DOI:
10.1039/C9NR08186K
(Paper)
Nanoscale, 2020,
12, 1728-1741
In situ analysis of liposome hard and soft protein corona structure and composition in a single label-free workflow†
Received
23rd September 2019
, Accepted 13th December 2019
First published on 18th December 2019
Abstract
Methodological constraints have limited our ability to study protein corona formation, slowing nanomedicine development and their successful translation into the clinic. We determined hard and soft corona structural properties along with the corresponding proteomic compositions on liposomes in a label-free workflow: surface plasmon resonance and a custom biosensor for in situ structure determination on liposomes and corona separation, and proteomics using sensitive nanoliquid chromatography tandem mass spectrometry with open-source bioinformatics platforms. Undiluted human plasma under dynamic flow conditions was used for in vivo relevance. Proof-of-concept is presented with a regular liposome formulation and two light-triggered indocyanine green (ICG) liposome formulations in preclinical development. We observed formulation-dependent differences in corona structure (thickness, protein-to-lipid ratio, and surface mass density) and protein enrichment. Liposomal lipids induced the enrichment of stealth-mediating apolipoproteins in the hard coronas regardless of pegylation, and their preferential enrichment in the soft corona of the pegylated liposome formulation with ICG was observed. This suggests that the soft corona of loosely interacting proteins contributes to the stealth properties as a component of the biological identity modulated by nanomaterial surface properties. The workflow addresses significant methodological gaps in biocorona research by providing truly complementary hard and soft corona compositions with corresponding in situ structural parameters for the first time. It has been designed into a convenient and easily reproducible single-experiment format suited for preclinical development of lipid nanomedicines.
Introduction
Nanoparticles (NPs) assimilate with their physiological milieu to assume a biological identity, the protein corona, which determines their safety and efficacy profile.1–3 Common representations of corona structure involve a dynamic soft corona (SC) of high exchange rate proteins and a more slowly exchanging hard corona (HC) composed of tightly-bound proteins with more specificity for the nanomaterial surface.1,4,5 The implications and proposed mechanisms of corona formation on different NPs have been extensively reviewed elsewhere,2,4,6 but it is important to note that a combined understanding of the physical structure and biological functionalities is required to predict their behaviour with clinical relevance. These attempts are complicated by possible nanomaterial-induced conformational changes in the bound proteins,7 which may escape the traditional methods of preclinical toxicology and can only be observed in in vivo.8 It was recently demonstrated that the corona controls NP interactions with immune cells and pre-coating with artificial coronas prepared in vitro15 or in vivo16 can be employed to extend their blood circulation times and control biodistribution. Identical NPs may also form coronas with different sizes and compositions in different individuals, due to the effect of individual variation and disease on plasma composition or protein structure.22–26 Since these “personalized” or “disease-specific” coronas may lead to different biological responses to the NPs, the therapeutic response and toxicity exhibited by NPs may vary between patients.
Progress has been made in the proteomic characterization of the corona, often by comparing different NPs as a function of time in a variety of physiological fluids,9,10 and more recently by contrasting in vitro and in vivo conditions in both humans and experimental animals.11–14 Several environmental corona-altering variables have been identified, e.g. dynamic flow conditions,17 temperature,18 and duration of exposure.10 The plasma used in vitro is most often diluted due to methodological constraints, and thus unable to mimic corona dynamics observed at physiological concentrations.19,20 Plasma is one of the most complex biological matrices, which further complicates studies on corona composition and kinetics.4,10,21 Removal of loosely bound proteins during sample processing, which also changes the relative composition of the residual proteins, have made it difficult to assess the biological relevance of the SC.20,27 Our limited holistic understanding of corona formation therefore hinders the development of nanomedicines and their successful translation into clinical use.2 Non-invasive in situ analysis is the pragmatic approach to overcome this challenge.
Multi-parametric surface plasmon resonance (MP-SPR) is an established technique to characterize organic biomolecular layers composed of a small number of interacting species.28–30 We pioneered its use to study corona formation and opsonin binding in situ on biosensor-immobilized liposomes in undiluted protein solutions.31 Herein, we present a workflow for undiluted human plasma to allow for simultaneous characterization of physical corona parameters (surface mass density, protein-to-lipid ratio, and thickness) by MP-SPR, and complementary SC and HC compositions with nanoliquid chromatography tandem mass spectrometry (nLC-MS/MS) and open-source bioinformatics platforms (Fig. 1). A novel biosensor with decylamine lipid anchors to capture the liposomes and repellent poly-N-[tris(hydroxy-methyl)methyl]acrylamide (pTHMMAA) moieties to reduce unspecific protein binding directly on the MP-SPR biosensor, permitting the elution of corona fractions for proteomics, is presented.32,33 Our objective was to use a straightforward proteomics approach that emphasizes fast processing of small sample amounts by focusing on the most enriched SC and HC protein species most likely to induce a biological response. The workflow was applied to study regular liposomes and light-triggered indocyanine-green (ICG) liposomes34 with and without polyethylene glycol (PEG) for proof-of-concept in preclinical development (Fig. S7†).
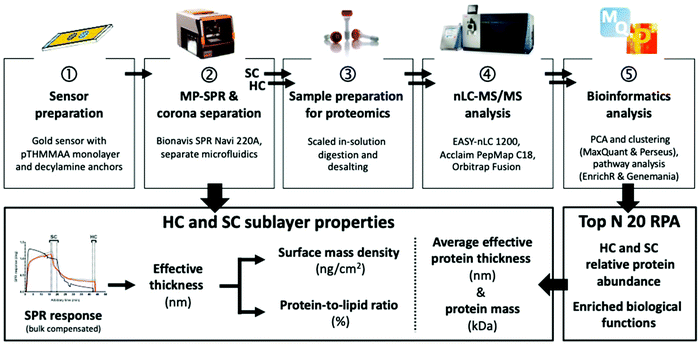 |
| Fig. 1 MP-SPR and quantitative proteomics workflow for liposomes. HC, hard corona; SC, soft corona; RPA, relative protein abundance. | |
Results and discussion
Liposomes are immobilized without modifications on a protein-repellent sensor
Fig. 2 presents the MP-SPR instrument and measurement setup, where no changes to the liposome formulations are needed for immobilization with the capture biosensor.
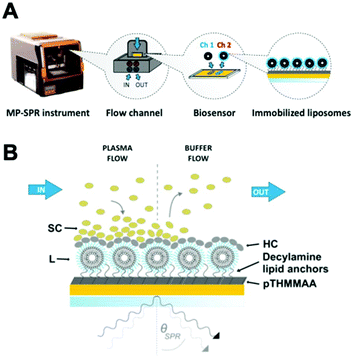 |
| Fig. 2 A. MP-SPR liposome immobilization on the pTHMMAA-BDA biosensor inside the flow channel. The liposomes are injected to the flow channels using an external peristaltic pump. The decylamine lipid chains capture them from inflow and immobilize them on the surface. Although in this proof-of-concept study the same liposome formulation was injected to both channels (Ch 1 and Ch 2), the setup permits parallel analysis of different formulations in the same measurement. B. MP-SPR measurement with the two main measurement stages for the soft corona (SC) and the hard corona (HC). | |
Lipid anchors capture liposomes from inflow by intruding the lipid bilayer without inducing leakage.31,35,36 The hydrophilic self-assembled monolayer surface of thiol-grafted pTHMMAA, functionalized with hydrophobic decylamine anchors (pTHMMAA-BDA) via bromoacetic linkers, forms liposome layers on the sensor without rupturing them into planar membrane surfaces and reduces protein binding directly on the sensor (Fig. S1–4†). The highly repellent properties of pTHMMAA are mainly attributed to the bulkiness and high hydrophilicity of its TRIS headgroups that, together with water molecules, limit hydrogen bonding interactions between proteins and the polymer.33 pTHMMAA-BDA sensors provide an advantage over carboxymethyl dextran gel matrix-based lipid anchor sensors (e.g. Biacore L1 chip35,36) by reducing unspecific protein binding on the sensor coating and permitting sample elution for proteomics studies with limited degradation over three runs (Fig. S5†).
All formulations have an almost neutral surface charge and were found positive for trace endotoxins originating from the starting materials and preclinical manufacture facilities, a common issue in nanomedical development37 (Table S1†). The molar composition of these liposome formulations is 90% phosphatidylcholines DPPC and DSPC, both commonly used due to their biocompatibility.38 Since ICG has been shown to form clusters with PEG on the surface of liposomes, the resulting effect on corona structure and composition could also be explored (Fig. S7†).
The liposomes display substantial differences in their binding amounts and kinetics during immobilization, as demonstrated in Fig. 3 and S6.† The non-pegylated liposome F1 with ICG localized in the lipid bilayer has the strongest affinity for the biosensor, which is decreased by both pegylation and absence of ICG. Since ICG is an amphiphilic compound,40 and ICG on F2 clusters within the PEG layer (Fig. S7†),34 interactions of the TRIS headgroups may influence the sensor binding of F2 in addition to capture by lipophilic anchors. F3 has the lowest affinity for the sensor surface, possibly due to the lack of ICG interference in the PEG layer, which retains an optimal repellent conformation. A dissociation phase is observed after buffer injection with the pegylated formulations F2 and F3, but not with the non-pegylated F1 due to surface lipophilicity. During immobilization, an increase exceeding 0.1 arbitrary units is consistently observed in the SPR peak minimum intensity in the full SPR angular scan curve measured at the 785 nm incident wavelengths of the SPR curve for F1 and F2, but not for F3 without ICG (Fig. S8†). These characteristic changes in the SPR peak minimum intensity measured at 785 nm can be attributed to the presence of ICG in the formulation, since the maximum absorption spectrum of ICG varies from 785 nm in monomeric to 690 nm in aggregate state.41,42
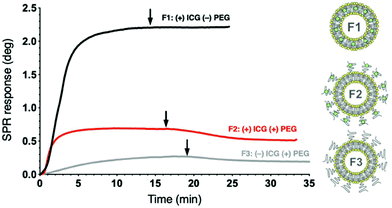 |
| Fig. 3 Representative liposome immobilization profiles for three ICG liposome formulations on pTHMMAA-BDA sensors at the 670 nm incident wavelength using MP-SPR (injection at 0 min). Arrows indicate buffer injection to remove loosely bound liposomes. Liposome illustrations adapted from a Frontiers publication under CC-BY 4.0.39 | |
Interactions of undiluted plasma with liposomes in situ display reproducible kinetic profiles
Fig. 2B and 4 depict the human plasma interaction measurement with MP-SPR with two measurement stages corresponding to the SC and HC, respectively. Citrate-anticoagulated fresh-frozen plasma collected within 24 hours (FFP24) is used for its in vivo relevance19 and availability from many national blood services as a pre-pooled product. The total protein concentration of the injected plasma, measured using the BCA assay, was 75.8 mg ml−1. After 15 to 20 min, the plasma injection is switched to buffer, which flushes off the SC for proteomics analysis and reveals the HC. After a wash out period, the liposomes and the tightly-bound HC proteins on their surface are eluted off the sensor's decylamine lipid anchors using Rapigest SF, an LC-MS/MS compatible surfactant. SC formation can be observed with all formulations following plasma injection with distinct and reproducible kinetic profiles (Fig. 4 and Fig. S9†).
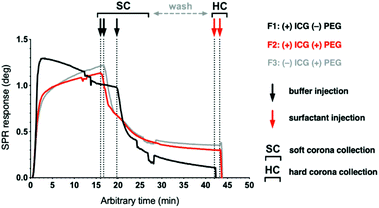 |
| Fig. 4 Sensogram of the plasma interaction following the liposome immobilization step, compensated for the bulk effect. Plasma injection (0 min) is followed by a buffer injection (↓) to wash off the loosely bound SC proteins and reveal the HC. The HC fraction is collected following an injection of the Rapigest SF surfactant ( ). The time points used for calculating layer properties are indicated with the vertical lines. | |
Interestingly, the plasma interaction profile of the non-pegylated F1 is different from the pegylated formulations: instead of a gradual increase without surface saturation, a gradual decrease is observed with F1. This suggests that the non-pegylated surface repels plasma proteins and other biomolecules after the initial protein layer is formed, whereas the PEG-surfaces constantly accumulate biomolecules on their surface and their deposition does not saturate within 20 min. When the plasma injection is switched to buffer to elute the SC and reveal the HC, the loosely bound biomolecules dissociate readily with similar kinetics from all the liposome surfaces. These results also demonstrate that the sensing depth of SPR, which is approximately half of the incident wavelength or 335 nm with a 670 nm laser as the lower wavelength, is sufficient to study corona formation on 120 nm liposomes.43,44
Biophysical analysis of protein “shells” for more accurate characterization of the hard and soft corona subsections
The real-time SPR sensograms have been compensated for bulk effect and the liposome immobilization levels have been adjusted to the same baseline. However, the residual amounts of adsorbed biomolecules in the corona, and the corresponding thicknesses on the different liposomes, should not be analysed directly from the plasma SPR responses because different amounts of liposomes are adsorbed on the sensor surface in each case. Therefore, to obtain comparable values, these are analysed in combination with the SPR responses obtained during the liposome immobilization stage (Fig. S6†). This permitted us to compare the amount of protein adsorbed to the amount of immobilized lipid, enabling the determination of effective corona thicknesses for each case. The SPR response right before the signal decreased as a result of switching from plasma to buffer, or from buffer to surfactant injection, was used to represent the SC and HC phases, respectively (indicated by the vertical lines in Fig. 4).29,45
Liposome diameters (d) were first estimated by software-assisted layer solving using two wavelengths (670 nm and 785 nm), and the contributions of changes in bulk liquid refractive index to the SPR responses were corrected simultaneously. The protein corona thicknesses were then determined using the formalism presented in ESI.† SC was considered as a denser embedded HC layer covered by loosely interacting proteins. Although protein binding to nanomaterial surfaces may employ crowding effects such as clustering,46 the analysis was conducted based on the assumption of random sequential adsorption (maximum surface coverage 54% for spheres in a 2D system).47 To determine the liposome amounts, either the light-absorbing properties of ICG (F1 and F2) or the ratio of the saturated liposome immobilization responses at two wavelengths (F3) were used. The relative amounts of protein and lipid were accounted for by assuming that the number of liposomes was equal to the number of spherical shells of protein coating the liposomes on the sensor. Using this equality, the effective corona thicknesses for the corona subsections (lp) were calculated, where the calculated thickness depends on the choice of mass density in the protein layer. For comparison, the average effective protein thicknesses of the HC and SC, based on their average molar mass values, are presented along with the modelled effective corona thicknesses (Table 1).
Table 1 MP-SPR biophysical analysis results for liposome diameter d, surface mass density Γ, and effective thickness of the protein layer lp with protein-to-lipid ratio (P/L %) of ICG liposome (L) soft (SC) and hard (HC) coronas, presented as a mean with S.E.M. The average effective protein thicknesses and molecular weights for SC and HC based on proteomics analysis are presented for comparison
|

|

|

|
L |
d nm |
86.63 ± 15.78 |
67.87 ± 31.20 |
89.13 ± 31.12 |
Γ ng cm−2 |
1557.64 ± 239.48 |
341.88 ± 26.42 |
199.97 ± 20.18 |
SC |
l
p nm |
4.04 ± 0.85 |
10.22 ± 1.38 |
18.16 ± 1.06 |
Γ ng cm−2 |
896.56 ± 55.73 |
731.16 ± 108.14 |
843.38 ± 1.26 |
P/L % |
0.76 ± 0.17 |
2.67 ± 0.54 |
5.21 ± 0.57 |
HC |
l
p nm |
0.94 ± 0.52 |
3.17 ± 0.07 |
4.34 ± 1.68 |
Γ ng cm−2 |
173.13 ± 71.38 |
168.91 ± 15.62 |
130.72 ± 44.32 |
P/L % |
0.14 ± 0.08 |
0.50 ± 0.02 |
0.75 ± 0.34 |
Average effective protein thickness and molecular weight: |
SC |
2.9 nm |
65 kDa |
HC |
3.1 nm |
77 kDa |
The calculation of the average molar mass of protein is based on the actual corona compositions obtained in the proteomics analysis, but it does not account for formulation-specific differences and corresponds to the estimated thickness of a single HC or SC protein layer assuming random sequential adsorption (eqn (S12)†). Since the SPR method averages over the entire layers of biomolecules on the liposome surface, and thicknesses (lp) denote layers with the same density, an effective corona thickness value that is below the average protein thickness after normalizing for liposome amount suggests that the biomolecules on their surfaces are not tightly packed. Surface mass densities (mass per unit area) are essentially the product of the thickness and optical density at one wavelength, detected at 670 nm to avoid any interference by ICG. When the HC or SC surface mass densities are compared to the corona thicknesses, the difference in the liposomal surface mass densities must be considered using the protein-to-lipid ratio (i.e. number of molecules) (Fig. 5 and Fig. S10†).
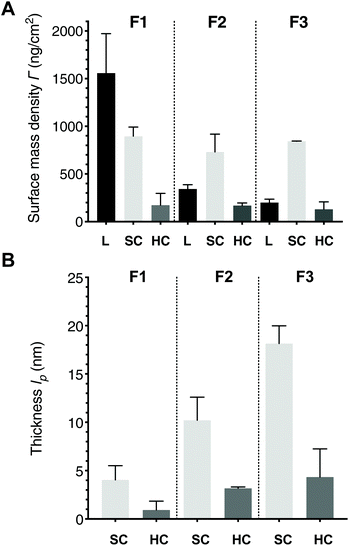 |
| Fig. 5 Comparison of surface mass densities between three liposome formulations based on optical parameters obtained at 670 nm: liposome (L), soft corona (SC), and hard corona (HC). B. Comparison of the effective corona thicknesses (lp). | |
Considering that liposomes are not immobilized as ideal spheres but are truncated as a result of their interaction with the surface and may shrink osmotically as a result of protein binding,11,48 the liposome layer diameters (d) are in good agreement with the 110 ± 20 nm to 120 ± 27 nm DLS bulk diameters (Table S1†). Comparing the calculated effective corona thicknesses (lp) to the size range of proteins in plasma, which is between 3–18 nm,49 the mean thicknesses suggest a 1–4 nm monolayer configuration for the HC, and 4–18 nm a structured organized configuration of at least protein bilayer thickness for the SC. Recent studies that have visualized in vivo coronas have noted that they appear not to coat the entire surface of the liposome, at least after separation and washing.5,14 Since the estimated effective average protein thickness for HC was 3.1 nm, a lower value for the effective corona thickness on F1 indicates that the proteins on the surface are not tightly packed into a single uniform protein layer but are spread out; the F2 and F3 effective HC thicknesses suggest more tightly packed monolayers of protein. The percentage protein-to-lipid ratio reflects the same trend, but F2 with PEG-clustered ICG showed remarkable consistency in HC formation, whereas there was less consistency in corona formation on F3 with PEG alone.
Interestingly, pegylation slightly decreases the SC surface mass density but does not influence the HC, and no significant difference is observed between the two pegylated formulations for SC or HC. However, the percentage protein-to-lipid ratios show that the pegylated formulation F3 accumulated almost twice the molar amount of proteins per amount of lipid comparing to F2, again most likely a reflection of the amphiphilic nature of ICG and its clustering within PEG. Several authors have noted that the corona continues to evolve under in vivo relevant conditions, where the amount of biomolecular species is not limited by scarcity and where biological processes affect the corona during its passage through different biological compartments (i.e. immune activation and enzymatic modifications).50,51 In our measurements, neither of the pegylated formulations saturated during the ca. 20 min incubation period (Fig. 4 and S9†). Considering that all our formulations tested positive for traces of endotoxins, it is probable that these surfaces induced complement system activation, which can increase the amount of bound biomolecules by as much as 25% due to surface deposition of opsonins.52,53 The slight differences between F2 and F3 in thickness and surface mass density, and the clear difference in protein-to-lipid ratios between the pegylated formulations demonstrate that ICG influences corona formation. Overall, the mass accumulated per area is very similar for all SC and HC, respectively, whereas clear differences between the liposome formulations are seen when the amount of bound protein is compared to the amount of lipid. The replicate data calculations are provided as ESI Table M1.†
Corona studies generally employ static incubation conditions where protein adsorption reaches equilibrium quickly.50 For instance, Walczyk et al. reported HC thicknesses of 11–12 nm before and 10.5 nm after extensive washing for differential centrifugal sedimentation isolated 100 nm surface-carboxylated polystyrene NPs, measured using TEM and DLS.54 More recent studies on unfunctionalized 140 nm polystyrene NPs obtained a HC thickness of 15 nm by TEM and 19 nm in human serum at 37 °C using multi-angle DLS.5 The recent study by Kokkinopoulou et al. on 140 nm polystyrene NPs visualized the SC and the HC using TEM with negative trehalose-metal staining, which yielded an average layer thickness between 70–100 nm for the entire corona.5 As the authors themselves stated, the corona was more accurately described as a loose network of proteins associated with the NP.5 Overall, the reported corona thicknesses in literature range from a few nanometres with individual proteins55 to a few hundred nanometres on sulfonated polystyrene NPs in plasma.56 In our case, the calculated corona thicknesses were limited by the assumption of constant density. Some variation depending on the choice of analysis method as well as the biophysical analysis approach is expected, but the results presented here clearly fall within the limits reported in the literature.
Contrary to our hypothesis, our analysis yielded the lowest SC thickness for the non-pegylated F1 and the highest for pegylated F3, with pegylated F2 at mid-range. Previously, authors have reported that a thinner corona forms on pegylated liposomes as opposed to uncoated counterparts, with a dependence on the PEG chain length,57,58 and dynamic flow conditions further reduce corona thickness on pegylated liposomes.17 For instance, Pozzi et al. showed that the hydrodynamic diameter of pegylated cationic liposomes at the start of the incubation in 1
:
1 diluted human plasma was similar to buffer. The original size difference between the uncoated and PEG2000-coated formulation was 30 nm, which could also suggest that the hydrated PEG shell is compressed and the volume is replaced by the adsorbed biomolecules. The first measurement point diameter for the uncoated formulation was about 50 nm higher (HC thickness of 25 nm).57 Interestingly, the size evolution of the uncoated liposome formulation did not show significant changes over time, whereas the thickness of the PEG2000-coated formulation increased by 10–20 nm. This would be consistent with the trend observed in Fig. 4, where the non-pegylated F1 liposome surface saturates instantly and begins shedding biomolecules already during the plasma interaction, whereas the amount of surface-bound molecules increases gradually on both pegylated formulations. Monopoli et al. have also demonstrated using 200 nm hydrophilic silica nanoparticles that plasma dilution increases the thickness of the hard corona.56 Thus, the HC thicknesses obtained in this study that are at the lower end of those reported in the literature could be partly explained by the use of undiluted plasma.
Proteomics snapshot compositions distinguish between complementary hard and soft corona subsections with possible biological implications
Proteomics data acquisition was done using a high-resolution nLC-MS/MS instrument, where each replicate measurement was analysed separately after protein digestion, since the lack of sufficient sensitivity has been proposed as an explanation for the earlier findings on a constantly shifting corona composition.4,10,59 A data-dependent Top N (N = 20) method that emphasizes abundance was considered preferable, since we expect that the most abundant corona proteins will distinguish the different corona types – and ensuing biological responses – most effectively. The structural properties and proteomic compositions of the eluted SC samples correspond to 15–20-minute plasma exposures. The SC in terms of biophysical analysis refers to the combination of a denser corona layer (embedded HC) together with a diffuse layer of weakly interacting proteins, but the eluted proteomics sample evidently cannot contain the HC. In the following sections, HC1 indicates the hard corona and SC1 the soft corona of F1, and so forth for the other two formulations with three replicates. In total, 189 plasma proteins were detected on a one-hour nLC gradient without fractionation, of which 130 were quantified in all three biological replicates of at least one corona type. On average 127 and 153 protein identifications were obtained for the SC and HC samples, respectively. Principal component analysis (PCA) using the top 2 components of all 189 identified human plasma proteins demonstrated that 71% of the variation in protein abundance clusters the samples into three main groups: SC, HC and the human plasma source (sampled both before and after injection to MP-SPR; Fig. S11†). In addition, within the SC, a very clear distinction could be observed between F2, and formulations F1 and F3 (Fig. S11 and 12†). The significant differences observed between the plasma and corona clusters indicate enrichment on the liposomes, while the separate HC and SC clusters suggest distinct biological roles and ensuing responses for these protein fractions.
After normalizing for total protein abundance, the 50 most abundant proteoforms clearly distinguished unbound plasma from the SC and HC corona fractions, representing 93% to 97% of the total identified plasma proteome from all samples (Fig. 6A). Comparing to plasma, two separate clusters of enriched proteins could be seen: proteins that were predominantly depleted in SC1 and SC3 (red box), and proteins that are highly enriched in HC1, HC2 and HC3, and moderately enriched in SC2 (black box). Several apolipoproteins (C-III, A-I, A-IV), fibrinogen (alpha, beta and gamma chains) and immunoglobulins were notably enriched in the coronas. Recent results by Amici et al. suggest that in vitro conditions exacerbate the binding of the three fibrinogen chains to liposomes compared to in vivo conditions, and the presence of apolipoproteins is increased.60
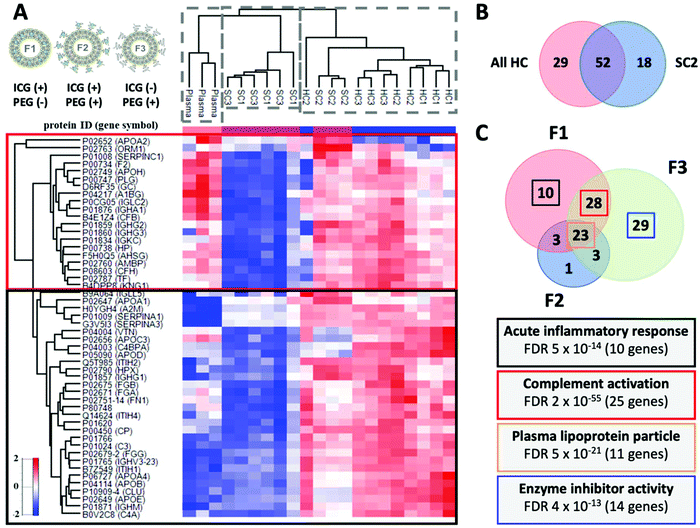 |
| Fig. 6 A. Corona composition with the Z-score normalized hierarchical cluster of the Top 50 most abundant proteins with protein identifications and gene symbols. The abundance profiles distinguish between HC, SC, and plasma. The red box shows proteins that are predominantly depleted in SC1 and SC3. The black box proteins are highly enriched in HC1, HC2 and HC3 and moderately enriched in SC2. Strikingly, these proteins are comparatively depleted in SC samples from F2 (SC2). Liposome illustrations adapted from a publication by Frontiers under CC-BY 4.0.39 B. Venn diagram of the differentially abundant proteoforms in HC and SC2 with “HC-type proteins”. C. Venn diagram of the differentially abundant proteoforms as a function of the liposome formulation and the associated biological functions. Highest number of differentially abundant proteins between HC and SC was observed with F3. The biological relevance of the HC versus SC changes with modulation of the liposome surface. Interestingly, the HC of the pegylated formulation F3 without ICG enriched 14 genes found linked to enzyme inhibition (APOC1, SERPINA3, ITIH4, PROS1, SERPIND1, AMBP, AGT, SERPINA1, APOC3, AHSG, SERPINF2, SERPINF1, APOC2, and APOA2). | |
Next, we performed a relative enrichment analysis using only those proteins with abundance values in at least three biological replicates (139 in total). Interestingly, F2 repeatedly produced a distinct SC2 composition, with protein annotations that were found depleted in the other SCs and containing “HC-type proteins” with 52 proteoforms in common with HCs and only 18 distinct for SC2 (Fig. 6B). A hierarchical cluster and Venn diagrams of the differentially abundant proteoforms between the specified contrasts are shown in Fig. S12 and S13,† whilst the lists of proteins are provided as ESI Tables P1, P2, and P3.† A relative log
2 fold-change analysis comparing HCs identifications to source plasma demonstrated that clusterin (7.9-fold) and apolipoprotein E (7.7-fold) are the most significantly enriched proteoforms (ESI Table P1†), and they are also significantly enriched when any HC is compared to their respective SCs (ESI Table P2†). This may partly reflect the effect of washing on relative HC composition: Kokkinopoulou et al. reported increasing relative abundances of clusterin, apolipoproteins A-I, C-II, and A-IV, and immunoglobulins in the HC with every additional washing cycle.5 As a result, immunoglobulins were the major enriched plasma component with a relative abundance of nearly 50%.5 Also, albumin is typically replaced by apolipoproteins (A-I, A-II, A-IV, and E) during incubation on hydrophobic surfaces.61,62
Notably, this simple Top N label-free quantification approach was sufficiently sensitive to identify protein annotations considered relevant by other studies in the field.17,60,63–65 This was achieved without upstream fractionation that is typically done to maximize the number of identified proteins. While these observations also point to some degree of protein specificity for the NP surfaces, they also underline the definition for HC as the “analytically accessible” proteins on the surface that are not removed by sample processing.4 In the same vein, the existence of a “softer” corona has remained disputed due to the fact that it has remained analytically inaccessible.4,5 The presented workflow therefore replicates typical HC compositions,17,60,63–65 along with truly matching SC compositions formed by weak protein–protein or protein–surface interactions.27,66
Biological functions modulated by liposome surface associate with immune response, lipid processing, and enzyme inhibition
Analysis of the biological functions associated with the HC protein signatures of all formulations with GeneMANIA, EnrichR, and FunRich indicated enrichment of plasma lipoprotein particle processing with 11 corresponding genes (Fig. 6C and S15†). This has implications for targeted drug delivery, since an apolipoprotein-enriched corona was shown to switch the cellular uptake mechanism and trafficking pathway of lipid nanoparticles towards LDL–receptor interactions and clathrin-mediated endocytosis.67 Based on the top-enriched biological functions, the most immunogenic formulation is F1, enriched with acute inflammatory response and complement activation, the latter property conjoining with F3 due to 25 common complement-related genes (Fig. 6C). The depletion of immune response-related genes in the HC2 is explained by its preference to enrich these proteins in the SC2, including classical and alternative complement pathway proteoforms and immunoglobulins. Therefore, the presence of clusterin on both HCs and notably SC2 may be linked to the presence of complement system components, owing to its role as a weak complement regulator or at least an interaction partner in common biological pathways68 (Fig. S15†). Excluding SC2, our multigroup ANOVA analysis of SC1/SC3 versus HC1/HC2/HC3 signatures identified strong but non-overlapping association with complement activation, echoing observations on the complement's flexibility to switch activation pathways69 (Fig. S16†). Circulating biological media has been shown to decrease the enrichment of complement system components,70 but our results also reflect the endotoxin-contamination of the sample material, which is a limitation but also reflects the state of affairs in nanomedicine development.37 However, comparing complement activation by F2 to a Doxil-replica liposome in the presence of endotoxins demonstrated a similar tendency for activation proceeding to the terminal pathway, at 1–4-fold compared to negative control for C3a and sC5b-9, when 2-fold is considered activation71 (Fig. S17†). Analysis of top biological functions reveals that the biological relevance of the HC versus SC changes with modulation of the liposome surface.
Stealth-mediating proteins enrich on liposomes regardless of pegylation and in the soft corona
Clusterin (apolipoprotein J), which has been shown to mediate the stealth effect of polyethylene glycol by reducing mononuclear phagocyte system (MPS) uptake,72–74 was significantly enriched on the HCs of all formulations regardless of pegylation. Therefore, it is interesting that comparative analysis of the distinct SC2 signature with the other SCs reveals a significant enrichment of clusterin and apolipoprotein E (Fig. S12 and 13†). This suggests that loosely interacting proteins may be relevant in mediating the stealth effect towards phagocytes, and that ICG-PEG clusters influence the SC composition in addition to its structural properties. Since all formulations contain trace endotoxins (F2 contained slightly less than F1 or F3), this difference in enrichment of immune system components is related to the ICG-PEG clusters rather than immunogenicity (Table S1†). Although the NP type may play a role, this contradicts the report by Winzen et al. that apolipoprotein A-I is a hard corona-specific protein, when complementary methods with separate workflows were used to study the HC and SC on hydroxyethyl starch nanocapsules.27 Interestingly, apolipoprotein A-I was also shown to inhibit NP cell uptake in a recent study by Kokkinopoulou et al., which was also observed as a result of pre-incubation with SC but not HC proteins.5
The non-pegylated F1 repelled protein binding after the formation of an initial layer of molecules, while the accumulation on both pegylated surfaces F2 and F3 was cumulative. Pegylation did not significantly influence surface mass density, but it increased the protein-to-lipid ratio and thickness of both HC and SC. The protein antifouling properties of the flexible PEG molecule coatings arise from steric repulsion and a hydration shell formed by water molecules interacting via hydrogen bonds.75,76 Zwitterionic materials, similar to the phosphorylcholines in the liposome phosphatidylcholine (PC) head group, have been explored as alternative hydrophilic coatings, especially for long-term biomedical applications where PEG's oxidation is an issue.76,77 They have been inspired by the antifouling properties of cell membranes, which form denser and thicker hydration shells with water via electrostatic interactions.78 Compared to hydrophilic molecules with repeat units such as PEG, zwitterionic coatings bind more water per molecule, which also decreases the risk of protein conformational changes as a result of transient surface adsorption.78 The molecular dynamics simulations with the ICG liposomes suggested that its presence in the lipid bilayer at a depth of 5–6 DPPC carbons destabilizes the layer slightly.34 As a result, water molecules could have better access to the zwitterionic PC headgroup of F1, forming a repellent hydration shell that outperforms the PEG2000 coatings with optimized densities on F2 and F3.
Recent results demonstrate that PEG harvests specific apolipoproteins as a result of preferential binding, which inhibit uptake and elimination by the MPS.79–81 The harvesting effect is observed in the tendency of F2 and F3 to attract more proteins per lipid to the surface and form thicker corona layers than F1. They show that while protein enrichment is modulated by surface coatings, accumulation of stealth-mediating apolipoproteins is not dependent on PEG. However, the top enriched biological functions for F1 were related to immune system activation, compared to the pegylated formulations which were associated with plasma lipoprotein binding and enzyme inhibitor activity corresponding with MPS uptake inhibition. Together with the observation on PEG's tendency to harvest more protein, these may suggest that more extensive binding and enrichment of these proteoforms is necessary for the activity of hydrophilic coatings. However, it is noteworthy that the proteoforms do not always distinguish between protein subtypes. For instance, clusterin exists in different forms in different biological compartments and is present in serum as a polydisperse mixture of oligomers with different molecular masses.82 These different forms may have different NP interaction modalities as well as biological functions. However, our results show that liposomal lipids may induce the enrichment of a similar “stealth apolipoprotein signature”, which accumulated on F1 at lower protein-to-lipid ratios but similar surface mass densities. Importantly, our results demonstrate that surface coatings and structural components such as lipids can also modulate both the structure and the protein composition of the SC. Especially the significant relative enrichment of clusterin and apolipoprotein E of the ICG-PEG coated F2 comparing to pegylated F3 demonstrates that this loose network of proteins may be relevant in mediating the stealth effect towards phagocytes, since this is what the cell sees first.
The preclinical workflow provides a convenient tool for holistic corona tailoring
Since there is no definitive agreement on what is considered a “full” SC, it is advantageous that the entire fraction of loosely interacting plasma proteins is eluted from MP-SPR and subjected to enrichment analysis, which looks at relative differences to identify nanomaterial-associated proteins. The truncated liposomes retain their convex shapes on the sensor and the interacting surfaces do not significantly differ from an in vivo experiment. While we agree with other authors that in vitro conditions do not fully emulate an in vivo corona,11,14 we argue that in situ coronas formed under in vivo relevant conditions are more accessible for preclinical development and provide more flexibility and convenience for the development scientists. The workflow complements other in situ approaches presented in the literature that do not require removal of “excess” proteins but require labeling, e.g. fluorescence correlation spectroscopy (FCS),8319F diffusion-ordered nuclear magnetic resonance (NMR) spectroscopy,84 and the asymmetric flow field-flow fractionation approach (AF4)85 as a notable label-free method for separation of the SC. By providing a crude assessment of packing density in the corona layers, the presented method combination offers insights previously limited to studies requiring sophisticated study setups to visualize the corona ex vivo.
Although not explored in this proof-of-concept study, the presented methods platform permits modifying the temperature, flow, and exposure time to explore their effects on the corona structure and composition. Considering the recent reports on selective enrichment of proteins necessary for stealth properties and the associated PEG density thresholds,58,86 this workflow provides a convenient method to study “personalized protein coronas”22–24 or apply the concept of “corona tailoring” directly into the preclinical development of nanomedicines. Diseases and individual variation in the plasma proteome is expected to be reflected in the composition of the corona sublayers, but the effect of disease-induced modifications23,24 on the protein affinity towards NPs should also be explored. With the method platform, this can be done using individual proteins and in undiluted complex protein solutions to assess the resulting effect on the corona. Treuel et al.87 demonstrated using albumin that carboxyl and amine modifications influenced its affinity towards the NP but also changed the binding orientation, which resulted in different protein layer thicknesses. For instance, protein glycation in diabetic patients88 may affect their affinity to NPs, which may similarly influence the structural properties of the corona in addition to its composition. The platform is also suitable for the creation of “artificial coronas” for immune cell evasion15 in dynamic incubation conditions, and assessing their stability over time under physiologically-relevant conditions. Notably, the effect of the pre-coating on the properties of the corona formed in the second incubation can be studied without separation steps or issues with NP aggregation. The effect of individual variation, disease, or pre-coating with artificial coronas on the SC should also be explored in the future.
In addition to exploring corona kinetics and dynamics, the protein-to-lipid ratios permit us to further explore the link between more accessible parameters such as “total protein binding” on nanomedicines and the pharmacokinetic properties that reflect their blood clearance.89–91 Moreover, the method can be used in molecular biology to study protein–lipid binding interactions (affinity and specificity) with unilamellar liposomes, eliminating the need for separation or additional quantification methods.92
To our knowledge, this is the first time that complementary compositions for a HC and SC have been provided in one workflow, while also providing information on the corresponding relative structural properties of these layers (thickness, protein-to-lipid ratio, and surface mass density), without batch or interexperimental variation. Moreover, this is accomplished using undiluted and flowing human plasma for increased in vivo relevance, since dilution is known to influence dynamic equilibrium reactions relevant for corona formation.31,93 Depleted, disease-state-associated, or patient-specific protein solutions may be used with the current digestion protocol, but cellular fractions require modifications to the microfluidics and additional separation steps. Both the SC and the HC can be studied at different time-points with sufficient sensitivity to analyse individual biological replicates. Sensor technologies can be developed for other nanoparticle technologies (excluding metallic). Hence, the presented workflow answers significant methodological limitations in the field.3,4,10,20,94 Moving “beyond the protein corona”, the workflow will be modified for other omics methodologies – for lipids, nucleic acids, and sugars – to provide a more holistic picture of biocorona formation in different biological matrices.1,95
Materials and methods
Preparation of ICG liposomes
The liposomes were prepared for immediate use with a previously reported protocol with modifications.34 1,2-Dipalmitoyl-sn-glycero-3-phosphocholine (DPPC), 1,2-distearoyl-sn-glycero-3-phosphocholine (DSPC), 1-stearoyl-2-hydroxy-sn-glycero-3-phosphocholine (Lyso PC), 1,2-distearoyl-sn-glycero-3-phosphoethanolamine (DSPE), and 1,2-distearoyl-sn-glycero-3-phosphoethanolamine-N [methoxy(polyethylene glycol)-2000] (DSPE-PEG) were bought from Avanti Polar Lipids, Inc. (Alabaster, AL, USA). All other compounds were bought from Sigma-Aldrich (St Louis, MO, USA). Briefly, the lipids were dissolved in chloroform at molar ratios of 75
:
15
:
10
:
4 for DPPC
:
DSPC
:
Lyso PC
:
DSPE or DSPE-PEG, respectively (10 μmol of total lipids). The liposomes were formed by a thin film hydration method followed by extrusion through a polycarbonate membrane. Chloroform was evaporated by heating to 60 °C and gradually reducing the pressure to 70 mbar under a nitrogen flow in a vacuum rotary evaporation system (Büchi R-114, Büchi Labortechnik AG, Flawil, Switzerland). The resulting thin lipid layer was hydrated at 60 °C for 1 h with 500 μL of HEPES buffer solution (20 mM HEPES, 140 mM sodium chloride, pH 7.4, 280 mOsm kg−1) including ICG (0.2 μmol). The formulations differ in regards of presence of PEG (formulations 2 and 3) and ICG (formulations 1 and 2). During the hydration, ICG is stabilized within the bilayer in case of formulation 1, or by clustering with PEG chains in case of formulation 2. The samples were then extruded 11 times (60 °C) through a polycarbonate membrane (pore size of 100 nm) with a syringe extrusion device (Avanti Polar Lipids). The samples were quickly cooled down and stored in a refrigerator. The samples were diluted with the HEPES buffer to the final lipid concentration of 1.5 mM prior to the measurements.
Physicochemical characterization
Liposome hydrodynamic diameters were measured in triplicate at 25 °C using a Zetasizer APS DLS instrument (Malvern Instruments, Malvern, United Kingdom). These are derived from relative signal intensity distributions, and are reported as a mean and S.D. of the particle size, and the polydispersity index (PDI). The liposome surface charge was determined by ζ-potential measurements in triplicate at 25 °C in DTS 1070 folded capillary cells (Malvern Instruments) using a Zetasizer Nano ZS instrument (Malvern Instruments). Surface charge is reported as the mean with S.D.
Endotoxin determination
The Pierce LAL chromogenic endotoxin quantitation kit (Thermo Fisher Scientific, Waltham, MA, USA) was used according to manufacturers’ instructions, with a Thermomixer C heat-block (Eppendorf AG, Hamburg, Germany) and endotoxin-free consumables for incubation. A SpectrostarNano plate reader (BMG Labtech, Ortenberg, Germany) was used to determine endotoxin content by linear fitting of A405 nm on the accompanying MARS software v 2.40. Endotoxin content is presented visually from (+) indicating 1.0 EU ml−1 to above range at (+++). Inhibition and enhancement controls and transmissivity measurements were conducted to rule out assay interference.
Plasma
Human plasma frozen within 24 hours (FFP24) was obtained under a research permit from the Finnish Red Cross Blood Service (Helsinki, Finland). O-type whole blood anticoagulated with 1
:
10 citrate-phosphate-dextrose (CPD) was separated at room temperature using a CompoMat G5 automatic blood processing system (Fresenius Kabi, Bad Homburg, Germany). The CPD-anticoagulated plasma may contain at most 0.030 mmol of citric acid monohydrate, 0.056 mmol of adenine, 2.30 mmol of glucose monohydrate, 14.6 mmol of glycine, 2.15 mmol of mannitol, 0.88 mmol of sodium chloride, 4.80 mmol of sodium citrate, and 1.43 mmol of sodium dihydrogen phosphate dihydrate, as well as trace amounts of di-2-ethylhexyl phthalate (DEHP) plastic softener used in the packaging. The plasma was frozen for transport, thawed at 37 °C for aliquoting in aseptic conditions within 36 hours of phlebotomy, and stored at −80 °C until use. The volunteer donors have provided their informed consent for research use but their gender and identities are anonymized by the supplier.
Liposome capture biosensor
Gold-plated SPR102-AU sensor chips (BioNavis Ltd, Tampere, Finland) were cleaned by boiling for 5 min in H2O2
:
NH3
:
H2O (volume ratios 1
:
1
:
5) and washing with Milli-Q water (Merck KGaA, Darmstadt, Germany). Adapting a protocol described earlier,28 the sensors were immersed in 0.2 mg ml−1 pTHMMAA (synthesized according to Albers et al.33) in a solution of 99.5% (w/w) ethanol (Altia Oyj, Rajamäki, Finland) and Milli-Q water (8
:
2) to form a self-assembled monolayer (SAM) by a thiol-graft-to-gold reaction at room temperature for 1 day. The SAM-surfaces were flushed with Milli-Q and ethanol 70% (w/w), and dried with N2 (g) (Oy Aga Ab, Espoo, Finland). The SAM pTHMMAA tris-ends were activated by 1 M bromoacetic acid (Merck KGaA) in 2 M sodium hydroxide (Honeywell Riedel-de-Haën, Morris Plains, NJ, USA) for 15 min, followed by 10 min in 80 mg ml−1 EDC (Merck KGaA) and 20 mg ml−1 NHS (Merck KGaA) in sodium diphosphate buffer (Honeywell Fluka, Morris Plains, NJ, USA). The sensors were immersed for 10 min in 30 mg ml−1 decylamine suspension and treated for 1 min with 1 M 2-aminoethanol (pH ∼ 8.5). The sensor was characterized with polarization modulation-infrared reflection–adsorption spectroscopy (PM-IRRAS), quartz crystal microbalance (QCM) and contact-angle measurements in addition to MP-SPR. The functionalized pTHMMAA-BDA sensors were washed and dried as described earlier and stored at 4 °C. Sensor development and characterization are described in detail in the ESI.†
Multi-parametric surface plasmon resonance (MP-SPR) studies
The measurements were conducted with an MP-SPR Navi™ 220A (BioNavis Ltd) using 670 nm and 785 nm incident laser. To enable the use of undiluted plasma, the autosampler loops were replaced by two flow channels with 0.51 mm Tygon LMT-55 (Saint-Gobain Performance Plastics, Paris, France) and 0.51 mm PEEK (IDEX Health & Science, Wallingford, CT, USA) tubing connected to a PEEK flow cell (BioNavis Ltd), with an Ismatec ISM596D digital peristaltic pump (Cole-Parmer GmbH, Wertheim, Germany). Liposome immobilization and plasma interaction measurements were conducted at a flow rate of 50 μL min−1 with the flow cell temperature at 20 °C. The pTHMMAA-BDA sensors were subjected to running buffer (HEPES 10 mM, NaCl 150 mM, pH 7.4) until the SPR sensogram signal stabilized. The same liposome formulation was injected into both channels for ca. 10 min, followed by running buffer to remove uncaptured liposomes. Human FFP24 plasma was thawed rapidly up to 90% in a 37 °C water bath and vortexed to ensure heat homogeneity before injection into both channels.31 After switching from plasma to buffer injection, the entire SC fraction (∼20 min corona) was collected into an Eppendorf Protein LoBind tube (Eppendorf AG), moved immediately to −20 °C. After the SPR signal stabilization, the running buffer was continued for 20–30 min to remove all loosely bound biomolecule species, followed by injection of a 0.1% Rapigest SF (Waters Inc., Milford, MA, USA) bolus and collection of the HC fraction. Flow-through plasma on a saturated uncoated sensor was used as the source control for proteomics analysis. Sensor-eluted corona and source plasma samples were tried overnight at 30 °C using an Eppendorf Concentrator Plus (Eppendorf AG) in aqueous solution mode, and digested or stored as pellets at −20 °C until digestion. All liposome formulations were measured in triplicate (‘biological replicates’) using dedicated sensors up to three times. The pTHMMAA-BDA sensors were rejuvenated with CHAPS 20 mM (Sigma-Aldrich), ethanol 70% (Altia Oyj) and Milli-Q water (Merck KGaA), and stored immersed in CHAPS at 4 °C. After use, the microfluidic system was washed with a mock sensor as described earlier, with CHAPS replaced by Hellmanex II 2% (Hellma Analytics, Müllheim, Germany).
MP-SPR biophysical analysis
MP-SPR Navi™ LayerSolver™ version 1.2.1 (BioNavis Ltd) was used for thickness d and refractive index n analysis using two wavelengths (670 nm and 785 nm). For the light-absorbing ICG liposomes, an algorithm was used to solve complex refractive index n + ik and thickness d simultaneously using the two different wavelength spectra from the same time point from multiple full SPR spectra in a numerical iteration procedure.29,45 SC refers to the combination of a denser corona layer (embedded HC) together with a diffuse layer of weakly interacting proteins.31 Calculations of surface-mass density (Γ) and effective layer thicknesses (lp) of the protein corona were conducted using analysis based on Jung model,96 as described in the ESI.† For non-ICG liposomes, LayerSolver™ was used only for correcting the bulk contribution in shift of SPR peak angular minimum during the injection of plasma. Diameters of non-ICG liposomes, derived from the ratios of Jung model responses from the two wavelengths, were used in the modelling.
Sample preparation for proteomics
Three independent biological replicates from all three formulations were resuspended after drying in 50 mM ammonium bicarbonate buffer (AMBIC), pH 7.8, supplemented with 0.1% RapiGest SF (Waters Inc.). The source plasma pool was solubilized in 0.2% RapiGest SF (Waters Inc.) prior to resuspension. The protein concentration of the source plasma and each sample was determined with a standard BCA protein assay kit (Thermo Fisher Scientific, Waltham, MA, USA). Samples were either diluted further or pipetted directly to a final volume of 50 μl in AMBIC, such that the final protein amount used for preparation of tryptic peptides was 7 μg. Tryptic peptides were prepared using In-Solution Tryptic Digestion and Guanidination Kit (Thermo Fisher Scientific) according to manufacturer's instructions but without the final guanidination step. After overnight digestions, formic acid was added ad 0.1% and incubated for 45 min at 37 °C to remove Rapigest SF, followed by centrifugation at 13
000 rpm for 15 min to remove particulate debris.
Nanoliquid chromatography tandem mass spectrometry
Samples were transferred into 250 μl autosampler microvials (Thermo Fisher Scientific) and loaded into an Easy-nLC 1200 (Thermo Fisher Scientific) coupled to an Orbitrap Fusion MS (Thermo Fisher Scientific). Chromatographic separation of about 100 ng of peptides was carried out in commercially packed Acclaim PepMap C18 columns (2 μm, 100 Å, 75 mm, 15 cm; Thermo Fisher Scientific). The peptides were loaded in buffer A (5% acetonitrile and 0.1% formic acid) and eluted with a 1 h linear gradient from 5% to 30% buffer B (80% acetonitrile and 0.1% formic acid). Three biological replicates were sequentially injected with two 15 min wash runs and a 1 h blank run alternated between distinct “treatments”. Mass spectra were acquired using a Top 20 data-dependent method with an automatic switch between full MS and MS/MS (MS2) scans. The Orbitrap analyser parameters for the full MS scan were resolution of 120
000 mass range of 350 to 1800 m/z, and AGC target of 4e5 ions, whereas those for MS2 spectra acquisition were resolution of 30
000, AGC target of 5e4 ions, with an isolation window of 2 m/z and dynamic exclusion of 30 s. Column chromatographic performance was routinely monitored with intermittent injections of 50 fmol of a commercially available BSA peptide mix (Bruker Corp., MA, USA), as well as evaluating double-wash runs for carry-over peptides.
Proteomics data processing and analysis
Protein groups identification and quantification were carried out with the MaxQuant97 software package v1.6.1.0, with the UniProtKB human FASTA file containing 86
725 entries to which 245 commonly observed contaminants and all reverse sequences were added. Each sample was injected 3 times, and the match between runs feature in MaxQuant was implemented to transfer identifications between technical replicates. Identification of co-fragmented peptides in all MS/MS spectra was enabled with the second peptides option in MaxQuant. All other peptide and protein identification parameters were used in their default settings in MaxQuant. Differential abundance analysis and hierarchical clustering were carried out with the Perseus98 data analysis software v. 1.5.6.0. Abundance values were log
2 transformed, protein identifications classified as being only identified by site, and reverse sequences and potential contaminants were filtered out from the main data frame. Additionally, only identifications with non-zero intensity values in all three biological replicates from at least one ‘treatment’ were retained for comparisons. Abundance intensities of identified proteins across each sample were median-normalized. For relative fold change estimation, missing intensity values of unidentified protein groups were replaced by random numbers drawn from a normal distribution to mimic low abundance measurements, using the impute from normal distribution function in Perseus. The lowest protein intensity values for each sample were assigned to the remaining proteins with missing quantification values to enable differential abundance estimation. Multiple hypothesis testing (ANOVA) was performed with a Benjamini–Hochberg FDR of 0.05. Only ANOVA-significant proteins were retained for two-sample comparisons via T-tests. Protein groups with a greater than 1.5-fold change in abundance between selected contrast sets were considered significantly different. Unless otherwise specified, all reported fold-changes are on a log
2 scale.
Pathway and additional data analysis
Gene set and functional enrichment analyses were carried out with Genemania (http://genemania.org/), EnrichR (http://amp.pharm.mssm.edu/Enrichr/) or the FunRich tool,99 as indicated. Additional data analysis and statistics was conducted with Prism 7.0d for Mac OS X (GraphPad Software Inc., La Jolla, CA, USA).
Conclusions
We present a workflow that combines in situ optical analysis of the protein corona by MP-SPR coupled with sensitive nLC-MS/MS analysis of the hard and soft corona subsections. The synthesis of a reusable pTHMMAA-BDA-biosensor for liposome capture and reduced sensor protein binding is presented. For proof-of-concept, corona formation on regular liposomes and light-triggered liposomes was studied under dynamic conditions in 100% human plasma, revealing significant formulation-dependent differences in the structure and composition of both corona subsections. Separate clusters for HC and SC suggest differences in the ensuing biological responses for these corona subtypes. The clinically-optimized formulation covered with PEG-ICG clusters (F2) formed a distinct SC signature; it contained proteins reported to mediate nanoparticle stealth properties, previously reported only in the HC. This highlights the importance of complementary soft corona characterization to obtain a holistic picture of the acquired biological identity. The relative enrichment of stealth-mediating proteins on all liposome surfaces suggests that lipids alone may induce their selective accumulation in the corona regardless of pegylation. Pegylated surfaces accumulated more molecules on their surfaces and formed thicker coronas, overperformed by the repellent properties of phosphatidylcholine groups on bare liposomes, but this “harvesting” may be necessary for the stealth effect mediated by hydrophilic coatings. While surface mass densities were similar for the corona subsections, thickness and protein-to-lipid values clearly discerned between the different formulations. Combined, both the physical structures and the proteomic compositions of the coronas were modulated by the surface coating of the liposome and the constituent lipid composition. Notably, this is the first time that truly complementary proteomic compositions for a hard and soft corona have been reported along with non-invasively obtained in situ physical parameters in one experiment. By facilitating studies on tailored, personalized, and artificial coronas with liposomes and lipid nanoparticles, the workflow has potential to advance nanomedical development and clinical translation especially in the field of drug delivery.
Conflicts of interest
There are no conflicts to declare.
Acknowledgements
The authors would like to thank Assistant Prof. Maria Pernemalm and Prof. Fredrik Höök for their valuable comments during the conduct of the research. The authors gratefully acknowledge financial support from Business Finland (LADDS, Light Activated Drug Delivery Systems, grant 4208/31/2015), the Academy of Finland (grant 1307088), the Ida Montin Foundation, the Inkeri and Mauri Vänskä Foundation, the Instrumentarium Science Foundation, and the Paulikki and Sakari Sohlberg Foundation.
References
- P. C. Ke, S. Lin, W. J. Parak, T. P. Davis and F. Caruso, ACS Nano, 2017, 11, 11773–11776 CrossRef CAS.
- G. Caracciolo, O. C. Farokhzad and M. Mahmoudi, Trends Biotechnol., 2017, 35, 257–264 CrossRef CAS PubMed.
- M. P. Monopoli, C. Åberg, A. Salvati, K. A. Dawson, C. Åberg, A. Salvati, K. A. Dawson, C. Aberg, A. Salvati and K. A. Dawson, Nat. Nanotechnol., 2012, 7, 779–786 CrossRef CAS PubMed.
- D. Docter, D. Westmeier, M. Markiewicz, S. Stolte, S. K. Knauer and R. H. Stauber, Chem. Soc. Rev., 2015, 44, 6094–6121 RSC.
- M. Kokkinopoulou, J. Simon, K. Landfester, V. Mailänder and I. Lieberwirth, Nanoscale, 2017, 9, 8858–8870 RSC.
- M. Hadjidemetriou and K. Kostarelos, Nat. Nanotechnol., 2017, 12, 288–290 CrossRef CAS PubMed.
- A. E. Nel, L. Mädler, D. Velegol, T. Xia, E. M. V. Hoek, P. Somasundaran, F. Klaessig, V. Castranova and M. Thompson, Nat. Mater., 2009, 8, 543–557 CrossRef CAS PubMed.
- B. Fadeel, H. Alenius and K. Savolainen, Toxicology, 2013, 313, 1–2 CrossRef CAS PubMed.
- D. Bonvin, U. Aschauer, D. T. L. Alexander, D. Chiappe, M. Moniatte, H. Hofmann and M. Mionić Ebersold, Small, 2017, 13, 1700409 CrossRef PubMed.
- S. Tenzer, D. Docter, J. Kuharev, A. Musyanovych, V. Fetz, R. Hecht, F. Schlenk, D. Fischer, K. Kiouptsi, C. Reinhardt, K. Landfester, H. Schild, M. Maskos, S. K. Knauer and R. H. Stauber, Nat. Nanotechnol., 2013, 8, 772–781 CrossRef CAS PubMed.
- M. Hadjidemetriou, Z. Al-Ahmady and K. Kostarelos, Nanoscale, 2016, 8, 6948–6957 RSC.
- A. Amici, G. Caracciolo, L. Digiacomo, V. Gambini, C. Marchini, M. Tilio, A. L. Capriotti, V. Colapicchioni, R. Matassa, G. Familiari, S. Palchetti, D. Pozzi, M. Mahmoudi and A. Laganà, RSC Adv., 2017, 7, 1137–1145 RSC.
- M. Hadjidemetriou, Z. Al-Ahmady, M. Mazza, R. F. Collins, K. Dawson and K. Kostarelos, ACS Nano, 2015, 9, 8142–8156 CrossRef CAS PubMed.
- M. Hadjidemetriou, S. McAdam, G. Garner, C. Thackeray, D. Knight, D. Smith, Z. Al-Ahmady, M. Mazza, J. Rogan, A. Clamp and K. Kostarelos, Adv. Mater., 2019, 31, 1803335 CrossRef PubMed.
- F. Giulimondi, L. Digiacomo, D. Pozzi, S. Palchetti, E. Vulpis, A. L. Capriotti, R. Z. Chiozzi, A. Laganà, H. Amenitsch, L. Masuelli, M. Mahmoudi, I. Screpanti, A. Zingoni and G. Caracciolo, Nat. Commun., 2019, 10, 3686 CrossRef PubMed.
- J. Lazarovits, S. Sindhwani, A. J. Tavares, Y. Zhang, F. Song, J. Audet, J. R. Krieger, A. M. Syed, B. Stordy and W. C. W. Chan, ACS Nano, 2019, 13, 8023–8034 CrossRef CAS PubMed.
- S. Palchetti, V. Colapicchioni, L. Digiacomo, G. Caracciolo, D. Pozzi, A. L. Capriotti, G. La Barbera and A. Laganà, Biochim. Biophys. Acta, Biomembr., 2016, 1858, 189–196 CrossRef CAS PubMed.
- M. Mahmoudi, A. M. Abdelmonem, S. Behzadi, J. H. Clement, S. Dutz, M. R. Ejtehadi, R. Hartmann, K. Kantner, U. Linne, P. Maffre, S. Metzler, M. K. Moghadam, C. Pfeiffer, M. Rezaei, P. Ruiz-Lozano, V. Serpooshan, M. A. Shokrgozar, G. U. Nienhaus and W. J. Parak, ACS Nano, 2013, 7, 6555–6562 CrossRef CAS PubMed.
- S. Schöttler, K. Klein, K. Landfester and V. Mailänder, Nanoscale, 2016, 8, 5526–5536 RSC.
- T. Cedervall, I. Lynch, S. Lindman, T. Berggard, E. Thulin, H. Nilsson, K. a. Dawson and S. Linse, Proc. Natl. Acad. Sci. U. S. A., 2007, 104, 2050–2055 CrossRef CAS PubMed.
- M. Pernemalm and J. Lehtiö, Expert Rev. Proteomics, 2014, 11, 431–448 CrossRef CAS PubMed.
- J. Ren, R. Cai, J. Wang, M. Daniyal, D. Baimanov, Y. Liu, D. Yin, Y. Liu, Q. Miao, Y. Zhao and C. Chen, Nano Lett., 2019, 19, 4692–4701 CrossRef CAS PubMed.
- M. J. Hajipour, J. Raheb, O. Akhavan, S. Arjmand, O. Mashinchian, M. Rahman, M. Abdolahad, V. Serpooshan, S. Laurent and M. Mahmoudi, Nanoscale, 2015, 7, 8978–8994 RSC.
- M. J. Hajipour, S. Laurent, A. Aghaie, F. Rezaee and M. Mahmoudi, Biomater. Sci., 2014, 2, 1210–1221 RSC.
- C. Corbo, R. Molinaro, M. Tabatabaei, O. C. Farokhzad and M. Mahmoudi, Biomater. Sci., 2017, 5, 378–387 RSC.
- G. Caracciolo, D. Caputo, D. Pozzi, V. Colapicchioni and R. Coppola, Colloids Surf., B, 2014, 123, 673–678 CrossRef CAS PubMed.
- S. Winzen, S. Schoettler, G. Baier, C. Rosenauer, V. Mailaender, K. Landfester and K. Mohr, Nanoscale, 2015, 7, 2992–3001 RSC.
- N. Granqvist, M. Yliperttula, S. Välimäki, P. Pulkkinen, H. Tenhu and T. Viitala, Langmuir, 2014, 30, 2799–2809 CrossRef CAS PubMed.
- N. Granqvist, H. Liang, T. Laurila, J. Sadowski, M. Yliperttula and T. Viitala, Langmuir, 2013, 29, 8561–8571 CrossRef CAS PubMed.
-
W. M. Albers and I. Vikholm-Lundin, in Nano-Bio-Sensing, Springer, 2011, pp. 83–125 Search PubMed.
- O. K. Kari, T. Rojalin, S. Salmaso, M. Barattin, H. Jarva, S. Meri, M. Yliperttula, T. Viitala and A. Urtti, Drug Delivery Transl. Res., 2017, 7, 228–240 CrossRef CAS PubMed.
- I. Vikholm-Lundin, R. Piskonen and W. M. Albers, Biosens. Bioelectron., 2007, 22, 1323–1329 CrossRef CAS PubMed.
- W. M. Albers, T. Munter, P. Laaksonen and I. Vikholm-Lundin, J. Colloid Interface Sci., 2010, 348, 1–8 CrossRef CAS PubMed.
- T. Lajunen, L.-S. Kontturi, L. Viitala, M. Manna, O. Cramariuc, T. Róg, A. Bunker, T. Laaksonen, T. Viitala, L. Murtomäki and A. Urtti, Mol. Pharm., 2016, 13, 2095–2107 CrossRef CAS PubMed.
- M. A. Cooper, A. Hansson, S. Löfås and D. H. Williams, Anal. Biochem., 2000, 277, 196–205 CrossRef CAS PubMed.
- G. Anderluh, M. Beseničar, A. Kladnik, J. H. Lakey and P. Maček, Anal. Biochem., 2005, 344, 43–52 CrossRef CAS PubMed.
- R. M. Crist, J. H. Grossman, A. K. Patri, S. T. Stern, M. a. Dobrovolskaia, P. P. Adiseshaiah, J. D. Clogston and S. E. McNeil, Integr. Biol., 2013, 5, 66–73 CrossRef CAS PubMed.
- T. Lajunen, R. Nurmi, D. Wilbie, T. Ruoslahti, N. G. Johansson, O. Korhonen, T. Rog, A. Bunker, M. Ruponen and A. Urtti, J. Controlled Release, 2018, 284, 213–223 CrossRef CAS PubMed.
- J. R. Ashton, J. L. West and C. T. Badea, Front. Pharmacol., 2015, 6, 256 Search PubMed.
- EMBL-EBI, ChEMBL compound properties for Indocyanine Green, https://www.ebi.ac.uk/chembldb/compound/inspect/CHEMBL1646.
- M. L. Landsman, G. Kwant, G. A. Mook and W. G. Zijlstra, J. Appl. Physiol., 1976, 40, 575–583 CrossRef CAS PubMed.
- J. F. Zhou, Proc. SPIE, 1994, 2128, 495–505 CrossRef.
- W. Knoll, Annu. Rev. Phys. Chem., 1998, 49, 569–638 CrossRef CAS PubMed.
- J. Homola, S. S. Yee and G. Gauglitz, Sens. Actuators, B, 1999, 54, 3–15 CrossRef CAS.
-
W. M. Albers and I. Vikholm-Lundin, Nano-Bio-Sensing, Springer, 2011, pp. 83–125 Search PubMed.
- F. Barbero, L. Russo, M. Vitali, J. Piella, I. Salvo, M. L. Borrajo, M. Busquets-Fité, R. Grandori, N. G. Bastús, E. Casals and V. Puntes, Semin. Immunol., 2017, 34, 52–60 CrossRef CAS.
- G. Zhang, Phys. Rev. E, 2018, 97, 1–9 Search PubMed.
- J. Wolfram, K. Suri, Y. Yang, J. Shen, C. Celia, M. Fresta, Y. Zhao, H. Shen and M. Ferrari, Colloids Surf., B, 2014, 114, 294–300 CrossRef CAS PubMed.
- H. Soo Choi, W. Liu, P. Misra, E. Tanaka, J. P. Zimmer, B. Itty Ipe, M. G. Bawendi and J. V. Frangioni, Nat. Biotechnol., 2007, 25, 1165–1170 CrossRef PubMed.
- M. Hadjidemetriou and K. Kostarelos, Nat. Nanotechnol., 2017, 12, 288–290 CrossRef CAS PubMed.
- F. Wang, L. Yu, M. P. Monopoli, P. Sandin, E. Mahon, A. Salvati and K. A. Dawson, Nanomedicine, 2013, 9, 1159–1168 CrossRef CAS PubMed.
- B. Nilsson, K. N. Ekdahl, T. E. Mollnes and J. D. Lambris, Mol. Immunol., 2007, 44, 82–94 CrossRef CAS PubMed.
- M. V. Carroll and R. B. Sim, Adv. Drug Delivery Rev., 2011, 1–11 Search PubMed.
- D. Walczyk, F. B. Bombelli, M. P. Monopoli, I. Lynch and K. a. Dawson, J. Am. Chem. Soc., 2010, 132, 5761–5768 CrossRef CAS PubMed.
- C. Röcker, M. Pötzl, F. Zhang, W. J. Parak and G. U. Nienhaus, Nat. Nanotechnol., 2009, 4, 577–580 CrossRef PubMed.
- M. P. Monopoli, D. Walczyk, A. Campbell, G. Elia, I. Lynch, F. Baldelli Bombelli and K. A. Dawson, J. Am. Chem. Soc., 2011, 133, 2525–2534 CrossRef CAS PubMed.
- D. Pozzi, V. Colapicchioni, G. Caracciolo, S. Piovesana, A. L. Capriotti, S. Palchetti, S. De Grossi, A. Riccioli, H. Amenitsch and A. Laganà, Nanoscale, 2014, 6, 2782–2792 RSC.
- N. Bertrand, P. Grenier, M. Mahmoudi, E. M. Lima, E. A. Appel, F. Dormont, J.-M. Lim, R. Karnik, R. Langer and O. C. Farokhzad, Nat. Commun., 2017, 8, 777 CrossRef PubMed.
- D. Docter, U. Distler, W. Storck, J. Kuharev, D. Wünsch, A. Hahlbrock, S. K. Knauer, S. Tenzer and R. H. Stauber, Nat. Protoc., 2014, 9, 2030–2044 CrossRef CAS PubMed.
- A. Amici, G. Caracciolo, L. Digiacomo, V. Gambini, C. Marchini, M. Tilio, A. L. Capriotti, V. Colapicchioni, R. Matassa, G. Familiari, S. Palchetti, D. Pozzi, M. Mahmoudi and A. Laganà, RSC Adv., 2017, 7, 1137–1145 RSC.
- M. Neagu, Z. Piperigkou, K. Karamanou, A. B. Engin, A. O. Docea, C. Constantin, C. Negrei, D. Nikitovic and A. Tsatsakis, Arch. Toxicol., 2017, 91, 1031–1048 CrossRef CAS PubMed.
- T. Cedervall, I. Lynch, M. Foy, T. Berggård, S. C. Donnelly, G. Cagney, S. Linse and K. A. Dawson, Angew. Chem., Int. Ed., 2007, 46, 5754–5756 CrossRef CAS PubMed.
- A. L. Barrán-Berdón, D. Pozzi, G. Caracciolo, A. L. Capriotti, G. Caruso, C. Cavaliere, A. Riccioli, S. Palchetti and A. Lagana, Langmuir, 2013, 29, 6485–6494 CrossRef PubMed.
- D. Pozzi, V. Colapicchioni, G. Caracciolo, S. Piovesana, A. L. Capriotti, S. Palchetti, S. De Grossi, A. Riccioli, H. Amenitsch and A. Laganà, Nanoscale, 2014, 6, 2782 RSC.
- D. Pozzi, G. Caracciolo, L. Digiacomo, V. Colapicchioni, S. Palchetti, A. L. Capriotti, C. Cavaliere, R. Zenezini Chiozzi, A. Puglisi and A. Laganà, Nanoscale, 2015, 7, 13958–13966 RSC.
- M. Lundqvist, J. Stigler, G. Elia, I. Lynch, T. Cedervall and K. a. Dawson, Proc. Natl. Acad. Sci. U. S. A., 2008, 105, 14265–14270 CrossRef CAS PubMed.
- L. Digiacomo, F. Cardarelli, D. Pozzi, S. Palchetti, M. A. Digman, E. Gratton, A. L. Capriotti, M. Mahmoudi and G. Caracciolo, Nanoscale, 2017, 9, 17254–17262 RSC.
- T. T. Hochgrebe, D. Humphreys, M. R. Wilson and S. B. Easterbrook-Smith, Exp. Cell Res., 1999, 249, 13–21 CrossRef CAS PubMed.
- I. Hamad, O. Al-Hanbali, A. C. Hunter, K. J. Rutt, T. L. Andresen and S. M. Moghimi, ACS Nano, 2010, 4, 6629–6638 CrossRef CAS PubMed.
- D. Pozzi, G. Caracciolo, L. Digiacomo, V. Colapicchioni, S. Palchetti, A. L. Capriotti, C. Cavaliere, R. Zenezini Chiozzi, A. Puglisi and A. Laganà, Nanoscale, 2015, 7, 13958–13966 RSC.
-
M. A. Dobrovolskaia and B. W. Neun, NCL Method ITA-5.2 Quantitative Analysis of Complement Activation, Nanotechnology Characterization Laboratory, National Cancer Institute, Frederick, 2010, pp. 1–10 Search PubMed.
- D. E. Owens and N. a. Peppas, Int. J. Pharm., 2006, 307, 93–102 CrossRef CAS PubMed.
- R. Gref, M. Lück, P. Quellec, M. Marchand, E. Dellacherie, S. Harnisch, T. Blunk and R. Müller, Colloids Surf., B, 2000, 18, 301–313 CrossRef CAS.
- S. Schöttler, G. Becker, S. Winzen, T. Steinbach, K. Mohr, K. Landfester, V. Mailänder and F. R. Wurm, Nat. Nanotechnol., 2016, 11, 372–377 CrossRef PubMed.
- W. R. Gombotz, W. Guanghui, T. A. Horbett and A. S. Hoffman, J. Biomed. Mater. Res., 1991, 25, 1547–1562 CrossRef CAS PubMed.
- M. He, K. Gao, L. Zhou, Z. Jiao, M. Wu, J. Cao, X. You, Z. Cai, Y. Su and Z. Jiang, Acta Biomater., 2016, 40, 142–152 CrossRef CAS PubMed.
- Y. Jiang, Y. Su, L. Zhao, F. Meng, Q. Wang, C. Ding, J. Luo and J. Li, Colloids Surf., B, 2017, 156, 87–94 CrossRef CAS PubMed.
- C. Leng, S. Sun, K. Zhang, S. Jiang and Z. Chen, Acta Biomater., 2016, 40, 6–15 CrossRef CAS PubMed.
- G. Settanni, J. Zhou, T. Suo, S. Schöttler, K. Landfester, F. Schmid and V. Mailänder, Nanoscale, 2017, 9, 2138–2144 RSC.
- G. Settanni, T. Schäfer, C. Muhl, M. Barz and F. Schmid, Comput. Struct. Biotechnol. J., 2018, 16, 543–550 CrossRef CAS PubMed.
- S. Giovanni, Z. Jiajia and S. Friederike, J. Phys.: Conf. Ser., 2017, 921, 12002 CrossRef.
- S. R. Matukumalli, R. Tangirala and C. M. Rao, Sci. Rep., 2017, 7, 1–13 CrossRef PubMed.
- L. Shang and G. U. Nienhaus, Acc. Chem. Res., 2017, 50, 387–395 CrossRef CAS PubMed.
- M. Carril, D. Padro, P. Del Pino, C. Carrillo-Carrion, M. Gallego and W. J. Parak, Nat. Commun., 2017, 8, 1542 CrossRef PubMed.
- C. Weber, J. Simon, V. Mailänder, S. Morsbach and K. Landfester, Acta Biomater., 2018, 76, 217–224 CrossRef CAS.
- S. Schöttler, K. Landfester and V. Mailänder, Angew. Chem., Int. Ed., 2016, 8806–8815 CrossRef.
- L. Treuel, S. Brandholt, P. Maffre, S. Wiegele, L. Shang and G. U. Nienhaus, ACS Nano, 2014, 8, 503–513 CrossRef CAS.
- H. Vlassara and M. R. Palace, J. Intern. Med., 2002, 251, 87–101 CrossRef CAS PubMed.
- A. Chonn, S. C. Semple and P. R. Cullis, J. Biol. Chem., 1992, 267, 18759–18765 CAS.
- C. D. Oja, S. C. Semple, A. Chonn and P. R. Cullis, Biochim. Biophys. Acta, Biomembr., 1996, 1281, 31–37 CrossRef.
- S. C. Semple, A. Chonn and P. R. Cullis, Biochemistry, 1996, 35, 2521–2525 CrossRef CAS PubMed.
-
M. M. Julkowska, J. M. Rankenberg and C. Testerink, in Methods in molecular biology, Clifton, N.J, 2013, vol. 1009, pp. 261–271 Search PubMed.
- M. Andersson, J. Andersson, A. Sellborn, M. Berglin, B. Nilsson and H. Elwing, Biosens. Bioelectron., 2005, 21, 79–86 CrossRef CAS.
- A. M. Nyström and B. Fadeel, J. Controlled Release, 2012, 161, 403–408 CrossRef PubMed.
- J. Müller, D. Prozeller, A. Ghazaryan, M. Kokkinopoulou, V. Mailänder, S. Morsbach and K. Landfester, Acta Biomater., 2018, 71, 420–431 CrossRef PubMed.
- L. S. Jung, C. T. Campbell, T. M. Chinowsky, M. N. Mar and S. S. Yee, Langmuir, 1998, 14, 5636–5648 CrossRef CAS.
- J. Cox, M. Y. Hein, C. A. Luber, I. Paron, N. Nagaraj and M. Mann, Mol. Cell. Proteomics, 2014, 13, 2513–2526 CrossRef CAS PubMed.
- S. Tyanova, T. Temu, P. Sinitcyn, A. Carlson, M. Y. Hein, T. Geiger, M. Mann and J. Cox, Nat. Methods, 2016, 13, 731–740 CrossRef CAS PubMed.
- M. Pathan, S. Keerthikumar, C. S. Ang, L. Gangoda, C. Y. J. Quek, N. A. Williamson, D. Mouradov, O. M. Sieber, R. J. Simpson, A. Salim, A. Bacic, A. F. Hill, D. A. Stroud, M. T. Ryan, J. I. Agbinya, J. M. Mariadason, A. W. Burgess and S. Mathivanan, Proteomics, 2015, 15, 2597–2601 CrossRef CAS PubMed.
Footnotes |
† Electronic supplementary information (ESI) available: Biophysical analysis and sensor details, liposome characterization, replicate data, additional proteomics results, and MIRIBEL checklist. See DOI: 10.1039/c9nr08186k |
‡ Current address: Laboratory of Pharmaceutical Technology, Department of Pharmaceutical Science, Tokyo University of Pharmacy & Life Sciences, Tokyo 192-0392, Japan. |
§ Current address: Division of Pharmaceutical Chemistry and Technology, Faculty of Pharmacy, University of Helsinki, P.O.B. 56, 00014 Helsinki, Finland. |
|
This journal is © The Royal Society of Chemistry 2020 |
Click here to see how this site uses Cookies. View our privacy policy here.