DOI:
10.1039/C9MO00160C
(Research Article)
Mol. Omics, 2020,
16, 156-164
Novel O-linked sialoglycan structures in human urinary glycoproteins†
Received
29th October 2019
, Accepted 27th January 2020
First published on 29th January 2020
Abstract
Glycopeptides represent cross-linked structures between chemically and physically different biomolecules. Mass spectrometric analysis of O-glycopeptides may reveal the identity of the peptide, the composition of the glycan and even the connection between certain sugar units, but usually only the combination of different MS/MS techniques provides sufficient information for reliable assignment. Currently, HCD analysis followed by diagnostic sugar fragment-triggered ETD or EThcD experiments is the most promising data acquisition protocol. However, the information content of the different MS/MS data is handled separately by search engines. We are convinced that these data should be used in concert, as we demonstrate in the present study. First, glycopeptides bearing the most common glycans can be identified from EThcD and/or HCD data. Then, searching for Y0 (the gas-phase deglycosylated peptide) in HCD spectra, the potential glycoforms of these glycopeptides could be lined up. Finally, these spectra and the corresponding EThcD data can be used to verify or discard the tentative assignments and to obtain further structural information about the glycans. We present 18 novel human urinary sialoglycan structures deciphered using this approach. To accomplish this in an automated fashion further software development is necessary.
Introduction
Golgi-derived protein glycosylation is among the most common post-translational modifications (PTMs). In the past three decades mass spectrometry has become the method of choice for PTM analysis, even in a high throughput manner.1 Glycopeptides represent crosslinked molecules from two biopolymer families with unique chemical and physical features that lead to different fragmentation behaviors depending on the type of MS/MS activation. Glycosidic bonds are weaker than peptide bonds. Thus, depending on the type of collisional activation, either only glycan fragments are formed mostly via single bond cleavages (ion trap CID),2,3 or some peptide fragmentation and smaller, less informative glycan fragments are observed (beam-type CID/HCD).3–5 In both cases, glycan fragmentation yields characteristic Y ions ([Nomenclature6]), the most abundant ones tend to be Y1 for N-glycopeptides7 and Y0 for O-glycopeptides.4 However, in beam-type CID peptide backbone fragments typically undergo gas phase deglycosylation without leaving any mark on the formerly modified Ser or Thr residue(s).4 Thus, these spectra may contain sufficient information for sequence identification, but assigning the glycosylation site is usually not possible. In the alternative, electron-transfer dissociation (ETD) activation almost exclusively peptide backbone fragments are formed,8 and very limited glycan fragmentation is observed (mostly sialic acid losses).9 In typical intact glycopeptide analysis mixtures of different complexity, depending on the enrichment method applied, are submitted to automated LC/MS/MS analyses, and the resulting data files are interpreted by different search engines that were more or less attuned to the specific task of glycopeptide identification.10–13 These searches produce a long list of glycopeptide assignments. Unfortunately, these lists may not be very reliable. As is the case with crosslinked peptides, and particularly because of reasons outlined above, one frequently does not get sufficient information in a single spectrum for the unambiguous assignment of both components or sometimes all components, since in O-glycopeptides multiple modifications frequently occur in a single sequence and the decorations may represent different glycans. In most cases, the search engine considers all permutations from the combination of peptides and glycans, and picks glycopeptide candidates based on the observed precursor mass and any observed peptide fragments, with the glycan assigned solely based on the mass difference between the precursor mass and unmodified peptide. However, errors in monoisotopic peak assignment; precursor ion interference; limited fragmentation; and unexpected side-reaction may all contribute to mis-assignments.14 In addition, a significant portion of the data will remain uninterpreted, partly because the structural information ‘hidden’ in the MS/MS spectra acquired with different activation techniques are handled separately. A newer MS/MS technique, EThcD enables recording spectra from intact glycopeptides that contain information about both the peptide and the glycan.15 ETD mostly results in peptide fragmentation, while the following gentle collisional activation leads mostly to single glycosidic bond cleavages.13,16 There might be a wealth of glycan structural information in these spectra, but presently search engines are not optimized to take advantage of these data. The glycan database itself could be the source of some problems. In most cases limited information is available about the protein-modifying glycans. The analysis of the glycan pool is still the best approach for detailed oligosaccharide characterization. However, significantly more sample might be necessary for such an exercise; minor components still might be lost in the process; and some structures, for example, the O-acetylated ones, will not survive the basic conditions necessary for the sugar release.
Mucin-type O-glycosylation consists of a Ser/Thr/(Tyr)-linked N-acetylgalactosamine (GalNAc) core that is usually extended into larger linear or branched oligosaccharides. These O-glycans in humans may contain N-acetylglucosamine(s) (GlcNAc), galactose(s) (Gal), fucose(s) (Fuc), additional GalNAc(s) and N-acetylneuraminic acid(s) (NeuAc).17 Some of the building blocks also may be further derivatized with sulfo or O-acetyl groups. There are 4 common mucin-type core structures.17 In human serum a-, mono- and disialo Galβ1,3GalNAc (core 1), represent the majority.18 There is no consensus sequence for this modification; potential glycosylation sites may be clustered, and multiply glycosylated sequences are common.
In the last few years we have been studying mucin-type O-glycosylation in human serum19–21 and more recently in urine samples.13,16 Our present workflow is based on lectin affinity chromatography using wheat germ agglutinin, which has enabled us to enrich a wide variety of glycopeptides from human urine. These mixtures have been analyzed by LC/MS/MS using EThcD activation. Database searches with the most common serum O-glycan compositions have yielded a less than 10% spectral assignment rate.13 Personal inspection of the data revealed some novel structures not previously reported in human urine and never linked to specified sites in specific proteins,16 but we knew that there is a lot more information in our datasets. Thus, we set out to investigate the potential occurrence of further unexpected glycan structures and used a rather simple but eventually fruitful approach.
In our data interpretation strategy, the first step is a database search with the EThcD data, where only the most common glycans are considered. This initial search delivers a set of somewhat reliable glycopeptide candidates that provide the basis for subsequent mining of both HCD and EThcD spectra. HCD spectra that feature the Y0 fragments of already identified glycopeptides could be of use (i) to provide additional confirmation of the peptide mass; (ii) to provide additional sequence confirmation in form of b and y-type peptide fragments; (iii) to discover new glycoforms: the mass increment may indicate a potential oligosaccharide composition. The usefulness of this approach was initially probed manually,16 and eventually a simple script was developed and used for this purpose (Supplement 1, ESI†). The potential new glycan structures were validated, their most likely structure was deciphered manually using both HCD and EThcD data. Obviously, the identity of the sugar units and their linkage positions cannot be assigned from these data.
Experimental
Sample preparation and mass spectrometry
We would like to emphasize that no acetate buffer or acetic acid containing solutions were used in any part of the protocol.
Urine samples were collected from 10 donors, 50 ml each (consent forms approved by the Hungarian Scientific and Research Ethics Committee, approval number: 1011/16). The previously published sample preparation protocol was followed.13 Briefly, the samples were centrifuged (5000g, 4 °C) to discard cells and other particles present in the urine; then the samples were concentrated on 10k MWCO cellulose filters to 250 μl (5000g, 4 °C). Subsequently proteins were reduced, alkylated, and digested with trypsin, then subjected to a 2-round glycopeptide enrichment using a wheat germ agglutinin affinity column collecting 3 glycopeptide fractions, the end of the flow-through peak, its shoulder and a fraction eluted by GlcNAc. Fractions were analyzed separately by LC-MS/MS using a Waters M-Class nanoUPLC on-line coupled to an Orbitrap Fusion Lumos Tribrid (Thermo Scientific) mass spectrometer. Peptides were desalted on a trap column (Waters Acquity UPLC MClass Symmetry C18 180 μm × 20 mm column, 5 μm particle size, 100 Å pore size; flow rate 10 μl min−1), and fractionated by a linear gradient of 10–30% B in 60 min (Waters Acquity UPLC M-Class BEH C18 75 μm × 250 mm column, 1.7 μm particle size, 130 Å pore size; solvent A: 0.1% formic acid/water; solvent B: 0.1% formic acid/ACN; flow rate: 300 nl min−1). MS/MS data were acquired using HCD product-ion dependent EThcD data acquisition mode. The HexNAc-specific oxonium ion, m/z 204.0867 among the 20 most abundant HCD fragments triggered EThcD acquisition. HCD spectra were acquired at 28% NCE, while supplemental activation in EThcD was set at 15% NCE. Each sample was analyzed twice, different precursors were selected for MS/MS in the consecutive LC/MS/MS experiments: (3+)–(5+) vs. (2+) ions, respectively. Trigger intensity threshold was set to 106 in a total cycle time of 3 s. All measurements were performed in the Orbitrap with a resolution of 60
000 and 15
000 for MS1 and MS/MS, respectively.
Database search
From the raw files mgf format peak lists were generated using Proteome Discoverer (Thermo Scientific, v2.2.0.388) requiring at least 40 peaks per spectrum. Spectra recorded with different activation techniques (HCD and EThcD) yielded separate peak list files. The EThcD peak lists were filtered using Protein Prospector's MS-Filter13,22 for the presence of the diagnostic oxonium ion m/z 292.1027 (mass tolerance was 10 ppm) which indicates the presence of sialic acid. The spectra that contained this oxonium ion among the 20 most abundant peaks were retained and used for database search with Protein Prospector v5.22.0. The human subset of SwissProt.2017.11.01 protein database (20417 entries), concatenated with random sequences for each protein, was searched. The enzyme was trypsin, allowing 1 missed cleavage, and semi-tryptic cleavages. Carbamidomethylation of Cys residues was defined as fixed modification. Variable modifications were acetylation, acetylation + oxidation, Met loss or Met loss + acetylation of protein N-termini, pyroglutamic acid formation from Gln at the N-termini of peptides and the oxidation of Met. For glycosylation the most frequently encountered serum glycans (HexNAcHex, HexNAcHexNeuAc, and HexNAcHexNeuAc2)18 and the disialo core 2 structure (HexNAc2Hex2NeuAc2), modifying Ser and Thr residues, were defined as common. Precursor and fragment mass tolerances were set to 5 and 10 ppm, respectively. For database searching the 80 most intense peaks were used from each spectrum and the maximum number of variable modifications per peptide was set to 2. Acceptance criteria: minimum scores: 22 and 15, maximum E-values: 0.01 and 0.05 for proteins and peptides, respectively.
GF-Hunter search
From the Protein Prospector search results a text file was created containing all unique peptide sequences only once, without the glycan, but with the other modifications retained. Nine sequences, which did not meet the acceptance criteria this time, but were unambigously identified in our previous study,16 were also included. This text file and the HCD peak list files (prefiltered for the presence of m/z 204.0867 indicative of glycosylation) were the input for GF-Hunter (Supplement 1, ESI†) that searched for the presence of Y0 (deglycosylated peptide) and Y1 (peptide + HexNAc) ions in the spectra with a mass tolerance of 10 ppm. Both ions had to be present in the same charge state, and among the 30 most intense peaks in a spectrum in order to be retained. Mass differences between the MH+ values determined and Y0 were assigned to glycan compositions from the built-in glycan list with a 10 ppm mass tolerance. The first glycan list was created from common structures, supplemented with further glycans deciphered by us previously from urine samples,16 and smaller fucosylated structures described in gastric mucins.23 Larger masses corresponding to potential common glycan combinations and Na-adducts were also included. The present list (included with the software in Supplement 1, ESI†) was gradually developed during this study.
Results
The workflow
Glycopeptides were enriched from human urine samples with a 2-step affinity chromatography protocol using wheat germ agglutinin. The resulting mixtures were analyzed with LC-MS/MS using HCD-fragment-dependent EThcD activation. Each sample was injected twice, first only higher charge states (z = 3–5) were selected for MS/MS analysis, and in the 2nd acquisition only doubly charged ions were fragmented. Earlier we have observed that the vast majority of the urinary glycopeptides were sialylated.13 Therefore, EThcD spectra featuring the diagnostic sialic acid fragment (m/z 292.1027) were used in primary database searches that were performed using Protein Prospector, and only the most common mucin-type glycans were permitted. Eventually the results were merged and identifications above the default cut-off parameters were accepted. In order to identify additional glycoforms of these peptide sequences, we developed a script (Supplement 1, ESI†), that using the identified peptide sequences as input, filters all HCD data for the presence of the gas-phase deglycosylated intact peptides (Y0) along with the corresponding GalNAc-modified sequences (Y1), and the scans featuring data for potential glycoforms are lined up in a user-friendly tabulated form (Supplement 2, ESI†). We found that requiring the presence of both these fragments increases the specificity of the filtering. From our 60 data files almost fourteen thousand HCD spectra were identified as data acquired on potential glycopeptides (Supplement 2, ESI†). The glycan structures were assigned from the mass differences between the peptides and the precursors subjected to MS/MS analysis using a glycan database that was gradually compiled based on manual evaluation of the MS2 data representing new glycoform candidates. We tentatively accepted assignments when the observed glycan mass matched within 10 ppm to a potential glycan composition. Initially, the glycan database composed of glycan masses of structures already identified16 and their combinations that may occur in the case of multiple glycosylation. Then in order to assess the existence of novel, unexpected structures we inspected the potential glycoforms of AVAVTLQSH ([342–350] of Protein YIPF3), as this peptide was present at a high level in all samples, and seems to feature only a single glycan, always on the Thr residue. O-Glycosylation of Thr-346 but not Ser-349 was also described in urine,24 cerebrospinal fluid25 and HeLa cell line.26 We gradually and manually increased the assigned glycan mass list, not only with sugar unit additions, but also O-acetylation of sialic acids, sulfation as well as Na-adduct formations were considered in the process. We proceeded with scrutinizing the potential glycoforms of other less abundant glycopeptides that featured a single potential modification site. From these 143DFTAAFPR150 of Hepatitis A virus cellular receptor 2 (Q8TDQ0) yielded novel glycan structures. Eventually the existence of 18 novel glycan structures was manually confirmed from the MS/MS data combined (Table S1 and Fig. S1–15 in Supplement 3 (ESI†), Fig. 1–3). One of these structures, with mass increment of 1515 Da (Fig. S5 in Supplement 3, ESI†), is not listed in the GF-Hunter Output, because Y1 showed a ∼13 ppm mass deviation, and thus, it was discovered with a less strict filtering. Potential alternative structures for five glycan compositions were also included in Supplement 3 (ESI†) and the diagnostic fragmentation differences are pointed out. The complete list of confirmed, individual glycan compositions is listed in Supplement 2 (ESI†).
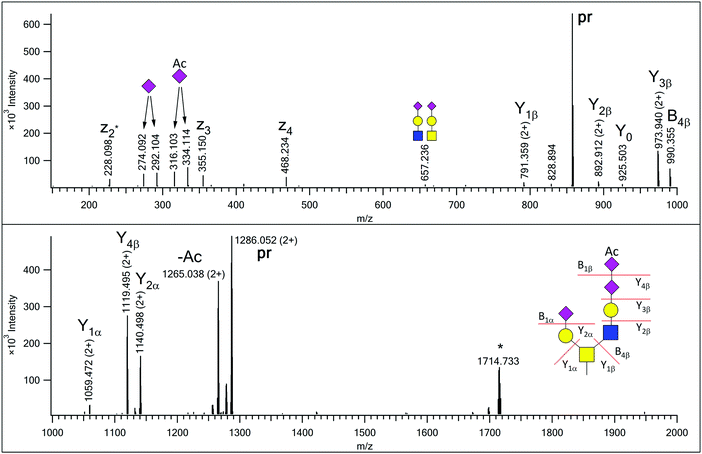 |
| Fig. 1 EThcD spectrum of AVAVT(HexNAc2Hex2NeuAc2NeuAcAc)LQSH. The precursor ion was at m/z 857.695(3+). Non-reducing-end (B) and reducing-end (Y) fragments are labeled according to the Domon–Costello nomenclature.6 Oxonium ions that represent terminal residues or are generated via multiple bond cleavages are labeled with cartoons, according to the CFG recommendations. The z2 + 1 fragment, formed via H-migration, is labeled as z2*. “pr” indicates all charge states of the precursor ion. The ion labeled with an asterisk is the charge-reduced form of a doubly charged co-eluting molecule. | |
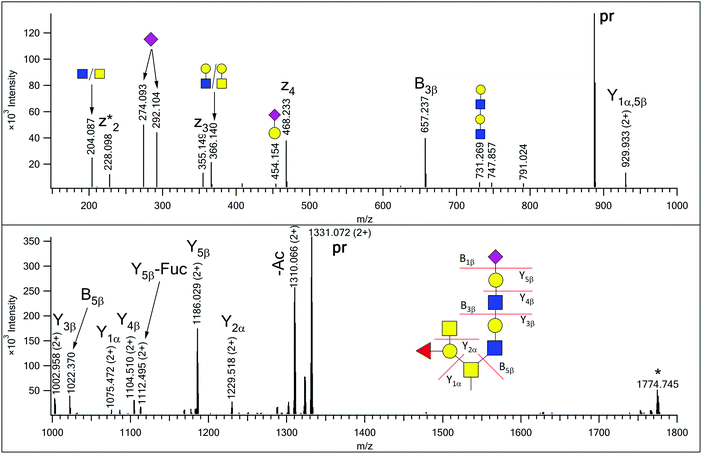 |
| Fig. 2 EThcD spectrum of AVAVT(HexNAc4Hex3FucNeuAc)LQSH. The precursor ion was at m/z 887.718(3+). Non-reducing-end (B) and reducing-end (Y) fragments are labeled according to the Domon–Costello nomenclature.6 Oxonium ions that represent terminal units or are generated via multiple bond cleavages are labeled with cartoons, according to the CFG recommendations. The z2 + 1 fragment, formed via H-migration, is labeled as z2*. “pr” indicates all the charge states of the precursor ion. The ion labeled with an asterisk is the charge-reduced form of a doubly charged coeluting molecule. There are two SDA-epitope-containing alternative structures for this glycan composition (see Supplement 3, page S16, ESI†), but the fragmentation observed does not support those structures. | |
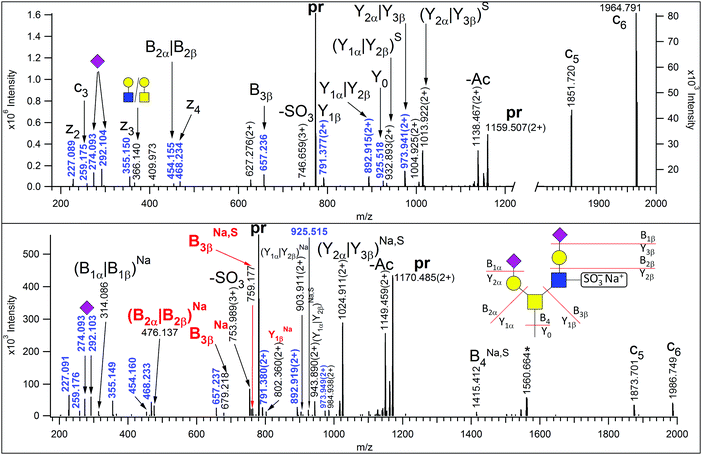 |
| Fig. 3 EThcD spectra of m/z 773.315(3+) (upper panel) and 780.640(3+) (lower panel) corresponding to AVAVT(HexNAc2Hex2NeuAc2Sulfo)LQSH and its sodium adduct, respectively. Non-reducing-end (B) and reducing-end (Y) fragments are labeled according to the Domon–Costello nomenclature.6 Oxonium ions that represent terminal residues or are generated via multiple bond cleavages are labeled with cartoons, according to the CFG recommendations. Ions indexed with Na and/or S are sodiated and/or the sulphate was retained. Masses printed in blue are assigned in the upper panel. The fragment ions decisive for sulfation site assignment are printed in red. When two fragments can be associated with the same m/z value, the labels are separated by a vertical line. The asterisk-labeled ion is the charge-reduced form of a doubly charged coeluting molecule. “pr” stands for all charge states of the precursor ions. | |
Novel glycan structures
In our earlier publication on urinary O-glycans we reported the existence of structures featuring disialic acid units as well as the occurrence of O-acetylation on N-acetylneuraminic acid.16 Now we identified two additional structures that featured both of these building blocks on a core 2 glycan (Table S1 in Supplement 3, ESI†). For the glycoform featuring a HexNAc2Hex2NeuAc2NeuAcAc composition we detected a single isoform, in which the GlcNAc-arm is capped with a disialic acid unit where the terminal N-acetylneuraminic acid is O-acetylated, and this assignment is justified by the presence of the B4β fragment (Fig. 1). In the related other glycan both terminating sialic acids are O-acetylated (Fig. S7, ESI†).
In our previous paper we reported the presence of blood-type antigens on different peptides. The AVAVTLQSH peptide was detected featuring a Type 3 A antigen, i.e. a glycan where the fucosylated galactose residue is directly linked to the core GalNAc of a Core 2 glycan (Fig. S15, upper panel in Supplement 3, ESI†).16 In the present study we discovered an extended version, where the GlcNAc-arm features an additional N-acetyllactosamine (Fig. 2), and also detected the isomeric version of the original structure, i.e. a Type 1 or 2 A antigen, where the A antigen is GlcNAc-linked (Fig. S15, lower panel in Supplement 3, ESI†). In addition, the second largest O-glycan discovered in this study (nominal mass 2391) also featured the A antigen on one of its antennae (Fig. S13 in Supplement 3, ESI†).
Last but not least, we detected one of the common structures, the disialo core 2 tetrasaccharide in a sulfated state (Fig. 3, upper panel), albeit the site of sulfation could not be determined unambiguously. However, MS/MS data were acquired from the sodium adduct of this glycopeptide as well (Fig. 3, lower panel). It has been reported that in sulfopeptides the sodium preferentially binds to the highly acidic sulfate group and thus, prevents its elimination, permitting modification site assignment.27 Indeed, the Y2β that was detected without the modification in the MS/MS spectrum of the protonated peptide, retained the sulfate when the sodium adduct was fragmented. Thus, we conclude that the GlcNAc residue of the β arm was sulfated.
Unfortunately, significantly more spectra feature the predicted Y0 and Y1 ions than the ones we could tentatively assign. When all deciphered structures, including Na-adducts, were considered 876 PSMs out of 1878 (47%) representing 51 different mass increments could be assigned to the AVAVTLQSH peptide (Supplement 2, ESI†). The success rate is a little bit better, 193 PSMs out of 365 (53%) assigned, for the DFTAAFPR sequence. Most of these assignments represent individual glycoforms. However, more glycoforms are present than masses on the list, because isomeric structures, for example, the blood-type antigen A isomers described here, have identical masses. In our previous publications we also reported positional isomers for two of the O-acetylsialic acid-containing structures.16 Some of the assignments, although valid, represent in-source fragments. PSMs featuring mass increments 494, 859, 901, 1021 and 1063 Da indicating HexNAcNeuAc, HexNAc2HexNeuAc, HexNAc2HexNeuAcAc, HexNAc2Hex2NeuAc and HexNAc2Hex2NeuAcAc compositions, respectively, are such products, and these conclusions can be validated comparing retention times (Fig. S16 and S17 in Supplement 4, ESI†). Glycoforms with different sialylation states do not co-elute,10,22 so if they are detected in the same MS1 scans then it indicates in-source fragmentation. However, we did detect ‘true’ partially sialylated structures. For example, the DFTAAFPR peptide featured mass additions of 1386, 1751 and 2116 representing HexNAc3Hex3NeuAc, HexNAc4Hex4NeuAc and HexNAc5Hex5NeuAc compositions, respectively (Fig. S4, S8 and S11 in Supplement 3, ESI†). We believe these glycoforms are not fragmentation products based on the retention time difference from the fully sialylated structures (Fig. S18 in Supplement 4, ESI†). A ±2 Da mass window, heavily populated around some confidently identified glycan masses indicates problems with the accurate assignment of monoisotopic masses. We investigated whether some of these masses corresponded to glycan structures bearing two fucoses instead of a sialic acid, but we could not find any convincing proof. For example, the 1313.4711 Da mass fits to a HexNAc2Hex2Fuc2NeuAc composition that may represent a core-2 Lewis y/b glycan. However, the reviewed data revealed faulty peak-picking and the disialo core-2 tetrasaccharide-bearing glycoform (data not shown). Other MS/MS data indicated the presence of certain peptides, but the mass difference could not be “translated” into meaningful O-glycan structures. Interestingly, 8 of the 144 peptides in the GF-Hunter input list harbor an N-glycosylation sequon. These peptides were originally assigned as bearing a characteristic mucin-type glycan. However, we cannot exclude additional/alternative N-glycosylation, and thus, the resulting additive glycan masses have to be interpreted accordingly. For example, 21 HCD spectra were assigned to the VGPVRPTGQDWNHTPQK peptide carrying a modification of ∼1353.5 Da. This latter mass might represent a glycan composition of HexNAc3Hex1NeuAc2 that can be “split” into 2 “meaningful” glycans: an O-linked core-1 tetrasaccharide (GalNAcGalNeuAc2) and a truncated N-glycan (GlcNAc2) (see Fig. S19 in Supplement 4, ESI†).
Other spectra might represent noncovalent adduct fragmentation28 or precursor ion interference due to the complexity of the mixture. In mixture spectra the glycopeptide whose Y0 and Y1 fragments led to the assignment might be just a minor component, while an unmodified peptide, another O- or N-glycopeptide may produce the majority of the fragment ions. The underlying peptide sequence of these glycopeptides might not have been identified in the primary search – that is most likely the case for N-glycopeptides, unless a database search aimed at their identification is performed separately. There are ∼750 scans in our glycopeptide candidates’ table (Supplement 2, ESI†) that are associated with multiple sequences identified in the primary search. More than half of these scans (∼460) were assigned to two different peptide pairs where even the high mass accuracy did not permit unambiguous differentiation (the MH+ differences within the peptide pairs were 11 and 2 ppm). Such situations obviously can be remedied whenever sufficient peptide fragmentation is detected. The other scans featured more than one Y0–Y1 pairs that matched to a ‘seed peptide’ accurately. In complex mixtures it is quite common that the MS/MS data derive from two or even more precursors. Thus, software has been developed to enable the identification of more than one peptide from a single MS/MS spectrum.29,30 Such feat can be achieved even from glycopeptide data (Fig. S20 and S21 in Supplement 4, ESI† illustrates the coelution of the two glycopeptides), albeit not in an automated fashion yet. In some spectra although the coveted Y0 and Y1 masses were detected within the required mass accuracy they happened to be the second isotope peaks of ion clusters that obviously cannot represent the originally identified peptide sequence. This observation confirms that relying on the mass accuracy alone is not sufficient. Considering fragmentation data and chromatographic behavior are absolutely necessary.
Finally, we created a graph displaying the distribution of the different AVAVTLQSH glycoforms based on the number of HCD spectra assigned to each (Supplement 2, ESI†). In good accordance with our earlier findings,13 only sialic acid containing structures were found, although for the HCD data used in GF-Hunter processing the presence of sialic acid was not a requirement. It is striking that the occurrence of the N,O-diacetylated neuraminic acid-containing glycoforms is equal or higher than that of their unmodified counterparts (i.e. carrying N,O-diacetylneuraminic acid instead of N-acetylneuraminic acid). This behavior seems to be unique for this particular peptide. The other N,O-diacetylneuraminic acid-containing glycoforms, confirmed until now, belong to 3 peptides: 93DVSTPPTVLPDNFPR107 of Insulin-like growth factor II (P01344), 247VWGQGQSPRPENSLER262 of Fractalkine (P78423), and 48WTHSYL53 of Basement membrane-specific heparan sulfate proteoglycan core protein (P98160). Each featured a core-1 structure modified with 3 sialic acids, as reported earlier.16 PSMs representing the ‘normal’ and N,O-diacetylneuraminic acid-containing modifications were at a comparable level (Supplement 2, ESI†). However, the more common mucin-type structures such as the disialo-core 1 or core 2 glycans were not detected with O-acetylation at all for these 3 peptides.
Discussion
Properly characterizing glycosylation microheterogeneity in natural sources is important in order to understand the biological role(s) of these PTMs. The assignment of mucin-type O-glycopeptides still represents a formidable challenge, even when only the most common glycans are considered. Numerous O-linked oligosaccharides may decorate secreted or membrane proteins. An impressive glycan database could be constructed from all human mucin-type structures ever reported. Perhaps the most diverse glycan populations have been described in gastric mucin studies with 70 and 258 glycans characterized,23,31 however, most of these did not feature sialic acids. The structures reported in this study and described by us earlier16 show that variations in sialylation may significantly increase the complexity. Performing database searches with so many glycans, especially because these have to be permitted in combination on the same sequences, may lead to impractically long searches with less reliable search results, especially when non-specific proteolytic cleavages also have to be considered – this is a common requirement for secreted proteins and body fluids in general. Thus, any measure that could restrict either the protein or the glycan database (preferentially both), will result in faster and more reliable data interpretation. Our iterative approach first used EThcD data to confidently identify glycopeptides featuring common mucin-type glycans. Then based on this information the HCD data were ‘filtered’ to find additional glycoforms. Finally, the HCD and corresponding EThcD data together were used to confirm the identity of the peptide and decipher the glycan structures. This process yielded 18 new glycan structures, a few of them above 2 kDa; quite unusual for O-glycosylation other than proteoglycans. To the best of our knowledge, this is the first large-scale study that reports the presence of such large O-glycans in a site-specific manner. Our approach ascertained that at least one EThcD spectrum (identified in the primary search) featured good enough peptide fragmentation for confident sequence and modification site assignment. The HCD data delivered by our script not only confirm the mass of the peptide and its glycosylation, but additional peptide fragments detected may strengthen the amino acid sequence assignment. In order to automatically label such potential fragments and visualize the results one could upload the filtered peak list in MS-Viewer of Protein Prospector,32 along with a properly adjusted table where all glycans are presented as neutral losses, however proper scoring of these results also has to be developed. A new feature of the program is the glycan oxonium ions’ assignment.33
Our approach may enable building a glycan database specific for the sample, without actually releasing and analyzing the glycans separately. Database searches with the adjusted glycan database would identify most of the ‘predicted’ glycoforms. Not all of them though, because peptide fragmentation might be limited in EThcD or HCD or both. Multiply modified sequences or peptides decorated with large glycan structures frequently produce almost exclusively glycosidic bond cleavages in HCD, and may not have sufficient charge density for efficient EThcD. Even in such cases we might be able to assign additional glycoforms considering retention times and just a few characteristic/diagnostic peptide fragments, in a manner similar to the spectral family approach used in earlier studies.34,35 At the same time we have to point out that without good quality ET(hc)D data the modification sites cannot be assigned, and usually only the combined glycan composition can be ascertained, not the identity of the individual glycans. Still, our approach can be used for ‘mapping’ these glycoforms in several data files permitting a semiquantitative representation of their distribution in different samples, with the reservation that the same sugar compositions may represent different glycan combinations. Such visualization of glycan distribution for an abundant glycopeptide drew our attention to the high level of glycoforms featuring N,O-diacetyl sialic acid on this particular sequence. Presently we cannot offer any hypothesis for the biological significance of this phenomenon. At the same time we think that this single ‘exceptional’ glycoform distribution also confirms that these structures are not chemical artifacts.
Low charge density or doubly charged precursor ions usually do not fare well in EThcD but may yield excellent HCD data. Unfortunately, in order to gain more information about the glycan structures, either ion trap CID or EThcD data of sufficient quality are needed, and the latter ones are a must for site assignments. In addition, different search engines weigh the presence or absence of Y0 in HCD spectra differently. It has been reported that sequences elongated at either termini, i.e. featuring a different Y0, may produce identical fragments with a shorter peptide, and that may lead to incorrect peptide and glycan assignments.6 Similarly, covalently modified peptides also may produce fragments that do not display the changes in the peptide mass leading to faulty assignments.36 Matching Y0-filtered results, search engine-assigned HCD and corresponding EThcD data may represent the perfect triage, where peptide mass, amino acid sequence confirmation, glycan size and modification site assignment are delivered automatically. For this site-assignment evaluation, like Protein Prospector's built in SLIP-score,37 and the introduction of structure-specific glycan fragmentation scoring should be integral parts of EThcD data interpretation. For this latter purpose, the lowest NCE permitted should be applied in order to prevent multiple cleavages.
Conclusions
We added 18 new glycans to the sialoglycan repertoire of urinary glycoproteins. Our data indicate that Thr-346 of YIPF3 protein may be decorated with 33 different mucin-type oligosaccharides. Among these the high level of sialic acid O-acetylation is especially interesting. Our approach, filtering for fragment ions representing the peptide unmodified or with the core GalNAc attached, can significantly speed up the identification of potential glycoforms, and it may lead to the discovery of novel glycan structures. It does not matter whether the primary identification data derive from EThcD or HCD data. However, in order to confirm the identity of the additional glycoforms, data acquired with different activation techniques, CID, HCD and EThcD data might be necessary in most cases; CID and EThcD may provide data on the glycan structure(s), while EThcD is necessary for the site assignment. Further software development is needed to utilize all the available information in an automated fashion. In highly glycosylated molecules peptide backbone fragments might not be present in any of the spectra. Thus, new data acqusition techniques also have to be developed.
Conflicts of interest
There are no conflicts to declare.
Acknowledgements
The authors wish to thank Robert Chalkley for his constructive comments. We thank the MTA Cloud (https://cloud.mta.hu/) for housing our Protein Prospector server. This work was supported by the following grants: the Economic Development and Innovation Operative Programmes GINOP-2.3.2-15-2016-00001, and GINOP-2.3.2-15-2016-00020 from the Ministry for National Economy.
References
- M. Ke, H. Shen, L. Wang, S. Luo, L. Lin, J. Yang and R. Tian, Identification, Quantification, and Site Localization of Protein Posttranslational Modifications via Mass Spectrometry-Based Proteomics, Adv. Exp. Med. Biol., 2016, 919, 345–382 CrossRef CAS PubMed.
- S. W. Wu, T. H. Pu, R. Viner and K. H. Khoo, Novel LC-MS2 product dependent parallel data acquisition function and data analysis workflow for sequencing and identification of intact glycopeptides, Anal. Chem., 2014, 86, 5478–5486 CrossRef CAS PubMed.
- J. Nilsson, Liquid chromatography-tandem mass spectrometry-based fragmentation analysis of glycopeptides, Glycoconjugate J., 2016, 33, 261–272 CrossRef CAS PubMed.
- J. Peter-Katalinić,
O-Glycosylation of proteins, Methods Enzymol., 2005, 405, 139–171 Search PubMed.
- G. Zauner, R. P. Kozak, R. A. Gardner, D. L. Fernandes, A. M. Deelder and M. Wuhrer, Protein O-glycosylation analysis, Biol. Chem., 2012, 393, 687–708 CrossRef CAS PubMed.
- B. Domon and C. E. Costello, A systematic nomenclature for carbohydrate fragmentations in FAB-MS/MS spectra of glycoconjugates, Glycoconjugate J., 1988, 5, 397–409 CrossRef CAS.
- K. F. Medzihradszky, Characterization of Protein N-Glycosylation, Methods Enzymol., 2005, 405, 116–138 CAS.
- L. M. Mikesh, B. Ueberheide, A. Chi, J. J. Coon, J. E. Syka, J. Shabanowitz and D. F. Hunt, The utility of ETD mass spectrometry in proteomic analysis, Biochim. Biophys. Acta, 2006, 1764, 1811–1822 CrossRef CAS PubMed.
- Z. Darula and K. F. Medzihradszky, Affinity enrichment and characterization of mucin core-1 type glycopeptides from bovine serum, Mol. Cell. Proteomics, 2009, 8, 2515–2526 CrossRef CAS PubMed.
- J. C. Trinidad, R. Schoepfer, A. L. Burlingame and K. F. Medzihradszky,
N- and O-glycosylation in the murine synaptosome, Mol. Cell. Proteomics, 2013, 12, 3474–3488 CrossRef CAS PubMed.
- B. L. Parker, M. Thaysen-Andersen, N. Solis, N. E. Scott, M. R. Larsen, M. E. Graham, N. H. Packer and S. J. Cordwell, Site-specific glycan-peptide analysis for determination of N-glycoproteome heterogeneity, J. Proteome Res., 2013, 12, 5791–5800 CrossRef CAS PubMed.
- R. C. Bollineni, C. J. Koehler, R. E. Gislefoss, J. H. Anonsen and B. Thiede, Large-scale intact glycopeptide identification by Mascot database search, Sci. Rep., 2018, 8, 2117 CrossRef PubMed.
- A. Pap, E. Klement, E. Hunyadi-Gulyas, Z. Darula and K. F. Medzihradszky, Status Report on the High-Throughput Characterization of Complex Intact O-Glycopeptide Mixtures, J. Am. Soc. Mass Spectrom, 2018, 29, 1210–1220 CrossRef CAS PubMed.
- Z. Darula and K. F. Medzihradszky, Analysis of Mammalian O-Glycopeptides-We Have Made a Good Start, but There is a Long Way to Go, Mol. Cell. Proteomics, 2018, 17, 2–17 CrossRef CAS PubMed.
- Q. Yu., B. Wang, Z. Chen, G. Urabe, M. S. Glover, X. Shi, L. W. Guo, K. C. Kent and L. Li, Electron-Transfer/Higher-Energy Collision Dissociation (EThcD)-Enabled Intact Glycopeptide/Glycoproteome Characterization, J. Am. Soc. Mass Spectrom, 2017, 28, 1751–1764 CrossRef CAS PubMed.
- Z. Darula, A. Pap and K. F. Medzihradszky, Extended Sialylated O-Glycan Repertoire of Human Urinary Glycoproteins Discovered and Characterized Using Electron-Transfer/Higher-Energy Collision Dissociation, J. Proteome Res., 2019, 18, 280–291 CAS.
-
I. Brockhausen and P. Stanley, “O-GalNAc glycans”, in Essentials of Glycobiology, ed. A. Varki, R. D. Cummings, J. D. Esko, P. Stanley, G. W. Hart, M. Aebi, A. G. Darvill, T. Kinoshita, N. H. Packer, J. H. Prestegard, R. L. Schnaar and P. H. Seeberger, Cold Spring Harbor Laboratory Press, 3rd edn, 2015–2017, ch. 10 Search PubMed.
- M. Yabu, H. Korekane and Y. Miyamoto, Precise structural analysis
of O-linked oligosaccharides in human serum, Glycobiology, 2014, 24, 542–553 CrossRef CAS PubMed.
- Z. Darula, F. Sarnyai and K. F. Medzihradszky,
O-Glycosylation sites identified from mucin core-1 type glycopeptides from human serum, Glycoconjugate J., 2016, 33, 435–445 CrossRef CAS PubMed.
- A. Pap, K. F. Medzihradszky and Z. Darula, Using “spectral families” to assess the reproducibility of glycopeptide enrichment: human serum O-glycosylation revisited, Anal. Bioanal. Chem., 2017, 409, 539–550 CrossRef CAS PubMed.
- A. Pap, A. Prakash, K. F. Medzihradszky and Z. Darula, Assessing the reproducibility of an O-glycopeptide enrichment method with a novel software, Pinnacle, Electrophoresis, 2018, 39, 3142–3147 CrossRef CAS PubMed.
- K. F. Medzihradszky, K. Kaasik and R. J. Chalkley, Characterizing sialic acid variants at the glycopeptide level, Anal. Chem., 2015, 87, 3064–3071 CrossRef CAS PubMed.
- Y. Rossez, E. Maes, T. Lefebvre Darroman, P. Gosset, C. Ecobichon, M. Joncquel Chevalier Curt, I. G. Boneca, J. C. Michalski and C. Robbe-Masselot, Almost all human gastric mucin O-glycans harbor blood group A, B or H antigens and are potential binding sites for Helicobacter pylori, Glycobiology, 2012, 22, 1193–1206 CrossRef CAS PubMed.
- A. Halim, J. Nilsson, U. Rüetschi, C. Hesse and G. Larson, Human urinary glycoproteomics; attachment site specific analysis of N- and O-linked glycosylations by CID and ECD, Mol. Cell. Proteomics, 2012, 11, M111.013649 CrossRef PubMed.
- A. Halim, U. Rüetschi, G. Larson and J. Nilsson, LC-MS/MS characterization of O-glycosylation sites and glycan structures of human cerebrospinal fluid glycoproteins, J. Proteome Res., 2013, 12, 573–584 CrossRef CAS PubMed.
- K. Tanimoto, K. Suzuki, E. Jokitalo, N. Sakai, T. Sakaguchi, D. Tamura, G. Fujii, K. Aoki, S. Takada, R. Ishida, M. Tanabe, H. Itoh, Y. Yoneda, M. Sohda, Y. Misumi and N. Nakamura, Characterization of YIPF3 and YIPF4, cis-Golgi Localizing Yip domain family proteins, Cell Struct. Funct., 2011, 36, 171–185 CrossRef CAS PubMed.
- K. F. Medzihradszky, S. Guan, D. A. Maltby and A. L. Burlingame, Sulfopeptide fragmentation in electron-capture and electron-transfer dissociation, J. Am. Soc. Mass Spectrom, 2007, 18, 1617–1624 CrossRef CAS PubMed.
- K. F. Medzihradszky, Noncovalent dimer formation in liquid chromatography-mass spectrometry analysis, Anal. Chem., 2014, 86, 8906–8909 CrossRef CAS PubMed.
- X. Chen, P. Drogaris and M. Bern, Identification of tandem mass spectra of mixtures of isomeric peptides, J. Proteome Res., 2010, 9, 3270–3279 CrossRef CAS PubMed.
- J. Wang, P. E. Bourne and N. Bandeira, Peptide identification by database search of mixture tandem mass spectra, Mol. Cell. Proteomics, 2011, 10, M111.010017 CrossRef PubMed.
- C. Jin, D. T. Kenny, E. C. Skoog, M. Padra, B. Adamczyk, V. Vitizeva, A. Thorell, V. Venkatakrishnan, S. K. Lindén and N. G. Karlsson, Structural Diversity of Human Gastric Mucin Glycans, Mol. Cell. Proteomics, 2017, 16, 743–758 CrossRef CAS PubMed.
- P. R. Baker and R. J. Chalkley, MS-viewer: a web-based spectral viewer for proteomics results, Mol. Cell. Proteomics, 2014, 13, 1392–1396 CrossRef CAS PubMed.
-
R. J. Chalkley OMICS this issue.
- N. Bandeira, Spectral networks: a new approach to de novo discovery of protein sequences and posttranslational modifications, BioTechniques, 2007, 42, 687–691 CrossRef CAS PubMed.
- A. Pap, K. F. Medzihradszky and Z. Darula, Using “spectral families” to assess the reproducibility of glycopeptide enrichment: human serum O-glycosylation revisited, Anal. Bioanal. Chem., 2017, 409, 539–550 CrossRef CAS PubMed.
- Z. Darula and K. F. Medzihradszky, Carbamidomethylation Side Reactions May Lead to Glycan Misassignments in Glycopeptide Analysis, Anal. Chem., 2015, 87, 6297–6302 CrossRef CAS PubMed.
- P. R. Baker, J. C. Trinidad and R. J. Chalkley, Modification site localization scoring integrated into a search engine, Mol. Cell. Proteomics, 2011, 10, M111.008078 CrossRef PubMed.
Footnote |
† Electronic supplementary information (ESI) available. See DOI: 10.1039/c9mo00160c |
|
This journal is © The Royal Society of Chemistry 2020 |