DOI:
10.1039/D0RA02061C
(Paper)
RSC Adv., 2020,
10, 20322-20337
Assessment of ampicillin removal efficiency from aqueous solution by polydopamine/zirconium(IV) iodate: optimization by response surface methodology†
Received
4th March 2020
, Accepted 13th May 2020
First published on 27th May 2020
Abstract
Polydopamine/zirconium(IV) iodate was prepared by incorporating polydopamine into zirconium iodate gel and studied as an effective adsorbent for ampicillin. In order to characterize the prepared composite, FTIR, XRD, TGA-DTA, SEM and TEM were used. The effects of experimental variables on ampicillin removal were examined using response surface methodology. The optimum conditions for ampicillin removal were 7, 130 min, 20 mg/20 mL and 50 mg L−1 for pH, contact time, adsorbent dose and initial ampicillin concentration, respectively. Under the optimum conditions, the maximum ampicillin removal percentage was found to be 99.12%. The Langmuir isotherm and pseudo-second-order kinetic models explained the removal process more appropriately. The maximum adsorption capacity at 303 K was 100.0 mg g−1. Thermodynamic study revealed that the ampicillin adsorption was spontaneous and endothermic in nature. The reusability of the prepared material was also explored.
1. Introduction
The occurrence of new emerging pollutants in the aquatic environment is of prime concern to researchers worldwide.1,2 Antibiotics are considered as emerging pollutants which have been found in various water systems.3 Antibiotics are of great importance as they have been used for the therapeutic treatment of infection related diseases in both humans and animals.4 The major sources of antibiotics in the aqueous environment mainly originate from hospital effluents, pharmaceutical industries and municipal wastewaters.5 Although the concentration of antibiotic residues in the environment is low but studies have revealed that these antibiotics are practically non-biodegradable and may result in the production of antibiotic resistant bacteria and antibiotic resistance genes.6 Ampicillin is a β-lactam, “broad spectrum” penicillin group antimicrobial agent which is used to treat against Gram-positive and Gram-negative bacteria.7 Ampicillin binds to peptidoglycan synthesizing enzymes and inhibits the synthesis of the cell wall.8 It is also helpful in the treatment of bacterial infections, such as ear infections, respiratory tract infections, endocarditis and to prevent group B streptococcal infections in newborns.9–11 It may also be used for utero therapy.12 Ampicillin is used for the treatment of clostridia infections in chickens.13 Therefore, the removal of these non-biodegradable antibiotics from environmental water has received great attention.
Several methods have been developed to deal with antibiotic contaminants in wastewater, including oxidation,14 degradation,15,16 electrodegradation,17 reverse osmosis,18 coagulation,19 nanofilteration membranes,20 photocatalytic degradation21 and adsorption.22 Adsorption is widely available technique due to its inherently high efficiency, operational simplicity and economic feasibility. However, the efficiency of adsorption method is highly affected by the properties of adsorbents. To date, several sorbents were utilized to adsorb penicillin group antibiotics from contaminated water. In recent years, low-cost naturally occurring biosorbents such as decaffeinated tea waste,23 palm bark biomass,24 rice husk,25 Rhizopus arrhizus and activated sludge26 were successfully utilized for the removal of antibiotics. Granular activated carbon was also tested to adsorb ampicillin from water at pH 6. The removal efficiency (73%) was poor and requiring 120 min of contact time.27 Carbon materials modified by liquid nitrogen treatment was used to remove ampicillin but its efficiency was 92.31% at pH 7.0.28 The adsorption behavior of ampicillin onto hydroxyapatite was investigated and the adsorption data followed the Freundlich isotherm model.29 Weng et al.30 have examined the adsorption characteristics of bentonite-supported nanoscale Fe/Ni for the decontamination of β-lactam antibiotics. The study revealed that only 85.1% of ampicillin was removed from aqueous solution. In addition, myristyltrimethylammonium intercalated montmorillonite was used as adsorbent with ampicillin adsorption capacity of 49.96 mg g−1.31 Rahardjo et al.32 have modified the bentonite by treating with cetyltrimethylammonium bromide and tested as sorbent to adsorb ampicillin. Single and multi-walled carbon nanotubes were adopted to extract penicillin G from aqueous solutions.33 Graphene sand composite and chitosan modified with Bi2O3/BiOCl were found to have selective affinity for the adsorption of ampicillin.34 Amino functionalized ordered mesoporous silica35 exhibited its potential for ampicillin removal.
Currently, synthesis of organic–inorganic composite materials is gaining attention because of their multifunctional and enhanced adsorption properties.36–39 Polydopamine is highly conjugated organic material and have abundant adhesive functional groups. It can be synthesized by the oxidative polymerization of dopamine in acidic and slightly alkaline conditions.40,41 Due to strong adhesion ability, versatile chemical reactivity and chemical stability of polydopamine, a variety of polydopamine based composite materials has been prepared for environmental applications.42,43 Moreover, the catechol groups and nitrogen heteroatoms of polydopamine are active sites for heavy metal ions and other organic pollutants through electrostatic, bidentate chelating or hydrogen bonding interactions.44 Recently, polydopamine based composite materials have demonstrated their potential in the removal of pollutants from aqueous solutions. Zeng et al.45 have designed a biosorbent based on pullulan and polydopamine for uptake of Cu2+, Co2+ and Ni2+ ions. Polydoapmine-functionalized graphene oxide was used for preparation of polyvinyl alcohol/chitosan/polydopamine–graphene oxide hydrogel.46 The material was found to have enhanced adsorption capacities for Cu2+, Pb2+ and Cd2+ ions. Zhao et al.47 have synthesized polydopamine/graphene oxide (PDA/GO) by self-polymerization of dopamine monomers on graphene oxide surface. PDA/GO has effectively removed U(VI) from aqueous solution. GO/PDA-β-cyclodextrin ultrafiltration membrane was used for rejection of methylene blue and uptake of trace Pb2+ ions from aqueous solution.48 Wang et al.49 have prepared polycaprolactone/polyethylene oxide@polydopamine composite with numerous functional groups for uptake of methylene blue and methyl orange. In this study, polydopamine with its reactive functional groups, imine and hydroxyl groups, was combined with thermally stable zirconium(IV) iodate to synthesize the composite material and explored its potential for the removal of antibiotics. The material was found to have higher adsorption capacity for ampicillin as compared to other adsorbents.27,31,32
The optimization of variables of analytical methods and adsorption processes50–52 has been accomplished mostly by one-factor-at-a time (OFAT) approach. Therefore, OFAT experimental designs for optimization of all variables are expensive and time consuming. Moreover, it does not give any interactional effects of variables on response. Alternatively, response surface methodology (RSM) can be useful to minimize the difficulties of OFAT approach. RSM is applied to study the relationships between the response and several independent factors using a second-degree polynomial model. Recently, RSM is used in the adsorptive removal processes for optimization of independent variables and determination of relative significance of several parameters.53–56
The aim of this work was to develop polydopamine/zirconium(IV) iodate (PDA/ZI) for ampicillin removal from water. The composite material was characterized by different analytical techniques including Fourier transform infrared spectroscopy (FTIR), X-ray diffraction (XRD), scanning/transmission electron microscopy (SEM/TEM), and thermogravimetric analysis-differential thermal analysis (TGA-DTA). Box–Behnken design (BBD) under RSM was utilized for optimizing and examining the effects of pH, contact time, adsorbent dose and initial ampicillin concentration on the percent ampicillin removal. Additionally, the studies of adsorption isotherms, kinetics, thermodynamics and the reusability of PDA/ZI composite were also performed.
2. Experimental
2.1 Reagents and materials
Ampicillin was procured from Sigma chemical company, St. Louis (USA). Dopamine hydrochloride and zirconium oxychloride were purchased from Himedia Laboratories (India) and Otto Chemie (India), respectively. Potassium iodate was obtained from Merck (India).
2.2 Characterization
FTIR spectrum of PDA/ZI composite was recorded on PerkinElmer FTIR spectrophotometer using KBr pellet method. The surface morphology of PDA/ZI composite and ampicillin loaded PDA/ZI was studied by scanning electron microscopy using a JEOL-JSM 6510LV scanning electron microscope. The TEM images were obtained through conventional transmission electron microscopy [JEM 2100 JEOL, Japan]. Thermal analysis was performed using a thermogravimetric analyzer (Shimadzu DTG-60H, Japan). The heating was carried in the range of 20 °C to 800 °C at a rate of 20 °C min−1 in nitrogen atmosphere. The powder X-ray diffractions were performed on Bruker AXS D8 advance diffractometer operating at 40 kV and 35 mA equipped with a Cu-Kα radiation. The scanning rate was fixed at 0.02° s−1 in 2θ range of 5° to 80°. A UV-Visible spectrophotometer (Shimadzu UV 1800, Japan) was used to determine the ampicillin in solutions. The measurement of pH of working solutions was performed using digital pH meter (model: Cyberscan pH 2100, Eutech instruments, Singapore). The sample solution was agitated at the desired temperature using a water bath shaker (Narang Scientific Works, India).
2.3 Synthesis of polydopamine/zirconium(IV) iodate (PDA/ZI)
The synthesis of polydopamine was conducted following the method of Zheng et al.57 For this, 0.3 g of dopamine hydrochloride was dissolved in 10 mL of 0.1 M HCl solution and heated at 80 °C for 20 h. The dark brown colored product was collected after cooling the content of the flask. Zirconium iodate was obtained on mixing 0.10 M ZrOCl2·8H2O (100 mL) and 0.10 M potassium iodate (100 mL) with stirring for 4 h at 27 °C (ref. 58) (Scheme 1). The gel of zirconium(IV) iodate was mixed with the prepared polydopamine with stirring for 8 h at 27 °C. Finally, the product was collected after filtration and dried at 50 °C in an oven (Scheme 2).
 |
| Scheme 1 Synthesis of zirconium(IV) iodate gel. | |
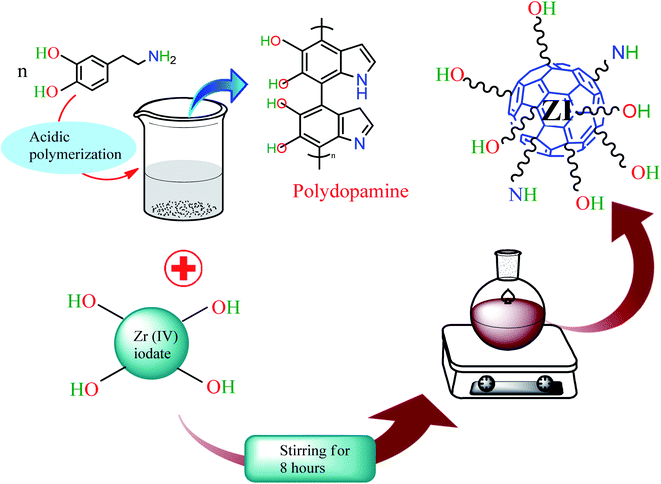 |
| Scheme 2 Synthesis of PDA/ZI composite. | |
2.4 Adsorption studies
For optimization of experimental variables, experiments were conducted according to Box–Behnken design. A known amount of adsorbent ranging from 0.01 to 0.03 g was added to 20 mL of ampicillin of varying concentration ranging from 30.0 to 70.0 mg L−1 in a 50 mL conical flask. The solution pH was adjusted from 4.0 to 10.0 using 0.10 M HCl or 0.10 M NaOH. The resulting mixture was stirred at different time intervals ranging from 50 to 210 min. Aliquots were taken from the system at different time intervals. The ampicillin concentration was determined spectrophotometrically at 511 nm.59 The adsorption capacity and percentage removal of ampicillin was calculated using the following equations: |
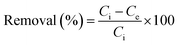 | (1) |
|
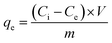 | (2) |
where, Ci and Ce (mg L−1) are the initial and equilibrium concentrations of ampicillin respectively, V and m are the solution volume (L) and mass of adsorbent (g), respectively.
2.5 Box–Behnken experimental design
BBD under RSM was applied to optimize the experimental variables (pH, contact time, adsorbent dose and initial concentration) to achieve the maximum response. Each factor was studied at three levels (Table S1†). A total of 29 experimental runs were needed for the development of BBD and the actual experimental design matrix was developed using Design-Expert 11.0 (trial version) software and is illustrated in Table 1.
Table 1 Box–Behnken design matrix with actual and predicted responses
Experimental run |
Independent variables |
Response |
A: pH |
B: contact time (min) |
C: adsorbent dose (mg per 20 mL) |
D: initial concentration (mg L−1) |
Actual removal (%) |
Predicted removal (%) |
1 |
7 |
130 |
20 |
50 |
99.12 |
99.12 |
2 |
7 |
210 |
20 |
70 |
82.29 |
81.95 |
3 |
7 |
50 |
10 |
50 |
58.57 |
58.64 |
4 |
7 |
210 |
20 |
30 |
99.12 |
99.11 |
5 |
4 |
210 |
20 |
50 |
78.88 |
79.10 |
6 |
7 |
130 |
20 |
50 |
99.12 |
99.12 |
7 |
10 |
130 |
20 |
70 |
63.05 |
62.93 |
8 |
7 |
50 |
20 |
30 |
77.09 |
77.49 |
9 |
7 |
130 |
10 |
70 |
61.77 |
62.33 |
10 |
7 |
130 |
20 |
50 |
99.12 |
99.12 |
11 |
7 |
210 |
10 |
50 |
83.6 |
83.27 |
12 |
7 |
130 |
20 |
50 |
99.12 |
99.12 |
13 |
7 |
50 |
20 |
70 |
57.26 |
57.32 |
14 |
4 |
130 |
20 |
70 |
58.05 |
57.96 |
15 |
4 |
130 |
10 |
50 |
59.36 |
59.35 |
16 |
10 |
130 |
10 |
50 |
64.36 |
64.18 |
17 |
7 |
130 |
10 |
30 |
82.6 |
82.49 |
18 |
7 |
130 |
20 |
50 |
99.12 |
99.12 |
19 |
7 |
210 |
30 |
50 |
99.12 |
99.17 |
20 |
4 |
130 |
20 |
30 |
77.88 |
78.12 |
21 |
10 |
130 |
30 |
50 |
80.15 |
80.22 |
22 |
7 |
130 |
30 |
70 |
81.29 |
81.23 |
23 |
7 |
130 |
30 |
30 |
99.12 |
98.39 |
24 |
10 |
130 |
20 |
30 |
79.88 |
80.09 |
25 |
10 |
210 |
20 |
50 |
80.25 |
80.66 |
26 |
4 |
130 |
30 |
50 |
77.88 |
78.11 |
27 |
4 |
50 |
20 |
50 |
54.65 |
54.06 |
28 |
7 |
50 |
30 |
50 |
77.09 |
77.54 |
29 |
10 |
50 |
20 |
50 |
59.85 |
59.45 |
The measured data were fitted to a second degree polynomial model which is expressed as:
|
 | (3) |
where,
Y,
Xi and
Xj presents response (predicted) and experimental variables.
β0,
βi,
βii and
βij are the regression coefficients for the intercept, linear, interaction and quadratic terms, respectively;
ε defines the error.
2.6 Desirability function
Desirability function60 was used to find the independent variables to reach simultaneously the optimum value. In this approach, each response was changed into individual desirability function, di, and establishing the optimization criteria. When di = 0, the response is considered undesirable whereas di = 1, exhibiting the response is completely desirable.
In order to obtain the individual desirability within an acceptable range of response values (Ru − Rl) where, Ru is the upper acceptable value and Rl is the lower, the transformations can be performed in several ways.
(i) The value of di was obtained from eqn (4) when the response has to be maximized.
|
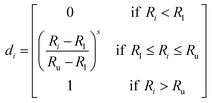 | (4) |
where
s is a power value called ‘weight’. Analyst set
s > 1 to judge the importance of ‘weight’ for
Ri to be close to the maximum.
Once the n variables (factors and responses) are converted into desirability functions, then the individual desirability scores are combined to give global desirability, D, according to the following equation:
|
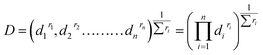 | (5) |
where
ri is the importance of each variable relative to the other. The global desirability is optimized to get the optimum set of input variables.
2.7 Error analysis
A statistical approach for comparing the fitness of adsorption isotherms and kinetic models was carried out by calculating the chi-square (χ2) and average percentage error (APE) values. These error functions employed are as follows: |
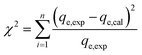 | (6) |
|
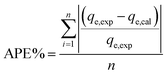 | (7) |
where, qe,exp is the experimentally obtained capacity, qe,cal is the capacity computed from the model and n defines the number of experimental runs. The best fitted model can be determined using the minimum values of χ2 and APE.
2.8 Desorption and reuse experiments
The reuse of PDA/ZI was explored by conducting sorption–desorption cycles. For desorption studies, 10 mL of NaOH solution (0.5 M) was added to the ampicillin loaded PDA/ZI (0.06 g). The mixture was shaken at 120 rpm for 20 minutes at room temperature. After filtration, ampicillin concentration in the filtrate was obtained by measuring absorbance at 511 nm. PDA/ZI was washed with distilled water till the washing became neutral and then treated with 0.10 M HCl solution for 1 h. After washing with distilled water, it was dried.
3. Results and discussion
3.1 Characterization
FTIR spectrum of PDA/ZI (Fig. 1) displays a broad absorption band in the range of 3600–3200 cm−1 which confirmed the presence of N–H and O–H groups of polydopamine. A strong band centred at 1618 cm−1 corresponds to benzene ring –C
C– stretching.61 The band at 1417 cm−1 is assigned to in-plane deformation of phenolic hydroxyls present in polydopamine.62 The band at 1340 cm−1 arises due to C–N stretching.63 The deformation vibration of C–H (aromatic) is revealed by the absorption bands peaking at 1284 cm−1 and 1121 cm−1.63 The absorption band centered at 785 cm−1 is indicative of iodate group in the material.64 The bands peaking at 623 cm−1 and 482 cm−1 are linked to Zr–O lattice vibration.65 The peak observed at 2029 cm−1 is assigned to N+–H deformation mode because the material was prepared in acidic conditions.63 These results suggested that the PDA/ZI composite was formed successfully.
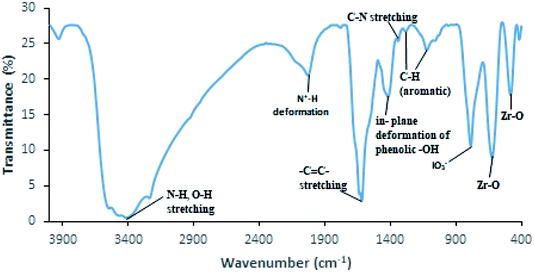 |
| Fig. 1 FTIR spectrum of PDA/ZI composite. | |
The X-ray diffraction pattern of PDA/ZI composite (Fig. S1†) shows few broad peaks at 2θ of 13.062, 26.383, 58.346 and 76.985 with d-spacing of 6.772, 3.375, 1.580 and 1.273 Å, respectively. This study showed the semi-crystalline nature of the material.
The thermal stability of PDA/ZI was examined in the range of 20° to 800 °C. TGA and DTA curves are shown in Fig. 2. In the first step, the weight loss was 8.44% up to 140 °C which is due to the elimination of external water present on the surface of composite. Similar observations were also reported for the removal of water from polyacrylamide zirconium(IV) iodate66 and zirconium(IV) selenoiodate.67 Secondly, weight loss of about 20.09% was observed in the temperature range of 140–280 °C. The weight loss is associated with the loss of I2 molecule, and decomposition of amino groups present in polydopamine polymeric backbone.68–70 This decomposition process is also confirmed by DTA curve with an exothermic peak at 210 °C. In the third step, the weight loss was about 12.30% from 280–520 °C. In this transition range, the degradation of main polydopamine chain occurs. Luo et al.71 have also reported the degradation of polydopamine chain in silver nanoparticles loaded polydopamine spheres. The weight loss between 520–580 °C (35.37%) was due to the formation of zirconium oxide. It is concluded that the adsorbent is thermally stable and can be used as an adsorbent for removal of ampicillin from water.
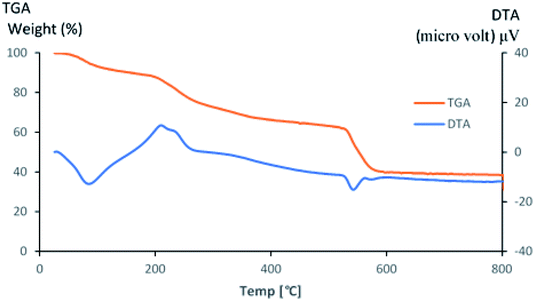 |
| Fig. 2 TGA and DTA curves of PDA/ZI composite. | |
SEM and TEM were used to study the morphology of the material. TEM image of PDA/ZI composite revealed that the average size of the particle was about 22 nm (Fig. 3a). The average size of the ampicillin loaded PDA/ZI composite particle (Fig. 3b) was found to be 36 nm which confirmed the adsorption of ampicillin onto PDA/ZI composite. SEM image of zirconium(IV) iodate (Fig. 4a) shows an irregular surface with pores of varying dimensions. The surface of the material became more irregular after incorporation of polydopamine (Fig. 4b). Additionally, the morphology of PDA/ZI has considerably changed after ampicillin adsorption (Fig. 4c). The morphological study has also pointed towards the successful adsorption of ampicillin onto PDA/ZI.
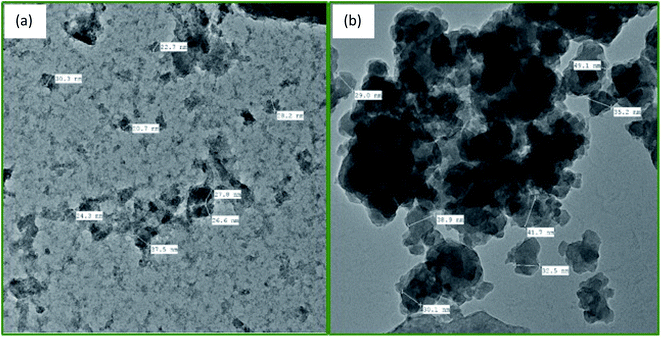 |
| Fig. 3 TEM images of (a) PDA/ZI composite, and (b) ampicillin loaded PDA/ZI. | |
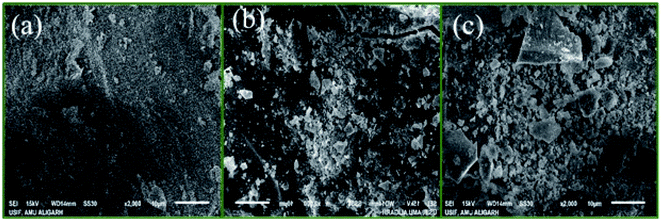 |
| Fig. 4 SEM images of (a) ZI, (b) PDA/ZI composite, and (c) ampicillin loaded PDA/ZI composite. | |
3.2 Box–Behnken statistical analysis and the model fitting
Box–Behnken design matrix includes pH (A), contact time (B), adsorbent dose (C) and ampicillin concentration (D) along with experimental response (percentage removal) (Table 1). The experimental data were fitted to polynomial models such as linear, 2-factor interaction and quadratic to obtain the regression equations and model statistics are shown in Table S2.† Model statistics were compared to find the best model representing the removal of ampicillin by PDA/ZI. The results revealed that the quadratic model has highest R2 (0.9996). Moreover, the results also showed higher F-value (4574.71) and lower values of standard deviation (0.41), predicted residual error sum of squares (13.80) and p-value (<0.0001) for quadratic model as compared to other models tested. Therefore, the measured data were fitted to second-order polynomial equation to correlate the response to the four selected variables and is expressed as: |
Y(% Removal) = −146.34442 + 32.039(A) + 0.607(B) + 4.4280(C) + 1.6475(D) − 0.0040(A × B) − 0.0228(A × C) + 0.0125(A × D) − 0.0009(B × C) + 0.0005(B × D) + 0.008(C × D) − 2.2217(A)2 − 0.0017(B)2 − 0.0866(C)2 − 0.0234(D)2
| (8) |
Analysis of variance (ANOVA) was adopted to judge the correctness of the model equation (Table 2). The results showed a high correlation between the measured and predicted responses because there is a closeness between R2 (0.9996) and adjusted R2 (0.9993). The values of F (2813.08) and p (<0.0001) for the model demonstrated its statistical significance. Moreover, the significance of each of the linear terms, the interaction terms and quadratic terms on the adsorption (%) of ampicillin was investigated on the basis of p-values. The model terms possessing p-values < 0.05 are regarded as significant. Here, all the linear terms, interaction terms and quadratic terms have p-value less than 0.05 and hence, all these terms are significant. The sign before the individual, interactive or quadratic terms as shown in eqn (8) indicated the positive or negative effect of model terms on the uptake of ampicillin. The model terms positively affecting on the removal efficiency were A, B, C, D, AD, BD and CD. On the other hand, the negative coefficient values of the interaction terms (such as AB, AC and BC) and quadratic terms (A2, B2, C2 and D2) indicated that these terms negatively affect the percentage removal of ampicillin. The plot of experimental response versus predicted response (Fig. S2†) showed the data points are homogeneously distributed on either side along the straight line. This indicated the adequacy and suitability of the quadratic model for analysis and optimization of ampicillin removal by PDA/ZI.
Table 2 ANOVA results and percent contributions of each variable for the developed response surface quadratic model
Source |
Sum of squares |
df |
Mean square |
F-Value |
p-Value prob > F |
Remark |
VIF |
PC |
Model |
6739.51 |
14 |
481.39 |
2813.08 |
<0.0001 |
Significant |
|
|
A – pH |
36.19 |
1 |
36.19 |
211.49 |
<0.0001 |
Significant |
1.0000 |
0.537 |
B – contact time |
1604.30 |
1 |
1604.30 |
9374.90 |
<0.0001 |
Significant |
1.0000 |
23.804 |
C – adsorbent dose |
908.11 |
1 |
908.11 |
5306.63 |
<0.0001 |
Significant |
1.0000 |
13.474 |
D – initial conc. |
1044.96 |
1 |
1044.96 |
6106.35 |
<0.0001 |
Significant |
1.0000 |
15.505 |
AB |
3.67 |
1 |
3.67 |
21.43 |
0.0004 |
Significant |
1.0000 |
0.054 |
AC |
1.86 |
1 |
1.86 |
10.89 |
0.0053 |
Significant |
1.0000 |
0.028 |
AD |
2.25 |
1 |
2.25 |
13.15 |
0.0028 |
Significant |
1.0000 |
0.033 |
BC |
2.25 |
1 |
2.25 |
13.15 |
0.0028 |
Significant |
1.0000 |
0.033 |
BD |
2.25 |
1 |
2.25 |
13.15 |
0.0028 |
Significant |
1.0000 |
0.033 |
CD |
2.25 |
1 |
2.25 |
13.15 |
0.0028 |
Significant |
1.0000 |
0.033 |
A2 |
2593.30 |
1 |
2593.30 |
15 154.25 |
<0.0001 |
Significant |
1.08 |
38.479 |
B2 |
757.11 |
1 |
757.11 |
4424.26 |
<0.0001 |
Significant |
1.08 |
11.234 |
C2 |
486.60 |
1 |
486.60 |
2843.50 |
<0.0001 |
Significant |
1.08 |
7.220 |
D2 |
567.06 |
1 |
567.06 |
3313.71 |
<0.0001 |
Significant |
1.08 |
8.414 |
Residual |
2.40 |
14 |
0.1711 |
|
|
|
|
|
Lack of fit |
2.40 |
10 |
0.2396 |
|
|
|
|
|
Pure error |
0.0000 |
4 |
0.0000 |
|
|
|
|
|
Cor total |
6741.90 |
28 |
|
|
|
|
|
|
Std. dev. |
0.4137 |
R2 |
0.9996 |
|
|
|
|
|
Mean |
78.92 |
Adjusted R2 |
0.9993 |
|
|
|
|
|
C.V.% |
0.5242 |
Predicted R2 |
0.9980 |
|
|
|
|
|
P |
13.80 |
Adeq precision |
151.5971 |
|
|
|
|
|
3.3 Interaction effects of variables and response surface
Response surface plots (3D) were used for determining the significance of binary interactions between the selected variables while keeping the other variables constant. Fig. 5a displays the combined effect of pH and contact time on percent ampicillin removal. At any fixed pH, the uptake increases with increasing contact time and 99.12% removal of ampicillin (maximum removal) was obtained at 130 min. The interactive effect of pH and adsorbent dosage on ampicillin removal (Fig. 5b) shows that on increasing the mass of adsorbent, the removal efficiency increases. The increase in the ampicillin removal percentage with increasing adsorbent dosage was expected because more active sites are available for ampicillin capture. The 3D-plot (Fig. 5c) displays the interactive effects of pH and ampicillin concentration on percent removal. The change in pH of the medium has a great impact on the surface of the adsorbent and ionization of ampicillin in solution. The pK1 (–COOH) and pK2 (–NH3+) of ampicillin are 2.5 and 7.3, respectively. Therefore, protonated ampicillin having –COOH and –NH3+ are deprotonated in the pH ranges 2–4 and 6–8, respectively.72 At any fixed initial concentration percent removal increases with increasing pH from 4 to 7 and then the removal efficiency gradually decreases up to pH 10. The maximum percent removal (99.12%) was observed at pH 7. This is because of the fact that at pH < 2.5, both adsorbent and ampicillin were positively charged, which retarded the adsorption of ampicillin onto PDA/ZI surface. At pH 7, ampicillin is deprotonated and providing negative sites which are available to interact with positive surface of PDA/ZI. Hence, at pH 7, maximum adsorption of ampicillin occurred. Further, at pH > 7 the adsorption of ampicillin was decreased due to the electrostatic repulsion between carboxylate group present in ampicillin and the negative surface charge of the adsorbent.73 Fig. 5d displayed the combined effect of adsorbent dose and contact time at pH 7 and initial concentration of ampicillin (50 mg L−1). The plot revealed that at lower adsorbent amounts, uptake decreases due to lesser number of binding sites for ampicillin. The interactive effect of ampicillin concentration and equilibrations time (Fig. 5e) suggested that uptake was increased with increasing initial ampicillin concentration at any fixed contact time. This is due to higher concentration gradient which facilitates the mass transfer from bulk solution to adsorbent surface.74 Fig. 5f shows the combined effect of concentration and adsorbent dose on uptake efficiency. Removal efficiency was found to increase with increasing concentration up to 50 mg L−1. Above 50 mg L−1, removal percentage decreases because the saturation rate increased and the more adsorption sites were being covered.
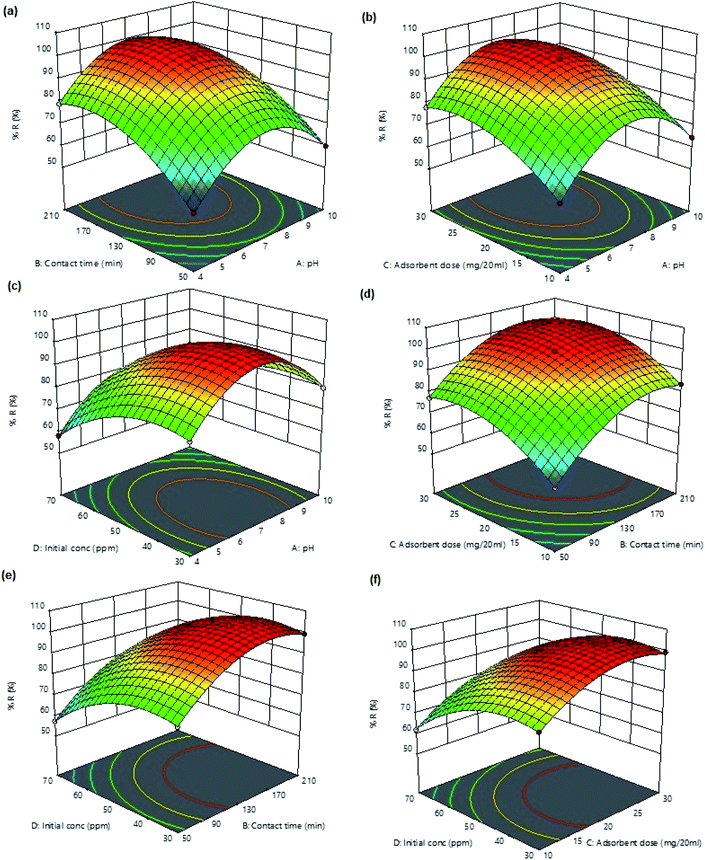 |
| Fig. 5 Response surface 3D plots for ampicillin percent removal versus (a) pH and contact time, (b) pH and adsorbent dose, (c) pH and initial concentration, (d) contact time and adsorbent dose, (e) contact time and initial concentration, and (f) adsorbent dose and initial concentration. | |
3.4 Verification of the model
Numerical optimization was studied by keeping independent variables in the selected ranges (pH: 4–10; contact time: 50–210 min; adsorbent dose: 20 mg/20 mL; initial concentration: 30–70 mg L−1) to obtain response at maximum level (Fig. S3†). The optimal conditions of pH, contact time, adsorbent dose and initial concentration of ampicillin were 7.0, 130 min, 20 mg/20 mL, and 50 mg L−1, respectively. RSM fitting model provided the predicted removal of 99.12%. Experiments in triplicate were conducted using the optimum values of independent variables and results were in well agreement with the predicted values. Therefore, RSM is accurate in predicting the removal efficiency of ampicillin by PDA/ZI.
3.5 Adsorption isotherms
Langmuir, Freundlich, Temkin and Dubinin–Radushkevich (D–R) isotherm models were applied to fit the adsorption data to understand the nature of interaction between ampicillin and PDA/ZI adsorbent at equilibrium. The non-linear equations of these isotherm models75 are given in Table 3. Microsoft Excel SOLVER function-spread sheet method76 was applied to evaluate the isotherm parameters. Fig. 6 and 7 show the plots of qe vs. Ce using experimental and predicted values by Langmuir and Freundlich isotherm models respectively. The values of KL, qm, KF and 1/n were computed by nonlinear regression analysis (Table 3). The characteristics of Langmuir isotherm is usually given by dimensionless parameter, RL.77 The numerical value of RL can be computed from eqn (9): |
 | (9) |
Table 3 Adsorption isotherm parameters obtained from non-linear fitting for adsorption of ampicillin onto PDA/ZI
Isotherm |
Temperature (K) |
Parameters |
Error function |
The experimental adsorption capacity for ampicillin (qe) are 99.946, 102.872, and 105.938 mg g−1 at 303, 313, and 323 K respectively. Where, qe and qm (mg g−1) are the adsorption capacity at equilibrium and simulated adsorption capacity, respectively. Ce (mg L−1) is the equilibrium concentration of ampicillin, KL (L mg−1) is the Langmuir adsorption constant. KF and n are the Freundlich constant and heterogeneity factor, respectively. AT (L g−1) is Temkin isotherm equilibrium binding constant, bT is Temkin isotherm constant associated with heat of sorption (J mol−1), R and T are universal gas constant (8.314 J mol−1 K−1) and temperature (Kelvin), respectively. KDR represents Dubinin–Radushkevich isotherm constant (mol2 J−2). |
Langmuir |
 |
|
qma (mg g−1) |
KL (L mg−1) |
RL |
R2 |
χ2 |
APE |
303 |
99.98 |
0.719 |
9.8 × 10−3 |
0.9965 |
1.16 × 10−5 |
−3.40 × 10−4 |
313 |
102.9 |
0.867 |
8.1 × 10−3 |
0.9974 |
7.62 × 10−6 |
−2.72 × 10−4 |
323 |
105.51 |
1.105 |
6.4 × 10−3 |
0.9997 |
1.73 × 10−3 |
4.04 × 10−3 |
![[thin space (1/6-em)]](https://www.rsc.org/images/entities/char_2009.gif) |
Freundlich |
qe = KFCe1/n |
|
qm (mg g−1) |
1/n |
KF |
R2 |
χ2 |
APE |
303 |
103.47 |
0.215 |
46.80 |
0.9940 |
0.12 |
−3.52 × 10−2 |
313 |
104.72 |
0.193 |
52.13 |
0.9938 |
0.03 |
−1.79 × 10−2 |
323 |
105.04 |
0.162 |
59.31 |
0.9979 |
7.61 × 10−3 |
8.48 × 10−3 |
![[thin space (1/6-em)]](https://www.rsc.org/images/entities/char_2009.gif) |
Temkin |
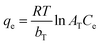 |
|
qm (mg g−1) |
AT (103) |
bT (103) |
R2 |
χ2 |
APE |
303 |
95.33 |
0.107 |
0.221 |
0.9954 |
0.21 |
4.60 × 10−2 |
313 |
96.74 |
0.282 |
0.249 |
0.9964 |
0.36 |
5.90 × 10−2 |
323 |
98.19 |
1.768 |
0.301 |
0.9973 |
0.56 |
7.31 × 10−2 |
![[thin space (1/6-em)]](https://www.rsc.org/images/entities/char_2009.gif) |
Dubinin–Radushkevich |
qe = (qm)exp(−KDRε2), where, 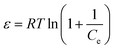 |
|
qm (mg g−1) |
KDR (10−8) |
E (KJ mol−1) |
R2 |
χ2 |
APE |
303 |
74.98 |
3.00 |
4.08 |
0.7907 |
6.24 |
0.25 |
313 |
80.99 |
2.00 |
5.00 |
0.9460 |
4.65 |
0.21 |
323 |
84.99 |
0.90 |
7.45 |
0.9808 |
4.14 |
0.20 |
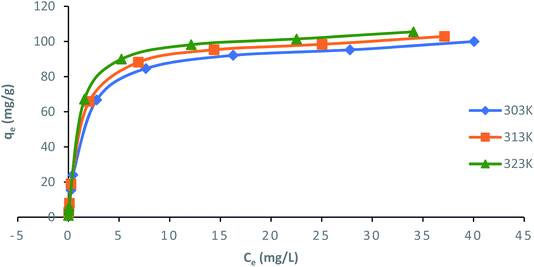 |
| Fig. 6 Nonlinear plots of Langmuir adsorption isotherm model for ampicillin adsorption onto PDA/ZI at different temperatures (pH: 7; contact time: 130 min; adsorbent dose: 1.0 g L−1). | |
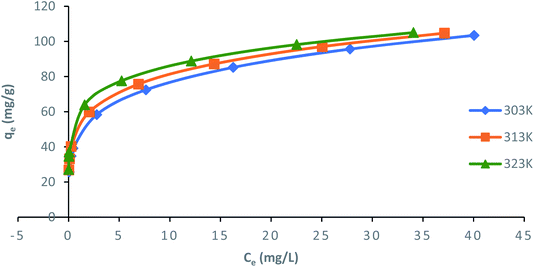 |
| Fig. 7 Nonlinear plots of Freundlich adsorption isotherm model for adsorption of ampicillin onto PDA/ZI (pH: 7; contact time: 130 min; adsorbent dose: 1.0 g L−1) at different temperatures. | |
The value of RL pointed towards the adsorption process as (i) irreversible when RL = 0, (ii) favourable when 0 < RL< 1, (iii) linear when RL = 1 and (iv) unfavourable when RL > 1. Herein, RL values demonstrated that the adsorption of ampicillin onto the surface of PDA/ZI was favourable. Similar observations were also reported for adsorption of chloramphenicol on modified activated carbon.78 Additionally, the value of 1/n computed from the Freundlich isotherm plot was less than 1 at all temperatures studied. This further demonstrated that ampicillin was favourably adsorbed onto PDA/ZI. The value of 1/n obtained from Freundlich isotherm for adsorption of tetracycline onto cellulose nanofibril/graphene oxide was reported to be less than 1 which was similar to this study.79
Temkin isotherm model suggested the effects of some adsorbate/adsorbent interactions. Due to adsorbate/adsorbent interactions the heat of adsorption decreases in a linear fashion with increasing surface coverage.80 Fig. 8 displays the plot of qe versus Ce for Temkin isotherm model and values of AT and bT were obtained by nonlinear regression analysis (Table 3). For examining the nature of adsorption (chemical/physical), D–R isotherm model was adopted to fit the measured data. The plot of qe versus Ce (Fig. S4†) was used to obtain the values of KDR and maximum adsorption capacity (qm) (Table 3). The mean free energy, E, per mole of adsorbate can be computed using eqn (10):81
|
 | (10) |
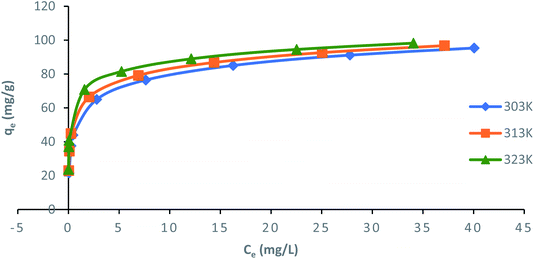 |
| Fig. 8 Non-linear plot of Temkin adsorption isotherm model for adsorption of ampicillin onto PDA/ZI (pH: 7; contact time: 130 min; adsorbent dose: 1.0 g L−1) at different temperatures. | |
The magnitude of E defines the type of adsorption reaction. If the value of E lies between 8–16 kJ mol−1 then the nature of adsorption is considered to be chemisorption type, while the values lower than 8 kJ mol−1 defines a physisorption process.82,83 Here, the values of E were found to be 4.08, 5.00 and 7.45 kJ mol−1 at 303, 313 and 323 K, respectively. This supports the fact that adsorption of ampicillin onto PDA/ZI composite may be favoured by physical forces. However, the R2 values were found to be 0.7907, 0.9460 and 0.9808 at 303, 313 and 323 K, respectively which indicated that the experimental data did not fit well with the D–R model. Therefore, the calculated mean free energy values from D–R model failed to justify the adsorption mechanism. Similar observation is reported in the literature.84
The values of R2 were 0.9965–0.9997, 0.9938–0.9979, 0.9954–0.9973 and 0.7907–0.9808 for Langmuir, Freundlich, Temkin and D–R models, respectively. The value of R2 may not be considered as the sole criterion to reject or accept a model.85,86 Therefore, the applicability of these models in describing the adsorption of ampicillin was further validated by error functions (χ2 and APE). Based on highest R2 and lowest χ2 and APE values, Langmuir isotherm model is most appropriate to describe the uptake of ampicillin onto PDA/ZI (Table 3).
3.6 Adsorption kinetic studies
In order to understand the mechanism of ampicillin uptake onto PDA/ZI composite, three kinetic models have been adopted to analyze the experimental kinetic data.
Surface kinetic models. The nonlinear equations of pseudo-first-order and pseudo-second-order kinetic models are given in Table 4.75 Nonlinear plots of qt (mg g−1) vs. t (min) for pseudo-first-order and pseudo-second-order model at 303, 313 and 323 K are shown in Fig. S5† and 9, respectively.
Table 4 Adsorption kinetic parameters obtained from non-linear fitting for adsorption of ampicillin onto PDA/ZI composite
Kinetic model |
Temp. (K) |
Parameters |
Error function |
The experimental values of adsorption capacity (qe,exp) are 49.56, 49.74, and 49.945 mg g−1 at 303, 313, and 323 K respectively, when C0 = 50 mg L−1. |
Pseudo-first-order |
qt = qe(1 − e−k1t) |
|
qe,cal (mg g−1) |
k1 |
R2 |
χ2 |
APE |
303 |
45.67 |
0.0356 |
0.9609 |
3.05 × 10−1 |
7.84 × 10−2 |
313 |
47.63 |
0.03615 |
0.9616 |
8.95 × 10−2 |
4.24 × 10−2 |
323 |
49.15 |
0.04099 |
0.9764 |
1.26 × 10−2 |
1.59 × 10−2 |
![[thin space (1/6-em)]](https://www.rsc.org/images/entities/char_2009.gif) |
Pseudo-second-order |
 |
|
qe,cala (mg g−1) |
k2 |
R2 |
χ2 |
APE |
303 |
49.70 |
0.0038 |
0.9934 |
3.95 × 10−4 |
−2.82 × 10−3 |
313 |
50.15 |
0.0036 |
0.9993 |
3.37 × 10−3 |
−8.24 × 10−3 |
323 |
50.34 |
0.0035 |
0.9982 |
3.12 × 10−3 |
−7.90 × 10−3 |
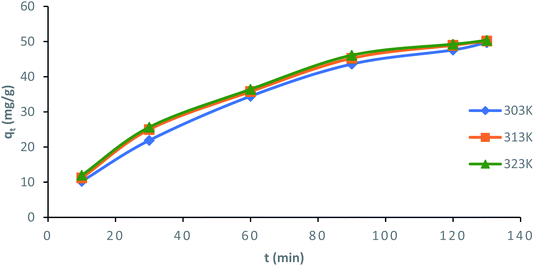 |
| Fig. 9 Nonlinear plot of pseudo-second-order kinetic model for the adsorption of ampicillin onto PDA/ZI composite (contact time: 130 min; adsorbent dose: 1.0 g L−1) at different temperatures. | |
The values of k1 and
for pseudo first order kinetic model were obtained by nonlinear regression analysis using Microsoft Excel SOLVER function-spread sheet method76 and summarized in Table 4. The values of correlation coefficient (R2) vary from 0.9609–0.9764. However, the values of
(simulated) deviated from the corresponding experimental qe values. These results demonstrated the poor fitting of the pseudo-first-order model to the measured data. In a similar fashion, the adsorption of tetracycline on activated carbon did not obey pseudo first-order kinetic model.87
The values of k2 and
for pseudo second order kinetic model were computed by nonlinear regression analysis and are given in Table 4. The values of R2 of the plots were found to be 0.9934, 0.9993, and 0.9982 at 303, 313, and 323 K, respectively, which showed that pseudo-second-order model fitted well with the adsorption data as compared to the pseudo-first-order kinetic model. Moreover, value of
was very close to the measured qe suggesting the best fit to the pseudo-second-order kinetic equation. The lower values of χ2 and APE (%) further confirmed the better fitting of pseudo-second-order kinetics to the measured data (Table 4). The adsorption of norfloxacin on hydrogen titanate nanobelts is controlled by pseudo second-order kinetic model.88 Therefore, the adsorption of ampicillin onto PDA/ZI was favoured by the chemisorption process.
Intra-particle diffusion model. The intra-particle diffusion model suggested that adsorption may occur in two or more steps89 and it can be represented as:where Kid is the intra-particle diffusion rate constant (mg g−1 min−1/2), and Cid (mg g−1) defines the boundary layer thickness. The values of Kid for first and second stages were computed from qt vs. t1/2 plots and are presented in Table 5. The first sharp slope of the line (Fig. 10) is due to the film diffusion of ampicillin from the bulk solution to the adsorbent surface. In the second stage, the low slope of the line was due to a slow diffusion rate of ampicillin into the pores of adsorbent. The linear portions of these curves (Fig. 10) show intercept of greater than zero which demonstrated that both film and intra-particle diffusion are playing significant role in the adsorption of ampicillin. It has been reported that the adsorption of sulfonamide antibiotics on carbon nanotubes was controlled by both surface adsorption (pseudo second order kinetic model) and intraparticle diffusion processes.90 These results support the findings of this study.
Table 5 Kinetic parameters of intraparticle diffusion model for adsorption of ampicillin onto PDA/ZI composite
Kinetic model |
Temp. (K) |
Parameters |
Cid |
Kid |
R2 |
Intraparticle diffusion |
303 |
I |
26.231 |
2.2219 |
0.9960 |
II |
36.267 |
1.1638 |
0.9994 |
313 |
I |
27.056 |
2.202 |
0.9964 |
II |
38.083 |
1.0146 |
0.9893 |
323 |
I |
27.507 |
2.3048 |
0.9856 |
II |
43.443 |
0.5631 |
0.9716 |
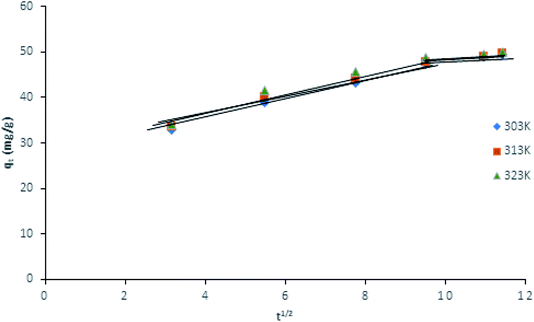 |
| Fig. 10 Intra-particle diffusion model for the adsorption of ampicillin onto PDA/ZI (contact time: 130 min; adsorbent dose: 1.0 g L−1) at different temperatures. | |
3.7 Adsorption thermodynamics
The uptake of ampicillin onto PDA/ZI was examined at 303, 313 and 323 K under the optimized experimental conditions. The thermodynamic parameters such as Gibbs free energy change (ΔG°), enthalpy change (ΔH°) and entropy change (ΔS°) were calculated by using the eqn (12) and (13): |
ΔG° = −RT ln Kc
| (12) |
|
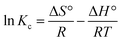 | (13) |
The adsorption equilibrium constant, Kc, was calculated using eqn (13) and following the method reported by Milonjic.91,92
|
 | (14) |
where
qe and
Ce are equilibrium adsorption capacity (mg g
−1) and equilibrium concentration of ampicillin in solution (mg L
−1), respectively.
The linear plot of ln
Kc versus 1/T (Fig. S6†) provided the values of ΔH° and ΔS°. The ΔG° values for the removal of ampicillin were found to be −29.302, −31.647 and −36.841 kJ mol−1 at 303 K, 313 K and 323 K, respectively, which indicated that the adsorption of ampicillin onto PDA/ZI needed low adsorption energy.93 Therefore, the uptake of ampicillin occurred favourably and spontaneously. The adsorption of ampicillin was endothermic in nature since the value of ΔH° (84.436 KJ mol−1) is positive.94,95 The positive value of ΔS° (373.922 J mol−1 K−1) depicts the increased degree of freedom at solid liquid interface (Table 6).
Table 6 Thermodynamic parameters for ampicillin adsorption onto PDA/ZI at different temperatures
Temperature (K) |
ΔG° (KJ mol−1) |
ΔH° (KJ mol−1) |
ΔS° (J mol−1 K−1) |
303 |
−29.302 |
84.436 |
373.922 |
313 |
−31.647 |
323 |
−36.841 |
3.8 Adsorption mechanism
The mechanism of ampicillin adsorption can be explained by taking into consideration the pH of the solution as it plays a significant role in the speciation of ampicillin as well as surface charge of the adsorbent. The pK values corresponding to –COOH and –NH3+ of ampicillin are 2.5 and 7.3, respectively. At pH < 2.5, both the adsorbent as well as ampicillin were positively charged. This electrostatic repulsion limited the adsorption of ampicillin in acidic conditions. At about neutral pH, the surface of the adsorbent was positively charged while negative charge on ampicillin was developed due to deprotonation of –COOH and –NH3+ groups72 and thus, electrostatic interaction occurs between adsorbent and ampicillin. Moreover, Zr(IV) can also interact with –COO− group of ampicillin. Additionally, π–π interaction between benzene ring of ampicillin and polydopamine can be expected (Scheme 3). Thus, these interactions are responsible for enhanced adsorption capacity.
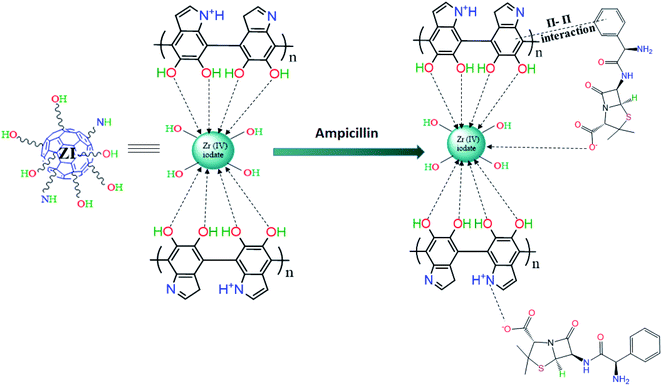 |
| Scheme 3 Mechanism of adsorption of ampicillin on PDA/ZI. | |
3.9 Desorption and reuse
For industrial applications, the regeneration and reuse of adsorbents are the major factors for the adsorption processes. In this study, reuse efficiency of PDA/ZI composite for ampicillin was studied up to eight cycles, the results of which are shown in Fig. 11. The desorption studies of ampicillin were carried out by varying the concentration of NaOH solution (0.1 M to 0.5 M). It was observed that the 0.5 M NaOH removed almost all of the ampicillin from the adsorbent surface (regeneration efficiency 99.12%) and the results reached a nearly constant value up to 5 cycles of adsorption–desorption. This result indicated that the PDA/ZI is a suitable adsorbent for removal of ampicillin.
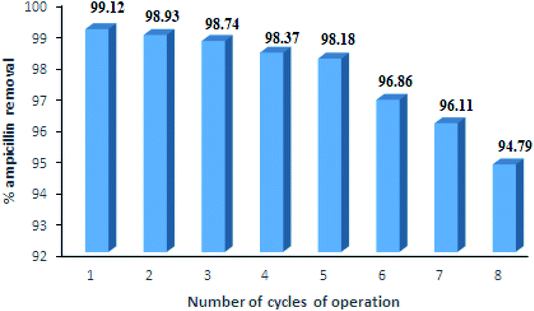 |
| Fig. 11 Reusable efficiency of PDA/ZI composite for ampicillin removal from aqueous solutions. | |
3.10 Comparative study
The maximum adsorption capacities of PDA/ZI and other existing adsorbents for the uptake of ampicillin are presented in Table 7. Two adsorbents namely natural bentonite and organobentonite show adsorption capacity of 50.35 and 86.55 mg g−1, respectively. All other adsorbents, except PDA/ZI, have adsorption capacity lower than 50.0 mg g−1. The material synthesized in this study (PDA/ZI) has higher adsorption capacity as compared to all other adsorbents mentioned in Table 7.
Table 7 Comparison of adsorption capacities of various adsorbents for ampicillin
S. no. |
Adsorbent |
pH |
Contact time |
Max. adsorption capacity (mg g−1) |
Ref. |
1 |
Granular activated carbon |
6 |
120 |
12.70 |
27 |
2 |
Montmorillonite |
7 |
24 h |
27.60 |
31 |
3 |
Myristyltrimethyl ammonium intercalated montmorillonite |
7 |
24 h |
49.96 |
31 |
4 |
Natural bentonite |
7.8 |
35 |
50.35 |
32 |
5 |
Organo bentonite |
7.8 |
35 |
86.55 |
32 |
6 |
Hydroxyapatite@C/Fe3O4 |
6 |
24 h |
3.49 |
96 |
7 |
Activated carbon (prepared from graphene slurry) |
— |
150 |
1.87 |
97 |
8 |
Polydopamine/zirconium(IV) iodate |
7 |
130 |
100.00 |
This study |
4. Conclusion
In this approach, polydopamine/zirconium(IV) iodate composite was prepared by incorporating polydopamine into zirconium(IV) iodate gel for removal of ampicillin. The effects of pH, contact time, adsorbent dose and initial ampicillin concentration, on percentage removal were examined and optimized using Box–Behnken design. The quadratic equation developed for the percent ampicillin removal showed a high correlation between measured and predicted values. The optimized values, at which maximum percent removal (99.12%) was obtained, were pH = 7, contact time = 130 min, adsorbent dose = 20 mg and initial concentration of ampicillin = 50 mg L−1. The isotherm studies pointed towards the best fit of Langmuir isotherm model to the measured data (R2 = 0.9926). The adsorption kinetics revealed that the uptake of ampicillin onto PDA/ZI is best represented by the pseudo-second-order adsorption mechanism. Intra-particle diffusion model was also analyzed and it was concluded that the uptake of ampicillin was generated by film and pore diffusion mechanisms. The feasibility and endothermic nature of adsorption process were confirmed by the thermodynamic parameters.
Conflicts of interest
There are no conflicts to declare.
Acknowledgements
UGC (DRS-II) and DST (FIST-PURSE) are acknowledged for facilities. Poornima Varhsney is thankful to A. M. U., Aligarh for providing fellowship to carry out this work.The reasearch work is fully funded by Aligarh Muslim University, Aligarh, India.
References
- C. Teodosiu, A.-F. Gilca, G. Barjoveanu and S. Fiore, J. Cleaner Prod., 2018, 197, 1210–1221 CrossRef CAS.
- M. Bilal, M. Adeel, T. Rasheed, Y. Zhao and H. M. N. Iqbal, Environ. Int., 2019, 124, 336–353 CrossRef CAS PubMed.
- G. Moussavi, A. Alahabadi, K. Yaghmaeian and M. Eskandaric, Chem. Eng. J., 2013, 217, 119–128 CrossRef CAS.
- Y. Ben, C. Fu, M. Hu, L. Liu, M. H. Wong and C. Zheng, Environ. Res., 2019, 169, 483–493 CrossRef CAS PubMed.
- M. Mezzelani, S. Gorbi and F. Regoli, Mar. Environ. Res., 2018, 140, 41–60 CrossRef CAS.
- J. L. Martinez, Environ. Pollut., 2009, 157(11), 2893–2902 CrossRef CAS.
- P. Acred, D. M. Brown, D. H. Turner and M. J. Wilson, J. Pharmacol., 1962, 18, 356–369 CAS.
- F. G. Rodgers, A. O. Tzianabos and T. S. Elliott, J. Med. Microbiol., 1990, 31(1), 37–44 CrossRef CAS.
- S. Adnan, D. L. Paterson, J. Lipman and J. A. Roberts, Int. J. Antimicrob. Agents, 2013, 42(5), 384–389 CrossRef CAS PubMed.
- B. D. Raynor, Primary Care Update for OB/GYNS, 1997, 4(4), 147–152 CrossRef.
- H. Lode, Int. J. Antimicrob. Agents, 2001, 18(3), 199–209 CrossRef CAS.
- D. D. Glover, D. Lalka and G. R. G. Monif, Infect. Dis. Obstet. Gynecol., 1996, 4, 43–46 CrossRef CAS PubMed.
- M. Kandeel, Int. J. Pharmacol., 2014, 10(6), 340–344 CAS.
- E. A. Serna-Galvis, J. Silva-Agredo, A. L. Giraldo-Aguirre, O. A. Florez-Acosta and R. A. Torres-Palma, Ultrason. Sonochem., 2016, 31, 276–283 CrossRef CAS PubMed.
- L. Chu, S. Zhuang and J. Wang, Radiat. Phys. Chem., 2018, 145, 34–38 CrossRef CAS.
- S. Norzaee, M. Taghavi, B. Djahed and F. K. Mostafapour, J. Environ. Manage., 2018, 215, 316–323 CrossRef CAS.
- M. D. H. Wirzal, A. R. M. Yusoff, J. Zima and J. Barek, Int. J. Electrochem. Sci., 2013, 8, 8978–8988 CAS.
- M. Gholami, R. Mirzaei, R. R. Kalantary, A. Sabzali and F. Gatei, Iran. J. Environ. Health Sci. Eng., 2012, 9(1), 19 CrossRef PubMed.
- K. J. Choi, S. G. Kim and S. H. Kim, J. Hazard. Mater., 2008, 151(1), 38–43 CrossRef CAS PubMed.
- I. Koyuncu, O. A. Arikan, M. R. Wiesner and C. Rice, J. Membr. Sci., 2008, 309, 94–101 CrossRef CAS.
- S. Babic, M. Perisa and I. Skoric, Chemosphere, 2013, 9(11), 1635–1642 CrossRef PubMed.
- M. B. Ahmed, J. L. Zhou, H. H. Ngo and W. Guo, Sci. Total Environ., 2015, 532, 112–126 CrossRef CAS PubMed.
- P. Gharbani, A. Mehrizad and I. Jafarpour, Pol. J. Chem. Technol., 2015, 17(3), 95–99 CAS.
- D. Balarak, A. Joghatayi, F. K. Mostafapour and H. Azarpira, Int. J. Life Sci. Pharma Res., 2017, 7(1), 9–16 Search PubMed.
- M. P. Oliva, C. Corrah, M. Jesoro and J. R. Barajas, MATEC Web Conf., 2019, 268, 01005 CrossRef CAS.
- Z. Aksu and O. T. Dede, Process Biochem., 2005, 40(2), 831–847 CrossRef CAS.
- P. D. Vecchio, N. K. Haro, F. S. Souza, N. R. Marcilio and L. A. Feris, Water Sci. Technol., 2019, 79, 2013–2021 CrossRef PubMed.
- Y. Wu, W. Liu, Y. Wang, X. Hu, Z. He, X. Chen and Y. Zhao, Int. J. Environ. Res. Public Health, 2018, 15, 2652 CrossRef CAS.
- A. C. Queiroz, J. D. Santos and F. J. Monteiro, Key Eng. Mater., 2005, 284–286, 387–390 CAS.
- X. Weng, W. Cai, R. Lan, Q. Sun and Z. Chen, Environ. Pollut., 2018, 236, 562–569 CrossRef CAS PubMed.
- M. Anggraini, A. Kurniawan, L. K. Ong, M. A. Martin, J.-C. Liu, F. E. Soetaredjo, N. Indraswati and S. Ismadji, RSC Adv., 2014, 4, 16298–16311 RSC.
- A. K. Rahardjo, M. J. J. Susanto, A. Kurniawan, N. Indraswati and S. Ismadji, J. Hazard. Mater., 2011, 190, 1001–1008 CrossRef CAS PubMed.
- S. Chavoshan, M. Khodadadi, N. Nasseh, A. H. Panahi and A. Hosseinnejad, Environ. Health Eng. Manage. J., 2018, 5(4), 187–196 CrossRef CAS.
- B. Priya, P. Raizada, N. Singh, P. Thakur and P. Singh, J. Colloid Interface Sci., 2016, 479, 271–283 CrossRef CAS PubMed.
- V. Nairi, L. Medda, M. Monduzzi and A. Salis, J. Colloid Interface Sci., 2017, 497, 217–225 CrossRef CAS PubMed.
- N. Rahman and M. F. Khan, Journal of Water Process Engineering, 2016, 9, 254–266 CrossRef.
- N. Rahman and M. F. Khan, J. Ind. Eng. Chem., 2015, 25, 272–279 CrossRef CAS.
- N. Rahman, U. Haseen and M. F. Khan, RSC Adv., 2015, 5, 39062–39074 RSC.
- Lutfullah, M. Rashid, U. Haseen and N. Rahman, J. Ind. Eng. Chem., 2014, 20, 809–817 CrossRef CAS.
- W. Zheng, H. Fan, L. Wang and Z. Jin, Langmuir, 2015, 31(42), 11671–11677 CrossRef CAS PubMed.
- J. Liebscher, R. Mrowczynski, H. A. Scheidt, C. Filip, N. D. Hadade, R. Turcu, A. Bende and S. Beck, Langmuir, 2013, 29, 10539–10548 CrossRef CAS PubMed.
- A. Pramanik, K. Gates, Y. Gao, Q. Zhang, F. X. Han, S. Begum, C. Rightsell, D. Sardar and P. C. Ray, ACS Appl. Nano Mater., 2019, 2, 3339–3347 CrossRef CAS.
- H. Gao, Y. Sun, J. Zhou, R. Xu and H. Duan, ACS Appl. Mater. Interfaces, 2019, 5, 425–432 CrossRef PubMed.
- J. An, W. Liang, P. Mu, C. Wang, T. Chen, Z. Zhu, H. Sun and A. Li, ACS Omega, 2019, 4, 4839–4847 CrossRef PubMed.
- Q. Zeng, X. Qi, M. Zhang, X. Tong, N. Jiang, W. Pan, W. Xiong, Y. Li, J. Xu, J. Shen and L. Xu, Int. J. Biol. Macromol., 2020, 145, 1049–1058 CrossRef CAS PubMed.
- T. Li, X. Liu, L. Li, Y. Wang, P. Ma, M. Chen and W. Dong, J. Polym. Res., 2019, 26, 281 CrossRef CAS.
- Z. Zhao, J. Li, T. Wen, C. Shen and X. Wang, Colloids Surf., A, 2015, 482, 258–266 CrossRef CAS.
- J. Wang, T. Huang, L. Zhang, Q. J. Yu and L. Hou, Environ. Technol., 2018, 39, 3055–3065 CrossRef CAS PubMed.
- C. Wang, J. Yin, R. Wang, T. Jiao, H. Huang, J. Zhou, L. Zhang and Q. Peng, Nanomaterials, 2019, 9, 116 CrossRef PubMed.
- N. Rahman, S. Sameen and M. Kashif, J. Mol. Liq., 2016, 222, 944–952 CrossRef CAS.
- Lutfullah, S. Sharma, N. Rahman and S. N. H. Azmi, Arabian J. Chem., 2016, 9, 51163–51169 Search PubMed.
- N. Rahman and U. Haseen, RSC Adv., 2015, 5, 7311–7323 RSC.
- N. Rahman and M. Nasir, J. Mol. Liq., 2020, 301, 112454, DOI:10.1016/j.molliq.2020.112454.
- N. Rahman, M. F. Khan and M. Nasir, Environmental Technology & Innovation, 2020, 17, 100634, DOI:10.1016/j.eti.2020.100634.
- N. Rahman and M. Nasir, ACS Omega, 2019, 4, 2823–2832 CrossRef CAS PubMed.
- N. Rahman and M. Nasir, Environ. Sci. Pollut. Res., 2018, 25, 26114–26134 CrossRef CAS PubMed.
- W. Zheng, H. Fan, L. Wang and Z. Jin, Langmuir, 2015, 31, 11671–11677 CrossRef CAS PubMed.
- S. A. Nabi, A. Islam and N. Rahman, Adsorpt. Sci. Technol., 1999, 17, 629–637 CrossRef CAS.
- I. Pasha S, F. Rehman, T. Mohammed, Y. Sanjyothi and S. Kumar, Int. J. Pharm. Res., 2012, 2, 47–50 Search PubMed.
- L. V. Candioti, M. M. De Zan, M. S. Camara and H. C. Goicoehea, Talanta, 2014, 124, 123–138 CrossRef PubMed.
- J.-H. Lin, C.-J. Yu, Y.-C. Yang and W.-L. Tseng, Phys. Chem. Chem. Phys., 2015, 17, 15124–15130 RSC.
- R. A. Zangmeister, T. A. Morris and M. J. Tarlov, Langmuir, 2013, 29, 8619–8628 CrossRef CAS PubMed.
- G. Socrates, Infrared characteristic group frequencies, John Wiley & Sons, New York, 1980, pp. 55–87 Search PubMed.
- P. Singh, J. P. Rawat and N. Rahman, Talanta, 2003, 59, 443–452 CrossRef CAS PubMed.
- M. Serdych and T. J. Bandsz, J. Phys. Chem. C, 2010, 114, 14552–14560 CrossRef.
- N. Rahman, U. Haseen and M. Rashid, Arabian J. Chem., 2017, 10, S1765–S1773 CrossRef CAS.
- V. K. Gupta, P. Singh and N. Rahman, Anal. Bioanal. Chem., 2005, 381, 471–476 CrossRef CAS PubMed.
- A. C. Bean, S. M. Pepper and T. E. Albrecht-Schmitt, Chem. Mater., 2001, 13, 1266–1272 CrossRef CAS.
- N. Rahman and M. Nasir, Journal of Water Process Engineering, 2017, 19, 172–184 CrossRef.
- A. P. Tiwari, D. P. Bhattarai, B. Maharjan, S. W. Ko, H. K. Kim, C. H. Park and C. S. Kim, Sci. Rep., 2019, 9, 2943 CrossRef.
- H. Luo, C. Gu, W. Zheng, F. Dai, X. Wang and Z. Zheng, RSC Adv., 2015, 5, 13470–13477 RSC.
- M. J. Zaworotko, H. H. Hammud, I. Abbas, V. C. Kravtsov and M. S. Masoud, J. Coord. Chem., 2006, 59, 65–84 CrossRef CAS.
- G. Wang, T. Wu, Y. Li, D. Sun, Y. Wang, X. Huang, G. Zhang and R. Liu, J. Chem. Technol. Biotechnol., 2012, 87, 623–628 CrossRef CAS.
- Z. W. Zeng, X. F. Tan, Y. G. Liu, S. R. Tian, G. M. Zeng, L. H. Jiang, S. B. Liu, J. Li, N. Liu and Z. H. Yin, Front. Chem., 2018, 6, 1–11 CrossRef PubMed.
- B. Nagy, C. Manzatu, A. Maicaneanu, C. Indolean, B. T. Lucian and C. Majdik, Arabian J. Chem., 2017, 10, S3569–S3579 CrossRef CAS.
- M. A. Hossain, H. H. Ngo and W. Guo, J. Water Sustainability, 2013, 4, 223–237 Search PubMed.
- M. Belhachemi and F. Addoun, Appl. Water Sci., 2011, 1, 11–117 CrossRef.
- J. Lach, Water, 2019, 11, 1141 CrossRef CAS.
- Q. Yao, B. Fan, Y. Xiong, C. Jin, Q. Sun and C. Sheng, Sci. Rep., 2017, 7, 45914 CrossRef CAS.
- H. I. Melendez-Ortiz, B. Puente-Urbina, J. A. Mercado-Silva and L. Garcia-Uriostegui, Int. J. Appl. Ceram. Technol., 2019, 16, 1533–1543 CrossRef CAS.
- A. Pandiarajan, R. Kamaraj and S. Vasudevan, New J. Chem., 2017, 41, 4518–4530 RSC.
- C. A. P. Almeida, N. A. Debacher, A. J. Downs, L. Cottet and C. A. D. Mello, J. Colloid Interface Sci., 2009, 332, 46–53 CrossRef CAS.
- M. T. Amin, A. A. Alazba and M. Shafiq, Sustainability, 2015, 7, 15302–15318 CrossRef CAS.
- N. Rahmam and U. Haseen, Ind. Eng. Chem. Res., 2014, 53, 8198–8207 CrossRef.
- M. Horsfall Jr and J. L. Vicente, Bull. Chem. Soc. Ethiop., 2007, 21, 349–362 Search PubMed.
- S. Shahmohammadi-Kalalsgh, H. Babazadeh, A. H. Nazemi and M. Manshouri, Caspian Journal of Environmental Sciences, 2011, 9, 243–255 Search PubMed.
- H. Saygili and F. Guzel, Ecotoxicol. Environ. Saf., 2016, 131, 22–29 CrossRef CAS PubMed.
- W. Li, J. Wang, G. He, L. Yu, N. Noor, Y. Sun, X. Zhou, J. Hu and I. P. Parkin, J. Mater. Chem. A, 2017, 5, 4352–4358 RSC.
- W. J. Weber Jr, J. C. Morris and J. Sanit, J. Sanit. Eng. Div., Am. Soc. Civ. Eng., 1963, 89, 31–38 Search PubMed.
- Y. Tan, B. Gao, H. Chen, Y. Wang and H. Li, J. Environ. Sci. Health, Part A: Toxic/Hazard. Subst. Environ. Eng., 2013, 48, 1136–1144 CrossRef PubMed.
- P. Zain Al-Salehin, F. Moeinpour and F. S. Mohseni-Shahri, Appl. Water Sci., 2019, 9, 172 CrossRef.
- S. K. Milonjic, J. Serb. Chem. Soc., 2007, 72, 1363–1367 CrossRef CAS.
- D. Balarak, F. K. Mostafapour, H. Azarpira and A. Joghataei, J. Pharm. Res. Int., 2017, 19, 1–9 CrossRef.
- Z. Li, M. Qi, C. Tu, W. Wang, J. Chen and A. J. Wang, Appl. Surf. Sci., 2017, 425, 765–775 CrossRef CAS.
- Y. Zheng, H. Ou, H. Liu, Y. Ke, W. Zhang, G. Liao and D. Wang, Colloids Surf., A, 2018, 537, 92–101 CrossRef.
- H. Yang, Q. Liu, S. Masse, H. Zhang, L. Li and T. Coradin, Chem. Eng. J., 2015, 275, 152–159 CrossRef CAS.
- R. Chitongo, B. O. Opeolu and O. S. Olatunji, Clean: Soil, Air, Water, 2019, 47, 1800077, DOI:10.1002/clen.201800077.
Footnote |
† Electronic supplementary information (ESI) available. See DOI: 10.1039/d0ra02061c |
|
This journal is © The Royal Society of Chemistry 2020 |
Click here to see how this site uses Cookies. View our privacy policy here.