Natural estrogens in surface waters of a catchment with intensive livestock farming in Switzerland†
Received
22nd July 2020
, Accepted 1st October 2020
First published on 2nd October 2020
Abstract
Natural estrogens such as 17α-estradiol (E2α), 17β-estradiol (E2β), estrone (E1), and estriol (E3), released to surface waters from both urban and agricultural sources, are endocrine disrupting for fish. Here, we assess the prevalence of livestock farming derived natural estrogens in tributaries and ponds in the agriculturally dominated catchment of Lake Baldegg, Switzerland. Passive samplers were deployed in the main tributary and daily time-proportional water samples were collected in five tributaries for 30 days at the beginning of the vegetation period. Furthermore, we took grab samples of 12 ponds in the catchment. Aqueous samples were liquid–liquid extracted, derivatized, and analysed with LC-MS/MS and stream water samples additionally with ERα-CALUX, a bioassay for assessing total estrogenic activity. Natural estrogens were regularly detected, with mean concentrations ranging from below the limit of detection to 0.55 ng L−1 for E2β and E1, respectively, and passive sampling and bioassay results largely confirmed these findings. Monte Carlo simulated mean natural estrogen concentrations underestimated measured ones by a factor of three to 11. An agricultural area's hydrological contribution and connectivity to surface waters seemed to be more important for the development of estrogen concentrations in streams than livestock densities in a catchment or the actual loads of slurry applied. Pond water occasionally contained natural estrogens in concentrations up to 8.6 ng L−1 for E2α. The environmental quality standards of the European Union (0.4 ng L−1 for E2β and 3.6 ng L−1 for E1) were never exceeded for longer than a day in tributaries, but E1 reached critical concentrations for aquatic organisms in ponds.
Environmental significance
Although exposure to elevated estrogen concentrations impacts fish, agriculture's contribution to the prevalence of estrogens in surface waters remains largely unknown. Combining different sampling approaches with biological and chemical assessment methods allowed us to monitor estrogens in small to medium-sized streams and ponds within an agricultural catchment. In streams, natural estrogen concentrations never reached critical concentrations for more than a day. In small ponds, however, we found natural estrogens in concentrations that may exert endocrine disrupting effects in aquatic organisms. In conclusion, agriculture is a source for natural estrogens in surface waters. In ponds, as well as in streams additionally impacted by wastewater treatment plant effluents, slurry-derived estrogens occasionally contribute to estrogen concentrations above EU environmental quality standards.
|
1. Introduction
Steroid hormones such as the natural estrogens 17α-estradiol (E2α), 17β-estradiol (E2 β), estrone (E1), and estriol (E3) impair reproduction and development of aquatic organisms.1,2 Agriculture may be a substantial source for natural estrogens in freshwaters.3 Slurry application on soil was found to release natural estrogens to surface waters.4–6 At the individual field scale, slurry derived estrogens were emitted to tile drains mainly through preferential flow after rain events and the export dynamics were comparable to those of dissolved phosphorus from slurry.6,7 To establish a link between natural estrogens in surface waters and agriculture, streams located in catchments with intensive livestock farming in the US8–10 and New Zealand11 or downstream and in close vicinity of farms12 or grazing areas13 were monitored. In these studies, water samples were taken per sampling site once or twice a year by collecting only grab samples or by combining grab sampling with passive polar organic chemical integrative samplers (POCIS) installed in streams.8,12 Increased estrogenic activity was reported for streams within agriculturally dominated catchments in the US,8–10 and downstream of farms12 and grazing areas.13 In contrast, in New Zealand surface waters natural estrogens were not found in concentrations above the EU environmental quality standards (EU EQS).11 Among the factors that might influence differences in monitoring data are variations in agricultural practices, catchment characteristics, sampling methods, frequencies, and periods, properties of surface waters, stability of targeted compounds, and analytical methods used. Furthermore, estrogens were quantified in stagnant water bodies,14 such as ponds, where dissolved oxygen availability is lower15 and hydrodynamics differ from those in running waters. Such differences might impact natural estrogen composition and concentrations in ponds.
In Switzerland, 55% of the agricultural area is potentially connected to surface waters, and 13% have a high connectivity.16 Consequently, slurry-derived natural estrogens are a potential risk for aquatic organisms. Unlike North America, where most of the previous surface water monitoring campaigns were conducted, slurry is rarely stored in slurry lagoons17 and administration of estrogens to livestock animals for growth promotion is forbidden in Europe.18 These factors might substantially influence the amount of estrogens emitted to the environment. Swiss regulation stipulates that slurry application is only allowed during the vegetation period. Therefore, slurry applications peak at the beginning of the vegetation period around March – the time when most Swiss fresh water fish species as well as amphibians hatch and are in early development.19,20 Whereas elevated estrogen concentrations in Swiss surface waters were attributed to wastewater treatment plant (WWTP) effluents,21,22 the contribution of agriculture remains unknown.
The aim of this study was to get for the first time a detailed picture about estrogen occurrence in streams from catchments dominated almost exclusively by intensive livestock farming in Europe. Samples were collected daily time-proportionally over a month during a period of frequent slurry applications and discharge was measured at the sampling sites. Opposite to most former studies, this sampling approach enabled us to quantify representative estrogen concentrations and emitted loads. Simultaneously, phosphorus was determined in stream water samples, which allowed comparing estrogen and phosphorus export dynamics at catchment scale to those observed at individual field scale in another study.6 For confirmation, we resorted to additional orthogonal chemical and biological methods to analyse the collected water samples. In concert, it was possible to evaluate long-term exposure of aquatic organisms to potential estrogenic pollution. In contrast to former studies, detailed agricultural, land use related, and hydrological data of the catchment and the experimental set-up allowed us to predict the mean monthly estrogen concentrations in streams with a Monte Carlo simulation and to estimate the agricultural contribution to the total natural estrogen load in streams at catchment scale.
The prevalence of natural estrogens in ponds in an agriculturally dominated landscape was assessed for the first time in this study. So far, the land use around stagnant water bodies was not included in the interpretation of the results. Ponds are of particular interest because they are the natural habitat of around 80% of the Swiss amphibians,23 another class of aquatic organisms susceptible to estrogenic pollution.24–26
2. Materials and methods
2.1 Study area
The study catchment of Lake Baldegg is dominated by intensive agriculture (2.4 livestock units (LSU) per hectare, Swiss average: 1.2 LSU ha−1).27 Phosphorus eutrophication of surface waters by slurries from intensive agriculture has been a problem for decades in this catchment.28 Current legislation allows slurry applications only during the vegetation period.20 Consequently, slurry-derived natural estrogens in streams are most likely to occur in the vegetation period and are probably highest in spring, when slurry applications are intensified. Therefore, the monitoring campaigns were conducted from February to April. Tributaries were monitored by passive sampling and daily time-proportional water samples. Grab samples were taken from ponds (Fig. 1).
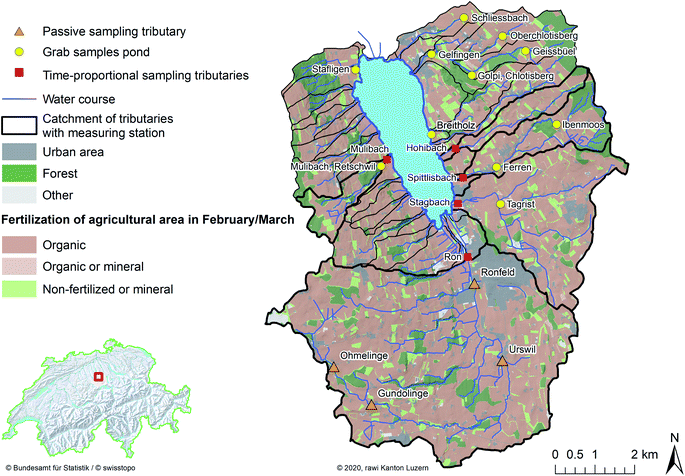 |
| Fig. 1 Map of the Lake Baldegg catchment (red empty square in bottom left map of Switzerland), and surface water sample locations. Passive samplers were deployed along the river Ron (orange triangles). Daily time-proportional water samples were collected from five Lake Baldegg tributaries (red squares). Grab samples were taken from ponds (yellow circles). Agricultural area was subdivided in cultures that were fertilized organically (dark brown), organically or mineral (light brown), or non-fertilized or mineral (light green) at the beginning of the vegetation period and were 62.4%, 0.3%, and 9.9% of the total Lake Baldegg catchment area, respectively. The sampling sites and land use in the catchment (Source: “© 2020, rawi Kanton Luzern”) were joined to landscape models, which contained administrative units, national boundaries and natural features such as watercourses and lake contours of Switzerland (Source: “Swiss Federal Office of Topography”)33 and to topographical catchment areas of Swiss surface waters (Source: “Federal Office for the Environment”).71 | |
At sampling sites along Lake Baldegg tributaries (passive sampling and time-proportional sampling), we can reasonably assume that most estrogens were agriculturally derived due to the following reasons: (1) by law, human wastewater has to be treated in WWTPs. An exception are remotely located farms. For them it is allowed to collect their domestic wastewater in the slurry pits connected to the farm.20 However, the human derived estrogen loads in slurry pits are marginal compared to the slurry volumes produced by livestock animals. (2) Tributaries upstream to the sampling sites were free of WWTP effluents, and (3) the application of sewage sludge (biosolids) as agricultural fertilizer is prohibited in Switzerland since 2006.29 Hence, we can exclude this input source of human estrogens in our study.
2.2 Sampling
2.2.1 Passive sampling.
Triplicates of passive samplers (Empore™ SPE Disks, SDB-RPS, Sigma – Aldrich Buchs, Switzerland) per sampling site were deployed at four sites along the river Ron, the 17 km long main tributary to Lake Baldegg: Ohmelinge (at 0.63 km distance from spring), Gundolinge (at 2.17 km), Urswil (at 7.17 km), and Ronfeld (at 9.17 km) (Fig. 1 (orange triangles), Table S5†). They were installed during two five-day periods: February 09 to February 13, 2018 (before the beginning of the vegetation period, when slurry application was prohibited), and March 22 to March 26, 2018 (after the beginning of the vegetation period, when slurry applications started). Passive samplers were conditioned and prepared according to Vermeirssen et al.30 Passive sampler conditioning and calibration is described in detail in the ESI, Chapter S2.1.1.† Sampling rates for calibrated compounds were 0.66 L d−1 for E2β and 0.68 L d−1 for E1. For E2α, we applied the same sampling rate as for E2β, i.e., 0.66 L d−1. The sampling rate of E3 was approximated by those of E2β and E1, and set at 0.67 L d−1 (ESI, Chapter S2.1.1†).
2.2.2 Time-proportional sampling.
We monitored five Lake Baldegg tributaries (starting from the tributary with the highest livestock density in its catchment in clockwise direction: Spittlisbach, Stagbach, Ron, Mulibach, and Hohibach (Fig. 1 (red squares), Table S6†)) daily from February 17 to March 18, 2019. The tributaries Spittlisbach, Stagbach, Mulibach, and Hohibach do not receive any regular WWTP effluents at all and for the Ron, the sampling site was located upstream of the WWTP discharge at Hochdorf. However, wastewater treatment overflows are connected to the rivers Ron and Stagbach. They are occasional sources for wastewater-derived natural and synthetic estrogens in these two tributaries, but agriculture remained the dominating estrogen source. Time-proportional water samples were collected over 24 h, using a submerged sampler (3 L volume) equipped with a regulator for continuous hydrostatic air pressure release from the sampler chamber to control the water sampling rate (Fig. S3†).31 For chemical and biological estrogen analysis, two subsamples (of approximately 200 mL) of stream water in the sampler were transferred into two 300 mL aluminum bottles (all used bottles were pure aluminium with a polypropylene screw cap with internal aluminium seal from Bürkle, Bad Bellingen, Germany). For phosphorous analysis, an aliquot of 500 mL stream water from the sampler was filled into a glass bottle. All samples were cooled during transport to the laboratory. Chemical estrogen analysis was performed immediately and subsamples for biological estrogen analysis were frozen at −20 °C. The stream water subsamples for phosphorus analysis were cooled at 4 °C and analysed within three days. The daily mean stream water discharge was measured at the sampling sites by the Canton of Lucerne.32
2.2.3 Pond sampling.
The occurrence of natural estrogens in 12 ponds located in the Lake Baldegg catchment (Fig. 1 (yellow circles)) was studied from March 25 to April 15, 2019. All ponds were at least partially surrounded by agricultural area. Three grab samples (1 L each) were taken at the inflow, the center and the outflow of each pond approximately 0.5 m below the water surface, using a plastic bucket attached to a telescopic pole. In absence of inflow or outflow, we took samples at two opposite peripheral locations and the center of the pond. For sampling of larger ponds, a rubber dinghy was used. The grab samples were combined in a 5 L plastic container and an aliquot (800 mL) was filled into a 1 L aluminum bottle. By repeating this procedure three times within one hour, triplicates were obtained for every pond. Furthermore, one pond was sampled weekly over a period of four weeks. The pond water samples were treated like stream water samples. The surface area of each pond was approximated by an ellipse. Ellipse radii, estimated with pond diameters, were extracted from a publicly available landscape model.33 Based on physical inspection during sampling, an average pond depth of 1.5 m was assumed to approximate the pond volume.
2.3 Chemical analyses
2.3.1 Chemicals.
17α-Estradiol-16,16,17-d3 (E2α-d3) was purchased from BOC Sciences (Shirley, USA). Estriol-3,16,17-d3 (E3-d3) was obtained from Toronto Research Chemicals (North York, Canada). Furthermore, E2α, E2β, 17β-estradiol-16,16,17-d3 (E2β-d3), E1, estrone-2,4,16,16-d4 (E1-d4), and E3, all in 1000 μg mL−1 methanol, were bought from A2S (Saint Jean d'Illac, France). A list of further chemicals used for chemical analysis is given in the ESI, Chapter S2.2.1.†
2.3.2 Natural estrogens: extraction from passive samplers.
Natural estrogens were extracted from Empore™ SDB-RPS disks analogous to Vermeirssen et al.34 After recollection from the field, they were placed in 8 mL vials filled with acetone (7 mL). In the laboratory, an aliquot of 0.1 ng of deuterated internal standard (100 μL of 1000 ng L−1 internal standard mixture of E2α-d3, E2β-d3, E1-d4, and E3-d3 in acetonitrile, 100 ng L−1 final concentration) was added to every sample and they were shaken for 30 min on an orbital shaker (100 rpm). Afterwards, the acetone extract was transferred to a new vial. The extraction of Empore™ SDB-RPS disks was repeated with methanol (7 mL). The acetone extract was evaporated to approximately 1 mL with N2, combined with the methanol extract and filtered with 0.45 μm, 25 mm diameter polytetrafluoroethylene (PTFE) filters (BGB Analytik, Boeckten, Switzerland). Prior to derivatization, the extracts were entirely evaporated to dryness with N2.
2.3.3 Natural estrogens: extraction from stream and pond water.
Natural estrogens in surface water samples were extracted as outlined by Backe35 with slight modifications. Surface water (30 mL) was filled into a 50 mL borosilicate vial, 12 cm high and 2.5 cm in diameter (Supelco, Sigma-Aldrich, Buchs, Switzerland). Sodium chloride (0.5 g) and 0.02 ng of deuterated internal standard (100 μL of 200 ng L−1 internal standard mixture of E2α-d3, E2β-d3, E1-d4, and E3-d3 in acetonitrile, 20 ng L−1 final concentration) were added to every sample. Target analytes were first extracted with 8 mL of 2
:
5 methanol
:
MTBE and mixed for 2 min on an orbital shaker (225 rpm). Subsequently, the supernatant was transferred to a borosilicate vial with a Pasteur pipette. Each surface water sample was liquid–liquid extracted for a second time with 4 mL 2
:
5 methanol
:
MTBE. Lastly, the two extracts were combined, evaporated to complete dryness with N2 and derivatized.
2.3.4 Natural estrogens: derivatization and analysis.
Target analytes in passive sampler and surface water extracts were derivatized with dansyl chloride and analysed with liquid chromatography coupled to a triple-quadrupole mass spectrometer as described in Rechsteiner et al.36 Internal standard calibration was used to quantify natural estrogens. Data processing was conducted with Agilent MassHunter QQQ Quantitative Analysis program version B.09.00.
2.3.5 Natural estrogens: method validation and quality assurance and control.
A detailed description of the determination of analytes' limits of detection (LOD), limits of quantification (LOQ), ion suppressions, instrument and method precisions, and absolute and relative recoveries is given in the ESI, Chapter S2.2.2.† Resulting values for these quality assurance and quality control parameters for surface waters were: limits of detection were ≤0.11 ng L−1 for all natural estrogens (LOD: E2α = 0.11 ng L−1, E2β = 0.10 ng L−1, E1 = 0.07 ng L−1 and E3 = 0.08 ng L−1); all natural estrogens were quantifiable above 0.36 ng L−1 (LOQ: E2α = 0.36 ng L−1, E2β = 0.34 ng L−1, E1 = 0.24 ng L−1 and E3 = 0.25 ng L−1); ion suppressions for natural estrogens in surface water ranged from −6 to 2% (ion suppression: E2α = −3%, E2β = 2%, E1 = −6% and E3 = −1%). Absolute and relative recoveries were above 80% for all substances (absolute recovery: E2α = 99%, E2β = 96%, E1 = 122%, and E3 = 100%; relative recovery: E2α = 125%, E2β = 100%, E1 = 109%, and E3 = 100%). Method precision ranged from 2 to 8% (E2α = 8%, E2β = 4%, E1 = 2%, and E3 = 5%). Instrument precision was for all target analytes ≤6% (E2α = 6%, E2β = 3%, E1 = 2%, and E3 = 4%). For passive samplers the LOQ were 0.01 ng L−1, 0.01 ng L−1, 0.02 ng L−1, and 0.01 ng L−1 for E2α, E2β, E1, and E3, respectively.
Stability of estrogens and potential contamination during sampling, storage and analysis were tested as explained in detail in the ESI, Chapter S2.2.3.† Concentration changes remained within method precision when stream water samples were stored at −20 °C for four weeks (Fig. S4†), but altered in percentages that were slightly above method precision during transport of the sample from the field to the laboratory (E2α = −9%, E2β = −7%, E1 = +9%, E3 = −8%). Short half-lives of estrogens in river water were reported previously (t1/2 = 0.2–9 d at 20 °C).37 No estrogen contamination occurred in field blanks and sampler blanks. A blank was produced for every extraction batch, i.e., a set of five or ten aqueous samples. In one of 20 extraction batches a contamination of 0.25 ng L−1 E1 was recorded. The concerned batch was rejected and reanalyzed.
2.3.6 Phosphorus
Phosphorus was photometrically determined with ammonium molybdate according to DIN EN ISO 6878, 2004 at Eawag, Switzerland. The method is described in the ESI, Chapter S2.2.4.†
2.4 Biological analysis using ERα-CALUX
17β-Estradiol equivalent concentrations (EEQbio) were determined with the in vitro ERα-CALUX bioassay in a subset of time-proportional water samples from Lake Baldegg tributaries (138 of the 150 samples). We decided for ERα-CALUX because it showed the best precision and repeatability among the commonly used estrogen receptor transactivation assays (YES, ERα-CALUX, MELN, T47D-Kbluc, ERα-GeneBLAzer).38 Furthermore, it is well suited for sensitive detection of estrogenic activities in native water samples, i.e., direct testing without filtration and/or enrichment, following a standardized protocol (ISO19040:3).39 Frozen water samples from the time-proportional sampling were thawed and subsamples filled into 8 mL glass vials. These were brought to Eawag, where ERα-CALUX was conducted with a 2.5 mL aliquot. The total measured estrogenic activity was expressed as ng L−1 EEQbio. An LOQ of 0.50 ng EEQ per L was previously determined for native water samples in ERα-CALUX, in accordance with the LOQ suggested by the ISO standard protocol.39 Further detailed information on the biological analysis, such as methodological aspects, LOQ determination, validity of the bioassay screening, the relative potency factors used and/or determined in this study, and chemical EEQ (EEQchem) calculations is provided in the ESI, Chapter S2.3.†
2.5 Statistical analyses
Statistical analyses were performed in R 3.6.3.40 Normal distribution of the data was assessed with a Shapiro–Wilk test (function shapiro.test) and by calculating the skewness of the data (function skewness, data was assumed to be normal distributed for −1 < skew value < 1).41 After log-transformation most non-normally distributed variables attained normal distribution (Table S14†). E2α and E2β degrades to E1 and afterwards to E3.42 Since degradation rates remained unknown, the total natural estrogen concentration (Etot) was used as response variable. The logarithm of Etot (“log(Etot)”) was regressed against the hydrological parameters and catchment characteristics as fixed effects with “catchment” and “sampling date” as crossed random effects. The linear mixed-effects model (LMM) was fitted using package lme4. The addition of an autoregressive term for “sampling date” did not significantly improve the model (likelihood ratio test, p-value = 0.18). Hydrological parameters were the logarithmised daily amount of rain (“log(rain)”) and the logarithmised daily discharge (“log(discharge)”) of each tributary (Fig. 3). Estrogen concentrations below LOQ were set to LOD for log-transformation. At days without rain, 0.2 mm of rain (minimal measured amount of rain multiplied by 0.5) was added to conduct a log-transformation. The assessed catchment characteristics were livestock densities of cattle and pig (“cattle” and “pig”) and percentage of organically fertilized (“org. fertilized”), surface water-connected (“SW-connected”), and hydrologically contributing agricultural areas (“hydr. contributing”) in a catchment (ESI, Chapter S1†). The catchment characteristics were included one at the time and their significance was assessed with a likelihood ratio test (function anova) (Table S15†). To calculate Pearson correlation coefficients (r) between total and individual natural estrogen concentrations and phosphorus concentrations in Lake Baldegg tributaries, we used the function cor (Table S16†).
2.6 Estimation of natural estrogen concentrations in tributaries including an uncertainty assessment
The exact location, volume, and time point of slurry applications by the about 280 individual farmers in the catchment during the monitoring campaign remained unknown. Therefore, only the mean natural estrogen concentrations in tributaries (cwater) from February 17 to March 18, 2019 could be estimated with the following equation (eqn (1)): |  | (1) |
where Nanimal is the number of dairy cows and heifers (Nanimal,cattle) and pigs (Nanimal,pig) in every catchment, Vslurry is the volume of slurry produced by one dairy cow or heifer (Vslurry,cattle) or pig (Vslurry,pig), respectively, cslurry is the average natural estrogen concentration in cattle (cslurry,cattle) and pig (cslurry,pig) slurries, EF are the emitted fractions of cattle (EF,cattle) and pig (EF,pig) slurry-derived natural estrogens applied on agricultural area to surface waters, Vdischarge is the cumulative discharge volume for every catchment and Aslurry is the average number of slurry applications per year.
From local authorities, the location and LSU of cattle and pigs were obtained for every farm in the catchment of Lake Baldegg. To derive Nanimal, the LSU of all farms in a tributary catchment were summed up and multiplied with a factor 1 for dairy cows, 0.6 for heifers and 0.45 for pigs (weighted average breeding pig (0.55) and fattening pig (0.17)).43 We assumed Nanimal as constant during the sampling period. Values for cslurry were determined in a previously conducted monitoring campaign (Table S9†).36 The emitted estrogen fraction (EF) was defined as natural estrogen loads recovered in drainage water of a tile-drained agricultural test field divided by the natural estrogen loads applied on an agricultural test field (Rechsteiner et al., submitted, see Table S10†).44 Values for Vslurry and Aslurry were obtained from Richner et al.45
A statistical distribution was attributed to all parameters which entailed uncertainties (Table S11†) and 1000 Monte Carlo simulations of cwater were obtained. The mean values and standard deviations of cwater were determined. Parameter values, and the sensitivity analysis are provided in the ESI, Chapters S2.4 and S3.2.†
3. Results and discussion
3.1 Natural estrogen occurrence as determined by passive sampling
All natural estrogens were detected with SDB-RPS Empore™ disks in the tributary Ron (Fig. 1 (orange triangles)) before (Fig. 2A) and after (Fig. 2B) the beginning of the vegetation period. Time weighted average (TWA) concentrations of all estrogens fluctuated within a range of 0.02 to 0.15 ng L−1 at all sampling sites and both time points, with the exception of Urswil where E1 and E2α reached 0.25 ng L−1 in February 2018 (Fig. 2A) and 0.60 ng L−1 in March 2018 (Fig. 2B), respectively. The sampling site in Urswil is particularly prone to emissions from agriculture, because it receives water from the sub-catchment with the highest fraction of organically fertilized, drained, and hydrologically contributing agricultural areas.46 A wastewater treatment overflow, located between Ohmelinge and Urswil, was never activated during our monitoring campaign. Downstream after Urswil, seven sub-tributaries enter the river Ron, dilute stream water between Urswil and Ronfeld and hence, might have caused lower estrogen concentrations in samples from the latter station.
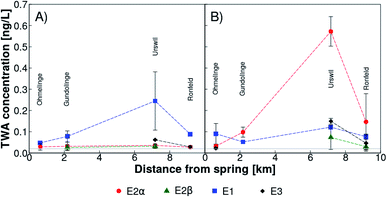 |
| Fig. 2 Time weighted average (TWA) concentrations of 17α-estradiol (E2α, red circles), 17β-estradiol (E2β, green triangles), estrone (E1, blue squares), and estriol (E3, black diamonds) determined with passive samplers (SDB-RPS Empore™ disks) along Lake Baldegg tributary Ron, Switzerland (locations of sampling sites are indicated in Fig. 1) before (February 2018, panel A) and after (March 2018, panel B) the beginning of the vegetation period. The grey horizontal line represents the maximal limit of quantification (LOQ). Values below LOQ were zeroed. | |
In passive samplers installed up- and downstream of livestock farms in the UK, E2β and E1 ranged across all sites from not detected to 0.89 ng L−1 and 0.10 to 4.9 ng L−1, respectively, and estrogenic activity was mostly higher downstreams. Farms were located close to small streams, had a high potential for farmyard run-off and livestock had free access to streams.12 None of this was the case along the tributary Ron. Furthermore, Swiss legislation prohibits slurry applications closer than 3 m from streams.20 Our findings support a previous study which found low estrogenicity in passive samplers installed upstream of a WWTP in a medium-sized Swiss river with an agriculturally dominated catchment.21
3.2 Natural estrogen occurrence as determined by time-proportional water sampling
All natural estrogens were detected in the daily collected time-proportional 24 h samples of Lake Baldegg tributaries (Fig. 3, Table S12†). Estrone was quantified most frequently (73 detects out of 150 samples, i.e., 49%). 17α-Estradiol, E2β, and E3 were above LOQ in 26%, 4%, and 35% of all samples analysed, respectively. The mean concentration of E2α, E1, and E3 ranged from <LOD to 0.55 ng L−1, while it was <LOD for E2β in all tributaries (Table S13†). The mean total estrogen concentration per catchment ranged from 0.51 ng L−1 to 1.1 ng L−1. The highest maximal concentration of E2α was found in the Spittlisbach (10.5 ng L−1, February 19, 2019, Fig. 3A) and of E2β and E1 in the Stagbach (0.61 ng L−1 and 3.5 ng L−1, respectively, February 21, 2019, Fig. 3B, Table S13†). In all tributaries, maximal E3 concentration never exceeded 2.0 ng L−1, which was measured in the Mulibach on March 05, 2019 (Fig. 3D and Table S13†).
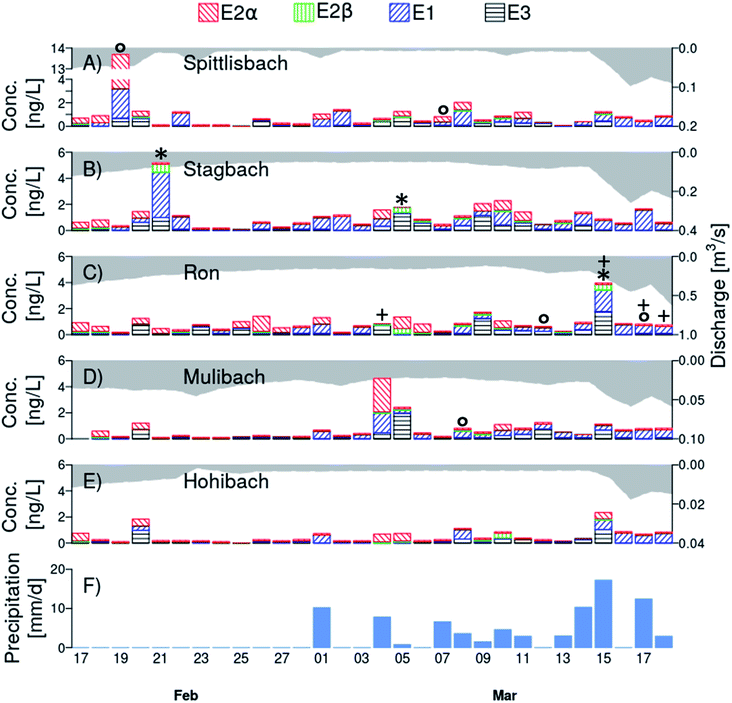 |
| Fig. 3 Daily concentration of 17α-estradiol (E2α, left diagonal red lines), 17β-estradiol (E2β, vertical green lines), estrone (E1, right diagonal blue lines), and estriol (E3, horizontal black lines) in Lake Baldegg tributaries (Spittlisbach (A), Stagbach (B), Ron (C), Mulibach (D), and Hohibach (E); for locations of sampling sites, see Fig. 1) between February 17 and March 18, 2019. Note that the scales on the left and right y-axes differ among tributaries. Discharge of every tributary is indicated in each panel (A)–(E) as grey area. Note that the y-axis of discharge is plotted on the right top down. The daily amount of precipitation in the catchment is shown in panel (F). Wastewater treatment overflows connected to the tributary Ron were active on sampling days marked with a “+“. Water samples in which either chemically or biologically determined 17β-estradiol equivalent concentrations were higher than 0.50 ng L−1 are marked with an “*” or “○“, respectively. Values below limit of detection (LOD) were zeroed. Concentrations between LOD and limit of quantification were set to LOD. | |
Toward the end of the measurement campaign the daily exported E2α loads and the relative contribution of E2α to the total natural estrogen concentration decreased, whereas the relative contribution as well as the daily exported E1 loads increased in most streams (Fig. S8–S10†). Former studies showed that microbes degrade E2β relatively fast to E1 in river water.47 In water-sediment systems, E2α is transformed to E2β, E1, and E3 under aerobic and anaerobic conditions.48 It is likely that initially prevailing E2α in the environment was degraded to E1, explaining their decreasing and increasing fractions, respectively, in tributaries over the time course of the monitoring campaign. No clear pattern in the fraction of E2β and E3 was discernible in neither time nor space.
Comparable to our finding, E1 and E3 were the prevailing estrogens in streams with an agriculturally dominated catchment in the USA and New Zealand.8,9,11 Maximal natural estrogen concentrations measured in streams strongly influenced by agriculture outside of continental Europe were higher (3.4 ng L−1 for E2β to 25 ng L−1 for E2α ng L−1).8–11,13 However, in the mentioned studies, samples were either collected in close proximity to farms12 or less frequent.9 In comparison, EEQs in small Swiss streams ranged from 0.04 ng L−1 to 0.85 ng L−1 downstream of WWTPs,49i.e., higher than in the present study in tributaries largely free of WWTP effluents (over all tributaries: mean EEQchem = 0.06 ng L−1).
3.2.1 Natural estrogen occurrence in light of catchment characteristics.
Hydrological parameters (Fig. 3) and catchment characteristics (Tables S1 and S2, ESI Chapter S1†) were examined for their ability to explain total natural estrogen concentrations in Lake Baldegg tributaries using a LMM. The percentage of surface water-connected (“SW-connected”) or hydrologically contributing (“hydr. contributing”) agricultural areas in the catchment had a significant effect on daily total natural estrogen concentrations (“SW-connected”: p-value = 0.03 and “hydr. contributing”: p-value = 0.02, Table S15†). The LMM with hydrological parameters and the catchment characteristic surface water-connected agricultural areas (“SW-connected”) had the highest R2. It explained 56% of the measured total natural estrogen concentrations in Lake Baldegg tributaries from February 17 to March 18, 2019 (marginal R2 = 0.27, Table S15†). The connectivity (“SW-connected”) of an agricultural area reflects its overland flow distance to surface waters (ESI Chapter S1†).16 The formation of fast flow components, i.e., preferential flow, saturation excess and Hortonian overland flow, of an agricultural area after a rain event is represented by the hydrological contribution of an agricultural area (ESI Chapter S1†).46,50 Other catchment characteristics, such as livestock densities and percentage of organically fertilized agricultural area were unrelated to total daily concentrations of natural estrogens. Consequently, we suggest that for natural estrogen emissions to Lake Baldegg tributaries the location of slurry application was more important than livestock densities in a catchment and the actual amount of slurry applied. However, for a more solid statement about the influence of catchment characteristics on natural estrogen concentrations in surface waters, more observations in catchments with contrasting hydrology and land use would be needed.
3.2.2 Correlation between natural estrogens and phosphorus?.
As slurry applications are a common source for natural estrogens4,7 and phosphorus51,52 in the environment and both are transported through rain-induced preferential flow processes,4,7,53 we hypothesized that phosphorus is a potential tracer for natural estrogens. Not only total estrogen concentrations, but also total phosphorus concentrations were slightly higher after the onset of rain events (Fig. 3F) (mean total phosphorus before and after the beginning of rain events were 17.0 and 27.2 μg L−1, respectively). The date on which slurry application was officially allowed (February 28, 2019) was nearly at the same time as the onset of rain events, which possibly accentuated higher total estrogen and phosphorus concentrations.
However, occasional concurrent peaks of natural estrogens (Fig. 3), total and dissolved phosphorus and orthophosphate (Fig. S11†) were observed in all tributaries even before March 01, 2019, e.g. on February 20 and 21 in Stagbach, Mulibach, and Ron. Additionally, we observed farmers to apply slurry before February 28, 2019. Still, the total phosphorus peaks during days without rain were only in the range of average concentrations for dissolved phosphorus measured in the catchment, meaning that no major slurry accident occurred during our monitoring campaign.46 Therefore, a plausible explanation for natural estrogen concentration peaks during days without rain is that farmers accidentally spilt small amounts of slurry into the tributaries or on shafts directly connected to the tributaries. After a major slurry accident, estrogen as well as phosphorus concentrations in streams are expected to exceed the ones measured here by at least a factor three to five.46
No direct correlation was found for both total and dissolved phosphorus concentrations and total natural estrogen concentrations (r < 0.5 in both cases, Table S16†). Several additional dissolved and/or total phosphorus pools, such as particulates from atmospheric deposition, earlier accumulated phosphorus in soil, or WWTP overflows may have contributed to those from slurry, and thereby override any more direct relationship between phosphorus and natural estrogens in tributaries.54 Therefore, at least at this scale of investigation, similar export dynamics of estrogens and phosphorus observed at individual field scale6 were not confirmed at catchment scale and the hypothesis that phosphorus is a potential tracer for natural estrogens in streams has to be rejected.
3.3 Estimation of natural estrogen concentrations in tributaries including an uncertainty assessment
Monte Carlo simulations allowed us to estimate the mean natural estrogen concentration derived from agriculture in Lake Baldegg tributaries, taking into account possible uncertainties associated with the input parameters (Table S11†). Mean concentrations of E2α, E2β, E1, and E3 were predicted to 0.03 ng L−1, 0.01 ng L−1, 0.03 ng L−1, and 0.07 ng L−1, respectively. These simulated concentrations were, on average, lower than measured mean concentrations by a factor seven for E2α, six for E2β, 11 for E1 and three for E3 (Fig. 4). Estimations based on different slurry application scenarios did not lead to a better convergence of modelled and quantified concentrations. In the catchment of the Mulibach, measured concentrations were underestimated the most. It remains unclear, why the catchment of the Mulibach reacted so differently. Among all catchments it is the steepest, and shows the highest percentage of surface water-connected and the lowest percentage of hydrologically contributing agricultural area in the catchment (Table S2†). Furthermore, the catchment of Mulibach has the highest pig-to-cattle ratio (Table S1†), and the lowest and second lowest number of cattle and pigs housed in a catchment, respectively. Given that measured concentrations were mostly close or below the LOQ, linking measured and estimated concentrations remains challenging. A sensitivity analysis on the parameters demonstrated that the estimation responded the most to a change in natural estrogen concentrations in livestock slurries followed by a change in the fraction of emitted natural estrogens to surface waters (Table S17†).
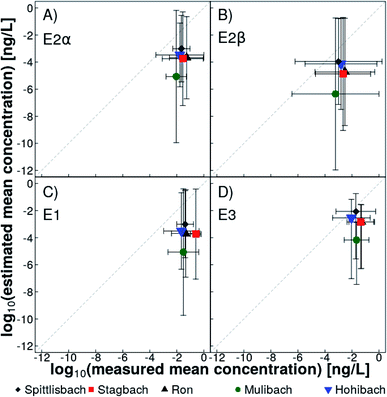 |
| Fig. 4 Estimated logarithmic mean concentrations of 17α-estradiol (E2α, panel A), 17β-estradiol (E2β, panel B), estrone (E1, panel C), and estriol (E3, panel D) plotted against measured logarithmic mean concentrations of E2α, E2β, E1, and E3 in the Lake Baldegg tributaries Spittlisbach (grey diamond), Stagbach (red square) Ron (black triangle), Mulibach (green circle), and Hohibach (blue triangle) from February 17 to March 18, 2019. Error bars represent the logarithmised standard deviation of the estimated and measured mean natural estrogen concentrations in Lake Baldegg tributaries. The 1 : 1 line is illustrated in grey as dotted line. | |
We recognize that the presented model could be improved using more information on the input parameters, for example, if estrogen concentration in slurry of every farm would be determined and every farmer would indicate the exact volume, location and timing of every slurry application. However, this would require a laborious monitoring of agricultural management and a completely different modelling concept. Additionally, emitted estrogen fractions applied in the simulation were determined throughout the year on an experimental test field with one specific soil type and drainage system, while several different ones are present in the catchment of Lake Baldegg. The emitted estrogen fractions in the catchment may differ from those determined on an experimental test field, because of different drainage efficiencies of fields and different pattern of rain events after slurry applications. Furthermore, our estimation ignored degradation of estrogens. The best fit between predicted and measured mean estrogen concentration was observed for E3 (Fig. 4), which is the final still estrogenic degradation product of all other natural estrogens.42 Rain events cause mobilization of natural estrogens through desorption and transport as well as dilution of the estrogen concentrations in surface waters. In the monitored tributaries, mobilization of natural estrogens to surface waters after rain events was only partially compensated by increasing discharge volumes, which was reflected in elevated estrogen concentrations after rain events (Fig. 3). The presented estimation accounted only for dilution, but not for mobilization, i.e., higher cumulative discharge volumes resulted in lower predicted estrogen concentrations in surface waters. A more sophisticated modelling approach including hydrodynamics and a correction term for estrogen mobilization could lead to a more accurate estimation of mean natural estrogen concentrations.
3.4 Comparison of chemically and biologically determined 17β-estradiol equivalent concentrations
To investigate if our chemical analytical method (i.e., LLE, derivatization and LC-MS/MS) possibly missed substances that cause estrogenicity, we additionally analysed samples with a bioassay. In 130 out of 138 analysed time-proportionally collected tributary water samples, i.e., 94% of the samples, EEQchem and EEQbio were below 0.50 ng L−1 (Table S12†), i.e., the LOQ of the latter.
For three samples, EEQchem were equal or above 0.50 ng L−1, while EEQbio were below 0.50 ng L−1 (February 21 in the Stagbach, March 05 in the Stagbach, March 15 in the Ron) (Fig. 3 marked with an “*”, Table S12†). The EEQchem of these samples ranged from 0.50 ng L−1 to 0.73 ng L−1, and thus were close to the LOQ of ERα-CALUX. Measuring near the LOQ becomes challenging for both chemical and biological analysis methods. Furthermore, the mismatch was observed in samples with the highest chemically determined E2β concentrations. One possible reason for the mismatch may be loss of E2β during storage. In stability experiments, where samples were stored for four weeks at −20 °C, we did not observe a degradation of E2β (Fig. S4†). Whereas water subsamples for the chemical analysis were extracted mostly immediately after sample collection, water subsamples for ERα-CALUX bioassays were stored at −20 °C and left at room temperature for 12 h prior to analysis. During the latter necessary equilibration to room temperature and transport prior to ERα-CALUX some degradation of E2β to less potent E1 might have occurred, as observed during transport from the field to the laboratory (ESI, Chapter S2.2.3†).
In five samples, the EEQchem was lower than the EEQbio (February 19 in the Spittlisbach, March 07 in the Spittlisbach, March 08 in the Mulibach, March 12 in the Ron, March 17 in the Ron) (Fig. 3 marked with an “○”, Table S12†). We can offer three possible reasons for the observed differences. Firstly, the focus of this study was on natural estrogens. Therefore, 17α-ethinylestradiol (EE2) – a typical wastewater contaminant – was not quantified and not included in EEQchem calculations. As a wastewater treatment overflow discharges into the river Ron, we speculate that increased estrogenicity potentially originated from wastewater-derived EE2, which was either directly discharged into tributaries (Ron, March 17, 2019), or resuspended from sediments.55 Secondly, phytoestrogens in surface waters might contribute to the total estrogenicity of the water samples.56 In particular, phytoestrogens derived from clover on grassland57,58 and soybeans in livestock fodder59,60 may be of relevance for the catchment of Lake Baldegg. High concentrations of equol are contained in slurry and hence, punctual peaks in streams caused by slurry applications are plausible. However, phytoestrogen emissions from clover on grassland are assumed to be more continuous.61 Thirdly, sample contamination during decanting and transport of the water samples before ERα-CALUX analysis cannot be entirely excluded.
Nonetheless, the determination of EEQbio in non-extracted water samples with ERα-CALUX confirmed that during our monitoring campaign in February and March 2019 in all tributaries, EEQbio exceeding the E2β EU EQS for chronic exposition (0.4 ng L−1)62 never lasted longer than a day and only occurred in very few samples (5 out of 138 samples). 17β-Estradiol equivalent concentrations determined in the T47D-KBluc assay (estrogen receptor transactivation assay using mammalian breast cancer cells) in samples of US streams associated with livestock operations ranged from non-detected to 4.6 ng L−1.8 In our study, maximal determined EEQbio in water samples of Lake Baldegg tributaries (river Ron on March 12, 2019: EEQbio = 2.0 ng L−1) was within this range.
3.5 Comparison of livestock and human derived natural estrogens in the Ron catchment
So far, the contribution of agriculturally derived natural estrogens to the total load in streams with mixed agricultural and human sources in the catchment has not been elucidated. Since E2α is not produced by humans,63 a direct comparison of agricultural and human contributions is only possible for E2β, E1, and E3. Moreover, such a comparison is in the present case restricted to the tributary Ron, which is the only catchment that receives natural estrogens form both sources. The WWTP of Hochdorf with close to 10
000 inhabitants27 discharges into the tributary Ron after our time-proportional sampling site. Even though no time-proportional samples could be collected downstream, literature data allowed to estimate estrogen concentrations downstream of the WWTP. As the WWTP has no advanced treatment step such as ozonation or activated carbon, we assumed the same removal rates as previously determined in a conventional Swiss WWTP, namely 91% for E2β, 58% for E1, and 75% for E3.64 Referring to Arlos et al.65 and Kostich et al.,66 we calculated that the population of Hochdorf emits 1.8 g of E2β, 2.7 g of E1, and 18.7 g of E3 in 30 days. We estimated that within a month, approximately 0.6 g of E2β, 0.7 g of E1, and 1.5 g of E3 are introduced to the environment through slurry applications in the Ron catchment (for details see Chapter 2.6 and ESI, Chapter S2.4†). As a result, livestock farming contributes to 25%, 21%, and 7% of the total E2β, E1, and E3 load in the Ron catchment, respectively. Dividing the estrogen load emitted from the WWTP of Hochdorf by the cumulative discharge volume of the tributary Ron, results in mean human E2β, E1, and E3 concentrations in the Ron of 0.30 ng L−1, 2.0 ng L−1, and 8.2 ng L−1, respectively. During our sampling campaign, the mean measured agriculturally derived concentration were 0.06 ng L−1 for E2β, 0.27 ng L−1 for E1, and 0.27 ng L−1 for E3 in the Ron (Table S13†). Consequently, only around 17% of the total E2β, 12% of the total E1, and 3% of the total E3 concentrations in the Ron were agriculturally derived from February 17 to March 18, 2019. Nevertheless, as slurry application, degradation rates, and discharge volumes vary throughout the year, the agricultural contribution to the total E2β, E1, and E3 loads and concentrations in the environment is not stable. Combined human and agricultural mean natural estrogen concentrations in Ron were 1.1 ng L−1 EEQchem (for calculations see ESI, Chapter S3.3†), which exceeds the EU EQS for E2β.62
3.6 Natural estrogens in ponds
17α-Estradiol (<LOQ −8.6 ng L−1, detected in five out of 12 sampled ponds) and E1 (<LOQ −5.2 ng L−1, detected in five out of 12 sampled ponds) were the natural estrogens mainly found in ponds. 17β-Estradiol and E3 were above the LOQ only once in two different ponds (Fig. 5). In the weekly sampled pond, E1 concentration increased gradually from 0.30 to 1.9 ng L−1 over a month (Fig. S13†). In contrast, concentrations of E2α, E2β, and E3 were always below the LOQ.
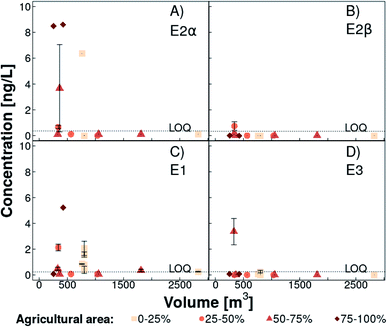 |
| Fig. 5 17α-Estradiol (panel A, E2α), 17β-estradiol (panel B, E2β), estrone (panel C, E1), and estriol (panel D, E3) concentrations in ponds in Lake Baldegg catchment (for location, see Fig. 1) in March and April 2019, plotted against their estimated volume. The coral shades and symbol shape indicate the percentage of agricultural area that is in circumference of 250 m around the ponds (light coral squares: 0–25% agricultural area, medium coral circles: 25–50% agricultural area, coral triangles: 50–75% agricultural area, dark coral diamonds: 75–100% agricultural area). Error bars represent the standard deviation among triplicate samples. Data points without error bars were either below limit of quantification (LOQ) or the standard deviation equaled zero. For pond Breitholz (volume: 803 m3), which was sampled weekly over four weeks, estrogen concentrations are shown per week. The dashed line indicates the LOQ for every substance. | |
Generally, natural estrogen concentrations above the LOQ were only found in the smaller ponds (Fig. 5). The ratio between the pond edge, where natural estrogens enter the pond, and the volume is decreasing with increasing pond size. Due to the general pond edge to volume relationship, we assume that dilution is higher in larger ponds, which could explain natural estrogen concentrations below the LOQ. The highest E2α and E1 were recorded in the pond which had the highest percentage of organically fertilized agricultural area in the catchment (89%, Fig. 5).
In contrast to stream water, E2α was the predominant estrogen (median 45% of the total estrogen concentration) in ponds followed by E1, E2β, and E3, with a median of 38%, 0%, and 0% of the total estrogen concentration, respectively (Fig. S12†). Two connected ponds exhibited higher E3 and lower E2α concentrations in the one downstream, which is in line with E3 being the final estrogenic degradation product of E2α.42
A final interpretation of these results is hampered by various possible influencing factors. A longer residence time of water in ponds in combination with differing microbial communities and lower dissolved oxygen availability in stagnant water15 could decelerate the degradation of E2α to E1 and of E1 to E3. Additionally, differences in cattle to pig ratios, estrogen composition in slurries applied, or hydrology are further possible explanations for dissimilar estrogen fingerprints in tributary and pond water (Fig. S12†). In Czech stagnant water bodies influenced by mixed sources, comparable E1 (≤7.1 ng L−1), E2β and E3 (<0.50 ng L−1) concentrations were found.14
4. Conclusion
During our sampling campaign, chemically and biologically determined EEQs in stream water never exceeded EU EQS for E2β62 for more than a day and more than fivefold. This result was confirmed by different sampling approaches combined with chemical and biological analyses of the samples. Slurry-derived natural estrogens alone did not cause long-term estrogenic contamination in Lake Baldegg streams. However, in combination with WWTP effluents, estrogen concentrations in streams above EU EQS occur.
During the sampling campaigns, only small rain events occurred. Likely, very intensive rain events shortly after slurry application and low base flow in tributaries or major slurry accidents could lead to higher estrogen loads in surface waters from agriculture in the here investigated catchment, as well as elsewhere. Many studies investigated the chronic exposure of fish to elevated estrogen concentrations,67 however, the effect of repeated short-term pulses of natural estrogens above EQS in surface waters requires further research.
The EU EQS of 3.6 ng L−1 for E1 (ref. 62) was exceeded in one of the sampled ponds. However, it has to be considered that we took only grab samples. For a more detailed risk assessment, ponds should be sampled more regularly. Due to longer residence times and lower dissolved oxygen availability, natural estrogens may prevail for longer periods of time in ponds. The role of E1 should not be underestimated, since in fish, its transformation to the more potent endocrine disruptor E2β was shown.68 Metabolic pathways of E2α and E3 in fish have not been elucidated so far. Moreover, estrogens exert endocrine disrupting effects also in amphibians, which breed and metamorphose in ponds at the beginning of the vegetation period.69
Reducing slurry applications prior to medium and strong rain events, limiting direct access of livestock to surface waters and applying slurry only in 3 m distance to surface waters are important measures to prevent natural estrogen emissions to surface waters. These measures are already implemented in Switzerland by law70 and good agricultural practice. Particularly, in catchments with a high percentage of hydrologically contributing and surface water-connected agricultural areas, agriculturally derived mean natural estrogen concentrations in streams were increased. In consequence, by reducing slurry applications on such areas, exposure to estrogenic compounds in surface waters could be further reduced.
Funding sources
The Swiss Federal Office for the Environment (FOEN) is acknowledged for the funding of this project (Contract No. 00.0445.PZ/P154-1654).
Conflicts of interest
There are no conflicts of interest to declare.
Acknowledgements
We would like to offer our special thanks to Francisca de Bruijn for her help during the sampling campaigns. A special thank goes to Josef Brunner, Robert Lovas and Sascha Künzli for the opportunity to use the Lake Baldegg tributaries monitoring network and for their support during the monitoring campaigns. We thank the entire team of the AuA-lab at Eawag for conducting the phosphorus analyses in the water samples. Johannes Hunkeler is acknowledged for answering questions regarding agricultural practices in the Lake Baldegg catchment. We appreciated the help with ArcGIS and map creation from Jonas Winizki. We would like to thank Andrea Schifferli and Nadine Bramaz for conducting bioassays. Advice and comments given by Lilian Gasser from statistical consulting at ETH Zurich were a great help to solve statistical questions. The first author is grateful for the assistance given by Franziska Blum, Shaine Vedova, Caroline Leuenberger and Dimitrios Stamatelatos during the passive sampling campaigns.
References
- A. D. Vethaak, J. Lahr, S. M. Schrap, A. C. Belfroid, G. B. Rijs, A. Gerritsen, J. de Boer, A. S. Bulder, G. C. Grinwis and R. V. Kuiper, Chemosphere, 2005, 59, 511–524 CrossRef CAS.
- E. Routledge, D. Sheahan, C. Desbrow, G. Brighty, M. Waldock and J. Sumpter, Environ. Sci. Technol., 1998, 32, 1559–1565 CrossRef CAS.
- A. C. Johnson, R. J. Williams and P. Matthiessen, Sci. Total Environ., 2006, 362, 166–178 CrossRef CAS.
- J. Kjær, P. Olsen, K. Bach, H. C. Barlebo, F. Ingerslev, M. Hansen and B. H. Sørensen, Environ. Sci. Technol., 2007, 41, 3911–3917 CrossRef.
- S. Dutta, S. Inamdar, J. Tso, D. S. Aga and J. T. Sims, J. Environ. Qual., 2010, 39, 1688–1698 CrossRef CAS.
- H. E. Gall, S. A. Sassman, B. Jenkinson, L. S. Lee and C. T. Jafvert, Water Res., 2015, 72, 162–173 CrossRef CAS.
- H. E. Gall, S. A. Sassman, B. Jenkinson, L. S. Lee and C. T. Jafvert, Hydrol. Processes, 2014, 28, 1318–1328 CrossRef CAS.
- D. A. Alvarez, N. W. Shappell, L. O. Billey, D. S. Bermudez, V. S. Wilson, D. W. Kolpin, S. D. Perkins, N. Evans, W. T. Foreman, J. L. Gray, M. J. Shipitalo and M. T. Meyer, Water Res., 2013, 47, 3347–3363 CrossRef CAS.
- M. Velicu and R. Suri, Environ. Monit. Assess., 2009, 154, 349–359 CrossRef CAS.
- E. P. Kolodziej, T. Harter and D. L. Sedlak, Environ. Sci. Technol., 2004, 38, 6377–6384 CrossRef CAS.
- L. A. Tremblay, J. B. Gadd and G. L. Northcott, Agric., Ecosyst. Environ., 2018, 253, 48–54 CrossRef CAS.
- P. Matthiessen, D. Arnold, A. C. Johnson, T. J. Pepper, T. G. Pottinger and K. G. T. Pulman, Sci. Total Environ., 2006, 367, 616–630 CrossRef CAS.
- E. P. Kolodziej and D. L. Sedlak, Environ. Sci. Technol., 2007, 41, 3514–3520 CrossRef CAS.
- T. Prochazkova, E. Sychrova, J. Vecerkova, B. Javurkova, A. Otoupalikova, M. Pernica, Z. Simek, M. Smutna, O. Lepsova-Skacelova and K. Hilscherova, Water Res., 2018, 136, 12–21 CrossRef CAS.
- G. Ice and B. Sugden, South. J. Appl. For., 2003, 27, 92–99 CrossRef.
- S. Alder, V. Prasuhn, H. Liniger, K. Herweg, H. Hurni, A. Candinas and H. U. Gujer, Land use policy, 2015, 48, 236–249 CrossRef.
-
Eurostat Statistics Explained, Archive: Agriculture - manure storage statistics, https://ec.europa.eu/eurostat/statistics-explained/index.php/Archive:Agriculture_-_manure_storage_statistics#Further_Eurostat_information, accessed January 2020 Search PubMed.
-
European Commission, Hormones in Meat, https://ec.europa.eu/food/safety/chemical_safety/meat_hormones_en, accessed September 2020 Search PubMed.
-
M. Breitenstein and A. Kirchhofer, Förderung der litho-rheophilen Fischarten der Schweiz–Factsheets zu Biologie und Förderungsmassnahmen, Federal Office for the Environment, 2010 Search PubMed.
-
Federal Act on the Protection of Waters
https://www.admin.ch/opc/de/classified-compilation/19910022/index.html, accessed January 2020 Search PubMed.
- E. L. Vermeirssen, M. J. F. Suter and P. Burkhardt-Holm, Environ. Toxicol. Chem., 2006, 25, 2413–2422 CrossRef CAS.
- H.-R. Aerni, B. Kobler, B. V. Rutishauser, F. E. Wettstein, R. Fischer, W. Giger, A. Hungerbühler, M. D. Marazuela, A. Peter and R. Schönenberger, Anal. Bioanal. Chem., 2004, 378, 688–696 CrossRef CAS.
-
B. Oertli, D. Auderset Joye, E. Castella, R. Juge and J.-B. Lachavanne, Diversité biologique et typologie écologique des étangs et petits lacs de Suisse, http://archive-ouverte.unige.ch/unige:26123, accessed August 2020 Search PubMed.
-
J. Tata, Recent Advances Steroid Hormone Action, 1987, pp. 259–283 Search PubMed.
- A. J. Perlman, A. P. Wolffe, J. Champion and J. R. Tata, Mol. Cell. Endocrinol., 1984, 38, 151–161 CrossRef CAS.
- I. Lutz, W. Kloas, T. A. Springer, L. R. Holden, J. C. Wolf, H. O. Krueger and A. J. Hosmer, Anal. Bioanal. Chem., 2008, 390, 2031–2048 CrossRef CAS.
-
Federal Statistical Office, Statistischer Atlas der Schweiz, https://www.atlas.bfs.admin.ch/maps/13/de/15070_112_7281_7263/23820.html, accessed August 2019 Search PubMed.
- R. Gächter and B. Wehrli, Environ. Sci. Technol., 1998, 32, 3659–3665 CrossRef.
-
Ordinance on the Avoidance and the Disposal of Waste, https://www.admin.ch/opc/en/classified-compilation/20141858/index.html, accessed August 2020 Search PubMed.
- E. L. Vermeirssen, R. I. Eggen, B. I. Escher and M. J.-F. Suter, CHIMIA International Journal for Chemistry, 2008, 62, 389–394 CrossRef CAS.
-
J. Bloesch, The Lakes Handbook, 2004, vol. 1, pp. 197–229 Search PubMed.
-
Canton of Lucerne Umwelt und Energie, Abfluss und Seepegel, https://uwe.lu.ch/themen/gewaesser/hydrometrie/abfluss_und_seepegel, accessed March 2020 Search PubMed.
-
Federal Office of Topography, swisstopo, https://shop.swisstopo.admin.ch/en/products/landscape, (accessed March 2020) Search PubMed.
- R. Burki, E. L. Vermeirssen, O. Körner, C. Joris, P. Burkhardt-Holm and H. Segner, Environ. Toxicol. Chem., 2006, 25, 2077–2086 CrossRef CAS.
- W. J. Backe, Environ. Sci. Technol., 2015, 49, 14311–14318 CrossRef CAS.
- D. Rechsteiner, S. Schrade, M. Zähner, M. Müller, J. Hollender and T. D. Bucheli, J. Agric. Food Chem., 2020, 68, 5545–5554 CrossRef CAS.
- M. D. Jürgens, K. I. Holthaus, A. C. Johnson, J. J. Smith, M. Hetheridge and R. J. Williams, Environ. Toxicol. Chem., 2002, 21, 480–488 CrossRef.
- P. Y. Kunz, E. Simon, N. Creusot, B. S. Jayasinghe, C. Kienle, S. Maletz, A. Schifferli, C. Schönlau, S. Aït-Aïssa and N. D. Denslow, Water Res., 2017, 110, 378–388 CrossRef CAS.
-
ISO 19040-3: 2018, Water quality — Determination of the estrogenic potential of water and waste water — Part 3: In vitro human cell-based reporter gene assay, https://www.iso.org/obp/ui/#iso:std:iso:19040:-3:ed-1:v1:en, accessed May 2020 Search PubMed.
-
R. C. Team, A language and environment for statistical computing, R Foundation for Statistical Computing, Vienna, Austria, 2012, https://www.r-project.org/, accessed February 2020 Search PubMed.
-
B. McCune, J. B. Grace and D. L. Urban, Analysis of ecological communities, MjM Software Design, Gleneden Beach, OR, 2002 Search PubMed.
- S. R. Hutchins, M. V. White, F. M. Hudson and D. D. Fine, Environ. Sci.
Technol., 2007, 41, 738–744 CrossRef CAS.
-
Verordnung über landwirtschaftliche Begriffe und die Anerkennung von Betriebsformen, https://www.admin.ch/opc/de/classified-compilation/19983381/index.html, accessed February 2020 Search PubMed.
- D. Rechsteiner, F. E. Wettstein, N. Pfeiffer, J. Hollender and T. D. Bucheli, Agric. Ecosyst. Environ. Search PubMed , submitted.
- W. Richner, S. Sinaj, C. Carlen, R. Flisch, C. Gilli, O. Huguenin-Elie, T. Kuster, A. Latsch, J. Mayer and R. Neuweiler, Agrarforsch. Schweiz., 2017, 8, 47–66 Search PubMed.
-
S. Stoll, C. von Arb, C. Jörg, S. Kopp and V. Prasuhn, Evaluation der stark zur Phosphor-belastung des Baldeggersees beitragenden Flächen, http://link.ira.agroscope.ch/de-CH/publication/41029, accessed June 2020 Search PubMed.
- K. I. Holthaus, A. C. Johnson, M. D. Jürgens, R. J. Williams, J. J. Smith and J. E. Carter, Environ. Toxicol. Chem., 2002, 21, 2526–2535 CrossRef CAS.
- J. A. Robinson, Q. Ma, J. P. Staveley, W. J. Smolenski and J. Ericson, Environ. Toxicol. Chem., 2017, 36, 621–629 CrossRef CAS.
- C. Kienle, E. L. Vermeirssen, A. Schifferli, H. Singer, C. Stamm and I. Werner, PLoS One, 2019, 14, e0226278 CrossRef CAS.
- C. Hahn, V. Prasuhn, C. Stamm, P. Lazzarotto, M. W. Evangelou and R. Schulin, Hydrol. Earth Syst. Sci., 2013, 17, 3679 CrossRef.
- K. Smith, D. Jackson and P. Withers, Environ. Pollut., 2001, 112, 53–60 CrossRef CAS.
- B. B. Coelho, R. Murray, D. Lapen, E. Topp and A. Bruin, Agric. Water Manag., 2012, 104, 51–61 CrossRef.
- R. Gächter, J. M. Ngatiah and C. Stamm, Environ. Sci. Technol., 1998, 32, 1865–1869 CrossRef.
- R. McDowell and A. Sharpley, Sci. Total Environ., 2001, 278, 113–125 CrossRef CAS.
- A.-K. Müller, K. Leser, D. Kämpfer, C. Riegraf, S. E. Crawford, K. Smith, E. Vermeirssen, S. Buchinger and H. Hollert, Water Res., 2019, 161, 540–548 CrossRef.
- C. C. Hoerger, F. E. Wettstein, K. Hungerbühler and T. D. Bucheli, Environ. Sci. Technol., 2009, 43, 6151–6157 CrossRef CAS.
- B. Boller, Agrarforschung, 1996, 3, 486–488 Search PubMed.
- E. Sivesind and P. Seguin, J. Agric. Food Chem., 2005, 53, 6397–6402 CrossRef CAS.
- R. Fletcher, Br. J. Nutr., 2003, 89, S39–S43 CrossRef CAS.
- M. Morrison, E. Cober, M. Saleem, N. McLaughlin, J. Fregeau-Reid, B. Ma, W. Yan and L. Woodrow, Crop Sci., 2008, 48, 2201–2208 CrossRef.
- C. C. Hoerger, F. E. Wettstein, H. J. Bachmann, K. Hungerbühler and T. D. Bucheli, Environ. Sci. Technol., 2011, 45, 6752–6760 CrossRef CAS.
-
Official Journal of the European Union, 2018, L 141, https://eur-lex.europa.eu/legal-content/EN/TXT/?uri=OJ%3AL%3A2018%3A141%3AFULL Search PubMed.
- W. H. Moos, J. A. Dykens, D. Nohynek, E. Rubinchik and N. Howell, Drug Dev. Res., 2009, 70, 1–21 CrossRef CAS.
- J. Margot, C. Kienle, A. Magnet, M. Weil, L. Rossi, L. F. de Alencastro, C. Abegglen, D. Thonney, N. Chèvre, M. Schärer and D. A. Barry, Sci. Total Environ., 2013, 461–462, 480–498 CrossRef CAS.
- M. J. Arlos, W. J. Parker, J. R. Bicudo, P. Law, P. Marjan, S. A. Andrews and M. R. Servos, Sci. Total Environ., 2018, 610–611, 1103–1112 CrossRef CAS.
- M. Kostich, R. Flick and J. Martinson, Environ. Pollut., 2013, 178, 271–277 CrossRef CAS.
- J. K. Leet, H. E. Gall and M. S. Sepúlveda, J. Appl. Toxicol., 2011, 31, 379–398 CrossRef CAS.
- G. T. Ankley, D. Feifarek, B. Blackwell, J. E. Cavallin, K. M. Jensen, M. D. Kahl, S. Poole, E. Randolph, T. Saari and D. L. Villeneuve, Environ. Sci. Technol., 2017, 51, 4705–4713 CrossRef CAS.
- M. R. Lambert, G. S. Giller, L. B. Barber, K. C. Fitzgerald and D. K. Skelly, Proc. Natl. Acad. Sci. U. S. A., 2015, 112, 11881–11886 CrossRef CAS.
-
Ordinance on the Reduction of Risks relating to the Use of Certain Particularly Dangerous Substances, Preparations and Articles, https://www.admin.ch/opc/en/classified-compilation/20021520/index.html, accessed February 2020 Search PubMed.
-
Federal Office for the Environment, FOEN geodata available, https://www.bafu.admin.ch/bafu/en/home/state/data/geodata.html, accessed May 2020 Search PubMed.
Footnote |
† Electronic supplementary information (ESI) available. See DOI: 10.1039/d0em00317d |
|
This journal is © The Royal Society of Chemistry 2020 |
Click here to see how this site uses Cookies. View our privacy policy here.