DOI:
10.1039/D0LC00252F
(Paper)
Lab Chip, 2020,
20, 4420-4432
Organotypic primary blood vessel models of clear cell renal cell carcinoma for single-patient clinical trials†
Received
12th March 2020
, Accepted 7th October 2020
First published on 26th October 2020
Abstract
Clear cell renal cell carcinoma (ccRCC) is a common genitourinary cancer associated with the development of abnormal tumor angiogenesis. Although multiple anti-angiogenic therapies have been developed, responses to individual treatment are highly variable between patients. Thus, the use of one-patient clinical trials has been suggested as an alternative to standard trials. We used a microfluidic device to generate organotypic primary patient-specific blood vessel models using normal (NEnC) and tumor-associated primary CD31+ selected cells (TEnC). Our model was able to recapitulate differences in angiogenic sprouting and vessel permeability that characterize normal and tumor-associated vessels. We analyzed the expression profile of vessel models to define vascular normalization in a patient-specific manner. Using this data, we identified actionable targets to normalize TEnC vessel function to a more NEnC-like phenotype. Finally, we tested two of these drugs in our patient-specific models to determine the efficiency in restoring vessel function showing the potential of the model for single-patient clinical trials.
Introduction
Clear cell renal cell carcinoma (ccRCC) is a common genitourinary cancer, with over 45
000 new cases each year in the US alone.1 Many ccRCC patients have abnormalities in the von Hippel–Lindau tumor suppressor (VHL) gene. However, not all patients present these mutations, which leads to a very heterogeneous population.2 Unsurprisingly, the response of ccRCC patients to first-line treatments is highly variable. More specifically, some patients show a sustained response, while other patients' conditions may worsen with therapy.3 Given the lack of consistent, effective therapies for metastatic ccRCC, current treatment paradigms largely rely on a trial and error approach to the initial selection of systemic treatments. However, a more individualized precision approach could be beneficial for these patients.4 Therapeutic approaches typically attempt to target the dense vascular networks characteristic of ccRCC,5 ideally achieving vessel normalization6 (i.e., “fixing” the abnormal features on tumor-associated vasculature). A return to normal vessel structure may restrict tumor-associated angiogenesis and, in turn, should reduce tumor growth and size.7
Currently, there are no effective methods to predict which ccRCC tumors will respond to different therapies. New methods could be instrumental in guiding clinical decision making and leading to the best possible disease outcome for each patient.4,8–10 Currently, stratification remains limited and is primarily done according to genetic profiling of mutations in patients,11 which frequently does not correlate with patient response.12,13 The application of a more integrative approach using functional readouts (e.g., cell viability, angiogenic sprouting, cell migration) for drug response has been presented as an attractive solution to this problem.14–17 Recently, microfluidic models have successfully reproduced angiogenesis and been used to test therapeutic drugs.18–20 However, personalized in vitro models that can accurately recapitulate vessel function (e.g., sprouting angiogenesis) is an unmet need in the field. Patient-specific models to test tailored subsets of treatments and guide treatment are scarce in the literature. The few existing examples focus on primary immune cells,21,22 or patient-specific spheroids with tumor cells.23 Notably, for ccRCC, Miller et al. reported a co-culture of primary patient-specific ccRCC epithelial cells with HUVEC vessels.19 However, few models have attempted to leverage primary patient-derived cells. We recently described the use of personalized normal and tumor-associated patient-derived endothelial vessel models (defining endothelial as CD31+ Epcam−) from ccRCC patients as an alternative to traditional angiogenesis assays.24 Additionally, we reported both models' capacity to mimic hallmarks differentiating normal from tumor-associated blood vessels. Finally, our model was able to reproduce a response or resistance to anti-angiogenics commonly used in clinical settings. However, a model that allows assessing restoration of normal vessel function in a patient-specific manner, which can help provide guidelines for patient stratification, remains a challenge. Hence, here we leverage a patient-specific model developed by us to identify successful precision medicine therapeutic alternatives.24
Building on our previously published work, we isolated both normal and tumor-associated endothelial cells (NEnC and TEnC, respectively) and established microfluidic tubular vessel models for three different patients. We further demonstrated the capacity of our model to mimic phenotypic differences between TEnC and NEnC vessels, including higher permeability coefficients in TEnC vessels than their normal counterparts. To target aberrant vasculature specifically, we have used a transcriptomics approach to identify differences between NEnC and TEnC vessels. TEnC vessels showed a more pro-angiogenic phenotype than their normal counterparts, with patient-specific responses and profiles unique to each patient. Using online databases, we have identified alternative drugs outside the current standard of care with the potential to target TEnC vasculature. We tested two of the identified drugs (i.e., nintedanib and sirolimus) on one of our patient-specific models to validate the results produced by gene profiling. Nintedanib and sirolimus are both currently undergoing clinical testing. Nintedanib is a small molecule multi-tyrosine kinase inhibitor that targets VEGFR1/2, FGFR1/2/3, and PDGFRα/β, while sirolimus is classified as a macrolide lactam and is a highly specific mTOR inhibitor. While these drugs are not a first-choice treatment for ccRCC, their mechanism of action targets the angiogenic process characteristic of this type of cancer. We observed that nintedanib treatment decreased sprout number and length and promoted recovery in vessel permeability.
In contrast, sirolimus was less effective in decreasing sprout numbers and length. Only at high doses was sirolimus capable of restoring vessel permeability, despite higher cell toxicity. Overall, our results demonstrate that our microfluidic vessel models can be used to functionally test and validate a tailored subset of drugs in a single-patient clinical trial approach. The insight and results of functional testing acquired through this methodology can eventually lead to patient stratification and a more effective standard of care for ccRCC.
Results and discussion
Establishment of the microfluidic vessel model for different patients
Tumor-associated vessels are different from their normal counterparts. Some of these differences rely on organization, angiogenic sprouting, and vessel permeability. In this paper, we used a patient-specific microfluidic organotypic model to identify specific gene expression abnormalities in tumor-associated endothelial cell (TEnC) vessels as compared to their normal counterparts (NEnC). We chose to characterize significant differences in gene expression between NEnC and TEnC vessels to identify actionable targets linked to specific treatments and, in turn, verify their efficacy in patient vessel models using functional readouts.
To this end, ccRCC tumor tissue and normal adjacent kidney tissue samples were collected from complete nephrectomies of three patients (histologic subtype and clinical information in Table S1†). Samples were mechanically dissociated and enzymatically digested. Endothelial cells were subsequently isolated using anti-CD31-conjugated magnetic microbead separation columns. CD31+ cells captured in the column were recovered and cultured in vitro until confluent. To verify the endothelial lineage of the isolated cells, RT-qPCR was performed in the cultured cells for CD31, CDH5 (i.e., VE-cadherin), and Epcam, and normalized to a control epithelial cell line. All cells demonstrated a significant increase in CD31 and CDH5 expression compared to a standard epithelial cell line, which is consistent with the desired endothelial cell lineage (Fig. S1†). Conversely, the expression of Epcam was significantly downregulated in TEnC than NEnC. Likewise, CD31, VE-cadherin, VEGFR2 and Epcam immunofluorescence and visual inspection of morphology were performed to assess cell lineage and disprove epithelial/fibroblast contamination of CD31+ populations (representative images in Fig. S2†). Both TEnC and NEnC were positive for VEGFR2, a canonical marker of endothelial cells. Endothelial cell stainings CD31 and VE-cadherin were homogeneous in the NEnC and TEnC samples, and higher expression was observed for NEnC than TEnC. However, both cells showed a less prevalent staining than HUVEC.25,26 These results could be explained by the high expression of these markers in HUVEC, whereas kidney endothelial cells typically show lower expression of cell-to-cell junctions.27,28 Further, TEnC cells showed a lower expression than NEnC, which is consistent with their dysregulated phenotype and is one of the initial steps of the angiogenic cascade.29 All in all, our results support the endothelial lineage of our cells. However, further characterization could be pursued in the future to elucidate the mechanisms underlying TEnC dysregulation and angiogenesis in RCC.
Next, the vessel models with NEnC and TEnC cells of three different patients (referred to as patient A, B, and C), were established in the LumeNEXT platform (Fig. 1A for a schematic of the procedure, Fig. 1B for a representative image of the device). Representative images of the lumens for each patient are shown in Fig. 1C–F and S4.† Qualitatively, we can see a higher prevalence of angiogenic sprouts in TEnC vessels, as well as heterogeneity between the angiogenic sprouting between TEnC models from different patients.
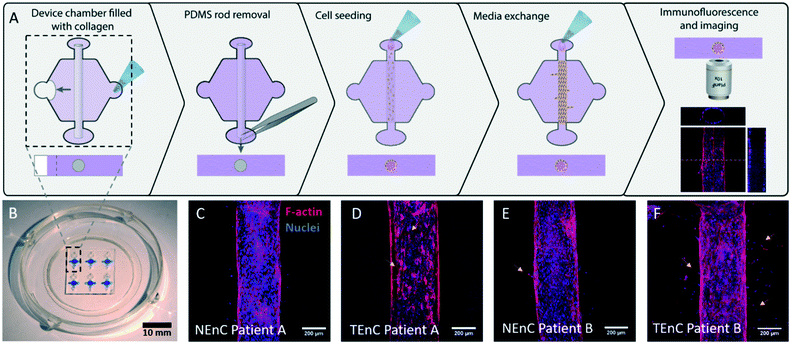 |
| Fig. 1 Schematic of methodology and images of the model. A) PDMS fabricated “LumeNEXT” microdevices were used for this work. The microchamber was filled with a collagen hydrogel polymerized around a PDMS rod, which is later removed to produce a tubular-shaped void in the hydrogel. Primary endothelial cells (both normal and tumor-associated, i.e., NEnC and TEnC, respectively) are used to line this void and produce a luminal structure. B) Photography of the microdevice. C–F) Confocal images showing the primary vessels in the model. C) NEnC from patient A shows higher confluency than D) TEnC from the same patient. Yellow arrows indicate endothelial disruptions. E) NEnC from patient B shows fewer sprouts (yellow arrows) than F) TEnC from the same patient. | |
Permeability differences between NEnC and TEnC vessels
Tumor-associated endothelial vessels are known to be phenotypically different from their normal counterparts.30,31 For example, low integrity and high diffusivity of tumor-associated vessels result in significant changes in their function, comparatively.6,30 To validate our model we measured permeability by perfusing our NEnC and TEnC vessels with 40 kDa fluorescently-conjugated dextran and tracking its diffusion out of the vessel over 15 min (Fig. 2A). We observed that, while the dextran was contained to the vessel region at time 0, the NEnC vessel retained a high quantity of dextran in the vessels after 15 min (Fig. 2B). Conversely, diffusion out of the vessel was higher in TEnC vessels (Fig. 2C). Specifically, in representative NEnC and TEnC diffusions, only 13% of the dextran fluorescence was observed 1000 μm from the center of the NEnC vessel (Fig. 2D), whereas it was 50% in the TEnC vessel (Fig. 2E). These results demonstrated our model's ability to recapitulate the characteristic leakiness of tumor-associated vessels as opposed to a higher barrier function of normal vessels. For a more thorough quantification of the differences in barrier function, we calculated the permeability coefficient for both types of vessels, as well as an empty vessel (cell-free collagen hydrogel tubular structure) as a control. This analysis revealed that NEnC vessels have the lowest permeability (6.0 ± 3.2 × 10−7 cm s−1). TEnC vessels show a 2.7-fold increase in permeability as compared to NEnC vessels (2.2 ± 1.8 × 10−6 cm s−1, **p = 0.004). Likewise, empty vessels show a 3.22-fold increase in permeability compared to TEnC vessels (6.9 ± 4.2 × 10−6 cm s−1, *p = 0.04) (Fig. 2F).
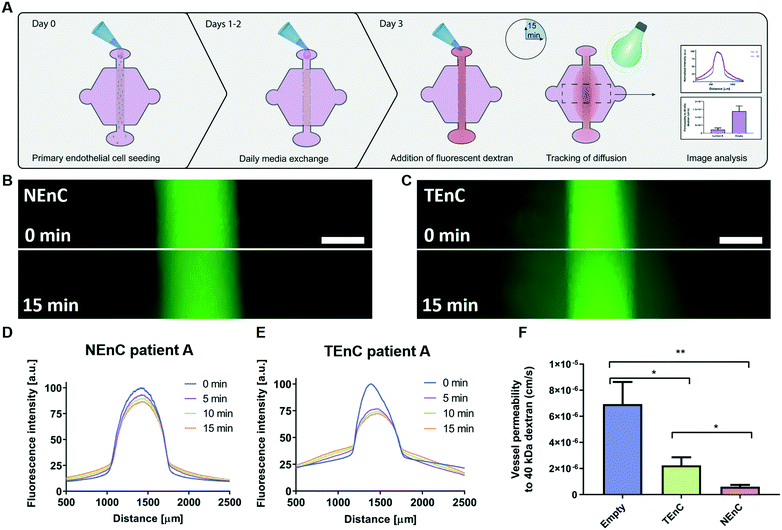 |
| Fig. 2 NEnC and TEnC vessel permeability. A) Schematic indicating the seeding procedure and dextran diffusion assays for permeability calculation. B) Representative images of 40 kDa dextran diffusion in NEnC and C) TEnC vessels over 15 min. D) Representative normalized intensity profiles of 40 kDa dextran diffusion, for NEnC and E) TEnC. F) Permeability values calculated for NEnC and TEnC compared to an empty vessel structure (as calculated via multiple t-tests). Data was pooled from all three patients. Scalebar = 200 μm. n = 6 vessels from at least 3 independent experiments. Bars represent average ± S.E.M. *p < 0.05; **p < 0.01. | |
Unsupervised clustergrams of qPCR profiles for NEnC and TEnC vessels
To better characterize the differences between the TEnC and NEnC vessel models and investigate patient-specific signatures of vascular normalization, we analyzed gene expression by RT-qPCR using a commercial panel. The analysis included 84 genes related to angiogenesis, and TEnC gene expression was normalized to NEnC.32 Therefore, we obtained a profile of gene dysregulation characterizing abnormal vasculature for the three patients. We identified significantly changed genes and presented them in a clustergram (Fig. 3). The three patients analyzed exhibited a different number of genes dysregulated (i.e., patients A and B presented smaller clustergrams than patient C). We observed that dysregulated genes were mostly constant throughout all TEnC vessels and NEnC vessels of the same patients.
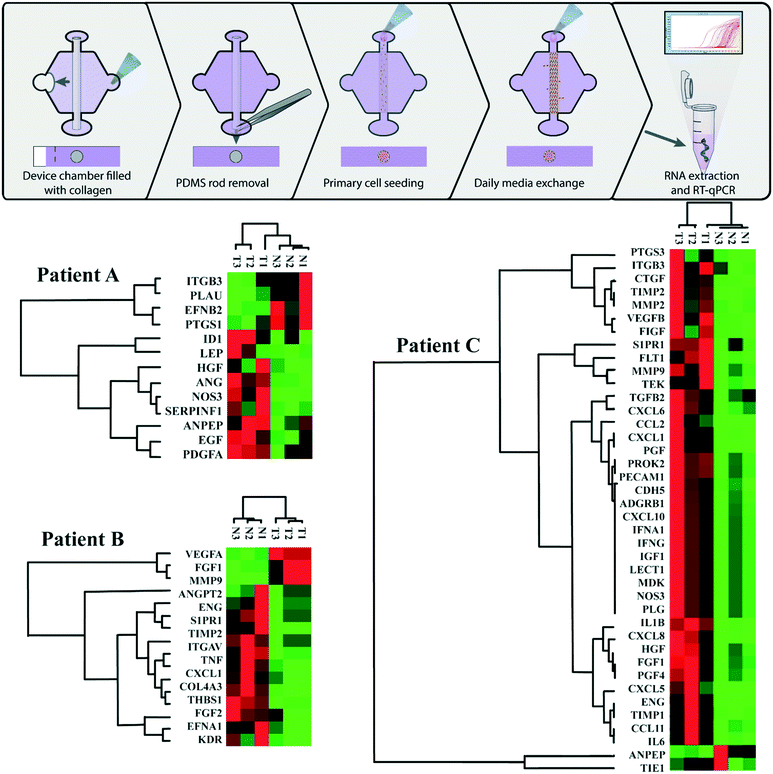 |
| Fig. 3 Unsupervised clustergram analysis of qPCR from TEnC vessels normalized to correlative NEnC vessels. (Top) Schematic of primary cell seeding and RT-qPCR experiment. (Bottom) Clustergrams were built using only those genes showing significant changes. N = 3 independent experiments per patient with 2 pooled vessels each. Sizes of clustergrams are different for the different patients. | |
We next looked at ontological distances in the clustergrams, a measurement of similarity in gene expression among different samples. Qualitatively, the distance among samples is variable. Specifically, patient B tumor (T) samples are grouped together, and similarly for the normal (N). However, this is not the case for all patients. Notably, in patients A and C, one of the TEnC samples is bracketed closer to NEnC samples. This observation is consistent with the high heterogeneity present in primary ccRCC tumors.
Among the significantly dysregulated genes, (i.e., in TEnC as compared to NEnC), we find canonical angiogenic intermediates and common drug targets (e.g., HGF in patient A, VEGFA in patient B, and VEGFC in patient C) (Fig. 4 for a more detailed view of the dysregulated genes).
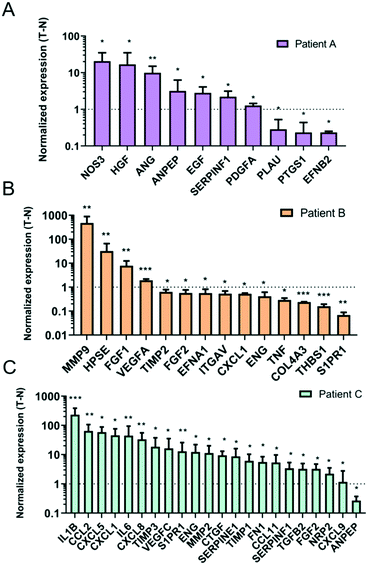 |
| Fig. 4 Significant changes in gene expression for each patient A–C) genes showing significant changes were plotted for patients A, B, and C to reflect the direction of the change. *p < 0.05; **p < 0.01; ***p < 0.001. | |
We also observed an upregulation of FGF1 and a less significant downregulation of FGF2 in TEnC samples. Both these genes are reportedly pro-angiogenic, although the net balance of their regulation results in a pro-angiogenic phenotype.33,34 Further, we observe that the significantly dysregulated genes include a small number of pro-angiogenic genes activated in patient A (i.e., VEGFA, FGF1, MMP9), and a longer list of downregulated angiostatic (i.e., anti-angiogenic) genes (e.g., ANGPT2, S1PR1, THBS1, ENG). Finally, the gene expression profile for patient C includes many upregulated cytokines and matrix-related proteins (e.g., MMP2, CXCL10, CCL2, IL6), which are reportedly pro-angiogenic, albeit unique in our study sample.35,36
Determination of angiogenic/angiostatic balance and functions of vessels
To determine the direction of the angiogenic/angiostatic balance for each of the studied patients, we cataloged our significantly dysregulated genes between pro-angiogenic and angiostatic (Fig. S5A†). Overall, two patients (i.e., A and C) had more upregulated than downregulated genes, whereas patient B showed a higher number of downregulated genes altogether. To characterize the angiogenic/angiostatic balance, we divided dysregulated genes between pro-angiogenic and angiostatic (Fig. S5B†). In pie graphs, we represented the percentage of genes contributing to angiogenesis in red shades (light for downregulated angiostatic genes and dark for upregulated pro-angiogenic genes). The same was done for those genes promoting angiostasis. Overall, pie sections depicted in red shades (pro-angiogenic) are more prominent for all three patients, indicating that TEnC vessels show a consistently more pro-angiogenic profile than NEnC vessels.
Following qualitative analysis of the gene expression data, we performed quantitative analysis, followed by an interpretation of potential gene functions activated in TEnC as compared to NEnC vessels. To this end, first, we plotted the fold change of the significantly dysregulated genes for each patient (Fig. 4A–C). Consistent with previous results, we observed that the composition of the gene expression profile was different for patients A, B, and C. Further, we observed that the upregulation changes were also very different for these patients. The highest upregulated gene for each patient was NOS3 (20 ± 8.3-fold change) for patient A, IL1B (231.715 ± 89.278-fold) for patient C, MMP9 (4.7 ± 2.4 ×102-fold) for patient C.
Next, we performed a gene enrichment analysis (Fig. S6A–C†); a bioinformatic analysis that calculates gene ontology (GO) terms (i.e., a definition for a group of specific cell functions). In other words, this analysis infers dysregulated cell functions from a given list of dysregulated genes.37 Since the downregulated genes would confound the search, we decided to run separate gene enrichment analyses for upregulated and downregulated genes. While this process was attempted with genes that were significantly downregulated, the number of genes was insufficient to provide results via GSEA analysis in 2 out of 3 patients. Therefore, we only present the upregulated genes in the analysis. We presented our data as –log10(p-value). In this graph, the higher the values, the more likely that the pathway referred to in the GO_term is dysregulated. This search revealed terms related to a pro-angiogenic phenotype, such as “GO_angiogenesis” or “GO_blood vessel morphogenesis” in all three patients. These results are consistent with the in vivo observation that angiogenesis is upregulated in these tumors as compared with normal tissue.
Interestingly, patient B showed a higher −log10(p-value) of GO_terms related to chemotaxis, or cell communication, “GO_positive regulation of locomotion” than to GO_terms related to angiogenesis. On the other hand, patient C had GO_terms more related to chemotaxis, such as “GO_positive regulation of locomotion” or “GO_regulation of chemotaxis”. These results are consistent with the abundant number of chemokines present in the dysregulated gene list of patient C (e.g., CCL2, CXCL5, IL6, CXCL8).
Identification of patient-specific actionable molecular targets
Next, we performed a pathway network analysis based on ccRCC databases displayed in cBioportal to identify dysregulated pathways and druggable nodes in these pathways. We did this analysis for all three patients (Fig. 5), retrieving different pathways in each case, as well as a few therapeutic options. Apart from the dysregulated molecules (e.g., EGF), we also included some drugs targeting molecule receptors, such as nintedanib, which targets EGFR and PDGFR. Interestingly, we found several options outside from current first-line options, such as mTOR inhibitors (i.e., sirolimus) or IL1B inhibitors (i.e., canakinumab). Many of these are FDA-approved for other pathologies and may arise as new therapeutic options for the benefit of individual patients. Further, we decided to assess the validity of our approach by two of the proposed therapeutic alternatives (i.e., nintedanib and sirolimus) using functional tests to determine the efficacy of the drugs in one of our patients.
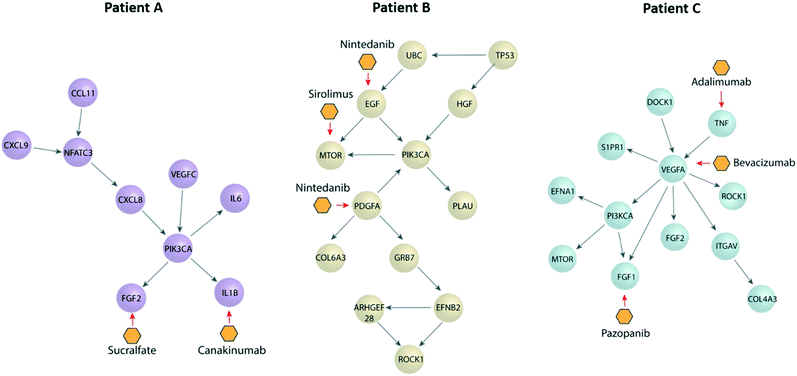 |
| Fig. 5 Pathway networks and potential treatments for each patient as suggested by cBioportal. Schematic pathway networks showing the most important transcription factors dysregulated in each patient, as well as their connections depicted as spheres. Potential treatments are depicted as the yellow hexagons, by their primary target. | |
Functional validation of patient-specific drugs in TEnC models
Anti-angiogenic drugs (e.g. nintedanib and sirolimus) specifically target different steps of the angiogenic pathway, resulting in a reduction of angiogenic sprout number and length, as well as in vessel permeability. Therefore, a model that allows for the evaluation of the effectiveness of drugs in several of the steps pertaining to the angiogenic cascade could help inform clinical decisions. Hence, we used one of our patient-specific models, along with the described readouts to validate our drug selection approach. We tested nintedanib and sirolimus on one of our patient-specific models (patient B, Fig. 6). While these two drugs are not currently used first-line treatments for ccRCC, our approach suggested they could be effective in patient B models. Nintedanib and sirolimus were hits for patient B nintedanib is a multi-tyrosine kinase inhibitor used in the last decade as a second-line treatment for idiopathic pulmonary fibrosis. Among other receptors, nintedanib targets VEGFR1–3, PDGFRα/β, and FGFR1–2.38 Sirolimus is an mTOR inhibitor, which has been used to treat ccRCC but are not recommended because of the better efficacy of VEGFR inhibitors in an average population of patients. Neither of these drugs is a current first-line RCC systemic treatment, but could potentially be effective in downregulating altered angiogenic pathways, specifically for patient B.
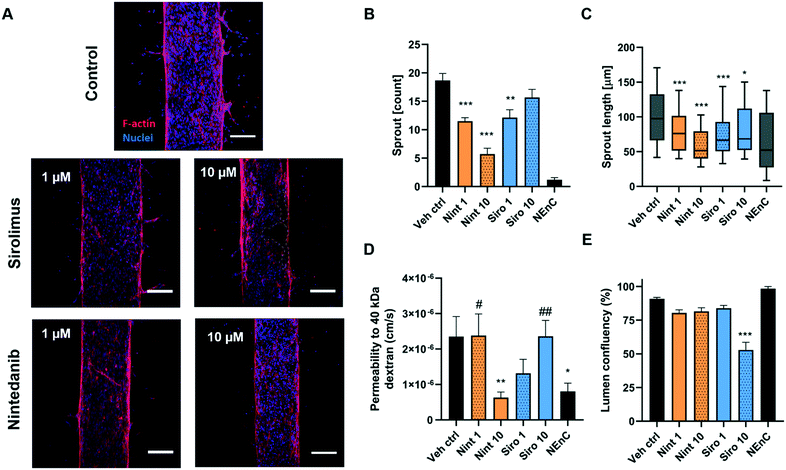 |
| Fig. 6 Test of drugs identified via cBioportal on the TEnC on patient B TEnC vessel models. A) Representative images of control and drug-treated TEnC vessel models. 1 and 10 μM of sirolimus (Siro) and nintedanib (Nint) were used and compared to vehicle control conditions. An example of endothelial disruption is shown in sirolimus 10 μm in dashed lines. B–E) Functional characterization of drug response to sirolimus and nintedanib using the following readouts: B) sprout count, C) sprout length, D) permeability values to 40 kDa fluorescently conjugated dextran. E) Vessel confluency quantified as the area occupied by phalloidin staining per vessel. N = at least 6 vessels from at least 2 independent experiments. Scalebar = 200 μm. Bars represent average ± S.E.M. * = significance as compared to vehicle control. *p < 0.05; **p < 0.01; ***p < 0.001. # = significance as compared to NEnC (in the permeability graph only). #p < 0.05; ##p < 0.01. | |
For this test, we seeded patient B vessels and added drug treatments at two concentrations (1 and 10 μM, labeled as “Nint 1”, “Nint 10”, “Siro 1” and “Siro 10”). After 3 days of incubation with the drug, we tested vessel permeability, as well as quantified vessel sprouting and confluency (Fig. 6A). We previously demonstrated that TEnC primary vessels show significantly lower vessel confluency than their normal counterparts.24 Therefore, this suggests that confluency may be used as a measurement of the restoration of normal vessel function. Likewise, lower confluency may be indicative of high toxicity, as confirmed via ATP luminescence-based assays (Fig. S7†). In this 2D assay, the ATP baseline is lower for all concentrations of sirolimus than for nintedanib. Interestingly, while the decrease in confluency in the models at 10 μM sirolimus points toward toxicity, this effect is not observed in the toxicity assay for sirolimus. Conversely, the toxicity observed in 2D for nintedanib does not show in vessel models.
There are many ways to present the data gathered from angiogenesis assays. Typical readouts include sprout length (presented as absolute distance, normalized distance or as the number of cells per sprout), sprout diameter (commonly used in long term experiments), or area occupied by sprouts (either presented as total area or as a percentage of the studied ROI).39,40 The choice of readout(s) will depend on the specific mechanism studied and the model utilized. Regarding sprout length, the use of raw numbers requires less processing and is more comparable among different conditions than normalized values. Conversely, the use of normalized values is typically used when ROIs differ in size.41 On the other hand, displaying sprout length may not be a reliable readout to indicate invasion, since cell size differs within a population. We were particularly interested in studying invasion distance and the number of sprouting events, as these readouts are some of the first steps in the angiogenic cascade. Since the size of our ROIs was consistent, we chose to use raw numbers to quantify sprouting in our models.
Representative images of immunofluorescence for treated and control vessels show a decrease in sprouting in the drug-treated conditions, especially at higher drug concentrations (Fig. 6A and B). While all conditions showed a decrease in the number of angiogenic sprouts, the quantification revealed that nintedanib 10 μM was the most effective treatment (5.7 ± 1.0 sprouts, vehicle control 19 ± 1.2 sprouts, 69% decrease). Interestingly, sirolimus 1 μM was more effective in decreasing sprout numbers than sirolimus 10 μM (35% and 16% decrease respectively).
However, when we assessed sprout length (Fig. 6C), we observed that 10 μM was more efficient in decreasing sprout length (78% decrease in 1 μM, and 22% decrease in 10 μM). Consistently, nintedanib 10 μM proved the most efficient treatment in decreasing sprout length (58 ± 5.0 μm) compared to vehicle control (100 ± 3.5 μm, 73% decrease). These results indicate a decrease in the angiogenic capabilities of the model due to drug treatment.
Next, we perfused our NEnC and TEnC vessels with a 40 kDa fluorescently-conjugated dextran and tracked its diffusion out of the vessel for 15 min (Fig. 6E). We observed that nintedanib 10 μM was most effective in reducing permeability (73% decrease from vehicle control) and recovering permeability levels to NEnC levels (6.3 ± 1.6 × 10−7 and 2.2 ± 1.8 × 10−6 cm s−1, respectively, p = 0.554), followed by sirolimus 1 μM (1.3 × 10−6 ± 4.0 × 10−7 cm s−1). Nintedanib 1 μM and sirolimus 10 μM showed no change in permeability compared to vehicle control and were significantly higher from NEnC permeability values (#p = 0.02 and ##p = 0.003, respectively). These results indicate that only nintedanib 10 μM was able to restore the TEnC to NEnC permeability levels.
Finally, we assessed the effect of the drugs on vessel confluency (Fig. 6F). Values higher than vehicle control would indicate an absence of vessel disruptions, consistent with NEnC phenotypes. Conversely, smaller values could indicate drug-induced toxicity. We only observed significant differences for sirolimus 10 μM, where vessel confluency plummeted to 53 ± 5.8% from the control (91 ± 1.1%). This result is consistent with a smaller length of sprouts and lower permeability (Fig. 6D and E). These results point toward nintedanib being a safer option than sirolimus at higher doses while having a superior anti-angiogenic effect in the models.
Interestingly, nintedanib was more effective in restoring normal vessel function than standard first-line therapies (i.e., sunitinib and pazopanib) (Fig. S9†). Sunitinib is a small molecule that inhibits multiple receptor tyrosine kinases, including platelet-derived growth factor receptors (PDGFRα and PDGFRβ) and vascular endothelial growth factor receptors (VEGFR1, VEGFR2 and VEGFR3).42 On the other hand, pazopanib is a second-generation multitargeted tyrosine kinase inhibitor that shares primary targets with sunitinib.43 We used the same dosages reported for sirolimus and nintedanib (i.e., 10 μM), which were validated for sunitinib and pazopanib in our previous work for this system, and found to be well-tolerated.24 We found that sunitinib significantly diminished lumen confluency (***p < 0.001). As for pazopanib, while it maintained higher confluency, it was still significantly different from the control condition (***p < 0.001). We calculated the permeability coefficient for pazopanib-treated vessels only, due to the poor performance of sunitinib. Consistent with our previous observations, the permeability coefficient was significantly lower than that of control vessels (*p = 0.04). Finally, we assessed sprouting events, and observed that sprouting did not change in number or length. All in all, pazopanib was not effective in restoring normal vessel function across readouts. These results reinforce our workflow, whereby targeting specific dysregulations could be more effective than a one-size-fits-all approach to ccRCC therapy.
Conclusions
ccRCC is a common genitourinary cancer with high inter- and intra-patient heterogeneity of response to systemic treatments. Although there are multiple approved treatments for ccRCC, there is considerable controversy concerning the proper selection, timing, and utilization of individual agents. Many clinical trials in the past few years have shown conflicting information regarding which patients are most likely to benefit from specific treatments. This evidence suggests that ccRCC may be ideal for a precision medicine approach, which can potentially help stratify patients who may benefit most from individual agents.
Single-patient clinical trials (also called N-of-1 clinical trials) consider an individual patient to investigate the efficacy or side-effect profiles of different interventions, in a data-driven effort to determine the optimal intervention for this individual patient. Some of the most common limitations for this kind of trial are design and repeatability constraints (i.e., the need to produce multiple measurements out of one subject, including appropriate controls). It is in this context that microfluidics can be an invaluable tool for an integrative approach in drug-testing.
Microfluidic models have demonstrated great potential in reproducing functional readouts (i.e., assays that use cell-based readouts, that result in quantifiable changes in cell behavior or response). Within microfluidic models, functional assays are possible and generally include viability assays after drug treatment, cell migration, cell permeability, or angiogenic sprouting response.
The concept of vessel normalization has long been a promise in the angiogenesis field. This approach highlights the differences between normal and tumor-associated vessels and presents anti-angiogenic therapies as a means to restore normal vessel function. However, to our knowledge, the application of this concept to transcriptional profiles has remained rather unexplored. We have shown that our microfluidic organotypic model can reproduce many hallmarks thoroughly defined for normal and tumor-associated endothelial vessels (i.e., different degrees of sprouting, differences in permeability), as well as the high inter and intra-patient heterogeneity described in the literature for ccRCC. The intra-patient heterogeneity was observed in the gene ontology diagrams, where some TEnC vessels were more closely related to NEnC vessels than others. On the other hand, inter-patient heterogeneity was observed in the disparity of gene expression profiles of TEnC vessels. This fact illustrates the necessity to generate patient-specific models and to define “vessel normalization” for each patient, regarding in each case the abnormalities observed in tumor-associated vessels as compared to normal ones.
Our preliminary results demonstrate that microfluidic models could be useful both in selecting appropriate drugs to restore normal vessel function in the clinic, as well as for narrowing down the drug selection and determining the most effective drug out of those available.
Particularly, in our proof of concept we showed that 10 μM nintedanib was the optimal treatment condition to normalize TEnC vessel models. Likewise, it showed a better safety profile (i.e., lower toxicity response) than sirolimus in our model. Interestingly, this response was different than in a 2D well plate, illustrating the differences existing in functional drug response between 2D and 3D models. Further, recent studies have demonstrated that 3D models recapitulate certain aspects of in vivo physiology (e.g., cell proliferation, drug response) better than 2D models,44 therefore reinforcing the argument in favor of 3D models.
Future studies should focus on obtaining pure and defined cell populations from primary samples. This is an unmet need in the field as evidenced by recent NIH grant calls, such as RFA-CA-06-004. In the future, clear-cut criteria for identifying and isolating these populations could be streamlined. Therefore, we could clearly delineate the crosstalk among different cell types in ccRCC. Advances in both microfluidic models and primary sample processing are required to fully implement functional precision medicine in the clinic, along with further clinical tests before implementation. Altogether, the approach presented in this article can guide precision medicine approaches leveraging functional readouts to inform patient treatment where clinical guidelines fail to choose among different treatment options.
Although most anti-angiogenic therapies have historically focused on the VEGF pathway, we now know that many other pathways are essential to the angiogenic response and can help fuel it in the absence of VEGF. This study has suggested that not all patients necessarily have dysregulation in the VEGF pathway, and therefore a one-size-fits-all approach of targeting this pathway may not suffice for many patients. In fact, a limitation of our study is that we included only angiogenesis-related targets in our transcriptomics analysis. In this paper, we were interested in providing a proof of concept for this precision medicine approach, although future work should test larger libraries of transcripts via RNA sequencing for a more in-depth validation of this methodology.
Particularly for the patient samples included in this proof of concept study, none of the patients treated with first-line therapies showed a sustained response. We corroborated these pieces of information in our model for pazopanib and sunitinib (i.e., two common first-line anti-angiogenic drugs) and demonstrated that none of them were effective in restoring normal vessel function. This approach could be adapted to precision medicine treatments, leveraging vessel models to stratify patients according to the pathways dysregulated and eventually to selecting non-first-line drugs that might prove to have a more durable effect. However, the number of investigated genes in this study and the number of patients assayed was limited. Therefore, more clinical investigation and thorough validation are still required to implement this approach, including the development of retrospective assays to validate the accuracy of the models in reproducing patient-response in vitro. Likewise, a comprehensive mimicry of angiogenic response and inhibition may require incorporating other microenvironmental factors, such as other cell components of the ccRCC tumor microenvironment. However, we believe that our study paves the way to a new approach in treating ccRCC in a more logical and informed approach.
Experimental
Microdevice fabrication
LumeNEXT microdevices were fabricated using standard soft lithography techniques, as previously described.45 Each PDMS layer was patterned with two heights: deep (500 μm) features, for the chamber and ledge, and shallow (250 μm) to hold the rod once the layers are together. This device has a hexagonal chamber (2.5 mm from opposing sides), with two side ports (∅ 0.75 mm) for hydrogel injection, and two differently sized ports (∅ 1.5 mm vs. ellipsoidal port of 0.75 and 1 mm axes) that hold a PDMS rod (∼∅ 0.35 mm), and are designed to enable passive pumping for fluid flow through the lumen. These and other dimensions are detailed in Fig. S8.†
Microdevice setup for vessel seeding
After assembly and UV-sterilization, devices were treated for 10 min with 1% poly(ethyleneimine) (Sigma-Aldrich, 03880) in water and with 0.1% glutaraldehyde (Sigma-Aldrich, G6257) in water for 30 min to enhance hydrogel attachment to the PDMS. Devices were washed at least three times with water and thoroughly dried before hydrogel injection. Type I rat tail collagen hydrogels were prepared at 6 mg ml−1 in ice to prevent premature gelation. Using a chilled tip, a mixture of 111.2 μl of collagen type I (10.76 mg ml−1, Corning, 354249); 3.5 μl NaOH 0.5 M (Fisher Scientific, S318); 20 μl PBS 10× (Fisher Scientific, BP3991) and 65.3 μl of dH2O was prepared and incubated on ice for 20 min. Provided the pH of the mixture be approximately 7.4, as checked via colorimetric strips (Capitol Scientific, PH1170-7), 6 μl of the mixture was injected into the LumeNEXT device, and polymerized for 10 min at room temperature, followed by 20 min at 37 °C. Afterward, the PDMS rods were pulled out of the polymerized collagen gel from the output port resulting in a tubular lumen structure (i.e., vessel) in the collagen gel.
Cell culture and isolation
Primary endothelial cells were grown in minimum essential medium (MEM) with L-glutamine, supplemented with 10% of FBS (Corning, 45000-734), 1% penicillin/streptomycin (Gibco, 15140122), 1% MEM vitamin mixture 100× (Lonza, 13-607C), 0.5% ITS (Roche, 11074547001), sodium pyruvate 1 mM (Lonza, 13-115E), 100 μM of MEM NEAA (ThermoFisher, 11140-050), 0.4 μg ml−1 of hydrocortisone (VWR, 101095-150), 5 ng ml−1 hEGF (Thermo-Fisher Scientific, PHG0311). The research protocol to obtain tumor and normal adjacent tissue following surgery at the University of Wisconsin Hospital (Madison, WI) was approved by the Institutional Review Board (2011-0719). Informed consent to use residual tissue was obtained from patients before surgery.
Primary endothelial cell isolation was performed as previously described.24 Briefly, a pathologist confirmed ccRCC diagnosis and tissue samples (ca. 3 cm3, a representative photograph of a piece of tissue in ESI† Fig. S1) were finely minced and digested. Digestion media was a mixture of MEM (Corning, 10-010-CV), 5 mg ml−1 collagenase (Thermo-Fisher 17100017), 5 mg ml−1 hyaluronidase (Sigma, H3506), 1 mg ml−1 of DNase I (Roche, 04716728001), and 1% penicillin–streptomycin. Samples were incubated in digestion media for 4 h at 37 °C. CD31 (i.e., platelet/endothelial cell adhesion molecule-1, also called PECAM-1) a classical marker of endothelial cells was used for the isolation of endothelial cells.46,47 Endothelial cells were isolated from the digestion mixture using anti-CD31-conjugated magnetic microbeads (Miltenyi Biotech, 130-091-935) and separation columns (Miltenyi Biotech, 130-042-401). CD31+ cells were seeded in 75 cm2 flasks (Corning, CLS430641U) at 3 × 104 cells per cm2.
Cell seeding in vessels
Primary endothelial cells were utilized for experiments at passage two to four, to avoid senescence observed after these passages. Each vessel was loaded with 2 μl of cell suspension at 50
000 cells per μl. The devices were then incubated for 45 min at 37 °C to allow for cell attachment. After that, vessels were washed three times, each with 10 μl of growth media to remove excess cells.
Immunofluorescence staining in 2D.
Primary cells grown on a glass-bottom well plate were fixed after 2 days of culture by incubating with 4% paraformaldehyde (EMScience, 15700) in PBS for 12 min. Unless specified otherwise, all the steps were performed at room temperature. Cells were washed three times with ice-cold 0.1% Tween in PBS for 5 min between every step. Cells were permeabilized with 0.1% saponin (TCI, S0019) in PBS for 10 min and blocked with 3% bovine serum albumin (BSA) (Sigma-Aldrich, A9056) in PBS for 1 h at 4 °C. Cells were incubated with 1 μg ml−1 anti-human CD31 (Abcam, ab9498), 1 μg ml−1 anti-human VEGFR2 (Abcam, ab39638), 2 μg ml−1 anti-human VE-cadherin (Abcam, ab33168) in 3% BSA overnight at 4 °C. Then, cells were incubated with 4 μg ml−1 AlexaFluor 488 goat anti-mouse (Thermo-FisherA-11029), 4 μg ml−1 647 goat anti-mouse (Thermo-Fisher A-11011), or PE-conjugated Anti-EpCAM [VU-1D9] (Abcam, ab112068) and 50 μg ml−1 DAPI (Life Technologies, D1306) in 3% BSA + 10% goat serum for 2 h at room temperature.
Immunofluorescence staining in vessels
Cell-lined vessels were fixed after four days of culture by perfusing 4% paraformaldehyde in PBS through the vessel and incubating for 30 min. Vessels were washed three times, with 0.1% Tween in PBS for 30 min between every step. Cells were then permeabilized with 0.2% Triton® X-100 for 30 min and blocked with 3% BSA in PBS overnight at 4 °C. Cells were incubated with human anti-VEcadherin (R&D, MAB9381) at 0.5 μg ml−1 overnight at 4 °C. Cells were then stained with 1
:
50 Texas Red®-X phalloidin (5 units), 4 μg ml−1 AlexaFluor 488 goat anti-mouse and 50 μg ml−1 DAPI in 3% BSA overnight at 4 °C. Finally, the vessels were washed to remove excess staining and minimize the background.
Fluorescence imaging
Diffusion assays and permeability calculations.
The barrier function of the vessel models was assessed by measuring solute diffusion across the vessel endothelium. A 1 μM solution of Texas Red dextran (40 kDa, D1845, ThermoFisher Scientific) was prepared in relevant media. For each vessel, 3 μL of dextran solution was injected through the small vessel port. Dextran diffusion was imaged with the Nikon TI® Eclipse inverted microscope every 5 min over 15 min.
Confocal imaging of vessels.
Fluorescent images were acquired using a Nikon TI® Eclipse inverted microscope (Melville, New York) and processed using the National Institutes of Health ImageJ software. Confocal images were acquired using a Leica SP8 3× STED super-resolution microscope (Wetzlar, Germany) in the UW-Madison Optical Imaging Core.
Image analysis.
Effective permeability coefficients were calculated using the following equation:48 | P = (1/Io)[(If − Io)/(tf − to)](D/4), | (1) |
where Io is the total initial intensity outside the vessel, If is the total intensity outside the vessel at 15 min, to is the initial time point, tf is the final time point of 15 min, and D is vessel diameter.
On the other hand, vessel confluency was calculated on phalloidin images after a binarization of images using a set threshold as previously described.24,49 Visual inspection of each threshold confirmed the accuracy in representing the initial image. Then, a consistent region of interest was defined to include only the vessel (i.e., not surrounding areas or sprouts). The percentage of pixels where the phalloidin signal was present was automatically measured for each vessel.
To perform our sprouting quantification, we adapted the methodology from previous studies,50 best illustrated in Wang et al.40 These authors used ImageJ to manually measure sprout length from the vessel to the furthest point of the sprout. If cells were escaped from the lumen and detached from a continuous sprout they were not considered.
Gene expression profiling of vessels
mRNA extraction.
NEnC and TEnC-lined vessels were cultured for three days. 2 vessels were pooled per condition and mRNA was extracted using a Dynabeads™ mRNA DIRECT™ Purification Kit (Thermo Fisher, 61011) according to the manufacturer's instructions. Briefly, collagen hydrogels containing vessels were transferred using isopropanol sterilized tweezers into 200 μl of lysis binding buffer. Collagen hydrogels were fragmented to ensure sample homogeneity by passing the sample repeatedly through a 23G needle until no hydrogel fragments were observable. Oligo-dT beads, previously resuspended in lysis buffer and 10 μl were added per sample. The sample with the beads was incubated for 6 minutes on ice. After that, two washes with 150 μl of buffer A and two more with buffer B were performed. Finally, the wash buffer was thoroughly aspirated, and the beads were resuspended in 12 μl of nuclease-free water (Ambion, AM9937).
HCT-116 cells (used as a control for CD31 status of the extracted cells) were seeded in 2D at 500
000 cells per well of a 6-well plate. RNA was extracted as with Dynabeads™ mRNA DIRECT™ Purification Kit, according to manufacturer's instructions for 2D cell cultures.
RT-qPCR.
mRNA was reverse transcribed to cDNA and pre-amplified using the RT2 PreAMP cDNA Synthesis Kit (Qiagen, 330451). mRNA from vessels was analyzed by qPCR using a Qiagen RT2 profiler angiogenesis panel (Qiagen, PAHS-024ZA) and RT2 SYBR® Green qPCR Mastermix (Qiagen, 330500) in a Roche 96-384 thermal cycler. As for HCT-116, they were analyzed using RT2 primer assay, by using the primers for CD31, EPCAM, CDH5, GAPDH and ACTB. Independent qPCR reactions were set for each of the primers, with 12.5 μl of RT2 SYBR® Green qPCR Mastermix, 10.5 μl of nuclease-free water, 1 μl of pre-amplification mix and 1 μl of RT2 primer. The conditions for the qPCR were the same as described for the RT2 profiler panel.
Data were analyzed using the 5 reference (housekeeping) genes provided for normalization using the Qiagen online software (http://pcrdataanalysis.sabiosciences.com/pcr/arrayanalysis.php).
GSEA and cBioportal.
We compiled the upregulated genes identified via qPCR with GSEA to perform a gene set enrichment analysis. To do this, we input the significantly dysregulated gene lists within the “investigate gene sets” option, using the target lists C4–C7 (computational gene sets, GO gene sets, oncogenic gene sets and immunologic gene sets). The top 10 gene sets were displayed. Our gene list submission provided a list of most probable GO terms along with their p-values (FDR q-value was set to less than 0.05). GO terms are presented as −log10 along with the number of genes used to compile the GO term list in GSEA. The same gene lists were compiled in cBioportal to generate the pathway networks and identify potential drug targets in our vessel models. The three largest databases of Renal Clear Cell Carcinoma were selected to match with known dysregulated pathways (TCGA Firehouse Legacy, Nature 2013 and PanCancer Atlas). Pathways were visualized with a minimal number of nodes and overlapped with actionable targets, with settings also set to a minimum. This automated analysis produced a list of the most likely dysregulated pathways and a small collection of drugs that would target these pathways.
Drug testing.
After 24 h of lining the vessel structures with primary cells, vessels were washed three times with primary cell media. Nintedanib (Selleckchem, S1010) or sirolimus (Pfizer®, Selleckchem, S1039) were resuspended at 10 mM in DMSO and diluted to final concentrations of 1 or 10 μM in primary cell media. 4 ml of drug solution was added per vessel plate, to ensure full coverage of the vessel microdevices. Drug conditions were compared to DMSO vehicle control (DMSO at 0.4% in primary cell media). The drugs were incubated for 72 h, and vessel permeability was then tested. Vessels were washed afterward and fixed for immunofluorescence staining.
Toxicity on patient B TEnC was tested via CellTiterGlo assay (Promega, G7570), as per manufacturer's instructions. 5000 TEnC cells from patient B were seeded in a white glass-bottom 96 well-plate. Nintedanib or sirolimus was added at different concentrations (10, 5, 2.5, 1.25, 0.625, 0.3125 μM) and incubated for 72 h before reading. Luminescence readouts were normalized to vehicle control (DMSO at 0.4% in primary cell media).
Statistical analysis.
All the experiments were repeated at least three times as independent biological repeats. All results are presented as the mean ± standard error of the mean. Data were analyzed using GraphPad Prism v8. Statistical significance was set at p < 0.05. *p < 0.05; **p < 0.01; ***p < 0.001. Shapiro–Wilk test assessed normality. Comparisons between two samples were performed with a Student's t-test with Welch's correction, after passing the Shapiro–Wilk normality test. Multiple comparisons by ANOVA were corrected using the Tukey test.
Author contributions
Conceptualization, MV; methodology, MV, JMA, ML; validation, MV; formal analysis, MV; investigation, MV, JC, JMA, resources, ML, EJA, DJB; data curation, MV, JMA; writing–original draft, MV, writing–review & editing, MV, JMA, ML, EJA, DJB; visualization, JMA; supervision, MV, EJA, DJB; project administration, MV, and DJB; funding acquisition, EJA, DJB;
Conflicts of interest
David J. Beebe holds equity in Bellbrook Labs L.L.C., Tasso Inc., Salus Discovery LLC, Lynx Biosciences Inc., Stacks to the Future L.L.C., Turba L.L.C., and Onexio Biosystems L.L.C. David J. Beebe is a consultant for Abbott Laboratories.
Acknowledgements
Authors acknowledge the use of the gene set enrichment analysis, GSEA software, and Molecular Signature Database (MSigDB) (Subramanian, Tamayo, et al. (2005), PNAS 102, 15545–15550, http://www.broad.mit.edu/gsea/). MV would also like to acknowledge Jeremy Cho for assistance with device fabrication and immunofluorescence and Elizabeth Clawson for providing helpful comments and edits. The authors acknowledge the financial support of the University of Wisconsin Carbone Cancer Center Support Grant P30 CA014520, NIH Moonshot Grant NCI R33CA225281 and the University of Wisconsin Optical Imaging Core.
Notes and references
- K.-J. Yu, S. K. Keskin, M. A. Meissner, F. G. Petros, X. Wang, L. D. Borregales, C. Gu, P. Tamboli, S. F. Matin, C. G. Wood and J. A. Karam, Cancer, 2018, 124, 4023–4031 CrossRef.
- J. M. Randall, F. Millard and R. Kurzrock, Cancer Metastasis Rev., 2014, 33, 1109–1124 CrossRef CAS.
- S. M. Crusz, Y. Z. Tang, S.-J. Sarker, W. Prevoo, I. Kiyani, L. Beltran, J. Peters, A. Sahdev, A. Bex and T. Powles, BMC Med., 2016, 14, 185 CrossRef.
- S. Signoretti, A. Flaifel, Y.-B. Chen and V. E. Reuter, J. Clin. Oncol., 2018, 36, 3553–3559 CrossRef CAS.
- E. Jonasch, J. Gao and W. K. Rathmell, BMJ, 2014, 349, g4797 CrossRef.
- P. Carmeliet and R. K. Jain, Nat. Rev. Drug Discovery, 2011, 10, 417–427 CrossRef CAS.
- M. Cully, Nat. Rev. Drug Discovery, 2017, 16, 87 CrossRef CAS.
- C. L. Cowey, C. Amin, R. S. Pruthi, E. M. Wallen, M. E. Nielsen, G. Grigson, C. Watkins, K. V. Nance, J. Crane, M. Jalkut, D. T. Moore, W. Y. Kim, P. A. Godley, Y. E. Whang, J. R. Fielding and W. K. Rathmell, J. Clin. Oncol., 2010, 28, 1502–1507 CrossRef CAS.
- T. K. Choueiri, B. I. Rini, J. M. G. Larkin, G. A. Bjarnason, G. Gravis, H. Gurney, J.-L. Lee, D. Keizman, J. B. A. G. Haanen, Y. Tomita, H. Uemura, L. Albiges, M. Schmidinger, M. B. Atkins, M. Mariani, M. Shnaidman, A. D. Pietro and R. J. Motzer, J. Clin. Oncol., 2017, 35, TPS4594 Search PubMed.
- R. J. Motzer, N. M. Tannir, D. F. McDermott, O. Aren Frontera, B. Melichar, T. K. Choueiri, E. R. Plimack, P. Barthelemy, C. Porta, S. George, T. Powles, F. Donskov, V. Neiman, C. K. Kollmannsberger, P. Salman, H. Gurney, R. Hawkins, A. Ravaud, M. O. Grimm, S. Bracarda, C. H. Barrios, Y. Tomita, D. Castellano, B. I. Rini, A. C. Chen, S. Mekan, M. B. McHenry, M. Wind-Rotolo, J. Doan, P. Sharma, H. J. Hammers, B. Escudier and I. CheckMate, N. Engl. J. Med., 2018, 378, 1277–1290 CrossRef CAS.
- A. Afghahi and G. W. Sledge, Jr., Cancer J., 2015, 21, 294–298 CrossRef CAS.
- B. M. Ghadimi, M. Grade, M. J. Difilippantonio, S. Varma, R. Simon, C. Montagna, L. Füzesi, C. Langer, H. Becker, T. Liersch and T. Ried, J. Clin. Oncol., 2005, 23, 1826–1838 CrossRef CAS.
- V. Prasad, Nature, 2016, 537, S63 CrossRef CAS.
- H. G. Augustin and G. Y. Koh, Science, 2017, 357, eaal2379 CrossRef.
- A. A. Friedman, A. Letai, D. E. Fisher and K. T. Flaherty, Nat. Rev. Cancer, 2015, 15, 747–756 CrossRef CAS.
- D. Senft, M. D. M. Leiserson, E. Ruppin and Z. A. Ronai, Trends Mol. Med., 2017, 23, 874–898 CrossRef.
- E. W. Esch, A. Bahinski and D. Huh, Nat. Rev. Drug Discovery, 2015, 14, 248–260 CrossRef CAS.
- N. Kuzmic, T. Moore, D. Devadas and E. W. K. Young, Biomech. Model. Mechanobiol., 2019, 18, 717–731 CrossRef.
- C. P. Miller, C. Tsuchida, Y. Zheng, J. Himmelfarb and S. Akilesh, Neoplasia, 2018, 20, 610–620 CrossRef CAS.
- S. Zeinali, C. A. Bichsel, N. Hobi, M. Funke, T. M. Marti, R. A. Schmid, O. T. Guenat and T. Geiser, Angiogenesis, 2018, 21, 861–871 CrossRef CAS.
- A. Pavesi, A. T. Tan, S. Koh, A. Chia, M. Colombo, E. Antonecchia, C. Miccolis, E. Ceccarello, G. Adriani, M. T. Raimondi, R. D. Kamm and A. Bertoletti, JCI Insight, 2017, 2, e89762 CrossRef.
- C. Pak, N. S. Callander, E. W. Young, B. Titz, K. Kim, S. Saha, K. Chng, F. Asimakopoulos, D. J. Beebe and S. Miyamoto, Integr. Biol., 2015, 7, 643–654 CrossRef CAS.
- C. Pauli, B. D. Hopkins, D. Prandi, R. Shaw, T. Fedrizzi, A. Sboner, V. Sailer, M. Augello, L. Puca, R. Rosati, T. J. McNary, Y. Churakova, C. Cheung, J. Triscott, D. Pisapia, R. Rao, J. M. Mosquera, B. Robinson, B. M. Faltas, B. E. Emerling, V. K. Gadi, B. Bernard, O. Elemento, H. Beltran, F. Demichelis, C. J. Kemp, C. Grandori, L. C. Cantley and M. A. Rubin, Cancer Discovery, 2017, 7, 462–477 CrossRef.
- J. A. Jimenez-Torres, M. Virumbrales-Munoz, K. E. Sung, M. H. Lee, E. J. Abel and D. J. Beebe, EBioMedicine, 2019, 42, 408–419 CrossRef.
- M. G. Lampugnani and E. Dejana, Thromb. Res., 2007, 120, S1–S6 CrossRef.
- R. Ronca, M. Benkheil, S. Mitola, S. Struyf and S. Liekens, Med. Res. Rev., 2017, 37, 1231–1274 CrossRef.
- J. Kalucka, L. P. de Rooij, J. Goveia, K. Rohlenova, S. J. Dumas, E. Meta, N. V. Conchinha, F. Taverna, L.-A. Teuwen and K. Veys, Cell, 2020, 180, 764–779 CrossRef CAS , e720.
- M. G. Lampugnani, E. Dejana and C. Giampietro, Cold Spring Harbor Perspect. Biol., 2018, 10(10), a029322 CrossRef CAS.
- B. Bussolati, I. Deambrosis, S. Russo, M. C. Deregibus and G. Camussi, FASEB J., 2003, 17, 1159–1161 CrossRef CAS.
- K. Hida, N. Maishi, C. Torii and Y. Hida, Int. J. Clin. Oncol., 2016, 21, 206–212 CrossRef CAS.
- S. Goel, D. G. Duda, L. Xu, L. L. Munn, Y. Boucher, D. Fukumura and R. K. Jain, Physiol. Rev., 2011, 91, 1071–1121 CrossRef CAS.
- C. Galambos, A. D. Minic, D. Bush, D. Nguyen, B. Dodson, G. Seedorf and S. H. Abman, PLoS One, 2016, 11, e0159005 CrossRef.
- M. De Palma, D. Biziato and T. V. Petrova, Nat. Rev. Cancer, 2017, 17, 457 CrossRef CAS.
- D. Ribatti, B. Nico, E. Crivellato, A. Roccaro and A. Vacca, Leukemia, 2007, 21, 44–52 CrossRef CAS.
- G. Neufeld and O. Kessler, Cancer Metastasis Rev., 2006, 25, 373–385 CrossRef CAS.
- M. V. Rojiani, J. Alidina, N. Esposito and A. M. Rojiani, Int. J. Clin. Exp. Pathol., 2010, 3, 775–781 Search PubMed.
- V. K. Mootha, C. M. Lindgren, K.-F. Eriksson, A. Subramanian, S. Sihag, J. Lehar, P. Puigserver, E. Carlsson, M. Ridderstråle, E. Laurila, N. Houstis, M. J. Daly, N. Patterson, J. P. Mesirov, T. R. Golub, P. Tamayo, B. Spiegelman, E. S. Lander, J. N. Hirschhorn, D. Altshuler and L. C. Groop, Nat. Genet., 2003, 34, 267–273 CrossRef CAS.
- G. M. Keating, Drugs, 2015, 75, 1131–1140 CrossRef CAS.
- J. Eglinger, H. Karsjens and E. Lammert, Inflammation Regener., 2017, 37, 1–9 CrossRef.
- W. Y. Wang, D. Lin, E. H. Jarman, W. J. Polacheck and B. M. Baker, Lab Chip, 2020, 20, 1153–1166 RSC.
- P. Nowak-Sliwinska, K. Alitalo, E. Allen, A. Anisimov, A. C. Aplin, R. Auerbach, H. G. Augustin, D. O. Bates, J. R. van Beijnum, R. H. F. Bender, G. Bergers, A. Bikfalvi, J. Bischoff, B. C. Böck, P. C. Brooks, F. Bussolino, B. Cakir, P. Carmeliet, D. Castranova, A. M. Cimpean, O. Cleaver, G. Coukos, G. E. Davis, M. De Palma, A. Dimberg, R. P. M. Dings, V. Djonov, A. C. Dudley, N. P. Dufton, S.-M. Fendt, N. Ferrara, M. Fruttiger, D. Fukumura, B. Ghesquière, Y. Gong, R. J. Griffin, A. L. Harris, C. C. W. Hughes, N. W. Hultgren, M. L. Iruela-Arispe, M. Irving, R. K. Jain, R. Kalluri, J. Kalucka, R. S. Kerbel, J. Kitajewski, I. Klaassen, H. K. Kleinmann, P. Koolwijk, E. Kuczynski, B. R. Kwak, K. Marien, J. M. Melero-Martin, L. L. Munn, R. F. Nicosia, A. Noel, J. Nurro, A.-K. Olsson, T. V. Petrova, K. Pietras, R. Pili, J. W. Pollard, M. J. Post, P. H. A. Quax, G. A. Rabinovich, M. Raica, A. M. Randi, D. Ribatti, C. Ruegg, R. O. Schlingemann, S. Schulte-Merker, L. E. H. Smith, J. W. Song, S. A. Stacker, J. Stalin, A. N. Stratman, M. Van de Velde, V. W. M. van Hinsbergh, P. B. Vermeulen, J. Waltenberger, B. M. Weinstein, H. Xin, B. Yetkin-Arik, S. Yla-Herttuala, M. C. Yoder and A. W. Griffioen, Angiogenesis, 2018, 21, 425–532 CrossRef.
- D. Huang, Y. Ding, Y. Li, W. M. Luo, Z. F. Zhang, J. Snider, K. Vandenbeldt, C. N. Qian and B. T. Teh, Cancer Res., 2010, 70, 1053–1062 CrossRef CAS.
- S. V. Keisner and S. R. Shah, Drugs, 2011, 71, 443–454 CAS.
- M. M. Morgan, M. K. Livingston, J. W. Warrick, E. M. Stanek, E. T. Alarid, D. J. Beebe and B. P. Johnson, Sci. Rep., 2018, 8, 7139 CrossRef.
- J. A. Jimenez-Torres, S. L. Peery, K. E. Sung and D. J. Beebe, Adv. Healthcare Mater., 2016, 5, 198–204 CrossRef CAS.
- M. P. Pusztaszeri, W. Seelentag and F. T. Bosman, J. Histochem. Cytochem., 2006, 54, 385–395 CrossRef CAS.
- P. Lertkiatmongkol, D. Liao, H. Mei, Y. Hu and P. J. Newman, Curr. Opin. Hematol., 2016, 23, 253–259 CrossRef CAS.
- V. H. Huxley, F. E. Curry and R. H. Adamson, Am. J. Physiol., 1987, 252, H188–H197 CAS.
- M. Virumbrales-Munoz, J. M. Ayuso, M. Olave, R. Monge, D. de Miguel, L. Martinez-Lostao, S. Le Gac, M. Doblare, I. Ochoa and L. J. Fernandez, Sci. Rep., 2017, 7, 11998 CrossRef.
- V. Van Duinen, D. Zhu, C. Ramakers, A. van Zonneveld, P. Vulto and T. Hankemeier, Angiogenesis, 2019, 22, 157–165 CrossRef CAS.
Footnote |
† Electronic supplementary information (ESI) available. See DOI: 10.1039/d0lc00252f |
|
This journal is © The Royal Society of Chemistry 2020 |