Establishing reactivity descriptors for platinum group metal (PGM)-free Fe–N–C catalysts for PEM fuel cells†
Received
31st March 2020
, Accepted 24th June 2020
First published on 24th June 2020
Abstract
We report a comprehensive analysis of the catalytic oxygen reduction reaction (ORR) reactivity of four of today's most active benchmark platinum group metal-free (PGM-free) iron/nitrogen doped carbon electrocatalysts (Fe–N–Cs). Our analysis reaches far beyond previous such attempts in linking kinetic performance metrics, such as electrocatalytic mass-based and surface area-based catalytic activity with previously elusive kinetic metrics such as the active metal site density (SD) and the catalytic turnover frequency (TOF). Kinetic ORR activities, SD and TOF values were evaluated using in situ electrochemical NO2− reduction as well as an ex situ gaseous CO cryo chemisorption. Experimental ex situ and in situ Fe surface site densities displayed remarkable quantitative congruence. Plots of SD versus TOF (“reactivity maps”) are utilized as new analytical tools to deconvolute ORR reactivities and thus enabling rational catalyst developments. A microporous catalyst showed large SD values paired with low TOF, while mesoporous catalysts displayed the opposite. Trends in Fe surface site density were linked to molecular nitrogen and Fe moieties (D1 and D2 from 57Fe Mössbauer spectroscopy), from which pore locations of catalytically active D1 and D2 sites were established. This cross-laboratory analysis, its employed experimental practices and analytical methodologies are expected to serve as a widely accepted reference for future, knowledge-based research into improved PGM-free fuel cell cathode catalysts.
Broader context
Polymer electrolyte membrane fuel cells (PEMFC) have reached the commercial stage and ever wider deployment is imminent. To further reduce the loading of platinum group metal (PGM) catalysts in PEMFC electrodes, PGM-free, iron and nitrogen-doped carbon oxygen reduction (ORR) electrocatalysts (Fe–N–C) were developed over past decades. Recent advances in activity and stability of Fe–N–C are impressive, yet methods to evaluate the number of catalytic active Fe sites at the surface and intrinsic turn over frequency remained elusive. This changed with the advent of CO cryo-sorption and in situ nitrite stripping techniques that yielded these intrinsic reactivity descriptors. Never before, however, have these two complementary specific adsorption/stripping techniques been compared and combined with other chemical and spectroscopic analytics for an in-depth analysis of catalytic reactivity of Fe–N–C ORR electrocatalysts. The present study addresses this issue and presents a comprehensive analysis of the reactivity of the four state-of-the-art Fe–N–C PEMFC electrocatalysts. The study provides a deeper understanding of the origin and difference in catalytic performance through the combination of a host of different surface sensitive and bulk analysis methods. The methodologies and analyses of this benchmark catalyst study will benefit future developments in Fe–N–C catalysis.
|
1. Introduction
Currently, proton exchange membrane fuel cells (PEMFCs) are on the verge of massive deployment, in the transport sector in particular, but still require expensive and scarce platinum-group-metal (PGM)-based electrocatalysts to promote the sluggish oxygen reduction reaction (ORR) occurring at the cathode of PEMFCs.1–8 This is the reason why much international effort is now being devoted to a rational design and development of lower-cost PGM-free ORR electrocatalysts. Large research consortia, such as the ElectroCat network9 funded by the US Department of Energy and the EU projects CRESCENDO10 and PEGASUS11 funded by the Fuel Cells and Hydrogen Joint Undertaking (FCH-JU), are working to meet specific performance targets. The latter are defined so that fuel cell stacks with PGM-free ORR catalysts become cost- and performance-competitive with PGM-based catalysts, even for the highly demanding automotive application.4–6,12–22 The most prominent example of PGM-free ORR electrocatalysts for acidic medium is the family of iron- (or cobalt-) and nitrogen-doped high surface area carbon matrix, typically referred as “Fe–N–C” catalysts, with atomically-dispersed Fe cations coordinated with nitrogen atoms as the recognized most active sites.5,23–32 Unlike PGM-based single atom catalysts, where the atoms exist in a carbon matrix as a sole atoms33,34 or dimeric compounds,35 iron generally has to be coordinated with hetero atoms. Several general approaches have been established in order to control the carbon micro and/or meso-porosity in M–N–C catalysts, a key for high performance: functionalisation of microporous carbon blacks with metal and N precursors,4 hard-templating of C and N precursors with e.g. silica,36 adding porogens before pyrolysis,37 using reactive gases such as ammonia or CO2 during pyrolysis,38 and last but not least by soft templating with e.g. metal organic frameworks39,40 or porous organic polymers.36,40–60 Despite the impressive achievements in the catalytic performance of Fe–N–C catalysts, further improvements in their ORR activity and, in particular, durability are needed before their large-scale deployment in commercial PEMFCs becomes a reality.12,26,61–63
Over the past decades, studies to identify more active Fe–N–C catalysts have largely relied on empirical approaches involving the systematic variation of elemental precursors and/or synthesis conditions to prepare Fe–N–C materials and their correlation with the resulting kinetic current density (Jkin) and other lump performance metrics of ORR catalysts.7,36,64–66 While this approach has had some success in the early stages of Fe–N–C materials development, it now seems to have reached its limitation, with stalled progress in the power and durability performance of Fe–N–C cathodes in PEMFCs in the last years, despite intense international efforts. Novel and more rational approaches are needed in order to deconvolute the overall activity and durability of Fe–N–C catalysts into the contributions arising from different Fe-based active sites, in order to identify the most active and/or most durable sites and to develop synthetic strategies to selectively optimize the number of such sites.31,67 The first step towards this goal implies the development of experimental methods that evaluate the number of Fe-based catalytic sites that are located at the surface of the catalyst (site density, SD). The SD value is then combined with the kinetic current density, Jkin, and elemental electric charge, e, in order to extract the average intrinsic turn over frequency (TOF) of the Fe-based active sites in a given Fe–N–C catalyst, according to68
|
Jkin [A g−1] = TOF [electron site−1 s−1] × SD [site g−1] × e [C electron−1] | (1) |
TOF and SD are fundamental descriptors of catalytic reactivity and can provide guidelines for the synthesis of more active catalysts. Efforts to improve the overall activity of a catalyst may now focus on synthetic strategies to increase, separately or combined, the SD value or to enhance the intrinsic TOF value of the active sites.
Theoretical–computational research has offered a much clearer, albeit not fully resolved, picture of the chemical structure of favorable, catalytically active Fe–Nx single metal sites.16,69 Advanced experimental analytical techniques such as 57Fe Mössbauer spectroscopy and high resolution STEM-EELS microscopy have now qualitatively proven the existence of such sites in active Fe–N–C materials.13–15,26,60,70–73 A serious hurdle in the rational improvement of the catalytic activity of Fe–N–C catalysts, however, has been the lack of suitable methods that accurately enumerate the electrochemically accessible Fe–Nx sites (SD). Even for model Fe–N–C materials comprising only Fe–Nx sites, the SD value cannot be accessed with the sole knowledge of the total Fe content, due to the location of a significant fraction of Fe–Nx sites not only on the surface but also in the bulk of the carbon matrix. This issue results from the pyrolytic process employed to form such active sites.
A range of spectroscopic methods based on X-rays and γ-rays have been applied in order to probe and quantify bulk and/or surface Fe-based sites, namely X-ray photoelectron spectroscopy (XPS), X-ray absorption spectroscopy (XAS) and 57Fe Mössbauer spectroscopy.7,13,15,31,63,70,71 However, there exist inherent shortcomings for each of these analysis methods. XAS and 57Fe Mössbauer spectroscopy are inherently bulk methods, so they identify both electrochemically accessible and inaccessible Fe-based sites. X-ray photoelectron spectroscopy (XPS) are element specific but not surface sensitive for carbon-based materials with high surface area, due to the escape path of several nm of photoelectrons through the carbon matrix.74 Synchrotron-based XPS with tuned energy of the X-rays has improved the surface sensitivity for carbon-based materials, and been successfully applied to study Fe–N–C materials.75,76 While synchrotron-based XPS can give information on surface elemental composition, it however cannot yield absolute numbers of metal-based sites in the overall sample. In addition, while XPS successfully distinguishes the presence of different oxidation states of a metal, it is not powerful at discriminating between different environments. For example, iron in ferric oxide and Fe(III)Nx sites cannot be distinguished with XPS, and the root for this is that the detected photoelectrons come from the core.
Adsorption/desorption techniques involving probe molecules are inherently well suited to count sites on the surface, yet often lack chemical specificity.77 Probe molecules such as CO,78 NO,79 CN− (ref. 80) or SCN− have been explored as surface probes for Fe–N–C materials under electrochemicalconditions, however none was successfully applied for a quantitative evaluation of surface sites.81 For example, both CN− and tris(hydroxymethyl)aminomethane (Tris) have been successfully employed in partial poisoning studies of Fe–Nx surface sites of Fe–N–C catalysts.80,82 This implies that counting the number of Tris molecules or cyanide ions irreversibly adsorbed on Fe–N–C (after washing the surface with electrolyte free of such probe species) would underestimate the total number of surface-located Fe-based sites, due to too weak adsorption on some sites. Recently, new complementary adsorption/desorption techniques were specifically developed for Fe–N–C materials and validated, one based on low-temperature CO adsorption83 and the other on ambient-temperature NO2−/NO adsorption.84 The ex situ, low-temperature CO cryo pulse chemisorption/desorption technique featured good specificity to Fe sites and resulted in reproducible SD values for different single metal active sites, in particular for Fe–N–C materials.68,85,86 The technique relies on rapid adsorption rates and strong binding at −80 °C between CO molecules and atomically dispersed single Fe–Nx sites embedded in a carbon framework. Possible pitfalls of this technique include overestimation, because it is not possible to show that ORR is blocked by CO and due to the possibility of single sites to bind more than one CO molecule. Also, initial poisoning of a fraction of the single Fe sites may alter the subsequent CO uptake amount, leading to undersampling. A careful pretreatment procedure is therefore necessary to desorb oxygenates quantitatively from all surface Fe-based sites site prior to CO uptake. A standardized thermal pretreatment protocol of Fe–N–C now ensures reproducible CO uptake values on oxygen-free Fe(II)Nx sites.85,86
Second, a complementary in situ electrochemical nitrite adsorption/NO electrostripping technique was put forward by Kucernak's group.77 The method relies on the very specific and strong interaction of Fe–Nx sites with nitrite anions resulting in NO adsorption, followed by electrochemical reductive stripping of NO into ammonia.87 Thus, a quantification of Fe–Nx sites is achieved by means of the stripping charge of the five-electron process. Issues related to this method include the fact that it requires a moderately acidic pH of about 5, which is less acidic than the conditions prevailing at a PEMFC cathode. Furthermore, although the majority of ORR current is blocked by NO adsorption (>70%), some ORR current remains suggesting the presence of multiple types of Fe–Nx sites. NO may poison only a fraction of the exposed sites due to its very high chemical specificity, which leads to undersampling. Together, the ex situ CO cryo probe technique and the in situ NO probe technique offer a powerful pair of complementary physico-chemical strategies to quantify the number of Fe–Nx sites on the surface of Fe–N–C catalysts. Together, both methods may yield a balanced and reliable range of quantitative values for (i) the SD and (ii) after combination with ORR activity measurements, for the TOF. This enables a rational, knowledge-driven improvement of the reactivity of Fe–N–C catalysts. However, hitherto these two SD probe techniques have never been combined to study and analyze the catalytic ORR reactivity of a same set of PGM-free catalysts to extract their SD and TOF values and to cross-compare the values obtained with the two techniques. Likewise, no study has hitherto attempted to draw useful correlations between the composition and structural or morphological characteristics of Fe–N–C catalysts and their fundamental reactivity parameters such as TOF and SD. The objectives of this contribution are to compare the SD and TOF values determined for several Fe–N–C catalysts with the nitrite stripping and CO cryo chemisorption techniques, as well as to establish novel structure–reactivity correlations, deconvoluting the reactivity into SD and TOF values, moving beyond the lump ORR activity descriptor used hitherto.
Here, we present the first comprehensive analysis of trends in the two fundamental descriptors of the electrocatalytic reactivity of today's state-of-art Fe–N–C catalysts, namely SD and TOF, as measured with the ex situ CO cryo probe technique and the in situ NO probe techniques. We then establish novel correlations between SD and/or TOF descriptors and several descriptors of the structure, morphology and/or elemental composition of Fe–N–C catalysts. What sets this study apart is not only the fact that the catalytic ORR reactivity of four of the most active Fe–N–C catalysts is deconvoluted into SD and TOF contributions, but also that the presented data, trends and conclusions are based on the combination of the independent analyses of four different laboratories. Furthermore, outcomes include both new and in part quite surprising correlations between the SD data resulting from ex situ CO and in situ NO techniques, as well as and more importantly previously unavailable fundamental insights into the origin of the catalytic ORR reactivity of these four benchmark catalysts.
More specifically, starting from the rotating ring-disk electrode (RRDE) based ORR mass activity (MA), we derive quantitative values for (i) SD for each benchmark catalyst, on a mass-basis and/or surface-area basis, and (ii) TOF values. In parallel, the Fe–Nx coordination environment and elemental composition in the bulk of the sample were determined by 57Fe Mössbauer spectroscopy and XPS, respectively. The pore structure and specific surface area were evaluated with nitrogen sorption isotherms. Previously inaccessible mass activity maps were established from the knowledge of the SD and TOF values of the catalysts, on the one hand, and between SD or TOF values and the experimentally determined type and quantity of Fe–Nx sites, on the other hand. Our analyses offer rational guidelines how to achieve further improvements in the PGM-free ORR activity in order to reach future targeted performance characteristics.
2. Experimental section
The present cross-laboratory study was carried out at the University of Padua, Imperial College London, the Institut Charles Gerhardt (CNRS – University of Montpellier – ENSCM), and at the Technical University Berlin. Some of the analyses were duplicated at different laboratories using distinct equipment. The experimental details are described below, by method and/or laboratory.
2.1 Benchmark catalysts
The four benchmark Fe–N–C catalysts investigated here were sourced from different laboratories and were used as received. Their detailed synthesis have been published in the literature. They are currently considered best-in-class Fe–N–C catalysts for PEMFC applications. They include a ZIF-derived catalyst from CNRS/University of Montpellier (henceforth referred to as CNRS),88,89 a polymerized di-amino naphthalene based catalysts from Imperial College London (ICL),77 two catalysts synthesized via hard templating with fumed silica, one from the University of New Mexico (UNM) and another one from Pajarito Powder Inc. (PAJ). The latter can be obtained as a commercial product under the name PMF-011904.
2.2 Physicochemical characterization
Nitrogen physisorption.
At one laboratory, nitrogen physisorption was performed in a Micromeritics ASAP 2020 instrument. 100 to 150 mg of the catalyst was inserted in a sample tube with glass wool and filling rods on top. Before the measurement, the samples were pre-treated under vacuum (300 °C, 20 h) to remove any species adsorbed on the sample. After cooling to room temperature, helium was backfilled into the sample tube. During the measurements, the sample was cooled to 77 K (liquid nitrogen). The Brunauer–Emmett–Teller (BET) equation was used to estimate the total surface area. Non-local density functional theory (2D-NLDFT) was used to model isotherms to calculate pore size distributions of microporous carbon materials with pores from 0.35 to 25 nm. For the analysis, an assumption of 2D model of finite slit pores having a diameter-to-width aspect ratio of 4–6–12 was made. At another laboratory, nitrogen physisorption was conducted on a Micromeritics Tristar II 3020. The analysis temperature was 77 K and the BET equation was also used to estimate the total surface area. The best region for the linear fit was determined by the Rouquerol method.90 Samples were degassed and dried overnight at 300 °C under flowing nitrogen prior to the measurement. Gases used were nitrogen (BIP plus-X47S) for drying and adsorption and He (BIP plus-X47S) for free-space measurement. Pore volume was determined as per NLDFT as implemented in the software Micromeritics “Microactive for Tristar II”. The model was based on a slit shaped pore.
X-ray photoelectron spectroscopy.
The XPS measurements were carried out in a custom-designed UHV system equipped with an EA 125 Omicron electron analyzer ending with a five channeltron detector, working at a base pressure of 10−10 mbar. The photoemission spectra were collected at room temperature using the Mg Kα line (hν = 1253.6 eV) of a non-monochromatised dual-anode DAR400 X-ray source. The survey spectra were acquired using 0.5 eV energy step, 0.5 s collection time, and 50 eV pass energy. Additionally, single components (C 1s, O 1s, N 1s, Fe 2p3/2) were acquired with the same parameters in order to increase accuracy of the calculation of surface composition (i.e. Fe 2p3/2 line was acquired 60 times). High resolution spectra were acquired using 0.1 eV energy steps, 0.5 s collection time, and 20 eV pass energy for the curves fitting.
57Fe Mössbauer spectroscopy.
57Fe Mössbauer spectra were measured with a Rh matrix 57Co source. The measurements were performed keeping both the source and the absorber at room temperature, unless otherwise mentioned. The spectrometer was operated with a triangular velocity waveform, and a gas filled proportional counter was used for the detection of the γ-rays. Velocity calibration was performed with an α-Fe foil. The spectra were fitted individually with appropriate combinations of Lorentzian lines. In this way, spectral parameters such as the isomer shift (IS) and the electric quadrupole splitting (QS), and the relative resonance areas (A) of the different components were determined. Isomer shift values are reported relative to α-Fe.
Elemental analysis (EA).
Elemental analysis was carried out using a Thermo Scientific Flash 2000 analyser.
Inductively coupled plasma-mass spectrometry (ICP-MS).
An Agilent Technologies 7700x ICP-MS was employed for inductively coupled plasma mass spectroscopy analysis. The samples (15 mg) for ICP analysis were treated with 2 mL of nitric acid (69% w/w) and heated at 100 °C for 1 h. The mixtures were diluted up to 40 g with Milli-Q water and after filtration, 2 mL of the solutions were analyzed. For ICP analysis, another protocol was tested using a microwave system CEM EXPLORER SP.D PLUS at a heating rate of 40 °C min−1 from room temperature to 220 °C with a pressure of 400 psi and a power a 300 W. In the latter method the samples were dispersed in 2 mL of nitric acid, 6 mL of hydrochloric acid (37% w/w) and 3 mL of sulfuric acid (93–98% w/w).
2.3 Electrochemical measurements
The electrochemical measurements consisted of the determination of the catalytic ORR activity and selectivity using rotating ring-disk electrode (RRDE) set-ups at two different geometric catalyst loadings of 0.2 and 0.8 mg cm−2 on the disk electrode, in order to study the influence of layer thickness on the catalyst performance. All laboratories involved in this study performed RRDE testing, and error bars originated from the variations of data across the laboratories.
Ink formulations.
The catalyst ink consisted of a slurry of the catalyst, isopropanol and ultrapure water in a water to isopropanol mass ratio of 1
:
1, and Nafion (5 wt%, Sigma-Aldrich). The catalyst content was either 0.5 wt% (0.2 mg cm−2 loading) or 2.0 wt% (0.8 mg cm−2 loading) of the total ink with a mass ratio of water to catalyst of 1
:
10 and 1
:
40 respectively. The ionomer to catalyst ratio is 1
:
2. The suspension was ultra-sonicated until a stable suspension was reached.
Electrochemical set-ups.
The electrolyte was 0.5 M H2SO4 (ANALR grade or EMSURE Merck Millipore, as available to all of the project partners). All the measurements were performed in a glass jacket cell at 25 °C with a reversible hydrogen electrode (RHE) reference electrode, a graphite counter electrode, and a glassy carbon disk with a platinum or gold ring as working electrode. The ring-disk electrodes were polished and cleaned in an ultra-sonication bath with isopropanol and ultrapure water. The cleaned electrodes were dried in nitrogen and the ink was deposited on the disk surface and dried at room temperature or in an oven at 50 °C.
Break-in procedures.
The activation of the catalyst was performed in N2-saturated electrolyte via cyclic voltammetry (0.0–1.0 VRHE, 10 mV s−1) with a minimum of five cycles until the change in capacitance in the 0.95–1.0 VRHE region between two successive scans was less than 2%.
ORR activity and selectivity measurements.
Cyclic voltammetry was performed in an O2-saturated electrolyte (0.925–0.00 VRHE, 1–2 mV s−1, rotation rate 1600 rpm, ring potential 1.5 VRHE) starting from open circuit potential (OCP) to the lower potential of 0.0 VRHE and a back scan to 0.925 VRHE. The scan rates are low enough to neglect non-faradaic currents.
Accelerated stress tests (AST).
AST was performed with a catalyst loading of 0.2 mg cm−2 in sequence with the oxygen reduction reaction (ORR) activity measurement. The electrolyte was saturated with nitrogen and cyclic voltammetry applied (0.60–0.925 VRHE, 100 mV s−1, 10
000 cycles).
Data analysis.
For the determination of the kinetic current density Jkin the forward and backward scans of the cyclic voltammetry of the disc current densities, J, were first averaged to correct for minimum interfacial capacitance at 1–2 mV s−1 and/or memory effects due to the direction of the scan. Then, the Koutecký–Levich equation was used to calculate the kinetic current density (Jkin) from the averaged geometric current density, J, at 0.80 and 0.85 VRHE, according to |
 | (2) |
|
 | (3) |
where Jlim is the diffusion-limited current density, measured at 0.20 VRHE. The following formula was used for quantifying the H2O2 production, with N being the collection efficiency of the ring-disk-electrode: |
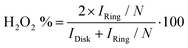 | (4) |
2.4
Ex situ and in situ evaluation of Fe surface site density (SD) and turnover frequency
CO cryo chemisorption measurements.
CO pulse chemisorption and temperature programmed desorption (TPD) were performed in a Thermo Scientific TPD/R/O 110 instrument. A weighed mass of 100 to 150 mg of catalyst was inserted between two pieces of quartz wool at the bottom of the internal quartz bulb. Before the measurement, the catalyst was pre-treated to remove any species strongly adsorbed on the metal-based sites on the surface, in particular O2. Pre-treatment of the catalyst begins with cleaning of the lines with helium (20 cm3 min−1, 30 min) and a consecutive ramp heating from 30 to 600 °C (10 °C min−1, 15 min hold time at 600 °C) and followed by cooling to room temperature. Pulse chemisorption at −80 °C (dry ice and acetone) consisted of 10 min line flushing (helium, 20 cm3 min−1), followed by six consecutive CO pulses injected by the automated sample loop (helium as a carrier gas, 20 cm3 min−1, loop volume was determined to be 0.341 mL) in intervals of 25 min.68,85 Prior to TPD analysis, three consecutive CO pulses are performed to ensure the saturation of the active centres with CO. Thereafter TPD (−80 °C to 600 °C, 10 °C min−1, hold time 10 min, He as carrier, 20 cm3 min−1) with a consecutive cooling to 30 °C (20° min−1) were performed.
For the catalyst surface areas and masses employed in this study, the CO cryo adsorption reached saturation after 3 pulses. The difference in peak areas (ΔA), corresponding to the adsorbed molar CO amount, can be calculated from the six individual baseline-corrected integral pulse areas A1,sample to A6,sample (formal physical unit of the integrated detector signal is [mV s]) according to:
|
 | (5) |
Using the injection of a known volume of CO gas, a calibration constant cf ≈ 4.14 × 10−7 mmol per unit area was derived. The calibration factor was henceforth used for the conversion between integral peak areas and molar CO amounts. In particular, the molar amount of adsorbed CO (NCO,ad), also referred to as the molar CO uptake, is the product of cf and ΔA. The mass-based molar amount of adsorbed CO, nCO, was then calculated by dividing by the mass of the catalyst sample inserted in the quartz tube of the chemisorption reactor, mcat, according to |
NCO,ad [nmol] = cf × ΔA × 106 | (6) |
|
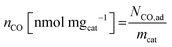 | (7) |
The mass-based site density with CO chemisorption (SDmass(CO)) was then calculated from nCOvia Avogadro's constant (NA) according to |
SDmass(CO) [sites gcat−1] = nCO [nmol mgcat−1] × NA [site mol−1] × 10−6 | (8) |
BET surface area-based SD values, SDBET(CO), with units of [site m−2], were obtained by dividing SDmass(CO) by the mass-specific surface area, ABET [m2 gcat−1].
The turnover frequency TOF(CO) was calculated from the catalyst mass-based kinetic current, Jkin,mass [A gcat−1], and the CO uptake-derived catalyst mass-based surface site density, SDmass(CO), or the adsorbed molar uptake of CO, nCO, according to
|
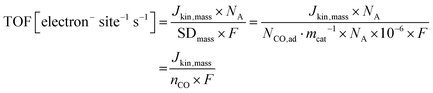 | (9) |
Jkin,mass was evaluated from the ratio between the mass-transport corrected geometric current density, Jkin [mA cm−2] and the geometric catalyst loading, Lgeo [mgCat cm−2] at either 0.80 or 0.85 VRHE.
The same turnover frequency (TOF) resulted from the mass-transport corrected, BET surface area-based kinetic current density Jkin,BET [mA mcat−2] and the BET surface area-based SD values, SDBET, according:
|
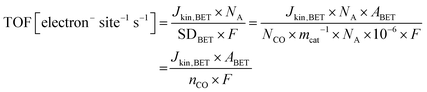 | (10) |
Nitrite reduction stripping.
Measurements were conducted with a conventional RRDE (Pine Instruments, model AFE6R1AU, with a mirror polished glassy carbon disk electrode and rotator model AFMSRCE), where the catalyst is deposited on the glassy carbon disk electrode. The catalyst loading was fixed at 0.2 mg cm−2 and 0.5
M acetate buffer at pH 5.2 was utilized as electrolyte. The detailed experimental steps were performed according to our previously reported steps including cleaning protocol, measurement protocol, and poisoning protocol.77,87 It is important to utilize a current integrator (or analog linear scan generator) when performing the stripping measurements as normal staircase voltammetry will not correctly measure the charges associated with these processes.
The number of stripped molecules was calculated via the electrochemical nitrite reduction stripping charge on Fe(II)Nx sites (Qstrip), assuming that the adsorption and stripping process follow these steps:
|
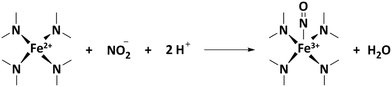 | (11) |
|
 | (12) |
The above mechanism identifies five electrons with the reduction of one adsorbed NO per site (nstrip = 5). Then, the areal site density, SDBET(NO2−), that is the number of Fe-based surface sites normalized to the catalyst surface area, was calculated as following: |
 | (13) |
where Qstrip is the coulometric charge in units of Coulomb associated with the NO stripping peak, nstrip is the number of electrons associated with the reduction of one nitrite ion, mcat is the mass of the catalyst, and ABET is the mass-specific surface area. Likewise, mass-based Fe surface site density, SDmass(NO2−), that is, the number of active sites per catalyst mass, was calculated as: |
 | (14) |
Nitrite adsorption significantly decreases the ORR performance of the catalyst, but does not entirely block ORR activity, leading to a 70–80% decrease in ORR activity over the relevant range of potentials (0.8–0.9 VRHE). This suggests that there is a range of sites responsible for the ORR activity, and that nitrite adsorption poisons those sites responsible for the majority of ORR current. Hence, in order to extract the turn over frequency at a given potential e.g. 0.80 VRHE, we use the difference in kinetic mass current (Jkin,mass) at that potential between the unpoisoned and poisoned state divided by the number of sites |
 | (15) |
TOF is determined at both 0.80 and 0.85 VRHE, utilizing the poisoned and unpoisoned kinetic currents at those potentials.
3. Results and discussion
3.1 Physico chemical and electrochemical characterisations
Nitrogen sorption and particle size measurements.
We note that these results were produced from a round robin test and so represent an average across laboratories. N2 physisorption was performed to determine the surface area and pore volume of the four selected catalysts (Fig. 1a, b and Table 1). All four catalysts showed high surface area in the range from 463 to 840 m2 g−1 and isotherms with well-defined hysteresis indicating the presence of mesopores (not shown here). The CNRS catalyst exhibits the highest BET surface area, for the most part due to micropores, followed by the UNM catalyst, which showed large pore volumes in the mesoporous range. Obtained from a similar hard templating technique, the PAJ catalyst displayed a slightly larger mesoporous volume but slightly lower microporous volume than UNM, and ranked in the middle in terms of BET area. The ICL catalyst, on the other hand, showed a balanced microporous/mesoporous pore volume and displayed the lowest BET area.
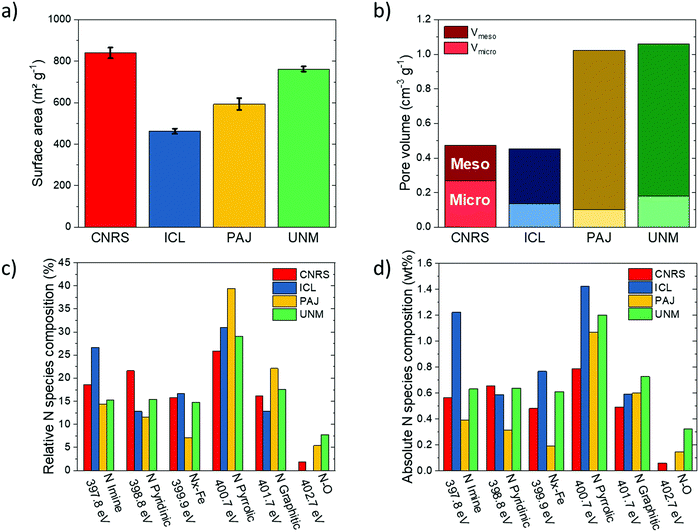 |
| Fig. 1 Physicochemical analyses of the four benchmark Fe–N–C catalysts: (a) BET specific area; (b) micro- and mesoporous volumes; (c) relative content of nitrogen species (% relative to total N) as detected in high-resolution N 1s XPS spectra, with assignments of nitrogen species of: Imine 397.8 eV, Pyridinic 398.8 eV, Nx–Fe 399.9 eV, Pyrrolic 400.7 eV, Graphitic 401.7 eV, N–O 402.7 eV; (d) absolute content of each nitrogen species in the catalysts by division of relative content from XP S with the wt% content of nitrogen as determined by elemental analysis. | |
Table 1 Overview of physicochemical and electrocatalytic properties of the four benchmarking PGM-free Fe–N–C electrocatalysts for the oxygen reduction reaction (ORR)
Method |
Unit |
Catalyst |
CNRS |
ICL |
PAJ |
UNM |
Note: the electrochemical data are cross-laboratory averaged values. Each catalyst was measured in at least two different laboratories with two to three repetitions for each catalyst loading. |
N2 physisorption |
|
Surface area |
m2 g−1 |
840 ± 26 |
463± 13 |
593 ± 28 |
763 ± 13 |
Micropore volume |
cm3 g−1 |
0.269 |
0.137 |
0.103 |
0.181 |
Mesopore volume |
cm3 g−1 |
0.203 |
0.317 |
0.92 |
0.88 |
|
57Fe Mössbauer |
Absorption area |
D1 |
% |
42 |
38 |
11 |
40 |
D2 |
% |
27 |
62 |
38 |
49 |
α-Fe |
% |
18 |
0 |
15 |
0 |
γ-Fe |
% |
10 |
0 |
36 |
11 |
Fe3C |
% |
3 |
0 |
0 |
0 |
Isomer shift |
D1 |
mm s−1 |
0.34 |
0.36 |
0.37 |
0.36 |
D2 |
mm s−1 |
0.45 |
0.55 |
0.40 |
0.41 |
α-Fe |
mm s−1 |
0.00 |
— |
— |
— |
γ-Fe |
mm s−1 |
−0.08 |
— |
−0.12 |
−0.08 |
Fe3C |
mm s−1 |
0.185 |
— |
— |
— |
|
Composition |
ICP-MS |
Fe |
wt% |
2.50 |
1.0 |
0.6 |
0.8 |
Elemental analysis |
C |
wt% |
76.39 |
76.57 |
84.45 |
84.49 |
H |
wt% |
1.08 |
1.15 |
0.81 |
0.82 |
N |
wt% |
3.04 |
4.59 |
2.71 |
4.13 |
S |
wt% |
0.23 |
1.39 |
— |
— |
|
XPS |
Surface composition |
C 1s |
at% |
91.51 |
86.78 |
95.43 |
91.46 |
O 1s |
at% |
5.98 |
9.99 |
2.01 |
4.91 |
N 1s |
at% |
2.15 |
3.06 |
2.3 |
3.37 |
Fe 2p3/2 |
at% |
0.36 |
0.16 |
0.25 |
0.26 |
Fraction of N species |
Imine (397.8 eV) |
% |
18.6 |
26.6 |
14.4 |
15.3 |
Pyridinic (398.8 eV) |
% |
21.6 |
12.8 |
11.6 |
15.4 |
Nx–Fe (399.9 eV) |
% |
15.8 |
16.7 |
7.1 |
14.8 |
Pyrrolic (400.7 eV) |
% |
25.9 |
31 |
39.4 |
29.1 |
Graphitic (401.7 eV) |
% |
16.2 |
12.9 |
22.1 |
17.6 |
N–O (402.7 eV) |
% |
1.9 |
— |
5.4 |
7.8 |
|
Electrochemistry via RRDE |
Intital activity 0.2 mg cm−2 |
J
lim (0.2 VRHE) |
mA cm−2 |
3.5 ± 0.3 |
4.77 ± 0.07 |
4.3 ± 0.6 |
3.9 ± 0.7 |
J
kin (0.80 VRHE) |
mA cm−2 |
0.40 ± 0.09 |
0.24 ± 0.06 |
0.465 ± 0.005 |
0.48 ± 0.43 |
J
kin (0.85 VRHE) |
mA cm−2 |
0.12 ± 0.06 |
0.05 ± 0.02 |
0.100 ± 0.001 |
0.10 ± 0.09 |
H2O2 (0.2 VRHE) |
% |
5 ± 2 |
8 ± 6 |
6 ± 4 |
7 ± 5 |
H2O2 (0.7 VRHE) |
% |
5 ± 3 |
14 ± 9 |
7 ± 3 |
8 ± 5 |
Activity after AST 0.2 mg cm−2 |
J
lim (0.2 VRHE) |
mA cm−2 |
3.1 |
4.2 ± 0.2 |
3.9 ± 0.5 |
3.41 ± 0.09 |
J
kin (0.80 VRHE) |
mA cm−2 |
0.4 |
0.22 ± 0.01 |
0.18 ± 0.07 |
0.13 ± 0.05 |
J
kin (0.85 VRHE) |
mA cm−2 |
0.09 |
0.05 ± 0.01 |
0.04 ± 0.02 |
0.03 ± 0.01 |
H2O2 (0.2 VRHE) |
% |
10 ± 2 |
8 ± 6 |
7 ± 3 |
10 |
H2O2 (0.7 VRHE) |
% |
14 ± 3 |
20 ± 13 |
19 ± 6 |
16 |
X-ray photoelectron spectroscopy.
The elemental composition and chemical state of the catalysts were analyzed by XPS, including the narrow scan regions at the N 1s and Fe 2p core levels (Fig. 1c, d and Fig. S1, ESI†). The Fe 2p XPS data displayed lower signal/noise ratio, because the signal intensity of Fe photoelectrons was close to the detection limit (not shown here). This is typical for Fe–N–C catalysts, due to the low site density of atomically dispersed Fe–Nx moieties. XPS Fe 2p core level analysis at higher resolution did give an estimation of the Fe amount located at or a few nm below the surface. This level of surface sensitivity allows us distinguishing Fe–Nx moieties at or within few nm from the surface from Fe species encapsulated by thick layers of carbon (>10 nm). The latter case is usual for metallic Fe or iron carbide particles in Fe–N–C materials, since they catalyze graphitization and reprecipitation of carbon around them during pyrolysis. Fe particles surrounded by a carbon layer >10 nm (longer than the path through which the corresponding photo-electrons may travel in carbon) are invisible by XPS. In contrast to the Fe 2p region, the N 1s region gave more insights on the qualitative and quantitative nitrogen content, allowing deconvolution the spectra into the contributions of functional groups into sp, sp2 and sp3 hybridized nitrogen atoms and N atoms involved in the Fe–Nx motifs. It should be noted that the multi-peak fitting of the N 1s core level region and the assignment of specific binding energies to functional groups remains a controversial topic of intense research and fitting variability across laboratories.13,91–94 In particular, the assignment and reliable quantification of N atoms involved in Fe–Nx moieties remains difficult, as it requires the knowledge of the exact coordination of the sites present in the sample. For example, the number of nitrogen atoms coordinating the Fe centers and the number of carbon atoms in the second coordination sphere can influence the N 1s binding energy.13,91 In addition, the average binding energy of N atoms coordinating Fe cations in Fe–Nx moieties overlaps that for amine nitrogens.13 Due to these difficulties, the Fe–Nx component is often not distinguished from the other nitrogen groups. Considering close binding energies calculated by DFT for slightly different Fe–Nx structures,91 we decided to limit ourselves to a conservative fitting procedure with one single component for Fe–Nx, to avoid data over-interpretation. Fig. S1b (ESI†) shows the high-resolution N 1s XPS spectra for the four benchmark catalysts that were fitted with a total of 6 components with fixed positions (see in Fig. 1c and d), assigning one binding energy only to the coordinative Fe–Nx structure. The lowest binding energy (BE) peak at 397.8 eV was assigned to imine or cyanide groups while the peak at 398.8 eV was attributed to pyridinic nitrogen and nitrogen-coordinated metal atoms in coordinative states such as Fe–N2 and Fe–N3. The peak at 399.9 eV in the metal-free samples was assigned to amine groups, whereas in the case of Fe-containing samples, it is considered to arise from both nitrogen of the mesomeric Fe–N4 configuration and amines. The peaks at 400.7 eV, 401.7 eV and 402.7 eV correspond to pyrrolic, graphitic and N–O nitrogen, respectively. The detailed quantitative analysis is summarized in Fig. 1c, d and Table 1.
Mössbauer spectroscopy.
57Fe Mössbauer spectroscopy was carried out in order to obtain qualitative and quantitative information on the different Fe species present in the bulk of the benchmark catalysts. As displayed in Fig. 2, each catalyst has a distinct Mössbauer fingerprint. The ICL sample showed exclusively the presence of the well-documented D1 and D2 quadrupole doublets,7,31 indicating the main or sole presence of atomically dispersed Fe–Nx sites. From the comparison between calculated and experimental values of quadrupole splitting (QS), the doublets D1 and D2 have recently been assigned by us to, mainly, high-spin Fe(III)N4 (with OH or O2 adsorbed on iron) and low- or medium-spin Fe(II)N4 sites, respectively.69 It is important however to note that the Mössbauer signature of high-spin Fe(III)N4 sites and nanosized ferric oxides is similar, the latter leading to a sextet spectral component only at very low temperature (<60 K) and/or in the presence of an external magnetic field.14 The minor presence of nanosized ferric oxides can therefore not be entirely excluded from room temperature Mössbauer spectroscopy measurements alone, but the TEM analysis shown later supports the absence of Fe clusters in the ICL sample. The UNM catalyst showed, in addition to D1 and D2, the minor presence of a singlet with isomer shift near 0 mm s−1, assigned to non-magnetic γ-Fe or to nanosized superparamagnetic α-Fe. In addition to D1, D2 and the same singlet component, the CNRS catalyst shows a small contribution of a sextet component that is unambiguously assigned to magnetic α-Fe. The PAJ catalyst displays a spectrum that differs considerably from the other spectra. While it contains the same four spectral components (D1, D2, the singlet and sextet assigned to γ-Fe and α-Fe), the singlet component is by far the majority species and, in addition, the relative content of D1 is lower than that of D2, an unusual feature for Fe–N–C catalysts. The Mössbauer results and analysis are in accordance with TEM characterization (Fig. S2 and S3, ESI†). No dense particles related to metallic Fe are observed in the TEM image of the ICL catalyst while large dark particles assigned mainly to α-Fe are seen for the CNRS catalyst. Surprisingly, the PAJ catalyst shows rather small dark particles, that we assign to γ-Fe nanoparticles from the Mössbauer spectroscopy analysis.
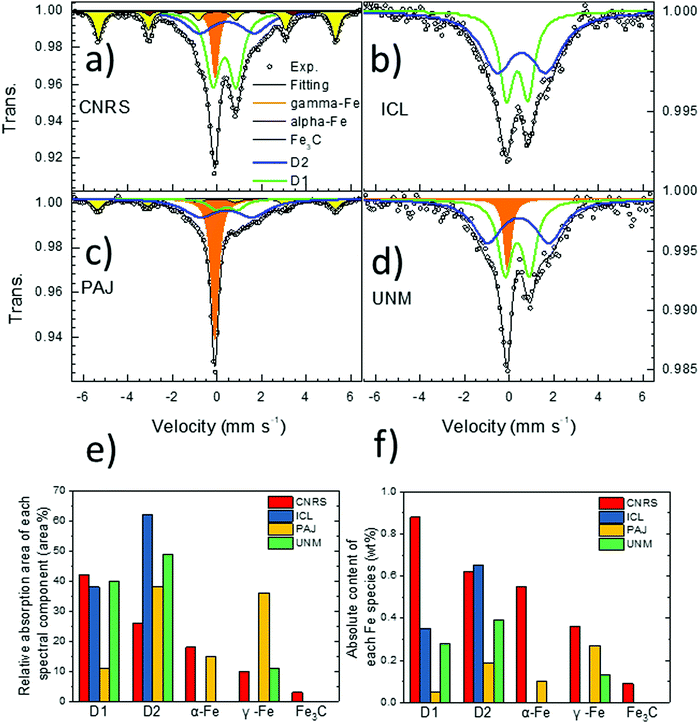 |
| Fig. 2 Comparison of the room temperature 57Fe Mössbauer spectra of the four benchmarking catalysts: (a) CNRS, (b) ICL, (c) PAJ, and (d) UNM. (e) Relative area for each spectral component relative to the total absorption area and (f) absolute wt% of each Fe species determined from the relative area, the Lamb–Mössbauer factor of each species and the total Fe bulk content as determined by ICP. In (a–d), the colour code identifies the individual spectral components, while in (e and f), the colour code identifies the catalysts. | |
Despite different overall shapes of the spectra, their fitting with unconstrained parameters (isomer shift, quadrupole splitting, hyperfine field and linewidth) resulted in spectral components with relatively common parameters among these four catalysts. As can be seen in Table 1 and Table S1 (ESI†), the isomer shift (IS) and quadrupole splitting (QS) of the doublet D1 ranges only from 0.34–0.37 mm s−1 and 0.75–1.10 mm s−1 for the four catalysts, respectively. While some small differences in the exact Fe coordination of D1 probably exist in these four catalysts, it nevertheless clearly makes sense to assign this particular spectral component to a single generic type of Fe–Nx moieties in the later discuss of the overall results. The doublet D2 with the larger QS-value has also relatively narrow range of IS and QS values across these four catalysts (Table 1 and Table S1, ESI†). While the singlet component has an IS corresponding either to nano-sized α-Fe or to non-magnetic γ-Fe, a low temperature Mössbauer measurement of the PAJ catalyst revealed that this singlet did not split into a sextet at 30 K, which excludes the assignment to a nanosized superparamagnetic γ-Fe phase (nanometric α-Fe particles usually become magnetically ordered at 30 K). For the sextets, the isomer shift and hyperfine field values derived from the fittings correspond perfectly to those for the reference compounds α-Fe and Fe3C, so that those assignments are completely unambiguous. The advantage of Mössbauer spectroscopy compared to X-ray diffraction for an identification of those phases lies in its sensitivity which allows for unambiguous detection of even very small amounts of such phases, which would be impossible with XRD.
Electrochemical measurements.
The ORR activity and selectivity of the four benchmark catalysts was measured in all four laboratories with conventional Rotating Ring Disk Electrode (RRDE) setups. Focus was placed on the initial ORR activity and H2O2 selectivity. The RRDE experiments were performed at 0.2 and 0.8 mg cm−2 geometric catalyst loading. Fig. 3a shows as an example one particular set of polarization curves and H2O2 selectivity curves of the four catalysts measured for a loading of 0.8 mg cm−2 in the potential range from 0.0 to 0.9 VRHE. It can be observed that the onset potential of oxygen reduction follows the order ICL < UNM < PAJ < CNRS whereas the opposite trend is observed for the diffusion-limited current density at 0.2 VRHE. It should be noted that the polarization curves and peroxide selectivity shown were measured in the same laboratory while data reported in Table 1 are averaged from measurements at several laboratories and for several layers of each catalyst. The benchmark catalysts exhibited generally low H2O2 production, except perhaps for the UNM catalyst with 10–11% below 0.5 VRHE. After Koutecký–Levich analysis of each curve (see Methods), the initial mass-based ORR activity of each catalyst was obtained, averaged across all four-laboratory data. Table 1 reports the mean ORR mass activity of each catalyst, at a potential of 0.80 or 0.85 VRHE and for catalyst loadings of either 0.2 or 0.8 mg cm−2. Initial mass-based activities are compared in Fig. 3b and Fig. S4 (ESI†). Due to the error margin, a comparison of the present catalysts, with similar activities, is not easily achievable. As observed in Fig. 3b, the PAJ catalyst exhibited the highest mass activity at the catalyst loading of 0.8 mg cm−2 whereas the ICL catalyst appears as the least active in such conditions. The CNRS and UNM catalysts showed comparable values. At 0.2 mg cm−2, the mass activity differences are within the experimental error and are hence harder to compare (Fig. S4a and c, ESI†). An accelerated stress test (AST) was also performed to evaluate the stability of the catalysts at 0.2 mg cm−2 in load-cycling conditions (Fig. S5, ESI† and Table 1). The CNRS and ICL catalysts exhibited better stability than the PAJ and UNM catalysts. The high stability of the ICL catalyst (comprising only Fe–Nx sites) to such a load-cycling AST in N2-saturated acid electrolyte is in agreement with the stability reported in similar conditions for two Fe–N–C catalysts derived from ZIF-8 and comprising also only atomically-dispersed Fe–Nx sites.95 Similarly, the poor stability of the PAJ catalyst to such a load-cycling AST in N2-saturated acid electrolyte is in line with the poor stability observed in similar conditions for one Fe–N–C catalyst derived from ZIF-8 but comprising only iron-carbide particles.95
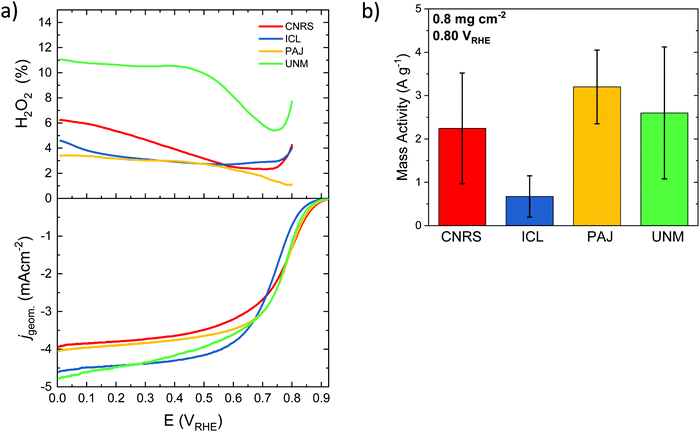 |
| Fig. 3 (a) Disk geometric current density measured with linear scan voltammetry (LSV) in RRDE setup and % peroxide derived from RRDE data. (b) Averaged mass-based activity at 0.8 VRHE. The measurements were performed in O2-saturated 0.5 M H2SO4 (pH 0.3) at a scan rate of 5 mV s−1 with a catalyst loading of 0.8 mg cm−2 and the rotating speed was set to 1600 rpm at 25 °C. The Pt ring was held at +1.5 VRHE. | |
The ORR selectivity of the catalysts was then studied at a loading of 0.2 and 0.8 mg cm−2 (Fig. S6, ESI†). The % H2O2 during ORR is generally low on the four benchmark catalysts, ranging from 2 to 8%, except for the ICL sample at low loading and at high potential, reaching ca. 14% (Fig. S6c, ESI†). The general trend of increased peroxide formation with decreased catalyst loading is observed, in accordance with previous reports on both PGM-free and PGM-based catalysts.96–99 Low catalyst loadings imply thin layers, which increases the probability of the formed peroxide molecule to escape the active layer before it can re-adsorb on another active site. Thick layers, in contrast, increase the probability of peroxide re-adsorption on a same or a different active site during its diffusion from the inner part of the active layer towards the bulk electrolyte.100 If re-adsorption occurs, then the initially formed peroxide molecule can be further reduced to water, or can decompose into O2 and water. In contrast to the impact of catalyst loading on the selectivity, no trend is observed in the selectivity as a function of the electrochemical potential, with similar % peroxide at 0.2 and 0.7 VRHE (Fig. S6a, b and c, d, ESI,† respectively), except for the ICL catalyst. The error bar on ICL sample regarding selectivity is however large.
3.2 Comparison between Fe surface site densities (SD) derived from ex situ CO cryo-adsorption and in situ reductive nitrite stripping
3.2.1 Evaluation of SD values and their normalization by the catalyst mass or BET area.
Recent reports have shown that SD values of Fe–N–C catalysts can be evaluated using either an ex situ CO cryo chemisorption or an in situ electrochemical nitrite (NO2−) adsorption/reductive stripping technique.68,77,85 The two methods operate in vastly different physical and chemical environments. The CO technique is a non-electrochemical environment and employs low temperature gas adsorption/thermal stripping of molecular CO on a solid, with the powders pre-annealed at 600 °C in argon. The NO2− technique is a “partial knock out technique” that blocks a fraction of the active surface Fe sites by NO adsorption, resulting immediately in lower ORR current densities. Subsequent reductive stripping and quantification of the number of the coordinated NO molecules in the same electrochemical environment enables the evaluation of a large fraction of the total accessible number of Fe sites under electrochemical conditions. SD values were evaluated using both techniques and reported after normalization by either the catalyst mass, referred to as SDmass, or by the BET surface area, referred to as SDBET (see Experimental methods).
More specifically, the ex situ CO cryo chemisorption method evaluated the SD value under the assumption that one adsorbed CO molecule corresponds to one Fe(II)Nx moiety at the surface of the pre-annealed, oxygen-free catalyst. Experimentally, the CO adsorption was found to be complete after three consecutive CO pulses at the chosen molar CO amounts per pulse. Consequently, the last three out of the total six CO pulses could be used as zero-uptake reference peaks for the CO uptake calculations (Fig. S7, ESI†). The experimentally derived CO uptakes on the four catalysts and the associated SD values are summarized in Table S2 (ESI†), while the temperature-programmed CO desorption profiles and peak assignments and interpretations are shown in Fig. S8 (ESI†). The in situ reductive NO2− adsorption in the form of adsorbed NO probe molecules and their subsequent reductive stripping to ammonia was performed at pH 5.2 using RDE measurements. Voltammetric scans were recorded before and after reductive NO poisoning from NO2− as well as after reductive removal of NO to ammonia, resulting in the full recovery of the NO-poisoned surface Fe sites (Fig. S9–S12, ESI†). Reductive NO2−/NO poisoning resulted in a significantly decreased catalytic ORR current density, while the catalytic ORR current density was almost completely recovered after reductive stripping of NO to ammonia (compare dashed blue and solid black curves in Fig. S9c–S12c, ESI†). Assuming that one NO molecule poisons one Fe surface site, the differential stripping current with and without NO poisoning yields the in situ SD values, which are summarized in Table S2 (ESI†).
Fig. 4a displays the first-ever direct comparison between experimental SDmass values obtained on one hand from the in situ nitrite reduction and on the other hand from the ex situ CO cryo chemisorption technique. It is noteworthy that despite the vastly different analysis methods, the experimental SDmass values ranged reproducibly on the same order of magnitude of 1019 sites gcatalyst−1. At the same time, the detailed SDmass(CO) values derived from gaseous CO cryo chemisorption were systematically (2× to 8×) greater than the SDmass(NO2−) values. This is largely attributed to Fe surface sites located inside gas accessible but electrochemically inaccessible pores that are likely to be less accessible for reductive NO2− adsorption and reductive NO stripping. Hence, these sites are not probed by the in situ reductive NO2− technique. In comparison, gaseous CO(g) has facile accessibility to Fe surface sites even inside the micropore structure of the dry solid catalysts. This hypothesis will be supported by numerous correlations below. The experimental NO2− and CO-based SDmass values can be regarded as lower and upper bounds of the surface Fe site density of each Fe–N–C catalyst, respectively. As such, the NO2− and CO-based site density values, for the first time, yield ranges of averaged reactivity descriptors, such as the intrinsic turn over frequencies of surface Fe sites of the benchmark Fe–N–C ORR catalysts.
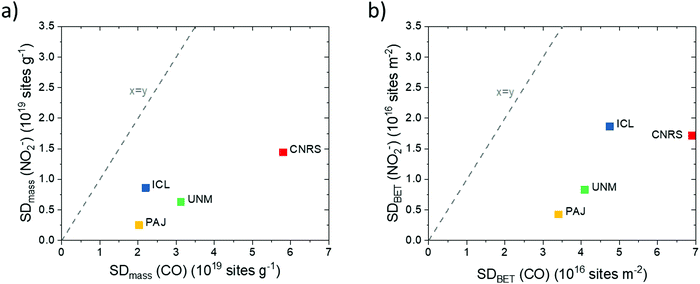 |
| Fig. 4 Comparison of ex situ and in situ Fe surface site density (SD) values of the four Fe–N–C catalysts obtained using CO-chemisorption and nitrite electrochemical reductive stripping. (a) SDmass values measured in situ vs. SDmass values measured ex situ; (b) SDBET values measured in situ vs. SDBET values measured ex situ. The y = x lines are indicated as dashed lines. Data were derived from measurements in Fig. S7–S12 (ESI†) and Table 1. | |
Fig. 4a reveals that the CNRS catalyst showed the largest SDmass value while the PAJ catalyst showed the smallest value, both in the ex situ and in situ SD metric. The ICL and UNM catalysts show intermediate SDmass values, with interchanged ranking according to which method is considered for the SDmass measurement. The underlying reason for such differences between the two methods is probably related to the difference between gas-phase accessibility (ex situ method) and electrochemical accessibility (in situ method). It seems reasonable to assume that the electrochemical surface will closely match the gas-phase surface for Fe–N–C materials with low BET surface area (low proportion of micropores) while the gap between the two concepts of accessibility will increase for materials of increasing BET areas (typically, with high micropore area). To evaluate this, the BET-normalized real Fe surface site density (SDBET(CO) and SDBET(NO2−)) were calculated and correlated to each other in Fig. 4b. Now, the ICL catalyst with its relatively low surface area of ∼400 m2 g−1 displays a large SDBET(NO2−) value, while the ICL, PAJ and UNM catalysts define a linear trend between the SDBET(NO2−) and SDBET(CO) values. In other words, for these three catalysts, the nitrite probe sampled an almost identical fraction of the total number of Fe sites that are available to CO via the gas phase. The absolute numbers of SD however significantly differ between the two methods (discussed later). The CNRS catalyst falls out of the trend defined by the three other catalysts in Fig. 4b. This can be explained if the electrochemical utilization of the Fe-based sites is significantly lower with the CNRS catalyst than with the three others. This in turn is well supported by the significantly higher ratio of micropore to mesopore volume of CNRS vs. other samples (Fig. 1b). The horizontal offset between SDBET(CO) of CNRS and the blue regression line is directly attributed to the effect of CO-accessibility of all Fe surface sites in micropores and NO2− inaccessibility of some of the Fe surface sites that are located deep inside micropores.
3.2.2 Correlating CO- and NO2−-derived turnover frequencies (TOF).
To compare intrinsic ORR reactivities between the catalysts, average catalytic turnover frequencies (TOFs) were calculated and correlated using experimental uncorrected SDmass(CO) values, SDmass(NO2−) values and mass-based kinetic current densities at different applied electrode potentials (0.80 and 0.85 VRHE) (Fig. 5). This TOF values are here to be seen as the average across all electrochemically active sites, and do not depend on whether they are evaluated using mass-based or BET-based SD and ORR activity values. Note that the CO-based TOF calculations used the ORR current densities from Fig. S4 (ESI†) and Table 1, while the NO2−-based TOF evaluations relied on the differential ORR current densities of the poisoned and the non-poisoned state of the catalyst at pH 5.2 (Fig. S9–S12, ESI†). Fig. 5 indicates a close positive correlation between the evaluated TOFs of both methods at both electrode potentials, with TOFs increasing in the order CNRS < ICL < UNM < PAJ.
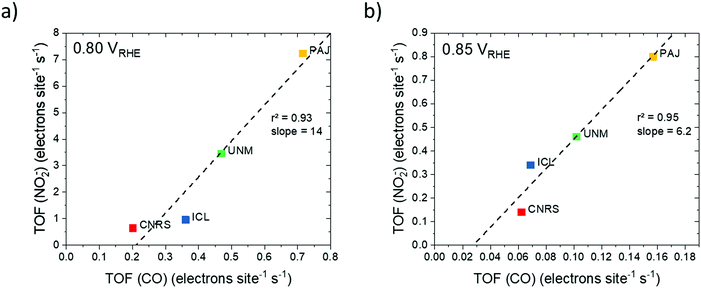 |
| Fig. 5 Correlations between the catalyst turnover frequencies (TOFs) derived from SDmass(CO) and SDmass(NO2−) values at (a) 0.80 VRHE and (b) 0.85 VRHE. | |
TOF values derived from NO2− stripping are 2× to 10× higher than the CO-derived TOFs, which follows from the ratio between SDmass(NO2−) and SDmass(CO). The lower TOFs derived from SDmass(CO) appear to originate in the overestimation of the catalytically active Fe surface site density. A more realistic average TOF values of each catalyst may lie in between the two TOF values in Fig. 5.
Looking at the TOFs derived from each probe technique separately, we note that the large TOFs of the PAJ catalysts may be attributed to an improved oxygen accessibility of the catalytically active Fe surface site under operating conditions. Another reason for the high TOFs may be the favorable molecular structure of some or all of the catalytically active Fe surface sites of PAJ, which leads to lower kinetic reaction barriers and hence faster catalytic turn over cycles. On the other hand, the CNRS catalyst exhibited the lowest TOFs in RDE testing due to its high proportion of micropores, expected to be hardly accessible due to non-wetting.
3.2.3 Fe surface site density (SD)–turnover frequency (TOF) reactivity maps.
Eqn (1) defines the experimental ORR mass activity of a catalyst as the product of the catalytic active site density, SD, and its intrinsic TOF. With values for SD and TOF at hand, SD–TOF reactivity maps can be generated to analyze the catalytic ORR reactivity (Fig. 6 and 7 display data at 0.80 VRHE, while Fig. S13 and S14 at 0.85 VRHE, ESI†). In SD–TOF reactivity maps each catalyst entry falls on its hyperbolic iso-mass activity or iso-BET surface area activity curve, depending whether SDmass or SDBET values are used. We propose SD–TOF reactivity maps as useful new comparative analysis tool to analyze and understand the origin of ORR reactivity of PGM-free electrocatalysts. Moreover, SD–TOF reactivity maps allow a knowledge-based correlation between catalyst synthesis parameters, such as temperature, time, precursor type, etc. and the two most important intrinsic reactivity descriptors. Variations in the mass or surface area-normalized kinetic reactivity can now be understood in terms of variations in the SD, or TOF, or both. Entire synthesis–reactivity pathways inside SD–TOF maps may trace the influence of individual synthetic parameter variations, and thus allow for a rational development of improved catalysts toward pre-defined target performances, as illustrated in Fig. 6.
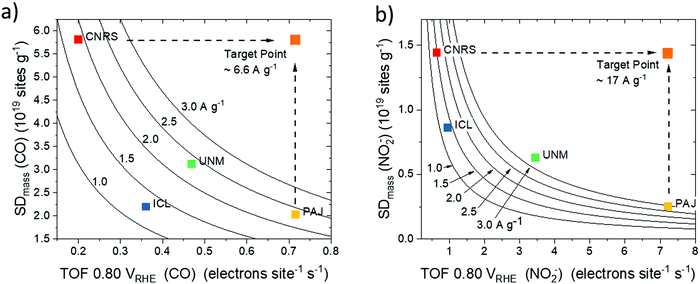 |
| Fig. 6 Site density–ORR turnover frequency maps (SD–TOF ORR reactivity maps) obtained by plotting the Fe surface site density (SD) and the corresponding TOF for each of the four catalysts with iso-mass activity hyperbolic curves at 0.80 VRHE: (a) SD–TOF reactivity map derived from SDmass(CO) (pH 1, activity values from Table 1) and (b) SD–TOF reactivity map derived from and SDmass(NO2−) (pH 5.2 Fig. S9–S12, ESI†). SD–TOF reactivity maps allow for a rational deconvolution and analysis of ORR reactivity of PGM-free catalysts. They also aid in establishing fundamental synthesis–reactivity relationships. Dashed arrows indicate examples of catalyst target performance superimposing the highest observed SD (CNRS) with the highest observed TOF (PAJ). | |
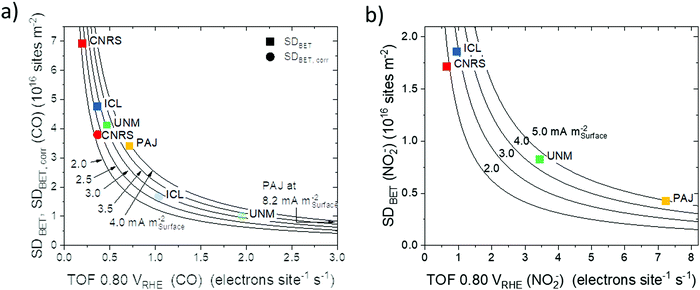 |
| Fig. 7 Areal site density, SDBET–ORR turnover frequency (TOF) reactivity maps with each of the four catalysts plotted on its hyperbolic iso-areal activity curve at 0.80 VRHE: (a) SDBET(CO)–TOF reactivity map (square symbols) and SDBET,corr(CO)–TOF reactivity map (round symbols) derived from CO cryo chemisorption (pH 1, activity values from Table 1) and (b) SDBET(NO2−)–TOF reactivity map derived from reductive NO2− stripping (pH 5.2 Fig. S9–S12, ESI†). | |
Fig. 6a and b show the catalyst mass-based SDmass–TOF reactivity maps derived from CO and NO2−, respectively. Both maps reveal a consistent trend in catalyst mass activity, Jkin,mass, at 0.80 VRHE in the order ICL < CNRS < UNM ≈ PAJ. Differences in Jkin,mass are due to the difference in measurement conditions, such as pH. Analysis of the SD–TOF maps demonstrates that the PAJ and CNRS catalysts both exhibited high and comparable Jkin,mass, however, the origin of their reactivity was quite different: While CNRS features many Fe sites at the surface with low average TOF, that is low intrinsic reactivity, PAJ offers fewer, yet intrinsically very active surface sites. This may be explained by the presence of many Fe surface sites in the micropores of CNRS, which failed to be accessible, and hence effective under electrochemical conditions. PAJ, on the other hand, appears to feature fewer Fe surface sites largely in macropores, where they are accessible and able to contribute to the experimental reactivity. UNM and ICL catalysts fall in between the two extreme cases, with their quantitative Jkin,mass–SD–TOF patterns varying between Fig. 6a and b due to the varying test conditions. From SD–TOF maps, catalyst development targets can be derived: Increasing the SD of the PAJ catalyst or, alternatively, introducing more sites of the PAJ type into CNRS, that is, accessible sites in macropores or sites that closely resemble the PAJ molecular structure, should be a rational strategy to arrive at the target points of 6.6 and 17 A g−1 in the respective maps of Fig. 6. Another interesting point to note is that catalysts with high TOF values, such as PAJ, fall into regions of the map where the iso-activity Jkin,mass curves are located more densely. Provided an absolute increase in SDmass, catalysts such as PAJ would gain a much larger increase in Jkin,mass than catalysts located at a more centered position of the same iso-activity curve. In other words, synthetic strategies to increase SDmass will have more impact on catalyst located on the right of the map than on catalysts located on the left.
A similar analysis was performed in the corresponding SDBET–TOF maps (Fig. 7a for SDBET(CO)–TOF and Fig. 7b SDBET(NO2−)–TOF) that both revealed a general trend in areal catalytic activity in the order CNRS < ICL < UNM < PAJ. The data points represented as squares in Fig. 7a indicate that CNRS and PAJ remained at the extremes, in the sense that CNRS exhibited, on average, the most Fe surface sites per catalyst surface area, while fewer Fe site per catalyst surface area of PAJ featured the highest average TOF and resulted in the highest ORR reactivity. The relatively low BET surface area of ICL reversed the site density trends of ICL and UNM in Fig. 7a. The areal ORR activity of ICL now trails that of UNM only slightly. In the SDBET(NO2−)–TOF map of Fig. 7b, CNRS and ICL now exhibit similar areal site densities, while ICL featured a 50% higher ORR activity, which gives testament to its effective catalytic Fe sites at the surface. Fig. 7a also includes CO-based areal site density data SDBET,corr (round symbols) that corrects for Fe surface sites that are inaccessible at ambient conditions and thus quantitatively matched the NO2−-derived SDBET values for non-microporous catalysts. While all trends were preserved, the data points increased their spread along the TOF scale, which now more closely match those of the NO2− derived map in Fig. 7b.
3.2.4 Identifying the molecular and chemical state and physical location of Fe surface sites.
In order to learn more about the molecular identity, chemical state and physical location of Fe surface sites in the four Fe–N–C catalysts, we analyzed a large number of correlations between various molecular, compositional, morphological characteristics and the Fe surface site densities (SDmass, SDBET) and their corresponding TOF values (Fig. 8, 9 and Fig. S15–S20, ESI†). More specifically, we analyzed relations between site densities and pore structure (micropore, mesopore and total pore volume) in Fig. 8, Fig. S15a, b, and S16 (ESI†), nitrogen species (pyridinic-N and pyrrolic-N) in Fig. S15c, d, and S17 (ESI†), Fe bulk content in Fig. 8, and TOF in Fig. S18 and S19, ESI.† Emphasis is placed on correlations between Fe surface site densities and the abundance of 57Fe Mössbauer spectroscopy-derived Fe–Nx sites (high spin D1 site and medium or low spin D2 site) in Fig. 9, Fig. S16, and S19 (ESI†).
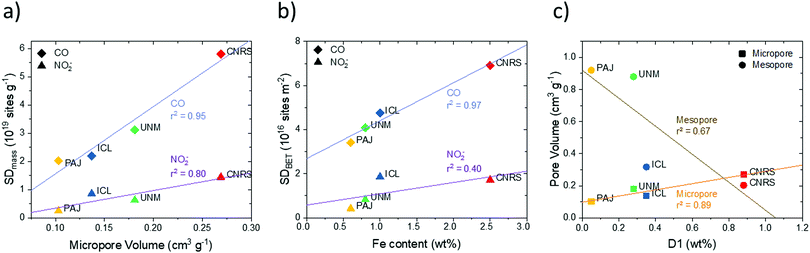 |
| Fig. 8 Correlations between the CO cryo adsorption-based Fe surface site density SDmass(CO) and NO2− stripping-based Fe surface site density SDmass(NO2−) and (a) the micropore volume, (b) the bulk Fe content, (c) correlation between pore volume with the weight ratio of the molecular D1 doublet obtained from 57Fe Mössbauer spectroscopy. | |
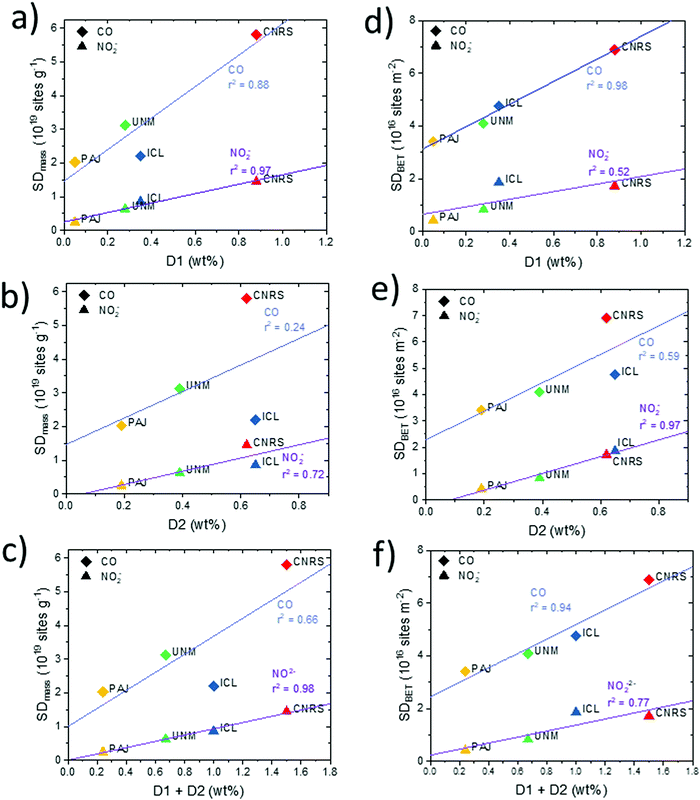 |
| Fig. 9 Correlations between Fe surface site densities, SDmass and SDBET, and the bulk weight abundance of the two principle molecular Fe sites, D1 and D2, derived from 57Fe Mössbauer spectroscopy doublets. (a–c) SDmass(CO), SDmass(NO2−), and (d–f) SDBET(CO) and SDBET(NO2−) plotted against the weight ratio of the molecular D1 site (a and d), the weight ratio of the molecular D2 site (b and e), the sum D1 + D2 (c and f). | |
Fe surface site density and physico-chemical surface properties.
Fe surface site densities, SDmass, displayed close correlations (r2 = 80–95%) with micropore volume (Fig. 8a, Fig. S15a and b, ESI†). The greater the micropore volume (PAJ to CNRS), the higher was the SDmass value for both the CO and NO2− probe techniques. However, the difference in the SDmass value between the CO and NO2− probe techniques increased with increasing micropore volume. In other words, the ratio of inaccessible Fe sites inside the micropores increased with the micropore volume (Fig. 8a). As expected, the number of inaccessible pore sites was largest for the ZIF-derived highly micro porous CNRS catalyst.
Generally, gaseous CO uptake-based SDmass(CO) values appear to correlate closer with pore-related catalyst characteristics (Fig. 8a and Fig. S15, ESI†), which is plausible given the generally better accessibility of pores to gaseous CO molecules. To support this conclusion further, we present the correlations of BET-based areal Fe surface site densities, SDBET, with surface nitrogen species (Fig. S17, ESI†): while their resulting r2 values are somewhat lower than those of the SDmass correlations, CO-based SDBET values again correlate much better with physico-chemical surface properties. For correlations between SD values and bulk properties, in contrast, this finding does no longer hold, as will be shown further below.
Plots of SDBET values against bulk Fe wt% content (Fig. 8b) show that overall Fe content followed the trend in SDBET(CO) for all four catalysts closely. The SDBET(NO2−) data, however, scale well only for PAJ, UNM, and ICL – the catalysts with limited microporosity – while the significantly larger bulk Fe content (∼2.5×) of CNRS appears offset from the other catalysts. It can be concluded that all the additional Fe bulk content of CNRS appears ineffective in raising the Fe surface site density sampled by the NO2− probe.
The correlation between pore structure and the weight content of the 57Fe D1 doublet (Fig. 8c) suggested that the high-spin Fe D1 sites are preferentially formed and hence located in micropores and less so in mesopores. That conclusion is also supported by the combined correlation between SDmass(CO), D1 wt% content and micropore volume illustrated in the 3D plot in Fig. S16 (ESI†).
Finally, the relations between pyrrolic N and Fe–Nx species and the TOF values, derived from CO and NO2−, is shown in Fig. S18 (ESI†). Consistent with eqn (1), the observed relations are strictly inverse to those found between the pyridinic- and pyrrolic N species and the SDBET values (Fig. S17, ESI†): PAJ and CNRS with the highest and lowest average TOF values showed the fewest and largest number of Fe–Nx sites near the surface, fully in line with the trends with pyridinic nitrogen species. UNM and ICL TOF values fit well into the N %–TOF trend lines in Fig. S18 (ESI†), following concomitantly the trends in SDBET, but not that of microporosity (Fig. 8a and c). Again, pyrrolic nitrogen atoms appear most abundant in PAJ with the largest TOF, but this does by no means imply that these Fe sites are constituted by coordinating pyrrolic N atoms. It rather indicates that the rather low pyridinic N content is still sufficient to constitute the N centers that coordinate Fe centers.
Fe surface site density and molecular Fe bulk moieties.
We now turn to an analysis of the relation between the catalyst mass- and BET-normalized Fe surface site densities, SDmass and SDBET, and the mass-normalized bulk abundance of the two main types of Fe–Nx moieties that are distinguished by 57Fe Mössbauer spectroscopy, referred to as “D1” and “D2” (Fig. 9). D1 and D2 differ due to the oxidation and electronic spin states of iron in Fe–Nx moieties, which in turn can be triggered by different local structures or different accessibility to O2.7,15,69 Both types of Fe sites have been repeatedly suspected to act as catalytically active sites, or at least as precursor sites, where oxygen adsorption and reduction occurs. The twelve correlations split into pairs of two, in particular those involving SDmass values (Fig. 9a–c), SDBET values (Fig. 9d–f) correlated with bulk ratios of D1 (a and d), D2 (b and e), and D1 + D2 (c and f).
Looking at Fig. 9a, c, d and f, SDmass(NO2−) follows D1 and D1 + D2 much better than SDmass(CO), which is plausible considering that D1 and D2 are porosity- and surface area-independent, mass-normalized bulk metrics, while SDmass(CO) values, unlike SDmass(NO2−) values, take micro porous morphology into account. Conversely, it is the surface area-corrected SDBET(CO), unlike SDBET(NO2−), that correlate very well with the abundance of D1 and D1 + D2 Fe sites. We conclude from the data that both SD probing methods appear sensitive for D1 type Fe sites, and D1 sites appear to exist both in meso- and micro-pores. Based on the trends in SDmass(NO2−), the NO2− probe technique samples Fe surface sites predominantly in mesopores, and likely also at the entry of micropores, but not deep inside micropores. This is supported by the data pattern in SDBET(NO2−) of Fig. 9d where the microporous CNRS catalyst is offset at much larger abundance of D1 at essentially constant values of the areal density SDBET(NO2−). Some recent researches demonstrated that the presence of Fe or Fe3C was beneficial for boosting the catalytic activity of Fe–Nx moieties through tuning the charge density of central iron atom in Fe–Nx moieties.101 The existence of Fe and/or Fe3C might contribute to the high ORR activity for the investigated catalysts. Only the ICL catalyst has a sole presence of atomically dispersed Fe–Nx sites from Mössbauer spectroscopy results.
Note that the two most active catalysts (UNM, PAJ) possess the fewest D1 Fe sites in the bulk and, accordingly, display the lowest Fe site density in three of four metrics, with SDmass(CO) of ICL being the only exception, likely due to its low surface area and low micro pore volume. This gives testament to the high intrinsic reactivity of their type of sites.
All catalysts except CNRS exhibit significantly larger bulk abundances of the molecular medium-spin D2 Fe site compared to those of D1 (PAJ 4×, UNM 1.5×, ICL 2×). ICL and CNRS, the two less active catalysts, display comparable large abundance of D2 (0.6–0.7 wt%). Only the areal density values of SDBET(NO2−), that is, the metric, the magnitude of which is least sensitive to porosity, correlate well with the bulk abundance of D2 (Fig. 9e). All others trace D2 content poorly. While both SD probing methods appear clearly sensitive to D2 Fe surface sites, the data also suggest that D2 Fe sites may be predominantly present in mesopores, and less so in micropores. Correlations with the combined abundances (D1 + D2) generally deteriorated compared to those with D1 alone, underlining the important role of D1 type Fe sites in the ORR process.
For completeness, we also analyzed the correlations of the CO- and NO2−-derived TOF values at 0.80 VRHE (Fig. 5) with D1, D2, and D1 + D2 as shown in Fig. S19 (ESI†). Average TOF values dropped with increasing weight content of bulk Fe sites, suggesting a decreasing mean efficacy of the Fe sites with increasing Fe site abundance. CNRS catalysts are offset at very low TOF values. In agreement with our conclusions above, this suggests that Fe sites in micropores (CNRS) appear highly ineffective catalytic sites, which, in turn, highlights the beneficial effect of meso- and possibly macro-pores as the physical location of effective Fe surface sites in PGM free Fe–N–C catalysts.
3.2.5 Catalyst Fe site utilization.
Only a portion of all D1 and D2 Fe–Nx sites of any catalyst is actually located at the catalyst (pore) surface, and can, in principle, act as catalytically active sites under ORR conditions. It would be quite useful to know the ratio of all Fe sites that are located at the catalyst surface and are potentially catalytic active sites. This ratio can be referred to as “site utilization factor” and can be used as a guiding metric in the design of improved Fe–N–C catalysts. Synthesis efforts should evolve toward utilization factor of unity, in which case all Fe sites could act as catalytic active sites.
The SDmass(CO) and SDmass(NO2−) metric probe and quantify the number of D1 and D2 Fe–Nx sites on the surface under ex situ and in situ conditions, respectively. SDmass(CO) and SDmass(NO2−) data in Fig. 10a (cf.Fig. 4) recall that under in situ NO2− conditions (presence of electrolyte and applied potential) the number of accessible, and hence potentially catalytic active Fe surface sites is smaller than the ex situ Fe surface site density suggests (see earlier discussion). This is why from SDmass(CO) and SDmass(NO2−) values a less and more stringent site utilization factor will ensue.
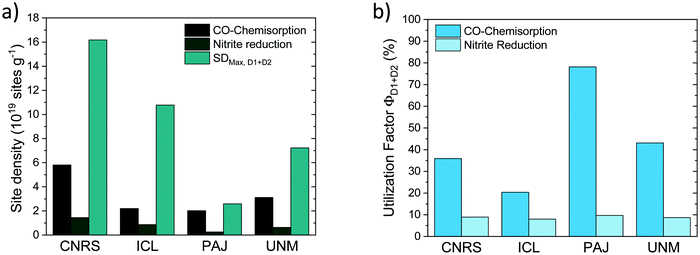 |
| Fig. 10 (a) Quantitative comparison of the maximum surface site density of D1 and D2 Fe sites (SDmax,D1+D2) and the Fe surface site densities, SDmass(CO) and SDmass(NO2−) of the four benchmark Fe–N–C catalysts; (b) Fe site utilization factors, ΦD1+D2(CO) and ΦD1+D2(NO2−) of the four benchmark Fe–N–C catalysts. | |
To derive experimental site utilization factors, we note that Mössbauer spectroscopy yields experimental quantitative estimates of the combined abundance (in weight%) of D1 and D2 Fe sites in bulk and surface of the catalysts, FeD1+D2. From FeD1+D2 the maximum possible mass-based Fe surface site density (SDmax,D1+D2) can be calculated as:
SDmax,D1+D2 [site gcat−1] = FeD1+D2 [wt%]/100/MFe × NA |
where MFe is the molar mass of iron, and NA is Avogadro's constant. Trends in SDmax,D1+D2 values for the four catalysts are illustrated in Fig. 10a with PAJ (∼2.2 × 1019 sites g−1) < UNM (7.2 × 1019 sites g−1) < ICL (11 × 1019 sites g−1) < CNRS (16 × 1019 sites g−1).
Normalizing SDmass(CO) and SDmass(NO2−) with SDmax,D1+D2 yields CO and NO2−-related site utilization factors, Φ, (Fig. 10b) according to:
ΦD1+D2(CO) = SDmass(CO)/SDmax,D1+D2 |
ΦD1+D2(NO2−) = SDmass(NO2−)/SDmax,D1+D2 |
While the magnitude of ΦD1+D2(NO2−) is significantly smaller (<10%), both types of site utilization factors exhibit the identical trend according: ICL < CNRS < UNM < PAJ. This is why the variations in utilization factors remained much smaller for the NO2−-related data set. ΦD1+D2(NO2−) represents the more stringent metric for guiding synthetic efforts. While ΦD1+D2(CO) of PAJ suggests that almost 80% of all Fe–Nx sites are located at the surface, its ΦD1+D2(NO2−) value demonstrates that just about 10% of Fe–Nx sites are electrochemically accessible. Both metric call for improved morphological and molecular catalyst designs that would deliberately place Fe–Nx sites in electrochemically accessible locations, such as meso pores. Once ΦD1+D2 values reach unity, further synthetic efforts to raise SD must remain ineffective and can give way to efforts to improve the (average) TOF values of (individual) Fe sites, in order to arrive at more active Fe–N–C ORR catalysts.
4. Conclusion
This cross-laboratory study compared and contrasted the physico-chemical properties and catalytic performance of four PGM-free Fe/N-doped carbon catalysts, Fe–N–C that efficiently catalyze the electrochemical reduction of molecular oxygen to water. The four selected catalysts, named PAJ, CNRS, UNM, and ICL were considered as state-of-art benchmark catalysts. What sets this study apart from previous similar studies, however, is the previously unachieved depth in the analysis of the origin of the catalytic reactivities. This was made possible by means of a deconvolution of catalytic performance metrics, such as the electrocatalytic mass activity (MA) and the surface area-based specific activity in terms of mass-normalized (SDmass) and BET surface area-normalized (SDBET) Fe surface site density (SD) and intrinsic catalytic turnover frequencies (TOF). This is the first time that Fe surface site densities and TOF values were evaluated and compared side-by-side for the same set of Fe–N–C catalysts using in situ nitrite reduction and ex situ CO cryo adsorption.
Initial ex situ characterization established the order of increasing kinetic mass-based ORR activity at 0.80 VRHE to ICL < CNRS < UNM < PAJ. Electrochemical NO2− adsorption/stripping measured a proportion of the surface Fe sites, electrochemically underutilizing the number of surface Fe sites potentially available, as disclosed by CO measurements. If we were able to access all the CO-accessible sites in an electrochemical environment, we could potentially boost the ORR activity by a factor of 2–4×.
Key conclusions include the first direct correlation of previously elusive SD values derived from CO and NO2− probing. Both techniques yield SDmass and SDBET estimates of the order of 1019 sites per gram catalyst and 1016 sites per m2 catalyst area, respectively. SD(CO) values were of larger magnitude due to the ready CO accessibility of Fe sites in the catalyst pore structure. TPD data further rationalized a deconvolution of SDBET(CO) data, which revealed a remarkable quantitative agreement between SDBET,corr(CO) and SDBET(NO2−) values.
SD–TOF reactivity maps were introduced as a data tool to analyze the origin of ORR reactivity of PGM-free Fe–N–C catalysts and aid in the design of more active ORR catalysts. These maps showed that PAJ exhibited the lowest Fe site density, which was offset by catalytically highly active site, which is why PAJ exhibited the highest mass-based ORR activity. CNRS, on the other hand, owing to its micropore structure featured the largest Fe SD at the lowest average TOF. The SD–TOF maps therefore suggested synthetic efforts to raise the SD of PAJ in order to achieve further improved ORR catalysts.
Correlations between Fe SD and (ex situ) physico-chemical surface and bulk properties of the catalysts led to the conclusions that (i) pyridinic nitrogen species are prevalent building blocks for Fe–Nx surface sites, (ii) high spin Fe sites (D1) are present in macro and micro pores, and (iii) medium spin Fe sites (D2) are present preferentially in mesopores and less so in micropores.
Finally, a Fe site utilization factor was introduced and evaluated for each catalyst from both the experimental CO- and NO2− based SD values. Site utilization factors derived from both SD techniques were fully consistent in their trends across the four catalysts. For PAJ, they suggested that roughly 80% of all available bulk Fe sites (D1 + D2) are located at the surface, while 10% remained available under operating ORR conditions. ICL, on the other hand, displayed 20% sites at the surface under ex situ conditions, and around 8% under operating conditions. Site utilization factors may be used as guidelines where synthetic optimization of catalyst morphologies is needed and when SD improvements are no longer necessary or effective.
In summary, this study represents a significant step forward in our analysis and understanding of the reactivity of PGM-free ORR catalysts. The proposed experimental methodologies and analytical data tools can easily be applied to new Fe–N–C ORR catalysts or even to metal–N–C single site catalysts for electrocatalytic processes other than the ORR. We expect that this work will help transform M–N–C catalysis research from the realm of empirical trial-and-error into a future, progressively more knowledge-based process.
Conflicts of interest
There are no conflicts to declare.
Acknowledgements
The research leading to the present results has received funding from the Fuel Cells and Hydrogen 2 Joint Undertaking under grant agreement no. 779366. This Joint Undertaking receives support from the European Union's Horizon 2020 research and innovation program, Hydrogen Europe and Hydrogen Europe research. P. S. acknowledges partial financial support by the German Federal Ministry of Education and Research under the German-Israeli battery program via the grant “Korrzellkat” with FKZ 03XP0251.
Notes and references
-
Handbook of Fuel Cells: Advances in Electrocatalysis, Materials, Diagnostics and Durability, ed. W. Vielstich, H. A. Gasteiger and H. Yokokawa, John Wiley & Sons Ltd, Chichester, West Sussex, UK, 2009 Search PubMed.
-
Handbook of Fuel Cells - Fundamentals, Technology, and Applications, ed. W. Vielstich, A. Lamm and H. Gasteiger, Wiley, Chichester, UK, 2003 Search PubMed.
-
N4-Macrocyclic Metal Complexes, ed. J. H. Zagall, F. Bedioui and J. P. Dodelet, Springer, New York, 2006 Search PubMed.
- M. Lefevre, E. Proietti, F. Jaouen and J. P. Dodelet, Science, 2009, 324, 71–74 CrossRef.
- F. Jaouen, E. Proietti, M. Lefevre, R. Chenitz, J. P. Dodelet, G. Wu, H. T. Chung, C. M. Johnston and P. Zelenay, Energy Environ. Sci., 2011, 4, 114–130 RSC.
- E. Proietti, F. Jaouen, M. Lefevre, N. Larouche, J. Tian, J. Herranz and J. P. Dodelet, Nat. Commun., 2011, 2, 416 CrossRef.
- U. I. Kramm, M. Lefevre, N. Larouche, D. Schmeisser and J. P. Dodelet, J. Am. Chem. Soc., 2014, 136, 978–985 CrossRef.
- M. Shao, Q. Chang, J.-P. Dodelet and R. Chenitz, Chem. Rev., 2016, 116, 3594–3657 CrossRef PubMed.
- S. T. Thompson, A. R. Wilson, P. Zelenay, D. J. Myers, K. L. More, K. C. Neyerlin and D. Papageorgopoulos, Solid State Ionics, 2018, 319, 68–76 CrossRef.
-
http://www.crescendo-fuelcell.eu/?jjj=1577517876158, Official FCH JU Website for Project CRESCENDO.
-
https://www.pegasus-pemfc.eu/, Official FCH JU Website for Project PEGASUS.
- U. Martinez, S. Komini Babu, E. F. Holby and P. Zelenay, Curr. Opin. Electrochem., 2018, 9, 224–232 CrossRef.
- K. Artyushkova, I. Matanovic, B. Halevi and P. Atanassov, J. Phys. Chem. C, 2017, 121, 2836–2843 CrossRef.
- S. Wagner, H. Auerbach, C. E. Tait, I. Martinaiou, S. C. N. Kumar, C. Kubel, I. Sergeev, H. C. Wille, J. Behrends, J. A. Wolny, V. Schunemann and U. I. Kramm, Angew. Chem., Int. Ed., 2019, 58, 10486–10492 CrossRef.
- U. I. Kramm, L. M. Ni and S. Wagner, Adv. Mater., 2019, 31, 1805623 CrossRef.
- U. Martinez, E. F. Holby, S. K. Babu, K. Artyushkova, L. Lin, S. Choudhury, G. M. Purdy and P. Zelenay, J. Electrochem. Soc., 2019, 166, F3136–F3142 CrossRef.
- U. Martinez, S. K. Babu, E. F. Holby, H. T. Chung, X. Yin and P. Zelenay, Adv. Mater., 2019, 31, 1806545 CrossRef PubMed.
- J. Z. Li, M. J. Chen, D. A. Cullen, S. Hwang, M. Y. Wang, B. Y. Li, K. X. Liu, S. Karakalos, M. Lucero, H. G. Zhang, C. Lei, H. Xu, G. E. Sterbinsky, Z. X. Feng, D. Su, K. L. More, G. F. Wang, Z. B. Wang and G. Wu, Nat. Catal., 2018, 1, 935–945 CrossRef.
- X. X. Wang, M. T. Swihart and G. Wu, Nat. Catal., 2019, 2, 578–589 CrossRef.
- L. Chong, J. G. Wen, J. Kubal, F. G. Sen, J. X. Zou, J. Greeley, M. Chan, H. Barkholtz, W. J. Ding and D. J. Liu, Science, 2018, 362, 1276–1281 CrossRef.
- Y. Y. Shao, X. L. Feng, L. M. Dai and J. P. Dodelet, Adv. Mater., 2019, 31, 1903622 CrossRef.
- V. P. Glibin, M. Cherif, F. Vidal, J. P. Dodelet, G. X. Zhang and S. H. Sun, J. Electrochem. Soc., 2019, 166, F3277–F3286 CrossRef.
- H. T. Chung, C. M. Johnston, K. Artyushkova, M. Ferrandon, D. J. Myers and P. Zelenay, Electrochem. Commun., 2010, 12, 1792–1795 CrossRef.
- K. M. G. Wu, C. M. Johnston and P. Zelenay, Science, 2011, 332, 443–447 CrossRef PubMed.
- G. Wu and P. Zelenay, Acc. Chem. Res., 2013, 46, 1878–1889 CrossRef PubMed.
- H. T. Chung, D. A. Cullen, D. Higgins, B. T. Sneed, E. F. Holby, K. L. More and P. Zelenay, Science, 2017, 357, 479–484 CrossRef PubMed.
- B. K. K. Artyushkova, B. Halevi, A. Knop-Gericke, R. Schlögl and P. Atanassov, Chem. Commun., 2013, 49, 2539–2541 RSC.
- H. G. Zhang, H. T. Chung, D. A. Cullen, S. Wagner, U. I. Kramm, K. L. More, P. Zelenay and G. Wu, Energy Environ. Sci., 2019, 12, 2548 RSC.
- Y. H. He, S. Hwang, D. A. Cullen, M. A. Uddin, L. Langhorst, B. Y. Li, S. Karakalos, A. J. Kropf, E. C. Wegener, J. Sokolowski, M. J. Chen, D. Myers, D. Su, K. L. More, G. F. Wang, S. Litster and G. Wu, Energy Environ. Sci., 2019, 12, 250–260 RSC.
- W. Wang, Q. Y. Jia, S. Mukerjee and S. L. Chen, ACS Catal., 2019, 9, 10126–10141 CrossRef CAS.
- J. K. Li and F. Jaouen, Curr. Opin. Electrochem., 2018, 9, 198–206 CrossRef CAS.
- N. Cheng, L. Zhang, K. Doyle-Davis and X. Sun, Electrochem. Energy Rev., 2019, 2, 539–573 CrossRef.
- S. Sun, G. Zhang, N. Gauquelin, N. Chen, J. Zhou, S. Yang, W. Chen, X. Meng, D. Geng, M. N. Banis, R. Li, S. Ye, S. Knights, G. A. Botton, T.-K. Sham and X. Sun, Sci. Rep., 2013, 3, 1775 CrossRef.
- P. Liu, Y. Zhao, R. Qin, S. Mo, G. Chen, L. Gu, D. M. Chevrier, P. Zhang, Q. Guo, D. Zang, B. Wu, G. Fu and N. Zheng, Science, 2016, 352, 797–800 CrossRef CAS.
- L. Zhang, R. Si, H. Liu, N. Chen, Q. Wang, K. Adair, Z. Wang, J. Chen, Z. Song, J. Li, M. N. Banis, R. Li, T.-K. Sham, M. Gu, L.-M. Liu, G. A. Botton and X. Sun, Nat. Commun., 2019, 10, 4936 CrossRef PubMed.
- A. Serov, K. Artyushkova and P. Atanassov, Adv. Energy Mater., 2014, 4, 1301735 CrossRef.
- U. I. Koslowski, I. Abs-Wurmbach, S. Fiechter and P. Bogdanoff, J. Phys. Chem. C, 2008, 112, 15356–15366 CrossRef CAS.
- R. Côté, J. Electrochem. Soc., 1998, 145, 2411 CrossRef.
- E. Proietti, F. Jaouen, M. Lefèvre, N. Larouche, J. Tian, J. Herranz and J.-P. Dodelet, Nat. Commun., 2011, 2, 416 CrossRef PubMed.
- S. Ma, G. A. Goenaga, A. V. Call and D. J. Liu, Chemistry, 2011, 17, 2063–2067 CrossRef CAS PubMed.
- M. J. Workman, A. Serov, L. K. Tsui, P. Atanassov and K. Artyushkova, ACS Energy Lett., 2017, 2, 1489–1493 CrossRef CAS.
- M. J. Workman, M. Dzara, C. Ngo, S. Pylypenko, A. Serov, S. McKinney, J. Gordon, P. Atanassov and K. Artyushkova, J. Power Sources, 2017, 348, 30–39 CrossRef CAS.
- R. Gokhale, Y. C. Chen, A. Serov, K. Artyushkova and P. Atanassov, Electrochim. Acta, 2017, 224, 49–55 CrossRef CAS.
- Y. C. Chen, R. Gokhale, A. Serov, K. Artyushkova and P. Atanassov, Nano Energy, 2017, 38, 201–209 CrossRef CAS.
- S. Kabir, K. Artyushkova, B. Kiefer and P. Atanassov, Phys. Chem. Chem. Phys., 2015, 17, 17785–17789 RSC.
- K. Artyushkova, C. Walker, W. Patterson and P. Atanassov, Electrocatalysis, 2014, 5, 241–247 CrossRef CAS.
- A. Serov, M. H. Robson, M. Smolnik and P. Atanassov, Electrochim. Acta, 2013, 109, 433–439 CrossRef.
- A. Serov, M. H. Robson, M. Smolnik and P. A. A. C. Information, Electrochim. Acta, 2012, 80, 213–218 CrossRef.
- G. Goenaga, S. Ma, S. Yuan and D.-J. Liu, ECS Trans., 2010, 33, 579–586 CrossRef.
- U. I. Kramm, J. Am. Chem. Soc., 2016, 138, 635–640 CrossRef PubMed.
- I. Martinaiou, T. Wolker, A. Shahraei, G. R. Zhang, A. Janssen, S. Wagner, N. Weidler, R. W. Stark, B. J. M. Etzold and U. I. Kramm, J. Power Sources, 2018, 375, 222–232 CrossRef.
- X. Yin, H. T. Chung, U. Martinez, L. Lin, K. Artyushkova and P. Zelenay, J. Electrochem. Soc., 2019, 166, F3240–F3245 CrossRef CAS.
- H. G. Zhang, S. Ding, S. Hwang, X. L. Zhao, D. Su, H. Xu, H. P. Yang and G. Wu, J. Electrochem. Soc., 2019, 166, F3116–F3122 CrossRef CAS.
- H. M. Barkholtz and D. J. Liu, Mater. Horiz., 2017, 4, 20–37 RSC.
- M. L. Xiao, J. B. Zhu, L. Ma, Z. Jin, J. Ge, X. J. Deng, Y. Hou, Q. G. He, J. K. Li, Q. Y. Jia, S. Mukerjee, R. Yang, Z. Jiang, D. S. Su, C. P. Liu and W. Xing, ACS Catal., 2018, 8, 2824–2832 CrossRef CAS.
- S. Ratso, N. R. Sahraie, M. T. Sougrati, M. Kaarik, M. Kook, R. Saar, P. Paiste, Q. Y. Jia, J. Leis, S. Mukerjee, F. Jaouen and K. Tammeveski, J. Mater. Chem. A, 2018, 6, 14663–14674 RSC.
- S. Ratso, M. T. Sougrati, M. Kaarik, M. Merisalu, M. Rahn, V. Kisand, A. Kikas, P. Paiste, J. Leis, V. Sammelselg, F. Jaouen and K. Tammeveski, ACS Appl. Energy Mater., 2019, 2, 7952–7962 CrossRef CAS.
- L. C. Pardo, N. R. Sahraie, J. Melke, P. Elsässer, D. Teschner, X. Huang, R. Kraehnert, R. J. White, S. Enthaler, P. Strasser and A. Fischer, Adv. Funct. Mater., 2018, 28(22), 1707551 CrossRef.
- N. Ranjbar Sahraie, J. P. Paraknowitsch, C. Göbel, A. Thomas and P. Strasser, J. Am. Chem. Soc., 2014, 136, 14486–14497 CrossRef CAS.
- G. P. Hao, N. R. Sahraie, Q. Zhang, S. Krause, M. Oschatz, A. Bachmatiuk, P. Strasser and S. Kaskel, Chem. Commun., 2015, 51, 17285–17288 RSC.
- R. Chenitz, U. I. Kramm, M. Lefevre, V. Glibin, G. X. Zhang, S. H. Sun and J. P. Dodelet, Energy Environ. Sci., 2018, 11, 365–382 RSC.
- Y. Y. Shao, J. P. Dodelet, G. Wu and P. Zelenay, Adv. Mater., 2019, 31, 1807615 CrossRef.
- C. H. Choi, H. K. Lim, M. W. Chung, G. Chon, N. R. Sahraie, A. Altin, M. T. Sougrati, L. Stievano, H. S. Oh, E. S. Park, F. Luo, P. Strasser, G. Drazic, K. J. J. Mayrhofer, H. Kim and F. Jaouen, Energy Environ. Sci., 2018, 11, 3176–3182 RSC.
- H. T. Chung, C. M. Johnston and P. Zelenay, ECS Trans., 2009, 25, 485–492 CrossRef CAS.
- K. Kumar, P. Gairola, M. Lions, N. Ranjbar-Sahraie, M. Mermoux, L. Dubau, A. Zitolo, F. Jaouen and F. Maillard, ACS Catal., 2018, 8, 11264–11276 CrossRef CAS.
- F. Jaouen and J.-P. Dodelet, J. Phys. Chem. C, 2007, 111, 5963–5970 CrossRef CAS.
- A. Zitolo, N. Ranjbar-Sahraie, T. Mineva, J. K. Li, Q. Y. Jia, S. Stamatin, G. F. Harrington, S. M. Lyth, P. Krtil, S. Mukerjee, E. Fonda and F. Jaouen, Nat. Commun., 2017, 8, 957 CrossRef PubMed.
- N. R. Sahraie, U. I. Kramm, J. Steinberg, Y. J. Zhang, A. Thomas, T. Reier, J. P. Paraknowitsch and P. Strasser, Nat. Commun., 2015, 6, 8618 CrossRef CAS.
- T. Mineva, I. Matanovic, P. Atanassov, M. T. Sougrati, L. Stievano, M. Clemancey, A. Kochem, J. M. Latour and F. Jaouen, ACS Catal., 2019, 9, 9359–9371 CrossRef CAS.
- L. Osmieri, R. K. Ahluwalia, X. H. Wang, H. T. Chung, X. Yin, A. J. Kropf, A. E. Y. Park, D. A. Cullen, K. L. More, P. Zelenay, D. J. Myers and K. C. Neyerlin, Appl. Catal., B, 2019, 257, 117929 CrossRef CAS.
- Q. Y. Jia, E. S. Liu, L. Jiao, S. Pann and S. Mukerjee, Adv. Mater., 2019, 31, 1805157 CrossRef.
- K. Artyushkova, A. Serova, H. Doan, N. Danilovic, C. B. Capuano, T. Sakamoto, H. Kishi, S. Yamaguchi, S. Mukerjee and P. Atanassov, J. Electron Spectrosc. Relat. Phenom., 2019, 231, 127–139 CrossRef.
- V. Armel, S. Hindocha, F. Salles, S. Bennett, D. Jones and F. Jaouen, J. Am. Chem. Soc., 2017, 139, 453–464 CrossRef.
- C. S. Fadley, J. Electron Spectrosc. Relat. Phenom., 2010, 178-179, 2–32 CrossRef.
- Y. Chen, I. Matanovic, E. Weiler, P. Atanassov and K. Artyushkova, ACS Appl. Energy Mater., 2018, 1, 5948–5953 CrossRef.
- M. J. Dzara, K. Artyushkova, S. Shulda, M. B. Strand, C. Ngo, E. J. Crumlin, T. Gennett and S. Pylypenko, J. Phys. Chem. C, 2019, 123, 9074–9086 CrossRef CAS.
- D. Malko, T. Lopes, E. Symianakis and A. R. Kucernak, J. Mater. Chem. A, 2016, 4, 142–152 RSC.
- D. v. Deak, D. Singh, J. C. King and U. S. Ozkan, Appl. Catal., B, 2012, 113–114, 126–133 CrossRef.
- J. L. Kneebone, S. L. Daifuku, J. A. Kehl, G. Wu, H. T. Chung, M. Y. Hu, E. E. Alp, K. L. More, P. Zelenay, E. F. Holby and M. L. Neidig, J. Phys. Chem. C, 2017, 121, 16283–16290 CrossRef CAS.
- M. W. Chung, G. Chon, H. Kim, F. Jaouen and C. H. Choi, ChemElectroChem, 2018, 5, 1880–1885 CrossRef CAS.
- M. S. Thorum, J. M. Hankett and A. A. Gewirth, J. Phys. Chem. Lett., 2011, 2, 295–298 CrossRef CAS.
- Y. Chen, K. Artyushkova, S. Rojas-Carbonell, A. Serov, I. Matanovic, C. Santoro, T. Asset and P. Atanassov, ACS Appl. Energy Mater., 2018, 1, 1942–1949 CrossRef.
- N. R. Sahraie, U. I. Kramm, J. Steinberg, Y. Zhang, A. Thomas, T. Reier, J. P. Paraknowitsch and P. Strasser, Nat. Commun., 2015, 6, 8618 CrossRef.
- D. Malko, A. Kucernak and T. Lopes, Nat. Commun., 2016, 7, 13285 CrossRef.
- F. Luo, C. H. Choi, M. J. M. Primbs, W. Ju, S. Li, N. D. Leonard, A. Thomas, F. Jaouen and P. Strasser, ACS Catal., 2019, 9, 4841–4852 CrossRef.
- N. D. Leonard, S. Wagner, F. Luo, J. Steinberg, W. Ju, N. Weidler, H. Wang, U. I. Kramm and P. Strasser, ACS Catal., 2018, 8, 1640–1647 CrossRef.
- D. Malko, A. Kucernak and T. Lopes, J. Am. Chem. Soc., 2016, 138, 16056–16068 CrossRef CAS PubMed.
- A. Zitolo, V. Goellner, V. Armel, M. T. Sougrati, T. Mineva, L. Stievano, E. Fonda and F. Jaouen, Nat. Mater., 2015, 14, 937–942 CrossRef CAS.
- V. Armel, J. Hannauer and F. Jaouen, Catalysts, 2015, 5, 1333–1351 CrossRef CAS.
-
F. Rouquerol, J. Rouquerol, K. S. W. Sing, P. Llewellyn and G. Maurin, Adsorption by Powders and Porous Solids: Principles, Methodology and Applications, Academic Press, Oxford, 2014 Search PubMed.
- S. Kabir, K. Artyushkova, A. Serov, B. Kiefer and P. Atanassov, Surf. Interface Anal., 2016, 48, 293–300 CrossRef CAS.
- W. Ju, A. Bagger, G. P. Hao, A. S. Varela, I. Sinev, V. Bon, B. Roldan Cuenya, S. Kaskel, J. Rossmeisl and P. Strasser, Nat. Commun., 2017, 8, 944 CrossRef PubMed.
- J. Y. Park, D. H. Kwak, K. B. Ma, S. B. Han, G. S. Chai, S. K. Kim, D. H. Peck, C. S. Kim, A. Kucernak and K. W. Park, J. Catal., 2018, 359, 46–54 CrossRef CAS.
- X. Zhang, C. Chen, J. Dong, R.-X. Wang, Q. Wang, Z.-Y. Zhou and S.-G. Sun, ChemElectroChem, 2018, 5, 3946–3952 CrossRef CAS.
- K. Kumar, P. Gairola, M. Lions, N. Ranjbar-Sahraie, M. Mermoux, L. Dubau, A. Zitolo, F. Jaouen and F. Maillard, ACS Catal., 2018, 11264–11276, DOI:10.1021/acscatal.8b02934.
- A. Bonakdarpour, M. Lefevre, R. Yang, F. Jaouen, T. Dahn, J.-P. Dodelet and J. R. Dahn, Electrochem. Solid-State Lett., 2008, 11, B105 CrossRef CAS.
- P. Teppor, R. Jäger, E. Härk, I. Tallo, U. Joost, M. Kook, P. Paiste, K. Šmits, K. Kirsimäe and E. Lust, J. Electrochem. Soc., 2018, 165, F1217–F1223 CrossRef CAS.
- X. Li, H.-J. Zhang, H. Li, C. Deng and J. Yang, ECS Electrochem. Lett., 2014, 3, H33–H37 CrossRef CAS.
- E. J. Biddinger, D. v. Deak, D. Singh, H. Marsh, B. Tan, D. S. Knapke and U. S. Ozkan, J. Electrochem. Soc., 2011, 158, B402–B409 CrossRef CAS.
- F. d. r. Jaouen and J.-P. Dodelet, J. Phys. Chem. C, 2009, 113, 15422–15432 CrossRef CAS.
- W. J. Jiang, L. Gu, L. Li, Y. Zhang, X. Zhang, L. J. Zhang, J. Q. Wang, J. S. Hu, Z. Wei and L. J. Wan, J. Am. Chem. Soc., 2016, 138, 3570–3578 CrossRef CAS.
Footnotes |
† Electronic supplementary information (ESI) available. See DOI: 10.1039/d0ee01013h |
‡ These authors contributed equally. |
|
This journal is © The Royal Society of Chemistry 2020 |
Click here to see how this site uses Cookies. View our privacy policy here.