DOI:
10.1039/D0LC00628A
(Paper)
Lab Chip, 2020,
20, 3772-3783
Time-resolved microwell cell-pairing array reveals multiple T cell activation profiles†
Received
17th June 2020
, Accepted 28th August 2020
First published on 31st August 2020
Abstract
The differences in behaviour between individual cells in a large population are often important, yet are masked in bulk analyses where only average parameters are measured. One unresolved question in the field of immunology is the extent to which important immunological phenomena such as immunodominance to cancer antigens correlates with the average activity of a population of antigen-specific T lymphocytes, or with the activity of individual “outlier” cells. Despite progress in single cell technologies, few platforms are available that can deliver time-resolved, functional analysis at single cell resolution, for these investigations. We have developed an accessible high-throughput platform to measure single T cell signalling in real time following time-controlled stimulation by live antigen presenting cells. The cell-trap array consists of thousands of individual microwells cast in an agarose block, which is biocompatible and permeable to nutrients. Single T cells are isolated in wells via passive sedimentation and size exclusion, achieving up to 90% occupancy. The device enables simultaneous activation of thousands of single CD8+ cells. Stimulation with soluble reagents (ionomycin, anti-CD3 antibodies) or antigen presenting cells leads to changes in intracellular calcium concentrations which were measured using calcium-chelating fluorophore dyes. The platform was used to demonstrate a range of activation profiles among individual cells of a cloned, antigen specific CD8+ T cell hybridoma in response to both nonspecific stimuli and specific, physiologically relevant antigen stimulation. The presence of two different activation profiles was demonstrated, together with rare outlier behaviour among cells that are essentially clonal.
Introduction
Antigen presenting cells (APCs) degrade proteins that originate from pathogens or cancer cells, loading peptide fragments, called epitopes, onto newly synthesised major histocompatibility complex (MHC) molecules. The resulting peptide
:
MHC (pMHC) complexes are then transported to the cell surface where they can be recognized by specific CD8+ cytotoxic T lymphocytes (CTL). Upon contact with APCs loaded with pathogenic antigens, these lymphocytes become activated, and can release granules containing cytotoxins into infected cells, killing them and preventing the spread of an infection to neighbouring cells, or the spread of cancer (Fig. 1). Cytotoxic T cells have receptors that are specific for a particular pMHC and growing evidence indicates that the precise specificity of CTL is important for its in vivo efficacy, be that clearing a viral infection or fighting cancer. Indeed, there are physiologically relevant differences between different CTLs with the same pMHC specificity; the precise mechanism underpinning this is not fully understood. Therefore, tools to measure T cell function at the single cell level are badly needed in order to fully investigate the correlation between CTL specificity and in vivo activity.
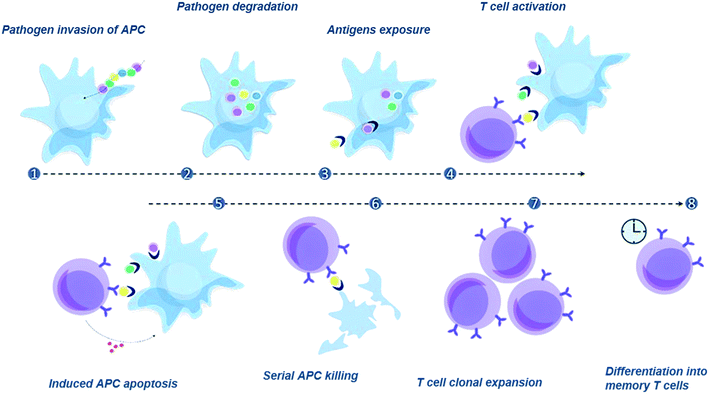 |
| Fig. 1 Schematic diagram demonstrating CD8+ T cell activation. 1. A pathogen enters an antigen presenting cell (APC); 2. its proteins are degraded into fragments called epitopes; 3. some epitopes (antigens) are bound to MHC molecules and exposed onto the cell surface; 4. T cells specific to the exposed epitopes contact the peptide-loaded APC, creating an immunological synapse; 5. the activated T cell secretes cytokines and cytotoxic granules; 6. cytotoxic granules induce apoptosis in the APC, and the T cell moves to kill the next infected cell (serial killing); 7. activated T cells undergo clonal expansion and acquisition of effector function; 8. after the expansion phase, up to 95% of the clone T cells become apoptotic, and the remaining clones differentiate to become memory T cells, providing long-lasting protection to the host. | |
Microfluidics and single cell technologies have provided new tools to investigate time-resolved single cell activation.1–10 High-throughput approaches include devices where two cell populations are sequentially trapped to create cell–cell contacts, or aqueous droplets where two cells are co-encapsulated and thereby interact. However, the transferability of such platforms to biomedical laboratories remains a challenge, particularly where specialist equipment or personnel are required.
Amongst the various technologies that allow isolation and analysis of single cells, three high throughput methods are used for single cell pairing, namely hydrodynamic trapping in a flow (closed system),11–16 sedimentation in micro-well arrays (open format)17–21 and containment in microfluidic droplets.22–24 Of these techniques, hydrodynamic traps and microwell arrays are the only techniques where the cell–cell contact time can be synchronised.
Voldman et al. developed a microfluidic trapping technology that can both couple and retrieve pairs of cells.12,25,26 Voldman and Dura also reviewed the range of microfluidic tools for the study of immune cell interactions2 and concluded that the open design of microwell arrays provides an easy method for single cell retrieval using micromanipulators. Compartmentalisation of the wells also enables analysis of excreted cytokines. However, disadvantages include limits on single wells occupancy (dictated by Poisson statistics) which lead to lower throughput per footprint compared to closed systems. Furthermore, microwells have been rarely considered for studies that involve time controlled cell–cell interactions. Synchronized vertical cell–cell contact using microwells has been achieved via centrifugation,17,18 but this doesn't allow analysis of the very first seconds of contact between cells, due to the time needed to wash the device and move it to a microscope. In fact, T cell activation occurs within seconds27 after encountering a target antigen, highlighting the need for a system that allows synchronized cell–cell contact with real-time recording of the first few minutes of interaction.
This paper describes a simple system that can be used to study multiple cell–cell interactions, enabling investigation of single cell activation in response to soluble reagents and epitope-presenting cells. The system consists of an agarose multi-well array that is used to trap thousands of individual lymphocytes. Epitope presenting cells are cultured as a monolayer on an opposing surface as shown in Fig. 2. The two surfaces are brought into contact to initiate signalling which is recorded using fluorescence. Microwell arrays are easy to fabricate and use; they do not require specialist equipment or fabrication techniques and can be scaled to accommodate a range of cell sizes and numbers, which can be an order of magnitude greater than typically screened with microfluidic devices. Multi-wells arrays have been used to capture single cell cytokines using capture antibodies patterned onto a lid,28 or to pair two cell populations using sequential centrifugation.17,18 However, this technology is particularly amenable to real-time measurement of single T cell activation upon synchronized contact with APCs. The novelty of the platform lies in its simplicity together with synchronised, timed control of multiple cell–cell interactions.
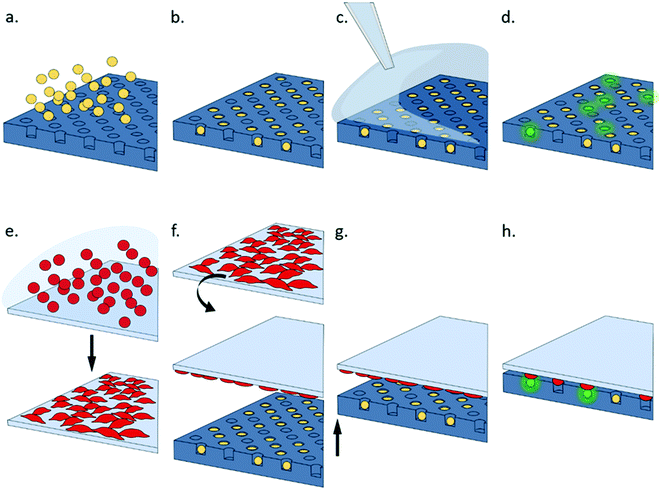 |
| Fig. 2 Working principle of the cell pairing platform. A suspension culture of T cell hybridoma cells are layered onto the agarose array (a), and excess cells washed away leaving up to 90% cell trapped (b). Adherent APC are grown to confluence on a flat (glass) surface (e) then pulsed with antigenic peptide epitope before being inverted (f). T cells are activated by either soluble stimulants pipetted onto the plate (c) or via contact with the monolayer of APCs (g); the time resolved activation of single cells is recorded with fluorescence microscopy (d, or h). | |
Methods
Cell size measurement
Cell trapping is based on size exclusion. Design optimisation required accurate determination of cell size, which was measured by single cell microfluidic impedance cytometry calibrated against polystyrene beads (see ESI† 1). For the cells used in this work, the average diameters were 12.84 ± 1.53 μm for B3Z (3 samples, 254 cells) and 12.37 ± 1.18 μm for K89 (3 samples, 725 cells).
Cell culture
RPMI 1640 culture medium (with L-glutamine, Gibco) was supplemented with 10% v/v heat-inactivated FBS (standard quality, EU approved, GE Healthcare), 1% v/v penicillin–streptomycin (Sigma-Aldrich), 1% v/v HEPES Buffer (1 M, Lonza), 1% v/v sodium pyruvate solution (100 mM, Sigma-Aldrich) and 500 nM β2-Mercaptoethanol (Sigma-Aldrich). Adherent K89 and suspension B3Z were cultured in complete RPMI at 37 °C, 5% CO2 and harvested as necessary to keep them in their log phase. 1 mM EDTA (Biowhittaker) was added in the passaging of K89, and the population was gently detached from the flasks mechanically, using a Pasteur pipette. Limiting dilution sub-cloning was initially used on the lymphocytes population to select for cells that were highly sensitive to SIINFEKL (SL8) epitope, and sensitivity assays were periodically run to ensure the functionality of the cell model (see ESI† 2).
Staining protocols
An early response to T cell activation is associated with an increase in intracellular levels of Ca2+.29 Lymphocytes were stained with calcium-dependent fluorophores to monitor activation. B3Z T cells were stained with Fluo-8AM (Abcam; excitation 490 nm, emission 520 nm, Kd = 390 nM), which binds to free calcium in the cytoplasm. This dye is suitable both for flow cytometry and fluorescence microscopy. Cells were incubated in growth media containing 5 μM Fluo8-AM for 30 minutes at 37 °C, then a further 30 minutes at room temperature. B3Z cells were then washed in fresh culture media 3 times before being resuspended. There is always a non-zero intracellular calcium level, so that the stained cell population has a non-zero basal fluorescence (referred to as mean fluorescence intensity zero, MFI0). Fluctuations in the cytoplasmic ion concentration result in a shift in the fluorescence signal.
Where specified, K89 cells were stained using a red membrane dye (PKH26; excitation 551 nm, emission 567 nm). Staining both cell populations helped in gating during flow cytometry and to confirm co-localization of activated T cells and APCs by imaging. In these cases, K89 were resuspended in 250 μl of diluent C; the suspension was mixed at a 1
:
1 ratio with 4 μM PKH26 solution prepared in diluent C, and the sample was left for 3.5 minutes with shaking at room temperature, followed by addition of 500 μl FBS. After another minute at room temperature, 1 ml of fresh media was added and the sample washed twice with fresh PBS.
Flow cytometry
Cells were analysed by Flow cytometry (BD Accuri™ C6) at 35 μl min−1; data was analysed with FlowJo. Viable cells were gated on forward scatter
:
side scatter (FSC
:
SSC), and single cells selected from the forward scatter-height
:
forward scatter-area (FSC-H
:
FSC-A) graph. B3Z were gated based on their basal green fluorescence MFI0, and their fluorescence signal monitored over time to assess any change in MFI (i.e. activation) after stimulation. Soluble stimulants (antiCD3 antibodies and ionomycin ionophores) were added to the sample after an initial measurement of MFI0. Flow was stopped, stimulant added to the sample with vortexing and the flow re-started. T cell signalling was monitored for 3 minutes after stimulation. For stimulation with antiCD3, the flow was stopped a second time, followed by addition of ionomycin then measurement for a final minute.
For biological stimulation with antigen presenting cells, K89 were pulsed with SL8 peptide by incubating the sample in growth media supplemented with different concentrations of epitope for 1 hour at 37 °C, 5% CO2. Cells were subsequently washed to remove unbound peptides and resuspended at the desired concentration in fresh medium. The minimum incubation time for optimal loading was 20 minutes. A chase experiment to test the duration of epitope exposure showed that 120 minutes after washing the cells (to remove unbound peptide), the exposed concentration was reduced by approximately 30% (see ESI† 3). For flow cytometry experiments, after recording the basal fluorescence of the B3Z population, data acquisition was stopped and K89 were added to the sample at a 1
:
1 cell ratio in 50 μl of media. To initiate cell–cell interactions, the sample was then spun at 2000 rpm (371 g) for 3 minutes, and quickly resuspended before restarting recording.
Serial dilution experiments were performed to define the appropriate concentrations of soluble stimulants (antiCD3 and ionomycin) and antigen concentrations for the single cell experiments (see ESI† 4 and Results).
Device design and fabrication
The cell trap consisted of 324 separate arrays with each array containing 4200 individual traps arranged in a hexagonal close packed grid as shown in Fig. 3a. Based on cell size measurements, the well diameter was set at 20 μm to ensure a single B3Z could be trapped. The horizontal shift between centres was 35 μm, and vertical shift between rows 30.31 μm. Trap arrays were made by pouring molten agarose onto a master made from SU8 (see ESI† 5). 2% w/v agarose solution was prepared by dissolving agarose powder (UltraPureTM Agarose, Invitrogen) in DPBS (Dulbecco's phosphate buffered saline, Sigma-Aldrich). The agarose was then cooled to 50 °C with continuous stirring and poured onto a master mould, to give a uniform thickness of approximately 4 mm. After cooling, the solid hydrogel edges were cut to size and the gel flipped onto a plastic support, where it was further diced into single devices to fit a 2 × 2 × 0.2 cm holder. The agarose devices could be stored at 4 °C for several days, without loss of feature definition.
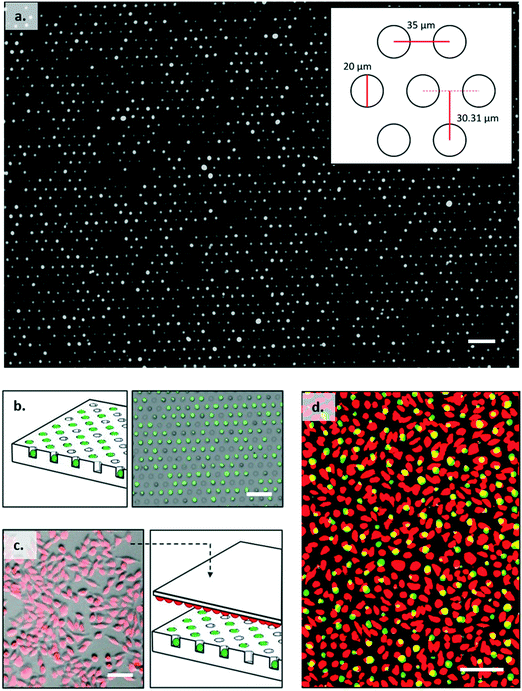 |
| Fig. 3 Experimental images of a T cell : APC array. (a) Image of an assembled trapping array containing B3Z T cells imaged using Fluo8-AM staining. (b and c) Schematic of the assembly mechanism: (b) Single T cells labelled with Fluo8-AM (green) are trapped in the hydrogel plate; (c) antigen presenting cells stained with PKH26 dye (red) are adhered to a flat surface and pulsed with SL8 antigen; (c and d) the slide is then flipped to face the T cell array. Cell contacts are formed by lifting the hydrogel plate against the flat surface. Scale bar is 100 μm. | |
Cell seeding
Cell trapping occurred by sedimentation of single cells into wells. The agarose microwell array was cut into 2 cm2 squares, each square consisted of 105
000 wells in total. A cell suspension was carefully pipetted onto the trapping array and the plate was incubated for 30 minutes at 37 °C, 5% CO2. Excess cells were removed from the surface by repeatedly washing the agarose surface with PBS using a wash bottle with the gel tilted at roughly 30°, and draining excess solution with absorbing paper from the bottom edge of the gel. Agar plates were checked with a microscope to make sure that there were no floating cells left (Fig. 3a and b). The agar array was then placed onto a customized holder. Cell loading was optimised by testing different seeding concentrations (see ESI† 6). For B3Z this was one million cells in 300 μl of culture media giving a 10
:
1 cell
:
well ratio. This led to 90% occupancy.
Coupling lid
Single cell activation experiments using antigen presenting cells were performed using a confluent layer of K89 cells grown on a glass slide (76 × 52 mm, VWR). Ultra-Thin Silicone Films (ELASTOSIL®, Silex) were cut to the size and bonded to the glass slide using oxygen plasma. After plasma-activating the unbound surface of the PDMS layer, a drop of 20 μg ml−1 fibronectin (Gibco) was spread on the surface and the slide was incubated for 30 minutes at room temperature, before washing off the excess solution with PBS. This substrate preparation was optimal for cell adhesion and subsequent displacement upon contact with the agarose wells. A suspension of K89 in media containing 1% FBS was then added to the substrate. Following a 2 hour incubation at 37 °C/5% CO2, unattached cells were washed away using fresh medium and the slide was ready for the imaging experiments. In experiments that required staining of the cells, K89 were stained with PKH26 membrane dye before being adhered onto the glass/PDMS surface.
Device assembly and image capture
The microwell array was placed in the holder shown in Fig. 4a and b, which consisted of a 5-axis aligner (New Focus, 9082, Newport) mounted onto a PMMA holder fitted for the microscope stage; on top of the aligner, a rotation stage (RP01, Thorlabs) was added, to give an extra degree of freedom in the positioning of the agarose array. Given that the agarose device is homogeneously flat, the only strict requirement is control in the Z-axis. For the experiment, initially the Z-axis was set to the lowest position. The inverted lid with the K89 cells (loaded with peptide) was magnetically clamped to the holder, and the latter carefully positioned on top of the agarose device, using spacers of the appropriate thickness, again held in position by magnets. The device was placed on the stage of a Zeiss AxioImager M2m confocal microscope fitted with a Zeiss EC Plan-Neofluar 5× objective, giving a final magnification of 50×. We used a lower magnification in order to maximise the throughput of our analysis. With the AxioCam MRm Rev.3 firewire digital cooled monochrome camera we were able to cover a field of view of 1789.23 μm × 1340.31 μm, having 1 pixel = 1.29 μm and a maximum lateral resolution of 2.082 μm. The microscope was focused onto the adherent cell layer, and the T cell array moved into contact by slowly moving the Z-axis of the micromanipulator. Contact between the surfaces was evaluated visually (an image of the surfaces before and after contact is shown in Fig. 4c and d), and once it was achieved, a time series (frame rate 2 fps, duration 2–5 minutes, exposure time approx. 100 ms) of images (FITC channel) was recorded.
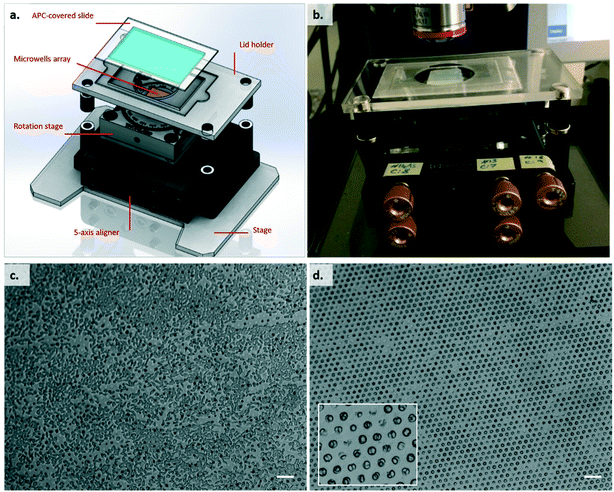 |
| Fig. 4 Diagram and photograph of the cell trapping array mounted onto a fluorescence microscope stage (a and b). Sequential images of the same plate pair before (c) and after (d) the agarose plate is sealed against the glass slide using the Z-axis control of the aligner, with the focus kept on the K89 cells. The K89 are naturally displaced to align with the wells when the agarose array is brought in contact, initiating synchronous contact between APC and single T cells. Scale bars 100 μm. | |
Stimulation with soluble reagents
Experiments with soluble stimulants (anti-CD3 antibodies and ionomycin), were performed without the need for the lid. 20 μl of soluble reagent were directly pipetted at the required concentration on top of the array. The fluorescence signal from single cells was recorded following stimulation, with time zero set as the point of stimulant addition. After approximately 3 minutes after anti-CD3 stimulation, 1 μM ionomycin was added to the same spot, and fluorescence recording continued for a further 1.5 minutes.
Data processing
The time series of fluorescent images was post-processed using ImageJ and MATLAB. Following background subtraction, cells were automatically located from their fluorescent signal using the “Find Maxima” built-in function of ImageJ. Circular ROIs were automatically designated around the maxima using a macro, adapted from the “DesignROI” macro, and assigned an arbitrary number of pixels as a diameter (using a 5× objective, 14 pixels was optimal for B3Z cells, see ESI† 7). The “Time Series Analyser” plug-in from ImageJ was used to extract the average fluorescence signal of each single cells over time as a matrix. The table, containing maxima coordinates with corresponding cell labels and their MFI, was processed in MATLAB. Signals were normalized to the initial value and the percentage increase in fluorescence above the basal signal was determined from (MFI − MFI0)/MFI0 × 100. Normalized data were corrected to compensate for gradual loss of fluorescence due to photobleaching and/or dye degradation inside the cell. This was done by using non-responding cells as a reference. The reduction in fluorescence signal from these cells was used to normalise the signals from cells of interest (responders). For the figures shown in this paper, all cells were manually selected. Automatic selection criteria using two methods were tested. A threshold method identified responders as cells with signals that, at any point, had a fluorescence value higher than an arbitrarily selected threshold. Alternatively, the slope method was based on two manually selected parameters: the intensity shift and the number of frames over which the shift occurred (time window). Cells were classified as responders when, at any point during the recording, an intensity shift greater than the selected one happened within the selected time window. These methods provided similar data but the manual selection method proved easier at identifying trends in response. In all cases, responders were automatically identified to a geometric location on the plate. Manual identification of hundreds of single cells of interest amongst thousands of traces could be performed within less than 30 minutes.
Results and discussion
T cell activation with ionophore and soluble anti-CD3 antibody
For this study, a biological model comprising two cell lines was used: the murine CD8+ T cell hybridoma B3Z and the mouse fibroblast cell line K89. B3Z has a T-cell receptor (TCR) specific for the hen egg ovalbumin (OVA) derived peptide SIINFEKL (single amino acid nomenclature, also referred to as SL8), presented by the H-2Kb MHC class I alloantigen molecules on the surface of antigen presenting cells. K89 expresses H-2Kb, and hence can be used as an APC in B3Z stimulation assays.30–32
T cells can also be stimulated using anti-CD3 antibodies which bind to the T cell receptor signalling complex, bypassing the need for cognate (pMHC) recognition.33–35 Ionomycin is a calcium ionophore that binds to extracellular ions, transporting Ca2+ across the cell membrane. It also triggers generation of IP3 in the cells, prompting the release of internal stores of calcium through the IP3, thereby activating T cells non-specifically. For this reason, intracellular calcium increase after ionomycin addition was used to validate the experimental system.
Fluo-8AM dosed B3Z T cells were arrayed into the trap, and stimulated with anti-CD3 antibody, followed by a second stimulation with ionomycin. Fig. 5a shows that anti-CD3 activation leads to an increase in cytosolic calcium release with a delay of approximately one minute for all responding cells, consistent with results obtained using flow cytometry (see ESI† 4). While only a fraction of the population of B3Z responded to anti-CD3, the response to the calcium ionophore ionomycin was widespread. For analysis, traces were normalized to MFI values at t = 25 s (addition of antiCD3). Therefore, the timescale in Fig. 5b–d was rearranged with a horizontal shift of 25 s.
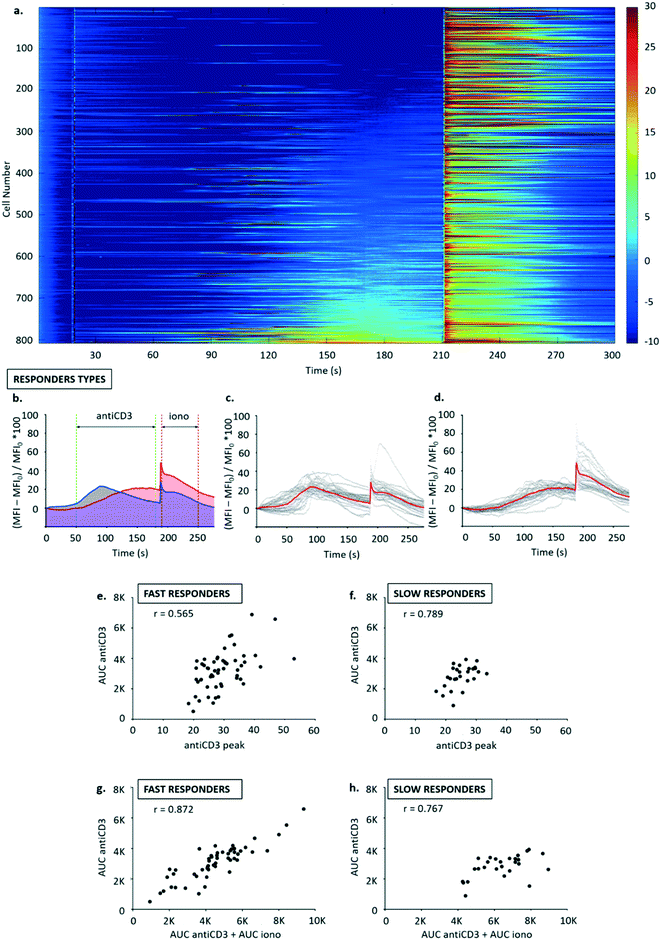 |
| Fig. 5 (a) Heatmap of fluorescent signals (MFI) recorded from a population of B3Z cells. Each row represents the time signal for a different lymphocyte, and the traces were sorted based on their average signal in the time window 30–180 s. (b–d) Signals were normalized as (MFI − MFI0)/MFI0 × 100 from the instant of antiCD3 addition (t = 25 s). Two activation profiles were classified from the dataset, by manually selecting traces belonging to the subgroups: “fast” and “slow” activators; the average signals of the two subpopulations are overlaid in (b) (blue = fast, red = slow) and shown separately in (c) “fast” and (d) “slow”. Correlation between key parameters was calculated from plots of: calcium released by anti-CD3 (AUC antiCD3) versus maximum reached during anti-CD3 activation (e and f); calcium released by anti-CD3 (AUC antiCD3) versus total calcium store (AUC antiCD3 + AUC iono) (g and h) for fast (e and g) and slow (f and h) responders. | |
Two different response profiles were observed as shown in Fig. 5b–d. A minority of cells (13.54 ± 1.24% of a total 2446 cells observed in 3 experiments) responded to anti-CD3 with a steep leading edge, a defined peak and a shallow trailing edge. Around 50% of the cells, however, showed a slow and steady increase in fluorescence (Fig. 5a). Subpopulations such as these could not be distinguished by flow cytometry, and illustrate the additional information achieved by continuous single-cell analysis.
The two types or responders seen in Fig. 5a (“fast” and “slow”) were manually binned into separate populations (see Fig. 5b–d), and three activation parameters were calculated for each population: (i) calcium release in the time window 50–180 s was quantified from area under curve following antiCD3 stimulation (AUC antiCD3); (ii) calcium release in the time window 190–250 s quantified as area under curve following ionomycin stimulation (AUC iono); (iii) peak calcium (antiCD3 peak) was calculated as the highest signal recorded in the time window following anti-CD3 stimulation (50–180 s).
Correlation analysis was performed for: calcium released with anti-CD3 (AUC antiCD3) vs. peak calcium (antiCD3 peak), to metricise the degree of similarity in the activation profiles of all T cells in the group (Fig. 5e and f); calcium released with anti-CD3 (AUC antiCD3) vs. total calcium store (AUC antiCD3 + AUC iono), to assess whether anti-CD3 triggers the release of the same proportion of the total calcium store across the population (Fig. 5g and h).
Generally, the correlation was good for the parameters selected. Homogeneity in activation profiles seemed to be higher in slow responders compared to fast responders (r = 0.789 vs. r = 0.565), and the proportion of total calcium store released following anti-CD3 stimulation seemed consistent in both groups (r = 0.872 for fast and r = 0.767 for slow responders). This may be physiologically relevant because it suggests that, especially in the minority of T cells that are “fast responders”, the likelihood of activation following a given input signal via TCR may be higher for T cells with a stochastically higher capacity for storing intracellular calcium.
T cell activation with live antigen presenting cells (APC) using flow cytometry
After recording the basal fluorescence of the B3Z population, K89 cells pulsed with SL8 peptide at a 1
:
1 cell ratio were added to the sample. The T cell – APC contact was induced by spinning down the sample, which was then resuspended and the fluorescence signal measured post stimulation. Different centrifugation speeds and durations resulted in different percentages of T cell activators (see ESI† 8), suggesting that the contact force plays a role in T cell activation (as supported by the literature36–39).
Synchronised T cell activation with live antigen presenting cells (APC)
Synchronous activation of B3Z T cells with monolayers of live APCs (cell line K89) were explored as outlined above. A control experiment using a cell-free PDMS substrate failed to elicit any response from T cells indicating that mechanical disturbance of the cells was not an issue (see ESI† 9).
The specificity of the APC response was demonstrated by comparing single-cell Ca2+ signals after contact with APCs and no peptide epitope with APC pulsed with 1 μM of the cognate epitope SIINFEKL. 70% of the B3Z cells trapped in the array and exposed to K89 pulsed with 1 μM SIINFEKL epitope (over a total of 1200 individual cells measured in a single field of view) showed a response, with very little background activation as shown in Fig. 6a and b (and in ESI† 9).
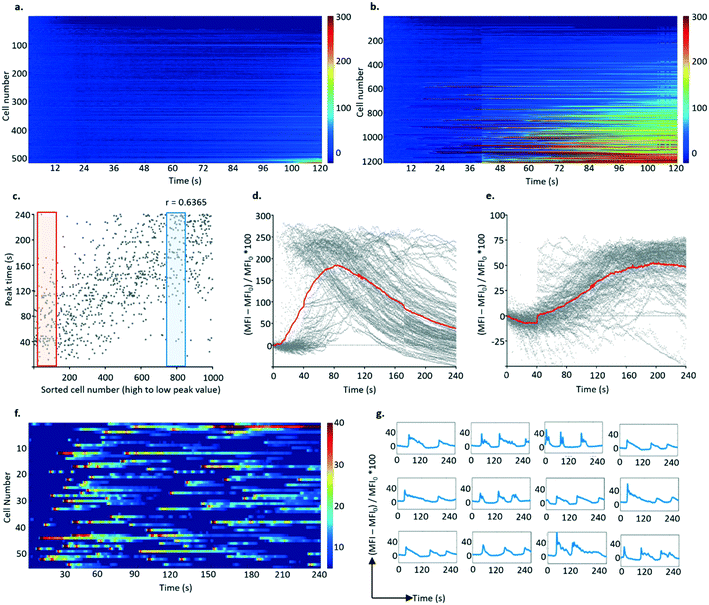 |
| Fig. 6 Activation profiles of single B3Z T cells following pairing with K89-covered lids. Heatmap of B3Z fluorescent signals after contact with K89, in absence (a) or presence (b) of 1 μM SIINFEKL. B3Z were trapped in agarose devices, while K89 were grown confluent onto a flat surface, flipped onto the hydrogel array. Comparison of two kind of T cell responses. B3Z profiles were sorted based on the peak value of their signalling profile, and (c) illustrates that cells that showed higher responses generally peaked earlier (Pearson correlation coefficient 0.6365). Two subpopulations of responders were selected, “fast” responders, gated in red and plotted in (d), and “slow” responders, gated in blue and plotted in (e). In both plots (d and e) the average of the responses is highlighted in red. Examples of cell traces that showed multiple peaks in response to K89 pulsed with 1 μM SL8 are reported in (f) in a heatmap format and in (g) as single traces. | |
Similar to observations with anti-CD3 stimulation, two kinds of responses were observed (Fig. 6c–e): fast responders (with rapid calcium accumulation occurring 30 s after stimulation, rising to a peak over the next 20 s followed by a slow decrease) and slow responders (where the fluorescence signal increased at 80 s from stimulation but to a lower level, which was reached at 150 s). In some experiments, a third type of activation profile was observed in the form of multiple peaks recorded during the 240 s window (Fig. 6f and g). Although individual activation “spikes” for these cells occurred in the same time window as for cells displaying a fast activation profile, the response of this population seems refractory to stimulation.
To confirm that activation only occurred in cells that were in close contact with APCs, the APCs were labelled with the red membrane dye PKH26 to enable co-visualisation with the T cells. ROIs were created around each individual K89 cell to filter the FITC time series, proving that the activated T cells shared the well with antigen presenting cells. Similarly, after analysing calcium oscillation of lymphocytes (from the FITC time series) and determining responders, their ROIs were superimposed on the PKH26 dye-labelled cell image, to identify the positions of K89 cells. As expected, activated B3Z cells could only be spotted in ROIs with PKH26 (red) signal, meaning that antigen presenting cells were in contact with the responders during the recording (see ESI† 10).
T cell activity is frequently quantified as a dose–response relationship using in vitro functional assays in which peptide ligand is titrated over 2 orders of magnitude or more in concentration. Therefore, the correspondence between the average activation of the bulk population versus activation at the single-cell level for B3Z was investigated. This experiment would reveal whether the measured activity of the bulk T cell population was dominated by the average activation profile or the activation profile of a particular subpopulation. Fig. 7a and b show the activation profile of B3Z cells in response to peptide in the 10 μM to 10 pM range measured by flow cytometry. The results are also presented as the % of cells exceeding an arbitrary threshold of fluorescence intensity (see Methods). In this experiment, the concentration of peptide required for half-maximal activation was between 1 and 100 nM.
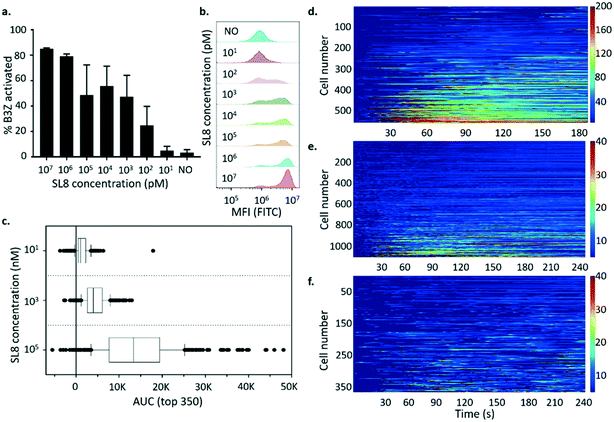 |
| Fig. 7 B3Z T cell activation after contact with K89 cells pulsed with different concentrations of SL8 peptide. Percentage of activators against different SL8 concentrations (events after pelleting the sample that are exceeding the basal fluorescence gate on MFI-FITC drawn on the sample before addition of K89, n = 3) are shown in (a), and an example of fluorescence histograms for each concentration in (b). “NO” represents the control of B3Z exposed to K89 in absence of peptide. Activation profiles of single B3Z T cells following pairing with K89-covered lids, where K89 were pulsed with different concentrations of SL8, were sorted based on the AUC after cell–cell pairing. The AUC of the top 350 responders for each concentration is shown in (c): the box plot covers data from 25th to 75th percentiles (with median highlighted), the whiskers include data from 10th to 90th percentiles. Heatmaps of single T cell activation profiles are shown in (d–f): (d) responders to K89 pulsed with 100 μM peptide (results from 5 recordings from different arrays, 9.77% responders identified); (e) activators stimulated with 1 μM epitope (results from 3 recordings from different arrays, 46.7% responders identified); (f) activators stimulated with 10 nM SL8 (results from 3 recordings from different arrays, 24.4% responders identified). | |
B3Z arrays were exposed to K89 pulsed with different concentrations of SIINFEKL peptide (100 μM, 1 μM and 10 nM) as shown in Fig. 7d–f. In these experiments, the dose–response relationship was clear when comparing the activation profiles of the top 350 responding cells in each array (Fig. 7c). However only a small percentage of trapped B3Z (9.77%) were activated in response to 100 μM peptide compared to 46.7% for 1 μM and 24.4% for 10 nM peptide. It is not clear whether this observation is significant, but it is consistent with a pro-zone effect observed in the literature, and described with mathematical models as bell-shaped T cell activation dose–response curves.40
When the activation profiles of individual B3Z were analysed in response to different concentrations of peptide, there were qualitative as well as quantitative differences. Most (93%) of the responders to APC pulsed with 100 μM peptide had a fast activation profile, with a single peak over the window of 240 s. At 1 μM, fewer (65%) of the responders had a single-peak profile, with 35% having a multiple-activation profile. This population was distinguished by multiple activation peaks with various amplitudes and rates (often 2–3 spikes during the recording time window, separated by approximately 100–150 s). When arrays were exposed to APC pulsed with 10 nM peptide, the percentage of single-peak profiles dropped further (47%), with the majority displaying multiple-activation profiles. This illustrates the increased depth of mechanistic detail that can be captured using single cell array measurements, and may indicate that at low levels of peptide antigen, as would be expected in a living organism, T cells are likely to achieve a critical activation threshold via the accumulation of multiple sub-threshold spikes rather than a single major spike. The pulsatile behaviour has been described previously in the literature,41,42 and very few systems12 can effectively show this heterogeneity in such a high-throughput yet simple way.
Conclusions
Biological heterogeneity is a fundamental property of cellular responses often masked by the use of a Gaussian distribution and mean parameters.43 Furthermore, it is becoming increasingly clear that individual cell behaviours are often more relevant for defining the biological property of the population than the average behaviour.44
With the goal of isolating and analysing single cells, several approaches have been developed in the past, including hydrodynamic traps,45–50 microwell arrays,18,19,51–53 droplet encapsulation,23,24,54–59 dielectrophoretic traps,60–62 digital microfluidics,63,64 adhesion onto functionalized patterned substrates47,65–69 and optical,70–74 magnetic64,75 or acoustic46,76–78 manipulation. Of these methods, only hydrodynamic trapping in a microfluidic chamber,11–16 sedimentation in micro-well arrays17–19 and co-encapsulation in droplets22–24 can be considered for high-throughput screening of cell–cell interactions.
While synchronized vertical cell–cell contact using microwells has been achieved by centrifugation,17,18 our platform is the first microwell device that allows synchronized cell contact and cell–cell interaction data to be captured from a well-defined time zero.
Our high-throughput cell array linked to a synchronised, physiologically relevant activation stimulus, and time-resolved response measurements, allowed us to distinguish three qualitatively different activation profiles among individual clonal T lymphocytes: fast, slow and pulsatile.
Similar patterns have been reported in the literature,42 also in response to other stimuli,79,80 but never at this scale of single cell analysis, with a throughput approximately 10-fold higher than that offered by existing platforms.12 Although the distinction between fast and slow responders could be prone to bias due to manual classification, the correlation between the abundance of stimulatory peptide
:
MHC present on APC and the fraction of responding T cells that show a “pulsatile” response profile suggests that in the presence of a low abundance of epitopes, cells are more likely to reach the activation threshold through multiple sub-threshold spikes.
Inter-cell variability within a clonal population could originate from stochastic differences in gene expression, growth phase of the cells or epigenetic alterations. To address this, an additional layer of information could be extracted from the current platform by integration with downstream analysis of individual T cells: for example with in situ lysis of lymphocytes and subsequent single cell western blotting.81 Alternatively, micromanipulators could be used to isolate the relevant T cells for expansion or further analysis using RNAseq, western blot and cytokine profiling.
Such high-density molecular information linked to time-resolved functional data achieved at single cell resolution will undoubtedly drive forward the understanding of physiologically relevant T cell responses to virus or tumours, and may prove vital for the development of therapeutics such as vaccines or immunomodulatory therapeutics.
Conflicts of interest
There are no conflicts to declare.
Acknowledgements
The authors wish to thank Dr Fabrizio Siracusa for technical help. This work was funded by CRUK Programme Grant A28279, and generous donations from Dr Norman Godinho.
References
- T. Q. Vu, R. M. B. de Castro and L. Qin, Lab Chip, 2017, 17, 1009–1023 RSC.
- B. Dura and J. Voldman, Curr. Opin. Immunol., 2015, 35, 23–29 CrossRef CAS.
- P. K. Chattopadhyay, T. M. Gierahn, M. Roederer and J. C. Love, Nat. Immunol., 2014, 15, 128–135 CrossRef CAS.
- M. Junkin and S. Tay, Lab Chip, 2014, 14, 1246 RSC.
- T. Konry, S. Sarkar, P. Sabhachandani and N. Cohen, Annu. Rev. Biomed. Eng., 2016, 18, 259–284 CrossRef CAS.
- T. W. Murphy, Q. Zhang, L. B. Naler, S. Ma and C. Lu, Analyst, 2018, 143, 60–80 RSC.
- X. An and N. Varadarajan, Curr. Opin. Chem. Eng., 2018, 19, 142–152 CrossRef.
- M. Rothbauer, H. Zirath and P. Ertl, Lab Chip, 2018, 18, 249–270 RSC.
- L. Valihrach, P. Androvic and M. Kubista, Int. J. Mol. Sci., 2018, 19, 807 CrossRef.
- Y. F. S. Seah, H. Hu and C. A. Merten, Mol. Aspects Med., 2018, 59, 47–61 CrossRef CAS.
- P. J. Lee, P. J. Hung, R. Shaw, L. Jan and L. P. Lee, Appl. Phys. Lett., 2005, 86, 223902 CrossRef.
- B. Dura, M. M. Servos, R. M. Barry, H. L. Ploegh, S. K. Dougan and J. Voldman, Proc. Natl. Acad. Sci. U. S. A., 2016, 113, E3599–E3608 CrossRef CAS.
- J.-P. Frimat, M. Becker, Y.-Y. Chiang, U. Marggraf, D. Janasek, J. G. Hengstler, J. Franzke and J. West, Lab Chip, 2011, 11, 231–237 RSC.
- S. Cui, Y. Liu, W. Wang, Y. Sun and Y. Fan, Biomicrofluidics, 2011, 5, 032003 CrossRef.
- C.-K. He, Y.-W. Chen, S.-H. Wang and C.-H. Hsu, Lab Chip, 2019, 19, 1370–1377 RSC.
- J. H. Jang, Y. Huang, P. Zheng, M. C. Jo, G. Bertolet, M. X. Zhu, L. Qin and D. Liu, J. Immunol., 2015, 195, 1320–1330 CrossRef CAS.
- L. Huang, Y. Chen, W. Huang and H. Wu, Lab Chip, 2018, 18, 1113–1120 RSC.
- L. Huang, Y. Chen, Y. Chen and H. Wu, Anal. Chem., 2015, 87, 12169–12176 CrossRef CAS.
- N. Zurgil, E. Afrimzon, A. Deutsch, Y. Namer, Y. Shafran, M. Sobolev, Y. Tauber, O. Ravid-Hermesh and M. Deutsch, Biomaterials, 2010, 31, 5022–5029 CrossRef CAS.
- Y. Zhou, N. Shao, R. Bessa de Castro, P. Zhang, Y. Ma, X. Liu, F. Huang, R.-F. Wang and L. Qin, Cell Rep., 2020, 31, 107574 CrossRef CAS.
- I. Wohl, N. Zurgil, Y. Hakuk, M. Sobolev and M. Deutsch, Eur. Biophys. J., 2019, 48, 267–275 CrossRef CAS.
- R. M. Schoeman, E. W. M. Kemna, F. Wolbers and A. van den Berg, Electrophoresis, 2014, 35, 385–392 CrossRef CAS.
- M. T. Chung, D. Núñez, D. Cai and K. Kurabayashi, Lab Chip, 2017, 17, 3664–3671 RSC.
- A. I. Segaliny, G. Li, L. Kong, C. Ren, X. Chen, J. K. Wang, D. Baltimore, G. Wu and W. Zhao, Lab Chip, 2018, 18, 3733–3749 RSC.
- B. Dura, S. K. Dougan, M. Barisa, M. M. Hoehl, C. T. Lo, H. L. Ploegh and J. Voldman, Nat. Commun., 2015, 6, 5940 CrossRef CAS.
- B. Dura, Y. Liu and J. Voldman, Lab Chip, 2014, 14, 2783 RSC.
- A. Brodovitch, P. Bongrand and A. Pierres, J. Immunol., 2013, 191, 2064–2071 CrossRef CAS.
- H. Park, H. Kim and J. Doh, Bioconjugate Chem., 2018, 29, 672–679 CrossRef CAS.
- M. Trebak and J.-P. Kinet, Nat. Rev. Immunol., 2019, 19, 154–169 CrossRef CAS.
- P. A. Negulescu, N. Shastri and M. D. Cahalan, Proc. Natl. Acad. Sci. U. S. A., 1994, 91, 2873–2877 CrossRef CAS.
- J. Karttunen, S. Sanderson and N. Shastri, Proc. Natl. Acad. Sci. U. S. A., 1992, 89, 6020–6024 CrossRef CAS.
- J. S. Blum, P. A. Wearsch and P. Cresswell, Annu. Rev. Immunol., 2013, 31, 443–473 CrossRef CAS.
- Y. Li and R. J. Kurlander, J. Transl. Med., 2010, 8, 104 CrossRef.
- B. S. Valitutti, M. Dessing, K. Aktories, H. Gallati and A. Lanzavecchia, J. Exp. Med., 1995, 181, 577–584 CrossRef.
- E. Donnadieu, G. Bismuth and A. Trautmann, J. Biol. Chem., 1992, 267, 25864–25872 CAS.
- Z. Ma and T. H. Finkel, Trends Immunol., 2010, 31, 1–6 CrossRef CAS.
- Z. Ma, D. E. Discher and T. H. Finkel, Front. Immunol., 2012, 3, 2–4 Search PubMed.
- P.-H. Puech, D. Nevoltris, P. Robert, L. Limozin, C. Boyer and P. Bongrand, PLoS One, 2011, 6, e22344 CrossRef CAS.
- R. Basu, B. M. Whitlock, J. Husson, A. Le Floc'h, W. Jin, A. Oyler-Yaniv, F. Dotiwala, G. Giannone, C. Hivroz, N. Biais, J. Lieberman, L. C. Kam and M. Huse, Cell, 2016, 165, 100–110 CrossRef CAS.
- M. Lever, H. Lim, P. Kruger, J. Nguyen, N. Trendel, E. Abu-Shah, P. K. Maini, P. A. van der Merwe and O. Dushek, Proc. Natl. Acad. Sci. U. S. A., 2016, 113, E6630–E6638 CrossRef CAS.
- C. Wülfing, J. D. Rabinowitz, C. Beeson, M. D. Sjaastad, H. M. McConnell and M. M. Davis, J. Exp. Med., 1997, 185, 1815–1825 CrossRef.
- M. Le Borgne, S. Raju, B. H. Zinselmeyer, V. T. Le, J. Li, Y. Wang, M. J. Miller and A. S. Shaw, J. Immunol., 2016, 196, 1471–1479 CrossRef CAS.
- A. Gough, A. M. Stern, J. Maier, T. Lezon, T. Shun, C. Chennubhotla, M. E. Schurdak, S. A. Haney and D. L. Taylor, SLAS Discovery, 2017, 22, 213–237 CAS.
- S. J. Altschuler and L. F. Wu, Cell, 2010, 141, 559–563 CrossRef CAS.
- D. Jin, B. Deng, J. X. Li, W. Cai, L. Tu, J. Chen, Q. Wu and W. H. Wang, Biomicrofluidics, 2015, 9, 014101 CrossRef CAS.
- K. A. Ohiri, S. T. Kelly, J. D. Motschman, K. H. Lin, K. C. Wood and B. B. Yellen, Lab Chip, 2018, 18, 2124–2133 RSC.
- L. Lin, Y. Chu, J. P. Thiery, C. T. Lim and I. Rodriguez, Lab Chip, 2013, 13, 714 RSC.
- W. Espulgar, Y. Yamaguchi, W. Aoki, D. Mita, M. Saito, J. Lee and E. Tamiya, Sens. Actuators, B, 2015, 207, 43–50 CrossRef CAS.
- J. Chung, Y.-J. Kim and E. Yoon, Appl. Phys. Lett., 2011, 98, 123701 CrossRef.
- D. Di Carlo, L. Y. Wu and L. P. Lee, Lab Chip, 2006, 6, 1445 RSC.
- J. F. Swennenhuis, A. G. J. Tibbe, M. Stevens, M. R. Katika, J. van Dalum, H. Duy Tong, C. J. M. van Rijn and L. W. M. M. Terstappen, Lab Chip, 2015, 15, 3039–3046 RSC.
- Y. Wang, P. Shah, C. Phillips, C. E. Sims and N. L. Allbritton, Anal. Bioanal. Chem., 2012, 402, 1065–1072 CrossRef CAS.
- J. R. Rettig and A. Folch, Anal. Chem., 2005, 77, 5628–5634 CrossRef CAS.
- A. Zinchenko, S. R. A. Devenish, B. Kintses, P. Colin, M. Fischlechner and F. Hollfelder, Anal. Chem., 2014, 86, 2526–2533 CrossRef CAS.
- J. F. Edd, D. Di Carlo, K. J. Humphry, S. Köster, D. Irimia, D. A. Weitz and M. Toner, Lab Chip, 2008, 8, 1262 RSC.
- S. Sarkar, P. Sabhachandani, D. Stroopinsky, K. Palmer, N. Cohen, J. Rosenblatt, D. Avigan and T. Konry, Biomicrofluidics, 2016, 10, 054115 CrossRef CAS.
- V. Chokkalingam, J. Tel, F. Wimmers, X. Liu, S. Semenov, J. Thiele, C. G. Figdor and W. T. S. Huck, Lab Chip, 2013, 13, 4740 RSC.
- S. Sarkar, V. Motwani, P. Sabhachandani, N. Cohen and T. Konry, J. Clin. Cell. Immunol., 2015, 334 DOI:10.4172/2155-9899.1000334.
- H. Hu, D. Eustace and C. A. Merten, Lab Chip, 2015, 15, 3989–3993 RSC.
- M. Şen, K. Ino, J. Ramón-Azcón, H. Shiku and T. Matsue, Lab Chip, 2013, 13, 3650 RSC.
- C. Wu, R. Chen, Y. Liu, Z. Yu, Y. Jiang and X. Cheng, Lab Chip, 2017, 17, 4008–4014 RSC.
- Y. Yoshimura, M. Tomita, F. Mizutani and T. Yasukawa, Anal. Chem., 2014, 86, 6818–6822 CrossRef CAS.
- A. Rival, D. Jary, C. Delattre, Y. Fouillet, G. Castellan, A. Bellemin-Comte and X. Gidrol, Lab Chip, 2014, 14, 3739–3749 RSC.
- D. Witters, K. Knez, F. Ceyssens, R. Puers and J. Lammertyn, Lab Chip, 2013, 13, 2047 RSC.
- C. Tu, B. Huang, J. Zhou, Y. Liang, J. Tian, L. Ji, X. Liang and X. Ye, Micromachines, 2016, 8, 1 CrossRef.
-
W. Yang, H. Yu, Y. Wang and L. Liu, in 2017 International Conference on Manipulation, Automation and Robotics at Small Scales (MARSS), IEEE, 2017, pp. 1–5 Search PubMed.
- S. A. Zawko and C. E. Schmidt, Lab Chip, 2010, 10, 379–383 RSC.
- M. Thery and M. Piel, Cold Spring Harb. Protoc, 2009, pdb.prot5255 Search PubMed.
- M. R. Dusseiller, D. Schlaepfer, M. Koch, R. Kroschewski and M. Textor, Biomaterials, 2005, 26, 5917–5925 CrossRef CAS.
- P.-F. Yang, C.-H. Wang and G.-B. Lee, Sci. Rep., 2016, 6, 22036 CrossRef CAS.
- M. Werner, F. Merenda, J. Piguet, R.-P. Salathé and H. Vogel, Lab Chip, 2011, 11, 2432 RSC.
- H. Xin, Y. Zhang, H. Lei, Y. Li, H. Zhang and B. Li, Sci. Rep., 2013, 3, 1993 CrossRef.
- Y. Yang, Y. Mao, K. Shin, C. O. Chui and P. Chiou, Sci. Rep., 2016, 6, 22630 CrossRef CAS.
- R. Burger, D. Kurzbuch, R. Gorkin, G. Kijanka, M. Glynn, C. McDonagh and J. Ducrée, Lab Chip, 2015, 15, 378–381 RSC.
- C. W. Shields, C. E. Livingston, B. B. Yellen, G. P. López and D. M. Murdoch, Biomicrofluidics, 2014, 8, 041101 CrossRef.
- M. Antfolk, S. H. Kim, S. Koizumi, T. Fujii and T. Laurell, Sci. Rep., 2017, 7, 46507 CrossRef CAS.
- F. Guo, Z. Mao, Y. Chen, Z. Xie, J. P. Lata, P. Li, L. Ren, J. Liu, J. Yang, M. Dao, S. Suresh and T. J. Huang, Proc. Natl. Acad. Sci. U. S. A., 2016, 113, 1522–1527 CrossRef CAS.
- B. Vanherberghen, O. Manneberg, A. Christakou, T. Frisk, M. Ohlin, H. M. Hertz, B. Önfelt and M. Wiklund, Lab Chip, 2010, 10, 2727 RSC.
- A. S. Kniss-James, C. A. Rivet, L. Chingozha, H. Lu and M. L. Kemp, Integr. Biol., 2017, 9, 238–247 CrossRef CAS.
- L. He, A. Kniss, A. San-Miguel, T. Rouse, M. L. Kemp and H. Lu, Lab Chip, 2015, 15, 1497–1507 RSC.
- C.-C. Kang, K. A. Yamauchi, J. Vlassakis, E. Sinkala, T. A. Duncombe and A. E. Herr, Nat. Protoc., 2016, 11, 1508–1530 CrossRef.
Footnote |
† Electronic supplementary information (ESI) available. See DOI: 10.1039/d0lc00628a |
|
This journal is © The Royal Society of Chemistry 2020 |