Secondary organic aerosol formation from evaporated biofuels: comparison to gasoline and correction for vapor wall losses†
Received
2nd March 2020
, Accepted 27th May 2020
First published on 19th June 2020
Abstract
With an ongoing interest in displacing petroleum-based sources of energy with biofuels, there is a need to measure and model the formation and composition of secondary organic aerosol (SOA) from organic compounds present in biofuels. We performed chamber experiments to study SOA formation from four recently identified biofuel molecules and mixtures and commercial gasoline under high NOx conditions: diisobutylene, cyclopentanone, an alkylfuran mixture, and an ethanol-to-hydrocarbon (ETH) mixture. Cyclopentanone and diisobutylene had a significantly lower potential to form SOA compared to commercial gasoline, with SOA mass yields lower than or equal to 0.2%. The alkylfuran mixture had an SOA mass yield (1.6%) roughly equal to that of gasoline (2.0%) but ETH had an average SOA mass yield (11.5%) that was six times higher than that of gasoline. We used a state-of-the-science model to parameterize or simulate the SOA formation in the chamber experiments while accounting for the influence of vapor wall losses. Simulations performed with vapor wall losses turned off and at atmospherically relevant conditions showed that the SOA mass yields were higher than those measured in the chamber at the same photochemical exposure and were also higher than those estimated using a volatility basis set that was fit to the chamber data. The modeled SOA mass yields were higher primarily because they were corrected for vapor wall losses to the Teflon® chamber.
Environmental significance
Biofuels are chemically distinct from fossil fuels and hence little is known of their ability to contribute to atmospheric formation of secondary organic aerosol (SOA). In this work, we measure and model the SOA formation from four next-generation biofuels and compare results to conventional gasoline. We find that (i) aromatic-rich fuels and those containing furanic compounds form more or as much SOA than gasoline and (ii) atmospheric SOA mass yields might be larger than those directly measured in environmental chambers on account of losses of vapors to the chamber walls.
|
1. Introduction
Volatile organic compounds (VOCs), emitted by anthropogenic and biogenic sources, undergo oxidation in the atmosphere to form secondary organic aerosol (SOA). SOA is an important fraction of fine particulate matter and consequently has adverse impacts on climate, air quality, and human health.1 Despite the large SOA contribution to fine particle pollution, there are large uncertainties surrounding the precursors, pathways, and properties of SOA.2 For instance, SOA mass concentrations are underestimated by chemical transport models in polluted urban environments3 and uncertainties in global burdens of SOA span nearly an order of magnitude.4 There is a continued need for more laboratory and field measurements to improve our understanding of SOA and for better models of SOA formation, transport, and fate in the atmosphere.
For reasons ranging from energy independence to environmental sustainability, there is ongoing interest in the production of biofuels from sustainable feedstocks to meet current and future energy demands.5 Ethanol, a biofuel sourced mainly from corn and sugarcane, is currently blended with gasoline (average of 10% by volume) for use in the transportation sector in the United States. With an eye towards the future, the United States Department of Energy recently invested in a large, multi-agency initiative titled Co-Optimization of Fuels and Engines (or Co-Optima) that aims to optimize biofuels and engines for improvements in engine performance while reducing tailpipe emissions.6 As part of the study focused on fuel selection, McCormick et al.7 developed a method to screen biofuel molecules and mixtures that could be blended with gasoline and have properties enabling more efficient engine designs and operating strategies. The selection criteria included limits on physical (e.g., boiling point), chemical (e.g., biodegradability), combustion (e.g., research octane number), and health-relevant (e.g., carcinogenicity) properties. However, they did not consider impacts from oxidation of these biofuel molecules and mixtures in the atmosphere and their ability to form SOA. Biofuel molecules, similar to those found in any liquid fuel, are emitted to the atmosphere, either through evaporative processes or as unburned species in the tailpipe. May et al.8 found that a third to a half of the non-methane organic compounds emissions from light-duty gasoline vehicles consisted of unburned fuel. The potential of a biofuel, or any other precursor, to form SOA depends on its molecular structure and volatility.9 If the SOA mass yield for a biofuel is larger than that for gasoline, SOA formation can negatively offset some of the environmental benefits that come from being optimized to reduce primary particle emissions in the tailpipe.
Researchers have typically relied on laboratory-based environmental chamber data to develop parameterizations to represent SOA formation in air quality models. To date, most SOA model parameterizations have not been corrected for losses of vapors to the walls of the Teflon® chamber, which can bias SOA production in chamber experiments.10–12 Furthermore, chamber experiments have historically used high initial VOC and oxidant concentrations to ensure abundant SOA production (>20 μg m−3) at levels above instrument detection limits (>1 μg m−3). These concentrations are significantly elevated compared to those found in the atmosphere, including most urban areas.13 Direct SOA parameterizations derived under these highly polluted conditions may overestimate SOA production in lower-concentration conditions, and may not reflect the magnitude and properties of SOA formed in the atmosphere.14,15 Experiments are often challenging to perform under atmospherically relevant conditions (the experiments in this work were also performed at elevated VOC levels). Thus, detailed models, such as those used in this work, that can simulate experimental artifacts (e.g., vapor wall losses) and the subsequent cascade of oxidation reactions (e.g., functionalization and fragmentation reactions), can help to translate chamber data (where VOC and SOA concentrations are elevated) to the more atmospherically-relevant conditions simulated in air quality models (where VOC and SOA concentrations are much lower).
In this work, we performed chamber experiments to study the SOA formation from four biofuels and one mixture of gasoline fuel with 10% ethanol by volume under high NOx conditions representative of those found in urban environments. The SOA formation was modeled using a state-of-the-science model that accounted for the influence of vapor wall losses and allowed us to determine atmospherically relevant SOA mass yields.
2. Methods
2.1 SOA measurements
Environmental chamber.
The SOA experiments were performed with the Colorado State University (CSU) environmental chamber. The CSU chamber consists of a temperature and relative-humidity controlled 10 m3 Teflon®-FEP (Fluorinated Ethylene Propylene) bag inside a steel-plywood enclosure. Based on chemical actinometry experiments, 80 UV-A black lights mounted inside the enclosure produced a maximum NO2 photolysis rate of ∼0.25 min−1 (Fig. S1†). The UV-A bulbs produced light with wavelengths between 315 and 400 nm with a peak intensity at 350 nm. The temperature inside the chamber was managed to below 28 °C using a 3.5 kW air conditioner.
Chamber operation.
Prior to beginning the experiment, the chamber was flushed with HEPA and activated-charcoal filtered air for at least 12 hours with the UV lights turned on. These measures were found to be sufficient in keeping the background contribution, especially from oxidation of desorbed vapors from the walls, to SOA formation to a minimum (<0.5 μg m−3). Ammonium sulfate particles were nebulized using an aerosol generation system (AGS, Brechtel Inc., CA) and injected into the chamber to provide the seed for SOA condensation. The initial seed surface area concentration in our experiments was 1100 ± 400 μm2 cm−3. Nitrous acid (HONO) was synthesized and added to the chamber following the methods of Ng et al.16 but with one minor modification. Clean air was bubbled through a fresh mixture of 50 mL of 10% H2SO4 and 25 mL of 1% NaNO2 solution and vented for ∼30 minutes, following which the bubbled air was added to the chamber for ∼30 minutes. The rationale for venting is described in the ESI† (S.1 HONO synthesis). HONO photolyzes under UV-A lights to yield a burst of hydroxyl radicals (OH) in the first hour of the experiment (>107 molecules cm−3) with a much lower concentration over the next few hours (∼106 molecules cm−3). The chamber experiments were performed with unburned fuel based on the observations of Jathar et al.17 that the unburned fuel system could serve as an appropriate, but a more convenient model, to study the SOA formation from tailpipe exhaust. Each fuel was studied in isolation and not as a blend with gasoline to determine each fuel's distinct potential to form SOA. The fuel was injected into the chamber by passing hot air (∼5 L min−1 at ∼200 °C) over the tip of a microliter syringe that was gradually depressed over the course of several minutes. After the fuel was injected, the contents of the chamber were allowed to mix for at least 45 minutes prior to the lights being turned on. The mixing time was determined based on observations of the time required for injected species (NO, NO2, toluene) to reach stable concentrations in the chamber. In each experiment, the contents of the chamber were irradiated by the lights for a maximum of six hours.
Gas and particle measurements.
Gas analyzers (42C and 49C, ThermoFisher Scientific, MA) measured concentrations of ozone (O3), NO, and
. The NOx instrument uses a molybdenum converter to convert NO2 and other reactive nitrogen species including HONO and peroxyacyl nitrates to NO prior to measurement,18 which is typically referred to as
.19 A scanning mobility particle sizer (SMPS, GRIMM Aerosol Technik, Austria) measured the aerosol size distribution between 32 and 717 nm. An aerosol chemical speciation monitor (ACSM, Aerodyne Research, MA) detected aerosol mass concentrations and the bulk chemical composition.20,21 There were no direct VOC measurements during the chamber experiments. Instead, we assumed a 100% injection efficiency to determine the initial VOC concentrations and used estimates of OH concentrations to determine the decay of the VOC species with time. The OH concentrations during the experiments were determined from simulations performed with the SAPRC gas-phase chemical mechanism;22,23 OH concentrations are typically estimated in chamber experiments using the measured decay of VOCs.24–26 Details of the OH concentration estimates can be found in the ESI† (S.2 SAPRC modeling). Briefly, a SAPRC simulation was performed by specifying the experiment-specific initial concentrations of NO, O3, and VOC(s) and chamber-specific photolysis rates for all species in SAPRC. The initial HONO concentration was adjusted until the SAPRC model predictions matched the observed concentrations of NO and O3. This method to estimate the OH concentrations was first validated against four chamber experiments performed with toluene where the toluene decay was measured with a gas chromatograph photoionization detector (GC-PID, SRI Instruments, CA). The SAPRC simulations were then used to determine OH concentrations for the fuel experiments. The implications of our inability to measure the VOCs in these experiments are presented in the ‘Discussion’ section.
Fuels and experimental matrix.
A total of twelve experiments were performed on five different fuels at different initial concentrations. Details of the experiments are presented in Table 1. Four of the fuel molecules and mixtures, namely diisobutylene, cyclopentanone, alkylfuran mixture, and an ethanol-to-hydrocarbon (ETH) mixture (referred to as Vertifuel in previous literature by Lunderman et al.27), can be synthesized from sustainable feedstocks and were chosen from a list of eight identified by McCormick et al.7 that were compatible with spark-ignition engines. These biofuels were deliberately picked since their molecular structures and composition indicated that they have some potential to form SOA. The rest were small alcohols (e.g., ethanol, propanol, butanol) and are expected to form little to no SOA. Diisobutylene is a mixture of 75% 2,4,4-trimethyl-1-pentene and 25% 2,4,4-trimethyl-2-pentene by mass. The alkylfuran mixture was 40% 2-methylfuran and 60% 2,5-dimethylfuran by mass. ETH is synthesized from ethanol,28 and resembles a petrofuel. Specifically, ETH is a complex mixture of alkanes (14.5%), aromatics (70%), alkenes (12%), oxygenates including ethanol (1%), and a small number of unknown compounds (2.5%); numbers in parentheses are mass percentages. To contrast the SOA formation from these select biofuels, we performed experiments with an E10 gasoline (gasoline blended with 10% ethanol by volume) prepared by blending ethanol into a commercial reformulated blendstock for oxygenate blending (RBOB) obtained from a petroleum refiner. A detailed speciation for all the fuels along with additional information (e.g., carbon number, kOH) is included in the ESI† (Excel spreadsheet). All five fuels (four biofuels and E10 gasoline) were sourced in small sterile vials from the National Renewable Energy Laboratory (NREL) and refrigerated at 4 °C prior to use. In addition to the fuel experiments, we performed two blank chamber experiments where all steps described earlier were undertaken, except no fuel was added to the chamber. These blank experiments produced very little SOA (<0.25 μg m−3) and this small SOA contribution was subtracted from the SOA formed during the fuel experiments.
Table 1 Gas and aerosol results for all experiments performed in this work. In order, we tabulate the fuel name, reaction rate constant with OH (kOH), average carbon number (C#), initial concentrations of VOC, NO,
, and seed, total VOC reacted, final SOA mass concentration and O
:
C, and final SOA mass yield
Date (2018) |
Fuel mol./mixture |
k
OH × 1012 (cm−3 s)a |
C# |
[VOC]0 (ppbv) |
[NO]0 (ppbv) |
[NO2]0b (ppbv) |
[Seed]0 (μm2 cm−3) |
[ΔVOC]c (ppbv) |
SOAc (μg m−3) |
SOA O : Cc |
SOA mass yieldc,e (%) |
Gathered and/or calculated from EPISuite (http://www.chemspider.com/).
All reactive N except NO.
End-of-experiment values.
SOA estimates based on SMPS data only.
Not-corrected for vapor wall losses. NM = not measured. BDL = below detection limit.
|
Mar 1d |
Cyclopentanone |
6.8 |
5.0 |
661 |
NM |
NM |
1780 |
134 |
BDL |
NM |
BDL |
Apr 3 |
Cyclopentanone |
6.8 |
5.0 |
1323 |
23 |
201 |
794 |
165 |
1 ± 1 |
1.31 |
0.2 ± 0.2 |
Mar 6 |
Diisobutylene |
58.8 |
8.0 |
374 |
41 |
155 |
975 |
302 |
2 ± 1 |
NM |
0.1 ± 0.1 |
Mar 20 |
Diisobutylene |
58.8 |
8.0 |
748 |
58 |
221 |
1158 |
524 |
2 ± 2 |
0.76 |
0.1 ± 0.1 |
Mar 3 |
Alkylfuran mix |
101.3 |
5.6 |
583 |
39 |
138 |
1065 |
409 |
23 ± 4 |
0.85 |
1.8 ± 0.3 |
Mar 11d |
Alkylfuran mix |
101.3 |
5.6 |
874 |
57 |
143 |
1261 |
494 |
27 ± 1 |
NM |
1.7 ± 0.1 |
Mar 15d |
Alkylfuran mix |
101.3 |
5.6 |
874 |
54 |
133 |
1088 |
381 |
14 ± 1 |
NM |
1.2 ± 0.1 |
Feb 27 |
ETH |
20.1 |
8.2 |
335 |
68 |
246 |
415 |
176 |
110 ± 16 |
0.51 |
16.4 ± 2.4 |
Mar 8 |
ETH |
20.1 |
8.2 |
223 |
43 |
157 |
1501 |
118 |
47 ± 6 |
0.60 |
10.4 ± 1.2 |
Apr 5 |
ETH |
20.1 |
8.2 |
447 |
37 |
186 |
1482 |
145 |
43 ± 7 |
0.59 |
7.8 ± 1.2 |
Mar 27 |
Gasoline |
12.7 |
7.3 |
867 |
NA |
NA |
1075 |
261 |
18 ± 3 |
0.69 |
2.0 ± 0.3 |
Mar 29 |
Gasoline |
12.7 |
7.3 |
650 |
49 |
176 |
931 |
196 |
13 ± 2 |
0.73 |
1.9 ± 0.3 |
Experimental data analysis.
The particle-wall-loss corrected SOA mass concentrations were determined for each experiment following Hildebrandt et al.29 The lower bound, particle-wall-loss corrected SOA estimate (SOAω=0) was calculated using the following equations by assuming that the SOA vapors only condensed on the suspended particles: |  | (1) |
| 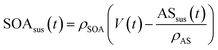 | (2) |
|  | (3) |
Eqn (1) and (2) calculate the suspended ammonium sulfate (ASsus) and SOA (SOAsus) mass concentrations and eqn (3) calculates the lower-bound, particle-wall-loss corrected estimate for the SOA mass concentration. In those equations, ρAS, the the density of ammonium sulfate, in g cm−3 (1.78 g cm−3), VASsus(0) is the volume concentration of the suspended ammonium sulfate at lights on in μm3 cm−3, kpar(t) is the time-dependent particle wall-loss rate in s−1, ρSOA is the SOA density in g cm−3 (assumed to be 1.4 g cm−3), and V(t) is the volume concentration of the suspended aerosol at time t in μm3 cm−3. kpar(t), was determined by fitting the change in the ammonium sulfate mass concentration measured by the ACSM. The upper bound, particle-wall-loss corrected SOA estimate (SOAω=1) was calculated using the following equation by assuming that the SOA vapors condensed on both the suspended particles and the particles deposited on the walls:
| 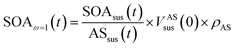 | (4) |
where

is the ratio of suspended SOA and ammonium sulfate concentrations directly measured by the ACSM at time
t.
We used the relationship developed by Canagaratna et al.,30 O
:
C = 0.079 + 4.31 × f44, to determine the SOA atomic O
:
C ratio from the mass fraction measured by the ACSM at a mass-to-charge ratio of 44. The end-of-experiment SOA mass yields were calculated as a ratio of the SOA formed and fuel reacted (i.e., ΔVOC). The amount of fuel reacted was determined using the following equation:
| ΔVOC = ΣiVOCi,0(1 − e−kOH,iOHexp) | (5) |
where VOC
i,0 is the initial concentration of species
i at lights on in μg m
−3,
kOH,i is the reaction rate constant of species
i with OH in cm
3 molecules
−1 s
−1, and OH
exp is the end-of-experiment OH exposure in molecules s cm
−3. The
kOH,i values were either determined from the primary literature (
e.g., see
ref. 31) or from the Estimation Program Interface Suite (EPISuite
32) and values for all VOCs have been tabulated in the ESI
† (Excel spreadsheet). The initial concentration for species
i was calculated from the normalized speciation for each fuel and the total volume of fuel injected into the chamber. These calculations assumed a 100% injection efficiency for the fuel into the chamber and no loss of fuel to the Teflon® walls prior to turning the lights on or photolysis from the UV-A lights. All VOC species in this work were sufficiently volatile (C* > 10
6 μg m
−3; C* is the effective saturation concentration
33) that they are unlikely to be lost to the walls of the chamber;
34 C*s for all VOC species are listed in the ESI
† (Excel spreadsheet). More recent work has suggested that oxygenated VOCs with C*s smaller than 10
6 μg m
−3 (
ref. 11 and 35) may partition into the chamber walls and hence these losses were accounted for in modeling the SOA formation (see ‘Vapor Wall Losses’ in next section).
2.2 SOA modeling
SOM-TOMAS model.
We used the recently developed Statistical Oxidation Model-TwO Moment Aerosol Sectional (SOM-TOMAS) model to simulate the SOA formation in our chamber experiments. The SOM simulates the gas-phase chemistry and calculates the thermodynamic properties of the oxidation products from SOA precursors.36 The TOMAS model uses a sectional approach to track the number and mass moments of the aerosol size distribution and simulates nucleation, coagulation, and condensation/evaporation.37 A brief description of the SOM and TOMAS models is provided below.
The SOM uses a carbon–oxygen grid to track the gas- and particle-phase organic species arising from VOC oxidation. Each cell in the carbon–oxygen grid represents a model organic species, which reflects the average properties (e.g. vapor pressure, reactivity) of all actual species with the same number of carbon (NC) and oxygen (NO) atoms that are produced from a given precursor. All gas-phase SOM species are assumed to be reactive towards OH. These reactions lead to either functionalization or fragmentation, resulting in movement through the carbon–oxygen grid. All SOM species properties (e.g., kOH, C*) are described in terms of NC and NO. Six adjustable parameters determine the chemistry and thermodynamics in each SOM grid: (i–iv) p1–p4, the yields of four functionalized products that add one, two, three, and four oxygen atoms to the carbon backbone respectively, (v) mfrag, the parameter that characterizes the fragmentation probability, Pfrag, and (vi) ΔLVP, the decrease in vapor pressure (or volatility) of the model species per addition of an oxygen atom. The probability of fragmentation (Pfrag) is designed to be a function of the O
:
C ratio of the model species and is parameterized as Pfrag = (O
:
C)mfrag. The volatility is represented using the effective saturation concentration (C*) and is parameterized as follows: C* = 10(−0.0337 × MWHC + 11.56 − NO × ΔLVP), where MWHC is the molecular weight of the hydrocarbon excluding the oxygen atoms. SOM is coupled to the TOMAS model,37,38 which tracks two moments (aerosol number and mass) across 36 size sections. For the mass moment, the particle phase of the SOM model species is tracked in each TOMAS size section. Particles within each size section are assumed to be internally mixed in composition. In this study, TOMAS simulates the kinetic condensation and evaporation of all SOM species for each TOMAS size section, and it also simulates coagulation of particles between and within size sections.
Model application.
For the fuels studies in this work, the SOM-TOMAS model was used to: (i) account for the influence of vapor losses to the walls of the Teflon® chamber and (ii) determine atmospherically relevant SOA mass yields. In the absence of any previous chamber data, SOM parameters were developed for the alkylfuran mixture, diisobutylene, and cyclopentanone, using the chamber data collected in this work. The SOM parameters were fit to reproduce the measured temporal evolution of the SOA mass concentrations. As the ETH and gasoline fuel compositions were known – and were primarily composed of previously studied SOA precursors (e.g., alkanes, aromatics) – historical SOM parameters were used to predict the SOA formation in these fuel experiments. Seven SOM grids, one for each SOA precursor class, were used to account for the diversity of organic compounds present in those fuels: linear alkanes (n-dodecane), branched alkanes (methylundecane), cyclic alkanes (hexylcyclohexane), benzene, toluene, lumped aromatics (m-xylene for ETH and o-xylene for gasoline), and polycyclic aromatic hydrocarbons (naphthalene). The SOM parameters for each grid are based on the species mentioned in the parentheses and these parameters are listed in Table S1.† This approach to use a single surrogate to represent the SOA formation from a class of VOC compounds is largely consistent with the approach used in other SOA models such as the volatility basis set (VBS).39–41 One of the differences between the SOM and VBS approaches is that in the SOM the parameters for the surrogate inform the statistical trajectory of the VOC oxidation in a carbon–oxygen grid and hence the surrogate and VOC (unless they are the same) are likely to have different SOA mass yields. In a VBS approach, all VOCs assigned to the same surrogate, by design, share the same SOA mass yield. A similar SOM grid-model setup was previously used and found to work quite well to model SOA formation in box42 and three-dimensional models.43–45
Vapor wall losses.
Loss of vapors to the walls of the Teflon® chamber is currently handled in the SOM-TOMAS model following Zhang et al.10 and Krechmer et al.11. Briefly, the first-order uptake to the walls is modeled using the rate coefficient kvap,on and the rate of release of vapors from the walls, kvap,off, is modeled using absorptive partitioning theory with the Teflon® wall serving as an absorbing mass with an effective mass concentration of Cwall. kvap,on and kvap,off for a model vapor species are calculated as follows: | 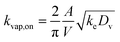 | (6) |
| 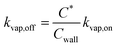 | (7) |
where A/V is the surface area to volume ratio for the Teflon® chamber in m−1, ke is the coefficient of eddy diffusion in s−1, and Dv is the gas-phase diffusion coefficient of the vapor molecule in m2 s−1. Based on the observations of Krechmer et al.,11Cwall was varied with the C* of the model species, with higher values used for more volatile species and vice versa: Cwall = 10
000 μg m−3 for C* > 104 μg m−3, Cwall = 16 × (C*)0.6 μg m−3 for C* < 104 μg m−3, and Cwall = 16 μg m−3 for C* < 1 μg m−3. ke for our CSU chamber was calculated following the methods described in Bian et al.,46 although a single ke value was calculated by combining the data from a subset of experiments instead of determining experiment-specific values. Details of the ke calculation can be found in the ESI† (S.3 Coefficient of eddy diffusion). We calculated a study-wide ke of 0.13 ± 0.02 s−1, which for a gas-phase diffusion coefficient of 4 × 10−6 m2 s−1, produced a kvap,on of 1.28 × 10−3 s−1, according to eqn (6). The time scale for gas-wall partitioning, or the inverse of kvap,on, was about 13 minutes and agreed well with the 7 to 13 minute gas-wall partitioning time scale calculated by Krechmer et al.11 for a slightly smaller Teflon® chamber (8 m3). We should note that the SOM parameters in Table S1† had to be refit to the original data since the earlier set of SOM parameters did not integrate the observations of Krechmer et al.11.
3. Results
3.1 Results from a sample experiment
Results from an example chamber experiment performed on the alkylfuran mixture are shown in Fig. 1. In Fig. 1(a), we show the evolution of the particle volume concentration as measured by the SMPS. Before the lights were turned on, the volume concentration increased with the injection of ammonium sulfate seed and decreased with steady loss of ammonium sulfate seed particles to the wall. After the lights were turned on, the volume concentration increased initially with condensation of SOA but decreased later on as SOA production stopped and ammonium sulfate + SOA particles and vapors were lost to the walls. Based on the loss of the ammonium sulfate seed particles to the walls, we estimated the suspended volume concentrations of the ammonium sulfate seed particles (dashed blue) and SOA (dashed orange) after the lights were turned on. In Fig. 1(c), we show the lower (w = 0) and upper (w = 1) bound SOA mass concentrations that were corrected for losses of particles to the walls. The upper bound estimate relied on the organic
:
ammonium sulfate ratio measured by the ACSM (shown in Fig. 1(b)). Vapor wall losses were modeled with the SOM-TOMAS model and those corrections are described later. The central SOA estimate, used henceforth, was calculated as an average of the lower and upper bound estimates. We estimated the uncertainty range by assuming that the lower and upper bounds were separated by four standard deviations; the ± ranges given for the SOA mass concentrations in Table 1 are one-quarter of this range and hence equivalent to the error. Unlike some previous chamber experiments,47,48 there was very little delay or ‘induction time’ between when the lights were turned on and when a sizable condensable SOA mass was detected by the particle instruments. This might have been on account of using a higher initial seed surface area for vapor condensation and/or a high OH concentration at the beginning of the experiment. SOA production was found to reduce particle losses in the ACSM,49 presumably because the SOA coating on the ammonium sulfate particles tended to reduce bounce in the vaporizer (Fig. S7†).
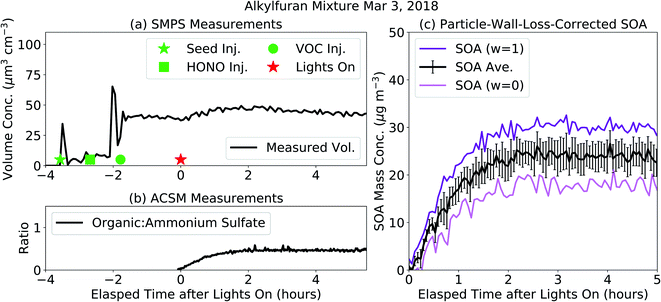 |
| Fig. 1 Aerosol results from the alkylfuran mixture experiment performed on Mar 3, 2018 that show the time evolution of the (a) total volume measured by the SMPS, (b) organic : ammonium sulfate ratio measured by the ACSM, and (c) particle-wall-loss corrected SOA mass concentration estimates. The volume concentration increase at two different times before the lights on can be attributed to two separate aerosol injections. The second aerosol injection was done to ensure sufficient seed concentrations for SOA condensation. | |
3.2 SOA from photooxidation of evaporated biofuels
Results from all the experiments performed in this work are summarized in Table 1. The end-of-experiment values in Table 1 were calculated three hours after the lights were turned on as there was very little change in the SOA mass and composition beyond three hours. This agrees well with the little to no change in estimated and modeled OH exposure, three hours after turning the lights on (Fig. S4(b)†). Despite large additions of the biofuel to the chamber (>370 ppbv or >1400 μg m−3), cyclopentanone and diisobutylene were found to produce very little SOA (<4.0 μg m−3). Their SOA mass yields were correspondingly quite low and did not exceed 0.2% for either biofuel. Lim and Ziemann50 measured an SOA mass yield of 4% for cyclohexane, a cyclic compound one larger in carbon number than cyclopentanone. A lower carbon number and increased susceptibility to fragmentation from the presence of a carbonyl group seems to have dramatically lowered the SOA mass yield for cyclopentanone in our work.51 The average SOA mass yield for diisobutylene (0.1%) in our experiments was slightly lower than that observed in previous experiments performed with 1-octene (an isomer of diisobutylene).52 The lower SOA mass yield likely indicates the role of carbon branching of the precursor on SOA formation.25
We observed much higher SOA mass yields for the alkylfuran mixture (average of 1.6%) when compared to those from cyclopentanone and diisobutylene. The SOA mass yields for the alkylfuran mixture (that contained species with carbon numbers of 5 and 6) were considerably larger than those for alkanes with similar carbon numbers, which have been shown to produce little to no SOA.50 Furans are heterocyclic compounds that contain a five-membered aromatic ring, yet their SOA mass yields were substantially lower than those for typical aromatic hydrocarbons measured under high NOx conditions; historical chamber yields for benzene, toluene, and xylenes have varied between 3 and 60%.10,16,53,54 The oxidation pathways and products from furan chemistry are hence expected to be different than those from aromatic hydrocarbons,55 noting that furan derivatives have been observed during the photooxidation of aromatic compounds.54,56,57
A handful of studies have performed chamber experiments with furanic compounds and reported on SOA mass yields. Two previous chamber studies have reported on SOA formation from 3-methylfuran, which is similar to the molecules present in our alkylfuran mixture (mixture of 2-methylfuran and dimethylfuran). Joo et al.58 measured an SOA mass yield of ∼2% for 3-methylfuran although the oxidation was performed with the nitrate radical and particulate organic nitrates (PONs) accounted for nearly 40% of the SOA formed. Strollo and Ziemann55 measured a much larger SOA mass yield from OH oxidation of 3-methylfuran (9–15%), but the substantially large SOA mass concentrations produced in those experiments (>2000 μg m−3) makes it difficult to compare the mass yields directly to our study.
The ETH produced the highest SOA mass yields (7.8–16.4%) amongst the four Co-Optima fuels. The high SOA mass yields, as we show later, can be attributed to the relatively large fraction of aromatic compounds (60%) in this fuel. The range in SOA mass yields reported in Table 1 for a given fuel molecule/mixture (≤factor of 2) was generally similar to the range observed in earlier studies where SOA mass yields from the same precursor were compared between experiments from the same chamber.24,29,59
The SOA O
:
C ratios are presented in Table 1. Unlike the SOA mass concentrations, the SOA O
:
C ratios varied little over the course of the experiment, suggesting, to first order, that the composition of the oxidation products in the particle phase did not change with time. Oxidation of cyclopentanone produced SOA with the highest O
:
C ratio (1.31) while oxidation of ETH produced SOA with the lowest O
:
C ratio (average of 0.56). The SOA O
:
C ratios are later shown to be useful in evaluating the model used in this work.
3.3 Comparisons to gasoline SOA
The SOA mass yields for gasoline in our experiments (average of 2.0%) compared reasonably well with chamber data from Jathar et al.17 and Chen et al.,60 who measured an SOA mass yield between 1 and 4%, and modeled data from Gentner et al.,61 who predicted an SOA mass yield of 2.3% (Jathar et al.17 and Gentner et al.61 studied summertime California gasoline while Chen et al.60 studied gasoline from China). Differences in the SOA mass yields between the studies could be attributed to small differences in the aromatic fraction and composition of the fuel studied. Overall, when compared to gasoline, two of the Co-Optima fuels – cyclopentanone and diisobutylene – had very low SOA mass yields (≤0.2%). The alkylfuran mixture had a slightly lower SOA mass yield compared to gasoline (average of 1.6% versus average of 2.0%). ETH had an average SOA mass yield of 11.5% that was six times higher than that for gasoline. Purely from an SOA perspective and assuming equivalent emissions of these species into the atmosphere, cyclopentanone and diisobutylene appear to be ideal candidates to be blended with gasoline. It is unclear if the alkylfuran mixture has any benefits over gasoline while ETH is likely to be a poor substitute. These conclusions are based on the assumption that the composition of the SOA precursors in the tailpipe is similar to the composition of the unburned fuel.8,17 Future work may need to examine the SOA formation from tailpipe exhaust.
3.4 Modeling the SOA formation and composition
We used the SOM-TOMAS model to fit or predict the SOA formation and composition in our chamber experiments while correcting for the influence of vapor wall losses. In the absence of any historical data, SOM parameters were developed based on representative chamber experiments performed on the alkylfuran mixture, diisobutylene, and cyclopentanone. Results from that exercise, where the model was fit to reproduce the evolution of the SOA mass concentrations, are shown in Fig. 2 (gasoline and ETH results are in Fig. 3). The SOM parameters are presented in Table 2. The SOM parameters were able to reproduce the measured SOA mass concentrations and O
:
C ratio (within 30%) for all experiments. We should note that the SOA O
:
C ratio was not used as a constraint during the fitting and the O
:
C agreement points to the ability of the SOM to capture the general features of the oxidation chemistry. We should note that the model performance for SOA mass concentrations and O
:
C was much better for the alkylfuran experiment compared to the diisobutylene and cyclopentanone experiments. This could be partly attributed to the observed variability and low SOA mass concentrations in the diisobutylene and cyclopentanone experiments.
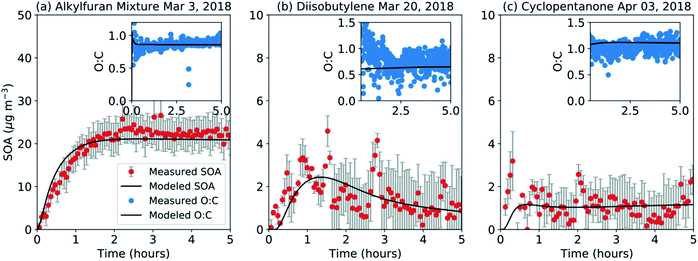 |
| Fig. 2 SOM-TOMAS model predictions based on parameter fits (solid black lines) compared to measurements (symbols) of SOA mass concentrations and SOA O : C for the (a) alkylfuran mixture, (b) diisobutylene, and (c) cyclopentanone. Model predictions for O : C are shown only after the first half hour as they were found to be unreliable at earlier times when the SOA mass concentrations were lower than 0.5 μg m−3. | |
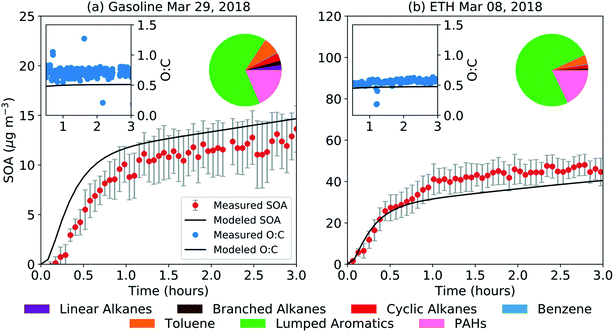 |
| Fig. 3 SOM-TOMAS model predictions (solid black lines) compared to measurements (symbols) of SOA mass concentrations and O : C for a representative (a) gasoline and (b) ETH experiment. The precursor contribution to SOA at the end of the experiment is shown as a pie chart in the top right corner of the panel. Note that the lumped aromatics were simulated with SOM parameters for o-xylene for gasoline and for m-xylene for ETH. | |
Table 2 SOM-TOMAS parameters determined by fitting to the experimental data presented in Fig. 2
Fuel |
m
frag
|
ΔLVP |
p
f1
|
p
f2
|
p
f3
|
p
f4
|
Alkylfuran mixture |
0.449 |
1.459 |
0 |
0.001 |
0.998 |
0 |
Diisobutylene |
0.277 |
1.509 |
0.946 |
0.042 |
0 |
0.013 |
Cyclopentanone |
0.434 |
1.613 |
0.369 |
0.256 |
0.329 |
0.047 |
The SOM-TOMAS model results for gasoline and ETH are shown in Fig. 3. Model predictions of the precursor contribution to the end-of-experiment SOA for both experiments are also shown in Fig. 3. A slightly different model configuration was used for the two experiments to optimize the model-measurement comparison. For the gasoline experiment, a model configuration that treated all C8 and higher single-ring aromatic compounds as low-yield aromatics (based on fits to o-xylene experiments) seemed to work best in reproducing the SOA mass concentration and O
:
C ratio. This configuration, however, did not work with the ETH experiment, and all C8 and higher single-ring aromatic compounds had to be treated as high-yield aromatics (based on fits to m-xylene experiments) to reproduce the measured SOA mass concentration and O
:
C ratio. The treatment of PAHs was kept the same for both fuels. The use of a xylene to model the SOA formation from C8 and larger single-ring aromatic compounds is consistent with its treatment in atmospheric models.39,41,62 That the single-ring aromatic compounds had to be treated in slightly different ways suggests that the aromatic composition between the two fuels was sufficiently different that they exhibited different potentials to form SOA. A closer examination of the aromatic composition indicated that the gasoline fuel had, on average, a slightly smaller aromatic carbon number (8.4 versus 8.7) and less alkyl substituents (0.61 versus 1.14 alkyl substituents per mole of fuel) than ETH. Aromatic carbon number and alkyl substituents on an aromatic ring have been found to influence SOA production.54,63 Our results imply that chemical mechanisms to model SOA formation need to consider the diversity in SOA potential for C8 and larger aromatic emissions, in addition to distinguishing between benzene, toluene, and larger aromatics. Regardless of the differences in the model configurations used for ETH and gasoline, non-benzene aromatic compounds contributed to more than 90% of the predicted SOA.
When the model was applied to experiments other than those shown in Fig. 2 and 3, the SOM-TOMAS model was able to reproduce the end-of-experiment SOA mass concentrations and O
:
C ratios for all fuels (Fig. S8†). This suggested that the fits developed for diisobutylene and the alkylfuran mixture and the treatment of C8 and larger single-ring aromatic compounds for gasoline and ETH worked well in reproducing the SOA formation in other experiments performed on the same fuel. We also performed simulations with the SOM-TOMAS model to assess the influence of the uncertainty in ke. Those results, shown in Fig. S9 and S10,† suggest that the predictions in the SOA mass concentrations and O
:
C ratios were relatively insensitive to the uncertainty linked to the vapor wall loss rate.
3.5 Atmospherically relevant SOA mass yields
The SOM-TOMAS model was used to simulate the atmospheric SOA mass yield by emitting a trace amount of precursor (∼1 pptv) into an ambient environment that had a constant organic aerosol mass concentration of 10 μg m−3 and an OH concentration of 1.5 × 106 molecules cm−3. Only a trace amount of precursor was added so that the SOA produced did not affect the organic aerosol mass concentration and the SOA mass yields between the different precursors could be compared at the same organic aerosol loading. A constant organic aerosol loading and OH concentration was used for simplicity while noting that both of these quantities change in the ambient environment with time. Vapor wall losses were turned off for these simulations. Results from those simulations, presented as an SOA mass yield with photochemical age, are shown in Fig. 4(a). These SOA mass yields are expected to be more atmospherically relevant than the chamber yields because vapors are not lost in the atmosphere as in a chamber and the calculations were performed at organic aerosol mass concentrations reflective of those found in typical urban environments. The atmospheric simulations predicted a similar ranking for the potential of the fuels to form SOA as the experimental data shown in Table 1, i.e., ETH had the highest SOA mass yield followed by the alkylfuran mixture and gasoline, and then by cyclopentanone and diisobutylene. However, at the same photochemical age as at the end of the experiment, the absolute SOA mass yields from the atmospheric simulations, shown in Fig. 4(b), were always equal to or higher than those measured in the chamber and reported in Table 1.
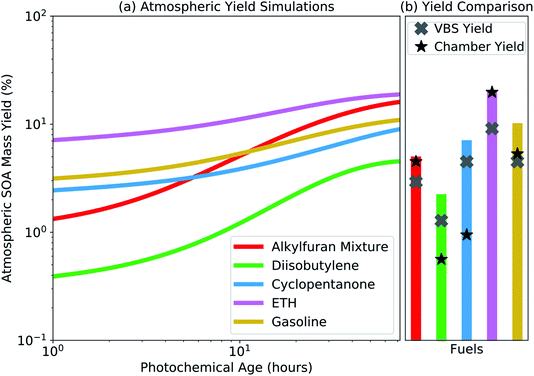 |
| Fig. 4 (a) SOA mass yields calculated from atmospheric simulations performed with the SOM-TOMAS model as a function of photochemical age for the five different fuels studied in this work. (b) Comparison of SOA mass yields from the SOM-TOMAS model predictions, chamber, and VBS fits. The SOM-TOMAS model predictions are those from panel (a) but corresponding to the photochemical age at the end of the chamber experiment. The SOA mass yields from the chamber are those measured at the end of the experiment. The SOA mass yields for the VBS are from fits to the chamber data but at an OA mass concentration of 10 μg m−3. | |
The difference in the SOA mass yields between the atmospheric simulations and the chamber experiments was a result of two competing effects. The SOA mass yields in the atmospheric simulations were expected to be higher than that in the experiments because the condensable and precursor vapors that were lost to the Teflon® walls in the chamber experiment were now allowed to contribute to SOA formation. On the other hand, the SOA mass yields in the experiments were expected to be higher than that in the atmospheric simulations because the SOA mass concentrations experiments except for cyclopentanone and diisobutylene were larger than 10 μg m−3, which allowed a larger fraction of the organic mass to partition into the particle phase. That the SOA mass yields in the atmospheric simulations at equivalent photochemical ages were higher than those reported in Table 1 suggested that the vapor-wall-loss effects more than offset the partitioning effects. This implies that the end-of-experiment SOA mass yields, despite being calculated at OA mass concentrations higher than those encountered in urban environments, are still likely to be lower than those that have been corrected for vapor wall losses and estimated at atmospherically relevant conditions. Although the SOA mass yields were higher in the atmospheric simulations than in the chamber experiments, the relative change in the SOA mass yields between the two seemed to vary with the fuel. These were very likely a result of the complex interplay of gas-phase chemistry and partitioning of the condensable vapors to the suspended particles and the walls of the Teflon® chamber.
In Fig. 4(b), we also compared predictions from the SOM-TOMAS model to predictions from a volatility basis set (VBS) model that was fit directly to the chamber data; VBS fits to the chamber data are shown in Fig. S11.† The VBS SOA mass yields, at an organic aerosol mass concentration of 10 μg m−3, did not vary with photochemical age and were lower than those predicted by the SOM-TOMAS model. The VBS SOA mass yields were lower because they did not account for the influence of vapor wall losses and, by assuming a constant volatility distribution, tended to underestimate the mass yields of the lower volatility species.
4. Discussion
The chamber experiments and numerical modeling performed in this work suggested that two of the Co-Optima fuels, namely cyclopentanone and diisobutylene, had a significantly lower potential to form SOA when compared to gasoline. A third fuel, the alkylfuran mixture, had the same SOA forming potential as gasoline. In contrast, ETH, on account of a large aromatic fraction, had a much higher potential to form SOA compared to gasoline. The Co-Optima initiative, so far, has ignored the atmospheric production of SOA from oxidation of compounds emitted from biofuel use. Our work shows that not only is the SOA potential significantly different between these biofuels but that some of them might be worse than gasoline (i.e., ETH or fuels with a high fraction of aromatic compounds). While biofuels might be environmentally more sustainable and optimized for combustion in spark-ignited engines, ignoring their impact on SOA formation may offset some of the projected environmental gains. An additional consideration that was not considered in this work is the potential of biofuel emissions to produce ozone (O3) in the atmosphere. Ozone is an atmospheric oxidant, a criteria pollutant, and a greenhouse gas that is produced during VOC oxidation in the presence of NOx.64 Aromatic compounds are important contributors to O3 production in urban environments65 and it is likely that ETH would contribute not only to SOA production but also O3 production.
We did not directly measure the VOC concentrations in this study because we did not have access to a complete suite of appropriate instrumentation. This prevented us from estimating the OH concentrations and exposure during the chamber experiments. These are important limitations of this study. By assuming a 100% injection efficiency and no losses of VOCs to the chamber walls, the SOA mass yields reported in this work represent a lower bound estimate. We attempted to reduce the uncertainty in the OH estimates by explicitly modeling the gas-phase chemistry and radical concentrations in each individual experiment using a chemical mechanism (i.e., SAPRC). While this modeling technique was evaluated for toluene photooxidation, the technique will need to be evaluated in the future for the other VOCs studied in this work. Another limitation of this work is that we did not measure or model the formation of oligomers in the condensed phase that likely play an important role in controlling the mass yields and properties of atmospheric SOA. Oligomers have been previously observed in the SOA formed from aromatic66 and heterocyclic compounds58 and subsequent work should attempt to understand the oligomeric composition of biofuel SOA.
In addition to being considered as a potential biofuel, furans and substituted furans account for a modest fraction of the gas-phase organic emissions from residential wood combustion67 and wildfires.68–70 The furan mixture studied herein had a measured SOA mass yield of ∼1.6% and an atmospherically relevant SOA mass yield of 10% after a day of photochemical aging. Although not as large as those for most aromatic compounds, the SOA mass yields were sufficiently large that furanic compounds could contribute modestly to biomass burning SOA.58 Furanic compounds, despite being much more reactive, are modeled as aromatic species in gas-phase chemical mechanisms used in atmospheric models.71 These models are thus likely to simultaneously underestimate the reactivity, but overestimate the SOA formation from furanic compounds. Both of these factors will tend to distort the magnitude and spatial distribution of the SOA from this class of compounds. Furanic compounds must be studied in much more detail to understand their potential to form SOA.
The atmospheric simulations performed in this work suggested that SOA mass yield data gathered in chamber experiments need to be interpreted using models, such as those used here, to account for the influence of vapor wall losses and to calculate atmospherically relevant SOA mass yields. We find that ignoring the influence of vapor wall losses, especially in smaller chambers where gas/wall partitioning timescales are on the order of minutes, as well as direct application of VBS-type parameterizations based on chamber data, may underestimate SOA production in air quality models.10 This finding, however, needs to be evaluated in the future by performing laboratory experiments at lower organic aerosol mass concentrations (<10 μg m−3) and where chamber wall losses can be minimized.
Author contributions
BK and SHJ designed the experimental study and YH and SHJ designed the modeling study. BK, LL, and MP performed the chamber experiments and analyzed chamber data. JV facilitated access to the SMPS. DKF facilitated access to the ACSM and Iodide-CIMS. LL and JM performed the HONO synthesis experiments. RM and MT provided the fuels and the fuel speciation data. JRP provided and advised on the use of the TOMAS model. AA and YH developed the SOA model. YH performed all data analysis. YH and SHJ wrote the paper with inputs from all co-authors.
Conflicts of interest
There are no conflicts to declare.
Acknowledgements
This work was partially supported by the National Oceanic and Atmospheric Administration (NA17OAR4310003 and NA17OAR4310001), Colorado Energy Research Collaboratory (37-2018), Department of Energy, Office of Science (DE-SC0017975), and the United States Environmental Protection Agency (RD839278). We would like to thank Dr Christopher D. Cappa and Dr Michael J. Kleeman with their help on the SOM-TOMAS model development. The authors acknowledge Oak Ridge National Laboratory for supplying the ETH sample. The National Renewable Energy Laboratory is operated by the Alliance for Sustainable Energy, LLC, for the U.S. Department of Energy (DOE), under Contract DE-AC36-08GO28308. Research at NREL was conducted as part of the Co-Optimization of Fuels & Engines (Co-Optima) project sponsored by the U.S. DOE Office of Energy Efficiency and Renewable Energy (EERE), Bioenergy Technologies and Vehicle Technologies Offices.
References
- J. L. Jimenez, M. R. Canagaratna, N. M. Donahue, A. S. H. Prevot, Q. Zhang, J. H. Kroll, P. F. DeCarlo, J. D. Allan, H. Coe, N. L. Ng, A. C. Aiken, K. S. Docherty, I. M. Ulbrich, A. P. Grieshop, A. L. Robinson, J. Duplissy, J. D. Smith, K. R. Wilson, V. A. Lanz, C. Hueglin, Y. L. Sun, J. Tian, A. Laaksonen, T. Raatikainen, J. Rautiainen, P. Vaattovaara, M. Ehn, M. Kulmala, J. M. Tomlinson, D. R. Collins, M. J. Cubison, E. J. Dunlea, J. A. Huffman, T. B. Onasch, M. R. Alfarra, P. I. Williams, K. Bower, Y. Kondo, J. Schneider, F. Drewnick, S. Borrmann, S. Weimer, K. Demerjian, D. Salcedo, L. Cottrell, R. Griffin, A. Takami, T. Miyoshi, S. Hatakeyama, A. Shimono, J. Y. Sun, Y. M. Zhang, K. Dzepina, J. R. Kimmel, D. Sueper, J. T. Jayne, S. C. Herndon, A. M. Trimborn, L. R. Williams, E. C. Wood, A. M. Middlebrook, C. E. Kolb, U. Baltensperger and D. R. Worsnop, Evolution of Organic Aerosols in the Atmosphere, Science, 2009, 326(5959), 1525–1529 CrossRef CAS PubMed.
- S. Fuzzi, U. Baltensperger, K. Carslaw, S. Decesari, H. Denier van der Gon, M. C. Facchini, D. Fowler, I. Koren, B. Langford, U. Lohmann, E. Nemitz, S. Pandis, I. Riipinen, Y. Rudich, M. Schaap, J. G. Slowik, D. V. Spracklen, E. Vignati, M. Wild, M. Williams and S. Gilardoni, Particulate Matter, Air Quality and Climate: Lessons Learned and Future Needs, Atmos. Chem. Phys., 2015, 15(14), 8217–8299 CrossRef CAS.
- P. L. Hayes, A. G. Carlton, K. R. Baker, R. Ahmadov, R. A. Washenfelder, S. Alvarez, B. Rappenglück, J. B. Gilman, W. C. Kuster, J. A. de Gouw, P. Zotter, A. S. H. Prévôt, S. Szidat, T. E. Kleindienst, J. H. Offenberg, P. K. Ma and J. L. Jimenez, Modeling the Formation and Aging of Secondary Organic Aerosols in Los Angeles during CalNex 2010, Atmos. Chem. Phys., 2015, 15(10), 5773–5801 CrossRef CAS.
- M. Shrivastava, C. D. Cappa, J. Fan, A. H. Goldstein, A. B. Guenther, J. L. Jimenez, C. Kuang, A. Laskin, S. T. Martin and N. L. Ng,
et al., Recent Advances in Understanding Secondary Organic Aerosol: Implications for Global Climate Forcing, Rev. Geophys., 2017, 55(2), 509–559 CrossRef.
- G. M. Morrison, S. Yeh, A. R. Eggert, C. Yang, J. H. Nelson, J. B. Greenblatt, R. Isaac, M. Z. Jacobson, J. Johnston, D. M. Kammen, A. Mileva, J. Moore, D. Roland-Holst, M. Wei, J. P. Weyant, J. H. Williams, R. Williams and C. B. Zapata, Comparison of Low-Carbon Pathways for California, Clim. Change, 2015, 131(4), 545–557 CrossRef.
-
J. T. Farrell, R. Wagner, D. Gaspar and C. Moen, Co-Optimization of Fuels & Engines: FY18 Year in Review, National Renewable Energy Lab. (NREL), Golden, CO (United States), 2019 Search PubMed.
- R. L. McCormick, G. Fioroni, L. Fouts, E. Christensen, J. Yanowitz, E. Polikarpov, K. Albrecht, D. J. Gaspar, J. Gladden and A. George, Selection Criteria and Screening of Potential Biomass-Derived Streams as Fuel Blendstocks for Advanced Spark-Ignition Engines, SAE Int. J. Fuels Lubr., 2017, 10(2), 442–460 CrossRef.
- A. A. May, N. T. Nguyen, A. A. Presto, T. D. Gordon, E. M. Lipsky, M. Karve, A. Gutierrez, W. H. Robertson, M. Zhang, C. Brandow, O. Chang, S. Chen, P. Cicero-Fernandez, L. Dinkins, M. Fuentes, S.-M. Huang, R. Ling, J. Long, C. Maddox, J. Massetti, E. McCauley, A. Miguel, K. Na, R. Ong, Y. Pang, P. Rieger, T. Sax, T. Truong, T. Vo, S. Chattopadhyay, H. Maldonado, M. M. Maricq and A. L. Robinson, Gas- and Particle-Phase Primary Emissions from in-Use, on-Road Gasoline and Diesel Vehicles, Atmos. Environ., 2014, 88, 247–260 CrossRef CAS.
- S. H. Jathar, N. M. Donahue, P. J. Adams and A. L. Robinson, Testing Secondary Organic Aerosol Models Using Smog Chamber Data for Complex Precursor Mixtures: Influence of Precursor Volatility and Molecular Structure, Atmos. Chem. Phys., 2014, 14(11), 5771–5780 CrossRef.
- X. Zhang, C. D. Cappa, S. H. Jathar, R. C. McVay, J. J. Ensberg, M. J. Kleeman and J. H. Seinfeld, Influence of Vapor Wall Loss in Laboratory Chambers on Yields of Secondary Organic Aerosol, Proc. Natl. Acad. Sci. U. S. A., 2014, 111(16), 5802–5807 CrossRef CAS PubMed.
- J. E. Krechmer, D. Pagonis, P. J. Ziemann and J. L. Jimenez, Quantification of Gas-Wall Partitioning in Teflon Environmental Chambers Using Rapid Bursts of Low-Volatility Oxidized Species Generated in Situ, Environ. Sci. Technol., 2016, 50(11), 5757–5765 CrossRef CAS PubMed.
- Y. Huang, R. Zhao, S. M. Charan, C. M. Kenseth, X. Zhang and J. H. Seinfeld, Unified Theory of Vapor-Wall Mass Transport in Teflon-Walled Environmental Chambers, Environ. Sci. Technol., 2018, 52(4), 2134–2142 CrossRef CAS PubMed.
- A. van Donkelaar, R. V. Martin, M. Brauer, R. Kahn, R. Levy, C. Verduzco and P. J. Villeneuve, Global Estimates of Ambient Fine Particulate Matter Concentrations from Satellite-Based Aerosol Optical Depth: Development and Application, Environ. Health Perspect., 2010, 118(6), 847–855 CrossRef CAS PubMed.
- J. E. Shilling, Q. Chen, S. M. King, T. Rosenoern, J. H. Kroll, D. R. Worsnop, P. F. DeCarlo, A. C. Aiken, D. Sueper, J. L. Jimenez and S. T. Martin, Loading-Dependent Elemental Composition of α-Pinene SOA Particles, Atmos. Chem. Phys., 2009, 9, 771–782 CrossRef CAS.
- T. Chen, Y. Liu, B. Chu, C. Liu, J. Liu, Y. Ge, Q. Ma, J. Ma and H. He, Differences of the Oxidation Process and Secondary Organic Aerosol Formation at Low and High Precursor Concentrations, J. Environ. Sci., 2019, 79, 256–263 CrossRef PubMed.
- N. L. Ng, J. H. Kroll, A. W. H. Chan, P. S. Chhabra, R. C. Flagan and J. H. Seinfeld, Secondary Organic Aerosol Formation from M-Xylene, Toluene, and Benzene, Atmos. Chem. Phys., 2007, 7(14), 3909–3922 CrossRef CAS.
- S. H. Jathar, M. A. Miracolo, D. S. Tkacik, N. M. Donahue, P. J. Adams and A. L. Robinson, Secondary Organic Aerosol Formation from Photo-Oxidation of Unburned Fuel: Experimental Results and Implications for Aerosol Formation from Combustion Emissions, Environ. Sci. Technol., 2013, 47(22), 12886–12893 CrossRef CAS PubMed.
- E. J. Dunlea, S. C. Herndon, D. D. Nelson, R. M. Volkamer, F. San Martini, P. M. Sheehy, M. S. Zahniser, J. H. Shorter, J. C. Wormhoudt, B. K. Lamb, E. J. Allwine, J. S. Gaffney, N. A. Marley, M. Grutter, C. Marquez, S. Blanco, B. Cardenas, A. Retama, C. R. Ramos Villegas, C. E. Kolb, L. T. Molina and M. J. Molina, Evaluation of Nitrogen Dioxide Chemiluminescence Monitors in a Polluted Urban Environment, Atmos. Chem. Phys., 2007, 7(10), 2691–2704 CrossRef CAS.
- R. R. Dickerson, D. C. Anderson and X. Ren, On the Use of Data from Commercial NOx Analyzers for Air Pollution Studies, Atmos. Environ., 2019, 214, 116873 CrossRef CAS.
- N. L. Ng, S. C. Herndon, A. Trimborn, M. R. Canagaratna, P. L. Croteau, T. B. Onasch, D. Sueper, D. R. Worsnop, Q. Zhang, Y. L. Sun and J. T. Jayne, An Aerosol Chemical Speciation Monitor (ACSM) for Routine Monitoring of the Composition and Mass Concentrations of Ambient Aerosol, Aerosol Sci. Technol., 2011, 45(7), 780–794 CrossRef CAS.
- R. Fröhlich, M. J. Cubison, J. G. Slowik, N. Bukowiecki, A. S. H. Prévôt, U. Baltensperger, J. Schneider, J. R. Kimmel, M. Gonin, U. Rohner, D. R. Worsnop and J. T. Jayne, The ToF-ACSM: A Portable Aerosol Chemical Speciation Monitor with TOFMS Detection, Atmospheric Measurement Techniques Discussions, 2013, 6767–6814 CrossRef.
-
W. P. L. Carter, Implementation of the SAPRC-99 Chemical Mechanism into the Models-3 Framework, Report to the United States Environmental Protection Agency, January 2000, 29 Search PubMed.
- C. Knote, J. Barré and M. Eckl, BEATBOX v1.0: Background Error Analysis Testbed with Box Models, Geosci. Model Dev., 2018, 561–573 CrossRef CAS.
- L. D. Yee, K. E. Kautzman, C. L. Loza, K. A. Schilling, M. M. Coggon, P. S. Chhabra, M. N. Chan, A. W. H. Chan, S. P. Hersey, J. D. Crounse, P. O. Wennberg, R. C. Flagan and J. H. Seinfeld, Secondary Organic Aerosol Formation from Biomass Burning Intermediates: Phenol and Methoxyphenols, Atmos. Chem. Phys., 2013, 13(16), 8019–8043 CrossRef.
- D. S. Tkacik, A. A. Presto, N. M. Donahue and A. L. Robinson, Secondary Organic Aerosol Formation from Intermediate-Volatility Organic Compounds: Cyclic, Linear, and Branched Alkanes, Environ. Sci. Technol., 2012, 46(16), 8773–8781 CrossRef CAS PubMed.
- W. Li, L. Li, C.-L. Chen, M. Kacarab, W. Peng, D. Price, J. Xu and D. R. Cocker, Potential of Select Intermediate-Volatility Organic Compounds and Consumer Products for Secondary Organic Aerosol and Ozone Formation under Relevant Urban Conditions, Atmos. Environ., 2018, 178, 109–117 CrossRef CAS.
- S. Lunderman, G. M. Fioroni, R. L. McCormick, M. R. Nimlos, M. J. Rahimi and R. W. Grout, Screening Fuels for Autoignition with Small-Volume Experiments and Gaussian Process Classification, Energy Fuels, 2018, 32(9), 9581–9591 CrossRef CAS.
- C. K. Narula, Z. Li, E. M. Casbeer, R. A. Geiger, M. Moses-Debusk, M. Keller, M. V. Buchanan and B. H. Davison, Heterobimetallic Zeolite, InV-ZSM-5, Enables Efficient Conversion of Biomass Derived Ethanol to Renewable Hydrocarbons, Sci. Rep., 2015, 5, 16039 CrossRef CAS PubMed.
- L. Hildebrandt, N. M. Donahue and S. N. Pandis, High Formation of Secondary Organic Aerosol from the Photo-Oxidation of Toluene, Atmos. Chem. Phys., 2009, 9(9), 2973–2986 CrossRef CAS.
- M. R. Canagaratna, J. L. Jimenez, J. H. Kroll, Q. Chen, S. H. Kessler, P. Massoli, L. Hildebrandt Ruiz, E. Fortner, L. R. Williams, K. R. Wilson, J. D. Surratt, N. M. Donahue, J. T. Jayne and D. R. Worsnop, Elemental Ratio Measurements of Organic Compounds Using Aerosol Mass Spectrometry: Characterization, Improved Calibration, and Implications, Atmos. Chem. Phys., 2015, 15(1), 253–272 CrossRef.
- R. Atkinson and J. Arey, Atmospheric Degradation of Volatile Organic Compounds, Chem. Rev., 2003, 103(12), 4605–4638 CrossRef CAS PubMed.
-
US EPA, Estimation Programs Interface Suite™, United States Environmental Protection Agency, Washington, DC, USA, 2019 Search PubMed.
- N. M. Donahue, A. L. Robinson, C. O. Stainer and S. N. Pandis, Coupled Partitioning, Dilution, and Chemical Aging of Semivolatile Organics, Environ. Sci. Technol., 2006, 40(8), 2635–2643 CrossRef CAS PubMed.
- D. Grosjean, Wall Loss of Gaseous Pollutants in Outdoor Teflon Chambers, Environ. Sci. Technol., 1985, 19(11), 1059–1065 CrossRef CAS PubMed.
- A. Matsunaga and P. J. Ziemann‡, Gas-Wall Partitioning of Organic Compounds in a Teflon Film Chamber and Potential Effects on Reaction Product and Aerosol Yield Measurements, Aerosol Sci. Technol., 2010, 44(10), 881–892 CrossRef CAS.
- C. D. Cappa and K. R. Wilson, Multi-Generation Gas-Phase Oxidation, Equilibrium Partitioning, and the Formation and Evolution of Secondary Organic Aerosol, Atmos. Chem. Phys., 2012, 12(20), 9505–9528 CrossRef CAS.
- P. J. Adams and J. H. Seinfeld, Predicting Global Aerosol Size Distributions in General Circulation Models, J. Geophys. Res., 2002, 107(19), 4370 CrossRef.
- J. R. Pierce, I. Riipinen, M. Kulmala, M. Ehn, T. Petäjä, H. Junninen, D. R. Worsnop and N. M. Donahue, Quantification of the Volatility of Secondary Organic Compounds in Ultrafine Particles during Nucleation Events, Atmospheric Chemistry and Physics Discussions, 2011, 14495–14539 CrossRef.
- B. N. Murphy, M. C. Woody, J. L. Jimenez, A. M. G. Carlton, P. L. Hayes, S. Liu, N. L. Ng, L. M. Russell, A. Setyan, L. Xu, J. Young, R. A. Zaveri, Q. Zhang and H. O. T. Pye, Semivolatile POA and Parameterized Total Combustion SOA in CMAQv5.2: Impacts on Source Strength and Partitioning, Atmos. Chem. Phys., 2017, 11107–11133 CrossRef CAS PubMed.
- M. Shrivastava, C. Zhao, R. C. Easter, Y. Qian, A. Zelenyuk, J. D. Fast, Y. Liu, Q. Zhang and A. Guenther, Sensitivity Analysis of Simulated SOA Loadings Using a Variance-Based Statistical Approach, J. Adv. Model. Earth Syst., 2016, 8(2), 499–519 CrossRef.
- S. J. Pai, C. L. Heald, J. R. Pierce, S. C. Farina, E. A. Marais, J. L. Jimenez, P. Campuzano-Jost, B. A. Nault, A. M. Middlebrook, H. Coe, J. E. Shilling, R. Bahreini, J. H. Dingle and K. Vu, An Evaluation of Global Organic Aerosol Schemes Using Airborne Observations, Atmos. Chem. Phys., 2020, 20(5), 2637–2665 CrossRef CAS.
- S. Eluri, C. D. Cappa, B. Friedman, D. K. Farmer and S. H. Jathar, Modeling the Formation and Composition of Secondary Organic Aerosol from Diesel Exhaust Using Parameterized and Semi-Explicit Chemistry and Thermodynamic Models, Atmos. Chem. Phys., 2018, 18(19), 13813–13838 CrossRef CAS.
- S. H. Jathar, C. D. Cappa, A. S. Wexler, J. H. Seinfeld and M. J. Kleeman, Simulating Secondary Organic Aerosol in a Regional Air Quality Model Using the Statistical Oxidation Model-Part 1: Assessing the Influence of Constrained Multi-Generational Ageing, Atmos. Chem. Phys., 2016, 16(4), 2309–2322 CrossRef CAS.
- C. D. Cappa, S. H. Jathar, M. J. Kleeman, K. S. Docherty, J. L. Jimenez, J. H. Seinfeld and A. S. Wexler, Simulating Secondary Organic Aerosol in a Regional Air Quality Model Using the Statistical Oxidation Model-Part 2: Assessing the Influence of Vapor Wall Losses, Atmos. Chem. Phys., 2016, 16(5), 3041–3059 CrossRef CAS.
- A. Akherati, C. D. Cappa, M. J. Kleeman, K. S. Docherty, J. L. Jimenez, S. M. Griffith, S. Dusanter, P. S. Stevens and S. H. Jathar, Simulating Secondary Organic Aerosol in a Regional Air Quality Model Using the Statistical Oxidation Model-Part 3: Assessing the Influence of Semi-Volatile and Intermediate-Volatility Organic Compounds and NOx, Atmos. Chem. Phys., 2019, 19(7), 4561–4594 CrossRef CAS.
- Q. Bian, A. A. May, S. M. Kreidenweis and J. R. Pierce, Investigation of Particle and Vapor Wall-Loss Effects on Controlled Wood-Smoke Smog-Chamber Experiments, Atmos. Chem. Phys., 2015, 15(19), 11027–11045 CrossRef CAS.
- C. Song, K. Na, B. Warren, Q. Malloy and D. R. Cocker, Secondary Organic Aerosol Formation from the Photooxidation of P- and O-Xylene, Environ. Sci. Technol., 2007, 41(21), 7403–7408 CrossRef CAS PubMed.
- K. M. Shakya and R. J. Griffin, Secondary Organic Aerosol from Photooxidation of Polycyclic Aromatic Hydrocarbons, Environ. Sci. Technol., 2010, 44(21), 8134–8139 CrossRef CAS PubMed.
- A. M. Middlebrook, R. Bahreini, J. L. Jimenez and M. R. Canagaratna, Evaluation of Composition-Dependent Collection Efficiencies for the Aerodyne Aerosol Mass Spectrometer Using Field Data, Aerosol Sci. Technol., 2012, 46(3), 258–271 CrossRef CAS.
- Y. B. Lim and P. J. Ziemann, Effects of Molecular Structure on Aerosol Yields from OH Radical-Initiated Reactions of Linear, Branched, and Cyclic Alkanes in the Presence of NOx, Environ. Sci. Technol., 2009, 43(7), 2328–2334 CrossRef CAS PubMed.
- H. J. Chacon-Madrid, K. M. Henry and N. M. Donahue, Photo-Oxidation of Pinonaldehyde at Low NOx: From Chemistry to Organic Aerosol Formation, Atmospheric Chemistry and Physics Discussions, 2012, 7727–7752 CrossRef.
- H. J. L. Forstner, R. C. Flagan and J. H. Seinfeld, Secondary Organic Aerosol from the Photooxidation of Aromatic Hydrocarbons: Molecular Composition, Environ. Sci. Technol., 1997, 31(5), 1345–1358 CrossRef CAS.
- A. W. H. Chan, K. E. Kautzman, P. S. Chhabra, J. D. Surratt, M. N. Chan, J. D. Crounse, A. Kürten, P. O. Wennberg, R. C. Flagan and J. H. Seinfeld, Secondary Organic Aerosol Formation from Photooxidation of Naphthalene and Alkylnaphthalenes: Implications for Oxidation of Intermediate Volatility Organic Compounds (IVOCs), Atmos. Chem. Phys., 2009, 9(9), 3049–3060 CrossRef CAS.
- L. Li, P. Tang, S. Nakao and D. R. Cocker III, Impact of Molecular Structure on Secondary Organic Aerosol Formation from Aromatic Hydrocarbon Photooxidation under Low-NOx Conditions, Atmos. Chem. Phys., 2016, 16(17), 10793–10808 CrossRef CAS.
- C. M. Strollo and P. J. Ziemann, Products and Mechanism of Secondary Organic Aerosol Formation from the Reaction of 3-Methylfuran with OH Radicals in the Presence of NOx, Atmos. Environ., 2013, 77, 534–543 CrossRef CAS.
- C. Bloss, V. Wagner, M. E. Jenkin, R. Volkamer, W. J. Bloss, J. D. Lee, D. Heard, K. Wirtz, M. Martin-Reviejo and G. Rea,
et al., Development of a Detailed Chemical Mechanism (MCMv3. 1) for the Atmospheric Oxidation of Aromatic Hydrocarbons, Atmos. Chem. Phys., 2005, 5(3), 641–664 CrossRef CAS.
- I. M. Al-Naiema, H. M. Roppo and E. A. Stone, Quantification of Furandiones in Ambient Aerosol, Atmos. Environ., 2017, 153, 41–46 CrossRef CAS.
- T. Joo, J. C. Rivera-Rios, M. Takeuchi, M. J. Alvarado and N. L. Ng, Secondary Organic Aerosol Formation from Reaction of 3-Methylfuran with Nitrate Radicals, ACS Earth Space Chem., 2019, 3(6), 922–934 CrossRef CAS.
- C. L. Loza, J. S. Craven, L. D. Yee, M. M. Coggon, R. H. Schwantes, M. Shiraiwa, X. Zhang, K. A. Schilling, N. L. Ng, M. R. Canagaratna, P. J. Ziemann, R. C. Flagan and J. H. Seinfeld, Secondary organic aerosol yields of 12-carbon alkanes, Atmos. Chem. Phys., 2014, 14, 1423–1439 CrossRef.
- T. Chen, Y. Liu, C. Liu, J. Liu, B. Chu and H. He, Important Role of Aromatic Hydrocarbons in SOA Formation from Unburned Gasoline Vapor, Atmos. Environ., 2019, 201, 101–109 CrossRef CAS.
- D. R. Gentner, G. Isaacman, D. R. Worton, A. W. H. Chan, T. R. Dallmann, L. Davis, S. Liu, D. A. Day, L. M. Russell, K. R. Wilson, R. Weber, A. Guha, R. A. Harley and A. H. Goldstein, Elucidating Secondary Organic Aerosol from Diesel and Gasoline Vehicles through Detailed Characterization of Organic Carbon Emissions, Proc. Natl. Acad. Sci. U. S. A., 2012, 109(45), 18318–18323 CrossRef CAS PubMed.
- E. Athanasopoulou, H. Vogel, B. Vogel, A. P. Tsimpidi, S. N. Pandis, C. Knote and C. Fountoukis, Modeling the Meteorological and Chemical Effects of Secondary Organic Aerosols during an EUCAARI Campaign, Atmos. Chem. Phys., 2013, 13(2), 625–645 CrossRef.
- J. R. Odum, T. P. W. Jungkamp, R. J. Griffin, H. J. L. Forstner, R. C. Flagan and J. H. Seinfeld, Aromatics, Reformulated Gasoline, and Atmospheric Organic Aerosol Formation, Environ. Sci. Technol., 1997, 31(7), 1890–1897 CrossRef CAS.
- P. S. Monks, A. T. Archibald, A. Colette, O. Cooper, M. Coyle, R. Derwent, D. Fowler, C. Granier, K. S. Law, G. E. Mills, D. S. Stevenson, O. Tarasova, V. Thouret, E. von Schneidemesser, R. Sommariva, O. Wild and M. L. Williams, Tropospheric Ozone and Its Precursors from the Urban to the Global Scale from Air Quality to Short-Lived Climate Forcer, Atmos. Chem. Phys., 2015, 15, 8889–8973 CrossRef CAS.
- A. C. Lewis, N. Carslaw, P. J. Marriott, R. M. Kinghorn, P. Morrison, A. L. Lee, K. D. Bartle and M. J. Pilling, A Larger Pool of Ozone-Forming Carbon Compounds in Urban Atmospheres, Nature, 2000, 405(6788), 778–781 CrossRef CAS PubMed.
- K. Sato, A. Takami, Y. Kato, T. Seta, Y. Fujitani, T. Hikida, A. Shimono and T. Imamura, AMS and LC/MS Analyses of SOA from the Photooxidation of Benzene and 1,3,5-Trimethylbenzene in the Presence of NOx: Effects of Chemical Structure on SOA Aging, Atmos. Chem. Phys., 2012, 12(10), 4667–4682 CrossRef CAS.
- E. A. Bruns, I. El Haddad, J. G. Slowik, D. Kilic, F. Klein, U. Baltensperger and A. S. H. Prévôt, Identification of Significant Precursor Gases of Secondary Organic Aerosols from Residential Wood Combustion, Sci. Rep., 2016, 6, 27881 CrossRef CAS PubMed.
- L. E. Hatch, W. Luo, J. F. Pankow, R. J. Yokelson, C. E. Stockwell and K. C. Barsanti, Identification and Quantification of Gaseous Organic Compounds Emitted from Biomass Burning Using Two-Dimensional Gas Chromatography–time-of-Flight Mass Spectrometry, Atmos. Chem. Phys., 2015, 1865–1899 CrossRef CAS.
- C. E. Stockwell, P. R. Veres, J. Williams and R. J. Yokelson, Characterization of Biomass Burning Emissions from Cooking Fires, Peat, Crop Residue, and Other Fuels with High-Resolution Proton-Transfer-Reaction Time-of-Flight Mass Spectrometry, Atmos. Chem. Phys., 2015, 15(2), 845–865 CrossRef.
- A. R. Koss, K. Sekimoto, J. B. Gilman, V. Selimovic, M. M. Coggon, K. J. Zarzana, B. Yuan, B. M. Lerner, S. S. Brown and J. L. Jimenez, Others. Non-Methane Organic Gas Emissions from Biomass Burning: Identification, Quantification, and Emission Factors from PTR-ToF during the FIREX 2016 Laboratory Experiment, Atmos. Chem. Phys., 2018, 18(5), 3299–3319 CrossRef CAS.
- W. P. L. Carter, Development of the SAPRC-07 Chemical Mechanism, Atmos. Environ., 2010, 44(40), 5324–5335 CrossRef CAS.
Footnote |
† Electronic supplementary information (ESI) available: Details on HONO synthesis, SAPRC modeling, particle wall losses, vapor wall losses, and fuel composition and properties. See DOI: 10.1039/d0em00103a |
|
This journal is © The Royal Society of Chemistry 2020 |
Click here to see how this site uses Cookies. View our privacy policy here.