DOI:
10.1039/C9EN00938H
(Critical Review)
Environ. Sci.: Nano, 2020,
7, 1041-1060
The rise of the nanomaterial metabolite corona, and emergence of the complete corona
Received
20th August 2019
, Accepted 23rd February 2020
First published on 24th February 2020
Abstract
Bio–nano interactions are a promising area of research, focused particularly on the interactions between proteins and nanomaterials (NMs). These interactions lead to the formation of the protein corona, a layer of proteins adsorbed to the surface of NMs; this corona determines the way in which cells within organisms “recognise” and interact with NMs. Corona formation has proven critical for cellular uptake, intracellular localization and toxicity arising from NMs. However, a major class of biological chemicals have been overlooked in the efforts to understand and predict the interactions of NMs with living organisms, namely metabolites, which are also involved in signaling cascades and toxic responses in biological systems. To date, very few studies have investigated this aspect of the NM corona, with initial work focusing upon the use of carbon nanotubes to clean persistent organic pollutants from contaminated air or water. As the protein corona field grew, limited interest in the metabolite corona began to emerge with investigations into the lipid composition of the corona around inhaled NMs and eventually more holistic analyses of the metabolite corona. We suggest that the metabolite corona co-exists with the protein one, since these smaller molecules can fit in between proteins, and indeed are often bound into protein complexes. We hypothesize that metabolites influence NMs impacts on molecular signaling and adverse outcome pathways. We show initial evidence that the metabolite corona is complementary to protein coronas, following similar rules of adsorption based on abundance and affinity leading to metabolite fingerprints akin to protein fingerprints. This work aims to highlight the metabolite corona as an important, but as yet greatly under represented, area of bio–nano interaction research, and encourage researchers to explore this aspect of the interactions between NMs and their surrounding biological environments.
Environmental significance
The protein corona has been extensively studied over the past decade; the adsorbed proteins confer a biological identity to nanomaterials and influences their uptake by cells and organisms. However, this protein centric view of the corona overlooks a significant group of molecules in environmental matrices, namely metabolites, which greatly outnumber proteins. These coronal metabolites are beginning to garner interest from the bio–nano interface community, although not always discussed in terms of the corona or bio–nano interface. This review encompasses early studies using carbon nanomaterials for the removal of persistent organic pollutants from water through to metabolomics style analysis of the metabolite corona acquired by nanomaterials in contact with the model organism Daphnia magna. The co-existence of metabolites and proteins in the corona is also discussed, leading to the concept of the complete corona.
|
1.0 Introduction
The protein corona has been an intense field of research over the past decade since its potential significance for toxicity, cellular entry and trafficking were realized. As a result, a wide range of studies have investigated its formation,1–4 effects on cellular localization,3,4 toxicological consequences and how various physico/chemico properties of NMs and biological matrices can influence its qualitative and quantitative protein constituents.5,6 The number and breadth of publications in this field have now enabled models to be constructed to predict the formation and composition of the corona and the interactions that the NM–corona complexes have in their local environs.6 However, one aspect of the corona that has been largely ignored to date, is the metabolite corona. Metabolites are orders of magnitude smaller than proteins, typically below 1000 Da whereas proteins are measured on the kDa scale, and metabolites are typically reactants, intermediaries and products of enzymatic activity. While the idea of interactions between metabolites and NMs is not a new one, as this is one of the applications of surface enhanced Raman spectroscopy (SERS)7,8 and several approaches to “clean” samples prior to analyses,8,9 the potential significance of metabolite constituents of the NM biomolecule corona in terms of NMs impacts on molecular signaling pathways and eventual adverse outcome pathways (AOPs) or targeting ability for nanomedicines has yet to be systematically explored.
To put the number of metabolites present in just humans into context it is worth bearing in mind that the latest genomics studies report 20
678 protein encoding genes,10 while the latest version of the human metabolome database details >114
000 metabolites.11 These metabolites are incredibly diverse in terms of structure and molecular formula compared to proteins which are polymers formed from a pool of 20 amino acids, or DNA/RNA which are formed from a pool of just 4 nucleotide monomers.12 While this doesn't strictly do the complexity of the proteome and genome justice, because it does not consider post translational modifications of proteins or acetylation and methylation patterns of DNA, the range of possible metabolites is much greater. Metabolites can vary in molecular weight, polarity and degree of saturation (number of double or triple bonds) thus providing a wide range of functions which causes different metabolites to be concentrated to different extents both within cells and between different biofluids and tissues.12 For example, highly polar, conjugated metabolites such as testosterone glucuronide is likely to be found at highest concentrations in urine through which it is excreted or in the liver where it is produced. Non-polar hydrophobic metabolites such as phospholipids are much more likely to be found at high concentrations in cell membranes and in the lung surfactant, potentially making them the first metabolites to be encountered by NMs.
Metabolites are several orders of magnitude smaller than proteins, with the metabolomics community typically defining a metabolite as a molecule which is required for metabolic reaction for the growth, function and maintenance of a cell or organism with a mass <1500 Da.13,14 These metabolites are vital components of a wide range of cellular functions such as energy metabolism through glycolysis and the Krebs cycle in animals or the Calvin cycle in plants, fatty acid, nucleotide and aromatic amino acid production via the pentose phosphate pathway15 and steroidogenesis to produce corticoids, androgen and estrogen steroids from cholesterol.16 While it is not possible to give an average concentration of metabolites in biological fluids they will range from milli/micromolar (carbohydrates, bile acids and amino acids etc.) to sub-attomolar (steroids, neurotransmitters etc.).11 As with protein signaling molecules, metabolites play vital roles in cell signaling; for example, eicosanoids, which are produced primarily from the fatty acid arachidonic acid, are a class of oxylipin, which play important roles in inflammation, pain perception, immune responses and cell growth, and interact with cell membrane G-coupled receptors.17,18 Interactions with cellular receptors are known to be a major mechanism for NM internalization,19–21 therefore it would not be a surprise to find that metabolites in the corona may also influence cellular uptake. Furthermore, many other metabolite signaling compounds, such as steroids, interact with receptors of the nuclear receptor class through which they regulate gene expression.22,23 As such, adsorption of metabolites and their transport to locations where they do not normally occur may represent a significant potential route of toxicity for NMs that is currently unexplored.
This degree of metabolite diversity is conserved between organisms, as metabolism is a highly conserved process with only relatively minor differences between species. For example, whereas 11-ketotestosterone is found at relatively low levels in mammals, it is the primary androgen in fish.24 When moving to the plantae kingdom, the level of diversity expands significantly due to the different metabolic pathways (photosynthesis versus ATP hydrolysis); here the number of predicted metabolites is an order of magnitude greater than in humans.25 These encompass metabolites used within the Calvin cycle for photosynthesis through to flavonoids, which are phenolic compounds involved in pigmentation, growth, defense and signaling.26 Furthermore, the rhizosphere, an area of soil surrounding plant roots is rich in root exudate and microorganisms known as the root microbiome, representing another rich source of not just plant metabolites but also metabolites from this microbiome.27 In addition to these endogenous metabolites which are produced by, and play vital roles in, metabolism in organisms, there are many exogenous metabolites present in the environment, sometimes referred to as xenometabolites or xenobiotics. Due to their ubiquity in the environment, xenometabolites may also bind to NMs, forming part of their acquired eco-corona, the term used to describe the biomolecular coating acquired by NMs in the environment.28–30 These can include molecules such as polyaromatic hydrocarbons, pharmaceuticals not removed by wastewater treatment plants or chemicals used in agriculture and their associated breakdown products or metabolites. Many of these xenobiotics are known to still be biologically active in the environment and have been demonstrated to affect reproductive behavior and neurochemistry of fish to name just a single organism.30–34 Metabolites have been confirmed to be present in aquatic organism secretions (e.g., daphnia conditioned medium),35 while waste water treatment plants release thousands of tons of human waste including proteins and metabolites into rivers and oceans around the world.36 There have been a number of proteomics studies of soils37,38 and water columns39 demonstrating that proteins are present in the wider environment. Given the prevalence of these chemicals in the environment they are strong contenders to form part of the eco-corona and thus offer a potential new route of exposure to a wide range of organisms and plants, making these interactions key to characterize.
Despite their prevalence in biological organisms and the environment, the interactions between NMs and metabolites have yet to be explored extensively by nanoscientists trying to understand the formation of the corona or the interaction of NMs with the organisms they become exposed to. It is highly likely that the recruitment of the protein corona is influenced by the presence of metabolites particularly given that many NMs are produced and purchased in solutions stabilized by citrate, a metabolite from the citric acid cycle, while others are stabilized with tannic acid, glutamic acid, oleic acid, salicylic acid, or trichloroacetic acid.40,41 This also means that data about metabolite–protein interactions in the corona has already been collected serendipitously in many studies but the significance of this information has been overlooked to date. The reverse is also a distinct possibility as many proteins are known to interact with both endogenous metabolites or drugs,42–44 and as such the binding of proteins onto NMs is likely to attract metabolites also. Exploring and understanding the role of the metabolites in the NM bio-/eco-corona is an exciting prospect as many metabolites, either naturally occurring in organisms such as amino acids, sugars and lipids or unnatural contaminants from human activity such as cosmetics, pharmaceuticals and agricultural waste can act as signaling molecules or toxicants.31 Thus, understanding the contribution of metabolites to the NM corona, elucidating the fraction of the total corona that constitutes metabolites, and potentially uncovering new metabolite driven mechanisms behind NM uptake, distribution and toxicity that have, to date, eluded researchers due to the lack of available information on the metabolites components of the corona, offers important new research directions. The various potential mechanisms of corona formation and interactions between metabolites and proteins and between the corona and NMs are illustrated schematically in Fig. 1, and include metabolite recruitment of other metabolites or proteins, or protein-induced recruitment of metabolites as has been established for protein-induced recruitment of other proteins. Although the evidence for the metabolite corona is quite sparse to date, there are tantalizing hints that similar patterns of interaction exist for metabolites as for proteins, including abundance driven initial binding and differential binding of metabolites based on their affinities for the NMs surface leading to NM metabolomic fingerprints, which we highlight in the sections below where relevant. Thus, we present here a conceptual framework for the metabolite corona, complementary to and indeed interwoven with, that of the protein corona. It will only be once a full understanding of all aspects of the complete bio-/eco-corona is achieved that the field can hope to expand into the tightly regulated world of medicine to deliver on the promise of novel nanomedicines and indeed novel agrochemicals.
 |
| Fig. 1 Schematic illustration of hypothetical mechanisms for formation of the complete corona around NMs. A wide range of possibilities exist for the corona to acquire metabolites and proteins. (a) The direct adsorption of proteins from the environment is the predominant model at the moment, however protein–protein interactions (b), metabolite adsorption (c), metabolite induced adsorption of proteins (d), protein induced recruitment of metabolites (e), metabolite induced recruitment of further metabolites (f) or more likely a complex combination of all aforementioned methods with elements such as metabolite induced conformational changes in proteins (g). | |
In much the same way that nanoscientists have leveraged the success of proteomics in the analysis of the protein corona, researchers will need to embrace metabolomics, a much younger omics science, to uncover the secrets of the metabolite corona. Indeed, it will be necessary to bridge both proteomics and metabolomics in order to begin to unwind the interactions between metabolites and proteins during the formation and evolution of the complete NM corona and interactions of the combined biomolecule corona with living organisms and the environment. In some cases, this will require additional instrumentation and expertise, particularly for researchers coming from the environmental background, however, researchers already involved in protein corona characterisation already possess the necessary equipment to investigate the metabolite corona. As such it may be possible to assess both in parallel and collect twice as much data from the same sample, and a workflow for this, including some of the relevant points to consider is presented, that captures in brief some of the main challenges and pitfalls to watch out for. Future instrument optimization may allow for simultaneous analysis of the complete corona.
This work aims to position the metabolite corona as a new and greatly underappreciated aspect of the bio–nano interface, which sits alongside and complements the protein corona. We introduce the metabolite corona as a key constituent of the complete corona, by discussing current research in this emerging field and looking ahead to the future whereby this aspect of the corona may unlock further understanding of NM uptake, distribution, toxicity and drug/agrochemical delivery.45 It is clear from the current research that there are key similarities between the metabolite corona and protein corona fingerprints, which this review aims to spotlight. These key properties of the metabolite corona, such as preferential binding based on surface affinities and evolution based on available metabolites and competition from other biomolecules, which shadow those of the protein corona are highlighted throughout. Key directions in which to focus future research are identified, including development of predictive models of the complete corona.
2.0 Targeted analysis of persistent organic pollutant/xenometabolites binding to NMs
While not presented as corona formation, the ability of small molecule pollutants, which are often referred to as xenometabolites, to interact with NMs has been investigated for at least 12 years, with initial studies investigating the sequestering of persistent organic pollutants (POPs) by carbon nanotubes (CNTs). Due to the potential applications of carbon NMs in environmental remediation for removal of POPs, several studies modelling the binding characteristics of dioxins, polyhalogenated compounds and poly aromatic hydrocarbons (PAH) have been carried out. It is worth noting that in real environmental systems larger molecules such as humic acid would also compete for the NM surface, and indeed natural organic matter (NOM) is a known sink for pollutants, such that co-binding of pollutants and humics could occur.46–49
The first paper to report the adsorption of xenometabolites to NMs was Long et al. in 2001 (ref. 50) who found that dioxins exhibited strong π–π interactions between the hexagonal arrays of atoms in the CNT and the benzene rings in dioxins. Since then a wide array of POPs have been found to adsorb to carbon NMs including polyhalogentaed51–53 and PAH54–56 compounds and that this adsorption is highly pH dependent,51–53 an important factor also for protein corona formation. As with the protein corona, several models have also been developed to investigate computationally how these POPs interact with NMs and demonstrated that the adsorption of PAHs correlated with CNT surface area, micropore volume, volume ratio between the mesopores versus micropores and planar versus non-planar structures.55,56 These surface-specific interactions are analogous to those reported previously for proteins, where mesoporous silica NMs resulted in different proteins binding compared to smooth silica NMs, and differential protein binding to different pore volumes.57
While these investigations were not explicitly focused on endogenous metabolites they highlight the fact that xenometabolite adsorption to NMs may play a critical role in biological and environmental systems. Furthermore, these studies highlighted the importance of characterizing the adsorption characteristics of these compounds due to the potential for increased toxicity to humans and the wider environment through so-called Trojan horse effects, as seen in the protein corona, whereby they are carried into organisms as the NMs are taken up.58–60 Due to the ubiquitous nature of both POPs and NMs in the natural environment it is highly likely that these NM–POP complexes are to be found in all cases where both are present. Thus, a thorough understanding of the (xeno)metabolite corona and how it interacts with biological systems is vital to comprehend the potential harm caused by the release of NMs into the ecosystem.
3.0 Moving towards understanding the role of endogenous metabolites in the metabolite corona
An important application areas for NMs in the 21st century is their role as nanomedicines. Researchers have already begun to investigate the potential of the NM protein corona as a tool to diagnose patients and to understand the distribution and uptake of nanomedicines and its role in determining the efficacy of such medications.61–63 While proteins play vital roles in the signaling pathways determining NM uptake and biodistribution, metabolites are also important in these signaling cascades, such as eicosanoids in inflammation, and in a wide range of metabolic processes. As such, characterizing the endogenous metabolite corona could unlock the potential of nanomedicines while also informing studies into human and ecological NM toxicity.
3.1 Lipid corona
The first class of chemicals to be investigated in terms of the NM metabolite corona were lipids. The lipid constituents of the corona are of particular interest as they represent an initial point of contact between NMs and living organisms in the form of the lipid bilayer in cell membranes64 or in lung surfactant for inhaled NMs.65,66 Lung surfactant is comprised of 90% lipids and just 10% proteins.66,67 While the abundance of compounds doesn't necessarily reflect the abundance in the corona, it is important to consider the fact that in cases of NM inhalation, the predominant class of molecule present in the lungs are lipids not proteins and that 25% of human NM exposure is via inhalation.68 This makes the metabolite corona potentially of huge significance in the lungs particularly if there is evidence that it affects the uptake of NMs. The lipid portion of lung fluid is comprised of phospholipids and cholesterol while there are 4 predominant proteins, namely surfactant protein-A (SP-A), SP-B, SP-C and SP-D. SP-A and SP-D, which are large hydrophobic proteins that play a role in innate host defense and bind to inhaled NMs and pathogens.69 SP-B and SP-C are smaller hydrophilic membrane proteins which reduce alveolar surface tension.69 These properties and functions of the protein surfactant are vital, which the metabolite corona may influence thus affecting alveolar surface tension and NM uptake by alveolar epithelial cells and macrophages.66
As with the environmental contaminants discussed above, several studies of endogenous metabolites were assessed as smaller well controlled groups of metabolites rather than in the complex mixtures that NMs are exposed to in reality. In 2012, Ruge et al., investigated the interplay between lung surfactant lipids and proteins and its effect on macrophage clearance of NMs.67 Here magnetite nanoparticles (mNPs) exposed to SP-A and SP-D significantly increased alveolar macrophage uptake. When exposed to the vesicles constructed from surfactant lipids in the absence of the surfactant proteins, creating a lipid corona, cellular binding of starch coated mNPs greatly increased compared to pristine mNPs, indicating that lipids in the corona are key for internalisation. However, phosphatidylcholine-coated mNPs did not show increased internalization, due to their already lipid like surface coating. Interestingly surfactant lipids diminished the effect of SP-A on mNP–macrophage interactions, potentially due to the role surfactant lipids play in the stimulation of immune cells; surfactant lipids and SP-A may also competitively bind to the surface of NMs leading to this reduced effect, demonstrating the importance of generating competitive binding data to enable accurate binding kinetics to be modelled.67 Computational modelling has also been used to investigate the inhalation route of exposure to silver and polystyrene NMs.66 Here course-grained molecular dynamic models determined that the lipids and proteins of the alveolar fluid immediately coat the NMs upon exposure. This immediate formation of a complex lipid–protein corona reflects the rapid formation of the protein corona, though it remains unclear if the metabolite corona also evolves over time in the same way the protein corona does.70 The lipid compositions acquired by 5 and 15 nm AgNPs were highly conserved, and the model showed that lipids formed a bilayer on the hydrophilic AgNPs but on the hydrophobic PS-NPs only a monolayer formed. For both NMs, the presence of surfactant didn't hinder the formation of the lipid corona. In fact, SP-B and SP-C were found to mediate the formation of the corona by interacting directly with the lipids within the corona (as per the model shown schematically in Fig. 1e). In the Ag-NM corona SP-B interacted with, and oriented parallel to, the bilayer whereas the helical fragment of SP-C adopted a transmembrane conformation. Due to the monolayer on the PS-NPs both SP-B and C orientated parallel to the lipid monolayer.66 This is a particularly critical finding as it demonstrates that protein conformation and/or orientation is highly dependent upon the presence and structure of lipids in the corona. While these aspects of the protein corona are currently poorly understood or studied it does suggest that a deeper understanding of these metabolite–protein interactions in the corona may be key for any biological functionality if it enables or hinders access to aptamers. An important question for future research is to explore whether lipid-mediation of surfactant-like protein binding to NMs is a general phenomenon and whether it applies also to less lipid-like proteins and to other NM types.
It is well documented in the protein corona literature that the NM surface chemistry is a significant determining property in the composition of the protein corona formed around a NM.71,72 This aspect of the formation of the metabolite corona has not yet been thoroughly investigated with just a single study focusing on the impact of NM composition on metabolite corona composition. Gold NMs with a core diameter of 14 nm were functionalized with either cationic or anionic surface modifications. Anionic modifications were made by coating the AuNPs with citrate (anionic) whereas two cationic coatings (16-(mercaptohexadecyl)-trimethylammonium bromide (MTAB) or poly(allyamine)hydrochloride (PAC)) were investigated. These NPs were exposed to 4 different vesicles made up of either 100% dipalmitoylphosphatidylcholine (DOPC), 9
:
1 DOPC
:
1,2-dioleoyl-sn-glycero-3-phospho-L-serine (DOPS), 9
:
1 DOPC
:
1,2-dioleoyl-sn-glycero-3-phospho-1-rac-glycerol, (DOPG) and 9
:
1 DOPC
:
1,2-dioleoyl-sn-glycero-3-phosphoethanolamine (DOPE) (Fig. 2).64 Previously a study using quartz crystal microbalance (QCM) measurements showed that DMPC and DOPC interact with the same gold NPs when exposed to lipid bilayers.73 Interestingly hydrodynamic size was only significantly increased for the PAC-AuNPs upon vesicle exposure suggesting the NMs had agglomerated. The zeta potential inverted when the AuNPs were introduced into the 9
:
1 DOPC
:
DOPS and 9
:
1 DOPC
:
DOPG vesicles, suggesting that the anionic lipids had interacted with the cationic AuNPs. However, for the 100% DOPC and 9
:
1 DOPC
:
DOPE vesicle exposures no change in zeta potential was observed, likely due to the zwitterionic nature of DOPC and DOPE maintaining a positive surface despite lipid binding. When exposed to DOPC the AuNPs bound 80% of the available lipids with PAC and MTAB modified NMs retuning very similar lipid coronas. When co-exposed to DOPS and DOPC the PAC modified AuNPs bound 95% of both lipids compared to <65% for the MTAB modified NPs. This suggests that surface modifications influence corona formation in complex mixtures and that metabolites may aid the recruitment of others and vice versa (as suggested in Fig. 1d and f). This co-recruitment of metabolites (or proteins) is an important consideration as past exposures are going to have significant implications in the evolution of the complete corona; co-adsorption of toxins (xenometabolites) to the corona is important for potential Trojan horse effects.
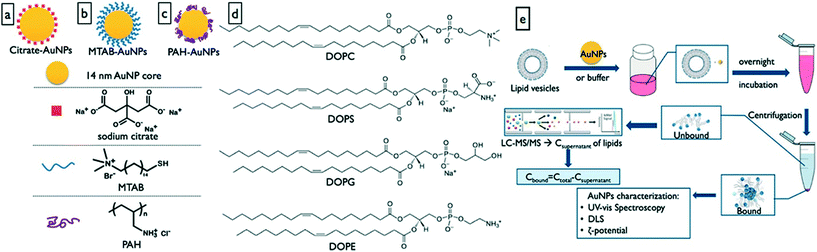 |
| Fig. 2 Investigation of the effect of NM surface functionalization on gold NM interactions with lipids, and isolation of the lipid corona. a) Citrate capped AuNPs, b) 16-(mercaptohexadecyl)trimethylammonium bromide capped AuNPs, c) poly(allylamine) hydrochloride capped AuNPs, d) vesicle phospholipid structures, and e) lipid corona characterisation workflow. Reprinted with permission from Zhang et al. Analytical Chemistry, 2018, 90, 14387, Copyright (2018) American Chemical Society.64 | |
The disparity between PAC and MTAB is due to differences in chemical structures: MTAB contains a quaternary ammonium head group and is less prone to forming hydrogen bonds, PAC is comprised of C16 chains more favorable for hydrophobic interactions with the lipid backbones. This confirms that surface modification/ligand binding plays a significant role in the formation of the metabolite corona. The anionic AuNPs only weakly interacted with the lipids with <5% adsorbing to the NMs.64 These findings suggest that manipulation of the NM physico/chemico properties influences the composition of the metabolite corona in much the same way it affects the protein corona. It remains to be seen how these properties affect interactions between the proteins and metabolites in the complete corona (see schematic in Fig. 1). However, it does potentially open the door for “designer” coronas through manipulation of NM properties with the goal of targeting specific tissues or having specific functions.
A significant recent publication quantified both the protein and lipid corona of polystyrene NMs while highlighting that a full characterization of the complete corona is likely to be key in future safe clinical applications of nanomedicines.45 Interestingly, cholesterol levels in the corona of polystyrene NMs were independent of the surface area but correlated with particle size. Furthermore, over a 24 h time period cholesterol levels decreased in the corona potentially due to lipid dissociation. Conversely, triglyceride concentrations in the corona had a correlation with surface area of the NMs. As with cholesterol there was a decrease in the concentration of triglycerides over time, this however was related to a decrease in apolipoprotein E which has a role in transporting lipids around the body. The authors noted the intriguing point that the binding mechanism between lipoproteins and NMs are not understood and that whether the lipids were present on the lipoprotein when it bound to the NM, or whether the lipids were adsorbed to the NM first which then attracted the lipoprotein, is not yet known.45 This perfectly highlights the need to characterise the metabolites within the corona as they have the potential to be key driving factors in the formation of the complete corona.
3.2 Targeted plasma metabolites
The blood is the most common biological matrix used for protein corona characterisation, as once within the body it's likely that NMs will become exposed to blood either after inhalation, ingestion or topical contact and thereby be systemically delivered around the body. Blood also represents one of the most commonly analysed biofluids in bioanalysis for drug development and biomarker discovery using proteomic and metabolomic approaches. As such, databases have been developed detailing the proteomic74,75 and metabolomic76,77 composition of human (and animal e.g. calf, rabbit etc.) blood making it an ideal candidate for coronal analysis.
A biological surface adsorption index (BSAI) has been proposed as a method to characterise competitive interactions between NMs and a selection of (xeno)metabolites. One such study proposed that the major factors driving adsorption of (xeno)metabolites to MWCNTs and 12 other NMs were primarily the lipophilicity of the (xeno)metabolites, their hydrogen bond basicity and their dipolarity/polarizability. Furthermore, this study demonstrated a strong binding capacity of 7 steroid hormones, progesterone, testosterone, estradiol, hydrocortisone, aldosterone, L-thyroxine and ethinyl estradiol, which play vital roles in regulating metabolism and act as important signaling molecules in biological systems, with ethinyl estradiol being the principal component in the contraceptive pill, an important endocrine disrupting chemical in the environment.22,78 Furthermore, the model predicted that DNA bases would also bind to NMs with a descending order of preference of adenine, thymine, guanine and cytosine, implying that NMs may interact with DNA, potentially being a source of mutations. The BSAI model builds upon the early binding studies of PAHs79 and is the first to model the binding properties of endogenous metabolites.
The presence of metabolites adsorbed to the surface of NMs was found to induce protein conformational changes that facilitated recognition and cellular uptake of the NMs.80 This study provides a critical insight into the significance of metabolites in the protein corona and leaves the door open for a host of follow-up work to begin to develop a full understanding of the roles that both proteins and metabolites play in the recruitment of each other into the corona and how they interact to bring about physiological effects in the body.
3.3 Dietary corona
Although very much an overlooked exposure route, dietary sources of NMs make up approximately 16% of human exposures to NMs, compared to 58% and 25% for topical and inhalation exposure, respectively.68,81 The high dietary exposure is typically through silica NMs which are used as a food additive (E551) and act as an anticaking agent.81 Furthermore, nano silver is being used as an antimicrobial and in some cases as a (supposed) supplement in health foods, nano selenium is used as an additive in green tea products, nano iron is used to treat contaminated water or as a supplement, and a variety of metal oxides are used as colorants or may elute from packaging materials.82 Due to the diverse compositions of diets around the world each meal or item of food represents a complex mixture of a vast array of biomolecules such as proteins and metabolites from a range of plant and animal species, making the dietary-corona potentially the most complex and diverse corona relevant for human exposure. Indeed the diversity of the food metabolome has been reviewed extensively.83–85 Despite the potential significance of this, just a single study has been performed thus far investigating interaction of NMs with dietary saccharides from honey and lipids from olive oil.81
Silica NM exposure to honey revealed a correlation between exposure time and honey concentration with more negative zeta potentials, however temperature appeared to have no effect on zeta potential. When run as mixtures of pure glucose, fructose, sucrose and maltose the zeta potential did not vary and the hydrodynamic size actually decreased suggesting other biomolecules in honey are responsible for the changes in DLS and for stabilizing the NMs. In honey, glucose and fructose interacted with the silica NMs in a concentration dependent manner at 25 °C, however zeta potential and hydrodynamic diameter were not affected by time but increased with increasing temperature. Sucrose and maltose were outcompeted by glucose and fructose (due to their higher concentration in honey) in the honey and showed no interaction, however when incubated with the NMs individually no preference for particular saccharides was observed.81 Upon exposure to olive oil DLS and zeta potential measurements were not possible due to the colour of the oil (which is excessively light scattering) and the fact that it lacks electrical conductivity. Instead, GC-MS was used to characterize the adsorption of lipids, which was found to be minimal and not correlated with temperature or exposure time. The poor interaction between lipids and the silica NMs was ascribed to the hydrophilic nature of the silica NMs generating repulsion between the lipids and silica.81 These findings highlight the importance of determining binding affinities for metabolites (as well as for proteins) individually and under competitive conditions, and assessing the nature of the binding, in order to facilitate the development of predictive models of the compete corona (proteins and metabolites).
The link between NMs taken up by edible plants and dietary exposure to the NMs has yet to be explored, although evidence is emerging of bioaccumulation in edible plants.86 The role of the metabolite corona in facilitating NM uptake into plants, their transformation in situ, and the impact of NMs on the metabolites themselves are all areas of active research currently linked to nano-enabled agriculture.
4.0 The application of untargeted analysis of the NM metabolite corona
Only via a full understanding of the protein and metabolite corona can their impact be understood and harnessed for better informed policies regarding NM use, disposal, safe-by-design NMs, and to exploit their full potential in nanomedicine. As such, a more holistic approach to analyzing the complete NM corona is required, with approaches to characterize and quantify the metabolite corona following in much the same manner as the protein corona is currently analyzed. In the case of the metabolite corona the field of metabolomics has a lot to offer,87 whereby a global analysis of metabolite (<1500 Da) are analyzed in as unbiased a fashion as possible.
4.1 Isolation of the metabolite corona
The first issue to arise when analyzing the metabolite corona is the generation of the sample for analysis following the incubation of the NMs in the desired matrix. There are two very different approaches to this: the first is a passive approach whereby the matrix is characterised prior to NM exposure and then again once the NMs have been pelleted (by centrifugation) and removed. Upon the second characterisation of the matrix following exposure it can be inferred that any drop in metabolite level is a result of adsorption to the NM, particularly if suitable controls are run to demonstrate that the metabolites do not adsorb to the reaction vessel. This technique will work very well for initial investigations using known standards for metabolites, either singly or as mixtures, and also offers the possibility to properly quantify the adsorption. However, in complex biological matrices where metabolite concentrations are dynamic this approach becomes more difficult, though would still work and drops in metabolite concentrations may still be detectable, however quantification of all metabolites then becomes unfeasible. This approach is also of no use in realistic exposure monitoring conditions as it would be impossible to analyse the matrix before and after exposure. The second, more direct, approach is to elute the metabolites from the NMs, as is done for protein corona analysis.35,88 There are a number of possible ways to do this, many of which have been applied in the metabolomics community to isolate metabolites from tissues or biofluids.89–91 The most likely to work are successive washes in solvents ranging from the polar, such as water, to the less polar solvents such as methanol and hexane.35 The chosen solvents would also be very much dependent upon the type of metabolite being isolated, for example the lipid corona will be more suited to an isopropanol or chloroform elution92 whereas the more polar fractions would be better suited to water and/or methanol.35 In cases where both the polar and non-polar portions of the corona need to be characterized then a biphasic extraction, such as the Bligh–Dyer,93 Folch94 and Matyash95 methods or their derivatives, where phase separation occurs between water and chloroform/MTBE with polar metabolites in the aqueous phase and lipids in the organic phase is preferred.91 These aforementioned methods also create a protein layer either at the solvent interface or at the bottom of the biphase, thus opening up the potential to be able to isolate the protein, polar metabolite and apolar metabolite portions of the complex corona. It is also likely that varying the pH of the solvent would have a significant effect on the elution of metabolites, either through hydrolysis of any bonds or changing the charge status of either the metabolite, NM or NM surface ligands thus releasing the metabolites.88 It may also be possible to dissolve the NM if the right pH and material is being used.96 Each NM and surface modified NM will require optimization of the metabolite corona isolation method, although general rules may emerge. As such it is recommended that future studies perform adequate method development to ensure that the full metabolite corona is being analysed rather than a small subset of the NM-bound metabolites. The aspects that require significant input will be which solvents and isolation techniques provide the most comprehensive overview of the metabolite corona, what steps are most prone to contamination and determination of whether any of the metabolites are adsorbed to the reaction or incubation vessels. A summary of these two approaches can be found in Table 1. It is also pertinent to point out that multi-omic sample preparation techniques are increasing in popularity in recent years with a number of studies developing and validating methods that isolate both the proteins and metabolites of a given sample in similar ways as described above.97–100 While it is likely that slight modifications may need to be made to these approaches to ensure the NMs themselves are also isolated to avoid injection into the analytical platforms, this is unlikely to be a major roadblock to the implementation to such approaches. Once the samples are prepared it is vital that they are correctly stored in order to prevent metabolite degradation. A general rule is to store metabolite samples at the lowest possible temperature available as many metabolites such as lipids degrade at room temperature or even in a fridge.101 While many methods are available for the analysis of metabolites in the literature it is vital that the NM bionano interactions and corona community follow the reporting guidelines published by the metabolomics community102 alongside those already in place in the NM community103–105 to ensure that high quality data is generated and to enable the data to be re-used by computational modelers to model the formation and interactions of the metabolite and eventually the complete corona.
Table 1 A comparison of passive and active isolation of the metabolite corona
|
Passive isolation |
Active isolation |
Pros |
• Straightforward approach |
• Can be applied to real world applications such as NPs found in the environment |
• Any biofluids can be assessed |
• No recovery study required in addition to a NP blank |
• Requires few injections as a before and after analysis isn't required |
• Same process for all metabolite class and NMs |
Cons |
• Not possible to perform in real world situations |
• A recovery study for each material and metabolite class is required |
• Requires more analyses, before and after exposure |
• Multiple wash steps are likely to be required to fully recover corona |
4.2 Instrumentation for metabolomics
A wide range of analytical techniques have been implemented in metabolomics to date, in several cases these technologies will be new to most nanoscientists, however some have seen extensive long-term use in several nanoscience labs. As such, several labs already contain the relevant instruments to characterise the metabolite corona. Here we provide an overview of the main approaches, the advantages and limitations of each approach, and the types of metabolites each is optimized for (Table 2).
Table 2 Analytical techniques for metabolite corona characterisation highlighting their advantages, disadvantages and target metabolites
Analytical technique |
Pros |
Cons |
Metabolites suitable for |
LC-MS – hydrophilic interaction liquid chromatography (HILIC) – reverse-phase (RP) |
Most popular method for metabolomics; wide range of different separation types possible (polar metabolites to lipids); common in many protein corona characterization labs |
Limited databases for metabolite identification as yet |
HILIC for polar metabolites such as amino acids, nucleotides, sugars, neurotransmitters, environmental contaminants |
RP for apolar metabolites such as: steroids, lipids, bile acids, environmental contaminants |
GC-MS |
Sensitive analysis; very reproducible; number of databases to identify metabolites; good for polar and volatile metabolites |
Non-volatile metabolites require derivatisation which is time consuming and adds expense |
Volative metabolites such as amino acids, nucleotides, PAHs, environmental contaminants, lipids |
CE-MS |
Highly sensitive due to low flow rates; well suited for high polar and highly charged metabolites can inject from very small sample values <5 μL |
Relatively expensive compared to LC-MS and GC-MS; fewer researchers with CE-MS experience; same limited databases for metabolite identification as LC-MS |
Well suited for highly charged polar metabolites such as amino acids, nucleotides, sugars, organic acids, neurotransmitters |
DIMS (direct-infusion mass spectrometry) |
Very high throughput |
No definitive identification of metabolites |
There are no specific classes of metabolites this suits, however those present at high concentrations are preferentially analysed |
NMR |
Ability to identify metabolites and their isomers; high throughput; good spectral databases |
Poor sensitivity when compared to mass spectrometry methods |
High abundance metabolites such as amino acids, sugars etc. however its poor with lipids in general |
4.2.1 Liquid chromatography-mass spectrometry (LC-MS).
LC-MS is the most common technique applied for metabolomics analysis106 and many reviews have discussed the use of LC-MS for metabolomics.106–108 LC-MS has already found use in NM metabolite corona analysis.92,96 In a typical separation using reversed phase chemistry, polar compounds elute first, typically with poor resolution, while more hydrophobic compounds elute later with better chromatographic resolution as the concentration of organic solvent increases over the run. This reversed phase separation is particularly suited for lipids and other non-polar metabolites such as steroids and bile acids. In the case of more polar metabolites such as amino acids or small organic acids hydrophilic interaction liquid chromatography (HILIC) is a more appropriate technique where less polar solvents, such as methanol or acetonitrile, are used at the start of the separation and move to the more polar water towards the end and require the use of a different column chemistry. The separated metabolites then enter the mass spectrometer and are typically ionized via electrospray ionization (ESI), which is a soft ionization technique that produces minimal fragments. However, mass spectrometers contain collision cells which enable the metabolites to be fragmented. These fragmentation patterns, when combined with accurate mass and retention order, can be used to help annotate (putatively identify) the metabolites.109 Unlike with the protein corona where extensive databases exist to help identify proteins, the metabolomics community does not yet have equivalent databases, making the identification of metabolites much more complex. However, a number of databases are emerging such as the Human Metabolome Database,77 Metlin110 and mycompoundID,111 which include accurate mass measurements and fragmentation patterns. Each database offers slightly different information as different types of fragmentation and/or different mass spectrometers were used, so there is still some work needed to integrate or compare across them.
Conveniently, many nanoscientists already have access to LC-MS, as nano liquid chromatography-mass spectrometry (nLC-MS) is also used to characterise the protein corona.3,112 While nLC-MS is not yet widely adopted for metabolomics it is growing in popularity31,113–117 and has proven to not only be highly reproducible but also very sensitive.32,118–120 Indeed, this technique has already had some success with determination of the lipid corona around nanofibrils, TiO2 and PS NMs.92
4.2.2 Gas chromatography-mass spectrometry (GC-MS).
GC-MS is a reasonably common technique in the metabolomics fields and has been extensively described in previous reviews.121–123 This technique is used to separate volatilized chemicals which are resistant to thermal degradation. These are separated using an inert carrier gas, typically helium, which flows through a GC column.12 Analytes are retained on the GC column and are eluted by changing the mass transfer between stationary phase and mobile phase in a temperature dependent manner. Over the course of the analyses the temperature increases in a similar way to the hydrophobicity of the mobile phase in LC-MS. Once eluted, the analytes enter a mass spectrometer that is usually equipped with electron ionization. This is a hard ionization technique and due to the high ionization energy applied, 70 eV, the analytes become heavily fragmented, often to such an extent that the parent ion is depleted.12 This high degree of fragmentation is critical to the identification of the analyte as each compound fragments in predictable patterns which can be screened against a number of well-established databases.124 To date, this approach has been used once for the metabolite corona around 4 metal oxide NMs.88
4.2.3 Capillary electrophoresis-mass spectrometry (CE-MS).
CE is a technology that has been used in colloid, particle and nanoscience labs for around 3 decades to characterise the size, surface charge and zeta potential of (nano)particles and has started to find a role in protein corona characterisation.87,125 When coupled to a mass spectrometer CE-MS is a valuable tool for determination and quantification of metabolomics, offering an orthogonal separation to both GC-MS and LC-MS.126–128 In CE separation, metabolites are separated based upon their size and charge over a fused glass capillary filled with a buffer solution under a high potential difference.87 This technique is particularly suited for small highly polar metabolites and is capable of injecting from a very small sample volume (<5 μL) and is extensively reviewed for metabolomics analysis.129–131 As with LC-MS, ionization is via ESI enabling metabolite database searches to be performed via the same (emerging) databases used for LC-MS based metabolomics.
4.2.4 Nuclear magnetic resonance (NMR).
NMR has been used for thousands of metabolomics studies and was initially the most widely applied technique due to its ability to analyse complex mixtures, to quantify metabolites, to elucidate chemical structures and the fact that it is extremely reproducible.132,133 However, the key limitation to the use of NMR is a lack of sensitivity which, to some extent, can be alleviated by larger magnetic field instruments or use of miniaturized or cryo-probes.132 While NMR has been used for NM characterization134 and to identify apolipoproteins135 and conformational changes in the proteins of the corona,136 it is still a relatively rare technique in NM studies and has not yet been directly applied to NM metabolite corona assessment, despite the relative abundance of NMR instrumentation in academia and industry. As with the other analytical techniques discussed, NMR based metabolomics has been extensively reviewed.121,137,138
4.3 Data analysis
Data analysis remains a bottleneck in untargeted metabolomics analysis, particularly with ESI-MS based techniques, due to the lack of comprehensive metabolite databases and extensive computational requirements.139,140 A number of open source software have been developed for chromatogram deconvolution, metabolite annotation and statistical analysis. These include XCMS141 and mzMine142 for deconvolution, normalization and visualization of the datasets with annotation packages possible to linked to them. Metaboanalyst is an online tool or can be accessed through R which will perform data transformation, normalization, imputation and univariate/multivariate statistical analysis of metabolomics datasets.143 More recently an open access end to end platform has been developed to encompass everything from peak picking to normalization, to missing value imputation, metabolite annotation and statistical analysis which offers a potential solution to this bottleneck.144 Experimental design and the research question are vital in order to perform the correct data analysis. To compare metabolite coronas between different NMs or matrices a classical metabolomics study can be performed with multivariate data analysis to highlight the metabolites that vary significantly between coronas and matrices. The multivariate analysis for metabolomics in these cases has already been extensively described and reviewed.145,146 However, a study just to identify what is present in the corona compared to a blank is more complex as missing values skew multivariate analysis meaning a peak picking and deconvolution step can be performed and metabolite annotation carried out (with adequate manual confirmation of peaks) or a known mixture of standards could be used to form a corona, which would allow for a targeted approach to be taken where specific masses are searched in the mass spectra.
4.4 Untargeted analysis of the metabolite corona
NMs have already started to find a niche in assisting in various metabolomics experiments in which they selectively bind to metabolites and thus concentrate them facilitating identification, as has been extensively reviewed by Zhang et al.8 Here, the use of NMs to carry out SERS, solid phase extraction (SPE) metabolite enrichment and for enhancing MALDI-MS ionization of metabolites are discussed in detail. An overview of all the untargeted studies using NMs for selective enrichment, including methods used and metabolites identified can be found in Table 3.
Table 3 Published metabolite corona studies including inadvertent ones where the NMs were utilized to “clean up” samples to reduce interference with target analytes
NM |
Biological matrix |
NM isolation method |
Corona isolation method |
Method for metabolite detection |
Metabolites found |
Ref. |
CNF: cellulose nano fibril, PS: polystyrene, SWCNT: single walled carbon nanotube, PC: phosphatidylcholine, PG: phosphatidylglycerol, PE: phosphatidylethanolamine, PI: phosphatidylinositol, PS: phosphatidylserine, SM: sphingomyelin, LPC: lysophosphatidylcholine, LPE: lyso phosphatidylethanolamine, DAG: diacylglycerol, TAG: triacylglycerol, GPL: glycerophospholipid, MGC: mono-glycerophosphocholines, MeOH: methanol, ESI: electrospray ionization, HCl: hydrochloric acid, MALDI-MS/MS: matrix assisted laser desorption ionization tandem mass spectrometry.
|
SWCNT |
Bronchoalveolar lavage fluid |
50% sucrose gradient |
Folch method |
LC-MS and MALDI-MS/MS |
5 PC, 4 PG, 3 PE, 2 PI, 2 PS, 1 SM |
65
|
TiO2, PS, CNF |
Serum and heavy cream |
Centrifugation |
Modified Folch method |
LC-MS |
6 LPC, 12 PC, 2 LPE, 6 PE, 13 DAG, 22 TAG |
92
|
Carbonate apatite, PS |
Serum, saliva, urine, FBS |
Centrifugation |
CA: particle digestion using HCl and methanol PS: 80% methanol |
LC-MS |
670 metabolites including: acyl carnitines, amino acids, aromatic compounds, bile acids, sugars, eicosanoids, glycerolipids, GPC, MGC, peptides, pyridines, fatty acids, sphingolipids, steroids and vitamins |
147
|
CuO, ZnO |
Cell media and simulated lung fluid |
Centrifugation |
Heating in HCl, mercaptoethanol and phenol |
GC-MS |
>200 metabolites including: carboxylic acids, primary aliphatic amines, amino acids and derivatives, aliphatic acyclic compounds, polyamines, phenols and derivatives and aromatic homomonocyclic compounds |
88
|
Anionic and cationic silica |
Urine |
N/A analysed in situ |
N/A analysed in situ |
NMR |
1-Methylhistidine, 1-methylguanidie, betaine, carnitine, creatinine, dimethylamine, dimethylglycine, lysine, trigonelline, trimethylamine N-oxide and arginine |
9
|
Amino modified PS |
Conditioned Daphnia magna culture medium |
Centrifugation |
2 × water wash, 1 × 2.5 : 1 MeOH : water |
Direct infusion mass spectrometry |
102 in +ve ESI 182 in −ve ESI including: amino acids, carbohydrates, fatty acids, glycolipids, nucleic acids and steroids |
35
|
4.4.1 Lipid corona.
Lipids have again been a class of high interest molecules for global analysis of NMs coronas. Initial work exposed SWCNT to mouse lung surfactant and the lipid corona was characterized using LC-MS, in addition to investigating the effect of the lipid corona on SWCNT phagocytosis by macrophages. LC-MS analysis of the corona identified phosphatidylcholines (PC), phosphatidylglycerols (PGs), phosphatidylinositols (PI), phosphatidylethanolamine (PE) and a sphingomyelin in the lipid corona however, they were found at different abundances to the lung surfactant itself.65 This reflects a well-known characteristic of the protein corona, that high affinity metabolites and proteins comprise the corona rather than the high abundance ones, however this implies that a similar evolution to the protein corona may also apply whereby initially high abundance lipids adsorb to be latter displaced by higher affinity lipids. Computational modelling of the binding mechanism suggested that the acyl chains of the phospholipids interact via hydrogen bonding with the SWCNT, with the polar head groups facing outwards into the biofluid (Fig. 3).65 In the absence of PG or PS or without both PS and PG macrophage internalization was reduced by 4, 9 and 18% respectively indicating that not only are phospholipids important for macrophage uptake of SWCNTs but the presence of lipid mixtures is synergistic, suggesting that the absence of one in the corona could prevent another from binding.65 This highlights a significant issue with the current protein centric view of the corona as currently the literature does not take into account the role of metabolites in cellular uptake. The synergistic effects seen between lipids is a particularly interesting property and opens up the field to investigate the possible synergistic effects of proteins and metabolites in NM uptake and distribution.
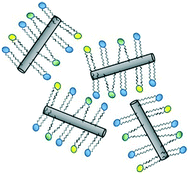 |
| Fig. 3 Predicted mode of adsorption of phospholipids to the walls of SWCNT via the acyl chains, as determined by molecular docking simulations.65 | |
A subsequent lipid corona investigation examined the adsorption of lipids to cellulose nanofibrils, titania and polystyrene NMs from serum and heavy cream to represent exposures to humans directly via blood and food products, respectively.92 A modified Folch method was applied to isolate the lipid corona with good recoveries being found across all NMs (>50%) when validated using a mixture of lipid standards.92 The lipid coronas isolated from the two matrices varied significantly with the serum coronas for all 3 NMs being comprised of 75% triacylglycerides (TAGs), 15–17% phospholipids and 7.5–11% diacylglycerides (DAGs) whereas for the heavy cream coronas for polystyrene and cellulose nanofibrils TAGs and DAGs made up 59% and 13–14% of the lipid corona with phospholipids accounting for a further 25% and lysophospholipids the remaining 2–3%. However, on titania the corona was comprised of 51% TAGs, 14% DAGs, 26% phospholipids and 9% lysophospholipids. These differences are thought to be due to the much more hydrophilic nature of the titania enabling up to 3 times more lysophospholipids to adsorb.92 While no explanation was given for these differences in TiO2 NM corona composition between cream and blood, it is possible that lysophospholipids are present in higher concentration in heavy cream than in blood and out-compete the other lipids for the hydrophilic titania NMs surface.
4.4.2 Metabolite corona.
The first metabolite corona study to use the term metabolomics was performed by Martel et al. in 2016.147 Here the corona acquired by an endogenous mineralo-organic NM, carbonate apatite, was investigated. These particles were exposed to a range of biofluids including plasma, saliva and urine all with a foetal bovine serum (FBS) concentration of 10%. The NMs were isolated and dissolved in acid, pelleted and dried and the metabolites solubilized for LC-MS analysis in 80% methanol. In total, 670 potential metabolites were uncovered from the analysis of the metabolite corona, with 377 being putatively identified based upon accurate mass matching with the human metabolome database. The metabolites putatively identified spanned a broad range of chemical classes including amino acids, bile acids, sugars, lipids, steroids, vitamins and carnitines. There were clear differences seen in concentrations between the different biofluids suggesting that the metabolite corona is biofluid dependent.147 This is a property of the metabolite corona which reflects the behavior of the protein corona, although it is too early days for analysis of whether trends in metabolite and protein coronas co-vary, although our expectation is that they will, given the multiple metabolite protein interactions inherent in biology, and the potential modes of complete corona formation shown in Fig. 1.
Pink et al.88 carried out extensive method development to isolate, derivatise, separate and detect 200 metabolite features from CuO, ZrO2, TiO2, ZnO and carbon black NMs incubated with cell culture medium and simulated lung fluid. Of the 200 features detected, 69% could be annotated based upon their retention index, mass and fragmentation patterns. Hierarchical clustering analysis (HCA) of these data primarily clustered NMs based upon the exposure media further strengthening the commonalities between the metabolite and protein coronas. However, the CuO and ZnO clustered only according to the NM composition suggesting that the physico-chemico properties of the NM are just as significant in corona formation as the biofluid they are exposed too. The first principal component, of a principal component analysis (PCA) scores plot, discriminated metabolite coronas based upon the presence of carboxylic acid, primary aliphatic amines, poly amines, plus other NH2 or COOH containing metabolites. The second component separation was driven by aromatic homo and monocyclic compounds, phenols and their derivatives, and pyrimidines.88 Interestingly both the Pink et al., and Martel et al., studies demonstrated that nucleotides adsorb into the NM corona which confirms BASI modelling suggesting nucleotides should adsorb strongly to NMs.79 This further raises the possibility that DNA and RNA may also bind, thus creating a DNA/RNA corona which could be of interest for novel biologics drugs or opening up the possibility that NMs could potentially act as a transfer mechanism of DNA sequences between organisms.88,147
Non-ferrous charged silica NMs were added to urine to enhance the quality of a urine NMR metabolomics analysis.148 The adsorption of metabolites to the anionic NMs eliminated several overlapping peaks from cationic metabolites including arginine which interferes with detection of lysine, histidine and glucose. As a result, the NMR analysis gained sensitivity, reduced peak broadening and reduced ambiguous metabolite identification. These anionic silica NMs were shown to have a particularly high affinity for metabolites with multiple methyl groups connected via a tertiary amine group such as trimethylamine N-oxide, trigonelline and creatinine. The opposite was true for cationic silica NMs which showed affinity for citric acid, lactase and shikimic acid.148 These findings offer an interesting insight into the metabolite corona, as they demonstrate that NM surface charge is equally important for this aspect of the corona as it is for determining the nature and composition of the protein corona.71 Furthermore, there is no ambiguity associated with isolating the metabolite corona from the NMs as this study was focused upon which metabolite signals were lost from the biofluid upon introduction of the NMs (i.e. using the first method described in section 4.1).
4.4.3 Metabolite eco-corona.
As with the protein corona, a metabolite eco-corona can form when NMs are exposed to environmental biofluids. This has recently been observed with amino-modified polystyrene NMs, commonly used to simulate microplastics or nanoscale plastic degradation products. Here the NMs were exposed to Daphnia magna conditioned medium to form a corona from the metabolites excreted by the daphnids as part of their normal water filtering activities. An extraction method comprising of 3 pure water wash steps and a 2.5
:
1 MeOH
:
H2O solubilization was assessed using SDS as a surrogate metabolite. Using a direct infusion selected ion monitoring (SIM)-stitch mass spectrometry approach149 a total of 284 features were detected that spanned 6 major chemical classes including amino acids, carbohydrates, fatty acids, glycolipids, nucleic acids and steroids. While only a small initial probe into the metabolite eco-corona, this offers an exciting prospect for further evaluation of the eco-corona particularly given the past studies investigating the role of NMs to mop up POPs.50,51,55 This could lead to unforeseen toxicities if the POPs form part of a dynamic corona which may then “unload” into various organisms which are normally exposed at lower doses based on equilibrium partitioning, or indeed are not exposed at all to these pollutants in normal settings.35 Importantly using a common freshwater organism model it is possible to compliment the metabolite corona data with the protein corona from the same organism;5,150 such studies with other model organisms may help pave the way to uncover similarities in behavior between the two corona classes, particularly with organisms from different environments.
5.0 Future perspectives – metabolite and complete NM coronas
The current knowledge on the metabolite corona supports the notion that this is a vital area of research that is yet to be exploited by nanoscientists for applications of NMs in the environment, agriculture and medicine, as well as for nanosafety assessment. It is clear that many of the NM properties that are known to influence the composition of the protein corona are also of significant importance to the formation of the metabolite corona, with properties such as NM elemental composition, surface charge, surface modification and biological environment being highlighted thus far.64,88,92,148 It is also becoming apparent that the NMs concentrate the metabolites they encounter based on preferential affinities in such a way that metabolite corona composition does not always reflect that of the matrix.
However, there is still a long way to go to fully understand the implications and effects of metabolite adsorption to NMs, and especially in terms of the interactions and cooperativity between metabolites and proteins. It will also become increasingly important to understand the relative contributions of proteins and metabolites to the corona, and how each is bound and recruited into the corona (i.e. direct interaction with the surface or via intramolecular interactions). To begin to do this, truly quantitative methods would be required for both the metabolite and protein coronas beginning with more simplistic standard solutions before progressing to the complex complete corona. These data may then be used to develop truly representative models of corona formation. We envision that the metabolite corona will follow a similar path as the protein corona in terms of interest and impact upon the nanoscience community, whereby in less than 10 years the number of papers increased exponentially from 35 published papers in 2009 to 234 in 2018 (PubMed “protein corona”). Factors such as NM curvature and structure have yet to be investigated from the metabolite perspective in addition to the time resolved evolution of the metabolite corona. From the protein corona we are well aware that proteins adsorb almost immediately to the surface of NMs following the introduction of biological matrices. These initial proteins are slowly exchanged for proteins with higher affinities for the NM surface, a significant direction for corona research would be to investigate the time evolution of the metabolites in the corona to inform corona kinetics models. Perhaps the most important consideration for future research is the co-variance of interactions between proteins and metabolites which are yet to be investigated to understand how each influence the other in the formation of the overall corona (Fig. 1), its interactions with organisms and its evolution as the NMs move through ecosystems and organisms. The combination of the metabolite and protein corona, or the complete corona, is likely the key to predicting NMs uptake, localization and impacts on molecular signaling and eventual adverse outcome pathways. It is likely also to be of vital importance for optimization of personalized medicine. Similarly, increased understanding of the protein and metabolite constituents of NOM or dissolved organic matter (DOM) is needed for characterization of environmental coronas; NM papers using NOM or DOM to date do not chemically characterise the NOM to identify the proteins and metabolites present in them, focusing only the role of NOM as a dispersant and characterising changes in NM diameter, dissolution or zeta potential. A key recommendation for the future is that researchers utilizing NOM/DOM provide chemical characterization of the protein and metabolite constituents as well as the humics.
Once the methods have been fully developed and validated for the characterisation of the metabolite corona around all types of NMs, we would expect that any studies which characterise the protein corona in the future will also look into the metabolite corona in parallel, given the similarity of approaches utilized and their likely co-variance. Knowledge of the complete corona will advance NMs applications in the clinical domain and facilitate applications of NMs for environmental remediation, as well as accelerating understanding of the implications of both aspects of the corona in terms of driving NM organism and cellular uptake, distribution, toxicity, bioaccumulation and dissolution. All of these metabolite corona data could then be used to build models to predict metabolite corona formation and predict its effect on biological systems in much the same way as models have been developed for the protein corona in an attempt to produce safe-by-design NM.6,151,152 Indeed, the real predictive power is likely to emerge from models of the complete corona, combining understanding of the presence and cooperativity of binding of both metabolites and proteins.
The ideal solution would be to characterise the protein and metabolite coronas of the same NMs for each exposure, as it would mean that no additional exposures would be required and twice as much data could be collected per sample.6 This would apply to both in vitro and in vivo studies, and to human and environmental samples. A proposed work flow based on current state of the art in metabolomics and proteomics is given below (Fig. 4) for initial progress going forward, allowing investigation of the two coronas (metabolite and protein) in parallel until such time as a method can be developed to isolate the protein and metabolites corona from the same NMs in the same vial. Among the challenges that need to be overcome in order to do this is to develop sample preparation and isolation methods that are compatible for both approaches; issues to be resolved include use of additional chemicals for reduction and alkylation of proteins that may displace metabolites in the corona for example, whereas washing the metabolite corona off with organic solvents may inadvertently cause a mis-characterization of the protein corona by selectively removing proteins such as albumin.153 Additionally, as new sample preparation techniques and analytical platforms develop it may become possible to simultaneously characterize the small and large biomolecules in the complete corona thus greatly increasing the data value from a single analysis.100 One potential solution to this are the biphasic extractions such as the Bligh and Dyer and the Matyash methods previously discussed which form a bilayer between polar and apolar metabolites but also forms a protein layer as an interface between the solvents or as a layer below the biphase. Once the metabolite phases have been removed for analysis this would leave a protein layer which could then be digested and analysed for the protein corona. While similar approaches have been used in proteomics and metabolomics analyses from the same sample97–99 this would require significant work to validate to ensure that the NMs are not mixed in with either biphase or the protein layer. As such the Bligh and Dyer approach may be the optimal starting point as the proteins are suspended between the two immiscible solvents with the NMs potentially sedimenting following the centrifugation step. Once these methods have been developed and suitably validated across a broad range of NMs then rapid complete corona analysis may be possible using high throughput methods which will enable high quality data to inform the fields of environmental nanoscience, nanomedicine and corona modelling.
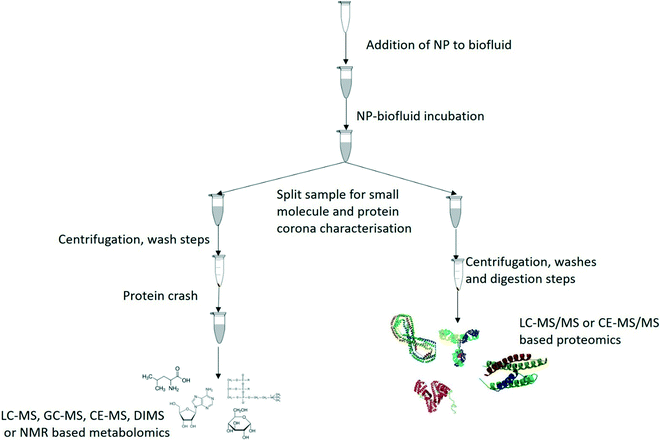 |
| Fig. 4 Proposed work flow for the parallel characterisation of metabolite and protein coronas from the same exposure media. | |
6.0 Summary
To date, research on the metabolite corona is sparse, focusing primarily on the lipid corona acquired by NMs during inhalation by humans, or interactions with xenometabolites either for remediation or to investigate potential changes in their bioavailability through binding to NMs. However, the studies that have been performed suggest that there are many similarities with the well-established protein corona in terms of specificity of binding and evolution over time and as a result of changes in the available biomolecules. As such, continued and increasing research on the metabolite corona and complete corona acquired by NMs has the potential to fill missing gaps in understanding of bio–nano interactions and the role of the corona and eco-corona in driving NMs interactions with biota. As knowledge develops, future regulatory guidelines on the use of NMs in agriculture and medicine, and broadly for environmental risk assessment, will increasingly require characterization of the complete corona as opposed to focusing on the single class of biological molecules that has dominated research to date, i.e., proteins. Metabolite corona research offers a rich vein of information to be tapped into to accelerate clinical and environmental applications and regulatory oversight of NMs in the future.
Conflicts of interest
There are no conflicts to declare.
Acknowledgements
The authors acknowledge funding from the European Union Horizon 2020 Programme (H2020) projects ACEnano (grant agreement No. 720952) and NanoCommons (grant agreement No. 731032).
References
- S. Tenzer, D. Docter, J. Kuharev, A. Musyanovych, V. Fetz, R. Hecht, F. Schlenk, D. Fischer, K. Kiouptsi, C. Reinhardt, K. Landfester, H. Schild, M. Maskos, S. K. Knauer and R. H. Stauber, Rapid formation of plasma protein corona critically affects nanoparticle pathophysiology, Nat. Nanotechnol., 2013, 8, 772–781 CrossRef CAS PubMed.
- J. Müller, J. Simon, P. Rohne, C. Koch-Brandt, V. Mailänder, S. Morsbach and K. Landfester, Denaturation via Surfactants Changes Composition of Protein Corona, Biomacromolecules, 2018, 19, 2657–2664 CrossRef PubMed.
- S. Tenzer, D. Docter, S. Rosfa, A. Wlodarski, J. Kuharev, A. Rekik, S. K. Knauer, C. Bantz, T. Nawroth, C. Bier, J. Sirirattanapan, W. Mann, L. Treuel, R. Zellner, M. Maskos, H. Schild and R. H. Stauber, Nanoparticle size is a critical physicochemical determinant of the human blood plasma corona: A comprehensive quantitative proteomic analysis, ACS Nano, 2011, 5, 7155–7167 CrossRef CAS PubMed.
- I. Lynch and K. A. Dawson, Protein-nanoparticle interactions, Nano Today, 2008, 3, 40–47 CrossRef CAS.
- F. Nasser and I. Lynch, Secreted protein eco-corona mediates uptake and impacts of polystyrene nanoparticles on Daphnia magna, J, Proteomics, 2016, 137, 45–51 CrossRef CAS PubMed.
- M. R. Findlay, D. N. Freitas, M. Mobed-Miremadi and K. E. Wheeler, Machine learning provides predictive analysis into silver nanoparticle protein corona formation from physicochemical properties, Environ. Sci.: Nano, 2018, 5, 64–71 RSC.
- B. Sharma, R. R. Frontiera, A. I. Henry, E. Ringe and R. P. Van Duyne, Mater. Today, 2012, 15, 16–25 CrossRef CAS.
- B. Zhang, M. Xie, L. Bruschweiler-Li and R. Brüschweiler, Nanoparticle-Assisted Metabolomics, Metabolites, 2018, 8, 21 CrossRef PubMed.
- B. Zhang, M. Xie, L. Bruschweiler-Li, K. Bingol and R. Brüschweiler, Use of Charged Nanoparticles in NMR-Based Metabolomics for Spectral Simplification and Improved Metabolite Identification, Anal. Chem., 2015, 87, 7211–7217 CrossRef CAS PubMed.
- M.-S. Kim, S. M. Pinto, D. Getnet, R. S. Nirujogi, S. S. Manda, R. Chaerkady, A. K. Madugundu, D. S. Kelkar, R. Isserlin, S. Jain, J. K. Thomas, B. Muthusamy, P. Leal-Rojas, P. Kumar, N. A. Sahasrabuddhe, L. Balakrishnan, J. Advani, B. George, S. Renuse, L. D. N. Selvan, A. H. Patil, V. Nanjappa, A. Radhakrishnan, S. Prasad, T. Subbannayya, R. Raju, M. Kumar, S. K. Sreenivasamurthy, A. Marimuthu, G. J. Sathe, S. Chavan, K. K. Datta, Y. Subbannayya, A. Sahu, S. D. Yelamanchi, S. Jayaram, P. Rajagopalan, J. Sharma, K. R. Murthy, N. Syed, R. Goel, A. A. Khan, S. Ahmad, G. Dey, K. Mudgal, A. Chatterjee, T.-C. Huang, J. Zhong, X. Wu, P. G. Shaw, D. Freed, M. S. Zahari, K. K. Mukherjee, S. Shankar, A. Mahadevan, H. Lam, C. J. Mitchell, S. K. Shankar, P. Satishchandra, J. T. Schroeder, R. Sirdeshmukh, A. Maitra, S. D. Leach, C. G. Drake, M. K. Halushka, T. S. K. Prasad, R. H. Hruban, C. L. Kerr, G. D. Bader, C. A. Iacobuzio-Donahue, H. Gowda and A. Pandey, A draft map of the human proteome, Nature, 2014, 509, 575–581 CrossRef CAS PubMed.
- D. S. Wishart, Y. D. Feunang, A. Marcu, A. C. Guo, K. Liang, R. Vázquez-Fresno, T. Sajed, D. Johnson, C. Li, N. Karu, Z. Sayeeda, E. Lo, N. Assempour, M. Berjanskii, S. Singhal, D. Arndt, Y. Liang, H. Badran, J. Grant, A. Serra-Cayuela, Y. Liu, R. Mandal, V. Neveu, A. Pon, C. Knox, M. Wilson, C. Manach and A. Scalbert, HMDB 4.0: The human metabolome database for 2018, Nucleic Acids Res., 2018, 46, D608–D617 CrossRef CAS PubMed.
- W. B. Dunn and D. I. Ellis, Metabolomics: Current analytical platforms and methodologies, TrAC, Trends Anal. Chem., 2005, 24, 285–294 CrossRef CAS.
- D. S. Wishart, D. Tzur, C. Knox, R. Eisner, A. C. Guo, N. Young, D. Cheng, K. Jewell, D. Arndt, S. Sawhney, C. Fung, L. Nikolai, M. Lewis, M. A. Coutouly, I. Forsythe, P. Tang, S. Shrivastava, K. Jeroncic, P. Stothard, G. Amegbey, D. Block, D. D. Hau, J. Wagner, J. Miniaci, M. Clements, M. Gebremedhin, N. Guo, Y. Zhang, G. E. Duggan, G. D. MacInnis, A. M. Weljie, R. Dowlatabadi, F. Bamforth, D. Clive, R. Greiner, L. Li, T. Marrie, B. D. Sykes, H. J. Vogel and L. Querengesser, HMDB: The human metabolome database, Nucleic Acids Res., 2007, 35, 521–526 CrossRef PubMed.
- R. Goodacre, S. Vaidyanathan, W. B. Dunn, G. G. Harrigan and D. B. Kell, Trends Biotechnol., 2004, 22, 245–252 CrossRef CAS PubMed.
- T. Wood, Cell Biochem. Funct., 1986, 4, 241–247 CrossRef CAS PubMed.
- W. L. Miller and R. J. Auchus, The molecular biology, biochemistry, and physiology of human steroidogenesis and its disorders, Endocr. Rev., 2011, 32, 81–151 CrossRef CAS PubMed.
- C. N. Serhan, J. Z. Haeggström and C. C. Leslie, Lipid mediator networks in cell signaling: update and impact of cytokines, FASEB J., 2018, 10, 1147–1158 CrossRef PubMed.
- C. M. Jenkins, A. Cedars and R. W. Gross, Cardiovasc. Res., 2009, 82, 240–249 CrossRef CAS PubMed.
- A. Salvati, C. Åberg, T. dos Santos, J. Varela, P. Pinto, I. Lynch and K. A. Dawson, Experimental and theoretical comparison of intracellular import of polymeric nanoparticles and small molecules: Toward models of uptake kinetics, Nanomedicine, 2011, 7, 818–826 CrossRef CAS PubMed.
- S. Lara, F. Alnasser, E. Polo, D. Garry, M. C. Lo Giudice, D. R. Hristov, L. Rocks, A. Salvati, Y. Yan and K. A. Dawson, Identification of Receptor Binding to the Biomolecular Corona of Nanoparticles, ACS Nano, 2017, 11(2), 1884–1893 CrossRef CAS PubMed.
- L. Treuel, X. Jiang and G. U. Nienhaus, J. R. Soc., Interface, 2013, 10, 20120939 CrossRef PubMed.
- W. Wahli and E. Martinez, Superfamily of steroid nuclear receptors: positive and negative regulators of gene expression, FASEB J., 1991, 5, 2243–2249 CrossRef CAS PubMed.
- B. L. Copple and T. Li, Pharmacol. Res., 2016, 104, 9–21 CrossRef CAS PubMed.
- E. Pretorius, W. Arlt and K. H. Storbeck, Mol. Cell. Endocrinol., 2017, 441, 76–85 CrossRef CAS PubMed.
- K. Saito and F. Matsuda, Metabolomics for Functional Genomics, Systems Biology, and Biotechnology, Annu. Rev. Plant Biol., 2010, 61, 463–489 CrossRef CAS PubMed.
- A. N. Panche, A. D. Diwan and S. R. Chandra, J. Nutr. Sci., 2016, 5, e47 CrossRef CAS PubMed.
- N. M. van Dam and H. J. Bouwmeester, Trends Plant Sci., 2016, 21, 256–265 CrossRef CAS PubMed.
- M. Markiewicz, J. Kumirska, I. Lynch, M. Matzke, J. Köser, S. Bemowsky, D. Docter, R. Stauber, D. Westmeier and S. Stolte, Changing environments and biomolecule coronas: Consequences and challenges for the design of environmentally acceptable engineered nanoparticles, Green Chem., 2018, 20, 4133–4168 RSC.
- N. Bonvallot, A. David, F. Chalmel, C. Chevrier, S. Cordier, J. P. Cravedi and D. Zalko, Metabolomics as a powerful tool to decipher the biological effects of environmental contaminants in humans, Curr. Opin. Toxicol., 2018, 8, 48–56 CrossRef.
- R. Al-Salhi, A. Abdul-Sada, A. Lange, C. R. Tyler and E. M. Hill, The xenometabolome and novel contaminant markers in fish exposed to a wastewater treatment works effluent, Environ. Sci. Technol., 2012, 46, 9080–9088 CrossRef CAS PubMed.
- A. David, A. Lange, A. Abdul-Sada, C. R. Tyler and E. M. Hill, Disruption of the Prostaglandin Metabolome and Characterization of the Pharmaceutical Exposome in Fish Exposed to Wastewater Treatment Works Effluent As Revealed by Nanoflow-Nanospray Mass Spectrometry-Based Metabolomics, Environ. Sci. Technol., 2017, 51, 616–624 CrossRef CAS PubMed.
- A. David, A. Abdul-Sada, A. Lange, C. R. Tyler and E. M. Hill, A new approach for plasma (xeno)metabolomics based on solid-phase extraction and nanoflow liquid chromatography-nanoelectrospray ionisation mass spectrometry, J. Chromatogr. A, 2014, 1365, 72–85 CrossRef CAS PubMed.
- A. M. Flores-Valverde, J. Horwood and E. M. Hill, Disruption of the steroid metabolome in fish caused by exposure to the environmental estrogen 17??-ethinylestradiol, Environ. Sci. Technol., 2010, 44, 3552–3558 CrossRef CAS PubMed.
- A. David, A. Lange, C. R. Tyler and E. M. Hill, Concentrating mixtures of neuroactive pharmaceuticals and altered neurotransmitter levels in the brain of fish exposed to a wastewater effluent, Sci. Total Environ., 2018, 621, 782–790 CrossRef CAS PubMed.
- K. Grintzalis, T. N. Lawson, F. Nasser, I. Lynch and M. R. Viant, Metabolomic method to detect a metabolite corona on amino functionalised polystyrene nanoparticles, Nanotoxicology, 2019, 1–12 Search PubMed.
- P. Zhang, Y. Shen, J.-S. Guo, C. Li, H. Wang, Y.-P. Chen, P. Yan, J.-X. Yang and F. Fang, Extracellular protein analysis of activated sludge and their functions in wastewater treatment plant by shotgun proteomics, Sci. Rep., 2015, 5, 12041 CrossRef PubMed.
- W. X. Schulze, G. Gleixner, K. Kaiser, G. Guggenberger, M. Mann and E. D. Schulze, A proteomic fingerprint of dissolved organic carbon and of soil particles, Oecologia, 2005, 142, 335–343 CrossRef PubMed.
- E. Zampieri, M. Chiapello, S. Daghino, P. Bonfante and A. Mello, Soil metaproteomics reveals an inter-kingdom stress response to the presence of black truffles, Sci. Rep., 2016, 6, 25773 CrossRef CAS PubMed.
- E. K. Moore, B. L. Nunn, D. R. Goodlett and H. R. Harvey, Identifying and tracking proteins through the marine water column: Insights into the inputs and preservation mechanisms of protein in sediments, Geochim. Cosmochim. Acta, 2012, 324–359 CrossRef CAS PubMed.
- I. L. Ardelean, L. B. N. Stoencea, D. Ficai, A. Ficai, R. Trusca, B. S. Vasile, G. Nechifor and E. Andronescu, Development of Stabilized Magnetite Nanoparticles for Medical Applications, J. Nanomater., 2017, 2017, 1–9 CrossRef.
- A. K. Singh, V. Viswanath and V. C. Janu, Synthesis, effect of capping agents, structural, optical and photoluminescence properties of ZnO nanoparticles, J. Lumin., 2009, 129, 874–878 CrossRef CAS.
- G. L. Trainor, The importance of plasma protein binding in drug discovery, Expert Opin. Drug Discovery, 2007, 2, 51–64 CrossRef CAS PubMed.
- D. L. Mobley and K. A. Dill, Structure, 2009, 17, 489–498 CrossRef CAS PubMed.
- R. D. Taylor, P. J. Jewsbury and J. W. Essex, A review of protein-small molecule docking methods, J. Comput.-Aided Mol. Des., 2002, 16, 151–166 CrossRef CAS PubMed.
- T. Lima, K. Bernfur, M. Vilanova and T. Cedervall, Understanding the Lipid and Protein Corona Formation on Different Sized Polymeric Nanoparticles, Sci. Rep., 2020, 10, 1129 CrossRef PubMed.
- J. Yang, P. Xu, L. Hu, G. Zeng, A. Chen, K. He, Z. Huang, H. Yi, L. Qin and J. Wan, Effects of molecular weight fractionated humic acid on the transport and retention of quantum dots in porous media, Environ. Sci.: Nano, 2018, 5, 2699–2711 RSC.
- W. Wu, G. Shan, Q. Xiang, Y. Zhang, S. Yi and L. Zhu, Effects of humic acids with different polarities on the photocatalytic activity of nano-TiO2 at environment relevant concentration, Water Res., 2017, 122, 78–85 CrossRef CAS PubMed.
- W. Kördel, M. Dassenakis, J. Lintelmann and S. Padberg, The importance of natural organic material for environmental processes in waters and soils (Technical Report), Pure Appl. Chem., 2007, 69, 1571–1600 Search PubMed.
- Y. L. Phyu, M. S. J. Warne and R. P. Lim, Toxicity of atrazine and molinate to the cladoceran Daphnia carinata and the effect of river water and bottom sediment on their bioavailability, Arch. Environ. Contam. Toxicol., 2004, 46, 308–315 CrossRef CAS PubMed.
- R. Q. Long and R. T. Yang, J. Am. Chem. Soc., 2001, 123, 2058–2059 CrossRef CAS PubMed.
- X. Peng, Y. Li, Z. Luan, Z. Di, H. Wang, B. Tian and Z. Jia, Adsorption of 1,2-dichlorobenzene from water to carbon nanotubes, Chem. Phys. Lett., 2003, 376, 154–158 CrossRef CAS.
- G. Aragay, F. Pino and A. Merkoçi, Chem. Rev., 2012, 112, 5317–5338 CrossRef CAS PubMed.
- M. Khajeh, S. Laurent and K. Dastafkan, Chem. Rev., 2013, 113, 7728–7768 CrossRef CAS PubMed.
- K. Yang, X. Wang, L. Zhu and B. Xing, Competitive sorption of pyrene, phenanthrene, and naphthalene on multiwalled carbon nanotubes, Environ. Sci. Technol., 2006, 40, 5804–5810 CrossRef CAS PubMed.
- K. Yang, L. Zhu and B. Xing, Adsorption of polycyclic aromatic hydrocarbons by carbon nanomaterials, Environ. Sci. Technol., 2006, 40, 1855–1861 CrossRef CAS PubMed.
- S. Zhang, T. Shao, S. S. K. Bekaroglu and T. Karanfil, The impacts of aggregation and surface chemistry of carbon nanotubes on the adsorption of synthetic organic compounds, Environ. Sci. Technol., 2009, 43, 5719–5725 CrossRef CAS PubMed.
- C. Vidaurre-Agut, E. Rivero-Buceta, E. Romaní-Cubells, A. M. Clemments, C. D. Vera-Donoso, C. C. Landry and P. Botella, Protein Corona over Mesoporous Silica Nanoparticles: Influence of the Pore Diameter on Competitive Adsorption and Application to Prostate Cancer Diagnostics, ACS Omega, 2019, 4, 8852–8861 CrossRef CAS PubMed.
- E. J. Park, J. Yi, Y. Kim, K. Choi and K. Park, Silver nanoparticles induce cytotoxicity by a Trojan-horse type mechanism, Toxicol. In Vitro, 2010, 24, 872–878 CrossRef CAS PubMed.
- I. L. Hsiao, Y. K. Hsieh, C. F. Wang, I. C. Chen and Y. J. Huang, Trojan-horse mechanism in the cellular uptake of silver nanoparticles verified by direct intra- and extracellular silver speciation analysis, Environ. Sci. Technol., 2015, 49, 3813–3821 CrossRef CAS PubMed.
- S. Sabella, R. P. Carney, V. Brunetti, M. A. Malvindi, N. Al-Juffali, G. Vecchio, S. M. Janes, O. M. Bakr, R. Cingolani, F. Stellacci and P. P. Pompa, A general mechanism for intracellular toxicity of metal-containing nanoparticles, Nanoscale, 2014, 6, 7052–7061 RSC.
- M. Hadjidemetriou and K. Kostarelos, Nanomedicine: Evolution of the nanoparticle corona, Nat. Nanotechnol., 2017, 12, 288–290 CrossRef CAS PubMed.
- R. Cai and C. Chen, The Crown and the Scepter: Roles of the Protein Corona in Nanomedicine, Adv. Mater., 2018, 1805740 Search PubMed.
- V. H. Nguyen and B. J. Lee, Protein corona: A new approach for nanomedicine design, Int. J. Nanomed., 2017, 12, 3137–3151 CrossRef CAS PubMed.
- X. Zhang, A. K. Pandiakumar, R. J. Hamers and C. J. Murphy, Quantification of Lipid Corona Formation on Colloidal Nanoparticles from Lipid Vesicles, Anal. Chem., 2018, 70(24), 14387–14394 CrossRef PubMed.
- A. A. Kapralov, W. H. Feng, A. A. Amoscato, N. Yanamala, K. Balasubramanian, D. E. Winnica, E. R. Kisin, G. P. Kotchey, P. Gou, L. J. Sparvero, P. Ray, R. K. Mallampalli, J. Klein-Seetharaman, B. Fadeel, A. Star, A. A. Shvedova and V. E. Kagan, Adsorption of surfactant lipids by single-walled carbon nanotubes in mouse lung upon pharyngeal aspiration, ACS Nano, 2012, 6, 4147–4156 CrossRef CAS PubMed.
- Q. Hu, X. Bai, G. Hu and Y. Y. Zuo, Unveiling the Molecular Structure of Pulmonary Surfactant Corona on Nanoparticles, ACS Nano, 2017, 11, 6832–6842 CrossRef CAS PubMed.
- C. A. Ruge, U. F. Schaefer, J. Herrmann, J. Kirch, O. Cañadas, M. Echaide, J. Pérez-Gil, C. Casals, R. Müller and C. M. Lehr, The interplay of lung surfactant proteins and lipids assimilates the macrophage clearance of nanoparticles, PLoS One, 2012, 7, e40775 CrossRef CAS PubMed.
- M. E. Vance, T. Kuiken, E. P. Vejerano, S. P. McGinnis, M. F. Hochella and D. R. Hull, Nanotechnology in the real world: Redeveloping the nanomaterial consumer products inventory, Beilstein J. Nanotechnol., 2015, 6, 1769–1780 CrossRef CAS PubMed.
-
I. Lynch, Role of the Biomolecule Corona in Nanoparticle Fate and Behavior, 2014, pp. 64–89 Search PubMed.
- C. Gräfe, A. Weidner, M. V. D. Lühe, C. Bergemann, F. H. Schacher, J. H. Clement and S. Dutz, Intentional formation of a protein corona on nanoparticles: Serum concentration affects protein corona mass, surface charge, and nanoparticle-cell interaction, Int. J. Biochem. Cell Biol., 2016, 75, 196–202 CrossRef PubMed.
- M. Lundqvist, J. Stigler, G. Elia, I. Lynch, T. Cedervall and K. A. Dawson, Nanoparticle size and surface properties determine the protein corona with possible implications for biological impacts, Proc. Natl. Acad. Sci. U. S. A., 2008, 105, 14265–14270 CrossRef CAS PubMed.
- H. Zhang, K. E. Burnum, M. L. Luna, B. O. Petritis, J. S. Kim, W. J. Qian, R. J. Moore, A. Heredia-Langner, B. J. M. Webb-Robertson, B. D. Thrall, D. G. Camp, R. D. Smith, J. G. Pounds and T. Liu, Quantitative proteomics analysis of adsorbed plasma proteins classifies nanoparticles with different surface properties and size, Proteomics, 2011, 11, 4569–4577 CrossRef CAS PubMed.
- L. L. Olenick, J. M. Troiano, A. Vartanian, E. S. Melby, A. C. Mensch, L. Zhang, J. Hong, O. Mesele, T. Qiu, J. Bozich, S. Lohse, X. Zhang, T. R. Kuech, A. Millevolte, I. Gunsolus, A. C. McGeachy, M. Doğangün, T. Li, D. Hu, S. R. Walter, A. Mohaimani, A. Schmoldt, M. D. Torelli, K. R. Hurley, J. Dalluge, G. Chong, Z. V. Feng, C. L. Haynes, R. J. Hamers, J. A. Pedersen, Q. Cui, R. Hernandez, R. Klaper, G. Orr, C. J. Murphy and F. M. Geiger, Lipid Corona Formation from Nanoparticle Interactions with Bilayers, Chem, 2018, 4, 2709–2723 CAS.
- N. L. Anderson and N. G. Anderson, The Human Plasma Proteome, Mol. Cell. Proteomics, 2002, 1(11), 845–867 CrossRef CAS PubMed.
- G. S. Omenn, D. J. States, M. Adamski, T. W. Blackwell, R. Menon, H. Hermjakob, R. Apweiler, B. B. Haab, R. J. Simpson, J. S. Eddes, E. A. Kapp, R. L. Moritz, D. W. Chan, A. J. Rai, A. Admon, R. Aebersold, J. Eng, W. S. Hancock, S. A. Hefta, H. Meyer, Y. K. Paik, J. S. Yoo, P. Ping, J. Pounds, J. Adkins, X. Qian, R. Wang, V. Wasinger, C. Y. Wu, X. Zhao, R. Zeng, A. Archakov, A. Tsugita, I. Beer, A. Pandey, M. Pisano, P. Andrews, H. Tammen, D. W. Speicher and S. M. Hanash, Overview of the HUPO Plasma Proteome Project: Results From the Pilot Phase With 35 Collaborating Laboratories and Multiple Analytical Groups, Generating a Core Dataset of 3020 Proteins and a Publicly-Available Database, Proteomics, 2005, 5(13), 3226–3245 CrossRef CAS PubMed.
- N. Psychogios, D. D. Hau, J. Peng, A. C. Guo, R. Mandal, S. Bouatra, I. Sinelnikov, R. Krishnamurthy, R. Eisner, B. Gautam, N. Young, J. Xia, C. Knox, E. Dong, P. Huang, Z. Hollander, T. L. Pedersen, S. R. Smith, F. Bamforth, R. Greiner, B. McManus, J. W. Newman, T. Goodfriend and D. S. Wishart, The human serum metabolome, PLoS One, 2011, 6(2), e16957 CrossRef CAS PubMed.
- D. S. Wishart, C. Knox, A. C. Guo, R. Eisner, N. Young, B. Gautam, D. D. Hau, N. Psychogios, E. Dong, S. Bouatra, R. Mandal, I. Sinelnikov, J. Xia, L. Jia, J. A. Cruz, E. Lim, C. A. Sobsey, S. Shrivastava, P. Huang, P. Liu, L. Fang, J. Peng, R. Fradette, D. Cheng, D. Tzur, M. Clements, A. Lewis, A. de Souza, A. Zuniga, M. Dawe, Y. Xiong, D. Clive, R. Greiner, A. Nazyrova, R. Shaykhutdinov, L. Li, H. J. Vogel and I. Forsythei, HMDB: A knowledgebase for the human metabolome, Nucleic Acids Res., 2009, 37, 603–610 CrossRef PubMed.
- A. M. Flores-Valverde, J. Horwood and E. M. Hill, Disruption of the steroid metabolome in fish caused by exposure to the environmental estrogen 17α-ethinylestradiol, Environ. Sci. Technol., 2010, 44, 3552–3558 CrossRef CAS PubMed.
- X. R. Xia, N. A. Monteiro-Riviere and J. E. Riviere, An index for characterization of nanomaterials in biological systems, Nat. Nanotechnol., 2010, 5, 671–675 CrossRef CAS PubMed.
- K. Prapainop, D. P. Witter and P. Wentworth, A chemical approach for cell-specific targeting of nanomaterials: Small-molecule-initiated misfolding of nanoparticle corona proteins, J. Am. Chem. Soc., 2012, 134, 4100–4103 CrossRef CAS PubMed.
- M.-R. Go, S.-H. Bae, H.-J. Kim, J. Yu and S.-J. Choi, Interactions between Food Additive Silica Nanoparticles and Food Matrices, Front. Microbiol., 2017, 8, 1013 CrossRef PubMed.
- B. S. Sekhon, Food nanotechnology - an overview, Nanotechnol., Sci. Appl., 2010, 3, 1–15 CAS.
- E. Trujillo, C. Davis and J. Milner, Nutrigenomics, proteomics, metabolomics, and the practice of dietetics, J. Am. Diet. Assoc., 2006, 106, 403–413 CrossRef CAS PubMed.
- S. Kim, J. Kim, E. J. Yun and K. H. Kim, Food metabolomics: from farm to human, Curr. Opin. Biotechnol., 2016, 37, 16–23 CrossRef CAS PubMed.
- J. M. Cevallos-Cevallos, J. I. Reyes-De-Corcuera, E. Etxeberria, M. D. Danyluk and G. E. Rodrick, Metabolomic analysis in food science: a review, Trends Food Sci. Technol., 2009, 20, 557–566 CrossRef CAS.
- C. M. Rico, S. Majumdar, M. Duarte-Gardea, J. R. Peralta-Videa and J. L. Gardea-Torresdey, Interaction of nanoparticles with edible plants and their possible implications in the food chain, J. Agric. Food Chem., 2011, 59, 3485–3498 CrossRef CAS PubMed.
- A. J. Chetwynd, E. J. Guggenheim, S. M. Briffa, J. A. Thorn, I. Lynch and E. Valsami-Jones, Current application of capillary electrophoresis in nanomaterial characterisation and its potential to characterise the protein and small molecule corona, Nanomaterials, 2018, 8, 99 CrossRef PubMed.
- M. Pink, N. Verma, C. Kersch and S. Schmitz-Spanke, Identification and characterization of small organic compounds within the corona formed around engineered nanoparticles, Environ. Sci.: Nano, 2018, 5, 1420–1427 RSC.
-
A. J. Chetwynd, W. B. Dunn and G. Rodriguez-Blanco, in Advances in Experimental Medicine and Biology, 2017, vol. 965, pp. 19–44 Search PubMed.
- H. Wu, A. D. Southam, A. Hines and M. R. Viant, High-throughput tissue extraction protocol for NMR- and MS-based metabolomics, Anal. Biochem., 2008, 372, 204–212 CrossRef CAS PubMed.
- J. Sostare, R. Di Guida, J. Kirwan, K. Chalal, E. Palmer, W. B. Dunn and M. R. Viant, Comparison of modified Matyash method to conventional solvent systems for polar metabolite and lipid extractions, Anal. Chim. Acta, 2018, 1037, 301–315 CrossRef CAS PubMed.
- J. Y. Lee, H. Wang, G. Pyrgiotakis, G. M. DeLoid, Z. Zhang, J. Beltran-Huarac, P. Demokritou and W. Zhong, Anal. Bioanal. Chem., 2018, 1–10 Search PubMed.
- E. G. Bligh and W. J. Dyer, A rapid method of total lipid extraction and purification, Can. J. Biochem. Physiol., 1959, 37, 911–917 CrossRef CAS PubMed.
- J. Folch, M. Llles and G. H. Sloane Stanley, A simple method for the isolation and purification of total lipides from animal tissues, J. Biol. Chem., 1957, 226, 497–509 CAS.
- V. Matyash, G. Liebisch, T. V. Kurzchalia, A. Shevchenko and D. Schwudke, J. Lipid Res., 2008, 49, 1137–1146 CrossRef CAS PubMed.
- J. Martel, C. Y. Wu, C. Y. Hung, T. Y. Wong, A. J. Cheng, M. L. Cheng, M. S. Shiao and J. D. Young, Fatty acids and small organic compounds bind to mineralo-organic nanoparticles derived from human body fluids as revealed by metabolomic analysis, Nanoscale, 2016, 8, 5537–5545 RSC.
- C. D. Nicora, K. E. Burnum-Johnson, E. S. Nakayasu, C. P. Casey, R. A. White, T. R. Chowdhury, J. E. Kyle, Y. M. Kim, R. D. Smith, T. O. Metz, J. K. Jansson and E. S. Baker, The MPLEx protocol for multi-omic analyses of soil samples, J. Visualized Exp., 2018, 135, e57343 Search PubMed.
- E. S. Nakayasu, C. D. Nicora, A. C. Sims, K. E. Burnum-Johnson, Y.-M. Kim, J. E. Kyle, M. M. Matzke, A. K. Shukla, R. K. Chu, A. A. Schepmoes, J. M. Jacobs, R. S. Baric, B.-J. Webb-Robertson, R. D. Smith and T. O. Metz, MPLEx: a Robust and Universal Protocol for Single-Sample Integrative Proteomic, Metabolomic, and Lipidomic Analyses, mSystems, 2016, 1(3), e00043-16 CrossRef PubMed.
- C. Coman, F. A. Solari, A. Hentschel, A. Sickmann, R. P. Zahedi and R. Ahrends, Simultaneous metabolite, protein, lipid extraction (SIMPLEX): A combinatorial multimolecular omics approach for systems biology, Mol. Cell. Proteomics, 2016, 15(4), 1453–1466 CrossRef CAS PubMed.
- A. Zougman, J. Wilson, L. Roberts and R. Banks, Detergent-free simultaneous sample preparation method for proteomics and metabolomics, J. Proteome Res., 2019 DOI:10.1021/acs.jproteome.9b00662.
- E. A. Palmer, H. J. Cooper and W. B. Dunn, Investigation of the 12-Month Stability of Dried Blood and Urine Spots Applying Untargeted UHPLC-MS Metabolomic Assays, Anal. Chem., 2019, 91(22), 14306–14313 CrossRef CAS PubMed.
- L. W. Sumner, A. Amberg, D. Barrett, M. H. Beale, R. Beger, C. A. Daykin, T. W.-M. Fan, O. Fiehn, R. Goodacre, J. L. Griffin, T. Hankemeier, N. Hardy, J. Harnly, R. Higashi, J. Kopka, A. N. Lane, J. C. Lindon, P. Marriott, A. W. Nicholls, M. D. Reily, J. J. Thaden and M. R. Viant, Proposed minimum reporting standards for chemical analysis: Chemical Analysis Working Group (CAWG) Metabolomics Standards Initiative (MSI), Metabolomics, 2007, 3, 211–221 CrossRef CAS PubMed.
- M. Faria, M. Björnmalm, K. J. Thurecht, S. J. Kent, R. G. Parton, M. Kavallaris, A. P. R. Johnston, J. J. Gooding, S. R. Corrie, B. J. Boyd, P. Thordarson, A. K. Whittaker, M. M. Stevens, C. A. Prestidge, C. J. H. Porter, W. J. Parak, T. P. Davis, E. J. Crampin and F. Caruso, Minimum information reporting in bio–nano experimental literature, Nat. Nanotechnol., 2018, 13, 777–785 CrossRef CAS PubMed.
- H. S. Leong, K. S. Butler, C. J. Brinker, M. Azzawi, S. Conlan, C. Dufès, A. Owen, S. Rannard, C. Scott, C. Chen, M. A. Dobrovolskaia, S. V. Kozlov, A. Prina-Mello, R. Schmid, P. Wick, F. Caputo, P. Boisseau, R. M. Crist, S. E. McNeil, B. Fadeel, L. Tran, S. F. Hansen, N. B. Hartmann, L. P. W. Clausen, L. M. Skjolding, A. Baun, M. Ågerstrand, Z. Gu, D. A. Lamprou, C. Hoskins, L. Huang, W. Song, H. Cao, X. Liu, K. D. Jandt, W. Jiang, B. Y. S. Kim, K. E. Wheeler, A. J. Chetwynd, I. Lynch, S. M. Moghimi, A. Nel, T. Xia, P. S. Weiss, B. Sarmento, J. das Neves, H. A. Santos, L. Santos, S. Mitragotri, S. Little, D. Peer, M. M. Amiji, M. J. Alonso, A. Petri-Fink, S. Balog, A. Lee, B. Drasler, B. Rothen-Rutishauser, S. Wilhelm, H. Acar, R. G. Harrison, C. Mao, P. Mukherjee, R. Ramesh, L. R. McNally, S. Busatto, J. Wolfram, P. Bergese, M. Ferrari, R. H. Fang, L. Zhang, J. Zheng, C. Peng, B. Du, M. Yu, D. M. Charron, G. Zheng and C. Pastore, On the issue of transparency and reproducibility in nanomedicine, Nat. Nanotechnol., 2019, 14, 629–635 CrossRef CAS PubMed.
- A. J. Chetwynd, K. E. Wheeler and I. Lynch, Best practice in reporting corona studies: Minimum information about Nanomaterial Biocorona Experiments (MINBE), Nano Today, 2019, 28, 100758 CrossRef.
- G. A. Theodoridis, H. G. Gika, E. J. Want and I. D. Wilson, Liquid chromatography-mass spectrometry based global metabolite profiling: A review, Anal. Chim. Acta, 2012, 711, 7–16 CrossRef CAS PubMed.
- H. G. Gika, I. D. Wilson and G. A. Theodoridis, LC-MS-based holistic metabolic profiling. Problems, limitations, advantages, and future perspectives, J. Chromatogr. B: Anal. Technol. Biomed. Life Sci., 2014, 966, 1–6 CrossRef CAS PubMed.
- M. R. Viant and U. Sommer, Mass spectrometry based environmental metabolomics: A primer and review, Metabolomics, 2013, 9, 144–158 CrossRef CAS.
- W. J. Nash and W. B. Dunn, From mass to metabolite in human untargeted metabolomics: Recent advances in annotation of metabolites applying liquid chromatography-mass spectrometry data, TrAC, Trends Anal. Chem., 2019, 120, 115324 CrossRef.
-
C. A. Smith, G. O'Maille, E. J. Want, C. Qin, S. A. Trauger, T. R. Brandon, D. E. Custodio, R. Abagyan and G. Siuzdak, in Therapeutic Drug Monitoring, 2005, vol. 27, pp. 747–751 Search PubMed.
- T. Huan, C. Tang, R. Li, Y. Shi, G. Lin and L. Li, MyCompoundID MS/MS Search: Metabolite Identification Using a Library of Predicted Fragment-Ion-Spectra of 383,830 Possible Human Metabolites, Anal. Chem., 2015, 87, 10619–10626 CrossRef CAS PubMed.
- R. Eigenheer, E. R. Castellanos, M. Y. Nakamoto, K. T. Gerner, A. M. Lampe and K. E. Wheeler, Silver nanoparticle protein corona composition compared across engineered particle properties and environmentally relevant reaction conditions, Environ. Sci.: Nano, 2014, 1, 238–247 RSC.
- A. J. Chetwynd, A. Abdul-Sada, S. G. Holt and E. M. Hill, Use of a pre-analysis osmolality normalisation method to correct for variable urine concentrations and for improved metabolomic analyses, J. Chromatogr. A, 2016, 1431, 103–110 CrossRef CAS PubMed.
- A. J. Chetwynd, A. Samarawickrama, J. H. Vera, S. A. Bremner, A. Abdul-Sada, Y. Gilleece, S. G. Holt and E. M. Hill, Nanoflow-Nanospray Mass Spectrometry Metabolomics Reveals Disruption of the Urinary Metabolite Profiles of HIV-Positive Patients on Combination Antiretroviral Therapy, JAIDS, J. Acquired Immune Defic. Syndr., 2017, 74, e45–e53 CrossRef CAS PubMed.
- D. R. Jones, Z. Wu, D. Chauhan, K. C. Anderson and J. Peng, A Nano Ultra-Performance Liquid Chromatography − High Resolution Mass Spectrometry Approach for
Global Metabolomic Profiling and Case Study on Drug-Resistant Multiple Myeloma, Anal. Chem., 2014, 86, 3667–3675 CrossRef CAS PubMed.
- Z. Li, J. Tatlay and L. Li, Nanoflow LC-MS for High-Performance Chemical Isotope Labeling Quantitative Metabolomics, Anal. Chem., 2015, 87, 11468–11474 CrossRef CAS PubMed.
- A. J. Chetwynd, L. A. Ogilvie, J. Nzakizwanayo, F. Pazdirek, J. Hoch, C. Dedi, D. Gilbert, A. Abdul-Sada, B. V. Jones and E. M. Hill, The potential of nanoflow liquid chromatography-nano electrospray ionisation-mass spectrometry for global profiling the faecal metabolome, J. Chromatogr. A, 2019, 1600(30), 127–136 CrossRef CAS PubMed.
- A. J. Chetwynd, A. David, E. M. Hill and A. Abdul-Sada, Evaluation of analytical performance and reliability of direct nanoLC-nanoESI-high resolution mass spectrometry for profiling the (xeno)metabolome, J. Mass Spectrom., 2014, 49, 1063–1069 CrossRef CAS PubMed.
- A. J. Chetwynd, A. Abdul-Sada and E. M. Hill, Solid-phase extraction and nanoflow liquid chromatography-nanoelectrospray ionization mass spectrometry for improved global urine metabolomics, Anal. Chem., 2015, 87, 1158–1165 CrossRef CAS PubMed.
- A. J. Chetwynd and A. David, A review of nanoscale LC-ESI for metabolomics and its potential to enhance the metabolome coverage, Talanta, 2018, 182, 380–390 CrossRef CAS PubMed.
- W. B. Dunn, N. J. C. Bailey and H. E. Johnson, Measuring the metabolome: current analytical technologies, Analyst, 2005, 130, 606 RSC.
- E. M. Lenz and I. D. Wilson, Analytical strategies in metabonomics, J. Proteome Res., 2007, 6, 443–458 CrossRef CAS PubMed.
- D. J. Beale, F. R. Pinu, K. A. Kouremenos, M. M. Poojary, V. K. Narayana, B. A. Boughton, K. Kanojia, S. Dayalan, O. A. H. Jones and D. A. Dias, Metabolomics, 2018, 14, 152 CrossRef.
- N. Schauer, D. Steinhauser, S. Strelkov, D. Schomburg, G. Allison, T. Moritz, K. Lundgren, U. Roessner-Tunali, M. G. Forbes, L. Willmitzer, A. R. Fernie and J. Kopka, GC-MS libraries for the rapid identification of metabolites in complex biological samples, FEBS Lett., 2005, 579, 1332–1337 CrossRef CAS PubMed.
- K. Faserl, A. J. Chetwynd, I. Lynch, J. A. Thorn and H. H. Lindner, Corona Isolation Method Matters: Capillary Electrophoresis Mass Spectrometry Based Comparison of Protein Corona Compositions Following On-Particle versus In-Solution or In-Gel Digestion, Nanomaterials, 2019, 9, 898 CrossRef CAS PubMed.
- M. C. Gulersonmez, S. Lock, T. Hankemeier and R. Ramautar, Sheathless capillary electrophoresis-mass spectrometry for anionic metabolic profiling, Electrophoresis, 2016, 37, 1007–1014 CrossRef CAS PubMed.
- W. Zhang, T. Hankemeier and R. Ramautar, Next-generation capillary electrophoresis–mass spectrometry approaches in metabolomics, Curr. Opin. Biotechnol., 2017, 43, 1–7 CrossRef CAS PubMed.
- R. Ramautar, G. W. Somsen and G. J. de Jong, CE-MS in metabolomics, Electrophoresis, 2009, 30, 276–291 CrossRef CAS PubMed.
- M. van Mever, T. Hankemeier and R. Ramautar, CE-MS for anionic metabolic profiling: an overview of methodological developments, Electrophoresis, 2019, 40(18–19), 2349–2359 CAS.
- R. Ramautar, G. W. Somsen and G. J. de Jong, CE-MS for metabolomics: Developments and applications in the period 2016–2018, Electrophoresis, 2019, 40, 165–179 CrossRef CAS PubMed.
- W. Zhang, M. C. Gulersonmez, T. Hankemeier and R. Ramautar, Sheathless Capillary Electrophoresis-Mass Spectrometry for Metabolic Profiling of Biological Samples, J. Visualized Exp., 2016, 116, e54535 Search PubMed.
- S. Moco, J. Vervoort, S. Moco, R. J. Bino, R. C. H. De Vos and R. Bino, Metabolomics technologies and metabolite identification, TrAC, Trends Anal. Chem., 2007, 26, 855–866 CrossRef CAS.
- B. Jiménez, E. Holmes, C. Heude, R. F. Tolson, N. Harvey, S. L. Lodge, A. J. Chetwynd, C. Cannet, F. Fang, J. T. M. Pearce, M. R. Lewis, M. R. Viant, J. C. Lindon, M. Spraul, H. Schäfer and J. K. Nicholson, Quantitative Lipoprotein Subclass and Low Molecular Weight Metabolite Analysis in Human Serum and Plasma by 1H NMR Spectroscopy in a Multilaboratory Trial, Anal. Chem., 2018, 90, 11962–11971 CrossRef PubMed.
- M. Carril, D. Padro, P. Del Pino, C. Carrillo-Carrion, M. Gallego and W. J. Parak, In situ detection of the protein corona in complex environments, Nat. Commun., 2017, 8, 1542 CrossRef PubMed.
- E. Hellstrand, I. Lynch, A. Andersson, T. Drakenberg, B. Dahlbäck, K. A. Dawson, S. Linse and T. Cedervall, Complete high-density lipoproteins in nanoparticle corona, FEBS J., 2009, 276, 3372–3381 CrossRef CAS PubMed.
- M. Lundqvist, I. Sethson and B. H. Jonsson, High-resolution 2D 1H-15N NMR characterization of persistent structural alterations of proteins induced by interactions with silica nanoparticles, Langmuir, 2005, 21, 5974–5979 CrossRef CAS PubMed.
- A. Vignoli, V. Ghini, G. Meoni, C. Licari, P. G. Takis, L. Tenori, P. Turano and C. Luchinat, Angew. Chem., Int. Ed., 2019, 58, 968–994 CrossRef CAS PubMed.
- K. Bingol, High-Throughput, 2018, 7, 9 CrossRef CAS PubMed.
- J. Chong and J. Xia, MetaboAnalystR: an R package for flexible and reproducible analysis of metabolomics data, Bioinformatics, 2018, 34, 4313–4314 CrossRef CAS PubMed.
- A. Vaniya and O. Fiehn, Using fragmentation trees and mass spectral trees for identifying unknown compounds in metabolomics, TrAC, Trends Anal. Chem., 2015, 69, 52–61 CrossRef CAS PubMed.
- C. A. Smith, E. J. Want, G. O'Maille, R. Abagyan and G. Siuzdak, XCMS: Processing mass spectrometry data for metabolite profiling using nonlinear peak alignment, matching, and identification, Anal. Chem., 2006, 78, 779–787 CrossRef CAS PubMed.
- M. Katajamaa, J. Miettinen and M. Orešič, MZmine: Toolbox for processing and visualization of mass spectrometry based molecular profile data, Bioinformatics, 2006, 22, 634–636 CrossRef CAS PubMed.
- J. Xia, I. V. Sinelnikov, B. Han and D. S. Wishart, MetaboAnalyst 3.0-making metabolomics more meaningful, Nucleic Acids Res., 2015, 43, W251–W257 CrossRef CAS PubMed.
- R. L. Davidson, R. J. M. Weber, H. Liu, A. Sharma-Oates and M. R. Viant, GigaScience, 2016, 5, 10 CrossRef.
- S. Wiklund, E. Johansson, L. Sjöström, E. J. Mellerowicz, U. Edlund, J. P. Shockcor, J. Gottfries, T. Moritz and J. Trygg, Visualization of GC/TOF-MS-based metabolomics data for identification of biochemically interesting compounds using OPLS class models, Anal. Chem., 2008, 80, 115–122 CrossRef CAS PubMed.
- B. Worley and R. Powers, Multivariate Analysis in Metabolomics, Curr. Metabolomics, 2012, 1, 92–107 Search PubMed.
- J. Martel, C. Y. Wu, C. Y. Hung, T. Y. Wong, A. J. Cheng, M. L. Cheng, M. S. Shiao and J. D. Young, Fatty acids and small organic compounds bind to mineralo-organic nanoparticles derived from human body fluids as revealed by metabolomic analysis, Nanoscale, 2016, 8, 5537–5545 RSC.
- B. Zhang, M. Xie, L. Bruschweiler-Li, K. Bingol and R. Brüschweiler, Use of Charged Nanoparticles in NMR-Based Metabolomics for Spectral Simplification and Improved Metabolite Identification, Anal. Chem., 2015, 87, 7211–7217 CrossRef CAS PubMed.
- A. D. Southam, R. J. M. Weber, J. Engel, M. R. Jones and M. R. Viant, A complete workflow for high-resolution spectral-stitching nanoelectrospray direct-infusion mass-spectrometry-based metabolomics and lipidomics, Nat. Protoc., 2017, 12, 255–273 CrossRef CAS PubMed.
- S. M. Briffa, F. Nasser, E. Valsami-Jones and I. Lynch, Uptake and impacts of polyvinylpyrrolidone (PVP) capped metal oxide nanoparticles on Daphnia magna: role of core composition and acquired corona, Environ. Sci.: Nano, 2018, 5, 1745–1756 RSC.
- C. D. Walkey, J. B. Olsen, F. Song, R. Liu, H. Guo, D. W. H. Olsen, Y. Cohen, A. Emili and W. C. W. Chan, Protein corona fingerprinting predicts the cellular interaction of gold and silver nanoparticles, ACS Nano, 2014, 8, 3439–3455 CrossRef PubMed.
- A. Afantitis, G. Melagraki, A. Tsoumanis, E. Valsami-Jones and I. Lynch, A nanoinformatics decision support tool for the virtual screening of gold nanoparticle cellular association using protein corona fingerprints, Nanotoxicology, 2018, 12, 1148–1165 CrossRef CAS PubMed.
- G. Liu, Y. Zhao, A. Angeles, L. L. Hamuro, M. E. Arnold and J. X. Shen, A novel and cost effective method of removing excess albumin from plasma/serum samples and its impacts on LC-MS/MS bioanalysis of therapeutic proteins, Anal. Chem., 2014, 86, 8336–8343 CrossRef CAS PubMed.
|
This journal is © The Royal Society of Chemistry 2020 |
Click here to see how this site uses Cookies. View our privacy policy here.