DOI:
10.1039/C9RA09583G
(Paper)
RSC Adv., 2020,
10, 7732-7739
Effective estimation of the inhibitor affinity of HIV-1 protease via a modified LIE approach†
Received
17th November 2019
, Accepted 6th February 2020
First published on 21st February 2020
Abstract
The inhibition of the Human Immunodeficiency Virus Type 1 Protease (HIV-1 PR) can prevent the synthesis of new viruses. Computer-aided drug design (CADD) would enhance the discovery of new therapies, through which the estimation of ligand-binding affinity is critical to predict the most efficient inhibitor. A time-consuming binding free energy method would reduce the usefulness of CADD. The modified linear interaction energy (LIE) approach emerges as an appropriate protocol that performs this task. In particular, the polar interaction free energy, which is obtained via numerically resolving the linear Poisson–Boltzmann equation, plays as an important role in driving the binding mechanism of the HIV-1 PR + inhibitor complex. The electrostatic interaction energy contributes to the attraction between two molecules, but the vdW interaction acts as a repulsive factor between the ligand and the HIV-1 PR. Moreover, the ligands were found to adopt a very strong hydrophobic interaction with the HIV-1 PR. Furthermore, the results obtained corroborate the high accuracy and precision of computational studies with a large correlation coefficient value R = 0.83 and a small RMSE δRMSE = 1.25 kcal mol−1. This method is less time-consuming than the other end-point methods, such as the molecular mechanics Poisson–Boltzmann surface area (MM/PBSA) and free energy perturbation (FEP) approaches. Overall, the modified LIE approach would provide ligand-binding affinity with HIV-1 PR accurately, precisely, and rapidly, resulting in a more efficient design of new inhibitors.
Introduction
The human immunodeficiency virus (HIV) causes acquired immunodeficiency syndrome (AIDS), which leads to the death of several million people worldwide.1 Although new patients have been reduced by around ∼40% compared to the peak in 1997, still ∼1.7 million new people have been infected in 2018.1 Moreover, globally, nearly 40 million people are currently living with HIV/AIDS, but only ∼23 million patients have access to antiviral drugs.1 The HIV/AIDS epidemic is still one of the most important global health issues. Therefore, the search for a new therapy to prevent HIV continuously attracts a large number of scientists. The scientists have tried to inhibit HIV by preventing viral reproduction through the use of fusion,2 reverse transcriptases,3 integrases,4 and protease inhibitors.5 Recently, the contemporary use of multiple drugs, which target several different HIV targets, are often employed to enhance the treatment efficiency, resulting in the decrease of patient mortality. This method is known as highly active antiretroviral therapy (HAART).6 Unfortunately, several resistance issues and unexpected side effects have been recorded over the treatment via HAART.7 Designing new inhibitors for HIV targets is still urgent.
The HIV-1 PR enzyme plays an important role in the synthesis of new viruses since it contributes to the cleavage of polyproteins to generate mature components of the HIV virion.8 Thus, the inhibition of HIV-1 PR is able to stop virus replication. Severally successful inhibitors have been designed for inhibiting HIV-1 PR and many of them were approved for HIV treatment, such as Amprenavir, Indinavir, Lopinavir, Nelfinavir, Ritonavir, and Saquinavir.9 HIV-1 PR inhibitors are currently used alongside reverse transcriptase inhibitors, resulting in an improvement in HIV-1 therapy. However, available HIV-1 PR inhibitors still have many side effects and their efficiency decrease with mutant viral strains.10,11 Thus, the search of a potential candidate to efficiently inhibit HIV-1 PR still attracts numerous efforts.
Computer-aided drug design (CADD) is a very useful method to shorten the development of new therapy.12 Several approaches have been established to determine the free energy difference of binding between the ligand and the receptor such as non-equilibrium molecular dynamics (NEMD),13,14 free energy perturbation (FEP),15,16 thermodynamic integration (TI),17,18 molecular mechanic/Poisson–Boltzmann surface area (MM/PBSA),19–21 linear interaction energy (LIE),22–25 fast pulling of ligand (FPL),26,27 biased sampling (US),28–30 deep learning,31 molecular docking,32,33 and quantitative structure–activity relationship (QSAR) approaches.34–36 Moreover, it is known that the accuracy of a ligand-binding affinity determination method is normally inversely proportional to the computational cost. In general, a computed method is employed on the number of ligands to analyse. In particular, the screening of million trial ligands is often performed using rapid and low accuracy approaches such as docking and QSAR methods. The testing binding affinity of hundred inhibitors is frequently carried out via an approximate method, which keeps blank between performance and accuracy, as known as MM/PBSA, LIE, FPL, and the US approaches. Time-consuming protocols including NEMD, FEP, and TI usually give results in agreement with experiments. These schemes are usually used to refine the results of an approximate method. Furthermore, the implementation of temperature/Hamiltonian replica exchange molecular dynamics simulations of the free energy perturbation method can present higher accuracy and precision results.37–39
Approximate methods are often employed to resolve the CADD problem. In particular, the MM/PBSA and LIE end-point approaches are very popular methods. In the MM/PBSA method, binding free energy is evaluated via a combination of molecular mechanics and continuum solvent.40–42 However, the accuracy of the MM/PBSA method depends on several factors that involve a continuum solvation approach, dielectric constant, and entropic determinations.43–45 Moreover, when the considered system is larger than 2000 atoms, the entropic estimation via normal mode analysis would cost a large CPU time. Therefore, the entropic calculation is usually carried out in the last snapshot of MD simulations rather than the whole conformation over the equilibrium interval.43–45 Thus, the statistical significance is unguaranteed. The selection of the dielectric constant also causes issues, resulting in a significant decrease of the obtained accuracy and precision.43–45 Overall, although the MM/PBSA method is able to estimate reasonable values of ligand-binding affinity, the absolute values do not correlate with the experiments.46–48
In addition, another end-point free energy estimation approach, LIE, gives successful results to different systems.49–56 In this approach, the binding free energy difference is computed based on the average of electrostatic and van der Waals (vdW) interaction energy differences of the inhibitor with its neighbouring atoms in various states involving the inhibitor in a solvated complex (bound state – noted as subscript b) and inhibitor in solvation (free state – noted as subscript f). In this context, we have computed the binding free energies of 33 inhibitors interacting with HIV-1 PR by using conventional molecular dynamics (MD) simulations and the LIE approach. In particular, the continuum model was employed to enhance the accuracy of the conventional LIE approach. In the improved LIE method, the contribution of polar free energy was also considered as an important factor controlling the ligand-binding affinity. The scaling factors for electrostatic, vdW and polar terms were fitted considering the binding process of 22 systems and then re-evaluated in 11 systems. The obtained results probably provide an appropriate way to screen a large number of ligands with affinity to HIV-1 PR.
Materials and methods
Initial conformation and parameterization of HIV-1 PR complexes
The three-dimensional structures of 33 HIV-1 PR protease inhibitor complexes (cf. Fig. 1) are deposited in protein data bank with PDB ID: 1AJV,57 1AJX,57 1D4J,58 1EBW,58 1EBZ,58 1EC0,59 1EC2,58 1EC3,58 1G2K,60 1G35,60 1OHR,61 1D4H,58 1T3R,62 1W5X,59 1W5Y,59 1XL5,63 2AQU,64 2BPX,65 2CEJ,66 2CEM,66 2CEN,66 2I0D,67 2Q5K,68 2QI0,69 2UXZ,70 3H5B,71 3NU3,72 3O9G,73 3OXC,74 4DJQ,75 4DJR,75 4U7Q,76 and 4U7V.76 HIV-1 PR and ions were parameterized using the GROMOS96 43a1 force field77 referring the previous works.26,78 In particular, the protonation states of Asp25A and Asp25B are assigned according to previous works26,79–83 or predicted via Propka 2.0 (ref. 84) since it has large effects on the ligand-binding affinity estimation. Moreover, the SPC water model was employed to represent water molecules.85 The parameters of the ligands were generated via the PRODRG2 webserver,86 but the atomic charges were determined by using the restrained electrostatic potential (RESP) approach87 calculated by molecular orbital quantum chemical calculations using the Hartree–Fock (HF) functional with the 6-31G(d) basis set.
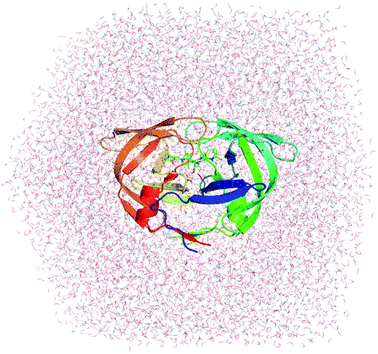 |
| Fig. 1 Initial structure used for MD simulations of Indinavir bound to HIV-1 PR (PDB ID: 2BPX). | |
Molecular dynamics (MD) simulations
GROMACS version 5.1.3 (ref. 88) was used to mimic the solvated complexes. In this solvated complex, the HIV-1 PR + inhibitor system was inserted into a periodic boundary dodecahedron box with a volume of ∼367 nm3 and consists of 1 HIV-1 PR molecule, 1 inhibitor, ∼10
880 water molecules, and ∼4–5 Cl− ions to neutralize the system (a total of ∼34
600 atoms). The ligand was put into a periodic boundary dodecahedron box with a volume of ∼74 nm3 and consists of 1 inhibitor molecule and 2400 water molecules (a total ∼7200 atoms). The simulation was carried out with parameters referring to the previous work,26,89 in which the non-bonded pair cut-off is 1.0 nm. The vdW cut-off is 1.0 nm and the PME method is used for electrostatic interactions. MD simulations were performed in turn according to the following steps: energy minimization, position restraint in NVT ensemble, equilibrate in NPT simulations, and production MD simulations. In particular, the energy minimization was carried out by using the steepest descent approach. NVT and NPT simulations were achieved with an MD length of 100 ps per simulation. During the NVT simulation, the complex was restrained via a small harmonic potential. MD simulations were completed in 20 and 5 ns, which correspond to the solvated complex and isolated ligand in solvation systems, respectively. MD simulations were performed 4 autonomous times to generate 4 independent trajectories with the same initial conformation but different generated velocities.
Data analysis
The root-mean-square-deviations (RMSDs) of all atoms in the starting conformation was determined. Hydrogen bonds (HB) are calculated based on the geometric determination. In particular, the distance between the acceptor (A) and the donor (D) is required to be less than 0.35 nm and the A–H–D angle should be larger than 135° (H is hydrogen). The vdW, electrostatic, and polar interactions were computed to construct estimation models for the LIE method (eqn (3)) via linear regression analysis. The MM/PBSA approach was also carried out to estimate the difference in binding free energy between HIV-1 PR and its inhibitors. The details of the calculations were mentioned in previous studies.90
Results and discussion
Structures and energies of complexes during MD simulations
The RMSD of all atoms in the solvated complex was evaluated to determine the equilibrium region (cf. Fig. S1 in ESI†), which was used to evaluate the free energy of interaction between the two molecules, HIV-1 PR and the inhibitor. The RMSD values were quickly enlarged during the first 2 ns of MD simulations and reached equilibrium circumstances after 5 ns of MD simulations, as shown in Fig. 2. RMSD values are smaller than 0.3 nm since the starting conformation is a native structure obtained through experiments. The solvated complex is rigidly stabilized during the last 10 ns of the MD simulations. The free energy estimation was calculated over the 10–20 ns interval of MD simulations. During this time, the protein–ligand interaction can be clarified with a two-dimensional protein–ligand interaction map using the last MD-generated conformations, as shown in Fig. S2 of ESI.† All maps mention that vdW interactions dominate over electrostatic interactions in all complexes. The RMSD of the solvated ligand system is rigidly similar to the complex. The energy calculation was carried out during the 2–5 ns interval of MD simulations.
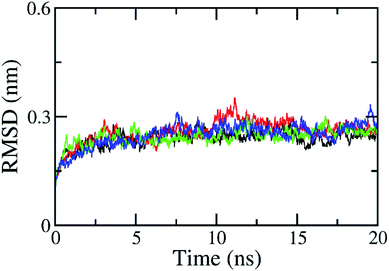 |
| Fig. 2 All-atom RMSD of HIV-1 PR + Indinavir (2BPX) over 4 independent MD trajectories with a length of 20 ns. The complex almost reaches equilibrium region after 5 ns. | |
In order to estimate the ligand-binding affinity to HIV-1 PR using the LIE approach, the difference of the mean electrostatic (ΔEcoub–f) and vdW (ΔEvdWb–f) interaction energy between the inhibitor and its neighbouring molecules in a solvated complex of HIV-1 PR (bound state) and in solvation (free state) were calculated over 4 independent MD trajectories. The magnitude of ΔEvdWb–f is significantly larger than ΔEcoub–f implying the domination of the hydrophobic interaction during the binding process of a ligand to HIV-1 PR, which is consistent with the previous works.27,78 Moreover, in the modified LIE approach, the influence of conformational entropy49 and solvent-accessible surface area (ΔGsur)50,91 have also been exercised. The entropic contribution was evaluated by a normal mode approximation. The difference in surface energy (ΔGsur) was determined by using the Shrake–Rupley method. Furthermore, the difference in the polar interaction energy (ΔGPB) was calculated as it is probably employed as a term in the LIE function. The ΔGPB was predicted by numerically resolving the linear Poisson–Boltzmann equation in a continuum solvent approximation. The results obtained are shown in Table 1. In particular, the average of ΔGsur, ΔGPB, and −TΔS values are computed as −8.93, 67.4, and 32.68 kcal mol−1, respectively, and are in good agreement with previous studies92–94 implying the validation of the calculated values.
Table 1 The free energy values obtained from experiments and MD simulationsa
Complexes |
ΔEcoub–f |
ΔEvdWb–f |
ΔGsur |
ΔGPB |
−TΔS |
ΔGEXP |
Experimental values were acquired using the formula ΔGEXP = RT ln Ki, where R is a gas constant, T is the absolute temperature, and Ki is the inhibition constant obtained from previous studies.58,59,62–76 The computational results were averaged over 4 independent trajectories. The unit of energy is kcal mol−1. |
1D4H |
28.20 |
−43.80 |
−9.54 |
80.18 |
34.73 |
−13.74 |
1T3R |
21.13 |
−34.10 |
−8.01 |
64.77 |
32.35 |
−14.91 |
1W5X |
37.64 |
−44.50 |
−9.63 |
79.57 |
37.07 |
−11.54 |
1W5Y |
23.52 |
−50.69 |
−9.71 |
84.82 |
34.80 |
−11.65 |
1XL5 |
23.72 |
−37.62 |
−8.99 |
58.82 |
30.73 |
−10.09 |
2AQU |
12.02 |
−35.68 |
−10.00 |
70.51 |
36.03 |
−12.79 |
2BPX |
−6.14 |
−32.15 |
−9.17 |
69.51 |
32.35 |
−12.90 |
2CEJ |
23.38 |
−36.10 |
−9.19 |
55.09 |
31.74 |
−11.83 |
2CEM |
16.61 |
−38.03 |
−9.15 |
61.60 |
35.32 |
−10.87 |
2CEN |
26.06 |
−44.79 |
−9.41 |
68.62 |
33.93 |
−11.39 |
2I0D |
24.06 |
−35.78 |
−8.92 |
81.24 |
33.01 |
−16.60 |
2Q5K |
6.24 |
−31.51 |
−8.97 |
65.86 |
30.65 |
−15.51 |
2QI0 |
16.38 |
−34.69 |
−8.17 |
60.51 |
28.75 |
−10.13 |
2UXZ |
33.49 |
−36.04 |
−9.60 |
49.54 |
33.58 |
−11.64 |
3H5B |
14.43 |
−32.45 |
−8.34 |
69.86 |
31.91 |
−13.73 |
3NU3 |
14.67 |
−30.94 |
−7.62 |
66.56 |
28.86 |
−13.48 |
3O9G |
6.20 |
−27.26 |
−8.48 |
75.99 |
30.45 |
−16.88 |
3OXC |
30.11 |
−36.22 |
−8.85 |
53.30 |
32.85 |
−11.54 |
4DJQ |
12.60 |
−28.06 |
−8.40 |
71.38 |
29.72 |
−14.16 |
4DJR |
24.18 |
−35.30 |
−8.31 |
77.05 |
29.54 |
−15.82 |
4U7Q |
29.57 |
−40.04 |
−10.18 |
74.49 |
41.44 |
−13.38 |
4U7V |
5.43 |
−31.10 |
−7.87 |
43.50 |
29.19 |
−7.54 |
Optimization of LIE equations
As mentioned above, in the original LIE theory, the difference in the binding free energy was estimated based on the difference of ΔEcoub–f and ΔEvdWb–f (eqn (1)) with the empirical coefficients of α = 0.180 and β ≈ 0.300–0.500, which are validated upon applied receptors such as P450CAM, potassium channel and aspartic proteases.95–99 Recent work on amyloid beta-peptide system confirmed that the coefficients are α = 0.288 and β = 0.049.89 The use of these coefficients to determine the binding free energy of HIV-1 PR + inhibitor produces a failure since the correlation coefficients are observed as R = 0.20, 0.14, and −0.43 corresponding to the set of empirical parameters of (0.180, 0.500), (0.180, 0.300), and (0.288, 0.049), respectively. Overall, the empirical coefficients are changed depending on the applied receptors. Moreover, although suitable empirical coefficients of the HIV-1 PR system represented with CHARMM force field100,101 (with α = 0.169 and β = 0.017)102 were used, an uncorrelated coefficient was observed between the experimental and computed values as R = −0.43.102 Furthermore, an improved LIE model (eqn (2)) involving surface free energy ΔGsur (with α = 0.014, β = 0.061, and γ = 0.042)103 was also considered to calculate the HIV-1 PR + inhibitor representative by the Amber99 force field.87,104 Unfortunately, the correlation coefficient between the computational and experimental values is R = 0.22. Therefore, the results obtained implied that the LIE model is also depended on the applied force field to represent the complex. |
ΔGLIE = αΔEvdWb–f + βΔEcoub–f
| (1) |
where ΔEvdWb–f = 〈VvdWl–s〉b − 〈VvdWl–s〉f and ΔEcoub–f = 〈Velecl–s〉b − 〈Velecl–s〉f are the difference of the mean electrostatic and vdW interaction energy between the inhibitor and its surrounding molecules in a solvated complex of the receptor (bound state) and in solvation (free state), respectively. |
ΔGLIE = αΔEvdWb–f + βΔEcoub–f + γΔGsur
| (2) |
where ΔGsur is the difference on the surface free energy, which was calculated by using the Shrake–Rupley method.
Because of the failure of the available empirical coefficients, an appropriate set of parameters was generated for HIV-1 PR + inhibitor represented by the GROMOS force field as follow:
|
ΔGLIE = αΔEvdWb–f + βΔEcoub–f + γΔGPB + δ
| (3) |
where Δ
GPB is the polar interaction free energy, which was estimated
via numerically resolving the linear Poisson–Boltzmann equation in continuum solvent approximation. In particular, the empirical coefficients
α,
β,
γ, and
δ are found to be of −0.92, −0.11, −0.47, and −23.06 kcal mol
−1, respectively (
eqn (3)), providing a correlation coefficient of
R = 0.84 and a root mean square error (RMSE) of 1.07 kcal mol
−1 (
Fig. 3) experimentally. In particular, the large negative value of
δ implies that the ligand adopts a very strong hydrophobic interaction with HIV-1 PR,
95,105 which is in good agreement with the two-dimensional protein–ligand maps (
cf. Fig. S2 in ESI
†). The negative value of
γ indicates that the polar interaction contributes to the attraction between HIV-1 PR and the inhibitor as well as the contribution of the electrostatic interaction (
β < 0). Consequently, the vdW interaction acts as a repulsive factor during the binding process since the parameter
α is smaller than zero.
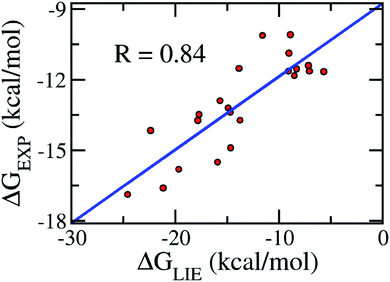 |
| Fig. 3 Correlation between experimental binding free energies and that calculated using the LIE model (eqn (3)) of the training set consisting of 22 complexes (Table 1). | |
Validation of the model
In order to confirm that our proposed coefficients including α, β, γ, and δ still work effectively with other systems, the approach was applied on the testing set consisting of 11 various inhibitors, which were taken randomly (Table 2). The mean values of free energy were calculated and are described in Table 2. The binding free energy between a ligand and HIV-1 PR was then evaluated according to the proposed model (eqn (3)). The results obtained suggested that the proposed LIE approach is rather robust. In particular, the correlation coefficient between the computed and experimental values is R = 0.83 (Fig. 4). The RMSE value was estimated as 1.25 kcal mol−1. Therefore, the accuracy and precision of the proposed model are appropriate to predict the ligand-binding affinity of a trail ligand to the HIV-1 PR system. Furthermore, the small RMSE means that the model can categorize different inhibitors that reveal similar binding free energies.
Table 2 The free energy values obtained from experiments and MD simulationsa
Complexes |
ΔEcoub–f |
ΔEvdWb–f |
ΔGsur |
ΔGPB |
−TΔS |
ΔGEXP |
The experimental values were acquired using the formula ΔGEXP = RT ln Ki, where R is a gas constant, T is the absolute temperature, and Ki is the inhibition constant obtained from previous studies.57–61 The simulated results were averaged over 4 independent trajectories. The unit of energy is kcal mol−1. |
1AJV |
13.49 |
−38.34 |
−8.42 |
50.09 |
26.77 |
−10.59 |
1AJX |
5.26 |
−29.40 |
−7.76 |
56.72 |
25.78 |
−10.86 |
1D4J |
17.70 |
−46.77 |
−9.72 |
79.69 |
35.78 |
−11.47 |
1EBW |
25.56 |
−33.65 |
−9.22 |
71.32 |
34.24 |
−12.42 |
1EBZ |
28.40 |
−42.67 |
−9.40 |
86.32 |
35.12 |
−12.90 |
1EC0 |
21.90 |
−45.44 |
−9.62 |
80.94 |
34.64 |
−11.66 |
1EC2 |
22.44 |
−36.36 |
−10.75 |
78.56 |
39.94 |
−13.73 |
1EC3 |
30.66 |
−42.82 |
−10.52 |
75.25 |
37.29 |
−12.40 |
1G2K |
14.40 |
−40.82 |
−9.14 |
58.10 |
30.38 |
−10.92 |
1G35 |
17.31 |
−42.16 |
−9.74 |
61.43 |
33.75 |
−11.17 |
1OHR |
11.94 |
−22.28 |
−7.93 |
45.88 |
27.87 |
−11.94 |
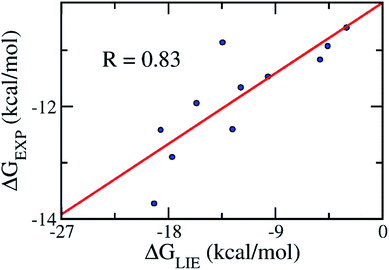 |
| Fig. 4 Correlation between the experimental binding free energies and that calculated using the LIE model (eqn (3)). The testing set consisted of 11 complexes (Table 2). | |
CPU time consumption
The estimation of ligand-binding affinity via the LIE scheme was indicated that it is required less computing resources than other end-point approaches such as MM-PBSA or FEP methods.89 In fact, in the LIE model, it was not necessary to perform additional computations to calculate the difference in the binding free energy alongside the production MD simulations. All of the free energy terms required to determine the binding free energy can be analyzed via the recorded conformations of the solvated complexes and the isolated ligands in the solution. Moreover, the estimation of the polar interaction energy caused the modified LIE to cost more CPU time than the original. However, since the HIV-1 PR + inhibitor is not very large, the numerical resolution of the Poisson–Boltzmann linear equation in continuum solvent approximation would not cost much CPU time. Furthermore, the applied united-atom GROMOS force field would require significantly less time-consuming CPU than the all-atom force field. In particular, one single calculation to predict the ligand-binding affinity to HIV-1 PR would consume ∼1 day for 4 independent trajectories using a single compute node with dual Xeon E5-2670 V3 with GPGPU acceleration.
Conclusions
Herein, a modified LIE approach has been successfully established to estimate the binding free energy of a ligand to the HIV-1 PR. The results obtained show a good correlation with the experimental data (R = 0.83) with a small RMSE (δRMSE = 1.25 kcal mol−1). We have found that the available LIE empirical coefficients are not able to adopt good correlation with the experimental values, especially the united-atom force field model employed to represent the systems.
Polar binding energy emerges as an important factor to control the accuracy in the LIE model. The electrostatic interaction energy contributes to the attraction between two molecules, but the vdW interaction acts as a repulsive factor between the ligand and HIV-1 PR. Furthermore, it was found that the ligands adopted a very strong hydrophobic interaction with the HIV-1 PR.
The CPU time consumption of the modified LIE model is larger than the original, since it spends computing resources to resolve the linear Poisson–Boltzmann equation to estimate the polar interaction free energy. However, the computing resource cost would be significantly lower than that required for the MM-PBSA or FEP methods.89
Obtaining an efficient scheme for rapidly and accurately estimating the ligand-binding affinity to the HIV-1 PR system is of great attraction. The proposed LIE model would enhance the development of HIV therapy.
Conflicts of interest
There are no conflicts to declare.
Acknowledgements
This research is funded by the Department of Science and Technology of Ho Chi Minh City under grant number 199/2016/HD-SKHCN.
References
- UNAIDS, Global HIV & AIDS statistics – 2019 fact sheet, 2019, https://www.unaids.org/en/resources/fact-sheet Search PubMed.
- H. Chong, J. Xue, Y. Zhu, Z. Cong, T. Chen, Y. Guo, Q. Wei, Y. Zhou, C. Qin and Y. He, J. Virol., 2018, 92, e00775-18 CrossRef PubMed.
- L. Tian, M.-S. Kim, H. Li, J. Wang and W. Yang, Proc. Natl. Acad. Sci. U. S. A., 2018, 115, 507 CrossRef CAS PubMed.
- S. J. Smith, X. Z. Zhao, T. R. Burke and S. H. Hughes, Antimicrob. Agents Chemother., 2018, 62, e01035-18 CrossRef PubMed.
- A. D. Badley, Cell Death Differ., 2005, 12, 924–931 CrossRef CAS PubMed.
- J. R. Brechtl, W. Breitbart, M. Galietta, S. Krivo and B. Rosenfeld, J. Pain Symptom Manage., 2001, 21, 41–51 CrossRef CAS.
- R. D. Moore and R. E. Chaisson, AIDS, 1999, 13, 1933–1942 CrossRef CAS PubMed.
- D. E. Clercq, Nat. Rev. Drug Discovery, 2007, 6, 1001–1018 CrossRef PubMed.
- D. E. Clercq, Int. J. Antimicrob. Agents, 2009, 33, 307–320 CrossRef PubMed.
- F. Clavel and A. J. Hance, N. Engl. J. Med., 2004, 350, 1023–1035 CrossRef CAS PubMed.
- D. D. Richman, Nature, 2001, 410, 995–1001 CrossRef CAS PubMed.
- G. R. Marshall, Annu. Rev. Pharmacol. Toxicol., 1987, 27, 193–213 CrossRef CAS PubMed.
- C. Jarzynski, Phys. Rev. Lett., 1997, 78, 2690–2693 CrossRef CAS.
- C. Jarzynski, Phys. Rev. E: Stat. Phys., Plasmas, Fluids, Relat. Interdiscip. Top., 1997, 56, 5018–5035 CrossRef CAS.
- R. W. Zwanzig, J. Chem. Phys., 1954, 22, 1420–1426 CrossRef CAS.
- D. L. Beveridge and F. M. DiCapua, Annu. Rev. Biophys. Biophys. Chem., 1989, 18, 431–492 CrossRef CAS PubMed.
- J. G. Kirkwood, J. Chem. Phys., 1935, 3, 300–313 CrossRef CAS.
- P. Kollman, Chem. Rev., 1993, 93, 2395–2417 CrossRef CAS.
- P. A. Kollman, I. Massova, C. Reyes, B. Kuhn, S. Huo, L. Chong, M. Lee, T. Lee, Y. Duan, W. Wang, O. Donini, P. Cieplak, J. Srinivasan, D. A. Case and T. E. Cheatham, Acc. Chem. Res., 2000, 33, 889–897 CrossRef CAS PubMed.
- B. Kuhn and P. A. Kollman, J. Med. Chem., 2000, 43, 3786–3791 CrossRef CAS PubMed.
- W. Wang and P. A. Kollman, Proc. Natl. Acad. Sci. U. S. A., 2001, 98, 14937–14942 CrossRef CAS PubMed.
- J. Åqvist, C. Medina and J.-E. Samuelsson, Protein Eng., 1994, 7, 385–391 CrossRef PubMed.
- J. Aqvist and J. Marelius, Comb. Chem. High Throughput Screening, 2001, 4, 613–626 CrossRef CAS PubMed.
- J. Åqvist, V. B. Luzhkov and B. O. Brandsdal, Acc. Chem. Res., 2002, 35, 358–365 CrossRef PubMed.
- H. L. N. d. Amorim, R. A. Caceres and P. A. Netz, Curr. Drug Targets, 2008, 9, 1100–1105 CrossRef PubMed.
- S. T. Ngo, H. M. Hung and M. T. Nguyen, J. Comput. Chem., 2016, 37, 2734–2742 CrossRef CAS PubMed.
- S. T. Ngo, M. T. Nguyen and M. T. Nguyen, Chem. Phys. Lett., 2017, 676, 12–17 CrossRef CAS.
- S. T. Ngo, K. B. Vu, L. M. Bui and V. V. Vu, ACS Omega, 2019, 4, 3887–3893 CrossRef CAS PubMed.
- M. Huang, W. Huang, F. Wen and G. Larson Ronald, J. Comput. Chem., 2017, 38, 2007–2019 CrossRef CAS.
- N. T. Lan, K. B. Vu, M. K. Dao Ngoc, P.-T. Tran, D. M. Hiep, N. T. Tung and S. T. Ngo, J. Mol. Graphics Modell., 2019, 93, 107441 CrossRef PubMed.
- G. Subramanian, B. Ramsundar, V. Pande and R. A. Denny, J. Chem. Inf. Model., 2016, 56, 1936–1949 CrossRef CAS PubMed.
- P. Ferrara, H. Gohlke, D. J. Price, G. Klebe and C. L. Brooks, J. Med. Chem., 2004, 47, 3032–3047 CrossRef CAS PubMed.
- M. Stahl and M. Rarey, J. Med. Chem., 2001, 44, 1035–1042 CrossRef CAS PubMed.
- H. H. Maw and L. H. Hall, J. Chem. Inf. Comput. Sci., 2002, 42, 290–298 CrossRef CAS PubMed.
- R. Gil-Redondo, J. Klett, F. Gago and A. Morreale, Proteins, 2010, 78, 162–172 CrossRef CAS PubMed.
- C. Coderch, Y. Tang, J. Klett, S.-E. Zhang, Y.-T. Ma, W. Shaorong, R. Matesanz, B. Pera, A. Canales, J. Jimenez-Barbero, A. Morreale, J. F. Diaz, W.-S. Fang and F. Gago, Org. Biomol. Chem., 2013, 11, 3046–3056 RSC.
- W. Jiang and B. Roux, J. Chem. Theory Comput., 2010, 6, 2559–2565 CrossRef CAS PubMed.
- Y. Meng, D. Sabri Dashti and A. E. Roitberg, J. Chem. Theory Comput., 2011, 7, 2721–2727 CrossRef CAS.
- W. Jiang, J. Thirman, S. Jo and B. Roux, J. Phys. Chem. B, 2018, 122, 9435–9442 CrossRef CAS.
- J. Wang, P. Morin, W. Wang and P. A. Kollman, J. Am. Chem. Soc., 2001, 123, 5221–5230 CrossRef CAS PubMed.
- B. Kuhn, P. Gerber, T. Schulz-Gasch and M. Stahl, J. Med. Chem., 2005, 48, 4040–4048 CrossRef CAS PubMed.
- R. Giulio, R. A. Del, D. Gianluca and S. Miriam, J. Comput. Chem., 2010, 31, 797–810 Search PubMed.
- T. Hou, J. Wang, Y. Li and W. Wang, J. Chem. Inf. Model., 2011, 51, 69–82 CrossRef CAS PubMed.
- L. Xu, H. Sun, Y. Li, J. Wang and T. Hou, J. Phys. Chem. B, 2013, 117, 8408–8421 CrossRef CAS PubMed.
- S. Genheden and U. Ryde, Expert Opin. Drug Discovery, 2015, 10, 449–461 CrossRef CAS PubMed.
- S. T. Ngo and M. S. Li, J. Phys. Chem. B, 2012, 116, 10165–10175 CrossRef CAS PubMed.
- C. Koukoulitsa, C. Villalonga-Barber, R. Csonka, X. Alexi, G. Leonis, D. Dellis, E. Hamelink, O. Belda, B. R. Steele, M. Micha-Screttas, M. N. Alexis, M. G. Papadopoulos and T. Mavromoustakos, J. Enzyme Inhib. Med. Chem., 2016, 31, 67–77 CrossRef CAS PubMed.
- S. Chakraborty and P. Das, Sci. Rep., 2017, 7, 9941 CrossRef PubMed.
- J. Wang, R. Dixon and P. A. Kollman, Proteins, 1999, 34, 69–81 CrossRef CAS.
- D. K. Jones-Hertzog and W. L. Jorgensen, J. Med. Chem., 1997, 40, 1539–1549 CrossRef CAS PubMed.
- I. D. Wall, A. R. Leach, D. W. Salt, M. G. Ford and J. W. Essex, J. Med. Chem., 1999, 42, 5142–5152 CrossRef CAS PubMed.
- M. S. Kumar, S. Johan, Å. Johan and K. Jaroslav, J. Comput. Chem., 2012, 33, 2340–2350 CrossRef PubMed.
- U. Uciechowska, J. Schemies, M. Scharfe, M. Lawson, K. Wichapong, M. Jung and W. Sippl, RSC Med. Chem., 2012, 3, 167–173 RSC.
- V. Durmaz, S. Schmidt, P. Sabri, C. Piechotta and M. Weber, J. Chem. Inf. Model., 2013, 53, 2681–2688 CrossRef CAS PubMed.
- V. Poongavanam and J. Kongsted, J. Mol. Graphics Modell., 2016, 70, 236–245 CrossRef CAS PubMed.
- M. van Dijk, A. M. ter Laak, J. D. Wichard, L. Capoferri, N. P. E. Vermeulen and D. P. Geerke, J. Chem. Inf. Model., 2017, 57, 2294–2308 CrossRef CAS PubMed.
- K. Bäckbro, S. Löwgren, K. Österlund, J. Atepo, T. Unge, J. Hultén, N. M. Bonham, W. Schaal, A. Karlén and A. Hallberg, J. Med. Chem., 1997, 40, 898–902 CrossRef PubMed.
- H. O. Andersson, K. Fridborg, S. Löwgren, M. Alterman, A. Mühlman, M. Björsne, N. Garg, I. Kvarnström, W. Schaal, B. Classon, A. Karlén, U. H. Danielsson, G. Ahlsén, U. Nillroth, L. Vrang, B. Öberg, B. Samuelsson, A. Hallberg and T. Unge, Eur. J. Biochem., 2003, 270, 1746–1758 CrossRef CAS PubMed.
- J. Lindberg, D. Pyring, S. Löwgren, Å. Rosenquist, G. Zuccarello, I. Kvarnström, H. Zhang, L. Vrang, B. Classon, A. Hallberg, B. Samuelsson and T. Unge, Eur. J. Biochem., 2004, 271, 4594–4602 CrossRef CAS PubMed.
- W. Schaal, A. Karlsson, G. Ahlsén, J. Lindberg, H. O. Andersson, U. H. Danielson, B. Classon, T. Unge, B. Samuelsson, J. Hultén, A. Hallberg and A. Karlén, J. Med. Chem., 2001, 44, 155–169 CrossRef CAS PubMed.
- S. W. Kaldor, V. J. Kalish, J. F. Davies, B. V. Shetty, J. E. Fritz, K. Appelt, J. A. Burgess, K. M. Campanale, N. Y. Chirgadze, D. K. Clawson, B. A. Dressman, S. D. Hatch, D. A. Khalil, M. B. Kosa, P. P. Lubbehusen, M. A. Muesing, A. K. Patick, S. H. Reich, K. S. Su and J. H. Tatlock, J. Med. Chem., 1997, 40, 3979–3985 CrossRef CAS PubMed.
- D. L. Surleraux, A. Tahri, W. G. Verschueren, G. M. Pille, H. A. de Kock, T. H. Jonckers, A. Peeters, S. De Meyer, H. Azijn, R. Pauwels, M. P. de Bethune, N. M. King, M. Prabu-Jeyabalan, C. A. Schiffer and P. B. Wigerinck, J. Med. Chem., 2005, 48, 1813–1822 CrossRef CAS PubMed.
- E. Specker, J. Böttcher, H. Lilie, A. Heine, A. Schoop, G. Müller, N. Griebenow and G. Klebe, Angew. Chem., Int. Ed., 2005, 44, 3140–3144 CrossRef CAS PubMed.
- J. C. Clemente, R. M. Coman, M. M. Thiaville, L. K. Janka, J. A. Jeung, S. Nukoolkarn, L. Govindasamy, M. Agbandje-McKenna, R. McKenna, W. Leelamanit, M. M. Goodenow and B. M. Dunn, Biochemistry, 2006, 45, 5468–5477 CrossRef CAS PubMed.
- S. Munshi, Z. Chen, Y. Li, D. B. Olsen, M. E. Fraley, R. W. Hungate and L. C. Kuo, Acta Crystallogr., Sect. D: Biol. Crystallogr., 1998, 55, 1053–1060 CrossRef PubMed.
- J. K. Ekegren, N. Ginman, Å. Johansson, H. Wallberg, M. Larhed, B. Samuelsson, T. Unge and A. Hallberg, J. Med. Chem., 2006, 49, 1828–1832 CrossRef CAS PubMed.
- A. Ali, G. S. K. K. Reddy, H. Cao, S. G. Anjum, M. N. L. Nalam, C. A. Schiffer and T. M. Rana, J. Med. Chem., 2006, 49, 7342–7356 CrossRef CAS PubMed.
- G. S. K. K. Reddy, A. Ali, M. N. L. Nalam, S. G. Anjum, H. Cao, R. S. Nathans, C. A. Schiffer and T. M. Rana, J. Med. Chem., 2007, 50, 4316–4328 CrossRef CAS PubMed.
- M. D. Altman, A. Ali, G. S. K. Kumar Reddy, M. N. L. Nalam, S. G. Anjum, H. Cao, S. Chellappan, V. Kairys, M. X. Fernandes, M. K. Gilson, C. A. Schiffer, T. M. Rana and B. Tidor, J. Am. Chem. Soc., 2008, 130, 6099–6113 CrossRef CAS PubMed.
- X. Wu, P. Öhrngren, J. K. Ekegren, J. Unge, T. Unge, H. Wallberg, B. Samuelsson, A. Hallberg and M. Larhed, J. Med. Chem., 2008, 51, 2586 CrossRef CAS.
- A. K. Ghosh, S. Leshchenko-Yashchuk, D. D. Anderson, A. Baldridge, M. Noetzel, H. B. Miller, Y. Tie, Y.-F. Wang, Y. Koh, I. T. Weber and H. Mitsuya, J. Med. Chem., 2009, 52, 3902–3914 CrossRef CAS PubMed.
- C.-H. Shen, Y.-F. Wang, A. Y. Kovalevsky, R. W. Harrison and I. T. Weber, FEBS J., 2010, 277, 3699–3714 CrossRef CAS PubMed.
- M. N. L. Nalam, A. Ali, G. S. K. K. Reddy, H. Cao, S. G. Anjum, M. D. Altman, N. K. Yilmaz, B. Tidor, T. M. Rana and C. A. Schiffer, Chem. Biol., 2013, 20, 1116–1124 CrossRef CAS PubMed.
- Y. Tie, A. Y. Kovalevsky, P. Boross, Y.-F. Wang, A. K. Ghosh, J. Tozser, R. W. Harrison and I. T. Weber, Proteins, 2007, 67, 232–242 CrossRef CAS PubMed.
- M. K. Parai, D. J. Huggins, H. Cao, M. N. L. Nalam, A. Ali, C. A. Schiffer, B. Tidor and T. M. Rana, J. Med. Chem., 2012, 55, 6328–6341 CrossRef CAS PubMed.
- J. Schimer, M. Pávová, M. Anders, P. Pachl, P. Šácha, P. Cígler, J. Weber, P. Majer, P. Řezáčová, H.-G. Kräusslich, B. Müller and J. Konvalinka, Nat. Commun., 2015, 6, 6461 CrossRef CAS PubMed.
- W. F. van Gunsteren, S. R. Billeter, A. A. Eising, P. H. Hunenberger, P. Kruger, A. E. Mark, W. R. P. Scott and I. G. Tironi, Biomolecular Simulation: The GROMOS96 Manual and User Guide, Vdf Hochschulverlag AG an der ETH, Zurich, 1996 Search PubMed.
- S. T. Ngo, B. K. Mai, D. M. Hiep and M. S. Li, Chem. Biol. Drug Des., 2015, 86, 546–558 CrossRef CAS PubMed.
- O. Aruksakunwong, P. Wolschann, S. Hannongbua and P. Sompornpisut, J. Chem. Inf. Model., 2006, 46, 2085–2092 CrossRef CAS PubMed.
- T. D. McGee, J. Edwards and A. E. Roitberg, J. Phys. Chem. B, 2014, 118, 12577–12585 CrossRef CAS PubMed.
- L. J. Hyland, T. A. Tomaszek and T. D. Meek, Biochemistry, 1991, 30, 8454–8463 CrossRef CAS PubMed.
- F. Pietrucci, F. Marinelli, P. Carloni and A. Laio, J. Am. Chem. Soc., 2009, 131, 11811–11818 CrossRef CAS PubMed.
- T. Hou, W. A. McLaughlin and W. Wang, Proteins, 2008, 71, 1163–1174 CrossRef CAS PubMed.
- M. Petrek, M. Otyepka, P. Banas, P. Kosinova, J. Koca and J. Damborsky, BMC Bioinf., 2006, 7, 316 CrossRef PubMed.
- H. J. C. Berendsen, J. P. M. Postma, W. F. van Gunsteren and A. J. Hermans, Intermolecular Forces, Reidel, Dordrecht, Jerusalem, Israel, 1981 Search PubMed.
- A. W. Schuttelkopf and D. M. F. van Aalten, Acta Crystallogr., Sect. D: Biol. Crystallogr., 2004, 60, 1355–1363 CrossRef PubMed.
- C. I. Bayly, P. Cieplak, W. Cornell and P. A. Kollman, J. Phys. Chem., 1993, 97, 10269–10280 CrossRef CAS.
- M. J. Abraham, T. Murtola, R. Schulz, S. Páll, J. C. Smith, B. Hess and E. Lindahl, SoftwareX, 2015, 1–2, 19–25 CrossRef.
- S. T. Ngo, B. K. Mai, P. Derreumaux and V. V. Vu, RSC Adv., 2019, 9, 12455–12461 RSC.
- S. T. Ngo, H. M. Hung, D. T. Truong and M. T. Nguyen, Phys. Chem. Chem. Phys., 2017, 19, 1909–1919 RSC.
- H. A. Carlson and W. L. Jorgensen, J. Phys. Chem., 1995, 99, 10667–10673 CrossRef CAS.
- H. Tzoupis, G. Leonis, A. Avramopoulos, T. Mavromoustakos and M. G. Papadopoulos, J. Phys. Chem. B, 2014, 118, 9538–9552 CrossRef CAS PubMed.
- J. Chen, Z. Liang, W. Wang, C. Yi, S. Zhang and Q. Zhang, Sci. Rep., 2014, 4, 6872 CrossRef CAS PubMed.
- K. R. Karnati and Y. Wang, J. Mol. Graphics Modell., 2019, 92, 112–122 CrossRef CAS PubMed.
- M. Almlöf, B. O. Brandsdal and J. Åqvist, J. Comput. Chem., 2004, 25, 1242–1254 CrossRef PubMed.
- F. Österberg and J. Åqvist, FEBS Lett., 2005, 579, 2939–2944 CrossRef PubMed.
- S. Bjelic, M. Nervall, H. Gutiérrez-de-Terán, K. Ersmark, A. Hallberg and J. Åqvist, Cell. Mol. Life Sci., 2007, 64, 2285–2305 CrossRef CAS PubMed.
- T. Hansson, J. Marelius and J. Åqvist, J. Comput.-Aided Mol. Des., 1998, 12, 27–35 CrossRef CAS PubMed.
- M. Almlöf, J. Carlsson and J. Åqvist, J. Chem. Theory Comput., 2007, 3, 2162–2175 CrossRef PubMed.
- A. D. MacKerell, N. Banavali and N. Foloppe, Biopolym, 2000, 56, 257–265 CrossRef CAS PubMed.
- K. Vanommeslaeghe, E. Hatcher, C. Acharya, S. Kundu, S. Zhong, J. Shim, E. Darian, O. Guvench, P. Lopes, I. Vorobyov and A. D. Mackerell Jr, J. Comput. Chem., 2010, 31 Search PubMed.
- D. Huang and A. Caflisch, J. Med. Chem., 2004, 47, 5791–5797 CrossRef CAS PubMed.
- D. Zhang, L. z. Yu, P. L. Huang, S. Lee-Huang and J. Z. H. Zhang, J. Chem. Theory Comput., 2010, 9, 471–485 CrossRef CAS.
- J. Wang, R. M. Wolf, J. W. Caldwell, P. A. Kollman and D. A. Case, J. Comput. Chem., 2004, 25, 1157–1174 CrossRef CAS PubMed.
- Y. Su, E. Gallicchio, K. Das, E. Arnold and R. M. Levy, J. Chem. Theory Comput., 2007, 3, 256–277 CrossRef CAS PubMed.
Footnote |
† Electronic supplementary information (ESI) available: All-atom RMSD during MD simulations. See DOI: 10.1039/c9ra09583g |
|
This journal is © The Royal Society of Chemistry 2020 |
Click here to see how this site uses Cookies. View our privacy policy here.