DOI:
10.1039/D0RA02406F
(Paper)
RSC Adv., 2020,
10, 18305-18314
A targeted neurotransmitter quantification and nontargeted metabolic profiling method for pharmacometabolomics analysis of olanzapine by using UPLC-HRMS†
Received
15th March 2020
, Accepted 21st April 2020
First published on 13th May 2020
Abstract
Neurotransmitters (NTs) are specific endogenous metabolites that act as “messengers” in synaptic transmission and are widely distributed in the central nervous system. Olanzapine (OLZ), a first-line antipsychotic drug, plays a key role in sedation and hypnosis, but, it presents clinical problems with a narrow therapeutic window, large individual differences and serious adverse effects, as well as an unclear mechanism in vivo. Herein, a simultaneous targeted NT quantification and nontargeted metabolomics method was developed and validated for pharmacometabolomics analysis of OLZ by using ultra-high-performance liquid chromatography coupled with high-resolution mass spectrometry (UPLC-HRMS). Considering the low physiological concentrations of NTs, a full MS scan and target selective ion monitoring (tSIM) scan were combined for nontargeted metabolomics and targeted NT quantification, respectively. By using this strategy, NTs at a very low physiological concentration can be accurately detected and quantified in biological samples by tSIM scans. Moreover, simultaneously nontargeted profiling was also achieved by the full MS scan. The newly established UPLC-HRMS method was further used for the pharmacometabolomics study of OLZ. Statistical analysis revealed that tryptophan, 5-hydroxytryptophan, 5-hydroxytryptamine, γ-aminobutyric acid etc. were significantly downregulated, while tyrosine was significantly upregulated, which suggested that OLZ could promote the downstream phase II reaction of 5-hydroxytryptamine, inhibit tyrosine hydroxylase activity, and increase the activity of γ-aminobutyric acid transaminase. In conclusion, this method could provide novel insights for revealing the pharmacodynamic effect and mechanism of antipsychotic drugs.
1. Introduction
Schizophrenia is a severe mental illness characterized by emotional reactions and deep confusion in the thinking process, occurs mostly in young adults, and affects approximately 1% of the world's population.1 At present, antipsychotic drugs (APDs) can be divided into two categories according to their pharmacological effects: typical and atypical.2 Atypical antipsychotics mainly include clozapine, quetiapine, olanzapine (OLZ), lurasidone, aripiprazole and ziprasidone.3 The World Federation of Societies of Biological Psychiatry recommended OLZ as the first-line drug for the treatment of schizophrenia.4 However, the mechanism of the specific action of OLZ is still not understood very clearly in vivo. Some studies have shown that OLZ acts on the receptors for a variety of monoamine neurotransmitters (NTs).5 For example, D2 receptor blockers are commonly affected by APDs6 and play a key role in schizophrenia by regulating NTs,7 which are specific endogenous metabolites that act as “messengers” in synaptic transmission and are widely distributed in the central nervous system.8 Moreover, metabolic disorders are more commonly observed in patients with schizophrenia, and the effects of OLZ on metabolism have been widely studied.9 OLZ has a low incidence of neurological side effects and risk of extrapyramidal symptoms,10 but has severe metabolic side effects in clinical treatment, including weight gain,11 dyslipidemia and insulin resistance.12,13
Metabolomics is the science of investigating the overall or seasonal changes in endogenous small molecule metabolites in biological systems after disturbances.14 Metabolomics has been applied to various fields of research, such as drug development, drug treatment response, individualized treatment and therapy monitoring.15,16 Methodological approaches for performing metabolomics experiments are classified into nontargeted and targeted analysis.17 Nontargeted metabolomics provides a large and complex amount of information for the overall analysis of all metabolite components and their levels in biological samples, with the advantage of obtaining relatively complete metabolite information.18 However, there are also obvious deficiencies: fluctuations in instrument parameter conditions affect data repeatability, and peak alignment errors occur in complex data preprocessing.19,20 It is impossible to detect and accurately quantify some key trace amounts of endogenous metabolites.21 Targeted metabolomics, with the advantages of high sensitivity, accuracy, measure a precisely targeted set of metabolites of low-content substances in complex biological samples.22 However, it is necessary to establish a specific analytical method for the detection of low-level metabolites in biological samples, avoiding in the loss of information on other metabolites. Recently, nontargeted metabolomics research was applied to identify biomarkers related to OLZ treatment by analyzing plasma in women with first-episode schizophrenia.23 However, the detection of specific NTs was not taken into consideration, and some low-abundance but important substances closely related to the disease and drug effects were missed. NTs are characterized by strong polarity and low content in biosamples. Some LC-MS methods have been developed for the targeted detection of certain NTs, such as acetylcholine,24 glutamate, acetylcholine, choline, dopamine, 5-hydroxytryptamine, and some metabolites.25,26
In this study, a simultaneous targeted NTs quantification and nontargeted metabolomics method was developed and validated for pharmacometabolomics analysis of OLZ by using ultra-high-performance liquid chromatography coupled with high-resolution mass spectrometry (UPLC-HRMS). We can detect the low physiological concentrations of NTs by a target selective ion monitoring (tSIM) scan and simultaneously acquire nontargeted metabolic profiling by the full MS (full MS) scan, and this method was further used for the pharmacometabolomics study of the antipsychotic drug, OLZ.
2. Experimental
2.1. Chemicals and reagents
HPLC grade methanol (MeOH), acetonitrile (ACN), and formic acid (FA) were purchased from Merck (Darmstadt, Germany), and pure water was obtained from Wahaha Group Co., Ltd (Hangzhou, China). Other chemicals were of analytical grade. Standard compounds, including L-tryptophan (Trp), L-tyrosine (Tyr), glutamine (Gln), 5-hydroxyindoleacetic acid (5-HIAA), 5-hydroxytryptophan (5-HTP), glutamate (Glu), taurine, L-3,4-dihydroxyphenylalanine (L-DOP), L-asparagine (Asn), 5-hydroxytryptamine (5-HT), acetylcholine (Ach), γ-aminobutyric acid (GABA), kynurenine, and ten stable isotope-labeled internal standards: γ-aminobutyric acid-d2, choline-d9, 5-hydroxytryptamine-d4, taurine-15N, indoleacetic acid-d2, glutamine-15N, glutamate-d5, L-tryptophan-d5, L-tyrosine-d2, L-asparagine-d1 were purchased from Sigma-Aldrich (St. Louis, MO, USA), and olanzapine (OLZ) bulk drug were purchased from Shanghai Yuan Ye Biotechnology Co., Ltd.
2.2. Standard solution
Stock solutions of the standards with concentrations ranging from 1 to 2 mg mL−1 were separately prepared in methanol/water (50/50, v/v). Standard working solutions were prepared by serial dilutions of the stock solutions to produce lower concentration levels. Ten stable isotopic internal standards were dissolved in methanol to obtain an IS mixture solution. Then, the IS mixture solution was diluted to a working concentration of 50 ng mL−1 for each IS. Both the standard stock solutions and IS working solutions were stored at 4 °C before use.
2.3. Clinical sample collection
17 Chinese adult volunteers employed in this pharmacometabolomics study were collected from bioequivalence tests of OLZ orally disintegrating tablets (SWDXX-201627) at Beijing Chao-Yang Hospital, Capital Medical University. This bioequivalence study (http://www.chinadrugtrials.org.cn/, identifier: CTR20170069) was conducted between May 16, 2017, and May 14, 2018, at a single study center in the Phase I clinical trial center of Beijing Chao-Yang Hospital. The present study was conducted in accordance with Declaration of Helsinki, International Conference on Harmonization (ICH) and Good Clinical Practice guidelines. All study documentation, such as study protocol and consent forms, were reviewed and approved by the Institutional Review Board of Beijing Chao-Yang Hospital (ethical approval number: 2016-Drug-43) before the initiation of the clinical trial. All subjects were provided with a written informed consent form before inclusion and were able to adhere to study restrictions issued by the Phase I Clinical Trial Center protocol. As the plasma samples used in this study were the remaining samples from the pharmacokinetic study, the pharmacometabolomic study was eligible for exemption from informed consent. The plasma samples collected at the timepoints of baseline and Cmax were used for UPLC-HRMS analysis. Specific sample information is shown in ESI Methods 1.2.†
2.4. Animals and drug treatments
For the animal experiments, twelve male Sprague-Dawley (SD) rats (6 weeks, body weight: 200 ± 20 g) were purchased from Vital River Laboratory Animal Technology Company (Beijing, China). The animals were maintained for a week with unlimited standard food and water at a constant room temperature of 22 ± 2 °C and 45–55% humidity under a 12 h light/dark cycle. In experiments, all animal procedures were performed in accordance with the Guidelines for Care and Use of Laboratory Animals of “the Institute of Materia Medica, Chinese Academy of Medical Science and Peking Union Medical College” and all experiments were conducted with the approval of the Animal Ethical Committee at the Institute of Materia Medica, Chinese Academy of Medical Science and Peking Union Medical College. After acclimating, all rats were randomly divided into control group and model group, with 6 animals for each group. Powder of OLZ was prepared in normal saline (50 mg mL−1) and orally administered to animals of model group (4 mg kg−1). The equal volume of saline was administered to rats of control group. Blood samples were collected from the eye sockets of the rats and placed in tubes containing the anticoagulant heparin sodium. Plasma samples were placed at 4 °C after gentle shaking. The supernatants were then collected by centrifugation at 4000 rpm at 4 °C for 10 min. The plasma samples collected at the timepoints of baseline and Cmax were used for UPLC-HRMS analysis.
2.5. Sample preparation
Standard working solutions were prepared by adding 10 μL of each concentration of the mixed standard solution dilutions in 50 μL purified water (blank matrix). Prepare the standard working solution according to the Plasma Sample Pretreatment. Quality control (QC) samples of the mixed standards at low, medium and high levels were prepared with different vials of standard working solutions. The frozen plasma samples were thawed at 4 °C before analysis. A pooled QC sample was prepared by mixing the same volume (10 μL) of each sample. 10 μL of MeOH solvent and 5 μL of mixed internal standards were added to each tube for the 50 μL plasma samples. Then, 150 μL of acetonitrile (stored at 4 °C; Merck, Darmstadt, Germany) was added, and the mixture was vortexed for 5 min on a vortex mixer (IKA, MS) at 2500 rpm and then centrifuged at 10
000 rpm and 4 °C for 5 min. The supernatant was transferred into a 1.5 mL centrifuge tube and evaporated to dryness in a Doprah A502200 (Xueyu Technology Co. Ltd.). The residues were reconstituted in 50 mL of reconstitution solution (acetonitrile/0.1% aqueous formic acid, 98
:
2, v/v), mixed for 5 min at 2500 rpm on a vortex mixer and then centrifuged at 10
000 rpm for 5 min. The supernatants were filtered with a 96-well plate filter (Captiva, Agilent Technologies, USA) for LC-MS analysis, and 5 μL was injected into the LC-MS/MS system.
2.6. UPLC-HRMS analysis
The analysis was performed on a two-dimensional ultra-high-performance liquid chromatography system (ACQUITY UPLC I-Class, Waters, Milford, MA, USA) coupled to a high-resolution quadrupole-orbitrap tandem mass spectrometer with a heated electrospray (HESI) probe (Q-Exactive, Thermo Fisher Scientific, Waltham, MA). Chromatographic separation was performed on a Waters HSS T3 (C18) column (2.1 × 100 mm, 1.8 mm) for the reversed-phase separation of weakly polar solutes and a Thermo Scientific Hypercarb porous graphitized carbon (PGC) column (2.1 × 150 mm, 3 mm) for supplemental separation of strongly polar solutes in the second dimension with two six-port-two-position valves. The mobile phase for the T3 column consisted of 0.1% formic acid water solution (A1) and acetonitrile (B1); the PGC column also consisted of 0.1% formic acid water solution (A2) and acetonitrile (B2). The injection volume was 5 mL, and the column oven temperature was maintained at 45 °C. The gradient conditions were from a previously reported.27
The instrumental setup is shown in ESI Fig. S1.† From 0 to 2.5 min at position A (Fig. S1A†), the T3 and PGC columns were simply coupled; thus, the components eluted from the T3 column were all automatically transferred and loaded to the PGC column for further subdivision. When the valves were switched to position B at 2.5 min (Fig. S1B†), pump 1 (P1) stopped flowing, while separation on the PGC column began. When the valves were switched to position C (Fig. S1C†) at 8.5 min, P1 started to operate again, leading to separation on the T3 column, while the PGC column underwent washing and pre-equilibration steps. The detailed elution gradient and flow rate are presented in ESI Table S1.†
MS analysis scanning was performed in positive and negative ion modes. The electrospray ionization source parameters were optimized as follows: sheath gas 40/45, auxiliary gas 11/10, auxiliary gas heater temperature 220 °C, spray voltage 3.5/−3.2 kV, capillary temperature 350 °C. Alternate settings for the full MS assay and tSIM assay were implemented as described below.
To relatively quantify polar metabolites with relatively low content and obtain mass spectral data containing as much information as possible on metabolite content, a combination of full MS and tSIM scanning was used, which consisted of a full MS scan (m/z 66.7–1000) and a tSIM scan. The resolution, AGC target, and maximum injection time (IT) for the full MS scan were set at 35
000, 3 × 106, and 100 ms, and those for the tSIM scans were set at 35
000, 3 × 106, and 200 ms, respectively. The window width of tSIM was 4 Da.
2.7. Data processing and statistical analysis
Accurate and relatively targeted quantification was performed using Xcalibur 2.2 (Thermo Fisher Scientific). All calibration curves were linear and weighted 1/x. For nontargeted analysis, the MM File Conversion 3.9 (http://mm-file-conversion.software.informer.com/3.9/) was used to convert the full scan raw data files from the LC-MS analysis to the mzXML format. Then, R 2.15.2 was applied to peak detection, filtering, alignment, scaling, and identification using XCMS software (http://masspec.scripps.edu/xcms/xcms.php) (the detailed parameters are shown in the ESI†). Multivariate statistical analysis was carried out using SIMCA-P 14.0 (Umetrics AB, Umeå, Sweden), which included principal component analysis (PCA) and orthogonal partial least squares discriminant analysis (OPLS-DA). Variables were considered to be potential markers, which satisfied the following conditions: variables with a VIP value ≥1.0; independent t-test (p < 0.05). Finally, the isotope and adduct ions were deleted according to the corresponding extracted ion chromatograms (XICs). Potential biomarkers were identified by searching the databases including HMDB (http://www.hmdb.ca/), MassBank (http://www.massbank.jp/), and Metlin (http://metlin.scripps.edu/), and then the high-resolution LC-MS/MS spectra were used for further identification. Then, available commercial standards were applied to further verify the potential structures. Finally, visually displayed heat maps were used to present the discriminatory power of potential biomarkers.
2.8. Method validation procedure
According to the US Food and Drug Administration (FDA) guidelines on bioanalytical method validation. Method validation needs to assess several quality parameters of the method, such as linearity, selectivity, accuracy, precision, stability and recovery. The details of the method validation procedure are shown in ESI Methods 1.3.†
3. Results and discussion
3.1. Methods development
The detection of NTs using HPLC-MS is highly challenging due to their strong polarity, short chromatographic retention time and low content. To simultaneously detect highly polar metabolites, including NTs, and weakly polar metabolites for comprehensive targeted quantification and nontargeted metabolomics, a dual UPLC-HRMS method was proposed, modified from our previously reported method. A T3 column with high column efficiency, good stability and large peak capacity was used as the first-dimension column, and the PGC column was used as the second-dimension column. Two six-way valves were used as the column switching valve. The first and secondary dimensional chromatographic mobile phases were both 0.1% formic acid water–acetonitrile systems. A T3 column was used for the reversed-phase separation of moderately and weakly polar solutes and a PGC column for the supplemental separation of strongly polar solutes. The total ion chromatographs (TICs) of actual plasma samples measured in positive and negative ion mode are shown in Fig. 1A and B. The typical extracted ion chromatographs (XICs) of some NTs and related metabolites in positive ion mode are shown in Fig. 1C and D. The results indicated that the plasma samples were well separated under the chromatographic conditions, and the peak shape was good. The number of features detected by LC-MS is shown in Fig. S2.†
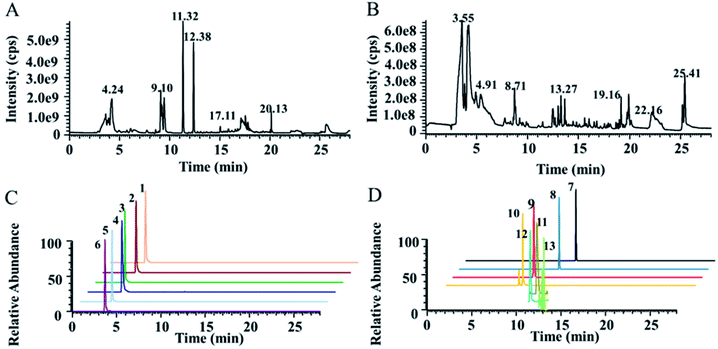 |
| Fig. 1 Typical metabolomics TICs obtained from humans in positive ion mode (A) and in negative ion mode (B) by UPLC-HRMS analysis. Typical XICs of some NTs and related metabolites obtained by LC-MS/MS in positive ion mode (C and D). Detailed information on typical XICs of 13 quantified metabolites in human plasma is shown in Table 1. | |
Furthermore, this method was specifically focused on the detection of antipsychotic drug mechanism-related endogenous metabolites, including most NTs and metabolic pathway-related metabolites. Thirteen endogenous metabolites, including NTs, were quantified. Large differences in the intensity of the MS signal of the 13 metabolites in human plasma were observed, as shown in Table 1. Here, we used a combination of a full MS scan and tSIM scan for data acquisition to improve the throughput for nontargeted metabolomics and the selectivity, sensitivity for low-level endogenous metabolites to achieve the quantification accuracy requirements of targeted metabolomics. The advantage of the tSIM scan is that the target ions can be scanned with a narrow sampling window width to eliminate interference from other ions and improve the signal-noise ratio of the target compound. The low-level endogenous metabolites 5-HTP, 5-HT and L-DOPA were acquired using the tSIM scan, and other metabolites were quantified using XIC in full MS. Take 5-HT as an example, as shown in Fig. S3.† The results indicated that the tSIM mode can effectively improve the peak shape and detection sensitivity (S/N) compared with the full MS scan. Therefore, the established method had the ability to quantify multiple metabolites with large differences in content to acquire more comprehensive metabolite information for pharmacometabolomics.
Table 1 The information and ion intensity of 13 quantified metabolites in human plasmaa
No. |
Compound name |
Formula |
Theoretical m/z |
Measured m/z |
Error (ppm) |
Ion intensity in human plasma |
RT (min) |
Abbreviations: L-tryptophan (Trp), L-tyrosine (Tyr), glutamine (Gln), L-asparagine (Asn), acetylcholine (Ach), 5-hydroxytryptophan (5-HTP), 5-hydroxyindoleacetic acid (5-HIAA), 5-hydroxytryptamine (5-HT), γ-aminobutyric acid (GABA), glutamate (Glu), and L-3,4-dihydroxyphenylalanine (L-DOPA). |
1 |
Glu |
C5H9NO4 |
148.0604 |
148.0598 |
−4.1 |
3.50 × 107 |
4.00 |
2 |
Gln |
C5H10N2O3 |
147.0764 |
147.0758 |
−4.1 |
6.00 × 107 |
3.78 |
3 |
GABA |
C4H9NO2 |
104.0706 |
104.0710 |
3.8 |
1.40 × 107 |
3.35 |
4 |
Ach |
C7H16NO2 |
146.1176 |
146.1178 |
1.4 |
1.00 × 108 |
3.88 |
5 |
Asn |
C4H8N2O3 |
133.0608 |
133.0602 |
−4.5 |
1.40 × 107 |
3.63 |
6 |
Taurine |
C2H7NO3S |
126.0219 |
126.0215 |
−3.2 |
3.50 × 106 |
3.69 |
7 |
Trp |
C11H12N2O2 |
205.0972 |
205.0964 |
−3.9 |
8.00 × 108 |
12.39 |
8 |
Kynurenine |
C10H12N2O3 |
209.0921 |
209.0915 |
−2.9 |
2.00 × 107 |
12.26 |
9 |
Tyr |
C9H11NO3 |
182.0812 |
182.0805 |
−3.8 |
7.00 × 108 |
9.11 |
10 |
L-DOPA |
C9H11NO4 |
198.0761 |
198.0765 |
2.0 |
5.00 × 104 |
8.60 |
11 |
5-HTP |
C11H12N2O3 |
221.0920 |
221.0915 |
−2.3 |
2.00 × 104 |
11.12 |
12 |
5-HT |
C10H12N2O |
177.1022 |
177.1029 |
4.0 |
6.00 × 104 |
10.88 |
13 |
5-HIAA |
C10H9NO3 |
192.0655 |
192.0651 |
−2.1 |
4.00 × 106 |
11.41 |
3.2. Method validation
3.2.1 Linearity. The linear regression equations, correlation coefficients and calibration ranges of the validated models are shown in Table S2.† The correlation coefficient (R2) of linear regressions was above 0.99 for all analytes. The calibration standards had a range of 0.1–20 μg mL−1 for Trp, GABA and taurine, 1–200 ng mL−1 for 5-HTP, 0.02–4 μg mL−1 for 5-HT, L-DOPA and Ach, 2–400 ng mL−1 for 5-HIAA, 0.01–2 μg mL−1 for kynurenine, 0.2–40 μg mL−1 for Tyr and Gln, 0.5–100 μg mL−1 for Glu, and 0.04–8 μg mL−1 for Asn.
3.2.2 Accuracy and precision. The intraday and interday accuracy and precision of the method are summarized in Tables S3 and S4.† The intraday and interday accuracy were 89.8–112.7% and 91.0–108.9%, respectively. The intraday and interday precision were 0.34–8.82% and 0.34–8.82%, respectively.
3.2.3 Extraction recovery. The results of the extraction recovery assay of the method at three concentration levels are shown in Table S5.† The recoveries of each compound at low, medium and high concentrations ranged from 71.9–114.8%, and the RSD values were less than 15%.
3.2.4 Stability studies. The freeze-thaw stability and autosampler stability for the QC samples of the method are summarized in Tables S6–S8.† The biases of the accuracy and precision of QC samples at three concentrations in this evaluation were lower than 20%. This suggests that all the assayed metabolites were stable in prepared samples in the autosampler at 4 °C for 12 h and 48 h and stable in frozen samples at −80 °C for three freeze-thaw cycles.
3.2.5 Validation for nontargeted metabolomics. These results demonstrated that this method was reliable to measure the metabolic changes associated with the intake of drugs. The details are shown in ESI Methods 1.3.†
3.3. Targeted analysis of NTs and related metabolites
The validated method was successfully applied to the determination of NTs and their related metabolites in healthy human and rat plasma before and after OLZ dosing. The comparison results from the measurement of the 13 metabolites associated with the mechanism of OLZ are shown in Fig. 2. In the tryptophan metabolic pathway (Fig. 3A), the contents of Trp, 5-HTP, 5-HT, 5-HIAA, kynurenine, and kynurenine acid were decreased significantly in human plasma compared to the preadministration levels. Notably, a decrease in Trp was observed after drug treatment, indicating that OLZ promotes the metabolism of tryptophan. It was reported that 5-HT and 5-HIAA were inhibitory NTs improving insomnia and extend sleep time.28,29 However, 5-HT and 5-HIAA were decreased after drug treatment, which suggested that OLZ may promote the downstream metabolism of 5-HT and could be related to downstream UDP-glucuronosyltransferase (UGT) enzymes.30,31 Glu is an excitatory amino acid.32 In the glutamate and γ-aminobutyric acid metabolic pathways (Fig. 3B), there were significant decreases in Glu and GABA levels, which can reduce neurotoxicity. GABA content decreased, but the downstream metabolite succinic acid semialdehyde increased, indicating that OLZ may increase the enzyme activity of γ-aminobutyric acid transaminase (GABA-T), thereby promoting GABA metabolism. In the dopamine and noradrenaline metabolic pathways (Fig. 3C), the levels of L-DOPA were significantly decreased, whereas the levels of Tyr were increased, which indicates that OLZ may inhibit tyrosine hydroxylase (TH) activity, inhibiting L-DOPA synthesis of the dopamine precursor and reducing dopamine synthesis. Thereby achieving anti-excitatory mania. Moreover, there was an increase in the content of the dopamine metabolites homovanillic acid (HVA) and 3,4-dihydroxyphenylacetic acid (DOPAC) after administration, which is further evidence that OLZ promotes the metabolism of the excitatory neurotransmitter dopamine (DA). Decreases in Ach and taurine concentrations were observed after administration. Asn levels were increased significantly (Fig. 2). The above results were focused on the analysis of three related target metabolic pathways based on the neurotransmitter hypothesis of schizophrenia pathogenesis. Compared to the human results, similar changed trends of NTs were reported in rat plasma samples. Trp, 5-HIAA, kynurenine, kynurenine acid, L-DOPA and GABA were decreased significantly in rat plasma with drug administration. But the results that the contents of 5-HTP and 5-HT were increased, the levels of Tyr were decreased in rats, indicated the species differences. UGT is encoded by a polymorphic gene, which may be differences in the types, contents and activities in different species. Rats and humans have different living environments, what's more rats are very sensitive for external stimuli, which easily caused the changes of metabolism in the body. Therefore, in the process of new drug development and evaluation, attention should be paid to the impact of differences between species.
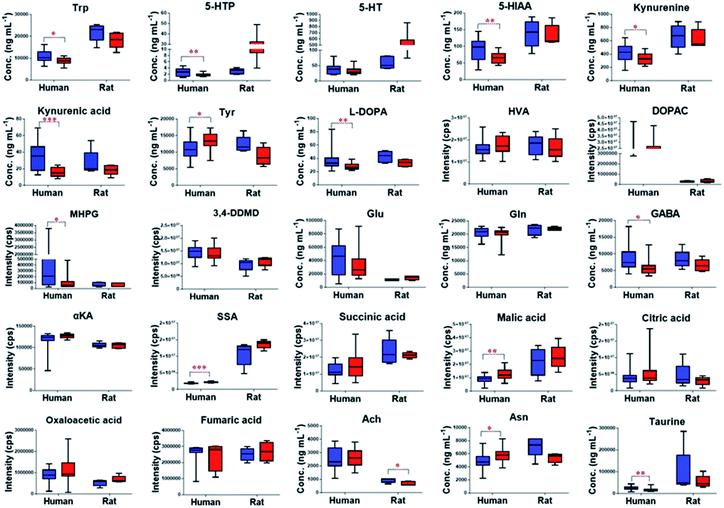 |
| Fig. 2 Contents of NTs and related metabolites in predosing ( ) and after-dosing ( ) samples. ***p < 0.001, **p < 0.01, *p < 0.05. Abbreviations: HVA: homovanillic acid, DOPAC: 3,4-dihydroxyphenylacetic acid, MHPG: 3-methoxy-4-hydroxyphenylglycol, 3,4-DDMD: 3,4-dihydroxymandelic acid, αkA: α-ketoglutaric acid, SSA: succinic semialdehyde. | |
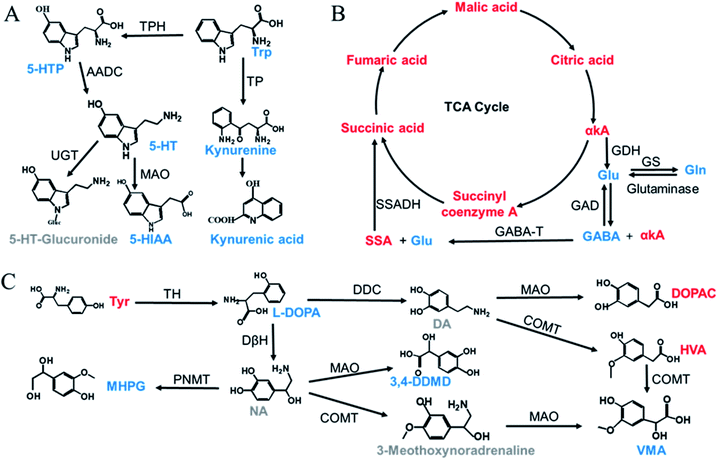 |
| Fig. 3 Three important target metabolic pathways related to the mechanism of action of OLZ (red: upregulation; blue: downregulation; gray: undetected). (A) Tryptophan metabolic pathway, (B) glutamic acid and γ-aminobutyric acid metabolic pathway, (C) dopamine and norepinephrine metabolic pathway. Abbreviations: DA: dopamine, NA: noradrenaline, VMA: vanillylmandelic acid, TPH: tryptophan hydroxylase, TP: tryptophan pyrrolase, AADC: aromatic L-amino acid decarboxylase, UGT: uridine diphosphate-glucuronosyltransferase, MAO: monoamine oxidase, SSADH: succinic semialdehyde dehydrogenase, GABA-T: γ-aminobutyric acid transaminase, GAD: glutamic acid decarboxylase, GS: glutamine synthetase, GDH: glutamate dehydrogenase, TH: tyrosine hydroxylase, DDC: dopadecarboxylase, COMT: catechol-O-methyl transferase, DβH: dopamine-β-hydroxylase, PNMT: phenylethanolamine N-methyltransferase. | |
3.4. Nontargeted metabolomics
To evaluate the clinical utility of this method, plasma samples collected from healthy volunteers before and after taking the antipsychotic drug (OLZ) were subjected to metabolomics analyses to discover the metabolic differences and in vivo effects of drug treatment. In order to prevent the influence of prototype drugs and metabolites on the grouping of models, they were excluded before modeling, and only the endogenous metabolites were included for statistical analysis. The projection results of each sample on the first principal component are shown in Fig. S4A and B.† It can be seen from the figure that the relative deviations of the peak areas of QC samples are controlled within the 2SD range. The OPLS-DA results indicated that the pre-dosing and after-dosing groups were clearly separated based on quantitative analysis. The results are shown in Fig. S5C and D.† The OPLS-DA model parameters R2Y (cum) = 94.1% and Q2 (cum) = 77.4% and model parameters R2Y (cum) = 98.3% and Q2 (cum) = 79.4% for the LC- (±) ESI-MS, respectively. Metabolites were selected for discrimination with differences (VIP value ≥1) and p < 0.05 in Student's t-tests. From the data obtained from the metabolic profiling analysis, 41 differential metabolites that contributed greatly to the group before and after administration were screened, 34 were upregulated after administration, and 7 were downregulated. The change rates of differential metabolites are shown in Fig. 4A and B.
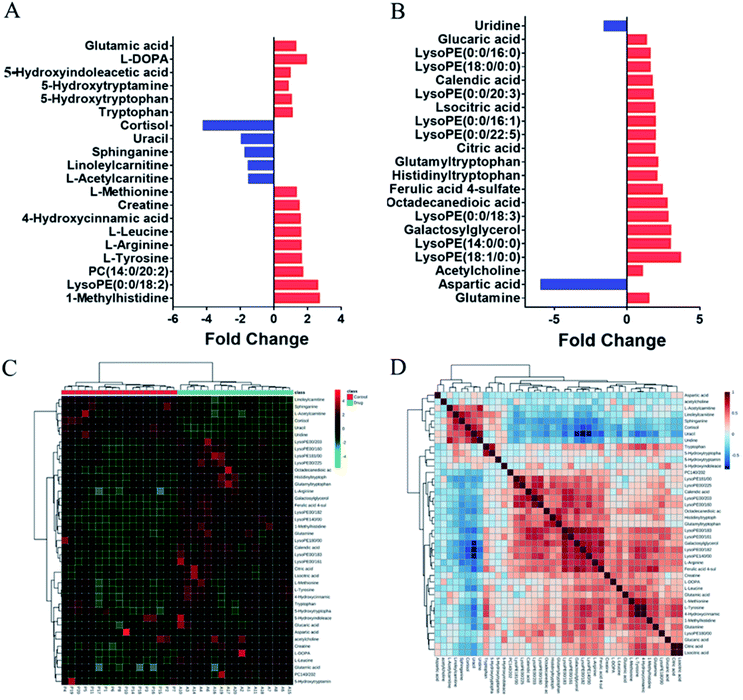 |
| Fig. 4 (A and B) Fold change of differential metabolites in after and before dosing. (C) Hierarchical clustering analysis (HCA) of 41 potential biomarkers. (D) Correlation analysis of potential biomarkers. | |
The differential metabolites in plasma were further identified by LC-MS/MS (Table S9†). Hierarchical cluster analysis (HCA) was used to visualize the changes in these potential biomarkers in each sample between the two groups, and the results indicated that these biomarkers could be used to distinguish between the pre-dosing and after-dosing groups (Fig. 4C). Correlation analysis was used to determine the association of identified differential metabolites, and the results are presented in Fig. 4D.
The altered metabolic pathways after OLZ drug treatment were further examined by MetaboAnalyst 4.0. The result is shown in Fig. S6.† Multiple metabolic pathways including alanine, aspartate and glutamate metabolism, glutamine and glutamate metabolism, arginine and proline metabolism, tryptophan metabolism, glycerophospholipid metabolism, tyrosine metabolism, sphingolipid metabolism, aminoacyl-tRNA biosynthesis, and nitrogen metabolism were identified. To more accurately explore changes in metabolic levels, we focused on the analysis of abnormal metabolic levels with pathway impact (PI) greater than 0.1. The details are shown in Table S10.† Alanine, aspartate and glutamate metabolism, tryptophan metabolism and tyrosine metabolism are related to the pathogenesis hypothesis NT theory of schizophrenia.33,34 Thus, changes in the NTs in the metabolic pathway can be used to explain the role of OLZ in the treatment of schizophrenia, which can provide a basis for further study of their mechanisms of action. Compared with the predosing group, the tricarboxylic acid (TCA) cycle showed increased succinic acid, fumaric acid, malic acid, oxaloacetic acid, citric acid, and α-ketoglutaric acid levels (Fig. 2), indicating that the energy supply changed after administration. This may be related to the side effects of OLZ. It is consistent with the previous results of the stimulating cell experiment of OLZ, which attempted to balance cell energy by promoting glucose uptake. The downregulation of AMPK in the absence of glucose reduction may result in a deleterious effect of OLZ on energy metabolism, which may be the basis for the well-known metabolic side effects of the drug.35 In the arginine and proline metabolic pathways, the arginine content was upregulated after administration, inline with previous studies showing that OLZ treatment of schizophrenia leads to upregulation of arginine and the synthesis of novel NTs. Agmatine is considered to play an important role in the development of schizophrenia, and its level decreases following antipsychotic treatment.36 In addition, arginine and proline can be transformed into ornithine and are very dependent on the energy and nitrogen sources provided by glutamine metabolism in biosynthesis.37 Disorders of glycerophospholipids and sphingolipid metabolism after administration may be associated with the well-known obesity and weight gain side effects of OLZ.38 Currently, there is evidence that taking OLZ daily for four weeks increases the levels of total cholesterol, free cholesterol, fatty acids and glycerol in the liver. In summary, OLZ may aggravate atherosclerosis by dysregulating liver lipid metabolism, aggravating hyperlipidemia and aortic inflammation.39 It has been reported that sphingolipids also play an important role in blood glucose balance and insulin resistance.40 Atypical anti-schizophrenia drugs can significantly decrease sphingolipid levels in the liver and significantly reduce the content of hepatic ceramide and sphingomyelin, as well as exerting specific effects on lipid species.
It should be noted that this targeted neurotransmitters quantification and nontargeted metabolic profiling method can be not only applied to the study of the metabolic changes in plasma samples, but also in cerebrospinal fluid or brain samples, as well as those samples from schizophrenia patients. What's more this method can also analyze the metabolism of other antipsychotic drugs, because antipsychotic drugs have some common characteristics in the mechanism of action. This method can simultaneously detect the metabolic changes in neurotransmitter substances and other metabolites with high coverage. Although our study conducted a pharmacometabolomics analysis of OLZ, which provided deep and comprehensive information on its metabolic alteration effects in vivo at the molecular level, it was not ruled out the interferes of diet, and the metabolic changes in biological samples associated with the occurrence of disease. Therefore, in the future, we will focus on the metabolic alterations and distribution information not only in the plasma but also in the whole body of animals and targeted organs using spatially-resolved metabolomics, to verify the function of key metabolites and the activities of related metabolic enzymes. It will provide a basis for individualized treatment and precise medication of schizophrenia using antipsychotic drugs.
In general, there are still deficiencies in researches on the pharmacometabolomics analysis of OLZ in the previous studies, which focused mostly on nontargeted metabolomics.41,42 Although the range of target metabolites detected was wide, it is impossible to high sensitively detect metabolites with special properties, such as NTs with strong polarity and very low physiological content. What's more some important metabolites closely related to the disease or drug action but with low endogenous abundance may be omitted. This method combined the two advantages of targeted metabolomics and nontargeted metabolomics analysis, and has been demonstrated to achieve simultaneous nontargeted profiling and targeted NTs quantitative analysis of the same biological sample in a single needle injection. It provides an effective and accurate research tool for metabolomics analysis.
Schizophrenia is a clinical syndrome composed of a group of symptoms, which is a multifactorial disease that is affected by both genetic and environmental factors, and its' mechanism is still unclear. There are three main biochemical hypotheses regarding the pathogenesis of schizophrenia: dopamine hypothesis; serotonin hypothesis; glutamate hypothesis. Based on the above hypothesis, a number of antipsychotic drugs have been developed, such as chlorpromazine, risperidone,43 and OLZ. In this study, we quantified the NTs with wide dynamic range by a tSIM scan and simultaneously acquire nontargeted metabolic profiling by the full MS scan, respectively, and discovered the metabolic changes especially the metabolic pathway on NTs in the body caused by drugs, further supporting the explanation of drug responses in molecular level.
It is well known that, as the first-line drug for the treatment of schizophrenia, OLZ has severe clinical problems in treatment, including narrow therapeutic window, large individual differences, weight gain, dyslipidemia and insulin resistance side effects. At present, there is no evaluation index of curative effect and individualized prognosis of such drugs. Therefore, this method would have the potential to provide novel insights into the molecular alterations for the treatment of neurodegenerative diseases and mental illness. It is expected to discover the comprehensive metabolic changes for the understanding of drug treatment response and the molecular mechanisms of drug effects in vivo, and providing a basis for individualized drug treatment and precise medication.
4. Conclusions
In this study, a simultaneous targeted NTs quantification and nontargeted metabolomics method was developed and validated for pharmacometabolomics analysis by UPLC-HRMS. By using this strategy, NTs with very low physiological content can be sensitively detected and accurately quantified in biological samples. Moreover, simultaneously nontargeted profiling were also achieved. Then the newly established UPLC-HRMS method was further applied to the pharmacometabolomics study of OLZ. Results showed that 5-HT, 5-HIAA, L-DOPA and GABA were decreased. The levels of Tyr were increased. Furthermore, nontargeted metabolomics analysis revealed altered metabolic pathways after drug treatment, including glycerophospholipid metabolism, sphingolipid metabolism, and the citrate cycle. These results can further provide a basis for studying the mechanism of OLZ in the treatment of schizophrenia.
Overall, these results demonstrated that this targeted NTs quantification and nontargeted metabolic profiling method has the advantages of efficiency and accuracy for pharmacometabolomics research of antipsychotic drugs. And it would be a powerful tool to provide novel insights into the molecular alterations of their action for the treatment of neurodegenerative diseases and mental illness.
Conflicts of interest
All authors report no conflicts of interest.
Acknowledgements
This work was supported by the National Natural Science Foundation of China (Grant No. 81773678), the Beijing Municipal Natural Science Foundation (project 7192074), and the CAMS Innovation Fund for Medical Sciences (Grant No. 2019-I2M-1-005).
Notes and references
- M. J. Owen, A. Sawa and P. B. Mortensen, Lancet, 2016, 388, 86–97 CrossRef.
- H. Y. Meltzer, Psychopharmacology, 2000, 148, 16–19 CrossRef CAS PubMed.
- P. Mackin and S. H. Thomas, BMJ, 2011, 342, d1126 CrossRef PubMed.
- A. Hasan, P. Falkai, T. Wobrock, J. Lieberman, B. Glenthoj, W. F. Gattaz, F. Thibaut and H. J. Möller, World J. Biol. Psychia., 2012, 13, 318–378 CrossRef PubMed.
- J. A. Lieberman, F. P. Bymaster, H. Y. Meltzer, A. Y. Deutch, G. E. Duncan, C. E. Marx, J. R. Aprille, D. S. Dwyer, X. M. Li, S. P. Mahadik, R. S. Duman, J. H. Porter, J. S. Modica-Napolitano, S. S. Newton and J. G. Csernansky, Pharmacol. Rev., 2008, 60, 358–403 CrossRef CAS PubMed.
- D. Amato, F. Canneva, P. Cumming, S. Maschauer, D. Groos, J. K. Dahlmanns, T. W. Grömer, L. Chiofalo, M. Dahlmanns, F. Zheng, J. Kornhuber, O. Prante, C. Alzheimer, S. von Hörsten and C. P. Müller, Mol. Psychiatry, 2018 DOI:10.1038/s41380-018-0114-5.
- L. Del Fabro, G. Delvecchio, A. D'Agostino and P. Brambilla, Hum. Psychopharmacol., 2019, 34, e2693 CrossRef PubMed.
- S. K. Jha, N. K. Jha, D. Kumar, R. Sharma, A. Shrivastava, R. K. Ambasta and P. Kumar, J. Alzheimer's Dis., 2017, 57, 1017–1039 CAS.
- X. Zhang, Y. Zhao, Y. Liu, Y. Yuan, H. Shao and X. Zheng, Biochem. Pharmacol., 2018, 158, 114–125 CrossRef CAS PubMed.
- J. A. Lieberman, T. S. Stroup, J. P. McEvoy, M. S. Swartz, R. A. Rosenheck, D. O. Perkins, R. S. Keefe, S. M. Davis, C. E. Davis, B. D. Lebowitz, J. Severe and J. K. Hsiao, N. Engl. J. Med., 2005, 353, 1209–1223 CrossRef CAS PubMed.
- M. Shobo, H. Yamada, T. Mihara, Y. Kondo, M. Irie, K. Harada, K. Ni, N. Matsuoka and Y. Kayama, Behav. Brain Res., 2011, 216, 561–568 CrossRef CAS PubMed.
- C. Kowalchuk, C. Teo, V. Wilson, A. Chintoh, L. Lam, S. M. Agarwal, A. Giacca, G. J. Remington and M. K. Hahn, Journal of Psychiatry & Neuroscience, 2017, 42, 424–431 Search PubMed.
- H. Li, M. Fang, M. Xu, S. Li, J. Du, W. Li and H. Chen, PLoS One, 2016, 11, e0167930 CrossRef PubMed.
- W. B. Dunn and D. I. Ellis, TrAC, Trends Anal. Chem., 2005, 24, 285–294 CrossRef CAS.
- D. S. Wishart, Nat. Rev. Drug Discovery, 2016, 15, 473–484 CrossRef CAS PubMed.
- Z. Zhang and W. Tang, Acta Pharm. Sin. B, 2018, 8, 721–732 CrossRef PubMed.
- A. Ribbenstedt, H. Ziarrusta and J. P. Benskin, PLoS One, 2018, 13, e0207082 CrossRef PubMed.
- S. Naz, M. Vallejo, A. García and C. Barbas, J. Chromatogr. A, 2014, 1353, 99–105 CrossRef CAS PubMed.
- E. C. Considine, G. Thomas, A. L. Boulesteix, A. S. Khashan and L. C. Kenny, Metabolomics, 2017, 14, 7 CrossRef CAS PubMed.
- A. C. Schrimpe-Rutledge, S. G. Codreanu, S. D. Sherrod and J. A. McLean, J. Am. Soc. Mass Spectrom., 2016, 27, 1897–1905 CrossRef CAS PubMed.
- W. B. Dunn, A. Erban, R. J. M. Weber, D. J. Creek, M. Brown, R. Breitling, T. Hankemeier, R. Goodacre, S. Neumann, J. Kopka and M. R. Viant, Metabolomics, 2013, 9, 44–66 CrossRef CAS.
- J. Zhou, H. Liu, Y. Liu, J. Liu, X. Zhao and Y. Yin, Anal. Chem., 2016, 88, 4478–4486 CrossRef CAS PubMed.
- Y. Qiao, L. Zhang, S. He, H. Wen, Y. M. Yu, C. H. Cao and H. F. Li, Neurosci. Lett., 2016, 617, 270–276 CrossRef CAS PubMed.
- P. Keski-Rahkonen, M. Lehtonen, J. Ihalainen, T. Sarajärvi and S. Auriola, Rapid Commun. Mass Spectrom., 2007, 21, 2933–2943 CrossRef CAS PubMed.
- M. S. Bergh, I. L. Bogen, E. Lundanes and Å. M. L. Øiestad, J. Chromatogr. B: Anal. Technol. Biomed. Life Sci., 2016, 1028, 120–129 CrossRef CAS PubMed.
- X. E. Zhao, Y. He, P. Yan, N. We, R. Wang, J. Sun, L. Zheng, S. Zhu and J. You, RSC Adv., 2016, 6, 108635 RSC.
- Y. Gao, Y. Chen, X. Yue, J. He, R. Zhang, J. Xu, Z. Zhou, Z. Wang, R. Zhang and Z. Abliz, Anal. Chim. Acta, 2018, 1037, 369–379 CrossRef CAS PubMed.
- J. Adrien, Sleep Medicine Reviews, 2002, 6, 341–351 CrossRef.
- F. G. Revel, J. Gottowik, S. Gatti, J. G. Wettstein and J. L. Moreau, Neurosci. Biobehav. Rev., 2009, 33, 874–899 CrossRef PubMed.
- T. K. Kiang, M. H. Ensom and T. K. Chang, Pharmacol. Ther., 2005, 106, 97–132 CrossRef CAS PubMed.
- N. Yang, R. Sun, X. Liao, J. Aa and G. Wang, Pharmacol. Res., 2017, 121, 169–183 CrossRef CAS PubMed.
- S. Xu, R. P. Gullapalli and D. O. Frost, Schizophrenia Research, 2015, 161, 452–457 CrossRef PubMed.
- H. Y. Meltzer and B. W. Massey, Curr. Opin. Pharmacol., 2011, 11, 59–67 CrossRef CAS PubMed.
- A. Demjaha, R. M. Murray, P. K. McGuire, S. Kapur and O. D. Howes, Am. J. Psychiatry, 2012, 169, 1203–1210 CrossRef PubMed.
- B. Stapel, A. Kotsiari, M. Scherr, D. Hilfiker-Kleiner, S. Bleich, H. Frieling and K. G. Kahl, J. Psychiatr. Res., 2017, 88, 18–27 CrossRef PubMed.
- B. Garip, H. Kayir and O. Uzun, Schizophrenia Research, 2019, 206, 58–66 CrossRef PubMed.
- W. Liu, A. Le, C. Hancock, A. N. Lane, C. V. Dang, T. W. Fan and J. M. Phang, Proc. Natl. Acad. Sci. U. S. A., 2012, 109, 8983–8988 CrossRef CAS PubMed.
- C. H. Chen, S. K. Shyue, C. P. Hsu and T. S. Lee, Cell. Physiol. Biochem., 2018, 50, 1216–1229 CrossRef CAS PubMed.
- R. C. Smith, J. P. Lindenmayer, Q. Hu, E. Kelly, T. F. Viviano, J. Cornwell, S. Vaidhyanathaswamy, S. Marcovina and J. M. Davis, Schizophrenia Research, 2010, 120, 204–209 CrossRef PubMed.
- K. Yuyama, S. Mitsutake and Y. Igarashi, Biochim. Biophys. Acta, 2014, 1841, 793–798 CrossRef CAS PubMed.
- V. L. Albaugh, T. C. Vary, O. Ilkayeva, B. R. Wenner, K. P. Maresca, J. L. Joyal, S. Breazeale, T. D. Elich, C. H. Lang and C. J. Lynch, Schizophrenia Bulletin, 2012, 38, 153–166 CrossRef PubMed.
- R. Kaddurah-Daouk, J. McEvoy, R. A. Baillie, D. Lee, J. K. Yao, P. M. Doraiswamy and K. R. Krishnan, Mol. Psychiatry, 2007, 12, 934–945 CrossRef CAS PubMed.
- O. Howes, R. McCutcheon and J. Stone, J. Psychopharmacol., 2015, 29, 97–115 CrossRef PubMed.
Footnotes |
† Electronic supplementary information (ESI) available. See DOI: 10.1039/d0ra02406f |
‡ These authors contributed equally to this work. |
|
This journal is © The Royal Society of Chemistry 2020 |
Click here to see how this site uses Cookies. View our privacy policy here.