DOI:
10.1039/D0MT00008F
(Paper)
Metallomics, 2020,
12, 876-890
Proteomic analysis of the S. cerevisiae response to the anticancer ruthenium complex KP1019
Received
8th January 2020
, Accepted 18th March 2020
First published on 24th April 2020
Abstract
Like platinum-based chemotherapeutics, the anticancer ruthenium complex indazolium trans-[tetrachlorobis(1H-indazole)ruthenate(III)], or KP1019, damages DNA, induces apoptosis, and causes tumor regression in animal models. Unlike platinum-based drugs, KP1019 showed no dose-limiting toxicity in a phase I clinical trial. Despite these advances, the mechanism(s) and target(s) of KP1019 remain unclear. For example, the drug may damage DNA directly or by causing oxidative stress. Likewise, KP1019 binds cytosolic proteins, suggesting DNA is not the sole target. Here we use the budding yeast Saccharomyces cerevisiae as a model in a proteomic study of the cellular response to KP1019. Mapping protein level changes onto metabolic pathways revealed patterns consistent with elevated synthesis and/or cycling of the antioxidant glutathione, suggesting KP1019 induces oxidative stress. This result was supported by increased fluorescence of the redox-sensitive dye DCFH-DA and increased KP1019 sensitivity of yeast lacking Yap1, a master regulator of the oxidative stress response. In addition to oxidative and DNA stress, bioinformatic analysis revealed drug-dependent increases in proteins involved ribosome biogenesis, translation, and protein (re)folding. Consistent with proteotoxic effects, KP1019 increased expression of a heat-shock element (HSE) lacZ reporter. KP1019 pre-treatment also sensitized yeast to oxaliplatin, paralleling prior research showing that cancer cell lines with elevated levels of translation machinery are hypersensitive to oxaliplatin. Combined, these data suggest that one of KP1019's many targets may be protein metabolism, which opens up intriguing possibilities for combination therapy.
Significance to metallomics
The ruthenium complex KP1019 has promising anticancer properties, yet its mechanisms of action are only partially understood. Using budding yeast as a model system, here we report the first global proteomic analysis examining the cellular response to this drug. One of the strongest signatures revealed by this work was elevated levels of proteins involved in ribosomal biogenesis (ribi) and translation, suggesting that KP1019 perturbs protein homeostasis. Previous studies showed that enhanced ribi gene expression is associated with increased sensitivity to oxaliplatin. Our observation of synergy between KP1019 and oxaliplatin suggests future promise for combination therapy.
|
Introduction
Certain metals, including iron and copper, serve essential functions in cells. However, at high levels, many metals are toxic. Although mechanisms of toxicity can vary, leading to divergent cellular responses,1,2 many metals have been shown to induce oxidative stress and DNA damage.3–6 Some metals have also been shown to induce protein misfolding and aggregation.7
In recent decades, the toxicity of a diverse array of metals has been harnessed in the production of anti-cancer drugs.8–11 Early examples include cisplatin and carboplatin, which are often used to treat testicular and ovarian cancers, as well as head and neck tumours. More recently, oxaliplatin has emerged as an effective treatment for gastrointestinal cancers,12,13 and it may be particularly effective in the context of combination therapies.14 Unfortunately, the utility of platinum-based drugs can be hindered by dose-limiting toxicity and development of drug resistance.15–17
Though not yet approved for regular use, three ruthenium complexes – NAMI-A, KP1019, and KP1339 – show significant promise in preclinical studies and early clinical trials.18,19 For example, indazolium trans-[tetrachlorobis(1H-indazole) ruthenate(III)], or KP1019 (Fig. 1), displays no dose-limiting toxicity20,21 and maintains potency against drug resistant cell lines.22 KP1019 caused tumour regression in animal models,23 and in a phase I clinical trial, the drug stabilized disease progression in five of six evaluable patients.20,21 However, this drug's mechanism of action remains poorly understood. In colorectal cancer cells, KP1019 has been shown to induce oxidative stress and DNA damage,24 yet in cervical carcinoma cells, the drug bound primarily to proteins in the cytosolic fraction.25 Furthermore, KP1019 not only bound genomic DNA but also accumulated in mitochondria of ovarian cancer cells.26
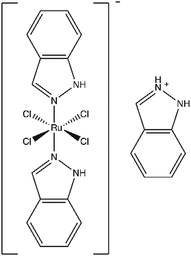 |
| Fig. 1 Structure of KP1019 (indazolium trans-[tetrachlorobis(1H-indazole)ruthenate(III)]). | |
Consistent with findings in cancer cell lines, KP1019 has been shown to inhibit growth, induce cell death, and damage DNA in the budding yeast Saccharomyces cerevisiae.27–29 Moreover, the drug bound readily with cytoplasmic proteins and mitochondria of fractionated yeast cells.30 KP1019's ability to induce stress is also consistent with its ability to activate the evolutionarily conserved stress-responsive kinase Hog131 and to increase lipid droplet formation.32 Combined, these studies suggest that KP1019 has multiple modes of action in yeast, much like it has in cultured mammalian cells. Thus, budding yeast appears to be an appropriate model organism for better characterizing KP1019's impact on cells.
When working with poorly characterized drugs, unbiased genome-wide approaches can help establish the targets of bioactive compounds.33,34 For example, transcriptional profiling of yeast helped uncover quinine's ability to perturb glucose transport35 and contributed to establishment of calcium mobility as a mechanism of action for the antifungal terpenoid phenol carvacrol.36
Given the success of such global approaches, we have used mass spectrometry to analyse the effects of KP1019 on the budding yeast proteome. Our results support previous findings, verifying activation of the DNA damage response, while revealing new physiological responses to the drug, such as morphogenesis, metabolic re-tooling, and induction of ribosomal biogenesis.
Materials and methods
Yeast strains and growth conditions
Yeast strains used in this study are listed in Table 1. Yeast were grown under standard conditions, at 30 °C using the rich medium yeast extract peptone dextrose (YPD) (1% yeast extract, 2% bacto-peptone, 2% dextrose) or synthetic complete media (SDC) as indicated.39 For experiments involving plasmids, a standard lithium acetate transformation protocol40 was used, and transformants were selected on minimal media lacking the component necessary for plasmid maintenance.
Table 1 Yeast strains used in this study
Strain |
Genotype |
Ref. |
BY 4742 |
MATα his3Δ1 leu2Δ0 lys2Δ0 ura3Δ0
|
Winzeler et al., 199937 |
yap1Δ
|
yap1::kanMX In BY4742 |
Winzeler et al., 199937 |
dun1Δ
|
dun1::kanMX In BY4742 |
Winzeler et al., 199937 |
ddc1Δ
|
ddc1::kanMX In BY4742 |
Winzeler et al., 199937 |
L4921 |
MAT a/α ura3-52/ura3-52 (Σ1278b strain background) |
Gimeno et al., 199238 |
Drug synthesis
KP1019 was synthesized using a protocol described previously27 and adapted from Lipponer et al.41 Briefly, 1 g RuCl3 × 3H2O was added to 20 ml of 12 M HCl and 20 ml of ethanol and refluxed for 1 hour. Once the reaction mixture had cooled, the ethanol was removed using a rotary evaporator, and 12 M HCl was added to give a final volume of 40 ml. The ruthenium solution was combined with 3.74 g of indazole that had been dissolved in 60 ml of 12 M HCl at 70 °C. This reaction mixture was heated for 15 minutes at 80–90 °C and cooled to room temperature with stirring. The resulting solid was filtered from the solution, and then stirred in approximately 150 ml of H2O at room temperature for 2 hours. The solid was filtered and subsequently washed with cold ethanol followed by cold diethyl ether. The drug was dried under vacuum for 18–24 hours. KP1019 purity was verified by UV-visible spectroscopy, elemental analysis and cyclic voltammetry.
Proteomic analysis
Proteomic analysis was carried out as previously,42 with minor changes included below.
Sample preparation.
For each of three biological replicates, 250 ml of wild-type yeast (BY 4742) were grown to mid-log phase (OD600 0.5–1.0) at 30 °C in SDC. The culture was then split and half was treated with 80 μg ml−1 KP1019 for 3 hours; the other half was left untreated. Samples were washed with water and cell concentrations were normalized. Cells were then pelleted and stored at −80 °C. The Y-PER Yeast Protein Extraction Reagent (Thermo Fisher, Waltham, MA) was used to extract proteins from the pellets. The protein fractions were quantified using Pierce BCA Protein Assay Kit (Thermo Fisher Scientific, Cat.# PI23225), ∼20 μg of protein per sample were then diluted to 35 μl using NuPAGE LDS sample buffer (1× final conc., Invitrogen, Cat.#NP0007). Proteins were then reduced with DTT and denatured at 70 °C for 10 min prior to loading everything onto Novex NuPAGE 10% Bis–Tris protein gels (Invitrogen, Cat.# NP0315BOX) and separated as a short stack (10 min at 200 constant V). The gels were stained overnight with Novex Colloidal Blue Staining kit (Invitrogen, Cat.# LC6025). Following de-staining, each lane was cut into single MW fractions and equilibrated in 100 mM ammonium bicarbonate (AmBc), each gel plug was then digested overnight with Trypsin Gold, Mass Spectrometry Grade (Promega, Cat.# V5280) following manufacturer's instruction. Peptide extracts were reconstituted in 0.1% formic acid/ddH2O at 0.1 μg μl−1.
Mass spectrometry.
Peptide digests (8 μL each) were injected onto a 1260 Infinity nHPLC stack (Agilent Technologies), and separated using a 75 micron I.D. × 15 cm pulled tip C-18 column (Jupiter C-18 300 Å, 5 micron, Phenomenex). This system runs in-line with a Thermo Orbitrap Velos Pro hybrid mass spectrometer, equipped with a nano-electrospray source (Thermo Fisher Scientific), and all data were collected in CID mode. The nHPLC was configured with binary mobile phases that included solvent A (0.1% FA in ddH2O), and solvent B (0.1% FA in 15% ddH2O/85% ACN), programmed as follows; 10 min @ 5%B (2 μl min−1, load), 90 min @ 5–40% B (linear: 0.5 nl min−1, analyse), 5 min @ 70% B (2 μl min−1, wash), 10 min @ 0% B (2 μl min−1, equilibrate). Following each parent ion scan (300–1200 m/z @ 60k resolution), fragmentation data (MS2) was collected on the top most intense 15 ions. For data dependent scans, charge state screening and dynamic exclusion were enabled with a repeat count of 2, repeat duration of 30 s, and exclusion duration of 90 s.
MS data conversion and searches.
The XCalibur RAW files were collected in profile mode, centroided and converted to MzXML using ReAdW v. 3.5.1. The mgf files were then be created using MzXML2Search (included in TPP v. 3.5) for all scans. The data was searched using SEQUEST, which was set for two maximum missed cleavages, a precursor mass window of 20 ppm, trypsin digestion, variable modification C @ 57.0293, and M @ 15.9949. Searches were performed with a species specific subset of the UniRef100 database.
Peptide filtering, grouping, and quantification.
The list of peptide IDs generated based on SEQUEST (Thermo Fisher Scientific) search results were filtered using Scaffold (Protein Sciences, Portland Oregon). Scaffold filters and groups all peptides to generate and retain only high confidence IDs while also generating normalized spectral counts (N-SC's) across all samples for the purpose of relative quantification. The filter cut-off values were set with minimum peptide length of >5 AA's, with no MH + 1 charge states, with peptide probabilities of >80% C.I., and with the number of peptides per protein ≥2. The protein probabilities were then set to a >99.0% C.I., and an FDR < 1.0. Scaffold incorporates the two most common methods for statistical validation of large proteome datasets, the false discovery rate (FDR) and protein probability.43–45 Relative quantification across experiments were then performed via spectral counting,46,47 and when relevant, spectral count abundances were then normalized between samples.48
Systems analysis.
Gene ontology assignments, clustering, and pathway analysis were carried out using Search Tool for Recurring Instances of Neighbouring Genes (STRING; https://string-db.org/),49 Protein ANalysis THrough Evolutionary Relationships (PANTHER; http://www.pantherdb.org/),50 and YeastMine (http://yeastmine.yeastgenome.org).51
Filamentation assay
A wild-type strain from the filamentation competent Σ1278b background52,53 was cultured overnight in SDC then spread onto SDC media containing the indicated concentrations of KP1019. After an 18 hour incubation at 30 °C colony morphology was documented using a Zeiss Axioskop 2 plus with Zeiss Axiovision software (Carl Zeiss AG, Oberkochen, Germany).
Oxidative stress assay
Wild-type yeast were cultured to mid-log phase then incubated with 50 μM 2′,7′-dichlorodihydrofluorescein diacetate (DCFH-DA; Sigma-Aldrich, St. Louis, MO) for 1.5 hours at 30 °C to pre-load the cells with the redox sensitive dye. The samples were then treated with 0 or 80 μg ml−1 KP1019 or 10 mM H2O2 for 2.5 hours. Samples were then analysed by flow cytometry using a BD Accuri C6 Flow Cytometer (Becton Dickson, Franklin Lakes, NJ). 10
000 events were collected for each sample.
Beta-galactosidase assays
The genotoxicity and proteotoxicity of KP1019 were verified using wild-type yeast strain BY4742 transformed with the pZZ2 RNR3-lacZ or HSE-lacZ reporter constructs, respectively.54,55 Specifically, transformed cells were cultured to mid-log phase (OD600 0.5–1.0) in selective media then treated with KP1019 dissolved in the same type of medium. Samples were incubated for 3 hours to allow for gene induction, at which point β-galactosidase activity was measured using the permeabilized cell assay described by Guarente.56
Heat shock assay
The heat shock sensitivity assay was adapted from Wang et al.57 Briefly, yeast were cultured to mid-log phase at 30 °C in SDC then treated with the indicated concentration of KP1019 for 2 hours. Cells were then washed with SDC and cell concentrations were normalized. Samples were then incubated at 52 °C, and aliquots were removed and pipetted onto YPD at the indicated times. Following a 48 hour incubation at 30 °C, images were captured using a scanner.
Drug sensitivity and synergy assays
Drug-containing SDC was subjected to 2-fold serial dilution across the wells of a microtitre plate. Overnight cultures of yeast were diluted to OD600 0.1 and subsequently diluted 20-fold more in SDC. An equal volume of diluted cell suspension was added to each well of the microtitre plate. After an 18 to 24 hour incubation at 30 °C, the growth of each strain at each concentration of drug was recorded as absorbance at 630 nm using a BioTek (Winooski, VT) microtitre plate reader. Data were fit with a sigmoidal 4-parameter logistic curve to determine the concentration of drug that inhibited growth by 50%.
For the synergy assay, overnight cultures of yeast were sub-cultured to mid-log phase (OD600 0.5–1.0) in SDC prior to transferring the cells to SDC containing the indicated concentrations of KP1019. Following a three-hour incubation with the drug, cells were harvested by centrifugation then resuspended in drug-free SDC. Samples were then normalized to OD600 0.1 and subsequently diluted 10-fold more in SDC. One part yeast suspension was then added to three parts oxaliplatin in SDC to achieve the desired final concentration of platinum drug. After an 18 to 24 hour incubation at 30 °C, yeast growth at each concentration of drug was recorded as described above. The resulting data were analysed using SynergyFinder.58
Results
Analysis of KP1019-dependent changes in protein abundance
To characterize the cellular response to the anticancer ruthenium complex KP1019, we used a proteomic approach to measure changes in protein abundance in yeast treated with 80 μg ml−1 KP1019, a concentration used in a prior transcriptomic study29 and previously shown to robustly inhibit yeast growth and arrest the cell cycle while causing only modest amounts of cell death.27 The yeast proteome was analysed after incubating the cells with KP1019 for 3 hours,59 the same duration of exposure used in prior transcriptomic studies of this drug.29,32 Ultimately, 3676 different proteins were detected across three biological replicates. To determine which proteins displayed a significant change in expression level, a student's t-test was performed and proteins with p-values less than 0.05 were considered to display statistically significant differences in abundance. In an attempt to enrich for physiologically significant changes in protein levels, we limited further analysis to proteins with a 1.5-fold or greater increase in abundance or a 25% or greater decrease in abundance, consistent with a prior study of stress-dependent changes in protein levels in yeast.60 Using these criteria, levels of 230 proteins increased and 200 proteins decreased following a three-hour incubation with the drug (Fig. 2). Amongst these 430 proteins, fold change ranged from a high of 19-fold induction for the heat shock protein Hsp104, which disaggregates proteins misfolded during stress,61,62 to a low of 24-fold repression for the spore wall protein Irc18.
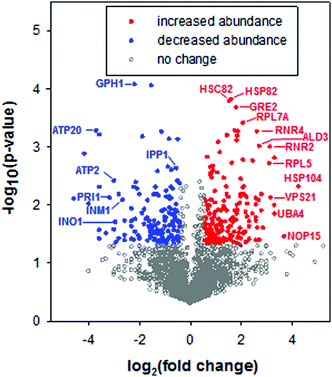 |
| Fig. 2 Volcano plot of proteomic data. Statistical significance was determined by a t-test across three biological replicates. Filled red circles correspond to proteins with statistically significant (p < 0.05) and ≥1.5 fold increases in abundance following a 3 hour treatment with the anticancer ruthenium complex KP1019. Filled blue circles correspond to proteins with statistically significant (p < 0.05) and ≥25% decreases in abundance. Open grey symbols correspond to proteins that did not meet these thresholds. Twenty-seven proteins were not detected in any of the no drug replicates but were detected in all of the drug trials; these proteins are not depicted in the volcano plot, due to this “infinite” fold change. However, these proteins were included in subsequent bioinformatics analyses. Similarly, 21 significantly (p < 0.05) repressed proteins that were not detected in any of the drug trials were included in bioinformatics analyses but are not included in the volcano plot. | |
To visualize networks of proteins impacted by KP1019, STRING was used to map each set of proteins (induced vs. repressed) onto the yeast interactome (Fig. 3A and 4A). Within STRING, clusters of proteins were identified using the MCL algorithm set to an inflation of 2.5, as a means of better separating clusters with distinct functions. The networks of increased and reduced abundance proteins both had significantly more interactions than expected with protein–protein interaction (PPI) enrichment p-values of 2 × 10−6 and 4 × 10−5, respectively. However, the proteins of increased abundance tended to be grouped into larger clusters containing up to 18 proteins (Fig. 3A), whereas the largest cluster identified for proteins with reduced abundance contained only 6 proteins (Fig. 4A). Network visualization and analysis in STRING was paired with statistical analysis by PANTHER, which identified and hierarchically organized biological process gene ontology (GO) terms that were significantly (FDR < 0.05) enriched among the proteins with increased and reduced abundance (Fig. 3B and 4B, respectively).
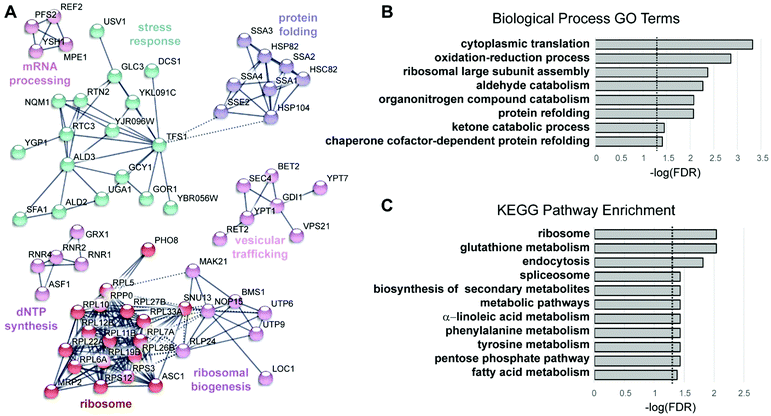 |
| Fig. 3 Functional analysis of proteins with elevated levels following KP1019 treatment. (A) STRING was used to map proteins of increased abundance onto the yeast interactome, using only known and predicted interactions with the highest confidence score (0.9). Nodes represent proteins of increased abundance only, and edges represent confidence. Associations between clusters are indicated with dashed edges. Clusters were established via the MCL algorithm with an inflation parameter of 2.5 to allow identification of a moderate number of clusters. Weak links and disconnected nodes are hidden for clarity, as are clusters with fewer than four nodes. Graphs represent the −log of the false discovery rate (FDR) for (B) the Biological Process GO terms at the highest hierarchical level identified by PANTHER and (C) the KEGG Pathway Enrichment as determined by STRING. Dashed lines indicate the cut-off for statistical significance (FDR = 0.05; −log(FDR) = 1.3). | |
 |
| Fig. 4 Functional analysis of proteins with reduced levels following KP1019 treatment. (A) STRING was used to map proteins of decreased abundance onto the yeast interactome as described for Fig. 3, except all clusters with three or more nodes are shown. (B) The Biological Process GO terms identified by PANTHER. Dashed line indicates the cut-off for statistical significance (FDR = 0.05; −log(FDR) = 1.3). | |
To identify transcription factors that may contribute to KP1019-dependent changes in protein levels, the pool of induced and repressed proteins were analysed in Yeastract (http://www.yeastract.com).63 Specifically, we applied Yeastract's TFRank functionality with a heat diffusion coefficient of 0.25 to avoid focusing on overly proximal or distal layers of regulation.64 Implicated transcription factors are presented in Fig. 5A. Since four of the top ten weighted transcription factors are known for their roles in filamentous growth and/or morphogenesis, we conducted a preliminary validation of the TFRank analysis by plating the filamentation competent strain ∑1278b52,53 on media containing varying concentrations of KP1019. As seen in Fig. 5B, the drug induced a dose-dependent increase in morphogenesis.
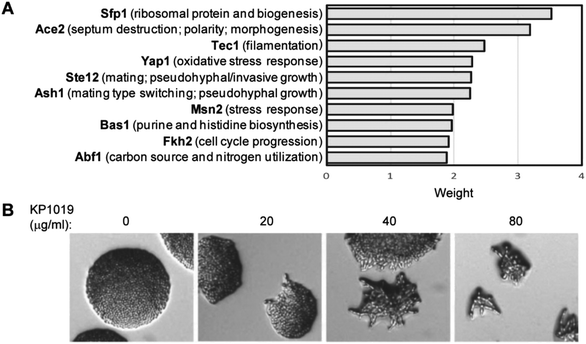 |
| Fig. 5 KP1019 induces morphogenesis in yeast. (A) All proteins with significant (p < 0.05) changes in abundance were analysed by the TFRank algorithm in Yeastract. Graph depicts scores for the ten most strongly implicated transcription factors. (B) Colony morphology of filamentation competent Σ1278b yeast grown overnight on the indicated concentrations of KP1019. | |
As will be discussed in more detail below, many of the findings generated through bioinformatics analyses revealed consistent themes, including activation of multiple stress response pathways. In fact, one of the largest clusters identified by STRING contains proteins involved in diverse biological processes including cellular detoxification (e.g. – Sfa165), RNA metabolism (e.g. – Rtc366), and signal transduction (e.g. – Tfs167) (Fig. 3A). Though seemingly dissimilar, most have been shown to be stress-responsive, increasing in abundance and/or changing localization following application of environmental stressors. For example, the glycogen branching enzyme Glc3, was previously shown to increase in abundance and form cytoplasmic puncta upon induction of DNA replication stress.68
KP1019 activates oxidative stress responses
Given that KP1019 has previously been shown to induce oxidative stress in colorectal carcinoma cells,24 it was not surprising to find “oxidation–reduction process” and “glutathione metabolism” amongst the enriched GO terms and Kyoto Encyclopedia of Genes and Genomes (KEGG) pathways, respectively (Fig. 3). Consistent with these findings, bioinformatics analyses uncovered additional evidence of metabolic retooling. For example, when proteins displaying drug-dependent reductions in abundance were analysed by STRING, “metabolic pathways” was identified as a significantly enriched KEGG pathway (FDR = 0.0001). Likewise, PANTHER revealed enrichment of proteins involved in purine nucleotide and alpha amino acid metabolism (Fig. 4B).
To better integrate these bioinformatics findings, metabolic enzymes displaying drug-dependent changes in abundance were mapped onto several intertwined metabolic pathways (Fig. 6A). This visualization reveals how these metabolic changes may coordinately regulate increased synthesis and/or cycling of the cellular antioxidant glutathione. In addition to increasing abundance of enzymes (Gsh2, Gtt1, and Glr1) directly involved in glutathione synthesis/cycling,69–71 KP1019 treatment increased levels of several enzymes in the pentose phosphate pathway (PPP), including the glucose-6-phosphate dehydrogenase Zwf1, which catalyses the pathway's rate-limiting step.72 Moreover, drug exposure lowered levels of proteins involved in inositol synthesis, potentially reducing the shunting of glucose-6-phosphate out of the PPP. Increased flux through the PPP could increase levels of NADPH,73 which is critical for glutathione regeneration.74,75 Furthermore, YeastMine uncovered significant changes to the S-adenosylmethionine (SAM) cycle (Benjamini–Hochberg corrected p-value = 0.002), which can be visualized as a cluster in Fig. 4A. This drug-dependent down-regulation might also contribute to antioxidant synthesis by increasing the proportion of de novo homocysteine available for conversion to cysteine and incorporation into GSH.
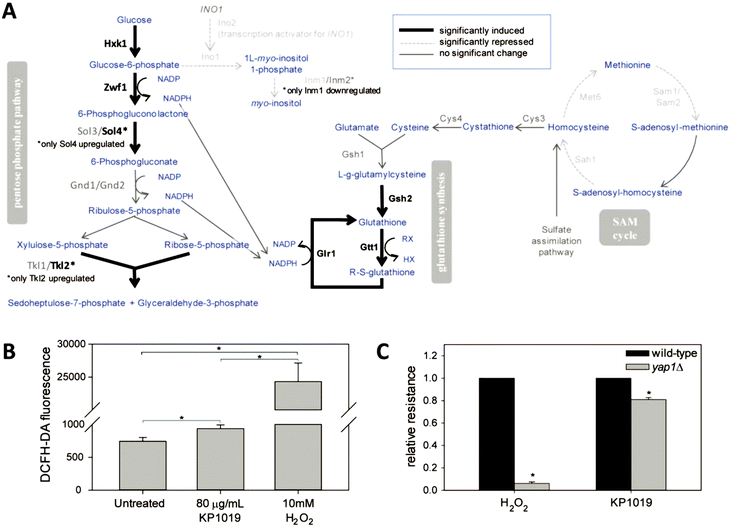 |
| Fig. 6 KP1019 induces oxidative stress resulting in metabolic retooling. (A) Changes in protein abundance are represented in the context of interacting metabolic pathways. Blue text corresponds to metabolic intermediates. (B) Yeast were pre-loaded with the redox sensitive dye DCFH-DA prior to treatment with KP1019 or hydrogen peroxide for 2.5 hours. Flow cytometry was used to measure the average fluorescence of 10 000 cells per condition per trial. (C) Drug sensitivity assays were conducted as described in Materials and Methods. The resulting IC50 values were used to calculate relative resistance by dividing each strain's IC50 by the IC50 of the corresponding wild-type control. Data presented in both graphs are the mean and standard deviation of three trials; asterisks indicate statistically significant differences (p < 0.01). | |
To more directly measure KP1019's ability to induce oxidative stress, we used the redox sensitive fluorophore DCFH-DA.76 As seen in Fig. 6B, KP1019 treated cells displayed elevated levels of fluorescence, consistent with drug-induced oxidative stress, albeit significantly weaker than the hydrogen peroxide positive control.
To gather independent confirmation of oxidative stress, we leveraged the results of the TFRank analysis, which implicated Yap1 (Fig. 5A), a major regulator of the yeast oxidative stress response and the yeast orthologue of the human transcription factor AP-1.75,77 Specifically, we examined the KP1019 sensitivity of yeast lacking YAP1. As seen in Fig. 6C, we confirmed that yap1Δ yeast are extremely sensitive to H2O2. Loss of YAP1 also caused a modest but statistically significant increase in sensitivity to KP1019, a result consistent with the modest induction of DCFH-DA fluorescence noted previously.
KP1019 induces the DNA damage response
Since several studies have shown that KP1019 damages DNA, it was surprising that the DNA damage response (DDR) was not among the enriched biological process GO terms. However, the ribonucleoside-diphosphate reductase (RNR) complex is visible in the STRING clustering (Fig. 3A), and it was one of the few specific cellular component GO terms that was significantly enriched (FDR = 0.01) for the list of proteins with increased abundance following KP1019 treatment. The RNR genes are well established as downstream effectors in the yeast DDR.78,79 To verify that elevated RNR levels in KP1019 treated cells was due to activation of the DDR, we examined expression of an RNR3-lacZ reporter construct in yeast lacking DDC1 and DUN1, which encode key mediators of the DDR.80–82 As can be seen in Fig. 7A, KP1019-dependent induction of this reporter for genotoxicity83 was blocked by disruption of the DDR. Consistent with previous studies,28,29 activation of the DDR appears to be physiologically significant, as deletion of DDC1 and DUN1 increases sensitivity to KP1019, decreasing the concentration of drug that inhibits growth by 50% (IC50) from 6.8 (wild-type) to 3.6 and 3.0 μg ml−1 for dun1Δ and ddc1Δ, respectively (Fig. 7B).
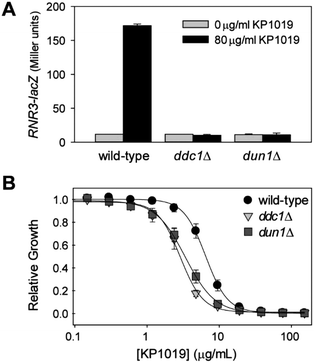 |
| Fig. 7 KP1019 activates the DNA damage response. (A) Yeast carrying an RNR3-lacZ reporter construct were treated with KP1019 for 3 hours prior to measuring β-galactosidase activity. (B) Yeast were grown overnight in the indicated concentrations of KP1019 then absorbance at 630 nm was measured to quantify culture density. Data presented in both panels are the mean and standard deviation of three trials. | |
KP1019 affects protein synthesis and folding
Among the most consistent themes that emerged from analysis of the proteomic data were protein (re)folding and protein synthesis. As can be seen in Fig. 3, KP1019 elevated the levels of chaperones that assist with protein (re)folding. Interestingly, subjecting the induced protein dataset to publication enrichment analysis in YeastMine revealed a potential connection to the heat shock response. Specifically, three of the top 5 hits (Benjamini Hochberg corrected p-values <0.0001) were published articles focused on the heat shock response.
In yeast, the heat shock response (HSR) involves elevated expression of chaperones. Consistent with activation of the HSR, we observed KP1019-dependent increases in the abundance of several heat shock proteins, including the HSP70 family members Ssa1-4 and the yeast HSP90 chaperones Hsp82 and Hsc82 (Fig. 3A). To determine whether the HSR transcriptional response might account for the changes in chaperone levels observed in our data, we monitored expression of a lacZ reporter under control of a heat shock element (HSE).84 Consistent with activation of the HSR, we observed a small but statistically significant drug-dependent increase in HSE-lacZ expression (Fig. 8A). Although a dose–response curve indicated that 80 μg ml−1 KP1019 (134 μM) results in maximal activation of this reporter construct (data not shown), the ability of 80 μg ml−1 KP1019 to induce expression was significantly lower than that of 100 μM cadmium (Fig. 8A). This concentration of cadmium was previously shown to derepress Hsf1,57 the evolutionarily conserved transcription factor and master regulator of S. cerevisiae HSR.75 Consistent with the modest induction of HSE-lacZ by KP1019, Hsf1 was only weakly implicated in the TFRank analysis of KP1019 response. Specifically, this transcription factor ranked 30th amongst the 100+ potential regulators reported by Yeastract. To determine whether the KP1019-dependent induction of chaperones was likely to be physiologically significant, we pre-treated yeast with KP1019 and then tested the yeast for heat tolerance. As can be seen in Fig. 8B, pre-treatment with KP1019 increased yeast tolerance to heat shock.
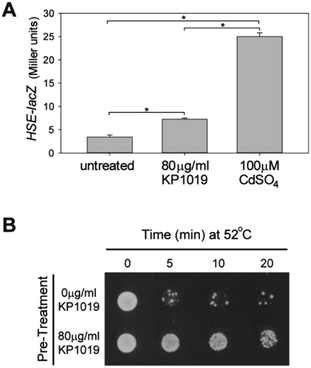 |
| Fig. 8 KP1019 activates the heat shock response. (A) Wild-type yeast carrying an HSE-lacZ reporter construct were treated with KP1019 or CdSO4 for 3 hours prior to measuring β-galactosidase activity. Bars and error bars represent the mean and standard deviation of 3 independent trials. Brackets and asterisks indicate statistically significant differences (p < 0.01). (B) Wild-type yeast were pre-treated with the indicated concentration of KP1019 prior to heat shock and plating on rich media to assess viability. | |
In addition to increasing abundance of proteins involved in protein (re)folding, clusters of ribosomal and ribosomal biogenesis proteins exhibited elevated levels (Fig. 3A). Specifically, the abundance of ribosomal and ribosomal biogenesis proteins in these intertwined clusters were increased an average of four-fold (range = 1.6 to 13-fold, not including Utp9, which was not detected in the absence of KP1019) Consistent with the visualization depicted in Fig. 3A, STRING's analytics revealed statistically significant (FDR = 0.002) enrichment of proteins associated with the cellular component GO term “cytosolic ribosome”. Likewise Yeastract identified the transcription factor Sfp1 – a major regulator of ribosomal gene expression85 – as a potential contributor to KP1019-dependent changes in protein abundance (Fig. 5A). Notably, the levels of Sfp1 itself increased four-fold in the drug treated samples.
Since elevated levels of translation machinery correlate with increased oxaliplatin sensitivity,86 and since KP1019 increases abundance of several ribosomal proteins (Fig. 3), we hypothesized that KP1019 might increase oxaliplatin sensitivity. As seen in Fig. 9A, treating yeast with 80 μg ml−1 KP1019 for three hours resulted in a roughly 60% decrease in the IC50 for oxaliplatin. To determine whether this effect was synergistic, we examined a wider range of KP1019 concentrations (Fig. 9B), analysing the resulting growth inhibition with the zero interaction potency (ZIP) model, which has a relatively low false positive rate and avoids the assumptions associated with more traditional additivity and independence models.87 According to this model a delta (δ) score of zero suggests no interaction between two drugs, whereas scores greater than zero indicate synergy and scores below zero correspond to antagonism. Although the average δ of 5.18 suggests only modest synergy between KP1019 and oxaliplatin, within the larger interaction landscape there is a region of synergy at moderately high doses of both drugs, peaking at δ = 25 (Fig. 9C).
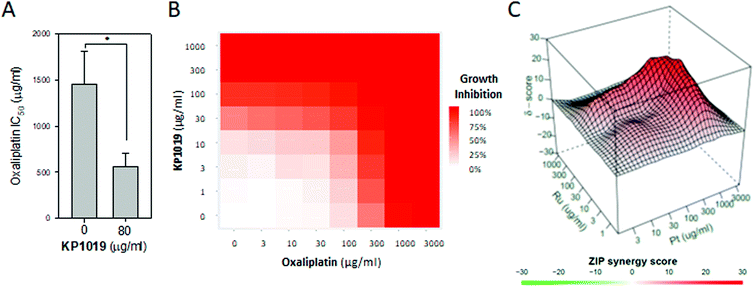 |
| Fig. 9 KP1019 sensitizes yeast to oxaliplatin. (A) Yeast were pre-treated with or without 80 μg ml−1 KP1019 for 3 hours. Then the oxaliplatin IC50 was determined as described in Fig. 7B. Data presented are the mean and standard deviation of three trials. (B and C) Yeast were pre-treated with the indicated concentrations of KP1019 for three hours prior to 18–24 hours of growth in the presence of oxaliplatin. Data shown are the dose response matrix (B) and interaction landscape (C) for one representative trial. | |
Discussion
In this study, we used the budding yeast S. cerevisiae as a model organism to examine the global effects of the promising anticancer ruthenium complex KP1019 at 80 μg ml−1, a concentration used in a prior transcriptomic study in yeast.29 Notably, this concentration is less than two-fold higher than the maximum plasma concentration (51.5 μg ml−1) observed in a Phase I clinical trial.20 Using a slightly higher concentration in S. cerevisiae is appropriate, as yeast often have elevated levels of resistance to antineoplastic agents.88
Consistent with previous findings, our results demonstrated that the drug significantly increased levels of proteins involved in genotoxic, proteotoxic, and oxidative stress responses.
With respect to the drug's impact on DNA, KP1019 binds model nucleotides in vitro89 and damages DNA in cancer24 and yeast27,28 cells; in S. cerevisiae, the resulting activation of the DNA damage response (DDR) leads to cell cycle arrest.29 Here, we observed induction of the ribonucleotide reductase complex (Fig. 3A) in a DDR-dependent manner (Fig. 7), and there was no evidence to suggest that these or other differences in protein levels were due solely to the drug's impact on cell cycle progression. These new data supporting KP1019-dependent activation of the DDR confirm prior studies, while validating our experimental design. We also noted that the drug induced filamentous growth in yeast (Fig. 5B), which may be a sign of replication stress.90
Interestingly, DNA damage and repair were not among the enriched GO terms identified in our bioinformatics analyses. Some lack of congruence with recent transcriptomic studies29,32 would be expected due to differences that arise when characterizing proteomes as opposed to transcriptomes. For example, osmotic or oxidative insults have been shown to impact translation, at times resulting in changes to the abundance of specific proteins.91–94 Divergence from transcriptomic studies may also stem from technical reasons. Although all three studies treated yeast with KP1019 for three hours prior to analysis, discrepancies included use of different wild-type yeast strains and varying concentrations of KP1019 (80 μg ml−1 (here and Bierle et al.29) vs.50 μg ml−1
32), and different cut-offs for bioinformatic analyses (e.g. – 1.5 (here and Golla et al.32) vs. 3.529 fold induction). Solubilizing the drug in DMSO, as in Golla et al.32 but not here, may also be a confounding variable, as DMSO impacts KP1019's stability (Cetto A and Stultz LK, personal communication). Regardless of the etiology of the variation between studies, our results support the existence of drug targets besides DNA. In particular, network and GO term analyses are consistent with the drug impacting proteins and redox processes.
In support of drug-dependent oxidative stress, we observed increased abundance of proteins involved in the pentose phosphate pathway and GSH synthesis (Fig. 3 and 6). Though these results are aligned with the oxidative stress observed in mammalian studies,24 increased expression of oxidative stress genes was not reported in the previous transcriptomic analyses in yeast.29,32 Regardless, we were able to verify induction of oxidative stress by measuring drug-dependent increases in fluorescence of the redox-sensitive dye DCFH-DA (Fig. 6B) and modest increases in sensitivity of yeast lacking Yap1 (Fig. 6C), the master regulator of the S. cerevisiae oxidative stress response.75,77 These findings are consistent with the “activation by reduction” hypothesis,95 according to which, KP1019 is a pro-drug that becomes bioactive after its central ruthenium atom is reduced from Ru3+ to Ru2+. Unlike most platinum-based drugs, which do not change oxidation states, KP1019 is more likely to be reduced in the low oxygen microenvironment of a poorly vascularized solid tumour. This localized activation may contribute to this ruthenium complex's selectivity for malignant tissues.95 An anticipated byproduct of KP1019 reduction would be reactive oxygen species (ROS), which could help mediate the drug's toxic effects. Although the more dramatic sensitivity of DDR mutants (Fig. 7B) relative to the Yap1 deletion strain (Fig. 6C) suggests DNA damage plays a greater role in the drug's toxicity, the physiological significance and origins of drug-induced ROS require further investigation, as the oxidative stress and DNA damage responses are highly intertwined. For example, oxidative stress is a well-known cause of DNA damage,96 and DNA damage leads to signalling-related increases in ROS.97,98
In addition to verifying KP1019's ability to induce DNA damage and oxidative stress, here we showed significant increases in levels of proteins involved in protein folding (Fig. 3). In fact, the chaperone Hsp104 showed the greatest fold increase in abundance following drug treatment (Fig. 2). Though analogous findings were not reported in previous transcriptomic studies,29,32 these observations are consistent with KP1019's reported binding to proteins in the cytosolic fraction of cancer cells.25 The drug's effects on proteins may be similar to those of other metals which perturb protein function by binding to thiols,5,6 displacing native metal cofactors,99 or causing aggregation.7,100 Additional impacts on proteins may be related to KP1019's effect on protein turnover. For example, our visualization of interactions between proteins with lowered abundance revealed a small cluster of proteins involved in the ubiquitin–proteasome system (Fig. 4A). Notably, deletion of RAD4 and RPT6 have previously been shown to increase accumulation of ubiquitinated proteins.101,102 Thus KP1019-dependent reductions in the levels of these proteins may contribute to the previously observed ability of the drug to result in global increases in ubiquitination.31
Regardless of the mechanism(s) by which KP1019 impacts protein folding and turnover, bioinformatics (YeastMine) analysis implicated the evolutionarily conserved transcription factor Hsf1 as a potential contributor to KP1019-dependent changes in protein levels. A reporter assay supported this conclusion (Fig. 8). In yeast and mammalian cells, Hsf1 is repressed by its interactions with Hsp70 chaperones.73,103 In S. cerevisiae, derepression can occur by misfolded proteins titrating Ssa1 (Hsp70) away from Hsf175 or by chemicals binding to or oxidizing Ssa1 thiols, thereby removing/displacing chaperones from Hsf1.57 Additional research will be required to determine which of these mechanisms, if any, applies to KP1019. The importance of drug-dependent perturbation of protein homeostasis relative to the drug's genotoxicity and ROS-inducing capabilities also remains an open question, as HSF1 is an essential gene in yeast,104 which precludes analysis of deletion strains.
Beyond alterations to protein (re)folding and turnover, we observed significant increases in levels of proteins involved in translation (Fig. 3). Although many stresses have previously been shown to repress expression of genes involved in ribosomal structure and biogenesis,105 recent transcriptomic analyses of KP1019,32 other ruthenium complexes,106,107 and the metal salts AgNO3, CdCl2, HgCl2, and ZnSO42 showed induction of ribosomal structural and biogenesis genes. Moreover, a re-analysis of published transcriptomic data,29 using a 1.5-fold (vs. 3.5-fold) cut-off for gene induction also revealed an enrichment of ribosomal genes. Specifically, PANTHER identified “cytosolic translation” as the biological process GO term with the lowest FDR (0.0002). Notably, low levels (0.02%) of the DNA damaging agent methyl methane sulfonate (MMS) have also been shown to induce expression of ribosomal proteins in yeast.60 Although MMS is capable of damaging proteins,108–110 a major mode of its action is DNA damage, raising the possibility that KP1019's impacts on ribosomal proteins/genes may be related to its genotoxicity. The induction of ribosomal proteins following KP101932 and MMS60 treatment has been linked to the transcription factor Sfp1, which was implicated in our bioinformatics analysis (Fig. 5A). As a “generalist,” Sfp1 responds to diverse stresses, including oxidative and osmotic stress,111 both of which are caused by KP1019 (Fig. 6 and Singh et al.31). However, precisely how KP1019 impacts translation remains unclear. For example, it is not yet known whether KP1019 affects expression of ribosomal RNAs and/or whether the drug impacts the pool of mRNAs being translated (i.e. – the translatome).
Regardless, since elevated levels of translation machinery correlate with oxaliplatin sensitivity,86 we hypothesized that KP1019-dependent increases in abundance of ribosomal and ribosomal biogenesis proteins might sensitize yeast to oxaliplatin. In fact, that was the case (Fig. 9), which is consistent with models for synergy which predict that agents acting on similar processes may be more likely to have synergistic (as opposed to additive or antagonistic) effects.112 Given that both oxaliplatin and KP1019 impact translation (Fig. 3, ref. 32 and 86) and that “translation addicted” cancer cell lines are hypersensitive to oxaliplatin,86 future studies might explore whether these same cell lines are hypersensitive to KP1019. If so, KP1019's effects on protein synthesis may explain its specificity for cancer cells, as many hematological and solid tumours have elevated levels of translation machinery.113–115 This specificity for translation-addicted cancer cells may, in turn, contribute to KP1019's lack of dose-limiting toxicity.
Conclusions
Here we report the first global proteomic analysis of the effects of KP1019 on eukaryotic cells. Our findings reveal a multi-faceted response that could be explained by the presence of both nuclear and non-nuclear targets. Although KP1019's ability to damage DNA is well-established in both cancer and yeast cells.24,27,28 here we provide the first evidence supporting KP1019-dependent induction of oxidative stress in yeast. This finding further supports the utility of yeast as a model for studying KP1019, as this drug has been shown to cause oxidative stress in colorectal cancer cells.24 The observed increases in chaperones and translation machinery suggest that proteins serve as a significant cytosolic target of the drug. These findings may have long-term clinical implications, as translation has emerged as a promising target for chemotherapeutics.115,116 Moreover, the ability of KP1019 to sensitize yeast to oxaliplatin suggests that KP1019 may be a robust component of combination therapy regimens.
Conflicts of interest
There are no conflicts to declare.
Acknowledgements
This work was supported by a National Institutes of Health award to the UAB Comprehensive Cancer Center [grant number P30CA013148], by the UAB Institutional Core Funding Mechanism, by a National Science Foundation award to Birmingham-Southern College (1229016), and by the BSC rise3 stipend program. We would also like thank Stephen Elledge (Harvard University), Kevin Morano (University of Texas Health Sciences Center), and Gerald Fink (MIT) for the generous gifts of reporter constructs and yeast strains.
References
- D. Hosiner, S. Gerber, H. Lichtenberg-Fraté, W. Glaser, C. Schüller and E. Klipp, Impact of acute metal stress in Saccharomyces cerevisiae, PLoS One, 2014, 9, e83330 CrossRef PubMed.
- Y. H. Jin, P. E. Dunlap, S. J. McBride, H. Al-Refai, P. R. Bushel and J. H. Freedman, Global transcriptome and deletome profiles of yeast exposed to transition metals, PLoS Genet., 2008, 4, e1000053 CrossRef PubMed.
- H. S. Kim, Y. J. Kim and Y. R. Seo, An overview of carcinogenic heavy metal: Molecular toxicity mechanism and prevention, J. Cancer Prev., 2015, 20, 232–240 CrossRef PubMed.
- A. Hartwig, Metal interaction with redox regulation: an integrating concept in metal carcinogenesis?, Free Radical Biol. Med., 2013, 55, 63–72 CrossRef CAS PubMed.
- M. Valko, M. Valko, H. Morris and M. T. D. Cronin, Metals, toxicity and oxidative stress, Curr. Med. Chem., 2005, 12, 1161–1208 CrossRef CAS PubMed.
-
P. B. Tchounwou, C. G. Yedjou, A. K. Patlolla and D. J. Sutton, Heavy metal toxicity and the environment, in Molecular, Clinical and Environmental Toxicology, ed. A. Luch, Springer, Basel, 2012, pp. 133–164 Search PubMed.
- M. Tamás, S. Sharma, S. Ibstedt, T. Jacobson and P. Christen, Heavy metals and metalloids as a cause for protein misfolding and aggregation, Biomolecules, 2014, 4, 252–267 CrossRef PubMed.
- U. Ndagi, N. Mhlongo and M. Soliman, Metal complexes in cancer therapy – an update from drug design perspective, Drug Des., Dev. Ther., 2017, 11, 599–616 CrossRef CAS PubMed.
- U. Jungwirth, C. R. Kowol, B. K. Keppler, C. G. Hartinger, W. Berger and P. Heffeter, Anticancer activity of metal complexes: involvement of redox processes, Antioxid. Redox Signaling, 2011, 15, 1085–1127 CrossRef CAS PubMed.
- P. C. A. Bruijnincx and P. J. Sadler, New trends for metal complexes with anticancer activity, Curr. Opin. Chem. Biol., 2008, 12, 197–206 CrossRef CAS PubMed.
- C. X. Zhang and S. J. Lippard, New metal complexes as potential therapeutics, Curr. Opin. Chem. Biol., 2003, 7, 481–489 CrossRef CAS PubMed.
- M. E. Royce and R. Pazdur, Novel chemotherapeutic agents for gastrointestinal cancers, Curr. Opin. Oncol., 1999, 11, 299–304 CrossRef CAS PubMed.
- A. Zaniboni and F. Meriggi, The emerging role of oxaliplatin in the treatment of gastric cancer, J. Chemother., 2005, 17, 656–662 CrossRef CAS PubMed.
- B. Gustavsson, G. Carlsson, D. Machover, N. Petrelli, A. Roth, H.-J. Schmoll, K.-M. Tveit and F. Gibson, A review of the evolution of systemic chemotherapy in the management of colorectal cancer, Clin. Colorectal Cancer, 2015, 14, 1–10 CrossRef PubMed.
- L. Kelland, The resurgence of platinum-based cancer chemotherapy, Nat. Rev. Cancer, 2007, 7, 573–584 CrossRef CAS PubMed.
- S. Dasari and P. B. Tchounwou, Cisplatin in cancer therapy: molecular mechanisms of action, Eur. J. Pharmacol., 2014, 740, 364–378 CrossRef CAS PubMed.
- M. G. Apps, E. H. Y. Choi and N. J. Wheate, The state-of-play and future of platinum drugs, Endocr.-Relat. Cancer, 2015, 22, R219–R233 CAS.
- R. Trondl, P. Heffeter, C. R. Kowol, M. A. Jakupec, W. Berger and B. K. Keppler, NKP-1339, the first ruthenium-based anticancer drug on the edge to clinical application, Chem. Sci., 2014, 5, 2925–2932 RSC.
- A. Bergamo, C. Gaiddon, J. H. M. Schellens, J. H. Beijnen and G. Sava, Approaching tumour therapy beyond platinum drugs: Status of the art and perspectives of ruthenium drug candidates, J. Inorg. Biochem., 2012, 106, 90–99 CrossRef CAS PubMed.
- F. Lentz, A. Drescher, A. Lindauer, M. Henke, R. A. Hilger, C. G. Hartinger, M. E. Scheulen, C. Dittrich, B. K. Keppler, U. Jaehde and Central European Society for Anticancer Drug Research-EWIV, Pharmacokinetics of a novel anticancer ruthenium complex (KP1019, FFC14A) in a phase I dose-escalation study, Anticancer Drugs, 2009, 20, 97–103 CrossRef CAS PubMed.
- C. G. Hartinger, M. A. Jakupec, S. Zorbas-Seifried, M. Groessl, A. Egger, W. Berger, H. Zorbas, P. J. Dyson and B. K. Keppler, KP1019, A new redox-active anticancer agent - Preclinical development and results of a clinical phase I study in tumor patients, Chem. Biodiversity, 2008, 5, 2140–2155 CrossRef CAS PubMed.
- P. Heffeter, M. Pongratz, E. Steiner, P. Chiba, M. A. Jakupec, L. Elbling, B. Marian, W. Körner, F. Sevelda, M. Micksche, B. K. Keppler and W. Berger, Intrinsic and acquired forms of resistance against the anticancer ruthenium compound KP1019 [Indazolium trans-[tetrachlorobis(1H-indazole) ruthenate(III)]] (FFC14A), J. Pharmacol. Exp. Ther., 2005, 312, 281–289 CrossRef CAS PubMed.
- M. R. Berger, F. T. Garzon, B. K. Keppler and D. Schmähl, Efficacy of new ruthenium complexes against chemically induced autochthonous colorectal carcinoma in rats, Anticancer Res., 1989, 9, 761–765 CAS.
- S. Kapitza, M. A. Jakupec, M. Uhl and B. K. Keppler, and B. Marian, The heterocyclic ruthenium(III) complex KP1019 (FFC14A) causes DNA damage and oxidative stress in colorectal tumor cells, Cancer Lett., 2005, 226, 115–121 CrossRef CAS PubMed.
- P. Heffeter, K. Böck, B. Atil, M. A. Reza Hoda, W. Körner, C. Bartel, U. Jungwirth, B. K. Keppler, M. Micksche, W. Berger and G. Koellensperger, Intracellular protein binding patterns of the anticancer ruthenium drugs KP1019 and KP1339, J. Biol. Inorg. Chem., 2010, 15, 737–748 CrossRef CAS PubMed.
- M. Groessl, O. Zava and P. J. Dyson, Cellular uptake and subcellular distribution of ruthenium-based metallodrugs under clinical investigation versus cisplatin, Metallomics, 2011, 3, 591 RSC.
- S. K. Stevens, A. P. Strehle, R. L. Miller, S. H. Gammons, K. J. Hoffman, J. T. McCarty, M. E. Miller, L. K. Stultz and P. K. Hanson, The anticancer ruthenium complex KP1019 induces DNA damage, leading to cell cycle delay and cell death in Saccharomyces cerevisiae, Mol. Pharmacol., 2013, 83, 225–234 CrossRef CAS PubMed.
- V. Singh, G. Kumar Azad, P. Mandal, M. A. Reddy and R. S. Tomar, Anti-cancer drug KP1019 modulates epigenetics and induces DNA damage response in Saccharomyces cerevisiae, FEBS Lett., 2014, 588, 1044–1052 CrossRef CAS PubMed.
- L. A. Bierle, K. L. Reich, B. E. Taylor, E. B. Blatt, S. M. Middleton, S. D. Burke, L. K. Stultz, P. K. Hanson, J. F. Partridge and M. E. Miller, DNA damage response checkpoint activation drives KP1019 dependent pre-anaphase cell cycle delay in S. cerevisiae, PLoS One, 2015, 10, e0138085 CrossRef PubMed.
- M. I. Webb and C. J. Walsby, EPR as a probe of the intracellular speciation of ruthenium(III) anticancer compounds, Metallomics, 2013, 5, 1624 RSC.
- V. Singh, G. K. Azad, A. Reddy M., S. Baranwal and R. S. Tomar, Anti-cancer drug KP1019 induces Hog1 phosphorylation and protein ubiquitylation in Saccharomyces cerevisiae, Eur. J. Pharmacol., 2014, 736, 77–85 CrossRef CAS PubMed.
- U. Golla, S. Swagatika, S. Chauhan, R. S. Tomar, U. Golla, S. Swagatika, S. Chauhan and R. Singh Tomar, A systematic assessment of chemical, genetic, and epigenetic factors influencing the activity of anticancer drug KP1019 (FFC14A), Oncotarget, 2017, 8, 98426–98454 CrossRef PubMed.
- T. R. Hughes, Yeast and drug discovery, Funct. Integr. Genomics, 2002, 2, 199–211 CrossRef CAS PubMed.
- S. C. dos Santos, M. C. Teixeira, T. R. Cabrito and I. Sá-Correia, Yeast toxicogenomics: genome-wide responses to chemical stresses with impact in environmental health, pharmacology, and biotechnology, Front. Genet., 2012, 3, 63 Search PubMed.
- S. C. dos Santos, S. Tenreiro, M. Palma, J. Becker and I. Sá-Correia, Transcriptomic profiling of the Saccharomyces cerevisiae response to quinine reveals a glucose limitation response attributable to drug-induced inhibition of glucose uptake, Antimicrob. Agents Chemother., 2009, 53, 5213–5223 CrossRef CAS PubMed.
- A. Rao, Y. Zhang, S. Muend and R. Rao, Mechanism of antifungal activity of terpenoid phenols resembles calcium stress and inhibition of the TOR pathway, Antimicrob. Agents Chemother., 2010, 54, 5062–5069 CrossRef CAS PubMed.
- E. A. Winzeler, D. D. Shoemaker, A. Astromoff, H. Liang, K. Anderson, B. Andre, R. Bangham, R. Benito, J. D. Boeke, H. Bussey, A. M. Chu, C. Connelly, K. Davis, F. Dietrich, S. W. Dow, M. El Bakkoury, F. Foury, S. H. Friend, E. Gentalen, G. Giaever, J. H. Hegemann, T. Jones, M. Laub, H. Liao, N. Liebundguth, D. J. Lockhart, A. Lucau-Danila, M. Lussier, N. M’Rabet, P. Menard, M. Mittmann, C. Pai, C. Rebischung, J. L. Revuelta, L. Riles, C. J. Roberts, P. Ross-MacDonald, B. Scherens, M. Snyder, S. Sookhai-Mahadeo, R. K. Storms, S. Véronneau, M. Voet, G. Volckaert, T. R. Ward, R. Wysocki, G. S. Yen, K. Yu, K. Zimmermann, P. Philippsen, M. Johnston and R. W. Davis, Functional characterization of the S. cerevisiae genome by gene deletion and parallel analysis, Science, 1999, 285, 901–906 CrossRef CAS PubMed.
- C. J. Gimeno, P. O. Ljungdahl, C. A. Styles and G. R. Fink, Unipolar cell divisions in the yeast S. cerevisiae lead to filamentous growth: Regulation by starvation and RAS, Cell, 1992, 68, 1077–1090 CrossRef CAS PubMed.
-
C. Kaiser, S. Michaelis and A. Mitchell, Methods in Yeast Genetics, Cold Spring Harbor Laboratory Press, Cold Spring Harbor, NY, 1994 Search PubMed.
-
R. D. Gietz and R. A. Woods, Yeast Protocols, Humana Press, New Jersey, 2006, pp. 107–120 Search PubMed.
- K. G. Lipponer, E. Vogel and B. K. Keppler, Synthesis, Characterization and Solution Chemistry of trans-Indazoliumtetrachlorobis(Indazole)Ruthenate(III), a New anticancer ruthenium complex. IR, UV, NMR, HPLC investigations and antitumor activity. crystal structures of trans-1-methyl-indazoliumtetrachlorobis-(1-methylindazole)ruthenate(III) and its hydrolysis product trans-monoaquatrichlorobis-(1-methylindazole)-ruthenate(III), Met.-Based Drugs, 1996, 3, 243–260 CrossRef CAS PubMed.
- M. R. Ludwig, K. Kojima, G. J. Bowersock, D. Chen, N. C. Jhala, D. J. Buchsbaum, W. E. Grizzle, C. A. Klug and J. A. Mobley, Surveying the serologic proteome in a tissue-specific kras(G12D) knockin mouse model of pancreatic cancer, Proteomics, 2016, 16, 516–531 CrossRef CAS PubMed.
- A. Keller, A. I. Nesvizhskii, E. Kolker and R. Aebersold, Empirical statistical model to estimate the accuracy of peptide identifications made by MS/MS and database search, Anal. Chem., 2002, 74, 5383–5392 CrossRef CAS PubMed.
- A. I. Nesvizhskii, A. Keller, E. Kolker and R. Aebersold, A statistical model for identifying proteins by tandem mass spectrometry, Anal. Chem., 2003, 75, 4646–4658 CrossRef CAS PubMed.
- D. B. Weatherly, J. A. Atwood, T. A. Minning, C. Cavola, R. L. Tarleton and R. Orlando, A heuristic method for
assigning a false-discovery rate for protein identifications from Mascot database search results, Mol. Cell. Proteomics, 2005, 4, 762–772 CrossRef CAS PubMed.
- W. M. Old, K. Meyer-Arendt, L. Aveline-Wolf, K. G. Pierce, A. Mendoza, J. R. Sevinsky, K. A. Resing and N. G. Ahn, Comparison of label-free methods for quantifying human proteins by shotgun proteomics, Mol. Cell. Proteomics, 2005, 4, 1487–1502 CrossRef CAS PubMed.
- H. Liu, R. G. Sadygov and J. R. Yates, A model for random sampling and estimation of relative protein abundance in shotgun proteomics, Anal. Chem., 2004, 76, 4193–4201 CrossRef CAS PubMed.
- T. Beißbarth, L. Hyde, G. K. Smyth, C. Job, W.-M. Boon, S.-S. Tan, H. S. Scott and T. P. Speed, Statistical modeling of sequencing errors in SAGE libraries, Bioinformatics, 2004, 20, i31–i39 CrossRef PubMed.
- D. Szklarczyk, J. H. Morris, H. Cook, M. Kuhn, S. Wyder, M. Simonovic, A. Santos, N. T. Doncheva, A. Roth, P. Bork, L. J. Jensen and C. von Mering, The STRING database in 2017: quality-controlled protein–protein association networks, made broadly accessible, Nucleic Acids Res., 2017, 45, D362–D368 CrossRef CAS PubMed.
- H. Mi, X. Huang, A. Muruganujan, H. Tang, C. Mills, D. Kang and P. D. Thomas, PANTHER version 11: expanded annotation data from Gene Ontology and Reactome pathways, and data analysis tool enhancements, Nucleic Acids Res., 2017, 45, D183–D189 CrossRef CAS PubMed.
- R. Balakrishnan, J. Park, K. Karra, B. C. Hitz, G. Binkley, E. L. Hong, J. Sullivan, G. Micklem and J. M. Cherry, YeastMine--an integrated data warehouse for Saccharomyces cerevisiae data as a multipurpose tool-kit, Database, 2012, 2012, bar062 CrossRef PubMed.
- P. J. Cullen and G. F. Sprague, Glucose depletion causes haploid invasive growth in yeast, Proc. Natl. Acad. Sci. U. S. A., 2000, 97, 13619–13624 CrossRef CAS PubMed.
- C. J. Gimeno and G. R. Fink, The logic of cell division in the life cycle of yeast, Science, 1992, 257, 626 CrossRef CAS PubMed.
- Z. Zhou and S. J. Elledge, Isolation of crt mutants constitutive for transcription of the DNA damage inducible gene RNR3 in Saccharomyces cerevisiae, Genetics, 1992, 131, 851–866 CAS.
- N. Santoro, N. Johansson and D. J. Thiele, Heat shock element architecture is an important determinant in the temperature and transactivation domain requirements for heat shock transcription factor, Mol. Cell. Biol., 1998, 18, 6340–6352 CrossRef CAS PubMed.
- L. Guarente, Yeast promoters and lacZ fusions designed to study expression of cloned genes in yeast, Methods Enzymol., 1983, 101, 181–191 CAS.
- Y. Wang, P. A. Gibney, J. D. West and K. A. Morano, The yeast Hsp70 Ssa1 is a sensor for activation of the heat shock response by thiol-reactive compounds, Mol. Biol. Cell, 2012, 23, 3290–3298 CrossRef CAS PubMed.
- A. Ianevski, L. He, T. Aittokallio and J. Tang, SynergyFinder: a web application for analyzing drug combination dose-response matrix data, Bioinformatics, 2017, 33, 2413–2415 CrossRef PubMed.
- L. Stultz, J. Mobley and P. Hanson, Budding yeast proteomic response to the anticancer ruthenium complex KP1019, Mendeley Data, 2020 DOI:10.17632/vrswrvjcy2.2.
- A. Mazumder, L. Q. Pesudo, S. Mcree, M. Bathe and L. D. Samson, Genome-wide single-cell-level screen for protein abundance and localization changes in response to DNA damage in S. cerevisiae, Nucleic Acids Res., 2013, 41, 9310–9324 CrossRef CAS PubMed.
- J. R. Glover and S. Lindquist, Hsp104, Hsp70, and Hsp40: A novel chaperone system that rescues previously aggregated proteins, Cell, 1998, 94, 73–82 CrossRef CAS PubMed.
- B. Bösl, V. Grimminger and S. Walter, The molecular chaperone Hsp104 – a molecular machine for protein diaggregation, J.
Struct. Biol., 2006, 156, 139–148 CrossRef PubMed.
- M. C. Teixeira, P. T. Monteiro, J. F. Guerreiro, J. P. Gonçalves, N. P. Mira, S. C. dos Santos, T. R. Cabrito, M. Palma, C. Costa, A. P. Francisco, S. C. Madeira, A. L. Oliveira, A. T. Freitas and I. Sá-Correia, The YEASTRACT database: an upgraded information system for the analysis of gene and genomic transcription regulation in Saccharomyces cerevisiae, Nucleic Acids Res., 2014, 42, D161–D166 CrossRef CAS PubMed.
- J. P. Gonçalves, A. P. Francisco, N. P. Mira, M. C. Teixeira, I. Sá-Correia, A. L. Oliveira and S. C. Madeira, TFRank: network-based prioritization of regulatory associations underlying transcriptional responses, Bioinformatics, 2011, 27, 3149–3157 CrossRef PubMed.
- M. Rosario Fernández, J. A. Biosca, A. Norin, H. Jörnvall and X. Parés, Class III alcohol dehydrogenase from Saccharomyces cerevisiae: Structural and enzymatic features differ toward the human/mammalian forms in a manner consistent with functional needs in formaldehyde detoxication, FEBS Lett., 1995, 370, 23–26 CrossRef.
- A. Savchenko, N. Krogan, J. R. Cort, E. Evdokimova, J. M. Lew, A. A. Yee, L. Sánchez-Pulido, M. A. Andrade, A. Bochkarev, J. D. Watson, M. A. Kennedy, J. Greenblatt, T. Hughes, C. H. Arrowsmith, J. M. Rommens and A. M. Edwards, The Shwachman-Bodian-Diamond syndrome protein family is involved in RNA metabolism, J. Biol. Chem., 2005, 280, 19213–19220 CrossRef CAS PubMed.
- R. Caesar and A. Blomberg, The stress-induced Tfs1p requires NatB-mediated acetylation to inhibit carboxypeptidase Y and to regulate the protein kinase A pathway, J. Biol. Chem., 2004, 279, 38532–38543 CrossRef CAS PubMed.
- J. M. Tkach, A. Yimit, A. Y. Lee, M. Riffle, M. Costanzo, D. Jaschob, J. A. Hendry, J. Ou, J. Moffat, C. Boone, T. N. Davis, C. Nislow and G. W. Brown, Dissecting DNA damage response pathways by analysing protein localization and abundance changes during DNA replication stress, Nat. Cell Biol., 2012, 14, 966–976 CrossRef CAS PubMed.
- E. Herrero, J. Ros, J. Tamarit and G. Bellí, Glutaredoxins in fungi, Photosynth. Res., 2006, 89, 127–140 CrossRef CAS PubMed.
- C. M. Grant, Role of the glutathione/glutaredoxin and thioredoxin systems in yeast growth and response to stress conditions, Mol. Microbiol., 2001, 39, 533–541 CrossRef CAS PubMed.
- M. B. Toledano, A. Delaunay-Moisan, C. E. Outten and A. Igbaria, Functions and cellular compartmentation of the thioredoxin and glutathione pathways in yeast, Antioxid. Redox Signaling, 2013, 18, 1699–1711 CrossRef CAS PubMed.
- I. Nogae and M. Johnston, Isolation and characterization of the ZWF1 gene of Saccharomyces cerevisiae, encoding glucose-6-phosphate dehydrogenase, Gene, 1990, 96, 161–169 CrossRef CAS PubMed.
- M. Ralser, M. M. Wamelink, A. Kowald, B. Gerisch, G. Heeren, E. A. Struys, E. Klipp, C. Jakobs, M. Breitenbach, H. Lehrach and S. Krobitsch, Dynamic rerouting of the carbohydrate flux is key to counteracting oxidative stress, J. Biol., 2008, 6, 10 CrossRef PubMed.
- M. D. Temple, G. G. Perrone and I. W. Dawes, Complex cellular responses to reactive oxygen species, Trends Cell Biol., 2005, 15, 319–326 CrossRef CAS PubMed.
- K. A. Morano, C. M. Grant and W. S. Moye-Rowley, The response to heat shock and oxidative stress in Saccharomyces cerevisiae, Genetics, 2012, 190, 1157–1195 CrossRef CAS PubMed.
- E. J. Yurkow and M. A. Mckenzie, Characterization of hypoxia-dependent peroxide production in cultures of Saccharomyces cerevisiae using flow cytometry: A model for ischemic tissue destruction, Cytometry, 1993, 14, 287–293 CrossRef CAS PubMed.
- W. S. Moye-Rowley, K. D. Harshman and C. S. Parker, Yeast YAP1 encodes a novel form of the jun family of transcriptional activator proteins, Genes Dev., 1989, 3, 283–292 CrossRef CAS PubMed.
- S. J. Elledge and R. W. Davis, DNA damage induction of ribonucleotide reductase, Mol. Cell. Biol., 1989, 9, 4932–4940 CrossRef CAS PubMed.
- S. J. Elledge, Z. Zhou, J. B. Allen and T. A. Navas, DNA damage and cell cycle regulation of ribonucleotide reductase, BioEssays, 1993, 15, 333–339 CrossRef CAS PubMed.
- J. Majka and P. M. J. Burgers, Yeast Rad17Mec3Ddc1: A sliding clamp for the DNA damage checkpoint, Proc. Natl. Acad. Sci. U. S. A., 2003, 100, 2249–2254 CrossRef CAS PubMed.
- V. M. Navadgi-Patil and P. M. Burgers, A tale of two tails: activation of DNA damage checkpoint kinase Mec1/ATR by the 9-1-1 clamp and by Dpb11/TopBP1, DNA Repair, 2009, 8, 996–1003 CrossRef CAS PubMed.
- Z. Zhou and S. J. Elledge,
DUN1 encodes a protein kinase that controls the DNA damage response in yeast, Cell, 1993, 75, 1119–1127 CrossRef CAS PubMed.
- X. Jia, Y. Zhu and W. Xiao, A stable and sensitive genotoxic testing system based on DNA damage induced gene expression in Saccharomyces cerevisiae, Mutat. Res., 2002, 519, 83–92 CAS.
- X.-D. Liu, P. C. C. Liu, N. Santoro and D. J. Thiele, Conservation of a stress response: human heat shock transcription factors functionally substitute for yeast HSF, EMBO J., 1997, 16, 6466–6477 CrossRef CAS PubMed.
- R. M. Marion, A. Regev, E. Segal, Y. Barash, D. Koller, N. Friedman and E. K. O'shea, Sfp1 is a stress-and nutrient-sensitive regulator of ribosomal protein gene expression, Proc. Natl. Acad. Sci. U. S. A., 2004, 101, 14315–14322 CrossRef CAS PubMed.
- P. M. Bruno, Y. Liu, G. Y. Park, J. Murai, C. E. Koch, T. J. Eisen, J. R. Pritchard, Y. Pommier, S. J. Lippard and M. T. Hemann, A subset of platinum-containing chemotherapeutic agents kills cells by inducing ribosome biogenesis stress, Nat. Med., 2017, 23, 461–471 CrossRef CAS PubMed.
- B. Yadav, K. Wennerberg, T. Aittokallio and J. Tang, Searching for drug synergy in complex dose-response landscapes using an interaction potency model, Comput. Struct. Biotechnol. J., 2015, 13, 504–513 CrossRef CAS PubMed.
- A. Stepanov, K. C. Nitiss, G. Neale and J. L. Nitiss, Enhancing drug accumulation in Saccharomyces cerevisiae by repression of pleiotropic drug resistance genes with chimeric transcription repressors, Mol. Pharmacol., 2008, 74, 423–431 CrossRef CAS PubMed.
- P. Schluga, C. G. Hartinger, A. Egger, E. Reisner, M. Galanski, M. A. Jakupec and B. K. Keppler, Redox behavior of tumor-inhibiting ruthenium(III) complexes and effects of physiological reductants on their binding to GMP, Dalton Trans., 2006, 1796–1802 RSC.
- Y. W. Jiang and C. M. Kang, Induction of S. cerevisiae filamentous differentiation by slowed DNA synthesis involves Mec1, Rad53 and Swe1 checkpoint proteins, Mol. Biol. Cell, 2003, 14, 5116–5124 CrossRef CAS PubMed.
- J. Warringer, M. Hult, S. Regot, F. Posas and P. Sunnerhagen, The HOG pathway dictates the short-term translational response after hyperosmotic shock, Mol. Biol. Cell, 2010, 21, 3080–3092 CrossRef CAS PubMed.
- W. Rowe, C. J. Kershaw, L. M. Castelli, J. L. Costello, M. P. Ashe, C. M. Grant, P. F. G. Sims, G. D. Pavitt and S. J. Hubbard, Puf3p induces translational repression of genes linked to oxidative stress, Nucleic Acids Res., 2014, 42, 1026–1041 CrossRef CAS PubMed.
- C. Vogel, G. Monteiro Silva and E. M. Marcotte, Protein expression regulation under oxidative stress, Mol. Cell. Proteomics, 2011, 10, M111.009217 CrossRef PubMed.
- D. Shenton, J. B. Smirnova, J. N. Selley, K. Carroll, S. J. Hubbard, G. D. Pavitt, M. P. Ashe and C. M. Grant, Global translational responses to oxidative stress impact upon multiple levels of protein synthesis, J. Biol. Chem., 2006, 281, 29011–29021 CrossRef CAS PubMed.
- C. G. Hartinger, S. Zorbas-Seifried, M. A. Jakupec, B. Kynast, H. Zorbas and B. K. Keppler, From bench to bedside – preclinical and early clinical development of the anticancer agent indazolium trans-[tetrachlorobis(1H-indazole)ruthenate(III)] (KP1019 or FFC14A), J. Inorg. Biochem., 2006, 100, 891–904 CrossRef CAS PubMed.
- B. Halliwell and O. I. Aruoma, DNA damage by oxygen-derived species Its mechanism and measurement in mammalian systems, FEBS Lett., 1991, 281, 9–19 CrossRef CAS PubMed.
- L. A. Rowe, N. Degtyareva and P. W. Doetsch, DNA damage-induced reactive oxygen species (ROS) stress response in Saccharomyces cerevisiae, Free Radical Biol. Med., 2008, 45, 1167–1177 CrossRef CAS PubMed.
- M. A. Kang, E.-Y. So, A. L. Simons, D. R. Spitz and T. Ouchi, DNA damage induces reactive oxygen species generation through the H2AX-Nox1/Rac1 pathway, Cell Death Dis., 2012, 3, e249 CrossRef CAS PubMed.
- A. Witkiewicz-Kucharczyk and W. Bal, Damage of zinc fingers in DNA repair proteins, a novel molecular mechanism in carcinogenesis, Toxicol. Lett., 2006, 162, 29–42 CrossRef CAS PubMed.
- W. Qin, N. Bazeille, E. Henry, B. Zhang, E. Deprez and X.-G. Xi, Mechanistic insight into cadmium-induced inactivation of the Bloom protein, Sci. Rep., 2016, 6, 26225 CrossRef CAS PubMed.
- Y. Li, J. Yan, I. Kim, C. Liu, K. Huo and H. Rao, Rad4 regulates protein turnover at a postubiquitylation step, Mol. Biol. Cell, 2010, 21, 177–185 CrossRef CAS PubMed.
- V. Sokolova, F. Li, G. Polovin and S. Park, Proteasome activation is mediated via a functional switch of the Rpt6 C-terminal tail following chaperone-dependent assembly, Sci. Rep., 2015, 5, 14909 CrossRef CAS PubMed.
- J. R. McConnell, L. K. Buckton and S. R. McAlpine, Regulating the master regulator: Controlling heat shock factor 1 as a chemotherapy approach, Bioorg. Med. Chem. Lett., 2015, 25, 3409–3414 CrossRef CAS PubMed.
- P. K. Sorger and H. R. Pelham, Yeast heat shock factor is an essential DNA-binding protein that exhibits temperature-dependent phosphorylation, Cell, 1988, 54, 855–864 CrossRef CAS PubMed.
- A. P. Gasch, P. T. Spellman, C. M. Kao, O. Carmel-Harel, M. B. Eisen, G. Storz, D. Botstein and P. O. Brown, Genomic expression programs in the response of yeast cells to environmental changes, Mol. Biol. Cell, 2000, 11, 4241–4257 CrossRef CAS PubMed.
- K. K. Jovanović, M. Tanić, I. Ivanović, N. Gligorijević, B. P. Dojčinović and S. Radulović, Cell cycle, apoptosis, cellular uptake and whole-transcriptome microarray gene expression analysis of HeLa cells treated with a ruthenium(II)-arene complex with an isoquinoline-3-carboxylic acid ligand, J. Inorg. Biochem., 2016, 163, 362–373 CrossRef PubMed.
- C. Licona, M.-E. Spaety, A. Capuozzo, M. Ali, R. Santamaria, O. Armant, F. Delalande, A. Van Dorsselaer, S. Cianferani, J. Spencer, M. Pfeffer, G. Mellitzer and C. Gaiddon, A ruthenium anticancer compound interacts with histones and impacts differently on epigenetic and death pathways compared to cisplatin, Oncotarget, 2017, 8, 2568–2584 CrossRef PubMed.
- L. C. Boffa and C. Bolognesi, Methylating agents: their target amino acids in nuclear proteins, Carcinogenesis, 1985, 6, 1399–1401 CrossRef CAS PubMed.
- T. J. Begley, A. S. Rosenbach, T. Ideker and L. D. Samson, Damage recovery pathways in Saccharomyces cerevisiae revealed by genomic phenotyping and interactome mapping, Mol. Cancer Res., 2002, 1, 103–112 CAS.
- A. P. Gasch, M. Huang, S. Metzner, D. Botstein, S. J. Elledge and P. O. Brown, Genomic expression responses to DNA-damaging agents and the regulatory role of the yeast ATR homolog Mec1p, Mol. Biol. Cell, 2001, 12, 2987–3003 CrossRef CAS PubMed.
- A. A. Granados, J. M. Pietsch, S. A. Cepeda-Humerez, I. L. Farquhar, G. Tkačik and P. S. Swain, Distributed and dynamic intracellular organization of extracellular information, Proc. Natl. Acad. Sci. U. S. A., 2018, 115, 6088–6093 CrossRef CAS PubMed.
- J. Jia, F. Zhu, X. Ma, Z. W. Cao, Y. X. Li and Y. Z. Chen, Mechanisms of drug combinations: interaction and network perspectives, Nat. Rev. Drug Discovery, 2009, 8, 111–128 CrossRef CAS PubMed.
- D. Ruggero, Translational control in cancer etiology, Cold Spring Harbor Perspect. Biol., 2013, 5, a012336 Search PubMed.
- N. Shenoy, R. Kessel, T. D. Bhagat, S. Bhattacharyya, Y. Yu, C. Mcmahon and A. Verma, Alterations in the ribosomal machinery in cancer and hematologic disorders, J. Hematol. Oncol., 2012, 5, 32 CrossRef CAS PubMed.
- M. Bhat, N. Robichaud, L. Hulea, N. Sonenberg, J. Pelletier and I. Topisirovic, Targeting the translation machinery in cancer, Nat. Rev. Drug Discovery, 2015, 14, 261–278 CrossRef CAS PubMed.
- P. R. Hagner, A. Schneider and R. B. Gartenhaus, Targeting the translational machinery as a novel treatment strategy for hematologic malignancies, Blood, 2010, 115, 2127–2135 CrossRef CAS PubMed.
|
This journal is © The Royal Society of Chemistry 2020 |
Click here to see how this site uses Cookies. View our privacy policy here.