DOI:
10.1039/D0RA01004A
(Paper)
RSC Adv., 2020,
10, 23066-23079
Heavy metal pollution and human health risk assessment at mercury smelting sites in Wanshan district of Guizhou Province, China†
Received
2nd February 2020
, Accepted 9th June 2020
First published on 17th June 2020
Abstract
The Wanshan district of Guizhou Province has a long history of mercury mining and smelting. Previous studies have been carried out on heavy metal (HM) pollution in the soil around Wanshan (such as in urban and farmland areas), but these studies have not been conducted at mercury smelting sites. In this study, the distribution characteristics of As, Be, Cd, Cr, Cu, Hg, Ni, Sb, Pb and Zn and their sources in the shallow stratum (<10 m) of the mercury smelting site in the Wanshan district were analyzed. Human health risks were evaluated using deterministic risk assessment (DRA) and probabilistic risk assessment (PRA) models. The contribution rates of different HM sources to human health risks were also calculated. The maximum HM concentration in mercury smelting site soil occured in the shallow soil (0–1 m), and the concentration sequences were as follows: 358.51 mg kg−1 (Hg) > 248.6 mg kg−1 (Zn) > 67.42 mg kg−1 (As) > 59.04 mg kg−1 (Ni) > 57.56 mg kg−1 (Pb) > 49.59 mg kg−1 (Cr) > 46.65 mg kg−1 (Sb) > 15.65 mg kg−1 (Cu) > 2.02 mg kg−1 (Be) > 0.78 mg kg−1 (Cd). The variable coefficients (CVs) were 1.64 (As), 0.67 (Be), 3.15 (Cd), 1.89 (Cr), 0.95 (Cu), 3.08 (Hg), 0.79 (Ni), 1.41 (Sb), 0.68 (Pb) and 1.13 (Zn), respectively. The HM concentrations in deep soils (9 m) still exceed the local background values, suggesting that heavy metals in shallow soil have migrated downward in the site. Three pollution sources identified with the shallow soil (0–1 m) HMs using the positive matrix factorization (PMF) model, were mercury smelting and coal combustion mixed sources (As, Hg and Zn), parent material sources (Ni, Cu, Cr, Cd and Sb) and wastewater discharge sources (Cu and Pb), respectively. DRA indicated that oral ingestion was the main pathway affecting the carcinogenic risk (CR) and hazard quotient (HQ) of heavy metals. The total-CR of twenty-five sampling points is between 1.219 × 10−6 and 3.446 × 10−4, and the total-HQ is between 0.37 and 43.56. PRA results indicated that DRA will underestimate the health risk of all populations in Guizhou Province, especially female, and BWa is the most influential variable for the PRA results. Smelting and coal combustion mixed sources contributed the most CR (99.29%) and with an HQ of 89.38% were the major sources of pollution affecting human health.
1. Introduction
Soil, as an important part of the terrestrial ecosystem, is not only the carrier of heavy metals but also the medium for heavy metals to spread to the atmosphere, organisms and water bodies.1 Heavy metals are persistent and accumulative in soil, and can inhibit the survival of plants and microorganisms, accumulate continuously through the food chain, and finally enter the human body through ingestion, thus threatening human health.2–4 In recent years, with the improvement of environmental protection, heavy metal pollution at industrial sites has attracted more attention, and heavy metal pollution remediation in the soil at industrial sites has become a popular topic.5–10
The heavy metal pollution characteristics, pollution source analysis and human health risk assessment at industrial contaminated sites are essential links in the treatment of contaminated soil. PMF model has been extensively applied to the analysis of heavy metal contamination sources in soil.11–13 Taking farmland soil around the Shuikoushan lead-zinc mine in Hunan Province as an example, Wei combined PMF model with spatial distribution of heavy metals concentration to determine that Pb, Zn, Cd and Sb mainly came from industrial activity sources such as lead-zinc mine mining and smelting (26.81%); As and Hg were mainly from agricultural activities such As sewage irrigation and agrochemical fertilizers (14.68%) and the main components of Cr, Ni, Co and Mo were parent material (24.41%).14 According to the analysis of heavy metal pollution sources of surface soil in Zhundong open-pit mining area by Blial, the contribution rates of coal burning, transportation, atmospheric dust removal, industrial emission and natural factors are 20.79%, 16.83%, 16.83%, 27.72% and 17.82%, respectively.15 Chen used PMF model to analyze the source and contribution rate of heavy metal pollution in suburban farmland, and the results showed that industrial and agricultural production (64.02%) is the main source of heavy metal in suburban farmland.16 Yang compared the analysis results of pollution sources among PMF model, MLR and Unmix models, showing that the analysis results obtained by PMF model are richer and more effective.17 Boroumandi used PMF model, principal component analysis (PCA) and cluster analysis (CA) to analyze the sources of heavy metals in the soil of Zanjan basin in Iran, showing that there are four sources of heavy metals in the soil in this area, which are mother material source, agricultural production source, industrial production source and industrial waste emission source. At the same time, the results show that the error of PMF model is smaller than that of PCA model, and the analytical results are more reasonable.18
Human health risk assessment of heavy metals can inform soil remediation at contaminated sites and provide remediation goals.19 Deterministic risk assessment (DRA) is the most commonly used method for carcinogenic risk and hazard quotient assessment of heavy metals in soil.20–22 By determining whether the carcinogenic risk and the hazard quotient of heavy metals exceed the risk acceptance level, the influence of heavy metals on human health can be judged.23,24 DRA use safety factors or conservative parameters to ensure that the actual risk is not underestimated. But its results often do not reflect the actual situation.25 Probabilistic risk assessment (PRA) provides a reasonable improvement to DRA by generating a range of risk values and the average estimate and degree of conservativeness of these estimates.26 In the PRA risk calculation, the 90–99.9% interval of the cumulative probability distribution is considered to be the maximum exposure within a reasonable range. The probability that the actual risk of the site is greater than this range is 0.1–10%. In recent years, some scholars have used pollution sources to quantitative assessment the human health risks, and human health risk assessments based on contamination sources of soil HMs at industrial sites are more directly responsive to the impact of industrial production on human health than deterministic risk assessments and probabilistic risk assessments.27,28 At contaminated industrial sites, the heavy metal pollution sources are mainly related to industrial productions.29 Different industrial production activities will produce different heavy metal pollution sources, which will have different effects on human health risk.30 Therefore, understanding the influence of industrial production activities on human health risks is of great significance not only to control pollution at the source but also to provide a reference for optimizing industrial production. In addition, industrial areas are distinguished from other areas, such as farmland, where there are often single pollution sources, the area is large, and the soil physical and chemical properties are relatively uniform. Due to the small overall areas of industrial contaminated sites, changes in soil physical and chemical properties also have a great impact on heavy metals. Therefore, the analysis of soil physical and chemical properties at industrial sites is also necessary.5
Based on these conditions, in this study, a typical mercury smelting site in Guizhou Province was selected. The concentrations of As, Be, Cd, Cr, Cu, Hg, Ni, Sb, Pb and Zn in the soil were analyzed. The sources of heavy metals were identified by PMF methods in combination with the production processes at contaminated industrial sites. Human health exposure parameters issued by the Ministry of Ecological Environment of the People's Republic of China and Exposure Factors Handbook of Chinese Population (Adult) were used to evaluate the human health exposure risk from different populations and pollution sources at contaminated sites. The objectives of the present study are, first, to obtain information on soil heavy metal contamination at mercury smelting sites; second, to identify the sources of heavy metals in soil at mercury smelting sites; and third, to analyze the effects of heavy metals on health risks in different populations and the contribution of different sources of contamination to human health risks.
2. Materials and methods
2.1 Study area
Guizhou Province has the largest mercury reserves in China, and the cinerite reserves contain approximately 8.81 × 104 t of mercury, among which the cinerite reserves in the Wanshan district account for 70% of the total mercury in Guizhou Province.31 Guizhou Province has conducted mercury smelting for thousands of years. Due to the simple mining and smelting process and backward environmental treatment measures, large amounts of mining waste rock and smelting slag are directly exposed to the environment, resulting in serious environmental pollution. China acceded the Minamata Convention on Mercury in 2013, which banned the mining of primary mercury mines, and pollution from mercury mining has been basically controlled, but the control measures for pollution cause by mercury smelting are still in their infancy. Based on this, the typical mercury smelting site in Wanshan district was selected as the research object. The mercury smelting site located in the Wanshan district industrial park, with 109°13′17′′ east longitude and 27°31′16′′ north latitude and an area of 42
620 square meters. The industrial site contains mercury chloride production workshops, mercury chloride catalyst production workshops and mercury processing production lines, as shown in Fig. 1. The site has been smelting mercury in a muffle furnace since 1951. With the adjustment of the production process, blast furnaces, tile furnaces, fluidized roasters and distillation furnaces are also used to refine mercury. Of these, blast furnaces and tile furnaces are the main mercury smelting method, smelting 47
050 tons of mercury, or 87.34% of the total mercury smelted, as shown in Fig. 1. There is no waste residue storage on this site, and the whole factory is out of production. The study area belongs to the subtropical monsoon climate, with four distinct seasons, and the dominant wind direction is northeast all year round.
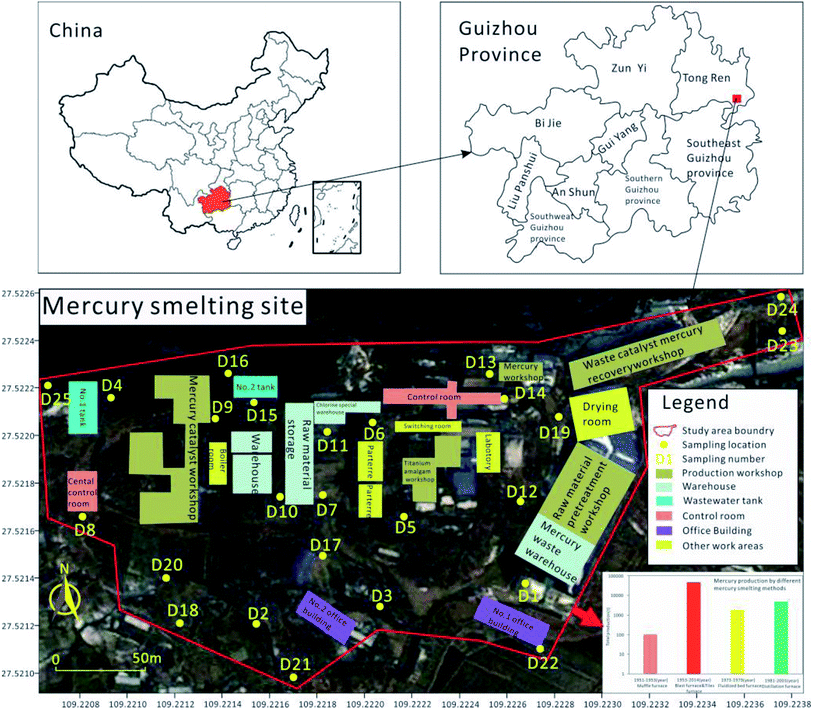 |
| Fig. 1 Location of the study area and layout of the sampling points. | |
2.2 Sample collection
A total of twenty-five soil boreholes were collected (Fig. 1). The sampling locations were determined according to the spatial distribution of pollution and hydrogeological information revealed in the confirmation sampling stage and adjusted according to the on-site XRF and other rapid detection equipment and pollution traces. The maximum sampling depth of 10 m, and the sample collection is divided into three categories. The first is to collect a sample of 20–50 cm of soil surface after removing concrete and plant roots. The second category is to collect one sample after each soil change layer of 20–50 cm. The third category is to collect a soil sample of the deepest point. However, according to the actual situation of the site, the sampling results of some boreholes will be different from the sampling principles. Among the samples, forty-four As, Be, Cd, Cr, Cu, Ni, Sb, Pb and Zn and ninety Hg test samples were collected (contains thirteen parallel samples).
2.3 Sample analysis
The soil samples were placed in an indoor ventilation area for natural air drying. After grinding, the samples were placed into an experimental bag with a 100 mesh nylon mesh screen for later use. To avoid human interference and contact with other metals, tools such as a wooden shovel, wooden stick and agate mortar were used during sample collection, mixing, grinding and crushing. The heavy metals As, Be, Cd, Cr, Cu, Ni, Sb, Pb and Zn in the soil samples were digested by the HNO3–HF–HClO4 method, and their concentrations were determined by ICP-MS. The Hg concentration was determined by atomic fluorescence spectrophotometry after (1
:
1) aqua digestion. All the chemical reagents used in the experiment were of excellent grade. All glassware and plastic ware were soaked in (1
:
1) dilute nitric acid for 24 h and washed with ultra-pure water. To ensure the accuracy of analysis, blank and parallel samples were taken throughout the experiment, and national standard soil reference material (gss-28) was added during the testing process for quality control. The recovery rates were ranged within 103% ± 6% of all HMs. The soil pH value was determined by the potentiometric method, and the total organic carbon (TOC) was analyzed by the high-temperature potassium dichromate oxidation-volumetric method.32
2.4 Data processing
2.4.1 Statistical analysis. Descriptive statistics for the sample data included mean value, standard deviation (SD), coefficient of variation (CV), skewness and kurtosis of HMs. SPSS 22.0 software was used to test the correlation between HMs and physical–chemical properties of soil by use of Pearson correlation analysis. A positive matrix factorization (PMF) model was used to explore the sources of heavy metals. All maps were created using ArcGIS 10.6 software, EPA PMF5.0 software and origin 9.1 software.
2.4.2 Positive matrix factorization (PMF) model. The PMF model principle is to decompose the original matrix Eik into two factor matrices Aij and Bik and the residual matrix εik using least iterative squares. The PMF model is computed by decomposing the original matrix Eik several times to obtain the optimal matrices A and B, which leads to the minimum value of the objective function Q.33 The basic formula of the model is as follows: |
 | (1) |
|
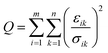 | (2) |
where, Eik is the concentration of the kth contaminant of the ith sample; Aij contributes to the ith sample in the jth source; Bik is the contribution of the kth pollutant in the jth source, ik is the random error. σik stands for Eik uncertainty.In this study, the US EPA PMF5.0 software was used to analyze the source of the contaminant, and the values of contaminant concentration and contaminant uncertainty were entered during the operation.34
There are two types of uncertainty values, when the contaminant concentration is less than or equal to the corresponding method detection limit (MDL), the uncertainty value is calculated as:
When the contaminant concentration is greater than the corresponding MDL, the value of the uncertainty is calculated as:
|
 | (4) |
where,
σ is the relative standard deviation;
C is pollutant concentration; MDL is the detection limit of the method.
15
2.4.3 Human health risk assessment. DRA models and related parameters referred to the technical guidelines for risk assessment of soil contamination of land for construction (Ministry of Ecology and Environment, 2019).35 As heavy metals are less volatile, oral ingestion, skin contact and inhalation of soil particles are considered as the main exposure routes. Probabilistic risk assessment (PRA) was carried out to analyze the influence of variabilities in exposure factors of different populations in Guizhou on the risk assessment results. Table 1 listed the specific expose parameters used in DRA and PRA, distributions for BWa, DAIRa and SAEa were obtained from Exposure Factors Handbook of Chinese Population (Adult).36 Distribution for PM10 was obtained from Monthly Environmental Quality Report of Guizhou Province (2019.1–2020.2).
Table 1 The specific expose parameters used in DRA and PRAa
Parameter symbol |
Unit |
DRA |
PRA |
Numerical |
Population of Guizhou Province |
Numerical |
— represents dimensionless. |
OSIRa |
mg d−1 |
100 |
— |
100 |
EDa |
a |
24 |
— |
24 |
EFa |
d·a−1 |
350 |
— |
350 |
Bwa |
kg |
61.8 |
Urban man |
Normal (mean = 63.2, 95% = 80.0) |
Urban women |
Normal (mean = 54.4, 95% = 70) |
Rural man |
Normal (mean = 59.4, 95% = 77.2) |
Rural women |
Normal (mean = 52.1, 95% = 68.1) |
ABSo |
— |
1 |
— |
1 |
ATca |
d |
27 740 |
— |
27 740 |
ATnc |
d |
2190 |
— |
2190 |
SAF |
— |
1 |
— |
1 |
SSARa |
mg cm−2 |
0.07 |
— |
0.07 |
SAEa |
Cm2 |
3022.87 |
Urban man |
Normal (mean = 3060, 95% = 3420) |
Urban women |
Normal (mean = 2700, 95% = 3060) |
Rural man |
Normal (mean = 2880, 95% = 3240) |
Rural women |
Normal (mean = 2520, 95% = 3060) |
Ev |
Time−1 |
1 |
— |
1 |
Ha |
cm |
161.5 |
— |
161.5 |
Hc |
cm |
113.15 |
— |
113.15 |
SERa |
— |
0.32 |
— |
0.32 |
DAIRa |
m3 d−1 |
14.5 |
Urban man |
Normal (mean = 17.7, 95% = 20.5) |
Urban women |
Normal (mean = 14.2, 95% = 16.0) |
Rural man |
Normal (mean = 16.9, 95% = 20.1) |
Rural women |
Normal (mean = 13.9, 95% = 15.8) |
PIAF |
— |
0.75 |
— |
0.75 |
fspi |
— |
0.8 |
— |
0.8 |
fspo |
— |
0.5 |
— |
0.5 |
EFIa |
d·a−1 |
262.5 |
— |
262.5 |
EFOa |
d·a−1 |
87.5 |
— |
87.5 |
PM10 |
mg m−3 |
0.119 |
— |
0.119 |
The human health risk contribution of each pollution source was quantitatively analyzed by using PMF model, and the mathematical expressions of the quantitative analysis are shown in eqn (5)–(14).37 The parameter values of each formula referred to the research results of the technical guidelines for risk assessment of soil contamination of land for construction (HJ 25.3-2019) and Li.38 Table S1† showed the hazard quotient reference dose and carcinogenic slope factor of the three exposure pathways of each pollutant.
|
 | (5) |
|
 | (6) |
|
 | (7) |
|
 | (8) |
|
 | (9) |
|
 | (10) |
|
Con(CRk)ij = Con(CRois)ij + Con(CRdsc)ij + Con(CRpis)ij
| (11) |
|
Con(HQk)ij = Con(HQois)ij + Con(HQdsc)ij + Con(HQpis)ij
| (12) |
|
 | (13) |
|
 | (14) |
where, Con
ij is the pollutant concentration of the
ith element and the
jth source; Con(CR
ois)
ij is the oral carcinogenic risk of the
ith element and the
jth source; Con(HQ
ois)
ij is the hazard quotient of the oral pathway of the
ith element and the
jth source; Con(CR
des)
ij is the carcinogenic risk of skin contact pathway from the
ith element and the
jth source; Con(HQ
des)
ij is the
ith element of the
jth source of skin contact route hazard quotient; Con(CR
pis)
ij is the carcinogenic risk of the
ith element and the
jth source in the respiratory inhalation pathway; Con(HQ
pis)
ij is the
ith element of the
jth source of respiratory inhalation pathway hazard quotient; Con(CR
k)
ij is the carcinogenic risk of the
ith element and the
jth source in three ways; Con(HQ
k)
ij is the third pathway of the
ith element and the
jth source is the
ith element of the
jth source of skin contact route hazard quotient; Con(total-CR
k)
ij is the total carcinogenic risk of the
ith element from the
jth source; Con(total-HQ
k)
ij is the total is the
ith element of the
jth source of skin contact route hazard quotient of the
ith element from the
jth source.
3. Results and discussion
3.1 Soil HMs concentrations
3.1.1 Vertical distribution of HMs concentration. The concentrations of ten heavy metals gradually decreased from the shallow-soil to the subsurface soil (Fig. 2). As, Hg and Sb had the highest concentrations, and in some sampling points, the concentrations of these three HMs exceeded the intervention values and controlling values.39–42 Hg was the most heavily contaminated heavy metal in the study area, which was consistent with the characteristics of the mercury smelting site.43 The concentrations of Cu, Ni, Pb, and Zn exceeded only the environmental background values of soil and did not exceed the intervention values. A few sampling points of Be, Cd, and Cr had concentrations exceeding the environmental background values of soil. The maximum concentrations of ten HMs in soil were located in the 0–1 m. It can be assumed that the shallow-soil (0–1 m) was the most polluted area at the site. However, the concentrations of As, Be, Cd, Cu, Hg, Ni, Sb, Pb and Zn in 9 m were still up to 36.97, 1.45, 0.37, 54.8, 17.83, 67.20,18.60, 76.46 and 509.93 mg kg−1, respectively, all exceed the background value. This indicated that there was a downward migration of heavy metals in shallow soil. Pearson correlation analysis (Table S2†) showed that As, Be and Hg were significantly correlated with TOC (P < 0.01), and the correlation coefficients were 0.730, 0.819 and 0.416, respectively. Cu was negatively correlated with pH value (P < 0.01), and the correlation coefficient was −0.473. Pb had a significant negative correlation with pH (P < 0.05), and Sb had a significant positive correlation with pH (P < 0.05). The concentration of soil HMs in the mercury smelting site was closely related to TOC and pH. Fig. 2 indicated both TOC and pH decrease with depth. Higher TOC may be the key factor leading to higher concentration of HMs in shallow-soil.44 In the deep part, the activity of soil heavy metals will increase with the lower of pH value, which will lead to the downward migration of soil HMs.
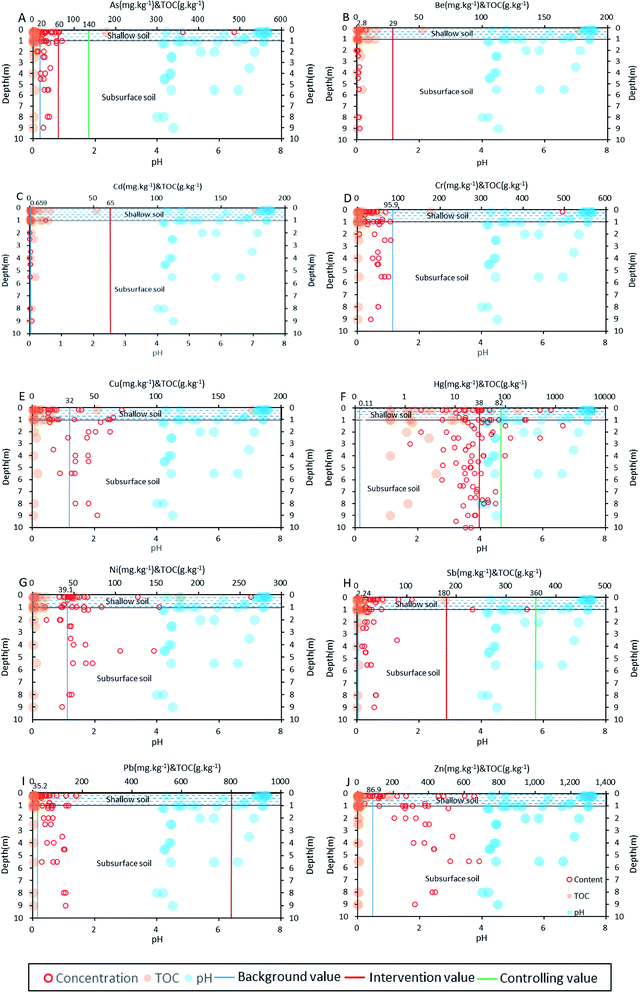 |
| Fig. 2 The varies of TOC, pH and ten HMs (As (A), Be (B), Cd (C), Cr (D), Cu (E), Hg (F), Ni (G), Sb (H), Pb (I) and Zn (J)) with depth. | |
3.1.2 HM concentration in the shallow soil (0–1.0 m). Based on the distribution of heavy metal pollution, in this study, the concentration of heavy metals in the shallow soil (0–1 m) was used to analyze the source of heavy metals and evaluate the risk to human health. The statistical results of the concentrations of ten HMs in twenty-five shallow soil (0–1 m) samples at the contaminated sites were shown in Table 2. The average concentrations of As, Cd, Hg, Ni, Sb, Pb and Zn were 67.42, 0.78, 358.51, 59.04, 46.65, 57.56 and 248.6 mg kg−1, respectively, all exceeding the background values. The average concentrations of Be, Cr and Cu are 2.02, 49.59 and 15.65 mg kg−1, which not exceeding the local soil background value. Among them, the soil pollution by Hg was the most serious, which consistent with the results of the analysis by Zhan.45 The variable coefficient (CV) values of As, Be, Cd, Cr, Cu, Hg, Ni, Sb, Pb and Zn were 1.64, 0.67, 3.15, 1.89, 0.95, 3.08, 0.79, 1.41, 0.68, and 1.13, respectively, and all were characterized by strong variation (CV > 0.5). This finding indicates that the local concentration of heavy metals varies greatly, the spatial variation is obvious, and the heavy metals are disturbed by external activities.46,47 The kurtosis and skewness values were used to characterize the double-tailed characteristics of the data. The HMs concentrations in soil under a natural state should follow a normal distribution.48 The skewness values of As, Cd, Cr, Hg, Ni and Sb were 3.28, 4.99, 4.8, 4.43, 3.83 and 4.13, respectively, and larger skewness values indicated more outliers produced by human activities, which change the distribution of data.
Table 2 Physico-chemical properties and HMs concentrations in the shallow soil of Wanshan district (n = 25)a
Character |
Min |
Max |
Mean |
Standard deviation (SD) |
Variable coefficient |
Kurtosis |
Skewness |
HMu represents the concentration of HMs in the urban soil around Wanshan mercury mine; HMf represents the concentration of HMs in farmland soil of Wanshan mercury mine. |
As (mg kg−1) |
1.17 |
485.69 |
67.42 |
110.33 |
1.64 |
10.37 |
3.28 |
Asu (mg kg−1) |
2.01 |
32.42 |
14.24 |
— |
— |
— |
— |
Asf (mg kg−1) |
95.11 |
160 |
117.6 |
21.87 |
— |
— |
— |
Be (mg kg−1) |
0.09 |
7.54 |
2.02 |
1.36 |
0.67 |
11.56 |
2.93 |
Cd (mg kg−1) |
0.09 |
12.56 |
0.78 |
2.46 |
3.15 |
24.96 |
4.99 |
Cdu (mg kg−1) |
0.26 |
2.22 |
0.87 |
— |
— |
— |
— |
Cdf (mg kg−1) |
0.27 |
0.51 |
0.43 |
— |
— |
— |
— |
Cr (mg kg−1) |
3.4 |
495 |
49.59 |
93.97 |
1.89 |
23.61 |
4.8 |
Cru (mg kg−1) |
308.21 |
377.80 |
353.22 |
— |
— |
— |
— |
Crf (mg kg−1) |
41.83 |
84.03 |
59.06 |
— |
— |
— |
— |
Cu (mg kg−1) |
0.09 |
71.92 |
15.65 |
14.89 |
0.95 |
8.25 |
2.58 |
Cuu (mg kg−1) |
26.35 |
56.83 |
41.45 |
— |
— |
— |
— |
Cuf (mg kg−1) |
34.22 |
59.97 |
43.77 |
8.5 |
— |
— |
— |
Hg (mg kg−1) |
5.57 |
5430 |
358.51 |
1102.47 |
3.08 |
20.6 |
4.43 |
Hgu (mg kg−1) |
0.08 |
67.88 |
14.15 |
— |
— |
— |
— |
Hgf (mg kg−1) |
3.09 |
8.05 |
4.29 |
1.37 |
— |
— |
— |
Ni (mg kg−1) |
15.15 |
263.32 |
59.04 |
46.82 |
0.79 |
16.28 |
3.83 |
Niu (mg kg−1) |
16.53 |
56.78 |
33.58 |
— |
— |
— |
— |
Nif (mg kg−1) |
14.42 |
24.74 |
18.8 |
3.1 |
— |
— |
— |
Sb (mg kg−1) |
7.28 |
341.74 |
46.65 |
65.54 |
1.41 |
18.67 |
4.13 |
Pb (mg kg−1) |
14.33 |
173.91 |
57.55 |
39.13 |
0.68 |
2.68 |
1.66 |
Pbu (mg kg−1) |
24.54 |
253.36 |
59.30 |
— |
— |
— |
— |
Pbf (mg kg−1) |
16.56 |
83.86 |
48.99 |
18 |
— |
— |
— |
Zn (mg kg−1) |
11.01 |
1304.76 |
248.60 |
280.37 |
1.13 |
7.88 |
2.56 |
Znf (mg kg−1) |
17.66 |
49.69 |
29.13 |
8.81 |
— |
— |
|
pH |
4.26 |
7.57 |
6.56 |
1.05 |
0.16 |
0.16 |
−1.10 |
pHu |
4.85 |
7.15 |
6.41 |
— |
— |
— |
— |
TOC (g kg−1) |
0.54 |
177.14 |
18.18 |
38.52 |
2.12 |
12.46 |
3.35 |
TOC of Guizhou Province (g kg−1) |
0.92 |
4.75 |
2.16 |
1.28 |
0.59 |
1.1 |
1.32 |
Compared with previous studies on heavy metal concentrations in the soil of urban and farmland areas around the Wanshan mercury mine,45,49 the maximum and average concentrations of Hg, Zn and Ni at the mercury smelting sites were higher than those in the urban and farmland areas. This difference was related to mercury production and the presence of smelters. The average concentrations of Cr and Cu at the mercury smelting sites were lower than those in the urban and farmland areas. The concentrations of As at mercury smelting sites was higher than that in urban areas but much lower than that in farmland, and the high As concentration in farmland was related to mercury mining in the Wanshan area.49 The concentrations of Pb and Cd at mercury smelting sites were higher than that in farmland but lower than that in urban areas. The characteristics of heavy metal concentrations in mercury smelting sites differed greatly from those in urban and farmland areas, so special treatment would be needed for the restoration process. The average pH value of the soil at the contaminated site was 6.56, slightly higher than that of urban soil. The mean value of TOC at the contaminated site was 18.18 g kg−1, about nine times the average TOC of the soil in Guizhou Province.50
3.2 Sources of HMs
3.2.1 Correlation analysis. HMs in soils mainly from parent materials and anthropogenic activities.51 There was a correlation between HMs from the same source.52 Significant or extremely significant correlations between HMs indicated the presence of homologous or compound contamination.53 Pearson correlation analysis of shallow-soil HMs concentration showed (Table 3) that As and Be, As and Zn were highly significant (P < 0.01) with correlation coefficients of 0.723 and 0.616, respectively, but Be and Zn were not correlated, indicated that As had two sources. Cu, Ni and Sb showed a significant correlation (P < 0.01) with a correlation coefficient greater than 0.6, indicating that the three HMs have the same source. Cd, Cr, Hg and Pb were not correlated with any other HMs.
Table 3 Pearson correlation analysis of soil HMs concentrations in shallow soila
|
As |
Be |
Cd |
Cr |
Cu |
Hg |
Ni |
Pb |
Sb |
Zn |
** was significantly correlated at the 0.01 level (bilateral). * significantly correlated at the 0.05 level (bilateral). |
As |
1 |
|
|
|
|
|
|
|
|
|
Be |
0.723** |
1 |
|
|
|
|
|
|
|
|
Cd |
−0.125 |
−0.295 |
1 |
|
|
|
|
|
|
|
Cr |
−0.012 |
0.053 |
−0.020 |
1 |
|
|
|
|
|
|
Cu |
0.009 |
0.116 |
−0.034 |
0.058 |
1 |
|
|
|
|
|
Hg |
0.121 |
0.336 |
−0.067 |
0.032 |
0.344 |
1 |
|
|
|
|
Ni |
−0.054 |
0.027 |
−0.211 |
−0.006 |
0.839** |
0.247 |
1 |
|
|
|
Pb |
−0.186 |
0.095 |
−0.108 |
−0.001 |
0.340 |
0.300 |
0.001 |
1 |
|
|
Sb |
−0.229 |
−0.148 |
−0.113 |
0.028 |
0.660** |
0.335 |
0.754** |
0.020 |
1 |
|
Zn |
0.616** |
0.249 |
−0.183 |
−0.079 |
0.421* |
0.219 |
0.468* |
−0.056 |
0.285 |
1 |
3.2.2 Pollution source analysis by PMF model. The US EPA PMF model was used to analyze data sets of ten HMs in shallow soil from twenty-five sampling points to identify pollution sources and the proportion of HMs in each source. To obtain the exact minimum value of the target function Q, set the F peak to −0.52 with a factor iteration of 20. Fig. S1† shows that the PMF predicted HMs concentration has a good correlation with the actual heavy metal concentration, indicating that the PMF simulation results were reasonable and feasible. Base on the strong explanatory ability and low Q value (0.3), three factors were selected (Fig. 3A).
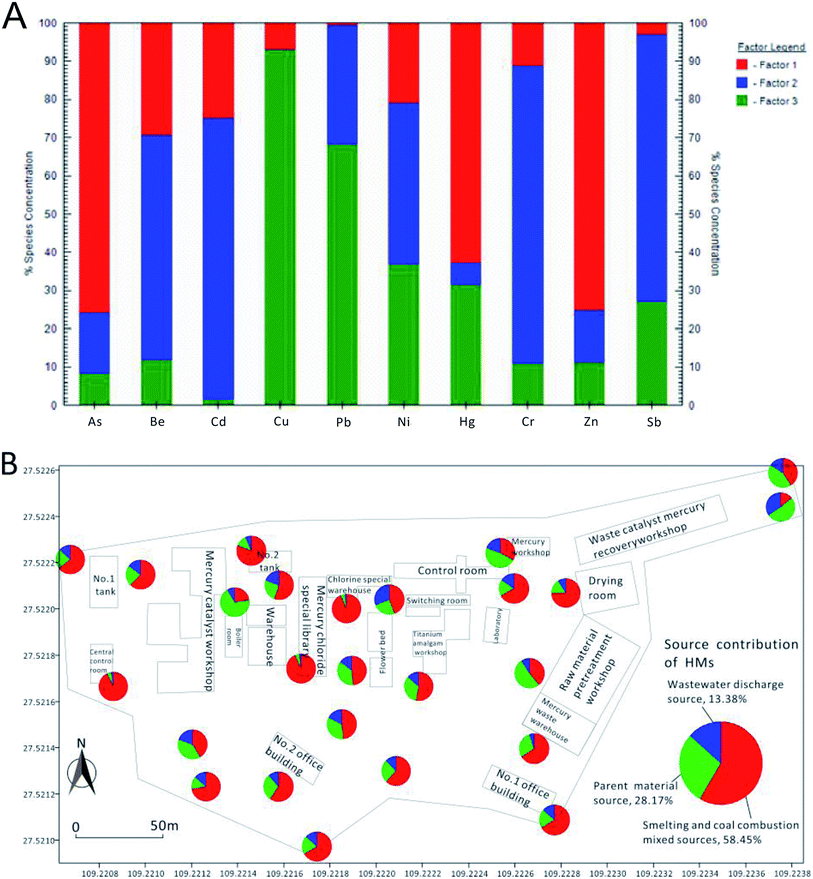 |
| Fig. 3 HMs sources analyzed by positive definite matrix (PMF) model (A) and contribution of different sources to HMs concentration (B). | |
As (75.7%), Hg (62.7%) and Zn (75.2%) have relatively high loads on factor 1. Hu showed that As was mainly anthropogenic in the soil around the Wanshan mercury mine, and Hg was associated with local mercury mining and smelting activities.49 Zhan believes that coal combustion was the main source of As in Wanshan's agricultural soil, while smelting was the main source of Hg and Zn.45 Guo showed that the soil As in the Wanshan mercury mining area was source of coal combustion, Hg was mainly source of smelting source and Zn was mainly source of transportation.54 A large amount of coal was used in the smelting of mercury in the site, and As was the identifying element of coal.55 At the same time, the blast furnace process produces a large amount of exhaust gases, which will spread to the southwest of the site with the atmosphere due to the prevailing northeast wind. This was the main reason why the higher levels of excess heavy metals at the site were mainly in the southwest (the distribution of ten HMs concentrations in shallow soil at the twenty-five sampling points was shown in Fig. S2†). Thus, factor 1 was primarily a source of mercury smelting and coal combustion.
Be (58.9%), Cd (73.5%), Cr (77.9%), Ni (42.2%) and Sb (69.7%) have relatively high loads on factor 2. The average concentration of Be, Cd and Cr did not exceeded the local soil background values, indicated that these three heavy metals mainly came from the parent material. Hu showed that Cr in the urban soil of the Wanshan district was mainly derived from the parent material.49 Gou found that Ni and Cr were mainly derived from parent material in the Wanshan mercury mining area.53 However, Cd in urban soil and farmland soil of Wanshan district were the result of mining and agricultural pollution,45,49 which was different from the sources at the mercury smelting site. Early studies showed that Ni in farmland soil of Wanshan district mainly derived from soil parent material and weathering products.56,57 Sb in soil was mainly derived from industrial “three waste” emissions and parent material.58,59 Correlation analysis showed a significant correlation between Sb and Ni in the soil at the mercury smelting site, and it assumed that Sb was also predominantly a soil-forming parent material source. Therefore, factor 2 represents the parent material source.
Pb (68.3%) and Cu (92.9%) have relatively high loads on factor 3. Zhan and Gou showed that Pb in the soil of Wanshan district was related to transportation.45,54 Hu showed that Pb in the Wanshan district have similar geochemical properties, and they coexist during the mineralization process and were closely associated with each other.49 However, for this mercury smelting site, due to the poor protective measures in the early stage of production, the wastewater, waste residue and waste gas were discharged into the soil and atmosphere without treatment. As a result, the soil at the site was seriously polluted. This verified that the maximum concentrations of Pb located in the No. 2 sewage tank. The results showed that Pb and Cu pollution at the site was mainly caused by wastewater discharge. Such pollution is also common at other industrial sites in China.59,60 Therefore, factor 3 represents the wastewater discharge source.
Fig. 3B showed the contribution of three sources to the average of ten HMs in the shallow-soil from 25 sampling sites. Smelting and coal combustion mixed sources was the main contributor to HMs pollution at the site (58.45%). Soil-forming parent material sources contributed 28.17% of the average HMs concentration, and wastewater discharge sources contribute 13.38% of the average HMs concentration.
3.3 Carcinogenic risk (CR) and hazard quotient (HQ) of HMs
3.3.1 CR and HQ in DRA. Four heavy metals, As, Be, Cd and Ni, were associated with CR, and As, Be, Cd, Cr, Cu, Hg, Ni, Sb, Pb and Zn have a HQ (Table S1†). According to HJ 25.3-2019-Technical guidelines for risk assessment of contaminated sites, this contaminated site was industrial land and belongs to category II land, and its HMs risk assessment considers only adult CR and HQ.Fig. 4A showed the CR values of the three exposure pathways of As, Ni, Cd and Be at twenty-five sampling points. The CR of three exposure pathways with As were all exceeded the acceptable level (1 × 10−6), among them, the CR of oral ingestion was the highest. It was worth noting that the CR value of oral ingestion with As at the D10 and D11 sampling points were at the upper limit of the tolerance interval (1 × 10−4), these sampling sites should be given special attention during soil remediation. The CR of the three exposure pathways of Be, Cd and Ni did not exceed the acceptable level (1 × 10−6).
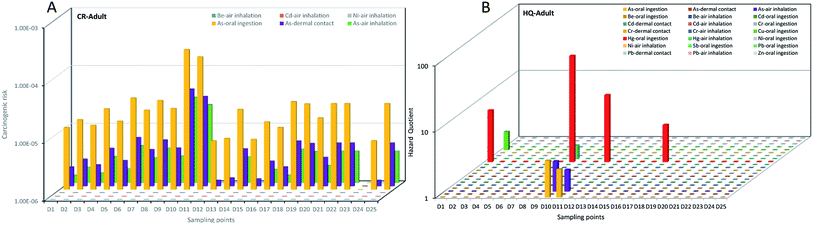 |
| Fig. 4 CR (A) and HQ (B) of ten heavy metals in twenty-five sampling sites of three pathways. | |
The HQ for the ten HMs in twenty-five borehole of three exposure pathways was listed in Fig. 4B. Hg was the main heavy metal that affects HQ in the site, and the HQ of Hg with oral ingestion at the D1, D8, D11 and D16 sampling points were the highest. In addition, the HQ of Hg with air inhalation at the D8 sampling points, Sb with oral ingestion at the D1 sampling points, and As with oral ingestion and air inhalation at the D10 and D11 sampling point are all upper limit of the tolerance interval of 1.
As, Hg and Sb were the major HMs that cause high CR and HQ of HMs in the soil at the mercury smelting site. This finding provides a target for soil remediation at the contaminated site.
3.3.2 CR and HQ in PRA. As the risk levels of As, Hg and Sb exceed the human health acceptable levels, these three HMs were taken as typical pollutants to carry out PRA. The probability distribution ranges of CR and HQ of three pollutants were shown in Fig. 5, all probabilities of health risk of four pollutants were greater than the tolerance interval. Under the same exposure scenario, the health risk of four populations in order of rural female > urban female > rural male > urban male. Using the exposure parameters recommended by the HJ 25.3-2019 for DRA will underestimate the health risk of all populations in Guizhou Province, especially female.
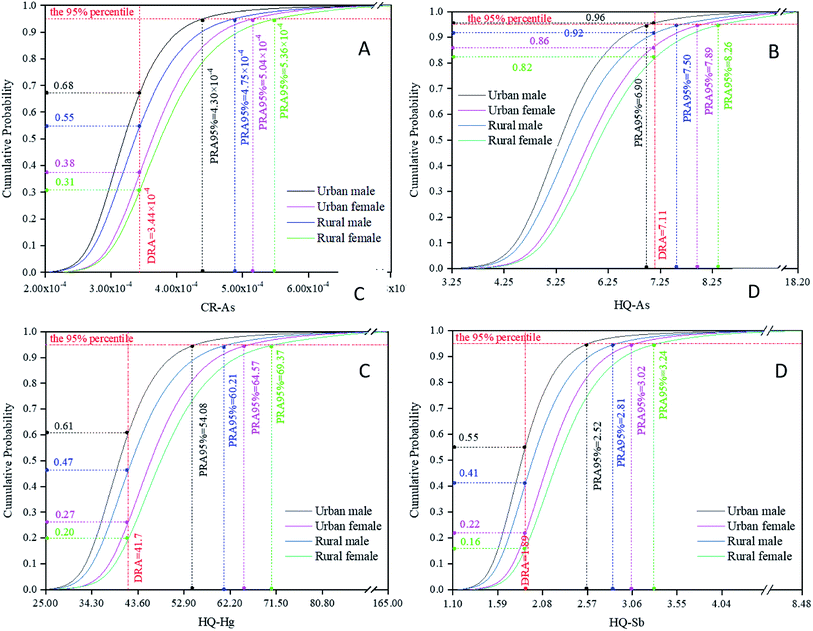 |
| Fig. 5 Comparison of the PRA and DRA results of As (A) and (B), Hg (C) and Sb (D). | |
For the CR of As (Fig. 5A), the risk values at the 95th percentile of the probability distributions of rural female, urban female, rural male and urban male were 0.56, 0.46, 0.38 and 0.25 times the DRA values. The DRA values were located only at the 31st, 38th, 55th and 68th percentiles of their probability distribution of each population. For the HQs of the three heavy metals (Fig. 5B–D), only the risk value at the 95th percentile of the As probability distribution of rural female (6.90) was slightly smaller the DRA value (7.11), and the DRA value was located at the 96th percentile of its probability distribution. The others were the opposite, and the risk values at the 95th percentile of the probability distributions were 0.05–0.16, 0.30–0.66 and 0.33–0.71 times the DRA values, and the DRA values were located at the 16th–55th, 20th–61st and 16th–55th percentiles of their probability distributions for As, Hg and Sb, respectively.
Taking the PRA of rural female as an example to carry out parameter sensitivity analysis, and the Spearman rank order correlation coefficient was used to explain sensitivity analysis results. For all the three heavy metals, BWa was the most influential variable (Fig. 6A), which was inversely proportional to the risk value. BWa (61.8 kg) used in DRA was located at the 44–55th percentile of its probability distribution (Fig. 6B), which was much greater than the value at the 5th percentile (46.39 kg), and it in turn causes the DRA result to be insufficiently conservative.
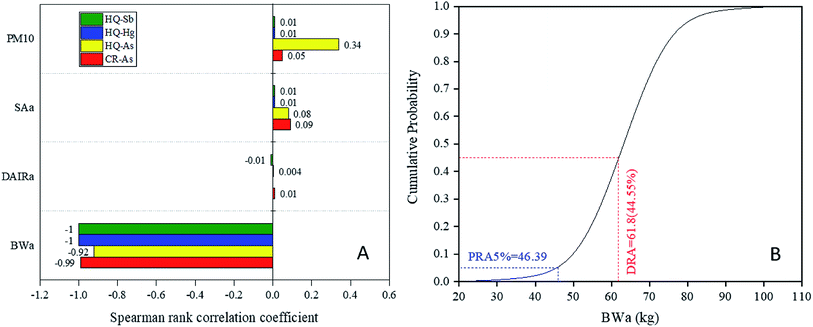 |
| Fig. 6 Sensitivity analysis for rural female (A) and (B). | |
3.3.3 CR and HQ in HMs sources. According to the sources of As, Be, Cd and Ni pollution, the CR at the twenty-five sampling sites contaminated by each source of were analyzed by DRA (Fig. 7A). The total CR value was between 1.219 × 10−6 and 3.446 × 10−4. The average total-CR of smelting and coal combustion mixed sources (As) and soil parent material sources (Be, Cd and Ni) were 4.779 × 10−5 and 3.15 × 10−7, with contribution rates of 97.32% and 2.68%, respectively. Smelting and coal combustion mixed sources was the main factor causing CR of HMs in the site.
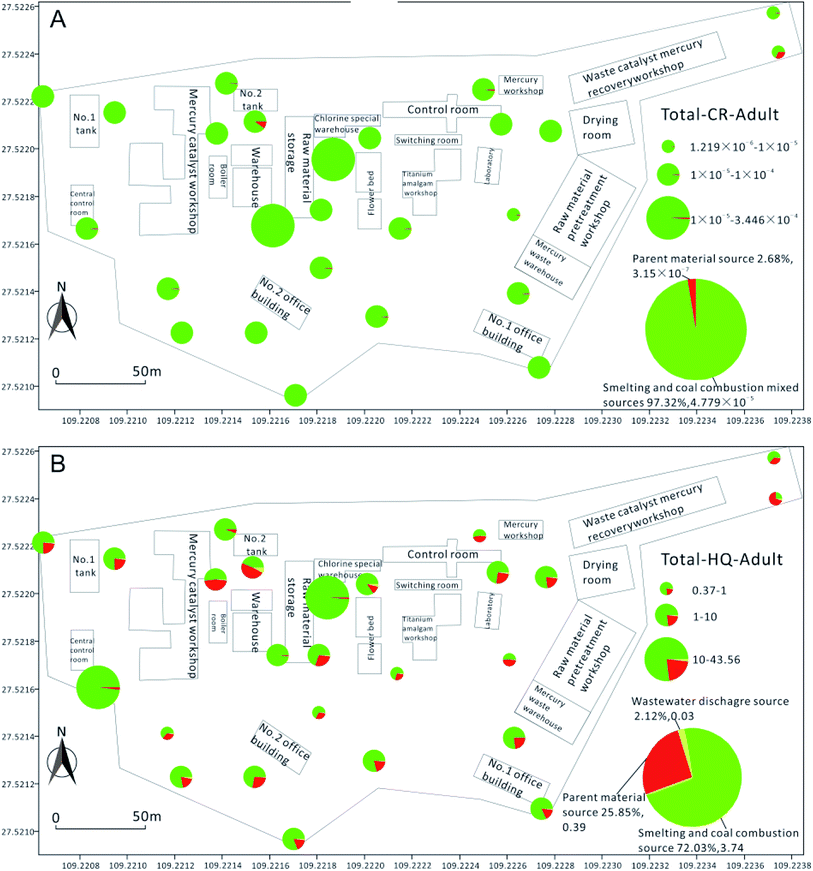 |
| Fig. 7 Contribution rate of CR (A) and HQ (B) of different pollution sources. | |
The interpolation maps of the HQ of three pollution sources was shown in Fig. 7B. The total- HQ of twenty-five sampling points was between 0.37 and 43.56. The total average HQ of the smelting and coal combustion mixed sources, soil parent material sources and wastewater discharge source were 3.74, 0.39 and 0.033, respectively, with contribution rates for the total average HQ of 72.03%, 25.85% and 2.12%, respectively. The smelting and coal combustion was the main pollution sources that affects the HQ of heavy metals at the site, too. The determination of major pollution sources can provide a reference for pollution protection, such as optimizing mercury smelting processes thereby reducing the CR and HQ of soil in the mercury smelting site.
4. Conclusion
In this study, the concentrations of ten heavy metals at depths of 0–10 m at typical mercury smelting sites were analyzed. The maximum concentrations of each heavy metal were mainly concentrated in the shallow-soil of 0–1 m. The higher organic carbon content was the main factor for the accumulation of HMs in the shallow soil, and the lower pH value of deep soil was a key factor affecting the transport of HMs from shallow soil to deep soil. Three pollution sources of the ten heavy metals in shallow soil were identified by PMF model, including mercury smelting and production mixed sources, parent material source and wastewater discharge source. DRA results indicated that As and Hg were the major heavy metals affecting CR and HQ in mercury smelting sites, respectively, with oral intake being the main route of risk to human health. The health risk of four populations in order of rural female > urban female > rural male > urban male, and BWa was the most influential variable that affects the results of PRA. DRA will underestimate the health risk of populations in Guizhou Province. Smelting and coal combustion mixed sources is the main pollution sources affecting human health in mercury smelting sites.
Conflicts of interest
We declare are no conflicts of interest.
Acknowledgements
Funding for the Soil Specific Topic (2018YFC1803001) and the Technical Topic on the Causes and Management of Site Soil Pollution (2018YFC1801401) are appreciated.
References
- M. A. Ashraf, M. J. Maah and I. Yusoff, Sci. World J., 2012, 1–11, DOI:10.1100/2012/125608.
- B. J. Alloway, Heavy metals in soils, London, Chapman and Hall., 1995 Search PubMed.
- Z. Mamata, H. Yimit, R. Zi, A. Ji and M. Eziz, Sci. Total Environ., 2014, 493, 1098–1111 CrossRef PubMed.
- P. A. Kabata and H. Pendias, Trace elements in soils and plants, London, CSC Press., 3rd edn, 2001 Search PubMed.
- P. Thavamani, M. Megharaj and R. Naidu, Environ. Monit. Assess., 2012, 184, 3875–3885 CrossRef CAS PubMed.
- X. Wang, J. Huang, Z. Liu and X. Yue, Environ. Sci., 2013, 34, 368–372 Search PubMed.
- W. Deng, X. Li, Z. An and L. Yang, Environ. Monit. Assess., 2016, 188, 1–8 CrossRef CAS PubMed.
- K. Zhang, C. Qiang and J. Liu, J. Soils Sediments, 2018, 18, 2044–2052 CrossRef CAS.
- W. Han, G. Gao, J. Geng, Y. Li and Y. Wang, Chemosphere, 2018, 197, 325–335 CrossRef CAS PubMed.
- A. Sungur, M. Soylak and H. Ozcan, Soil Sediment Contam., 2019, 28, 213–227 CrossRef CAS.
- J. Ai, N. Wang and J. Yang, Environ. Sci., 2014, 35, 3530–3536 Search PubMed.
- C. Li, M. Sun, C. Song, P. Tao, Y. Yin and M. Shao, J. Soil Contam., 2017, 26, 45–58 CrossRef CAS.
- Y. Zhou, C. Qiang, S. Deng, J. Wan, S. Zhang, T. Long, Q. Li, Y. Lin and Y. Wu, Environ. Sci., 2018, 39, 3884–3892 Search PubMed.
- Y. Wei, G. LI, Y. Wang, Q. Zhang, B. Li, S. Wang, J. Cui, H. Zhang and Q. Zhou, J. Agro-Environ. Sci., 2018, 37, 2549–2559 Search PubMed.
- I. Bilal, A. Abdugheni, Q. Shi, S. Liu, K. Nicati and H. Li, Trans. Chin. Soc. Agric. Eng., 2019, 35, 185–192 Search PubMed.
- Z. Chen, Y. Hua and W. Xu, J. Environ. Sci., 2020, 40, 276–283 Search PubMed.
- B. Yang, L. Zhou and N. Xue, Sci. Total Environ., 2013, 43, 31–37 CrossRef PubMed.
- M. Boroumandi, M. Khamehchiyan and M. Reza, Geopersia, 2019, 9, 293–304 Search PubMed.
- Z. Li, Y. Qian and M. Wang, Environ. Eng., 2014, 32, 831–835 Search PubMed.
- I. U. Rehman, M. Ishaq, L. Ali, S. Khan, I. Ahmad, I. U. Din and H. Ullah, Ecotoxicol. Environ. Saf., 2018, 154, 127–136 CrossRef PubMed.
- Y. Huang, Y. Teng, N. Zhang, Z. Fu and W. Ren, Hum. Ecol. Risk Assess., 2018, 24, 1312–1326 CrossRef CAS.
- G. Y. Hadzi, G. A. Ayoko, D. K. Essumang and S. K. D. Osae, Environ. Geochem. Health, 2019, 41, 1–23 CrossRef PubMed.
- P. Lan, D. Wan and F. Dong, Hunan Agric. Sci., 2019, 4, 59–63 Search PubMed.
- H. Wu, Y. Yang, H. Li, Q. Li, F. Zhang and Y. Ba, Int. J. Environ. Health Res., 2020, 30, 174–186 CrossRef CAS PubMed.
- T. Xia, L. Jiang, X. Jia, M. Zhong and J. Liang, Front. Environ. Sci. Eng., 2014, 8, 441–450 CrossRef CAS.
- E. D. Bruce, A. A. Abusalih, T. J. Mcdonald and R. L. Autenrieth, J. Environ. Sci. Health, Part A: Toxic/Hazard. Subst. Environ. Eng., 2007, 42, 697–706 CrossRef CAS PubMed.
- X. Peng, G. Shi, G. Liu, J. Xu, Y. Tian, Y. Zhang, Y. Feng and A. G. Russell, Environ. Pollut., 2016, 221, 335–342 CrossRef PubMed.
- J. Liu, Y. Liu, Y. Liu, Z. Liu and A. Zhang, Ecotoxicol. Environ. Saf., 2018, 164, 261–269 CrossRef CAS PubMed.
- P. Guo, Y. Lei, Q. Zhou, C. Wang and J. Pan, Environ. Sci., 2015, 36, 3447–3456 CAS.
- L. Cao, T. Zhang and J. Fu, J. North Univ. China, Nat. Sci. Ed., 2017, 38, 209–216 Search PubMed.
- Q. Li, F. Guo, C. Mo, X. Zhao, R. Zhang and H. Liao, Ecological Science, 2013, 32, 235–240 Search PubMed.
- F. Li, X. Li, P. Wu, L. Chen, B. Gou and Z. Qi, Soils, 2009, 41, 49–53 CAS.
- P. Paatero and U. Tapper, Chemom. Intell. Lab. Syst., 1993, 18, 183–194 CrossRef CAS.
- W. Ge, Q. Cheng, C. Chai, L. Zeng, J. Wu, Q. Chen, X. Zhu and D. Ma, Environ. Sci., 2017, 38, 1587–1596 Search PubMed.
- M. E. E. PRC, Technical guidelines for risk assessment of soil contamination of land for construction, China Environment Publishing Group, 2019 Search PubMed.
- X. Zhao and X. Duan, Exposure factors handbook of Chinese population, China environment press., 2014 Search PubMed.
- P. Qi, C. Qu, S. Albanese, A. Lima, D. Cicchella, D. Hope, P. Cerino, A. Pizzolante, H. Zheng, J. Li and B. D. Vivo, J. Hazard. Mater., 2020, 383, 1–10 CrossRef PubMed.
- Q. Li, Q. Yao, X. Huang, Y. Zhang, X. Liu, W. Song and X. Wang, J. Southwest Univ., Nat. Sci. Ed., 2019, 41, 120–129 Search PubMed.
- W. Qi and J. Cao, Soils Bull., 1991, 5, 19–20 Search PubMed.
- Y. Zhang, J. Wang and H. Zhang, J. Guizhou Norm. Univ, 2011, 29, 20–25 Search PubMed.
- Q. Chen, S. Xu, J. Chen and Z. Wu, Environ. Sci. Technol., 2014, 27, 1–4 Search PubMed.
- H. Cheng, K. Li, M. Li, K. Yang, F. Liu and X. Cheng, Earth Sci. Front., 2014, 21, 265–306 CAS.
- Q. Lin and J. He, Environ. Sci. Technol., 1998, 4, 23–31 Search PubMed.
- D. Lv, H. Wang, Y. Pan and L. Wang, J. Nat. Sci. Hunan Normal Univ., 2010, 1, 77–81 Search PubMed.
- T. Zhan, Y. Huang, Y. Teng, T. He, W. Shi, C. Hou, Y. Luo and Q. Zhao, Chin. J. Soil Sci., 2017, 48, 474–480 Search PubMed.
- X. Zhao, L. Li, H. Yang, H. Yang and S. Tan, Acta Geosci. Sin., 2012, 33, 331–340 CAS.
- F. Chen, Z. Dong, C. Wang, X. Wei, Y. Hu and L. Zhang, Environ. Sci., 2017, 38, 4360–4369 Search PubMed.
- Y. Mamat, P. Adil and A. Sidik, Chinese Agricultural Science Bulletin, 2017, 33, 64–69 Search PubMed.
- G. Hu, L. Zhang, J. Qi, J. Yang, Y. Yu, H. Zheng, F. Chen, M. Chen, C. Wang and H. Li, J. Ecol. Environ. Sci., 2015, 24, 879–885 Search PubMed.
- D. Yin, Soil, 1988, 3, 31–33 Search PubMed.
- Y. Xu, J. Zhang and H. Ke, Geol. Bull. China, 2014, 33, 1097–1105 CAS.
- F. Cheng, J. Cheng, H. Sang, J. Yu, L. Xi and S. Pi, Environ. Sci., 2013, 34, 1062–1066 Search PubMed.
- G. Guo, F. Wu, F. Xie and R. Zhang, J. Environ. Sci., 2012, 24, 410–418 CrossRef CAS.
- T. Gou and Y. Ruan, Environ. Prot. Chem. Ind., 2019, 1–7 Search PubMed.
- Y. Chen, L. Weng, J. Ma, X. Wu and Y. Li, J. Agro-Environ. Sci., 2019, 38, 2219–2238 Search PubMed.
- Y. Wang, Y. Bai and J. Wang, Environ. Sci., 2014, 35, 2714–2720 Search PubMed.
- S. Liu, Q. Wu, X. Cao, J. Wang, L. Zhang, D. Cai, L. Zhou and N. Liu, Environ. Sci., 2016, 37, 270–279 Search PubMed.
- W. Luan, Z. Song, X. Cui, S. Li, W. Wang, X. Wen and W. Li, Chin. J. Soil Sci., 2010, 5, 152–156 Search PubMed.
- Y. Wu, Y. Jia, M. Fang, H. Liu, Z. Mi, X. Wang, W. Lin and X. Tong, Shanghai Chem. Ind., 2012, 11, 31–36 Search PubMed.
- T. Xie, C. Hu, Y. Chen, Q. Xu and G. Wan, J. Agric. Resour. Environ., 2017, 35, 155–160 Search PubMed.
Footnote |
† Electronic supplementary information (ESI) available. See DOI: 10.1039/d0ra01004a |
|
This journal is © The Royal Society of Chemistry 2020 |
Click here to see how this site uses Cookies. View our privacy policy here.