DOI:
10.1039/D0RA03697H
(Paper)
RSC Adv., 2020,
10, 21530-21534
Confirmatory non-invasive and non-destructive identification of poison ivy using a hand-held Raman spectrometer
Received
24th April 2020
, Accepted 21st May 2020
First published on 5th June 2020
Abstract
Poison ivy (Toxicodendron radicans) is a forest understory plant that grows throughout the United States, Canada and Mexico. The plant contains urushiol oils, a mixture of pentadecylcatechols, that cause severe allergic reactions on skin including reddish inflammation, uncoloured bumps and blistering. Such allergic reactions develop within hours or days, which facilitates unknowing spread of the urushiol inside the house. This enables continuous contact with urushiol extending the length of time of the rash. It should be noted that apart from extensive washing with soap and cold water, there is no direct way to treat urushiol-induced allergic reactions. In these circumstances, the best practice is to avoid contact with the plant. However, differentiating poison ivy from other plants requires sophisticated botanical experience that is not possessed by a vast majority of people. To overcome this limitation, we developed a confirmatory, label-free, non-invasive and non-destructive approach for detection and identification of poison ivy. We show that using a hand-held Raman spectrometer, 100% accurate identification of this species can be performed in only one second. We also demonstrate that in combination with partial least square discriminant analysis (PLS-DA), Raman spectroscopy is capable of distinguishing poison ivy from more than fifteen different plant species, including weeds, grasses and trees. The use of a hand-held spectrometer on a motorized robotic platform or an unmanned aerial vehicle (UAV) can be used for automated surveillance of household and agricultural spaces enabling confirmatory detection and identification of this dangerous plant species.
Introduction
Poison ivy (Toxicodendron radicans) is a plant species that is broadly spread across North America.1 It produces urushiol that causes severe allergic reactions on skin including reddish inflammation, uncoloured bumps and blistering. Urushiol is a mixture of 1-(alkyl)- or 1-(alkenyl)-2,3-dihydroxybenzenes, where the alkyl/alkenyl group is C15–C17 saturated or unsaturated hydrocarbons.2 Once adsorbed by the skin, urushiol is captured by immune system dendritic cells that migrate to lymph nodes where they present it to T-lymphocytes.3 Activated T-lymphocytes migrate to the skin areas rich with urushiol where, through the release of cytokines, they develop an autoimmune skin inflammation known as urushiol-induced contact dermatitis. The immune response typically develops within hours to days, which facilitates unknowing spread of the urushiol inside the house or through personal belongings, such as cell phones and clothes.1 Thus, a person may continuously come in contact with urushiol present on door knobs, desks, etc., substantially extending the length of time of the rash.
The problem of poison ivy can be solved by timely detection, identification, and elimination of plants from publicly accessible areas. Such detection and identification typically require substantial botanical expertise that is not possessed by most people. The alternative is an image recognition software, which has been steadily improving since the 2000's.4 In particular, the yearly PlantCLEF competition has pushed the field of species image recognition: between 2007 and 2017, over 10
000 species were added to the dataset with the best tools able to classify 84% of samples.5 Additionally, a variety of mobile applications have been developed for plant species identification.6,7 Although such image recognition approaches enable fast species identification, they are not always accurate. Plant genotyping can overcome this limitation, enabling highly accurate species identification. However, these methods are destructive, time-consuming and labor-intensive.
Our group recently showed that Raman spectroscopy (RS) can be used for label-free, non-invasive and non-destructive identification of six different corn varieties.8 This identification is based on unique chemical composition of these varieties that can be probed by Raman spectroscopy, an analytical technique that is based on inelastic light scattering.9 Our group also showed that in addition to identification of plant varieties, RS can be used for confirmatory diagnostics of fungal diseases on corn, wheat and Sorghum.10,11 We further showed that RS could be also used for detection of viral diseases of wheat and rose, as well as the bacteria that cause Huanglongbing (HLB or Citrus Greening) on citrus trees.12–14 These diagnostics are based on detection of pathogen-induced changes in structure and composition of plant molecules. Such changes are unique for each species of pathogens.9
In this work, we investigate the potential of RS for confirmatory, non-invasive and non-destructive identification of poison ivy. Such identification is important in both natural forest and agricultural ecosystems. Using a hand-held Raman spectrometer, we collected spectra from leaves of poison ivy, as well as leaves of several wild (tree: water oak; shrub: buckbrush; ivy: saw greenbrier; grasses: palmer amaranth and white crownbeard) plant species grown in a forest in College Station, TX. We also compared Raman spectrum of poison ivy to the spectra collected from nine different cultivated species, including orange, grapefruit, roses, wheat, peanut, corn, marijuana, hemp, and potato. Lastly, we used partial least square discriminant analysis (PLS-DA) to discriminate between Raman spectra from poison ivy and other species.
Experimental
Plants
Poison ivy (Toxicodendron radicans), water oak (Quercus nigra) white crownbeard (Verbesina virginica), buckbrush (Symphoricarpos orbiculatus) and saw greenbrier (Smilax bona-nox) are naturally occurring species in the forest/park area in College Station, TX; hemp and marijuana plants were grown at Evergreen Enterprises LLC located in Denver, CO; leaves of orange and grapefruit trees were collected from plants grown in RioFarms, Monte Alto, TX and Texas A&M University-Kingsville Citrus Center, Weslaco, TX. Potato, roses (The Double Knock Out®), palmer amaranth and corn plants were grown in greenhouses located in College Station, TX; wheat plants were grown in a greenhouse in Amarillo, TX, whereas peanuts plants were grown in a greenhouse in Stephenville, TX.
All analyzed samples were fresh live plants. No special sample preparation was made.
Raman spectroscopy
Raman spectra were taken with a hand-held Resolve Agilent spectrometer equipped with 830 nm laser source (beam diameter ∼ 2 mm). The following experimental parameters were used for all collected spectra: 1 s acquisition time, 495 mW power, and baseline spectral subtraction by device software. From two to four spectra were collected from each leaf on the adaxial side of the leaf. In total, 20 to 131 surface spectra from each plant species were collected. Spectra shown in the manuscript are raw baseline corrected, without smoothing.
Multivariate data analysis
All Raman spectra were imported into MATLAB and analyzed with the add-on PLS_Toolbox (Eigenvector Research Inc.). Spectra were normalized to total area and the mean offset was removed at each wavenumber before use in partial least squares discriminant analysis (PLS-DA).
Results and discussion
Raman spectra collected from six different wild plant species exhibited vibrational bands that could be assigned to cellulose (520, 915, 1047, 1115, 1326 cm−1), pectin (747 cm−1), carotenoids (1000, 1155, 1525 cm−1), phenylpropanoids (1047, 1326, 1601–1630 cm−1), xylan (1184 and 1218 cm−1), protein (1000 and 1654 cm−1), as well as to aliphatic (1218, 1288, 1382, 1440, 1488 cm−1) and carbonyl/ester (1690–1717 cm−1) groups (Table 1). We found that intensities of these bands vary between both non-normalized and normalized spectra collected from different plant species. For instance, Raman spectra collected from buckbrush leaves exhibited the most intense 1601–1630 cm−1 bands (phenylpropanoids), while intensity of these bands was the lowest in the spectrum of palmer amaranth (Fig. 1). We have also found that palmer amaranth showed a unique vibrational band at 1085 cm−1, which was not evident in Raman spectra of any other plant species. Although buckbrush, palmer amaranth and white crownbeard exhibited an intense band at 1690 cm−1, other species did not have a band at this frequency. We have also found that Raman spectra collected from leaves of poison ivy exhibited a unique band at 1717 cm−1, which was not evident in the Raman spectra of other plant species. Raman spectra collected from this plant species appeared to have the highest intensity of carotenoids (1000, 1155, 1525 cm−1) relative to five other wild plant species analyzed in our work. These results demonstrate that wild plant species have distinctly different Raman fingerprints that can be used for their identification. It should be noted that spectroscopic analysis of poison ivy reported in this manuscript was performed in April. Future studies are required to reveal possible changes in the spectra of this dangerous plant species that could take place in summer and fall. It is also important to reveal possible differences between spectroscopic signatures of poison ivy that was grown in different geographic locations to make such Raman-based identification of poison ivy robust and reliable.
Table 1 Vibrational bands and their assignments for leaves
Band |
Vibrational mode |
Assignment |
520 |
ν(C–O–C) glycosidic |
Cellulose15 |
747 |
γ(C–O–H) of COOH |
Pectin16 |
915 |
ν(C–O–C) in plane, symmetric |
Cellulose, phenylpropanoids15 |
1000 |
ν3 (C–CH3 stretching) and phenylalanine |
Carotenoids, proteins17,18 |
1047 |
ν(C–O) + ν(C–C) + δ(C–O–H) |
Cellulose, phenylpropanoids15 |
1085 |
ν(C–O) + ν(C–C) + δ(C–O–H) |
Carbohydrates19 |
1115 |
νsym(C–O–C), C–OH bending |
Cellulose15 |
1155 |
C–C stretching; ν(C–O–C), ν(C–C) in glycosidic linkages, asymmetric ring breathing |
Carotenoids,20 cellulose21 |
1184 |
ν(C–O–H) next to aromatic ring + σ(CH) |
Xylan22,23 |
1218 |
δ(C–C–H) |
Aliphatic,24 xylan22 |
1265 |
Guaiacyl ring breathing, C–O stretching (aromatic) |
Phenylpropanoids25 |
1288 |
δ(C–C–H) |
Aliphatic24 |
1326 |
δCH2 bending vibration |
Cellulose, phenylpropanoids26 |
1382 |
δCH2 bending vibration |
Aliphatic24 |
1440 |
δ(CH2) + δ(CH3) |
Aliphatic24 |
1488 |
δCH2 bending vibration |
Aliphatic24 |
1525 |
–C C– (in plane) |
Carotenoids27,28 |
1601–1630 |
ν(C–C) aromatic ring + σ(CH) |
Phenylpropanoids29,30 |
1654 |
Amide I |
Proteins31 |
1690–1717 |
ν(C O) |
Carboxyl/ester groups32 |
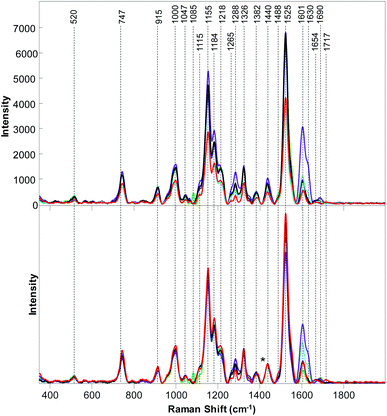 |
| Fig. 1 Baseline-corrected (top) and area normalized (bottom) Raman spectra collected from poison ivy (red), palmer amaranth (green), water oak (blue), white crownbeard (marine), buckbrush (purple) and saw greenbrier (black). | |
Next, we used PLS-DA to determine whether RS can be used for the qualitative identification of these wild plant species based on spectroscopic signatures collected from their leaves. Our results show that poison ivy can be distinguished from all other analyzed plant species with 100% accuracy, Table 2. Moreover, PLS-DA results demonstrate that all wild species except buckbrush can be identified with 100% accuracy (accuracy of buckbrush identification was 98.2%). These findings suggest that RS can be used for highly accurate detection and identification of wild plant species, including poison ivy.
Table 2 Accuracy of classification by PLS-DA wild plant species
|
Correct, % |
Palmer amaranth |
Poison ivy |
Water oak |
White crownbeard |
Buckbrush |
Saw greenbrier |
Palmer amaranth |
100 |
30 |
0 |
0 |
0 |
0 |
0 |
Poison ivy |
100 |
0 |
52 |
0 |
0 |
1 |
0 |
Water oak |
100 |
0 |
0 |
52 |
0 |
0 |
0 |
White crownbeard |
100 |
0 |
0 |
0 |
55 |
0 |
0 |
Buckbrush |
98.2 |
0 |
0 |
0 |
0 |
55 |
0 |
Saw greenbrier |
100 |
0 |
0 |
0 |
0 |
0 |
52 |
The question to ask is whether RS can be also used to distinguish poison ivy in agricultural ecosystems. To answer this question, we collected Raman spectra from nine different cultivated plant species, Fig. 2 and 3. We have found that leaves of cultivated plants exhibit similar spectroscopic profiles. The obtained spectra were dominated by vibrational bands that could be assigned to the same classes of scaffold molecules that were present in the leaves of wild species. Only Raman spectra collected from marijuana plants exhibited substantial differences in the observed vibrational bands, which could be assigned to delta-9-tetrahydrocannabinolic acid (THCA), the precursor to the psychoactive compound present in these plants.33
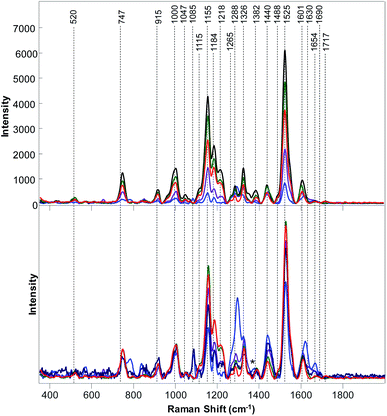 |
| Fig. 2 Baseline-corrected (top) and area normalized (bottom) Raman spectra Raman collected from poison ivy (red), orange (green), marijuana (blue), roses (black), and peanuts (purple). | |
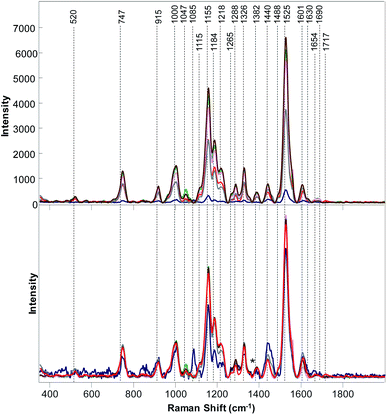 |
| Fig. 3 Baseline-corrected (top) and area normalized (bottom) Raman spectra Raman collected from poison ivy (red), wheat (green), potato (pink), hemp (marine blue), grapefruit (grey) and corn (brown). | |
We have also found that intensities of vibrational bands that could be assigned to cellulose, pectin, carotenoids, phenylpropanoids, xylan, protein, as well as aliphatic and carbonyl/ester groups varied a lot from one plant species to another. For instance, intensities of carotenoid vibrations were found to be the most intense in the spectra of orange, potato and corn, whereas the intensities of these vibrations have been found to be substantially less intense in the Raman spectra of marijuana and hemp. Some of the plant species, such as roses, peanuts and hemp exhibited an intense amide I vibration that can be assigned to proteins, whereas other spectra collected from other plant species did not exhibit an intense vibration in this spectral region. It should be noted that the discussed above 1717 cm−1 band that has been observed in the spectrum of poison ivy was not evident in any of the spectra of cultivated plant species. This suggests that this band can be used to identify this dangerous plant species based on the spectroscopic fingerprint of its leaves.
We used PLS-DA to enable quantitative identification of poison ivy among all analyzed cultivated plants. Our results demonstrate that poison ivy can be distinguished from all cultivated plant species with 100% accuracy, Table 3. Moreover, PLS-DA results demonstrate that wheat, peanuts, marijuana, potato, and hemp can be identified with 100% accuracy, whereas roses, oranges, grapefruit and corn can be identified with 97.7%, 97.9%, 97.9% and 98.4% accuracy, respectively. These findings suggest that RS can be used for highly accurate detection and identification of poison ivy agricultural ecosystems.
Table 3 Accuracy of classification by PLS-DA cultivated plant species
|
Correct, % |
Wheat |
Peanuts |
Marijuana |
Roses |
Potato |
Poison ivy |
Orange |
Hemp |
Grapefruit |
Corn |
Wheat |
100 |
20 |
0 |
0 |
0 |
0 |
0 |
0 |
0 |
0 |
0 |
Peanuts |
100 |
0 |
50 |
0 |
2 |
0 |
0 |
0 |
0 |
0 |
0 |
Marijuana |
100 |
0 |
0 |
20 |
0 |
0 |
0 |
0 |
0 |
0 |
0 |
Roses |
97.7 |
0 |
0 |
0 |
131 |
0 |
0 |
0 |
0 |
0 |
0 |
Potato |
100 |
0 |
0 |
0 |
0 |
24 |
0 |
0 |
0 |
0 |
0 |
Poison ivy |
100 |
0 |
0 |
0 |
0 |
0 |
57 |
0 |
0 |
0 |
0 |
Orange |
97.9 |
0 |
0 |
0 |
1 |
0 |
0 |
47 |
0 |
1 |
0 |
Hemp |
100 |
0 |
0 |
0 |
0 |
0 |
0 |
0 |
22 |
0 |
0 |
Grapefruit |
97.9 |
0 |
0 |
0 |
0 |
0 |
0 |
1 |
0 |
47 |
0 |
Corn |
98.4 |
0 |
0 |
0 |
0 |
0 |
0 |
0 |
0 |
0 |
63 |
Conclusions
Our results demonstrate that RS can be used for confirmatory, non-invasive and non-destructive identification of poison ivy among both wild and cultivated plant species. These findings also suggest that RS can be used for automated identification of this dangerous plant in both forest and agricultural ecosystems. One can envision that coupling of RS with robotic platform or a UAV will enable automated surveillance of household and agricultural spaces enabling confirmatory detection and identification of this dangerous plant species. Rapid development of portable Raman spectrometers also offers a possibility to make a pocket device that any farmer or property owner can have in their possession.34,35 Once equipped with a spectroscopic library of plant species, such units can be used for daily inspection of plants in parks, forests and agricultural ecosystems.
Conflicts of interest
There are no conflicts to declare.
Acknowledgements
This study was supported by funds from Texas A&M AgriLife Research, Texas A&M University Governor’s University Research Initiative (GURI) grant program of (12-2016/M1700437). We are grateful to Prof. Michael Kolomiets for the help with identification of wild plant species, as well as Prof. Michael Kolomiets and John Bennett for growing corn. We are also grateful to Prof. Kranthi Mandadi and Shankar Pant for assistance with collection of Raman spectra from grapefruit and orange trees, as well as potatoes; Prof. Charles Simpson and John Cason for assistance with collection of spectra from peanuts; Prof. David Byrne and Kevin Ong, as well as Madalyn Shires for provided roses; Colin Filter for provided marijuana and hemp. Prof. Charles Rush for provided wheat and Prof. Muthu Bagavathiannan for provided palmer amaranth plants.
References
- https://www.poison-ivy.org/.
- E. Hodgson, in Progress in Molecular Biology and Translational Science, ed. D. B. Teplow, Academic Press, 2012, vol. 112, pp. 373–415 Search PubMed.
- M. D. Gober, R. Fishelevich, Y. Zhao, D. Unutmaz and A. A. Gaspari, J. Invest. Dermatol., 2008, 128, 1460–1469 CrossRef CAS PubMed.
- C. Yang, S. O. Prasher, J. Landry and A. DiTommaso, Can. Agric. Eng., 2000, 42, 147–152 Search PubMed.
- A. Joly, H. Goëau, C. Botella, S. Kahl, M. Poupard, M. Servajean, H. Glotin, P. Bonnet, W.-P. Vellinga, R. Planqué, J. Schlüter, F.-R. Stöter and H. Müller, International Conference of the Cross-Language Evaluation Forum for European Languages, 2019 Search PubMed.
- H. Goëau, P. Bonnet, A. Joly, V. Bakić, J. Barbe, I. Yahiaoui, S. Selmi, J. Carré, D. Barthélémy and N. Boujemaa, Advances in Information Retrieval, 2013 Search PubMed.
- A. Angelova, S. Zhu, Y. Lin, J. Wong and C. Shpecht, NEC Labs Am. Tech. Rep., 2012 Search PubMed.
- M. Krimmer, C. Farber and D. Kurouski, ACS Omega, 2019, 4, 16330–16335 CrossRef CAS PubMed.
- C. Farber, M. Mahnke, L. Sanchez and D. Kurouski, Trends Anal. Chem., 2019, 118, 43–49 CrossRef CAS.
- V. Egging, J. Nguyen and D. Kurouski, Anal. Chem., 2018, 90, 8616–8621 CrossRef CAS PubMed.
- C. Farber and D. Kurouski, Anal. Chem., 2018, 90, 3009–3012 CrossRef CAS PubMed.
- L. Sanchez, S. Pant, Z. Xing, K. Mandadi and D. Kurouski, Anal. Bioanal. Chem., 2019, 411, 3125–3133 CrossRef CAS PubMed.
- L. Sanchez, S. Pant, M. S. Irey, K. Mandadi and D. Kurouski, J. Raman Spectrosc., 2019, 1–6 Search PubMed.
- C. Farber, M. Shires, K. Ong, D. Byrne and D. Kurouski, Planta, 2019, 250, 1247–1254 CrossRef CAS PubMed.
- H. G. Edwards, D. W. Farwell and D. Webster, Spectrochim. Acta, Part A, 1997, 53, 2383–2392 CrossRef.
- A. Synytsya, J. Čopíková, P. Matějka and V. Machovič, Carbohydr. Polym., 2003, 54, 97–106 CrossRef CAS.
- N. Tschirner, K. Brose, M. Schenderlein, A. Zouni, E. Schlodder, M. A. Mroginski, P. Hildebrandt and C. Thomsen, Phys. Status Solidi, 2009, 246, 2790–2793 CrossRef CAS.
- D. Kurouski, R. P. Van Duyne and I. K. Lednev, Analyst, 2015, 140, 4967–4980 RSC.
- M. R. Almeida, R. S. Alves, L. B. Nascimbem, R. Stephani, R. J. Poppi and L. F. de Oliveira, Anal. Bioanal. Chem., 2010, 397, 2693–2701 CrossRef CAS PubMed.
- H. Schulz, M. Baranska and R. Baranski, Biopolymers, 2005, 77, 212–221 CrossRef CAS PubMed.
- E. Wiercigroch, E. Szafraniec, K. Czamara, M. Z. Pacia, K. Majzner, K. Kochan, A. Kaczor, M. Baranska and K. Malek, Spectrochim. Acta, Part A, 2017, 185, 317–335 CrossRef CAS PubMed.
- U. P. Agarwal, Front. Plant Sci., 2014, 5, 1–12 Search PubMed.
- Y. S. Mary, C. Y. Panicker and H. T. Varghese, Orient. J. Chem., 2012, 28, 937–941 CrossRef CAS.
- M. M. Yu, H. G. Schulze, R. Jetter, M. W. Blades and R. F. Turner, Appl. Spectrosc., 2007, 61, 32–37 CrossRef CAS PubMed.
- Y. Cao, D. Shen, Y. Lu and J. Huang, Ann. Bot., 2006, 97, 1091–1094 CrossRef CAS PubMed.
- H. G. Edwards, D. W. Farwell and D. Webster, Spectrochim. Acta, Part A, 1997, 53, 2383–2392 CrossRef.
- G. Devitt, K. Howard, A. Mudher and S. Mahajan, ACS Chem. Neurosci., 2018, 9, 404–420 CrossRef CAS PubMed.
- F. Adar, Spectroscopy, 2017, 32, 12–20 CAS.
- L. Kang, K. Wang, X. Li and B. Zou, J. Phys. Chem. C, 2016, 120, 14758–14766 CrossRef CAS.
- U. P. Agarwal, Planta, 2006, 224, 1141–1153 CrossRef CAS PubMed.
- L. Sanchez, A. Ermolenkov, X.-T. Tang, C. Tamborindeguy and D. Kurouski, Planta, 2020, 251, 64 CrossRef CAS PubMed.
- N. B. Colthup, L. H. Daly and S. E. Wiberley, Introduction to Infrared and Raman Spectroscopy, Academic Press, 3rd edn, 1990 Search PubMed.
- L. Sanchez, C. Filter, D. Baltensperger and D. Kurouski, RSC Adv., 2020, 10, 3212–3216 RSC.
- SpectrEcology, https://shop.spectrecology.com/product/raman-spectrometer-rental-idraman-785nm-handheld-raman/?attribute_pa_rental-period=month%26gclid=CjwKCAjwqpP2BRBTEiwAfpiD-ydG4yaVcy4bMRyoVr6e87FGX5oOhMm1XuJinDiyR57OyZxxzJMvLBoCP_wQAvD_BwE, accessed on May 20, 2020.
- Agiltron, https://agiltron.com/product/handheld-raman-spectrometer, accessed on May 20, 2020.
|
This journal is © The Royal Society of Chemistry 2020 |
Click here to see how this site uses Cookies. View our privacy policy here.