DOI:
10.1039/D0RA05267A
(Paper)
RSC Adv., 2020,
10, 29675-29681
Production of high-complexity frameshift neoantigen peptide microarrays†
Received
15th June 2020
, Accepted 2nd August 2020
First published on 11th August 2020
Abstract
Parallel measurement of large numbers of antigen–antibody interactions are increasingly enabled by peptide microarray technologies. Our group has developed an in situ synthesized peptide microarray of >400
000 frameshift neoantigens using mask-based photolithographic peptide synthesis, to profile patient specific neoantigen reactive antibodies in a single assay. The system produces 208 replicate mircoarrays per wafer and is capable of producing multiple wafers per synthetic lot to routinely synthesize over 300 million peptides simultaneously. In this report, we demonstrate the feasibility of the system for detecting peripheral-blood antibody binding to frameshift neoantigens across multiple synthetic lots.
1 Introduction
Highly sensitive and highly specific blood-based assays for cancer detection are a much sought after diagnostic goal. Analysis of antibody–antigen interactions via a peptide microarray has a long history using low density, medium density, and high density peptide microarrays.1–22 Recently, our group has demonstrated that in cancer, the normal RNA transcription and splicing error correction mechanisms are less effective leading to an increase in aberrant peptide production including frameshift antigens (FSAs).13,23 The FSAs have been found to be highly immunogenic cancer neoantigens, that can elicit both T cell and antibody responses and drive effective anti-tumor immune responses.24–27 The errors in RNA transcription and splicing significantly affect the transcriptome of cancer cells. Unlike neoantigens from DNA mutations, there are many shared FSA neoantigens resulting from RNA errors, and these shared neoantigens generate antibodies that can be detected by FS peptide (FSP) arrays.13,23 We have shown that FSAs have potential use in therapeutic cancer vaccines.13,23,26 While the high density (HD) peptide microarrays produced by light directed peptide synthesis using Roche-Nimblegen Maskless Array (NMA) synthesis is a powerful discovery system, it is difficult to produce large numbers of peptide microarrays for large scale antigen discovery or diagnostic development.17 Our group has been producing HD peptide microarrays for a number of years using photolithographic peptide synthesis in which UV light generates a photo-acid at an exposed spot for peptide chemistry.28 Due to the promising results on the NMA peptide microarrays to identify antibodies that were reactive against neoantigens, we developed a photolithographic synthesis system that produces 208 HD peptide microarrays of 409
600 peptides per microarray. This neoantigen peptide library can be used to comprehensively profile a patient's antibody binding profile towards the possible FSAs that can arise from RNA-based errors. The FSAs are synthesized as 15-mer FSPs that span the full-length antigen, using all 20 amino acids (aa). This requires the use of 300 photolithographic masks, a noted challenge of photolithographic peptide array production systems.3 We show that we can synthesize a library of this complexity at a scale compatible with the development of a diagnostic assay. We demonstrate that this system can be used to produce large numbers of peptide microarrays. We then evaluated the performance of the system by screening serum from a single donor over many replicate peptide arrays.
2 Materials and methods
2.1 Materials and reagents
The following reagents were used as received from the supplier: (3-glycidyloxypropyl)trimethoxysilane (GPTMS), N-methyl-2-pyrrolidine (NMP), 1-hydroxybenzotriazole (HOBt), diisopropylcarbodiimide (DIC), poly methyl methacrylate (PMMA), propylene glycol methyl ether acetate (PGMEA), isopropanol (IPA), dimethylformamide (DMF), trifluoroacetic acid (TFA), methanol (MeOH), dichloromethane (DCM), methoxyethoxy acetic acid (MEAA), trifluoromethanesulfonic acid (TFMSA).
2.2 Peptide microarray synthesis
Silicon wafers are etched (Alta Microtec, Los Gatos, CA) with alignment marks for photomask alignment and dicing marks for dicing each wafer into 13 slides in which each slide contains 16 identical peptide microarrays. The wafer is polished and thermally oxidized, resulting in a 250 nm-thick silicon oxide layer. Wafers are plasma-cleaned and derivatized by chemical vapor deposition of GPTMS. The epoxy-functionalized surface is further modified with a solution of 4,7,10-trioxa-1,13-tridecanediamine (Sigma Aldrich, St. Louis, MO) in NMP, at 60 °C overnight, resulting in a layer of primary and secondary amines for peptide synthesis. The amino groups are coupled with a solution of Boc-glycine/HOBt/DIC in NMP and different density peptide surfaces are produced at this step through addition of a mixture of Boc-Gly and MEAA. Based on the library of sequences being synthesized, photomasks are selected in a predetermined order and the amino acid–mask pairs are assigned accordingly. The arrays are fabricated on a P8000 track system (C&D Semiconductor, San Jose, CA) that consists of (i) a spin-coater with a heated-chuck for post exposure baking, photoresist stripping, amino acid coupling, and removal of coupling solution; (ii) a chill plate to cool the wafer to room temperature; (iii) a spin-coater for photoresist coating; and (iv) a hot plate for pre-exposure baking. The arrays are photo-activated at each cycle using a model 800 mask aligner (OAI, Inc, Milpitas, CA). The photoresist solution used in this synthesis contains a photo acid generator, a sensitizer, and PMMA in PGMEA.
In a cycle of synthesis, the wafer surface is washed first on the spin-coater (i) with NMP, acetone, and then IPA before the photoresist solution is applied to the surface on spin-coater (ii). The wafer is then baked on the hotplate at 80 °C, cooled, and placed onto the mask aligner for alignment and UV exposure to deprotect the Boc groups from the exposed peptide features. After exposure, the wafer is baked for 90 seconds at 80 °C on the spin-coater (i) to ensure complete deprotection of Boc-groups from patterned features. A coupling solution is prepared that contains each amino acid, HOBt, and DIC in NMP. After post-exposure baking and photoresist removal on spin-coater (i), the activated amino acid solution is added to the wafer surface and coupled while the wafer is on spin-coater (i). A cycle of synthesis is complete after the coupling solution is washed off with NMP, acetone, and then IPA. Complete fabrication of a wafer of 208 peptide microarrays takes 300 synthetic cycles. At the completion of synthesis, the completed wafer is diced into slides (Advotech, Tempe, AZ).
A “low-high TFMSA” method is used to remove the side chain protection groups.29 The slides are placed in a glass slide rack in a glass container, washed with DMF, acetone, and IPA. The slides are each dried with a stream of nitrogen and placed back in a glass slide rack in a glass container. The container is then chilled in an ice bucket, the low cleavage solution (50% TFA, 30% DMS, 10% TFMSA, 8% m-cresol, 2% EDT), made in a separate ice bucket, is added to the container and shaken for 3 hours at 0–5 °C. The slides are washed with MeOH, dried with nitrogen, and treated in the same container with the high cleavage solution (83.25% TFA, 4.25% thioanisol, 2.5% EDT, and 10% TFMSA) for 1.5 hours at RT. The slides are then washed with MeOH, DCM, IPA thoroughly and dried with nitrogen before use in antibody binding assays.
2.3 Serum collection
The collection and use of all human serum for research presented here was approved by the Institutional Review Board of Arizona State University (ASU), protocol No. 0912004625. Informed consent was obtained from all human subjects. Blood was collected from a healthy donor and the serum was separated.
2.4 Antibody binding assay
Antibody binding assays are performed at room temperature. Generally, arrays were washed in 1× PBST for 30 minutes and then incubated with blocking/dilution buffer for 30 minutes. Arrays were incubated with 1
:
100 diluted serum overnight at room temperature. After serum incubation, the arrays were washed 3× in 1× PBST, 10 minutes per wash. Serum IgG binding was detected by Cy3-conjugated goat anti-human secondary antibody (Jackson ImmunoResearch Cat#109-165-098). Arrays were incubated with 4 nM secondary antibody in 0.75% casein/PBST for 2 hours, washed 3× in 1× PBST for 10 minutes per wash, 2 minutes each in 40% and 100% isopropanol and then dried by centrifuging at 800 RPM for 2 minutes. Fluorescent signal of the secondary antibody was detected by scanning with an Innoscan 910 (Innopsys, France). Images were grid with Mapix (Innopsys) to extract the raw RFU values for each peptide and these data were exported into a SAS database. The resulting data was analysed in JMP and figures were prepared using GraphPad Prism or JMP.
3 Results and discussion
3.1 High density FSP microarray production
The 409
600 peptide library that was designed as 15aa long peptides is composed from all 20 amino acids and requires a total of 300 synthetic steps with one photolithographic mask per step. The peptide library was designed to analyze antibody binding to the 190
865 FSAs that are predicted to arise from transcriptional errors at microsatellite loci (n = 7452) and from exon splicing errors (n = 174
756) as well as 8657 predicted FSAs from the canine transcriptome. Predicted FSAs that are longer than 15aa were synthesized as non-overlapping 15-mers. For example, for a 32 amino acid predicted FSA, there would be two FSPs synthesized on the peptide microarray: FSP1 (1–15aa) and FSP2 (16–30aa). This library is designed to maximize the number of total FSAs that can be screened against a single sample rather than on a more comprehensive analysis of antibody reactivity that would be possible using a tiled peptide array in which there are overlapping amino acids.
While the peptide microarray synthesis is technically challenging, the photolithographic synthesis process produces 208 replicate peptide microarrays in a single wafer for a total of over 85 million peptides synthesized per run. The wafer is designed such that 16 replicate arrays are arranged in a microscope slide-like format enabling processing using standard laboratory equipment. Due to the small feature size (8 micron × 8 micron), analysis of the synthetic yield of each peptide is not performed (Fig. 1). Additionally, we chose not to sample a subset of peptides for additional analysis as it is difficult to generalize observations across 409
000 peptides of significant sequence diversity. Boc chemistry was used with procedures described in our previous work.28 However, for this library, we used all 20 amino acids rather than the reduced amino acid sets favored in our previous designs.
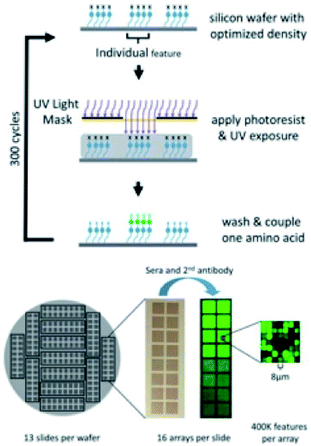 |
| Fig. 1 Schematic of photolithographic peptide synthesis process (top) and peptide microarrays produced per silicon wafer (bottom). | |
3.2 Analysis of dose responsive antibody binding to FSP microarrays
Our previous HD peptide microarrays have used high amine surface density to produce highly avid surfaces in order to maximize capture of antibodies that recognize mimotopes, otherwise known as the “immunosignature effect”.30–32 However, in order to reduce the surface avidity, a reduced amine density surface was prepared through the first step coupling of a mixture of Boc-Gly and MEAA in place of the standard Boc-Gly coupling. This reduces the number of start-points for peptide synthesis, effectively reducing potential surface avidity effects. This should enable the measurement of antibody–peptide interactions under conditions close to the 1
:
1 binding regime. This is a necessary requirement for identification of the target peptide antigen and not of a lower affinity mimotope.
To assess the performance of the less avid peptide microarray surface, we screened a serum sample from a healthy donor (ND30) using a method similar to our previously published procedures,13,23 with a few key changes. First, the assay incubation time was increased from one hour to overnight to allow high affinity peptide–antibody interactions to approach a binding equilibrium.33,34 Next, an IgG specific, anti-Fc secondary antibody was used to ensure measurement of only IgG binding. This is important as different immunoglobulins can have different roles in the anti-tumor immune response.35 Finally, the neoantigen reactive IgG we intend to measure are predicted to be infrequent and of low concentration, therefore we increased the serum concentration well above what is typically used for immunosignature (IMS) analysis.36 As such, we diluted the serum sample from 1
:
100 to 1
:
21
600 times and ran each dilution on triplicate microarrays in order to evaluate the dose responsiveness of antibody–antigen binding (Fig. 2a). Examination of the cell plot in which each peptide (y-axis) is plotted versus serum dilution (x-axis) demonstrates that the vast majority of peptides do not bind (blue) but observed binding (red) is reproducible across peptide microarrays as demonstrated by correlation analysis between replicate arrays (Fig. 2b). This contrasts with IMS peptide arrays in which a large portion of the peptide microarray binds with a broad range of intensities.36 This difference becomes more clear when the relative fluorescence units (RFU) for each peptide at each serum dilution was averaged and the maximum signal observed on each peptide array, the 99.5 percentile and median RFU of the entire array were plotted as a function of sample dilution (Fig. 2c). As can be seen, the average maximum observed signal approached detector saturation (65
536 RFU) at 1
:
100 dilution and the 99.5 percentile signal of the top 2048 peptides increased while the full array median was not significantly changed. These data demonstrate that the peptide microarray can capture dose-responsive antibody-antigen binding and that the majority of signal is low-level and non-specific. These data suggest that the high complexity peptide array has much lower surface avidity than our previous peptide microarrays.
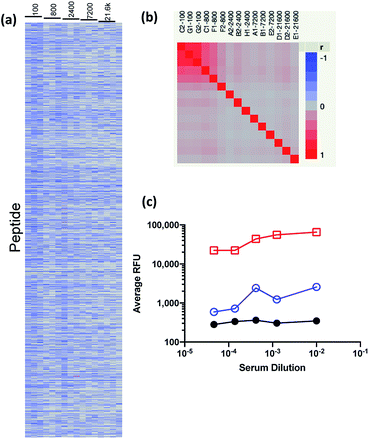 |
| Fig. 2 Analysis of normal donor serum on 409 600 peptide microarray. (a) Cell plot of each peptide array (column) by each peptide (row) where red indicates high binding and blue indicates low binding. (b) Correlation between replicate peptide arrays versus serum dilution. Red indicates high correlation and blue indicates low correlation. (c) The maximum observed signal (red squares), 99.5 percentile (blue circles) and the peptide array median (black circles) for the averaged RFU as a function of serum dilution. | |
Next, we examined IgG binding on a per peptide basis to identify peptides that exhibited dose-responsive binding. The average RFU per peptide at 1
:
100 (y-axis) was plotted versus the average RFU at 1
:
800 (x-axis) to identify peptides with significant binding at both dilutions (Fig. 3a). The binding for each peptide at 1
:
2400 dilution was overlaid (color scale) and it can be seen that peptides that were high binding in 1
:
100 and 1
:
800 dilutions were the highest binding peptides at 1
:
2400. The mean RFU of a cohort of these peptides were plotted as a function of serum dilution and it was found that they were indeed dose responsive (Fig. 3b).
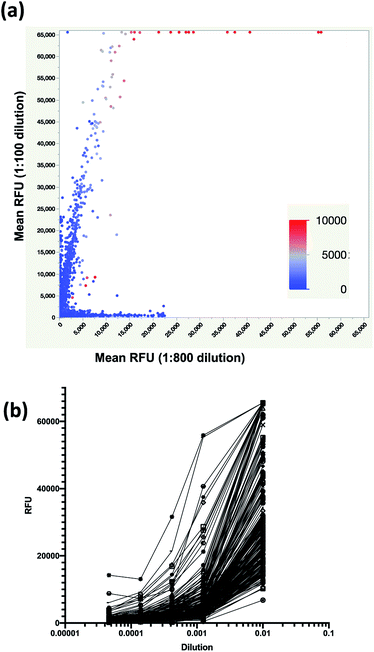 |
| Fig. 3 Identification of dose responsive peptides from a serum sample. (a) Scatterplot of mean RFU of triplicate arrays for 1 : 100 (y-axis) versus 1 : 800 (x-axis) dilution with each data point colored by mean RFU for the 1 : 2400 dilution (color bar) where red indicates high binding and blue indicates low binding. (b) Average RFU from triplicate arrays as a function of serum dilution for 225 dose responsive peptides. | |
3.3 Peptide surface density study
We next explored the effect of peptide surface avidity using a modified synthetic protocol in which a single silicon wafer was prepared with four different amine densities separated into quadrants. Each array was designed such that only two unique peptide sequences were synthesized yet replicated several thousand times per peptide array. This experimental design produced over 4700 copies each of two peptides: APLARPRSRPAPAA and the closely related APLRRGRSWIMPSSF. These peptides were identified from the experiment described in Fig. 3. Upon completion of the wafer synthesis, ND30 was applied at the same dilution series as before to one slide from each amine surface density. Data from replicate peptides were averaged and the RFU for each peptide was plotted as a function of dilution and amine surface density (Fig. S1†). The peptide arrays produced on surfaces with lower amine densities, had lower signals and exhibited dose dependent binding as expected. The avidity effect of the high density surface becomes apparent at lower serum dilutions, which is expected as this is the same surface density previously reported for the avidity driven immunosignature effect.30 The 1/10 density surface did not show the avidity driven binding that was evident on the high-density surface and confirms that the peptide surface used in Fig. 2 and 3 has negligible avidity driven effects. This 1/10 density surface was then chosen for further studies of peptide synthesis and assay reproducibility.
3.4 Peptide array reproducibility across synthesis lots
As antibody–antigen microarrays suffer from a number of well-known reproducibility limitations due to mixing fluidics and antibody–antigen binding kinetics,33,34 we examined the distribution of binding values for replicate arrays at 1
:
100, 1
:
800, and 1
:
2400 dilution for both peptide sequences and observed a large spread in measured fluorescence values (Fig. 4a). We then examined binding as a function of peptide microarray column and found that there is some positional heterogeneity in the binding observed that is likely caused by imperfect mixing within the gasketed microarray assay chamber. The lowest binding is observed near the margins of the peptide microarray which are the replicate spots that are closest to the array gasket (Fig. 4b and c). This indicates that there is reduced mixing near the gasket. These observations are in line with other reports and justify further assay development to eliminate the limitations in mixing of the current assay protocol.
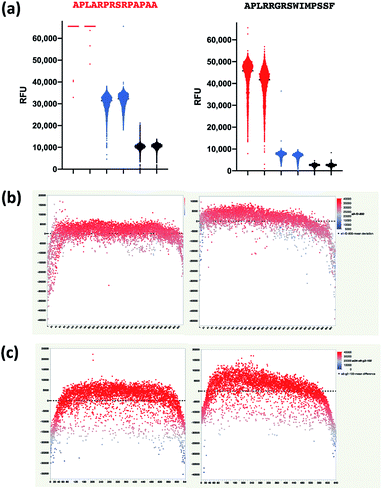 |
| Fig. 4 Mixing effects in IgG–peptide binding. (a) Distribution of RFU values for 4752 replicate spots of APLARPRSPAPAA (left) and 4702 spots of APLRRGRSWIMPSSF (right) for 1 : 100 dilution (red), 1 : 800 dilution (blue), and 1 : 2400 dilution (black). Difference in RFU from the array mean for each replicate peptide (y-axis) versus column position within the microarray (x-axis) at (b) the 1 : 800 dilution for APLARPRSRPAPAA and (c) the 1 : 100 dilution for APLRRGRSWIMPSSF. Each spot is coloured according to its RFU. | |
However, with this limitation in mind, we evaluated the performance of the 409
600 peptide microarray across multiple synthetic runs. We synthesized four additional wafers for a total of 832 replicate microarrays and then applied serum from ND30 at 1
:
100 dilution to a total of 18 replicate peptide microarrays. The mean RFU and standard deviation for each peptide-feature was calculated and is shown in Fig. 5. A simple linear regression of the standard deviation against the mean yields a line with a slope of 0.948× and an R2 of 0.699, indicating that most peptides do not have reproducible binding. However, as the mean signal increases, the standard deviation decreases demonstrating that high affinity binding on the arrays is reproducible.
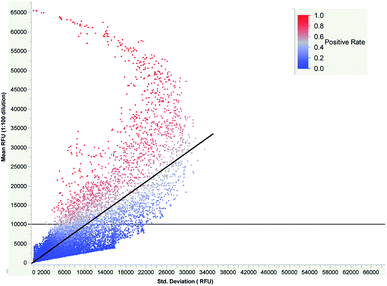 |
| Fig. 5 Plot of peptide mean RFU (y-axis) across 18 arrays versus the standard deviation (x-axis) for 4 096 000 peptides. The positive rate of each peptide is indicated on the color scale bar from 100% positive (red) down to 0% positive (blue). | |
This neoantigen peptide microarray is designed to detect whether a patient sample is positive for an IgG that reacts with a given FSP. Given the reproducibility observed in Fig. 5, we sought to set a threshold feature signal-intensity to score a peptide as positive for IgG binding. We constructed a simple binding model of 1
:
1 antibody–peptide binding under equilibrium conditions, which might not be the case for every peptide under the current 17 hour assay incubation time,33 to estimate expected binding that would be observed. If a single IgG clone has a KD = 100 pM and a concentration of 25 pM in the dilute serum sample, estimated from reported breast cancer autoantibody concentrations,37 the expected RFU would be ∼13
000 RFU (Fig. S4†). Guided by this, we set a peptide binding threshold of 10
000 RFU and scored a peptide as positive if it exceeded this threshold on a peptide microarray. We then calculated the positive rate by dividing the number of times the peptide was positive by the total number of arrays and overlaid the peptide positive rate on Fig. 5. As can be seen, the peptides that had high positive rates, generally had mean RFU > 10
000 and standard deviations that were less than 50% RSD. When this data was examined in light of the imperfect mixing that occurs within a peptide microarray assay chamber, it was found that the peptides that were positive in 16 out of 18 arrays were evenly distributed positionally within the microarray (Fig. S5†). These data demonstrate that despite the non-optimal fluidics in the current assay system, that the reproducibility of antibody–peptide binding across synthetic runs is sufficiently reproducible to achieve biologically informative results.
4 Conclusions
In this work we demonstrated that photolithographic synthesis could be used to produce a library of 15 mer peptides using all 20 amino acids and that these microarrays could be used to probe antibody reactivity against peptides corresponding to FS neoantigens. While the system reproducibility calls for improvement in the technology development cycle, this work does highlight a number of important points about the system: first, the microarray cassette assay demonstrates a high level of positional bias due to imperfect mixing. This limitation could be overcome with a number of modifications to the assay. Transition to a larger volume, microwell based system should reduce the observed mixing driven artifacts and there are a number of approaches that have been reported to improve microarray assay mixing that should be relevant to this system. Second, the clear impact of peptide amine density on binding suggests that further refinements can be made to the array surface to increase affinity driven antibody binding while maintaining low levels of avidity driven binding. Third, the amount of peptide synthesized per feature is far less than the amount of antibody in solution for those peptides with saturated binding. This is demonstrated by the two-peptide experiment in which despite there being 4700 copies of the peptide present versus the standard peptide microarray, all copies of the highest affinity peptide (APLARPRSRPAPAA) were saturated at the 1
:
100 dilution. If this IgG clone was present at low concentrations, one would expect the signal in the two-peptide microarray to decrease if it was depleted from solution. Finally, one of the powers of the photolithographic peptide synthetic system is the large numbers of peptide microarrays enable more detailed studies of assay performance. Simple dose response studies are easily accessible due to the large number of replicate microarrays produced. New experimental microarrays can be produced from a single set of photolithographic masks to guide platform improvement. In summary, this report suggests that it is possible to produce hundreds to thousands of reproducible FS peptide microarrays that could enable cancer neoantigen discovery and potentially diagnostic development with further platform development.
Conflicts of interest
S. A. J. and L. S. hold patents and pending patents with respect to frameshift antigens and FSP arrays. S. A. J. is a founder of Calviri which is commercializing these diagnostic arrays. K. S. and J. R. B. are employees of Calviri. All authors have ownership interests in Calviri.
Acknowledgements
This work was supported by a sponsored research agreement from Calviri, Inc and from funds from the Peptide Array Core in the Center for Innovations in Medicine at Arizona State University. The authors thank James Boyd at HealthTell for synthesis of the peptide arrays used for the surface density studies.
Notes and references
- C. A. Parada, J. Osbun, S. Kaur, Y. Yakkioui, M. Shi, C. Pan, T. Busald, Y. Karasozen, L. F. Gonzalez-Cuyar, R. Rostomily, J. Zhang and M. Ferreira, Sci. Rep., 2018, 8, 2098 CrossRef PubMed.
- J. Atwater, D. S. Mattes, B. Streit, C. Von Bojničić-Kninski, F. F. Loeffler, F. Breitling, H. Fuchs and M. Hirtz, Adv. Mater., 2018, 30, 1801632 CrossRef PubMed.
- D. S. Mattes, N. Jung, L. K. Weber, S. Bräse and F. Breitling, Adv. Mater., 2019, 31, 1806656 CrossRef PubMed.
- D. S. Mattes, S. Rentschler, T. C. Foertsch, S. W. Münch, F. F. Loeffler, A. Nesterov-Mueller, S. Bräse and F. Breitling, Small Methods, 2018, 2, 1700205 CrossRef.
- M.-J. Garcia-Bonete, M. Jensen, C. V. Recktenwald, S. Rocha, V. Stadler, M. Bokarewa and G. Katona, Sci. Rep., 2017, 7, 16816 CrossRef PubMed.
- A. Lingel, B. L. Bullard and E. A. Weaver, Sci. Rep., 2017, 7, 14912 CrossRef PubMed.
- V. Qendro, D. H. Lundgren, S. Palczewski, P. Hegde, C. Stevenson, L. Perpetua, A. Latifi, J. Merriman, G. Bugos and D. K. Han, Proteomics, 2017, 17, 1600318 CrossRef PubMed.
- L. K. Weber, A. Isse, S. Rentschler, R. E. Kneusel, A. Palermo, J. Hubbuch, A. Nesterov-Mueller, F. Breitling and F. F. Loeffler, Eng. Life Sci., 2017, 17, 1078–1087 CrossRef CAS PubMed.
- B. Ridder, D. S. Mattes, A. Nesterov-Mueller, F. Breitling and M. A. R. Meier, Chem. Commun., 2017, 53, 5553–5556 RSC.
- L. K. Weber, A. Palermo, J. Kügler, O. Armant, A. Isse, S. Rentschler, T. Jaenisch, J. Hubbuch, S. Dübel, A. Nesterov-Mueller, F. Breitling and F. F. Loeffler, J. Immunol. Methods, 2017, 443, 45–54 CrossRef CAS PubMed.
- K.-Y. Sim, S.-H. Park, K. Y. Choi, J. E. Park, J. S. Lee, B. C. Kim, J. Gwak, W. K. Song, K. H. Lee and S.-G. Park, Sci. Rep., 2019, 9, 4587 CrossRef PubMed.
- C. J. Petell, A. T. Pham, J. Skela and B. D. Strahl, Sci. Rep., 2019, 9, 6265 CrossRef PubMed.
- J. Zhang, L. Shen and S. A. Johnston, Sci. Rep., 2018, 8, 17366 CrossRef PubMed.
- V. I. Lyamichev, L. E. Goodrich, E. H. Sullivan, R. M. Bannen, J. Benz, T. J. Albert and J. J. Patel, Sci. Rep., 2017, 7, 12116 CrossRef PubMed.
- Y. Yan, N. Sun, H. Wang, M. Kobayashi, J. J. Ladd, J. P. Long, K. C. Lo, J. Patel, E. Sullivan, T. Albert, G. E. Goodman, K.-A. Do and S. M. Hanash, Cancer Res., 2019, 79, 1549 CrossRef CAS PubMed.
- A. E. Zhou, A. A. Berry, J. A. Bailey, A. Pike, A. Dara, S. Agrawal, E. M. Stucke, A. Ouattara, D. Coulibaly, K. E. Lyke, M. B. Laurens, M. Adams, S. Takala-Harrison, J. Pablo, A. Jasinskas, R. Nakajima, A. Niangaly, B. Kouriba, A. K. Kone, J. A. Rowe, O. K. Doumbo, M. A. Thera, J. J. Patel, J. C. Tan, P. L. Felgner, C. V. Plowe and M. A. Travassos, mSphere, 2019, 4, e00097–e00019 CrossRef CAS PubMed.
- N. Mishra, A. Caciula, A. Price, R. Thakkar, J. Ng, L. V. Chauhan, K. Jain, X. Che, D. A. Espinosa, M. Montoya Cruz, A. Balmaseda, E. H. Sullivan, J. J. Patel, R. G. Jarman, J. L. Rakeman, C. T. Egan, C. B. E. M. Reusken, M. P. G. Koopmans, E. Harris, R. Tokarz, T. Briese and W. I. Lipkin, mBio, 2018, 9, e00095–e00018 CrossRef CAS PubMed.
- M. Rowe, J. Melnick, R. Gerwien, J. B. Legutki, J. Pfeilsticker, T. M. Tarasow and K. F. Sykes, PLoS Neglected Trop. Dis., 2017, 11, e0005882 CrossRef.
- D. Brambilla, M. Chiari, A. Gori and M. Cretich, Analyst, 2019, 144, 5353–5367 RSC.
- S. Buus, J. Rockberg, B. Forsström, P. Nilsson, M. Uhlen and C. Schafer-Nielsen, Mol. Cell. Proteomics, 2012, 11, 1790–1800 CrossRef PubMed.
- S. J. Carmona, M. Nielsen, C. Schafer-Nielsen, J. Mucci, J. Altcheh, V. Balouz, V. Tekiel, A. C. Frasch, O. Campetella, C. A. Buscaglia and F. Agüero, Mol. Cell. Proteomics, 2015, 14, 1871–1884 CrossRef CAS PubMed.
- T. Osterbye, M. Nielsen, N. L. Dudek, H. Ramarathinam, A. W. Purcell, C. Schafer-Nielsen and S. Buus, J. Immunol., 2020, 205, 290–299 CrossRef CAS.
- L. Shen, J. Zhang, H. Lee, M. T. Batista and S. A. Johnston, Sci. Rep., 2019, 9, 14184 CrossRef PubMed.
- K. Bauer, N. Nelius, M. Reuschenbach, M. Koch, J. Weitz, G. Steinert, J. Kopitz, P. Beckhove, M. Tariverdian, M. von Knebel Doeberitz and M. Kloor, Mol. Cell. Proteomics, 2013, 62, 27–37 CAS.
- J. C. Dudley, M. T. Lin, D. T. Le and J. R. Eshleman, Clin. Cancer Res., 2016, 22, 813–820 CrossRef CAS PubMed.
- M. Peterson, S. N. Murphy, J. Lainson, J. Zhang, L. Shen, C. W. Diehnelt and S. A. Johnston, BMC Immunol., 2020, 21, 25 CrossRef CAS PubMed.
- S. Turajlic, K. Litchfield, H. Xu, R. Rosenthal, N. McGranahan, J. L. Reading, Y. N. S. Wong, A. Rowan, N. Kanu, M. Al Bakir, T. Chambers, R. Salgado, P. Savas, S. Loi, N. J. Birkbak, L. Sansregret, M. Gore, J. Larkin, S. A. Quezada and C. Swanton, Lancet Oncol., 2017, 18, 1009–1021 CrossRef CAS.
- J. B. Legutki, Z.-G. Zhao, M. Greving, N. Woodbury, S. A. Johnston and P. Stafford, Nat. Commun., 2014, 5, 1–7 Search PubMed.
- J. P. Tam, W. F. Heath and R. B. Merrifield, J. Am. Chem. Soc., 1983, 105, 6442–6455 CrossRef CAS.
- P. Stafford, R. Halperin, J. B. Legutki, D. M. Magee, J. Galgiani and S. A. Johnston, Mol. Cell. Proteomics, 2012, 11, M111.011593 CrossRef PubMed.
- R. F. Halperin, P. Stafford and S. A. Johnston, Mol. Cell. Proteomics, 2010, 10, M110.000786 CrossRef PubMed.
- K. A. Navalkar, S. A. Johnston and P. Stafford, J. Immunol. Methods, 2015, 417, 10–21 CrossRef CAS.
- W. Kusnezow, Y. V. Syagailo, S. Ruffer, N. Baudenstiel, C. Gauer, J. D. Hoheisel, D. Wild and I. Goychuk, Mol. Cell. Proteomics, 2006, 5, 1681–1696 CrossRef CAS.
- W. Kusnezow, Y. V. Syagailo, I. Goychuk, J. D. Hoheisel and D. G. Wild, Expert Rev. Mol. Diagn., 2006, 6, 111–124 CrossRef CAS.
- G. V. Sharonov, E. O. Serebrovskaya, D. V. Yuzhakova, O. V. Britanova and D. M. Chudakov, Nat. Rev. Immunol., 2020, 20, 294–307 CrossRef CAS PubMed.
- P. Stafford, Z. Cichacz, N. W. Woodbury and S. A. Johnston, Proc. Natl. Acad. Sci. U. S. A., 2014, 111, E3072–E3080 CrossRef CAS PubMed.
- L. Bassaro, S. J. Russell, E. Pastwa, S. A. Somiari and R. I. Somiari, Cancer Genomics Proteomics, 2017, 14, 427–435 CAS.
Footnotes |
† Electronic supplementary information (ESI) available. See DOI: 10.1039/d0ra05267a |
‡ Current address: Caris Life Sciences, Inc., Phoenix, AZ, USA. |
|
This journal is © The Royal Society of Chemistry 2020 |