DOI:
10.1039/D0RA01397H
(Paper)
RSC Adv., 2020,
10, 15514-15522
Insights into the competitive adsorption of pollutants on a mesoporous alumina–silica nano-sorbent synthesized from coal fly ash and a waste aluminium foil†
Received
13th February 2020
, Accepted 26th March 2020
First published on 20th April 2020
Abstract
A highly efficient and low-cost alumina–silica nano-sorbent was fabricated and characterized to understand the key factors responsible for its superiority over the existing adsorbents in treating the industry-discharged wastewater for the removal of dyes and heavy metals. As compared to the properties of raw fly ash, the following fundamental improvements were observed for the alumina–silica nano-sorbent: (a) transformation of throttled mesopores into slit-type pores, (b) increment in the surface area by 65-fold, (c) change in the morphology from spherical particles to a flake-type structure with sharp edges, (d) reduction in the average crystal size from 61.143 to 27.176 nm, and (e) increase in the pore volume from 0.005 to 0.50 cm3 g−1. These desired properties of the nano-sorbent were obtained by blending a waste aluminium foil with fly ash. This process increased the ratio of alumina to silica from 0.59
:
1 to an optimum ratio of 1.9
:
1, beyond which the particles agglomerated and the pore volume reduced. Eventually, the precipitated hydroxides were calcined at 700 °C that favoured the formation of γ-alumina. Moreover, this heat treatment changed its crystallinity and morphology of γ-alumina, which abruptly enhanced its activity towards the pollutants. The obtained product (nano-sorbent) was tested for the removal of lead and malachite green from a model wastewater solution over a wide range of initial pollutant concentrations and adsorbent dosages. After observing almost complete removal capacity and reusability for the pollutants, we propose this synthesized adsorbent as a universal material for treating industrial wastewater.
1. Introduction
Water pollution originating from several anthropogenic industrial sources is a global concern. The toxic and harmful pollutants such as dyes and heavy metals are the major components in the discharge of dye, leather, textile, paper, food and electroplating processing industries.1 Usually, dyes are difficult to decompose and their presence reduces the penetration of sunlight in water, which affects the intensity of photosynthesis in aquatic plants and subsequently damages the aquatic ecosystems. Moreover, dyes and heavy metals there are proven to have strong carcinogenic, mutagenic and toxic effects towards aquatic organisms, human beings and ecological environments.2,3 In this light, lead (Pb(II)) is an extremely toxic heavy metal, which causes encephalopathy, anaemia and liver damage, and affects the central nervous system.4–6 On the other hand, malachite green (MG) is a synthetic dye of basic nature, which is extensively used in the textile industry and in curing bacterial or fungal infections and parasites in fish. Malachite green has the potential to cause cancer, respiratory diseases and teratogenicity.7,8 Typically, the textile industries dispose a sizable amount of Pb(II) and malachite green through their effluents.9 Therefore, the development of an effective method to eliminate malachite green and Pb(II) from industrial effluents is a crucial task for preserving the environment. The present work is carried out with this intention.
There are several methods available to eliminate the pollutants from wastewater; however, most of these methods involve high installation and operating costs, complicated operations, partial separations and the production of huge sludge. In addition, the proper separation of pollutants from a multicomponent mixture is an arduous and expensive task using traditional techniques. Howbeit, adsorption is attested as an economical and robust technique with a modest operational procedure, which is effectually applicable for a wide variety of pollutants.10,11 The industrial wastewater naturally comprises multicomponent pollutants with a wide range of concentration.
Till date, research on adsorption processes is more concentrated on the mono-component system that either contains heavy metals or dyes.12,13 The application of adsorption to deal with a multicomponent mixture of pollutants is still at an preliminary level due to the limited research emphasis on the same. Therefore, there is an urgent need to understand and evaluate the nature of the multicomponent adsorption in the view of formulating an industry scale unit to treat the wastewater.
In search of efficient and low-cost adsorbents, bio-adsorbents from agricultural wastes (such as lotus seedpod,14 orange peel,15 barley straw,16 leaf powder17 and other agricultural waste-based adsorbents18) have already shown an appreciable efficiency in removing dyes and heavy metals, but they have the drawbacks of enhancing chemical oxygen demand (COD), biological oxygen demand (BOD) and total organic carbon (TOC) in water.19 Concurrently, activated carbon offers huge production and reactivation cost.17,20 The inorganic adsorbents from industrial solid wastes have claimed huge research attention due to their economic-nature and successful applications. Steel slag was used efficiently for the removal of Cd and Mn ions from industrial wastewater.21 Fertilizers and steel plant wastes were successfully used for removing dyes,22 Pb(II)23 and bromophenols24 by adsorption. Red mud was used to prepare porous adsorbents for the adsorption phosphorus and diclofenac.25 Besides, the growth and development of an industrial sector is greatly dependent on the extent of power generation and consumption. Majority of the industries rely on the thermal power plants for the generation of energy. However, these power plants mostly utilize relatively inefficient carbon-based fuels, such as coal, which are further responsible for emitting an enormous amount of fly ash in the environment. The fly ash creates significant environmental risk and serious disposal problems. Moreover, the inappropriate disposal of fly ash causes soil degradation and groundwater contamination. The fly ash particles are small enough to cause severe air pollution that leads to eye irritation, skin and respiratory diseases.26 Therefore, the recycling of coal fly ash is a vital aspect for safe and efficient solid waste management. Simultaneously, this offers significant economic and environmental benefits. Fly ash is used in concrete production, ceramic industry,27 soil amelioration,28 valuable metal recovery,29 zeolite syntheses,30 catalyst supports,31 and adsorbents for water treatment.32 Moreover, fly ash is a potential adsorbent for dyes and heavy metals because it is composed of significant amounts of alumina and silica.33
Therefore, considering the aforementioned couple of issues, an attempt is made to fabricate an adsorbent from coal fly ash that can purify wastewater from the industry. Through this approach, we are utilizing the waste (i.e., coal fly ash) to treat another waste (i.e., wastewater containing dyes and heavy metals). Till date, the adsorption capacity of plant fly ash is increased either by a base–acid treatment or through the impregnation method.32 The present research proposes a novel approach to synthesize mesoporous alumina–silica nano-sorbents from the precipitates of the alkali extraction from a mixture of coal fly ash and a waste aluminium foil. A comprehensive characterization of the as-prepared material is performed to examine their crystallinity, morphology and porosity. The as-synthesised nano-sorbent is employed to remove a multicomponent mixture of Pb(II) and malachite green from wastewater. Here, the effective parameters of adsorption are optimized through response surface methodology (RSM) with central composite design (CCD). The experimental results have proved that the adsorption process involves monolayer formation and is endothermic in nature, which is controlled by both physical and chemisorption phenomena. Besides, the nano-sorbent has a pretty high adsorption capacity for both malachite green (1655.2 mg g−1) and lead (326.2 mg g−1), with reasonable reusability. Therefore, the present experimental investigation along with modelling can provide important contributions towards the effluent treatment at an industrial scale.
2. Experimental
2.1 Materials
The raw coal fly ash was collected from Durgapur Project Limited, India. The coal fly ash has an alumina to silica ratio of 0.517
:
1. The waste aluminium foil was collected from the local market. The detailed specifications of all chemicals used in the experiments are presented in Table S1.†
2.2 Synthesis of adsorbents
The raw coal fly ash was first washed with Millipore water and dried at 403 K for 8 h. The dried fly ash was then mixed with the desired quantity of the scrap aluminium foil and soaked in a 2 M NaOH solution at ambient condition for 24 h. This process was repeated three times. Subsequently, the liquid was separated via vacuum filtration. A 1 N H2SO4 solution was added to the filtrate to get a white precipitate of silicon hydroxide and aluminium hydroxide. The slurry was washed with double distilled water. Afterwards, the material was calcined at 973 K for 4 h to obtain the alumina–silica nano-sorbents. The alumina to silica ratio in the as-prepared adsorbents was varied by changing the weight ratio of fly ash and aluminium foil.34 Different adsorbents were designated as SiA-1, SiA-2, SiA-3 and SiA-4, according to their alumina to silica weight ratios as 0.56
:
1, 1.9
:
1, 3.81
:
1 and 6.03
:
1, respectively.
2.3 Characterization
The details of the adsorbent characterization are documented in the ESI.†
2.4 Adsorption experiment
2.4.1 Adsorption isotherm and kinetics. The adsorption isotherms were studied at three different temperatures (283 K, 303 K and 313 K) and the initial concentration for the single component was varied from 10 to 500 mg L−1 for binary components, and from 10 to 350 mg L−1 for Pb(II) and MG. The adsorption kinetics for Pb(II) and MG solutions was also studied at different initial concentrations of single and binary component solutions.
2.4.2 Experimental design. The adsorption uptake was optimized via central composite design (CCD) by varying the parameters such as pH (2 to 8), temperature (283 to 313 K), initial concentration of adsorbate (10 to 100 mg L−1), and adsorbent dosage (0.25 to 2.5 g L−1). The Design Expert software version 11 was used for the optimization of the process by statistical analysis. A second-degree polynomial was fitted to correlate the independent parameters to the response as: |
 | (1) |
where Y represents the responses (adsorption uptake); b0, bi, bij and bii are the coefficients for constant, linear, interaction and the quadratic contributions, respectively, in eqn (1). The terms xi, xj are the coded values of the independent variables and ε is the error between predicted and experimental values. By simultaneously varying multiple factors, it is possible to locate the region of optimum responses. The values of the coefficients of the model for different responses were evaluated by utilizing the multiple regression analysis technique.35–38
3. Results and discussion
3.1 Selection of adsorbent
The best adsorbent was selected by comparing the adsorption capacities of raw fly ash, SiA-1, SiA-2, SiA-3 and SiA-4. It is observed that the adsorbent SiA-2 with an alumina to silica weight ratio of 1.9
:
1 shows the maximum removal capacity for both Pb(II) and MG (Fig. 1). Beyond this alumina–silica weight ratio, particle agglomeration reduces the pore volume, which leads to a decrease in the adsorption capacity. Thus, the successive experiments were performed using SiA-2. The effects of pH and adsorbent dosage are shown in Fig. S7 and S8,† respectively.
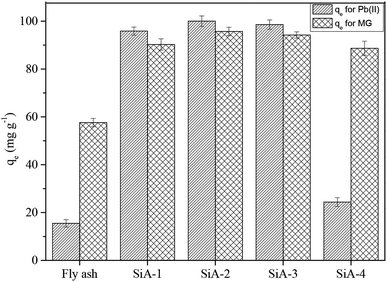 |
| Fig. 1 Comparison of the adsorption capacity of different adsorbents for Pb(II) and MG (initial concentration of Pb(II) and MG: 100 mg L−1 each; adsorbent dosage: 1 g L−1; pH: 6, temperature: 303 K). | |
3.2 Adsorption equilibrium isotherm
The equilibrium adsorption isotherms for MG and Pb(II) in single and binary solutions are shown in Fig. 2(a–d). The higher initial concentration facilitates the driving force to overcome the resistance between liquid and solid phases by increasing the collision between the adsorbate molecules and the adsorbent.39,40 The equilibrium adsorption isotherms obtained for single and binary component solutions for three different temperatures show that the adsorption capacity of SiA-2 increases at higher temperatures.
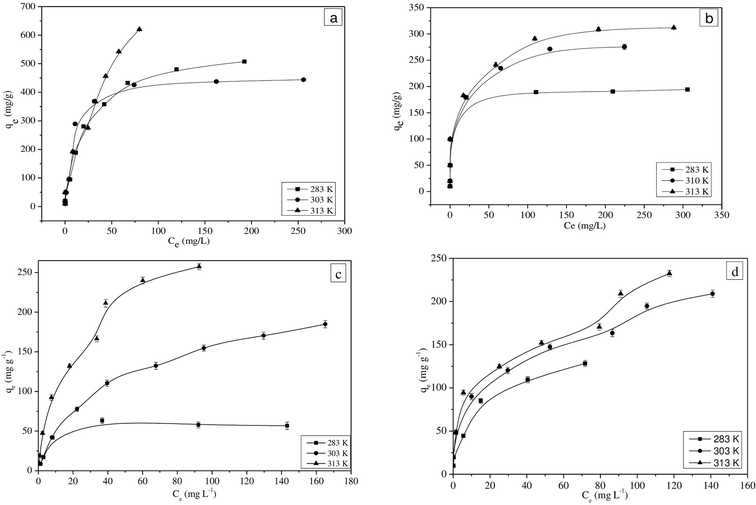 |
| Fig. 2 Adsorption isotherms of (a) MG in single, (b) Pb(II) in single, (c) MG in binary and (d) Pb(II) in binary component solutions (pH: 6; adsorption dosage: 1 g L−1). | |
The Langmuir, Freundlich and Temkin adsorption models41,42 were utilized to fit the adsorption data for a single component. The extended Langmuir isotherm was used for binary component system.43
It is observed that the Langmuir isotherm fitted well with a good correlation coefficient for the single-component adsorption. However, the extended Langmuir isotherm has a good correlation coefficient when it is fitted with binary component adsorption data. The results are shown in Tables S3 and S4† for single and binary component systems, respectively.
In a single component, the MG adsorption shows maximum adsorption capacities of 478.9, 584.3 and 1655.2 mg g−1 at 283, 303 and 313 K, respectively. However, the maximum adsorption capacities for MG in the binary mixture are found to be as 103.7, 391 and 445 mg g−1 at 283, 303 and 313 K, respectively. These experimental results prove that the MG adsorption capacity of SiA-2 is significantly lower for the binary as compared to the single component. However, the Pb(II) adsorption capacity of SiA-2 was significantly higher in the case of binary as compared to that in the single-component solution. In a single component, the adsorption capacities for Pb(II) are found to be 193.6, 291.5 and 326.2 mg g−1 at 283, 303 and 313 K, respectively, and in the case of binary adsorption, these capacities are 231.27, 521.87 and 615.86 mg g−1 at 283, 303 and 313 K, respectively. It is observed that Pb(II) adsorption considerably increases for binary adsorption. This phenomenon confirms the synergetic effect of the presence of MG on the adsorption of Pb(II), while there is an antagonistic effect of the presence of Pb(II) on MG adsorption. The possible reasons behind this observation may be the larger size of MG molecules that causes difficulty in its adsorbtion into the pores. The adsorption process is largely influenced by the charge density of cations. Therefore, Pb(II) with a higher charge density show a higher rate of ion exchange.44,45
3.3 Adsorption kinetics
The adsorption uptake as a function of time for MG and Pb(II) in single and binary components is shown in Fig. 3a–d. The experimental data shows a good agreement with pseudo-first-order, pseudo-second-order and Elovich adsorption kinetic models.41 The correlation coefficients and other adsorption kinetic parameters are presented in Tables S5–S7.†
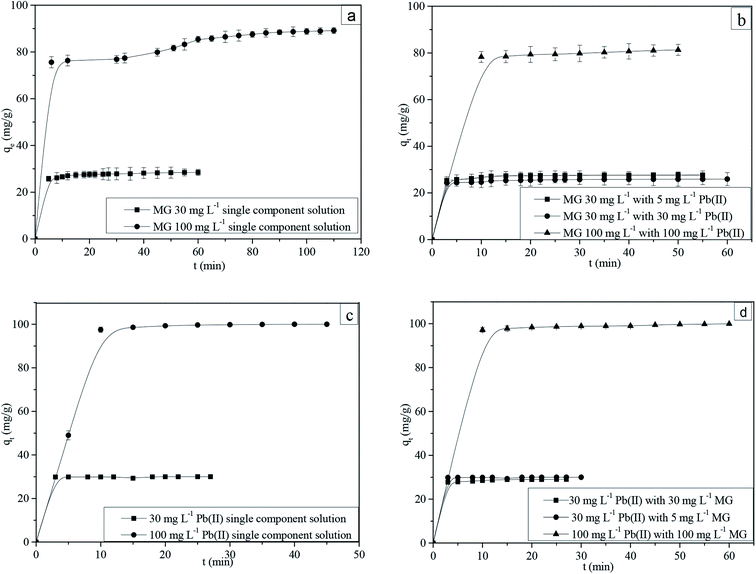 |
| Fig. 3 Adsorption kinetics of (a) MG in single, (b) MG in binary, (c) Pb(II) in single and (d) Pb(II) in binary component solutions (pH: 6; adsorption dosage 1 g L−1; temperature: 303 K). | |
It is observed that, for an intra-particle diffusion model, the qe vs. t1/2 has multiple linearities (Fig. S9a–d†). At the initial step, the linear plot represents the external film diffusion; the second step represents pore diffusion from the adsorbent surface to the interior of the pores, and the third step reveals adsorption on the active sites.46
3.4 Molecular insight to adsorption mechanism
Fig. 4a and b show the optimized structure of SiA-2 and MG, respectively, via universal force field (UFF). The desorption process was initiated by the Na+ ion-exchange method. Pb(II) is attached with SiA-2 at a minimum distance of 3.69 Å and the contact angle was 74.5°, as shown in Fig. 4c. However, MG is attached to SiA-2 having a minimum bond length of 3.571 Å and the contact angle is 84.3°, as shown in Fig. 4d. The high adsorption energy for Pb(II) (306.35 kJ mol−1) and MG (586.228 kJ mol−1) indicates chemisorption.47–49 The higher adsorption energy for MG indicates a higher capacity for MG in single-component adsorption. In the case of the binary adsorption of Pb(II) and MG on SiA-2 (Fig. 4e), the adsorption energy was 791.8 kJ mol−1.
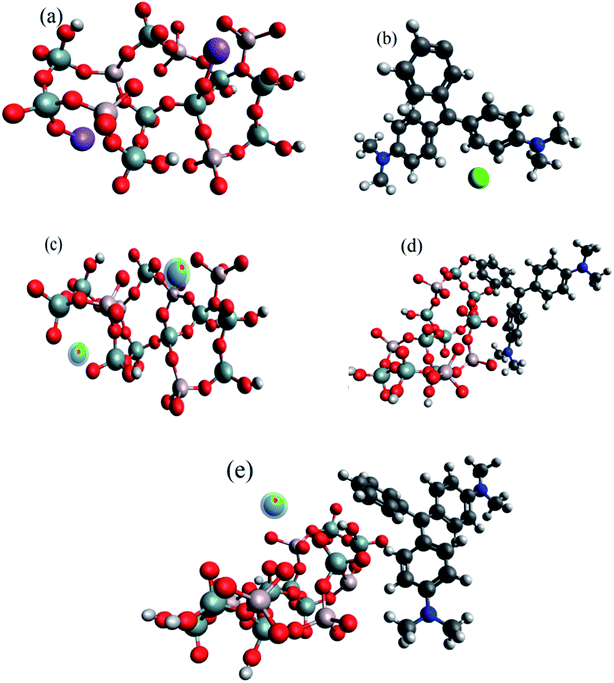 |
| Fig. 4 Molecular structure of (a) SiA-2, (b) malachite green, molecular insight into the adsorption mechanism for (c) Pb(II) adsorption on SiA-2, (d) MG adsorption on SiA-2 and (e) binary adsorption of Pb(II) and MG on SiA-2. The red, grey, pink, light grey, dark grey, blue, green, purple and blue with red dot spheres represent oxygen, silicon, aluminium, hydrogen, carbon, nitrogen, chlorine, sodium and lead, respectively. | |
3.5 Design of experiment
The CCD consists of 2n factorial runs with 2n axial runs and nc centre (8 replicates) runs. The complete range of experiments and independent variables are presented in Table S8.† The total number of experimental runs are expressed as N and the number of factors as n.36,50 The total number of experiments are represented by eqn (2): |
N = 2n + 2 × n + nc = 25 + 2 × 5 + 8 = 50
| (2) |
Consequently, 50 sets of experimental runs were performed. The results are tabulated in Table S9.† The independent variables are coded with (−1, 1), where −1 shows the low level and 1 shows the high level. Both the responses, i.e. the adsorption uptake of Pb(II) and MG are recorded for all preformed experimental runs. The ANOVA from the statistical analysis for the Pb(II) and MG uptakes are given in Tables S10 and S11,† respectively.
3.5.1 Regression models. The adsorption uptake of Pb(II) (Y1) and MG (Y2) can be represented by the second-degree polynomials as follows: |
Y1 = 38.68 − 21.03A + 12.73B − 1.77C + 7.65D + 2.53E − 3.44AB − 2.31AC + 4.99AD + 2.15AE − 1.91BC + 3.64BD + 2.27BE − 0.6743CD − 2.06CE + 0.2357DE + 8.25A2 + 2.00B2 − 0.9295C2 − 4.77D2 − 1.57E2
| (3) |
|
Y2 = 34.47 − 7.00A − 4.66B + 13.74C + 2.32D + 0.6621E + 7.38AB − 2.06AC − 3.39AD + 0.5244AE − 4.40BC + 1.77BD − 2.33BE + 0.1300CD + 0.5258CE − 1.27DE
| (4) |
where the adsorption parameters: adsorbent dosage, initial Pb(II) concentration, initial MG concentration, pH, and temperature are represented by A, B, C, D and E, respectively.The analysis of variance (ANOVA) for the model analysis of Pb(II) and MG are described in Tables S10 and S11,† respectively. A Quadratic model for the Pb(II) uptake and a two-factor interaction (2FI) model for the MG uptake are suggested. The positive term in the model signifies synergistic effects, while the negative sign indicates antagonistic effects on the adsorption process. The R2 values for eqn (3) and (4) are 0.930 and 0.923, respectively. It shows a reasonably good agreement between the predicted and experimental values from the model. The difference between adjusted and predicted R2 values is less than 0.2 for both the responses, which indicates that both the equations are appropriate. Adeq precision measures the signal to noise ratio as 21.80 for the Pb(II) uptake and 22.89 for the MG uptake. This implies sufficient adequate signals. The P value of less than 0.05 indicates that the model terms are significant and the P value greater than 0.10 indicates that model terms are not significant. The F values for the developed model for the Pb(II) and MG uptakes are 18.68 and 27.20, respectively, and the P values for both of the cases are less than 0.05, which indicates that the model terms are significant. Table S10† confirms that the significant terms of the model for the Pb(II) uptake (Y1) are A, B, D, AD, BD, A2, D2. Simultaneously, Table S11† indicates that for the MG uptake (Y2) the significant model terms are A, B, C, D, AB, AD, BC, BE.
The plots of experimental versus predicted values for the adsorption uptake of Pb(II) and MG are shown in Fig. S10a and b,† respectively. It is observed that the predicted values are close enough to the experimental values, which confirm the satisfactory correlation between the adsorption operating parameters and the adsorption uptake of Pb(II) and MG.50,51
3.5.2 Interaction effects of different parameters on the Pb(II) and MG uptake. The variation of response with two selected parameters is represented by response surfaces 3D plots in Fig. 5a, b and 6a–d, for Pb(II) and MG, respectively. The maximum predicted response lies in the surface-confined in the smallest curve of the 3D contour diagram.
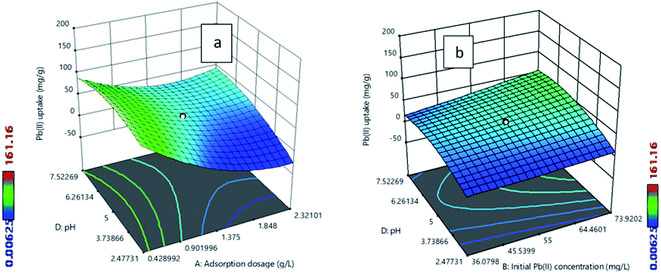 |
| Fig. 5 3D response plots for interaction effect of (a) the adsorbent dosage–pH (initial Pb(II) concentration: 55 mg L−1; initial MG concentration: 55 mg L−1; temperature: 298 K) and (b) initial Pb(II) concentration–pH (adsorbent dosage: 1.375 g L−1; initial MG concentration 55 mg L−1; temperature: 298 K) on Pb(II) uptake. | |
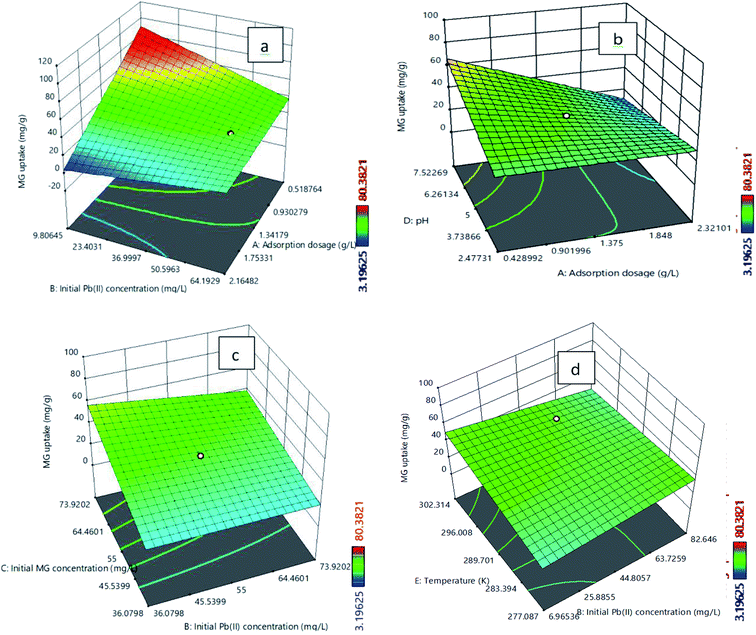 |
| Fig. 6 3D response plot for the interaction effect of (a) adsorbent dosage–initial Pb(II) concentration, (initial MG concentration: 55 mg L−1; pH: 5; temperature: 298 K) (b) adsorbent dosage–pH (initial Pb(II) concentration: 55 mg L−1; initial MG concentration: 55 mg L−1; temperature: 298 K), (c) initial Pb(II) concentration–initial MG concentration (adsorbent dosage: 1.375 g L−1; pH: 5; temperature: 298 K) and (d) initial Pb(II) concentration–temperature (adsorbent dosage: 1.375 g L−1; pH: 5; initial MG concentration: 55 mg L−1) on the MG uptake. | |
3.5.3 Optimization of adsorption parameters. The maximum adsorption of Pb(II) and MG with the optimum values of operating parameters were calculated by the point prediction method. The adsorption experiments were further conducted with the optimized process parameters and the comparison between the predicted and experimental values are shown in Table 1. It is found that the experimental results are in quite good agreement with the predicted results.
Table 1 Optimum process variables for adsorption of Pb(II) and MG
Ada (g L−1) |
C0 Pb(II)b (mg L−1) |
C0 MGc (mg L−1) |
pH |
Temp.d (K) |
Pb(II) uptake (mg g−1) |
MG uptake (mg g−1) |
Pred.e |
Exp.f |
Pred.e |
Exp.f |
Adsorbent dosage. Initial Pb(II) concentration. Initial MG concentration. Temperature. Predicted. Experimental. |
1.375 |
55 |
55 |
5 |
298 |
38.68 |
38.45 |
34.47 |
34.26 |
3.6 Thermodynamic analysis
The in-depth analysis of inherent energy changes associated with adsorption processes was determined by the thermodynamic analysis. The changes in the Gibbs free energy (ΔG0), enthalpy (ΔH0) and entropy (ΔS0) are calculated and reported in Table S12† (the equations are described in ESI Section S4†). The endothermic nature of the adsorption process is ensured by the positive values of ΔH0. Simultaneously, during the adsorption process, the randomness at the solid–liquid interface is confirmed by the positive values of ΔS0. The negative value of ΔG0 confirms the spontaneous nature of the process.46,52,53 However, the positive value of ΔG0 implies that the adsorption process is not spontaneous, thus requiring the mechanical agitation.54
3.7 Regeneration
The reusability of the adsorbent was checked by the regeneration of SiA-2 by desorbing Pb(II) and MG. SiA-2 was suspended in a dilute HCl solution (0.01 mol L−1) for 1 h to desorb Pb(II).55 Then, SiA-2 particles were washed with distilled water. Then, the particles were suspended in a C2H5OH–water solution for 1 h to desorb MG. Thereafter, the adsorbent was washed again with distilled water and dried in a hot air oven at 383 K for 8 h. The regenerated adsorbent was further used for the simultaneous removal of Pb(II) and MG. In Fig. S11,† it is shown tha, after five cycles of adsorption, the adsorption efficiency of SiA-2 was reduced by only 15.05% and 10.07% for Pb(II) and MG, respectively. This demonstrates that SiA-2 particles have considerably good reusability for the Pb(II) and MG adsorption.
4. Comparison of Pb(II) and MG adsorption on different adsorbents with SiA-2
The adsorption capacities of different adsorbents for Pb(II) and MG are available in the literature. However, it is observed that SiA-2 offers a better adsorption capacity than many other adsorbents. Moreover, SiA-2 has a satisfactory level of reusability. The comparative study is documented in Table 2 for Pb(II) and MG adsorption.
Table 2 Comparison of the Pb(II) and MG adsorption capacity with different adsorbents
Pollutant |
Adsorbents |
Adsorption uptake (mg g−1) |
Reference |
Pb(II) |
SiA-2 |
326.2 |
Present research |
γ-Alumina |
65.67 |
56 |
Al(OH)3/(PAA-co-PAM) |
106.2 |
57 |
HCl-treated Egyptian kaolin |
34.5 |
58 |
Acid activated red mud |
6.0273 |
59 |
Cu-MOFs/Fe3O4 |
219.00 |
60 |
MG |
SiA-2 |
1655.2 |
Present research |
Cu-MOFs/Fe3O4 |
113.67 |
60 |
Bentonite |
178.6 |
61 |
Copper sulfide nanorods loaded on activated carbon |
47.892 |
62 |
Boron-doped mesoporous carbon nitride |
310 |
63 |
Melamine-MOF |
122.0 |
64 |
Magnetic graphene oxide decorated with persimmon |
560.58 |
65 |
5. Conclusion
In this study, a novel methodology is proposed for the synthesis of a mesoporous alumina–silica nano-sorbent from coal fly ash and a waste aluminium foil. The in-depth characterizations of the nano-sorbent confirm the drastic changes in surface area, pore structure, pore volume, morphology and crystalline structure, which facilitate its adsorption capacity. The nano-adsorbent is successfully utilized to remove the toxic pollutants, namely Pb(II) and MG, from single to multi-component solutions. The as-synthesized nano-adsorbent has an excellent adsorption capacity and reusability. The nature of adsorption is investigated by analysing the equilibrium adsorption isotherms and adsorption kinetics. The resemblance to the Langmuir isotherm model enlightens the monolayer adsorption mechanism; however, the nature of adsorption kinetics suggests that the process is affected by both the chemisorption and physical adsorption. Simultaneously, the applicability of the intra-particle diffusion model confirms the multiple steps of adsorption. Besides, the endothermic nature of the adsorption process is confirmed by the negative values of enthalpy change via a detailed thermodynamic analysis. Moreover, RSM is employed to decide the optimum operating parameters for the adsorption of noxious heavy metal and dye from the binary solutions. The as-synthesized nano-sorbent has exhibited high adsorption capacities for both lead and malachite green as (1) for single component 326.2 and 1655.2 mg g−1, respectively, and (2) for binary components 615.86 and 445.03 mg g−1, respectively. More importantly, the observed adsorption capacities of the as-synthesized adsorbent for the concerned pollutants are reasonably higher than those reported in the literature. This apart, the as-synthesized adsorbent shows 15.05% and 10.07% reduction in adsorption capacity after five cycles of reuse for Pb(II) and MG, respectively. These observations have attested the effective and economical nature of the adsorbent, which has a potential application in treating industrial wastewater.
Conflicts of interest
There are no conflicts to declare.
References
- D. Shen, J. Fan, W. Zhou, B. Gao, Q. Yue and Q. Kang, J. Hazard. Mater., 2009, 172, 99–107 CrossRef CAS PubMed.
- L. Zou, P. Shao, K. Zhang, L. Yang, D. You, H. Shi, S. G. Pavlostathis, W. Lai, D. Liang and X. Luo, Chem. Eng. J., 2019, 364, 160–166 CrossRef CAS.
- F. Ding, M. Gao, T. Shen, H. Zeng and Y. Xiang, Chem. Eng. J., 2018, 349, 388–396 CrossRef CAS.
- T. Brudey, L. Largitte, C. Jean-Marius, T. Tant, P. C. Dumesnil and P. Lodewyckx, J. Anal. Appl. Pyrolysis, 2016, 120, 450–463 CrossRef CAS.
- M. J. Pirouz, M. H. Beyki and F. Shemirani, Food Chem., 2015, 170, 131–137 CrossRef CAS PubMed.
- P. S. De Velasco Maldonado, V. Hernández-Montoya, A. Concheso and M. A. Montes-Morán, Appl. Surf. Sci., 2016, 386, 381–388 CrossRef CAS.
- H. Zhang, F. Zhang and Q. Huang, RSC Adv., 2017, 7, 5790–5799 RSC.
- B. Ekka, S. R. Nayak, P. Dash and R. K. Patel, AIP Conf. Proc., 2016, 1724, 020011 CrossRef.
- M. Guner and O. Yucel, J. Appl. Sci., 2005, 5, 1843–1849 CrossRef CAS.
- P. Assefi, M. Ghaedi, A. Ansari, M. H. Habibi and M. S. Momeni, J. Ind. Eng. Chem., 2014, 20, 2905–2913 CrossRef CAS.
- J. R. De Andrade, M. F. Oliveira, M. G. C. Da Silva and M. G. A. Vieira, Ind. Eng. Chem. Res., 2018, 57, 3103–3127 CrossRef CAS.
- P. Saha, S. Chowdhury, S. Gupta and I. Kumar, Chem. Eng. J., 2010, 165, 874–882 CrossRef CAS.
- J. Ma, F. Li, T. Qian, H. Liu, W. Liu and D. Zhao, Chem. Eng. J., 2017, 315, 191–200 CrossRef CAS.
- Y. Liu, Q. Gao, S. Pu, H. Wang, K. Xia, B. Han and C. Zhou, Colloids Surf., A, 2019, 568, 391–401 CrossRef CAS.
- J. Liu, Y. Wang, Y. Fang, T. Mwamulima, S. Song and C. Peng, J. Mol. Liq., 2018, 250, 468–476 CrossRef CAS.
- E. Pehlivan, T. Altun and S. Parlayici, Food Chem., 2012, 135, 2229–2234 CrossRef CAS PubMed.
- K. G. Bhattacharyya, J. Sarma and A. Sarma, J. Hazard. Mater., 2009, 165, 271–278 CrossRef CAS PubMed.
- I. Anastopoulos, I. Pashalidis, A. Hosseini-Bandegharaei, D. A. Giannakoudakis, A. Robalds, M. Usman, L. B. Escudero, Y. Zhou, J. C. Colmenares, A. Núñez-Delgado and É. C. Lima, J. Mol. Liq., 2019, 295, 1–17 CrossRef.
- C. L. Chen, X. K. Wang and M. Nagatsu, Environ. Sci. Technol., 2009, 43, 2362–2367 CrossRef CAS PubMed.
- A. E. Rider, K. Ostrikov and S. A. Furman, Aust. Phys., 2013, 50, 162–165 Search PubMed.
- H. Abd El-Azim, M. M. El-Sayed Seleman and E. M. Saad, J. Environ. Chem. Eng., 2019, 7, 102915 CrossRef CAS.
- A. Bhatnagar and A. K. Jain, J. Colloid Interface Sci., 2005, 281, 49–55 CrossRef CAS PubMed.
- A. Bhatnagar, A. K. Jain, A. K. Minocha and S. Singh, Sep. Sci. Technol., 2006, 41, 1881–1892 CrossRef CAS.
- A. Bhatnagar, J. Hazard. Mater., 2007, 139, 93–102 CrossRef CAS PubMed.
- X. Li, M. Ji, L. D. Nghiem, Y. Zhao, D. Liu, Y. Yang, Q. Wang, Q. T. Trinh, D.-V. N. Vo, V. Q. Pham and N. H. Tran, J. Mol. Liq., 2019, 112286 Search PubMed.
- Z. T. Yao, M. S. Xia, P. K. Sarker and T. Chen, Fuel, 2014, 120, 74–85 CrossRef CAS.
- M. Erol, S. Küçükbayrak and A. Ersoy-Meriçboyu, J. Hazard. Mater., 2008, 153, 418–425 CrossRef CAS PubMed.
- H. Lee, H. S. Ha, C. H. Lee, Y. B. Lee and P. J. Kim, Bioresour. Technol., 2006, 97, 1490–1497 CrossRef CAS PubMed.
- F. Arroyo, N. Pérez Camacho, P. Coca and C. Fernández-Pereira III, World Coal Ash, Conf. Proc., 2009, 1–13 Search PubMed.
- W. Feng, Z. Wan, J. Daniels, Z. Li, G. Xiao, J. Yu, D. Xu, H. Guo, D. Zhang, E. F. May and G. Li, J. Cleaner Prod., 2018, 202, 390–400 CrossRef CAS.
- D. Jain, C. Khatri and A. Rani, Fuel Process. Technol., 2010, 91, 1015–1021 CrossRef CAS.
- M. Karanac, M. Đolić, Đ. Veljović, V. Rajaković-Ognjanović, Z. Veličković, V. Pavićević and A. Marinković, Waste Manag., 2018, 78, 366–378 CrossRef CAS.
- G. H. Bai, W. Teng, X. G. Wang, J. G. Qin, P. Xu and P. C. Li, Trans. Nonferrous Met. Soc. China, 2010, 20, s169–s175 CrossRef CAS.
- A. Chatterjee, J. K. Basu and A. K. Jana, Powder Technol., 2019, 354, 792–803 CrossRef CAS.
- M. Roosta, M. Ghaedi and M. Mohammadi, Powder Technol., 2014, 267, 134–144 CrossRef CAS.
- M. Roosta, M. Ghaedi, N. Shokri, A. Daneshfar, R. Sahraei and A. Asghari, Spectrochim. Acta, Part A, 2014, 118, 55–65 CrossRef CAS PubMed.
- N. Zainudin, K. Lee, A. Kamaruddin, S. Bhatia and A. Mohamed, Sep. Purif. Technol., 2005, 45, 50–60 CrossRef CAS.
- A. V. Palodkar, K. Anupam, S. Banerjee and G. Halder, Environ. Prog. Sustainable Energy, 2017, 36, 1597–1611 CrossRef CAS.
- L. Zhang, H. Zhang, W. Guo and Y. Tian, Appl. Clay Sci., 2014, 93–94, 85–93 CrossRef CAS.
- A. Ozer, G. Akkaya and M. Turabik, Dyes Pigm., 2006, 71, 83–89 CrossRef.
- H. Mazaheri, M. Ghaedi, A. Asfaram and S. Hajati, J. Mol. Liq., 2016, 219, 667–676 CrossRef CAS.
- S. Deng and Y. P. Ting, Langmuir, 2005, 21, 5940–5948 CrossRef CAS PubMed.
- N. M. Mahmoodi, B. Hayati and M. Arami, J. Chem. Eng. Data, 2010, 55, 4638–4649 CrossRef CAS.
- S. Wang and E. Ariyanto, J. Colloid Interface Sci., 2007, 314, 25–31 CrossRef CAS PubMed.
- E. Erdem, N. Karapinar and R. Donat, J. Colloid Interface Sci., 2004, 280, 309–314 CrossRef CAS PubMed.
- Q. Song, Y. Fang, Z. Liu, L. Li, Y. Wang, J. Liang, Y. Huang, J. Lin and L. Hu, Chem. Eng. J., 2017, 325, 71–79 CrossRef CAS.
- Y. Sun, S. Yang, Y. Chen, C. Ding, W. Cheng and X. Wang, Environ. Sci. Technol., 2015, 49, 4255–4262 CrossRef CAS PubMed.
- J. Lan, D. Cao, W. Wang and B. Smit, ACS Nano, 2010, 4, 4225–4237 CrossRef CAS PubMed.
- S. Kerisit and C. Liu, Environ. Sci. Technol., 2014, 48, 3899–3907 CrossRef CAS PubMed.
- J. N. Sahu, J. Acharya and B. C. Meikap, J. Hazard. Mater., 2009, 172, 818–825 CrossRef CAS PubMed.
- K. Mahalik, J. N. Sahu, A. V. Patwardhan and B. C. Meikap, J. Hazard. Mater., 2010, 182, 603–610 CrossRef CAS PubMed.
- Y. Liu, C. Yan, Z. Zhang, H. Wang, S. Zhou, W. Zhou, H. Wang, Y. Liu, W. Zhou, Z. Zhang, C. Yan, Z. Zhang, H. Wang, S. Zhou and W. Zhou, Fuel, 2016, 185, 181–189 CrossRef CAS.
- Y. Liu, J. Chem. Eng. Data, 2009, 54, 1981–1985 CrossRef CAS.
- R. M. Ali, H. A. Hamad, M. M. Hussein and G. F. Malash, Ecol. Eng., 2016, 91, 317–332 CrossRef.
- M. A. Khan, M. Otero, M. Kazi, A. A. Alqadami, S. M. Wabaidur, M. R. Siddiqui, Z. A. Alothman and S. Sumbul, J. Hazard. Mater., 2019, 365, 759–770 CrossRef CAS PubMed.
- A. Bhat, G. B. Megeri, C. Thomas, H. Bhargava, C. Jeevitha, S. Chandrashekar and G. M. M. Madhu, J. Environ. Chem. Eng., 2015, 3, 30–39 CrossRef CAS.
- Q. Zhao, L. Ren, H. Zhou, T. Cao and P. Chen, Chem. Emg. J., 2014, 250, 6–13 CAS.
- S. A. Drweesh, N. A. Fathy, M. A. Wahba, A. A. Hanna, A. I. M. Akarish, E. A. M. Elzahany, I. Y. El-sherif and K. S. Abou-el-sherbini, Biochem. Pharmacol., 2016, 4, 1674–1684 CAS.
- M. K. Sahu, S. Mandal, S. S. Dash, P. Badhai and R. K. Patel, J. Environ. Chem. Eng., 2013, 1, 1315–1324 CrossRef CAS.
- Z. Shi, C. Xu, H. Guan, L. Li, L. Fan, Y. Wang and L. Liu, Colloids Surf., A, 2018, 539, 382–390 CrossRef CAS.
- S. Ayhan, E. Bulut, M. Özacar and I. A. Şengil, Microporous Mesoporous Mater., 2008, 115, 234–246 CrossRef.
- E. Sharifpour, H. Zare, M. Ghaedi and A. Asfaram, Ultrason. Sonochem., 2018, 40, 373–382 CrossRef CAS PubMed.
- E. B. Azimi, A. Badiei and J. B. Ghasemi, Appl. Surf. Sci., 2019, 469, 236–245 CrossRef.
- N. Yin, K. Wang, Y. Xia and Z. Li, Desalination, 2018, 430, 120–127 CrossRef CAS.
- M. Gao, Z. Wang, C. Yang, J. Ning, Z. Zhou and G. Li, Colloids Surf., A, 2019, 566, 48–57 CrossRef CAS.
Footnote |
† Electronic supplementary information (ESI) available. See DOI: 10.1039/d0ra01397h |
|
This journal is © The Royal Society of Chemistry 2020 |
Click here to see how this site uses Cookies. View our privacy policy here.