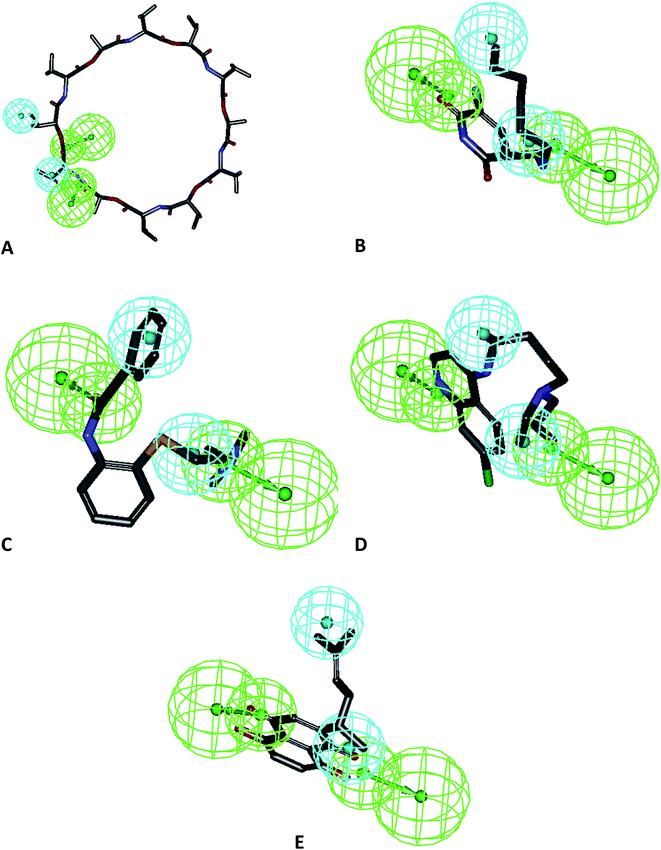 |
| Fig. 3 Mapping of test set compounds on Hypo-1: (A) vanilomycin, (B) carmafour, (C) cinanserin, (D) hydroxychloroquine and (E) shikonin. | |
Table 3 The predicted fit values and activity scales of the external test set compounds. The reported compounds were classified based on their EC50 as most active +++ (0–30 μM), moderately active ++ (30.1–50 μM), and least active + (50.1–150 μM)
Compound name |
Fit value |
Predicted scale |
Reported scale |
EC50 |
Carmofur |
3.07649 |
+++ |
+++ |
1.82 |
Cinanserin |
3.41556 |
+++ |
+ |
124.9 |
Disulfiram |
2.76355 |
+++ |
+++ |
9.35 |
Ebselen |
1.85441 |
++ |
+++ |
0.67 |
HCQ |
3.69414 |
+++ |
NA |
NA |
PX12 |
2.36422 |
+++ |
+++ |
21.32 |
Shikonin |
3.73749 |
+++ |
+++ |
15.75 |
TDZD |
2.29467 |
+++ |
+++ |
2.15 |
Tideglusib |
2.30247 |
+++ |
+++ |
1.5 |
3.3 Pharmacophore based virtual screening
After the triple validation, the pharmacophore model was implemented as a query for PBVS experiments using the Skellochem, FDA-approved Drug and Drugs from Natural Resources databases. Additionally, the Across database was used to further design the library for targeted synthesis as will be reported in the synthesis part of our other manuscript. Our group has documented the virtual screening protocol well in the past for multiple targets essential to different diseases. The ligand pharmacophore mapping protocol was used to screen these databases with filters for the most active compounds, with a fit value range between 2.5–4. A total number of 100 known drug molecules were prioritized from this screening and the top 66 based on their mapping were further studied in molecular docking experiments. The preparations of the database were carried out using the ‘prepare ligands’ protocol of DS 2020.
3.4 Molecular docking
3.4.1 Molecular docking of training set compounds. The structure-based studies were carried out using Molegro Virtual Docker 4.0, well-reported software, and results from our group have been published in our previous studies of various targets.14–22 The docking protocol from our previous studies was again validated using co-crystal ligand docking in the binding site of the protein. The scores for standard co-crystal ligands for the training set of compounds are included in Table 4 for further comparison and prioritize the scores of drugs from the virtual screening. The binding interactions of the training and test sets, along with virtually identified compounds, were studied to extensively validate our protocol. The template docking, or a structure-based pharmacophore, was also developed and used to study and screen the features of the co-crystal ligand with targeted proteins, along with its binding scores, as the internal standard for prioritization of leads and prediction of binding affinities as compared to standard co-crystal ligands for training set compounds (Table 4), test set compounds (Table 5) and external test set compounds (ESI Table 1†). The two crystal structures of the important COVID-19 enzymes, viz. the crystal structure of COVID-19 main protease (Mpro) in complex with an inhibitor N3 (PDB ID 6LU7) and 3CLpro bound to non-covalent inhibitor 1A (PDB ID 4YOI), were identified from the literature and the recently reported 6LU7 was used for docking purposes. The binding of these molecules was analyzed for the binding site conserved residues of these proteins as reported with co-crystal ligands in literature. Although we started our work in December 2019 with PDB ID 4YOI, we selected 6LU7 for this manuscript after an internal comparison of the results from 4YOI which are not reported in this manuscript. The double validation was desired for the targeted proteins to minimize the error in the results. The binding site was assigned according to the active site residues, viz. Phe140, Asn142, Gly143, Cys145, His163, His164, Met165, Glu166, Gln189, and Thr190 for PDB ID 6LU7.
Table 4 The docking scores of the training set compounds under study
Ligand |
MolDock score |
Rerank score |
Docking score |
Similarity score |
Chloroquine |
−114.978 |
−92.5441 |
−261.472 |
−147.734 |
Cycloheximide |
−111.981 |
−95.7982 |
−245.775 |
−129.837 |
Emetine |
−122.442 |
−97.7131 |
−339.048 |
−217.275 |
Exalamide |
−101.31 |
−86.0816 |
−227.935 |
−127.459 |
Hycanthone |
−109.707 |
−95.6088 |
−290.382 |
−177.462 |
Lycorine |
−107.671 |
−75.0677 |
−266.956 |
−149.724 |
Promazin |
−97.0912 |
−81.0416 |
−245.361 |
−149.471 |
Propranalol |
−95.2749 |
−79.7205 |
−243.4 |
−148.829 |
Trilorene |
−129.096 |
−95.8643 |
−317.374 |
−188.474 |
Zoxazolamine |
−68.8333 |
−55.9818 |
−179.447 |
−111.572 |
6LU7 ligand |
−159.941 |
−114.863 |
−8.98507 |
−486.921 |
Table 5 Molecular docking scores of all test set compounds using template docking protocol based on similarity with reference ligand
Ligand |
MolDock score |
Rerank score |
Docking score |
Similarity score |
4-Hydroxy chalcone |
−77.8585 |
−70.1986 |
−265.67 |
−188.48 |
Alprenolol |
−94.2214 |
−79.7208 |
−238.851 |
−143.365 |
Antimycin |
−117.878 |
−104.221 |
−337.716 |
−220.788 |
Berbamine |
−120.311 |
−98.79347 |
−345.907 |
−227.734 |
Phenyl mercuric acetate |
−68.8508 |
−58.4608 |
−184.388 |
−115.875 |
Cetylpyridinium |
−81.407 |
−63.7001 |
−252.532 |
−172.229 |
Chloropyramine |
−92.5222 |
−80.7619 |
−254.611 |
−163.322 |
Conessine |
−98.7959 |
−58.5235 |
−288.742 |
−192.76 |
Desipramine |
−86.5117 |
−72.4371 |
−238.397 |
−153.176 |
Dihydroxy acetyl |
−114.699 |
−43.4549 |
−342.943 |
−229.103 |
Diperidon |
−139.883 |
−116.888 |
−413.704 |
−275.333 |
Doxazosin |
−89.4616 |
−72.7456 |
−268.799 |
−180.266 |
Harmine |
−81.5181 |
−66.3686 |
−213.9 |
−131.439 |
Loperamide |
−87.8964 |
−53.1829 |
−353.971 |
−267.323 |
Monensin |
−137.415 |
−98.1262 |
−407.187 |
−270.843 |
Mycophenoic acid |
−111.679 |
−93.0382 |
−274.334 |
−164.1 |
Mycophenolate |
−134.681 |
−111.598 |
−397.7 |
−263.794 |
Oligomycin |
−74.3467 |
−25.4185 |
−324.684 |
−248.783 |
Papaverine |
−109.986 |
−86.4359 |
−283.187 |
−173.788 |
Phenazopyridine |
−77.551 |
−69.6718 |
−216.598 |
−139.639 |
Pristimerin |
−96.9969 |
−75.2007 |
−316.129 |
−217.741 |
Pyrvinium pamoate |
−151.909 |
−127.372 |
−346.018 |
−193.82 |
Salinomycin sod |
−153.401 |
−123.915 |
−494.644 |
−337.353 |
Terandine |
−104.198 |
−55.1371 |
−325.496 |
−222.293 |
Ticlopidine |
−89.0208 |
−75.621 |
−232.645 |
−144.404 |
Vanilomycin |
−38.4419 |
−11.707 |
−82.0148 |
−43.8819 |
The standard molecules used for development of the pharmacophore model as well as in clinical practices were also docked along with the internal co-crystal ligands to analyze the binding affinity and probable effect on the Mpro or 3CLpro using 6LU7. The binding interactions of these ligands may influence the future for these drugs as probable targets for protein crystallographers. Our study explained very well the binding of these drugs (MolDock scores), comparative binding to a standard ligand (similarity score), binding respective to a co-complexed ligand (docking score), and verified scores through this data (rerank score), along with the binding affinity in kJ mol−1.
The docking run was carried out for all reported compounds used for the development of the pharmacophore model. The MolDock, rerank, docking, and similarity scores are presented in Tables 5 and 6 for the compounds used in the pharmacophore experiments and the virtual screening-identified compounds. The most active compounds from the dataset used in the pharmacophore modeling experiments, lycorene (Fig. 4A) and hycanthon (Fig. 4B), showed hydrogen bond interactions with conserved residues at the binding site of Mpro with HBI with the amino acids Ser143, Gly144, Leu141, His164, His165, and Cys145. The hycanthon showed additional binding interactions with His164. Both of these compounds showed hydrophobic interactions with the amino acids His163, His164, Met165, Glu166, Gln189, and Thr190. The higher binding scores of these molecules are due to their interactions with important amino acids and their hydrophobic interactions with amino acids. The observed lower activities of vanilomycin (Fig. 4C) compared to the other molecules may be due to the large ring structure of the molecule and lower hydrogen bond interactions of the drug with important amino acids. This drug's activity may improve if the ring is broken due to biotransformation, but that is not a matter of study in this manuscript. The top 66 of the top 100 compounds from the FDA-approved drug database were prioritized for docking experiments using the fit value filter (3–4) and were mapped on the pharmacophore model. The top drugs from these 66 docked poses are discussed in the following section.
Table 6 The molecular docking results of the virtual screening-based FDA-approved drugs prioritized molecules identified during the study
Sr no. |
Ligand |
MolDock score |
Rerank score |
Docking score |
Similarity score |
1 |
Aspartame |
−129.954 |
−106.59 |
−274.806 |
−144.96 |
2 |
Fluvoxamine |
−135.771 |
−96.6365 |
−278.138 |
−139.263 |
3 |
Pantoprazole |
−133.092 |
−102.277 |
−331.781 |
−192.732 |
4 |
Torasemide |
−138.501 |
−111.807 |
−299.368 |
−151.745 |
5 |
Pipobroman |
−86.7665 |
−71.4506 |
−188.056 |
−101.898 |
6 |
Ranolazine |
−126.937 |
−103.559 |
−359.856 |
−233.799 |
7 |
Cabergoline |
−168.008 |
−123.317 |
−358.298 |
−188.338 |
8 |
Carmustine |
−86.0684 |
−67.0939 |
−163.787 |
−78.156 |
9 |
Ethambutol |
−95.7324 |
−74.5407 |
−171.837 |
−71.1444 |
10 |
Clobazam |
−92.2753 |
−64.4408 |
−253.209 |
−161.716 |
11 |
Meprobamate |
−89.8846 |
−70.1335 |
−183.89 |
−88.0827 |
12 |
Thiethylperazine |
−129.145 |
−105.519 |
−320.837 |
−193.746 |
13 |
Carisoprodol |
−116.049 |
−92.1891 |
−219.45 |
−97.3266 |
14 |
Sorafenib |
−142.531 |
−113.778 |
−380.394 |
−233.509 |
15 |
Darifenacin |
−54.5361 |
48.8406 |
−318.882 |
−262.529 |
16 |
Cinalukast |
−116.891 |
−62.911 |
−332.984 |
−204.711 |
17 |
Cisapride |
−141.037 |
−116.779 |
−383.177 |
−242.699 |
18 |
Imatinib |
−151.955 |
−126.045 |
−438.477 |
−286.422 |
19 |
Stavudine |
−96.4495 |
−82.0152 |
−219.296 |
−116.038 |
20 |
Pirenzepine |
−105.275 |
−75.9533 |
−302.71 |
−193.117 |
21 |
Loperamide |
−113.212 |
−48.633 |
−380.345 |
−268.168 |
22 |
Donepezil |
−119.174 |
−87.2512 |
−336.186 |
−219.146 |
23 |
Primaquine |
−103.71 |
−85.7877 |
−251.63 |
−146.001 |
24 |
Rabeprazole |
−116.278 |
−95.9976 |
−348.436 |
−232.992 |
25 |
Pioglitazone |
−122.649 |
−99.5867 |
−334.632 |
−207.822 |
26 |
Nefazodone |
−143.332 |
−112.682 |
−448.559 |
−305.528 |
27 |
Propafenone |
−127.166 |
−100.896 |
−325.772 |
−199.7 |
28 |
Domperidone |
−145.189 |
−98.4695 |
−369.321 |
−216.364 |
29 |
Acebutolol |
−129.353 |
−105.281 |
−261.847 |
−131.645 |
30 |
Levomethadyl acetate |
−127.156 |
−116.3751 |
−231.818 |
−175.635 |
31 |
Gemfibrozil |
−102.262 |
−84.5029 |
−234.93 |
−129.442 |
32 |
Oxybenzone |
−93.1873 |
−81.9793 |
−239.662 |
−143.627 |
33 |
Bupranolol |
−104.384 |
−84.311 |
−241.542 |
−138.347 |
34 |
Tofisopam |
−120.390 |
−116.166 |
−288.161 |
−161.36 |
35 |
Oseltamivir |
−116.888 |
−90.6791 |
−269.786 |
−145.391 |
36 |
Niclosamide |
−106.634 |
−86.2728 |
−285.866 |
−180.079 |
37 |
Valsartan |
−146.678 |
−91.319 |
−343.665 |
−196.903 |
38 |
Bortezomib |
−120.396 |
−95.0133 |
−379.268 |
−258.813 |
39 |
Isoetharine |
−101.959 |
−79.664 |
−233.287 |
−126.583 |
40 |
Lovastatin |
−150.987 |
−119.2 |
−338.447 |
−185.958 |
41 |
Gefitinib |
−132.461 |
−109.42 |
−384.446 |
−253.236 |
42 |
Indomethacin |
−138.481 |
−106.98 |
−318.151 |
−179.726 |
43 |
Lansoprazole |
−116.967 |
−92.7688 |
−346.258 |
−230.186 |
44 |
Dipivefrin |
−145.967 |
−114.056 |
−287.587 |
−138.183 |
45 |
Droperidol |
−142.567 |
−114.334 |
−371.802 |
−225.464 |
46 |
Tolmetin |
−108.906 |
−86.7135 |
−257.899 |
−145.77 |
47 |
Bentiromide |
−138.671 |
−120.759 |
−403.212 |
−265.418 |
48 |
Labetalol |
−121.342 |
−98.8951 |
−322.866 |
−203.348 |
49 |
Amodiaquine |
−140.028 |
−112.717 |
−289.1 |
−150.6 |
50 |
Nicardipine |
−144.13 |
−105.635 |
−415.538 |
−267.425 |
51 |
Simvastatin |
−135.57 |
−98.3448 |
−326.099 |
−191.316 |
52 |
Trimethobenzamide |
−122.876 |
−101.48 |
−308.771 |
−187.42 |
53 |
Fluvastatin |
−151.559 |
−116.4 |
−349.643 |
−190.249 |
54 |
Capecitabine |
−127.478 |
−99.999 |
−302.545 |
−175.768 |
55 |
Cilostazol |
−111.214 |
−81.0146 |
−358.573 |
−243.96 |
56 |
Flecainide |
−148.612 |
−116.236 |
−346.253 |
−199.106 |
57 |
Metoclopramide |
−121.99 |
−95.5672 |
−255.64 |
−133.377 |
58 |
Ergonovine |
−129.265 |
−104.514 |
−282.181 |
−151.342 |
59 |
Bambuterol |
−153.331 |
−123.629 |
−290.998 |
−136.86 |
60 |
Alfuzosin |
−139.782 |
−115.967 |
−373.886 |
−235.767 |
61 |
Cinitapride |
−130.586 |
−111.053 |
−352.432 |
−222.269 |
62 |
Ibutilide |
−140.641 |
−97.0092 |
−279.257 |
−128.063 |
63 |
Acetophenazine |
−131.713 |
−106.403 |
−342.675 |
−206.539 |
64 |
Olsalazine |
−144.487 |
−121.151 |
−294.586 |
−147.22 |
65 |
Nebivolol |
−101.377 |
−85.8583 |
−345.029 |
−239.789 |
66 |
Lucanthone |
−111.759 |
−91.616 |
−284.419 |
−169.83 |
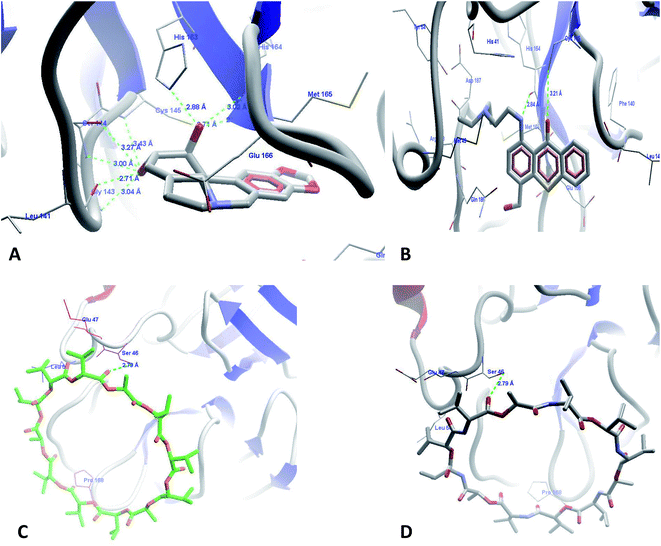 |
| Fig. 4 The molecular docking interactions of (A) lycorene, (B) hycanthone, (C) and (D) vanilomycin with target protein 6Lf. | |
3.4.2 Molecular docking of prioritized drugs. The top predicted compounds from the PBVS were docked in the protein Mpro using the default parameters; the binding site defined in the docking of all compounds was used in the development of the pharmacophore model. The scores from the docking experiments of the FDA-approved drug list are presented in Table 6. The top-scoring drug from this screening was cabergoline, which showed a MolDock score of −168.008 and a rerank score of −123.317. The cabergoline (Fig. 5A) showed hydrogen bond interactions with amino acids at the binding site, viz. Ser144, His163, Glu166, and Cys145. It also showed hydrophobic interactions with His163, His164, Met165, Glu166, Gln189, Thr190, and Glu192. The N-(ethylcarbamoyl)acetamide and N,N-dimethylpropan-1-amine side chains showed hydrophobic interactions with these amino acids while the parent ergoline nucleus with –NH functionality showed hydrogen bond interactions and additional hydrophobic interactions with amino acids like Phe140. The higher binding scores of this drug are due to its higher hydrogen bond interactions and hydrophobic interactions at the binding site of the target proteins. Literature also confirms our findings, as the cabergoline molecule is comprised of a fused indoloquinoline nucleus which has been previously identified as a possible antiviral agent, such as the natural product ‘mapicine ketone’, which may play an important role in inhibiting anti-SARS-CoV-2.33–35
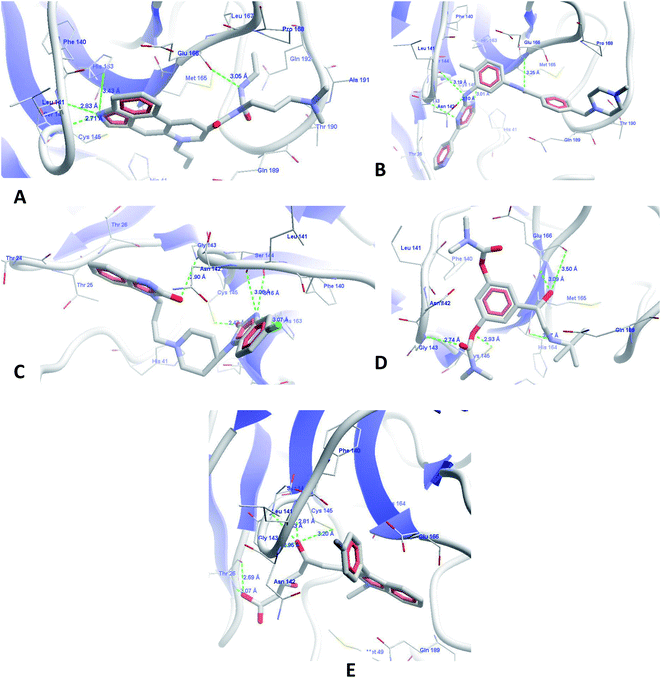 |
| Fig. 5 Molecular docking interactions of (A) cabergoline, (B) imatinib, (C) domperidone, (D) bambuterol and (E) fluvastatin. | |
The next identified FDA approved drug from the database is imatinib (Fig. 5B), which binds with the target protein with a MolDock score of −151.955 and a rerank score of −126.045. The drug showed a cascade of hydrogen bond interactions with Leu141, Ser144, Cys145, His163, and Glu166. It forms good hydrophobic contacts with amino acids Thr26, Phe140, Pro168, Met165, Thr190, Gln189, and Asn142. The aromatic rings of the imatinib form a butterfly-like structure in the binding site for hydrophobic interactions with amino acids. The methylpiperazine ring also showed additional hydrophobic interactions. This finding from our study is well supported from the literature, as imatinib (4-[(4-methylpiperazin-1-yl)methyl]-N-[4-methyl-3-[(4-pyridin-3-ylpyrimidin-2-yl)amino]phenyl]benzamide) is very well reported for SARS-CoV inhibition with an EC50 of 9.823 μM, which ultimately confirms the authenticity of our research and the application of our discovered compounds for SARS-CoV-2 inhibition.36
The next drug identified from screening was domperidone (Fig. 5C) with a MolDock score of −145.189 and a rerank score of −98.4695. The drug showed HBI with Leu141, Gly143, Ser144, Cys145 and His163 and hydrophobic interactions with Thr26, Thr24, Thr25, Met165, and Phe140. The higher scores are representative of the good binding affinity of this drug for Mpro. The benzimidazole nucleus has been documented in literature for antiviral activity against hepatitis C and non-nucleoside reverse transcriptase inhibitors. The chemical structure and our results well support the findings that the drug could be effective in the inhibition of SARS-CoV-2.37–42
Fluvastatin, an HMG-CoA inhibitor, was the next molecule to show higher binding scores (Fig. 5E), with MolDock and rerank scores of −151.559 and −116.4, respectively. The drug also showed similar binding interactions with the amino acids present at the active site along with hydrophobic and pi–pi stacking interactions. The statin class of drugs has also been documented by randomized routes in patients with hepatitis-3; this supports our analysis and raises the probability of an anti-SARS-CoV-2 receptor as per our predictions.43,44
The last drug from our top identified drugs is bambuterol (Fig. 5D) from the PBVS, which also showed higher MolDock and rerank scores of −153.331 and −123.629, respectively. The drug showed HBI with amino acids Leu141, Gly143, Ser144, Cys145, Glu166, and His164. It also showed pi–pi stacking and hydrophobic interactions with Leu27, Phe140, His164, Met165, and Gln189. Although the bambuterol nucleus has not yet been reported for antiviral functions, with regard to our observations and literature, we hope that the drug might have a strong inhibitory effect on SARS-CoV-2.
4. Conclusion
In the current situation of SARS-CoV-2 infection, multiple trials are ongoing on different available drugs, such as antivirals (HIV protease inhibitors). The selection of these drugs is based on a hit and trial basis and no justification is used for the repurposing of these drugs. This approach may be effective initially, but may trigger problems later in relapse or the resistance of viruses to concurrent infections. The validated in silico protocol was therefore established in this study on the basis of a dataset of drugs with known inhibitory potentials for the target pathogen in the micromolar region. The extracted features were used to identify the novel use of existing drugs from the FDA-approved drug database. The study resulted in 66 drugs which were used for various targets with strong binding affinity and interactions at the binding site of the target protein. The top five identified drugs with higher docking scores and fit values, viz. a MolDock score of −168.008 and rerank scores of −123.317 to 145.189 to −98.46, may be promising compounds. The top leads from this data, D2 receptor agonist cabergoline (fused indoloquinoline nucleus), tyrosine kinase inhibitor imatinib (4-[(4-methylpiperazin-1-yl)methyl]-N-[4-methyl-3-[(4-pyridin-3-ylpyrimidin-2-yl)amino]phenyl]benzamide), D2 receptor antagonist domperidone (benzimidazole nucleus), HMG-CoA inhibitor fluvastatin (E,3R,5S)-7-[3-(4-fluorophenyl)-1-propan-2-ylindol-2-yl]-3,5-dihydroxyhept-6-enoic acid), and beta 2 adenoreceptor agonist bambuterol ([3-[2-(tert-butylamino)-1-hydroxyethyl]-5-(dimethylcarbamoyloxy)phenyl]N,N-dimethylcarbamate), showed the highest potential to inhibit the virus through Mpro. Such top-scoring compounds have a high probability of inhibiting Mpro. Top identified drugs in our study, such as imatinib, have already been documented for SARS-CoV inhibition at 9.823 μM, which further validates our analysis and results and improves the probability of drug repurposing, validating our procedure.
Conflicts of interest
The authors claim that the researchers in this study have no conflict of interest.
Acknowledgements
The authors would like to acknowledge the support of the King Khalid University through a grant RCAMS/KKU/001/20 under the Research Center for Advanced Materials Science at King Khalid University, Saudi Arabia. The authors are also like to acknowledge BIOVIA for granting us SARS-CoV-2 Discovery Studio Academic Research License Suite for the project work.
References
- A. S. Agrawal, T. Garron, X. Tao, B. H. Peng, M. Wakamiya, T. S. Chan, R. B. Couch and C. T. Tseng, J. Virol., 2015, 89, 3659–3670 CrossRef CAS.
- Z. Zhao, F. Zhang, M. Xu, K. Huang, W. Zhong, W. Cai, Z. Yin, S. Huang, Z. Deng, M. Wei, J. Xiong and P. M. Hawkey, J. Med. Microbiol., 2003, 52, 715–720 CrossRef CAS.
- WHO organization, Weekly Operational Update on COVID-19, 2020 Search PubMed.
- S. Pushpakom, F. Iorio, P. A. Eyers, K. J. Escott, S. Hopper, A. Wells, A. Doig, T. Guilliams, J. Latimer, C. McNamee, A. Norris, P. Sanseau, D. Cavalla and M. Pirmohamed, Nat. Rev. Drug Discovery, 2019, 18, 41–58 CrossRef CAS.
- C. Liu, Q. Zhou, Y. Li, L. V. Garner, S. P. Watkins, L. J. Carter, J. Smoot, A. C. Gregg, A. D. Daniels, S. Jervey and D. Albaiu, ACS Cent. Sci., 2020, 6, 315–331 CrossRef CAS.
- C. A. Devaux, J. M. Rolain, P. Colson and D. Raoult, Int. J. Antimicrob. Agents, 2020, 55, 105938 CrossRef CAS.
- E. De Clercq and G. Li, Clin. Microbiol. Rev., 2016, 29, 695–747 CrossRef.
- A. M. Sayed, A. R. Khattab, A. M. AboulMagd, H. M. Hassan, M. E. Rateb, H. Zaid and U. R. Abdelmohsen, RSC Adv., 2020, 10, 19790–19802 RSC.
- A. T. Ton, F. Gentile, M. Hsing, F. Ban and A. Cherkasov, Mol. Inf., 2020, 39(8), e2000028 CrossRef.
- A. B. Gurung, M. A. Ali, J. Lee, M. A. Farah and K. M. Al-Anazi, Life Sci., 2020, 117831 CrossRef CAS.
- T. Huynh, H. Wang and B. Luan, J. Phys. Chem. Lett., 2020, 11(11), 4413–4420 CrossRef CAS.
- O. Altay, E. Mohammadi, S. Lam, H. Turkez, J. Boren, J. Nielsen, M. Uhlen and A. Mardinoglu, Iscience, 2020, 101303 CrossRef CAS.
- P. K. Panda, M. N. Arul, P. Patel, S. K. Verma, W. Luo, H.-G. Rubahn, Y. K. Mishra, M. Suar and R. Ahuja, Sci. Adv., 2020, 6, eabb8097 CrossRef.
- S. Varshney, K. Shankar, M. Beg, V. M. Balaramnavar, S. K. Mishra, P. Jagdale, S. Srivastava, Y. S. Chhonker, V. Lakshmi and B. P. Chaudhari, J. Lipid Res., 2014, 55, 1019–1032 CrossRef CAS.
- S. Srivastava, R. Sonkar, S. K. Mishra, A. Tiwari, V. Balramnavar, S. Mir, G. Bhatia, A. K. Saxena and V. Lakshmi, Lipids, 2013, 48, 1017–1027 CrossRef CAS.
- S. Satish, A. Srivastava, P. Yadav, S. Varshney, R. Choudhary, V. M. Balaramnavar, T. Narender and A. N. Gaikwad, Eur. J. Med. Chem., 2018, 143, 780–791 CrossRef CAS.
- S. Rajan, S. Satish, K. Shankar, S. Pandeti, S. Varshney, A. Srivastava, D. Kumar, A. Gupta, S. Gupta and R. Choudhary, Metabolism, 2018, 85, 1–13 CrossRef CAS.
- A. Gupta, V. K. Singh, D. Kumar, P. Yadav, S. Kumar, M. Beg, K. Shankar, S. Varshney, S. Rajan and A. Srivastava, Metabolism, 2017, 73, 109–124 CrossRef CAS.
- V. M. Balaramnavar, R. Srivastava, N. Rahuja, S. Gupta, A. K. Rawat, S. Varshney, H. Chandasana, Y. S. Chhonker, P. K. Doharey and S. Kumar, Eur. J. Med. Chem., 2014, 87, 578–594 CrossRef CAS.
- V. M. Balaramnavar, I. A. Khan, J. A. Siddiqui, M. P. Khan, B. Chakravarti, K. Sharan, G. Swarnkar, N. Rastogi, H. Siddiqui and D. P. Mishra, J. Med. Chem., 2012, 55, 8248–8259 CrossRef CAS.
- K. Ahmad, V. M. Balaramnavar, M. H. Baig, A. K. Srivastava, S. Khan and M. A. Kamal, CNS Neurol. Disord.: Drug Targets, 2014, 13, 1346–1353 CrossRef CAS.
- K. Ahmad, V. M. Balaramnavar, N. Chaturvedi, S. Khan, S. Haque, Y.-H. Lee and I. Choi, Molecules, 2019, 24, 1827 CrossRef.
- P. W. Sprague, Perspect. Drug Discovery Des., 1995, 3, 1–20 CrossRef CAS.
- B. R. Brooks, C. L. Brooks, 3rd, A. D. Mackerell, Jr., L. Nilsson, R. J. Petrella, B. Roux, Y. Won, G. Archontis, C. Bartels, S. Boresch, A. Caflisch, L. Caves, Q. Cui, A. R. Dinner, M. Feig, S. Fischer, J. Gao, M. Hodoscek, W. Im, K. Kuczera, T. Lazaridis, J. Ma, V. Ovchinnikov, E. Paci, R. W. Pastor, C. B. Post, J. Z. Pu, M. Schaefer, B. Tidor, R. M. Venable, H. L. Woodcock, X. Wu, W. Yang, D. M. York and M. Karplus, J. Comput. Chem., 2009, 30, 1545–1614 CrossRef CAS.
- B. R. Brooks, R. E. Bruccoleri, B. D. Olafson, D. J. States, S. a. Swaminathan and M. Karplus, J. Comput. Chem., 1983, 4, 187–217 CrossRef CAS.
- G. W. Milne, M. C. Nicklaus, J. S. Driscoll, S. Wang and D. Zaharevitz, J. Chem. Inf. Comput. Sci., 1994, 34, 1219–1224 CrossRef CAS.
- J. J. Irwin and B. K. Shoichet, J. Chem. Inf. Model., 2005, 45, 177–182 CrossRef CAS.
- R. Thomsen and M. H. Christensen, J. Med. Chem., 2006, 49, 3315–3321 CrossRef CAS.
- D. K. Gehlhaar, D. Bouzida and P. A. Rejto, Fully automated and rapid flexible docking of inhibitors covalently bound to serine proteases, in International Conference on Evolutionary Programming, Springer, Berlin, Heidelberg, 1998, pp. 449–461 Search PubMed.
- S. E. S. John, S. Tomar, S. R. Stauffer and A. D. Mesecar, Bioorg. Med. Chem., 2015, 23, 6036–6048 CrossRef.
- Z. Jin, X. Du, Y. Xu, Y. Deng, M. Liu, Y. Zhao, B. Zhang, X. Li, L. Zhang, C. Peng, Y. Duan, J. Yu, L. Wang, K. Yang, F. Liu, R. Jiang, X. Yang, T. You, X. Liu, X. Yang, F. Bai, H. Liu, X. Liu, L. W. Guddat, W. Xu, G. Xiao, C. Qin, Z. Shi, H. Jiang, Z. Rao and H. Yang, Nature, 2020, 582, 289–293 CrossRef CAS.
- L. Shen, J. Niu, C. Wang, B. Huang, W. Wang, N. Zhu, Y. Deng, H. Wang, F. Ye, S. Cen and W. Tan, J. Virol., 2019, 93(12), e00023-19 CrossRef.
- P. Das, X. Deng, L. Zhang, M. G. Roth, B. M. A. Fontura, M. A. Philips and J. K. De Brabander, ACS Med. Chem. Lett., 2013, 4(6), 517–521 CrossRef CAS.
- I. Pendrak, S. Barney, R. Wittrock, D. M. Lambert and W. D. Kingsbury, J. Org. Chem., 1994, 59(9), 2623–2625 CrossRef CAS.
- R. A. Mekheimer, M. A. Al-Sheikh, H. Y. Medrasi and K. U. Sadek, RSC Adv., 2020, 10, 19867–19935 RSC.
- J. Dyall, C. M. Coleman, B. J. Hart, T. Venkataraman, M. R. Holbrook, J. Kindrachuk, R. F. Johnson, G. G. Olinger Jr., P. B. Jahrling, M. Laidlaw, L. M. Johansen, C. M. Lear-Rooney, P. J. Glass, L. E. Hensley and M. B. Frieman, Antimicrob. Agents Chemother., 2014, 58, 4885–4893 CrossRef.
- Y. Luo, J. P. Yao, L. Yang, C. L. Feng, W. Tang, G. F. Wang, J. P. Zuo and W. Lu, Bioorg. Med. Chem., 2010, 18(14), 5048–5055 CrossRef CAS.
- R. Zou, K. R. Ayres, J. C. Drach and L. B. Townsend, J. Med. Chem., 1996, 39(18), 3477–3482 CrossRef CAS.
- M. Tonelli, G. Paglietti, V. Boido, F. Sparatore, F. Marongiu, E. Marongiu, P. L. Colla and R. Loddo, Chem. Biodiversity, 2008, 5(11), 2386–2401 CrossRef CAS.
- A. M. Monforte, A. Rao, P. Logoteta, S. Ferro, L. D. Luca, M. L. Barreca, N. Iraci, G. Maga, E. D. Clercq, C. Pannecouque and A. Chimirri, Bioorg. Med. Chem., 2008, 16(15), 7429–7435 CrossRef CAS.
- M. Tonelli, M. Simone, B. Tasso, F. Novelli, V. Boido, F. Sparatore, G. Paglietti, S. Pricl, G. Giliberti, S. Blois, C. Ibba, G. Sanna, R. Loddo and P. L. Colla, Bioorg. Med. Chem., 2010, 18(8), 2937–2953 CrossRef CAS.
- T. Bader, J. Fazili, M. Madhoun, C. Aston, D. Hughes, S. Rizvi, K. Seres and M. Hasan, Am. J. Gastroenterol., 2008, 103(6), 1383–1389 CrossRef CAS.
- D. A. Sheridan, S. H. Bridge, M. M. E. Crossey, D. J. Felmlee, F. I. Fenwick, H. C. Thomas, R. D. G. Neely, S. D. Taylor-Robinson and M. F. Bassendine, Liver Int., 2014, 34(5), 737–747 CrossRef CAS.
- T. Bader, L. D. Hughes, J. Fazili, B. Frost, M. Dunnam, A. Gonterman, M. Madhoun and C. E. Aston, J. Viral Hepatitis, 2013, 20(9), 622–627 CrossRef CAS.
Footnote |
† Electronic supplementary information (ESI) available. See DOI: 10.1039/d0ra06038k |
|
This journal is © The Royal Society of Chemistry 2020 |