Thermochemical unification of molecular descriptors to predict radical hydrogen abstraction with low computational cost†
Received
14th July 2020
, Accepted 22nd September 2020
First published on 23rd September 2020
Abstract
Chemistry describes transformation of matter with reaction equations and corresponding rate constants. However, accurate rate constants are not always easy to get. Here we focus on radical oxidation reactions. Analysis of over 500 published rate constants of hydroxyl radicals led us to hypothesize that a modified linear free-energy relationship (LFER) could be used to predict rate constants speedily, reliably and accurately. LFERs correlate the Gibbs activation-energy with the Gibbs energy of reaction. We calculated the latter as the sum of one-electron transfer and, if appropriate, proton transfer. We parametrized specific transition state effects to orbital delocalizability and the polarity of the reactant. The calculation time for 500 reactions is less than 8 hours on a standard desktop-PC. Rate constants were also calculated for hydrogen and methyl radicals; these controls show that the predictions are applicable to a broader set of oxidizing radicals. An accuracy of 30–40% (standard deviation) with reference to reported experimental values was found suitable for the screening of complex chemical systems for possibly relevant reactions. In particular, potentially relevant reactions can be singled out and scrutinized in detail when prioritizing chemicals for environmental risk assessment.
1. Introduction
Free radicals are produced (photo)chemically in living cells, surface water, electrochemical devices and many other systems. A significant number of free radicals are reactive and oxidize molecules via diverse reaction pathways. They are important in the context of electrochemistry,1 environmental science2 and biology,3 and there is an interest to understand reaction pathways and to quantify the rates of reaction. Experimental methods to determine the rate constant, kr, of a chemical reaction are in general laborious.4 Besides the cost of instrumentation and lab space, measuring a single rate constant with proper controls and data evaluation easily takes a skilled experimenter 3 days.
With 100
000+ chemicals to be assessed,5,6 there is a growing need to supplement experiments with computational methods to reduce costs and increase the sample variation and throughput.7–9 With this large number of chemicals, not all the reaction rate constants and pathways can be calculated with ultimate precision. Instead, we need ‘cheap’ calculus methods which are accurate and precise enough to (1) determine the rate constants of the bulk of reactions and (2) identify those reactions that require further, more detailed and expensive scrutiny.
Prediction of kr is possible via, for example, ‘machine learning’ approaches which use a variety of algorithms and easily retrievable topological, electrostatic, and energetic parameters.2 The approaches yield relationships of mixed quality that depend on the type of radical and substrate considered. Many machine learning methods are ‘black box’ approaches, not supporting facile interpretation in terms of reaction mechanisms. In addition, parameters can be ill-defined for molecules that are complex or outside the model's domain of applicability.10–12 A different line of research aims to predict kr mechanistically. ‘Ab initio’ methods base calculations of kr on an accurate and precise determination of the free energy of activation ΔG‡. The rate constant, kr, of a chemical reaction is described by the Eyring-equation as13
| 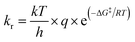 | (1) |
Δ
G‡ is the free energy of activation,
R the universal gas constant,
T the absolute temperature and
q a statistical factor. The
ab initio methods involve the exploration of free energy changes along the reaction coordinate but the time-dependent three-dimensional interactions involved take much computing power for a full description.
14 Simplifications might use static configurations for reactants or transition states, but require foreknowledge on the reaction pathway. Moreover, the statistical factor
q (
eqn (1)) has to be described separately;
q differs between non-spherical ‘complex’ reactants.
15
Simplifications of the Eyring-equation are free-energy relationships, made famous by the work of Hammett and Taft.16,17 For reactions with a closely similar rate-determining step, LFER stipulates that differences in Gibbs energy of activation, or the ratio of rate constants kr1/kr2 for two substrates 1 and 2, are proportional to the difference in the reaction free energy change ΔGr, eqn (2) and Fig. 1:
| 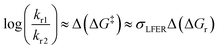 | (2) |
where
σLFER is the substituent (fitting) constants.
16,18 This approach has been successful because it uses semi-empirical parameters, hence requires less effort as compared to
ab initio methods. Thermodynamic values, such as the Gibbs energies of reaction Δ
Gr, are often available from literature or comparatively easy to estimate computationally and are, therefore, ‘cheap’.
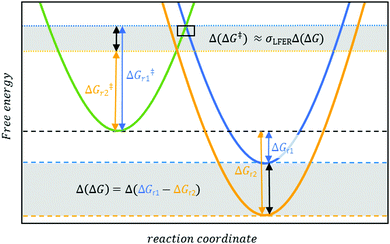 |
| Fig. 1 Linear free energy relationships establish that, in a first approximation, the difference in activation energies Δ(ΔG‡) (dotted lines) is proportional (σLFER as the proportionally constant) to the differences in Gibbs energies Δ(ΔG). (dashed lines) between reactions, e.g. r1 (blue curve) and r2 (yellow curve). The concept16 offers the prospect of efficient computational methods to predict ΔG‡ and, by extension, kr. The rectangle symbol at the intercept between green and blue curves refers to Fig. 2. | |
LFER predictions are possible for reactions of chemicals with conventional, ‘non-exotic’, substituents19 and when σLFER values are available (Fig. 1). Many LFERs developed over the years predict Δ(ΔG‡) with reasonable accuracy. Comparison of Δ(ΔGr) with experimental data allowed to approximate σLFER for various radicals (e.g. O2˙−/HOO˙ and aryl radicals20,21). Curiously, for those radicals, σLFER was identical (i.e. within 2 standard deviations). Given these findings,18,20–22 we hypothesized σLFER to be a constant factor for a broader set of radicals. This implies that all radical-induced one-electron transfers have a similar rate-determining step.
The prediction of Δ(ΔG‡) via LFER is a successful, albeit crude estimation, eqn (2). Changing solvents, i.e. different dielectric constants of the environment, is for instance known to alter product distribution and reaction kinetics.23 This is direct evidence that charge polarization in the transition state (TS) can affect the Gibbs energies of activation for a specific transition state, and thus promote/inhibit a specific reaction pathway. In other words, there are additional, usually smaller, contributions to the activation energy, Δ(ΔG‡TS), that we need to consider,24eqn (3):
| Δ(ΔG‡) = σLFERΔ(ΔGr) + Δ(ΔG‡TS) | (3) |
Thus, the practical problem is the development of computationally efficient calculus of the quantum chemical influences on the transition states hidden in the term Δ(Δ
G‡TS),
Fig. 2:
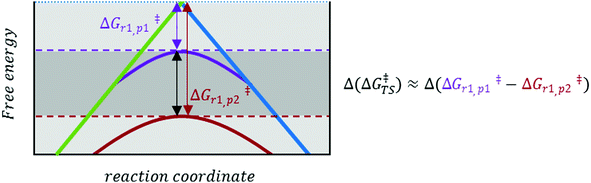 |
| Fig. 2 Enlarged version of the black rectangle in Fig. 1. Depending on the nature and environment of the reaction site, a specific pathway (p1 or p2, indicated by purple and red curves, respectively) may be favoured. This is indicated by a ‘perturbation’ Δ(ΔG‡TS), of the ‘normal’ LFER behaviour (LFER depicted in Fig. 1). | |
We therefore chose to calculate rate constants with a modified LFER approach that combines the ‘cheap’ calculus of the free-energy of reaction with selective ‘ab initio’-style additions. The selection reflects an analysis of over 500 published reactions of hydroxyl radicals. The resulting factors governing the rate constants originally caused us to embrace LFER terminology for the explanation of our work.
In our study, we investigated the details in transition states for one-electron oxidations, i.e. reactions that involve the net transfer of a single electron and, possibly, a single proton. We explain our selection of easily accessible parameters to successfully calculate and predict Δ(ΔG‡TS). With it, we estimate rate constants for one-electron transfer, or radical oxidation, reactions.
2. Methods
Linear free-energy relationships (LFERs) assume that Δ(ΔGr) relates to kr1/kr2 under the condition of an ‘identical’ mechanism, the same number of reacting sites and similar geometry. Eqn (2) allows for calculation of Δ(ΔG‡) and kr1/kr2 based on knowledge of Δ(ΔGr).
For a successful use of LFER, all parameters for the basic eqn (2), prominently the proportionality constant σLFER, need to be derived. Our parametrization process, together with the introduction of all the modifications (Δ(ΔG‡TS) and the statistical factor q, eqn (1) and (3), respectively), is described step by step in the Sections 2.1–2.3.
The parametrization was developed with known reactions of HO˙ radicals, a case with ample experimental data (ESI,† S1), and opting for a model with a minimal set of parameters that would allow for ‘cheap’ and reliable calculation of unknown rate constants. We chose to calculate the Gibbs energy of reaction, ΔGr, as per reactive site, which ensures the same number of reacting sites in the LFER (i.e. one). The total reactivity of the molecule is then calculated as the sum of the reactivities of all individual R–H bonds.
Subsequently, the approach as outlined above was validated with data from reactions of HOO˙, H˙ and CH3˙ (see Results section). In the validation process, the derived value for σLFER and the calculated values for Δ(ΔGr) and q were used to predict kr1/kr2, which in turn was compared to experimental data.
2.1. Gibbs energy calculations: ΔGr
Eqn (2) predicts that prominent influences on Δ(ΔG‡) are the Gibbs energy of the reaction Δ(ΔGr) and the scaling factor σLFER. Empirically, we used values for Δ(ΔG‡) for one-electron oxidation reactions (e.g. blue and yellow in Fig. 1) to quantify the relevance of Δ(ΔGr). Thereby, we also determined σLFER. As we calculated the values for Δ(ΔG‡) per reaction site, they do not include the statistical factor (Section 2.3).
Perturbation theory leads to a formula to calculate ΔGr based on ‘frontier orbital’ interaction and charge transfer.25 In its simplified form (S3, ESI†), the reaction free energy change for oxidation reactions is described as:
| ΔGr = ΔGET,ox − ΔGCT = ΔGET,ox − ΔGPT | (4) |
with possible driving forces being oxidative electron transfer (ET,ox) and additional “charge transfer” (CT),
e.g. proton-transfer (PT). The reactions considered do not imply a change in net charge. Therefore,
eqn (4) reflects negligible changes in the Gibbs-energy of solvation.
Any parametrization routine starts with the parameters of large influence and subsequently proceeds those with smaller impact. The most prominent contributions to the activation energy, ΔG‡, are correlated to ET and PT, i.e. their contributions are most influential. By definition, no reactions occur without electrons. Hence, we began with the calculation of the contribution of ET.
2.1.1. Transfer of the electron.
The ΔG for ET relates to interactions between the highest occupied molecular orbital (HOMO) and the lowest unoccupied molecular orbital (LUMO) of the closed-shell molecule R− and the singly occupied molecular orbital (SOMO) of the radical X˙, the so-called “frontier orbitals”.26 Outer-sphere ET is frontier-controlled (energetically and spatially) and does not involve charge-transfer complexes.27–29 We describe oxidative ET via the energy difference in the frontier orbitals, ESOMO − EHOMO as: | 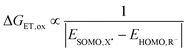 | (5) |
For computational details and associated uncertainties, we refer to the ESI† (S2). EHOMO (pure electron transfer) reflects the lower limit of the rate constant (Fig. S3A, ESI† and Fig. 6A). The majority of radical redox reactions are several orders of magnitude faster than that. In other words, the assumption of pure electron transfer often underestimates how favorable the reaction is, i.e. it overestimates the Gibbs activation energy of reaction.
2.1.2. Transfer of the proton.
Compared to outer-sphere ET, hydrogen abstraction (HA) involves additionally the transfer of a positive charge not the result of the frontier electron (eqn (5) and eqn (S1), ESI†). If a formal transfer of both an electron and a proton takes place, i.e. with proton-coupled electron transfer (PCET) or hydrogen atom transfer (HAT), energies of proton dissociation (pKa-values) need to be included. The overall result of the reaction does not involve charge transfer or even charge separation.
The homolytic bond dissociation enthalpy relates to the pKa of R–H and the electron affinity of the radical R˙.30–32 This applies to X–H as well. The enthalpy change of a reaction between R–H and X˙ involves an analysis of the relative bond strengths (blue rectangles in Fig. 3). Given the proton dissociation constants pKa(R–H) and pKa(X–H) of the conjugate hydrogen donor X–H of the attacking radical X˙, respectively, eqn (6):
| EpK = 2.3RT [pKa(R–H) − pKa(X–H)] | (6a) |
Frontier orbital (
EHOMO,
eqn (5)) and charge transfer parameters (p
Ka) can, therefore, be combined into Δ
Gr:
| 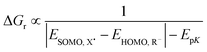 | (6b) |
If the p
Ka's of R–H and X–H are equal, we return to
eqn (5) (pure ET). We obtained p
Ka values from the literature and, when unavailable, by regression with atomic charges (S2, ESI
†). The thermodynamic cycle in
Fig. 3 reflects graphically the calculation of Δ
Gr values for HA:
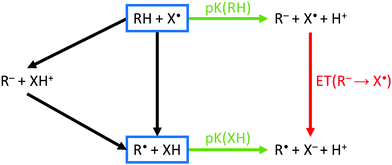 |
| Fig. 3 Thermochemical cycle for H-abstraction. The reaction driving force for both sequential (left) or concerted (center) mechanisms is described using proton transfer (pKa, green arrows) and electron transfer (red arrow). Electron transfer in absence of protolysis (black). The colors of arrows correspond to the data in Fig. S3 (ESI†). Depending on the energies of proton/electron-transfer, an ionic or a non-ionic transition state is preferred, and the mechanism is sequential or concerted. | |
2.2. Quantum chemical corrections
In the Hammett/Taft equation (eqn (2)), σLFER is introduced to compare between congeneric chemicals, i.e. chemicals with ‘the same’ reaction mechanism, which we know is not the case for the reactions we include here: an electron transfer is not a hydrogen abstraction. In order to apply the LFER assumption, we need a correction for different mechanisms (purple and red in Fig. 2). Therefore, we chose to include the parameter Δ(ΔG‡TS) into eqn (3) with the assumption that Δ(ΔG‡TS) is distinctly smaller than σLFERΔ(ΔGr).
2.2.1. Transition state stabilization: Δ(ΔG‡TS).
In order to obtain ‘experimental’ values for Δ(ΔG‡TS) we compared our values for σLFERΔ(ΔGr) obtained above with Δ(ΔG‡), in eqn (7a): | 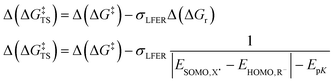 | (7a) |
with EpK = 2.3RT[pKa(R–H) − pKa(X–H)] (Section 2.2.2). Remember that Δ(ΔG‡) relates back to Section 2.1, eqn (7b): | 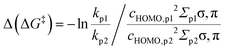 | (7b) |
where cHOMO2 and summation terms (eqn (8b)) characterize the statistical factor q (Section 2.3).
We calibrated Δ(ΔG‡TS) on ET and then introduced a correction for the involvement of protons, i.e. ‘the polarization of the bond’ in PCET and HAT. The so obtained range for Δ(ΔG‡TS) involving reactions with OH˙ was ∼13 kJ mol−1.
2.2.2. Parametrization of Δ(ΔG‡TS).
Contributions for the TS required refinement. For the calculus, we parametrized Δ(ΔG‡TS) using a computationally inexpensive method. The idea is to find a value that is proportional to the ‘experimental’ one and to derive the proportionality constant (here ‘z’, see below). Assuming Δ(ΔG‡TS) ≪ σLFERΔ(ΔGr), its accuracy does not need to be as high as the latter, allowing for simplifications.
Charge polarization and solvent interactions in the TS depend on the mechanism (e.g. HAT, PCET), which depends on the type of H-donor involved. We distinguished types via atom-specific Fukui delocalizability indices.33 For computational details and associated uncertainties, we refer to S2 (ESI†). The index, computed for the R–H electron pair, can denote whether the H-atom has neighboring π-electrons (π) or not (σ). π-Transition states associate to allylic hydrogens (sp2 hybridized centers) and σ-transition states to non-allylic hydrogens (sp3 hybridized centers), Table T2 (ESI†).
To parametrize specific TS effects, we introduced a global scaling parameter z. We calculated the correction for the transition state stabilization with zσ = 0 for π-transition states and zπ = 0 for σ-transition states, eqn (8):
In
eqn (7),
μ is the local dipole moment surrounding R–H and
z was left for radical-specific empirical fitting. The slope of the lines in
Fig. 4 is the scaling parameter
z, denoting the strength of the proportionality between Δ(Δ
G‡TS) and the local dipole moment within the molecule. Note that if either
zσ =
μ = 0 or
zπ = 0, Δ(Δ
G‡TS) ≈ 0 (
Fig. 4). In this case, Δ
G‡ is directly proportional to Δ
G (which follows from
eqn (2)). As the dipole moment is tied to solvation free energies, the proportionality characterizes charge polarization in the TS.
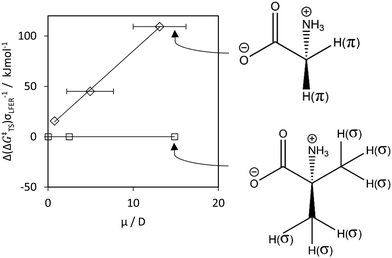 |
| Fig. 4 Δ(ΔG‡TS) for HA by OH˙ (y-axis) versus the local dipole moment μ (x-axis). We calculated Δ(ΔG‡TS) viaeqn (8), by taking the difference between 2 values: with μ, and with μ = 0, i.e. Δ(ΔG‡TS) = Δ(ΔG‡) – Δ(ΔG‡(μ = 0)). Δ(ΔG‡TS) characterizes the offsets between the LFERs in Fig. S8E (ESI†). Diamonds and squares denote π- and σ-type hydrogens, respectively solid lines denote data fits. We used the fit to parametrize the contribution by μ to ΔG‡. Molecules illustrate the combined impact of σ, π and μ on Δ(ΔG‡TS). | |
2.3. The statistical factor q
In the Eyring equation (eqn (1)) q is a statistical factor related to the reaction probability. Following eqn (8), we treated q as a product function: | q = qΣ(π,σ)qCHOMO(π,σ)2 | (9) |
q prominently includes the number of abstraction sites Σ(π,σ), Fig. S9-2 (ESI†). For compounds with only equivalent H atoms, i.e. symmetric molecules, we can use simple corrections based on symmetry.19 We derived the expected values for kr depending on σΔGr and the reaction pathway (TS effects, in Section 2.2). We filtered the data used for parametrization based on symmetry (see Table T2, ESI†) and then express qΣ(π,σ) for the resulting compounds as: | 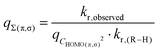 | (10) |
wherein the denominator is the expected rate constant for a single R–H bond (π = σ = 1). We can see that the values for qΣ(π,σ) vary by a factor 10, Fig. 5A. We thus used the number of ‘chemically equal’ hydrogen atoms Σ(π,σ) to account for this, Fig. 5A:
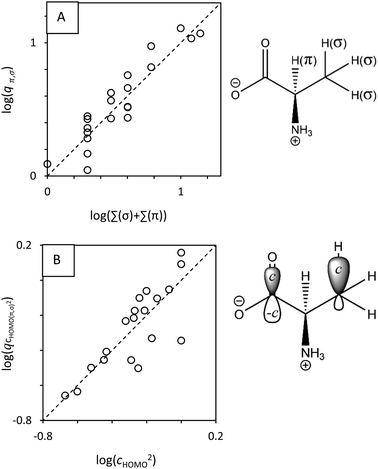 |
| Fig. 5 (A) The statistical factor q (on the y-axis), calculated using experimental data and eqn (8), is the number of equivalent H atoms, wherein π and σ denote the presence of either resonance stabilized or non-resonance stabilized mechanisms (which do not have neighboring π-electrons), respectively. The molecule illustrates π and σ: there are 3 equivalent σ hydrogens; 1 π hydrogen. (B) The statistical factor q (on the y-axes), calculated using experimental data and eqn (8). q correlates with the squared atomic orbital coefficient. The dashed line denotes theoretical relationships for symmetric molecules. | |
q can also be understood as the accessibility of abstraction sites, i.e. it comprises also the ‘effectiveness’ of collisions, the so-called quantum-mechanical ‘transmission’. By extension, the overlap between frontier orbitals is characteristic of the space in which the electron can move within the TS.26,34 Thus, we can express qCHOMO(π,σ)2 as function of squared AO coefficients, cHOMO2 (Fig. 5B):
| 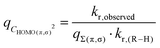 | (11) |
wherein the numerator is the expected rate constant for the molecule reflecting similar-sized orbitals (
cHOMO(π,σ)2 = 1). We then see that the values for
qCHOMO(π,σ)2 vary by almost a factor 10,
Fig. 5B.
We obtained atomic orbital (AO) coefficients (denoted as cHOMO, eqn (S1), ESI†) from quantum-mechanical calculations, denoting the ‘size’ or ‘shape’ of orbitals (Fig. 5B). Computational details and associated uncertainties are provided in S2 (ESI†). We thereby used the data for congeneric H-donors, to take into account the dependence of qCHOMO(π,σ)2 on AO coefficients, Fig. 5B.
3. Results
3.1. Linear free energy relationships
Within the (crude) model of LFER only (eqn (2)), ΔGr can predict ∼95% of the kr values (N ≈ 500) within ±1 order of magnitude of the expected value (Fig. S3B, ESI,†p < 1 × 10−5), i.e. for these 95%, the estimation of the activation energy ΔG‡ was accurate within 6 kJ mol−1.
Using symmetry-filtered data (Section 2.1), we found σLFER = log(kr)/ΔGr = 2.3 eV−1, i.e. the rate constant doubles if the Gibbs energy of the reaction decreases by 13 kJ mol−1. We obtained σLFER values (±2SD) of 2.4(±0.2) and 2.1(±0.2) p(kr)/eV for H˙ (Fig. 8) and 2.6 (±0.6) p(kr)/eV for CH3˙ (Fig. S12, ESI†). These values do no statistically differ from those for OH˙ or OOH˙/O2˙−
21 (within two SDs).
3.2. Transition state stabilization: Δ(ΔG‡TS)
For π-type systems, our calculated Δ(ΔG‡) shows a systematic deviation from the known rate data for water that is dependent on the dipole moment μ. In other words, hydrogen abstraction from non-polar hydrogen donors shows lower Δ(ΔG‡TS) compared to HA from polar and zwitterionic π-type hydrogen donors, Fig. 5. Parametrization of this effect resulted in a satisfactory fit of the rate constants, Fig. 6C.
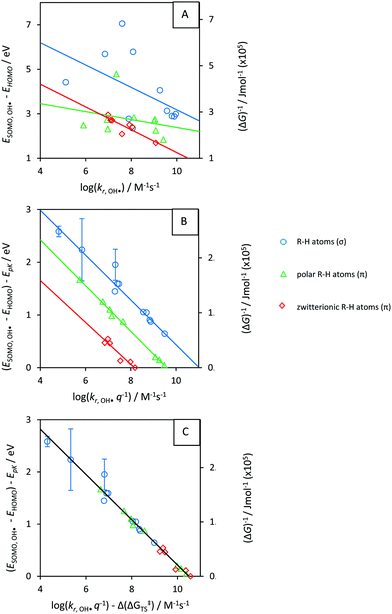 |
| Fig. 6 Rate constants (kr) for reaction with OH˙ (x), versus reaction energies (y). (A) krversus frontier orbital energies; (B) ΔG‡versus ΔGr; (C) ΔG‡ − Δ(ΔG‡TS) versus ΔGr. Only symmetric compounds (with equivalent R–H groups) are taken into account. Energies were converted using 100 kJ mol−1 ≙ 1.04 eV and 0 kJ ≙ −8 eV ≈ ESOMO(OH˙). Lines are data fits. Error bars indicate uncertainty from predicted pKa (Chemaxon program35). Full parametrization in Fig. S8 and Table T2 (ESI†). | |
As a validation, we used the parametrized result to predict kr. for OOH˙, H˙ and CH3˙. Aqueous-phase abstraction by H˙ of a π-type H atom from a zwitterionic compound is ≈200 times slower (≈2.3 in log units, Fig. 7) than for a σ-type H atom. The difference in terms of Δ(ΔG‡) is ≈13 kJ mol−1 (≈100 kJ mol−1 in terms of Δ(ΔGr)). The values match those for Δ(ΔG‡TS) for HA involving OH˙ (Fig. S8E, ESI†) and CH3˙ (Fig. S12A, ESI†). However, propene (purple star in Fig. 7) did not fit our prediction (see Discussion, Section 4.3.1). S4 (ESI†) provides additional controls.
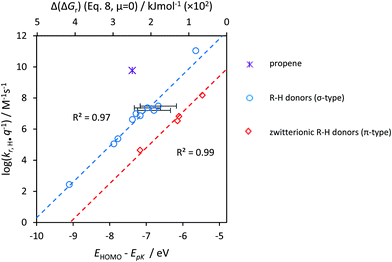 |
| Fig. 7 The product of the rate constant (kr) and the statistical factor q, logarithmically transformed, for reaction with H˙(y) versus ΔGr(x). Dashed lines denote the LFERs. Red diamonds are π-type zwitterionic R–H and blue circles are σ-type R–H. Error bars indicate uncertainty introduced upon prediction of pKa.35 Only compounds with equivalent X–H groups are taken into account. The purple star is propene (outlier, discussed in Section 4.3.1). 100 kJ mol−1 ≙ 1.04 eV; 0 kJ ≙ −5 eV, details in S2; Table T3 (ESI†). | |
3.3. The influence of radical electron affinity ex
As explained above, we developed our method with reactions of OH˙ radicals, where ample data is available. The predictions were very satisfactory. However, when we extended our database with controls for HOO˙, H˙, and CH3˙ (Tables T2–T5, ESI†), some minor systematic, radical-specific deviations from prediction became evident.
Eqn (8) contains 2 radical-specific properties: pKa(X–H) and ESOMO,X˙. The error, therefore, is introduced in one of the two values. As we used the experimentally determined value of pKa(X–H) for HOO˙, H˙ and HO˙, we assume the error to relate to our calculation of ESOMO,X˙ (S2, ESI†). We then decided to derive experimental values for ESOMO,X˙ (via fitting) and obtained an ‘electron affinity’, ex. If we compare the two values, Fig. 8, they are identical for the OH˙ radical: eOH˙ = 8.0 (±0.5) ≈ ESOMO,OH˙ = 8.0 (±0.2) eV. For best intercomparison between radicals, ESOMO,X˙ has to be replaced with ex in eqn (8):
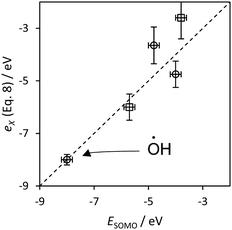 |
| Fig. 8 Comparison of the electron affinity, ex, with ESOMO,X˙. The dashed line denotes interaction between frontier orbitals allowing oxidative outer-sphere electron transfer. Data for O2˙− (both abstraction and addition reactions) and HOO˙s indicated by squared symbols were taken from ref. 21. p < 0.05 (2SD). | |
4. Discussion
With our method for calculus, we can estimate rate constants of one-electron oxidation processes with a statistical reproducibility of R2 = 0.98 and with a confidence (±1 SD, i.e. 68%) of 30–40%. In other words, the activation energy is predicted with an accuracy of around 1 kJ mol−1. We want to compare the result to errors from experimental data: rate data for fast kinetics with rate constants >106 M−1 s−1 are often reported with precisions better than 10%. However, results of different labs routinely yield a distinctly higher scatter (20–40%). One may assume, therefore, that the accuracy of data often cannot keep up with their reported precision. Our calculations have accuracies comparable to the accuracy of preliminary experiments.
Our method uses a minimal set of parameters to predict kinetic rate constants, though similar (but substantially larger) sets recur in both machine learning and ab initio methods. Because of the minimization, parameters may embed more than 1 influence. However, for our examples and controls, the parametrization appears sufficient. Rather than quantifying explicit contributions to Δ(ΔG‡) via ‘expensive’ ab initio methods, our parametrization is computationally ‘cheap’. Calculations were carried out on a standard desktop computer and for the ±40% prediction accuracy they took 5 seconds per molecule. Such a low-cost approach is very handy for selecting possibly relevant reactions in a complex reaction scheme. It enables, for example, large-scale screening of aquatic reactivity in environmental chemistry36,37 that can single out possibly relevant reaction pathways. This is the application for which the current method was developed. Then, in a second step, more accurate and precise data can be acquired either by experimental work, more sophisticated LFER-based methods,22,24,38,39 or even extensive ab initio calculation.
We initially assumed that the influence of ET and PT would be largest, whereas other influences were assumed to be distinctly smaller. Statistical evaluation of data supports this notion (Fig. S10, ESI†). Thus, our parametrization mainly relies on the assumption of a linear free-energy relationship between Gibbs energy of reaction and the Gibbs activation-energy of the same reaction. The former is given by the sum of the electron transfer and, if applicable, a proton transfer. We calculated the energies of electron and proton transfer via the energies of frontier orbitals and pKa values, respectively. We combined the terms via a thermodynamic cycle and Klopman's equation.25
The exact values for activation energies were then refined by estimation of relative (de-)stabilization of transition states by local delocalization and polarization. This process is reiterated over all possible reactive sites of a molecule. The measured rate constant then is equal to the sum of all rate constants for individual reactive sites. Our initial hypothesis of a closely similar rate determining step for the one-electron oxidations by HOO˙, H˙, CH3˙ and HO˙ appears to be correct; our method is applicable for multiple radicals (Fig. 6–8 and Fig. S12, ESI†).
4.1. Statistical factor
With symmetric molecules, inclusion of the statistical factor q via Σ(σ) + Σ(π) (see Fig. 3A) led to better correlations between kr and ΔG between and within H-donor types (Fig. S8C compared to Fig. S8B, ESI†). Similar findings were reported for unsaturated organics.40,41 The inclusion of q via cHOMO (Fig. S8D, ESI†) marginally improved the correlations (Fig. S10, ESI†), whereas it slightly converged the offsets of the regressions for π-type X–H compared to σ-type X–H bonds (Fig. S8D compared to Fig. S8C, ESI†). In contrast, the statistical factor q (as described via cHOMO,R−, within a factor 5) did not improve the LFER for ET (N = 36, Fig. S2 and Table T1, ESI†). Note that we can also interpret the influence of cHOMO in terms of ΔGrvia Klopman's equation (eqn (S1), ESI†).25
If q was included in the calculation model, the slopes of the LFERs (i.e. the substituent constants, σLFER) for OH˙ converged to ≈2.3 p(kr)/eV (Fig. 6B and Fig. S2, S8D (ESI†)). The slopes do not differ between π and σ H-atom types. Thus, q improves the prediction accuracy of kr. The overall reaction rate constant is a combination of the accessibility of each individual hydrogen atom that can be abstracted (i.e. q), and depends on the reaction dynamics.42–44 Depending on the dynamics, symmetry arguments (Table T2, ESI†) may reduce the computational effort needed.
4.2. Driving forces ΔGr
4.2.1. Electron transfer versus hydrogen abstraction.
For electron transfer by OH˙ (eqn (2) and (3)), the σLFER (Hammett substituent constant) value, ±2SD, is 2.2 ± 0.2 p(kr)/eV, similar to that of HOO˙/O2˙− (σLFER = 2.1–2.5 p(kr)/eV) including ET.21 Using a thermodynamic cycle (eqn (6)), we found that slopes of the LFERs (σLFER) for OH˙ involving different types of H-donors all converged to ∼2.3p(kr)/eV (0.024p(kr)/(kJ mol−1)). Thus, it appeared to us that the values for ET and HA do not significantly differ. For both, it implies doubling of the rate constant with every −13 kJ mol−1 decrease of ΔGr.
HA by OH˙ is usually faster than ET.45,46 Hence, the observed pathway is usually HA (similar for addition, Fig. S14, ESI†). Our calculations give clear hints on the expected reaction mechanism. For example, the inclusion of ET only in the calculus may predict a very low rate constant as compared to the case of PCET/HAT. In that case, the contribution from proton-transfer is significant, and ET is not the prominent mechanism. In other words, the influence of pKa on the ΔGr values (eqn (6)) is non-negligible.
If experimental data are available, and if the calculated rate constant is significantly lower than the observed one, a misconception about the mechanism is to be expected. An obvious case is the reaction of the hydrogen atom with propene (outlier in Fig. 7). There, the observed reaction with H˙ is probably addition,47 not an oxidation (Tables T2–T5, ESI†). Compared to OH˙ and OOH˙, which react as electron acceptors with most organic chemicals (Fig. S3B, ESI,†
21), H˙ and CH3˙ might not (eqn (4)). Provided a correct assumption of mechanism, the applicability of the method is retained and eqn (8) can predict kr for different radicals (Fig. 6–8).
The influence of pKa on the reaction rate (eqn (6)) can be interpreted as a Coulombic interaction between the hydrogen atom of R–H and X˙, e.g. the oxygen atom of OH˙. A larger value for QH (lower pKa for R–H) implies greater electrostatic stabilization and higher kr. Provided that the solvent is inert, a higher pKa of R–H implies a proportionally lower contribution of proton transfer to ΔGr. (eqn (6)). Within the model of LFER, HA is expected to be faster than ET if the reaction is more favorable thermodynamically, i.e. the products including H2O (HA) are more stable than those including OH− (ET).
4.2.2. Comparison with other methods.
Machine learning and ab initio methods require separate models and simulations for individual chemicals. For example, charge-transfer interactions are described explicitly in ab initio methods, which consider charge transfer as a function of time and distance. Charge-based descriptors also recur in many QSPR models.2,48 However, proton transfer can also be characterized by static (cheap) descriptors; the use of pKa (Section 2.2.2) takes less computational effort.
LFERs for ET and HA exist for OH˙, OOH˙/O2˙−, H˙ and CH3˙, with similar σLFER values (Table T5, ESI†). Thus, the individual LFERs are not phenomenological,18 and the potential energy curves of the reactants can be described by a uniform method, e.g. a harmonic oscillator (depicted in Fig. 1) or Morse potential. In contrast, the relevance of EHOMO and MoRSE potential2,49,50 parameters and the QSPR model algorithm architecture differs between radical species. As MoRSE derives from molecular vibrational structure,51,52 specification of potential curves24,53 might facilitate extrapolation to a broader set of radicals.
The optima in kr for OH˙, OOH˙, H˙ and CH3˙ generally correspond to cases when |ESOMO − EHOMO| → 0 (Fig. 8); the reactivity sequence (Tables T2–T4, ESI†) is in line with previous studies.54–57ESOMO relates to electron accepting/donating ability, electrophilicity (S5, ESI†)54 and ‘electron affinity’, ex (Fig. 8 and Fig. S11, S12, ESI†).54 We did not consider specific terms for orbital shapes of radicals (eqn (S1) and (S2), ESI†) in the current study. Machine learning methods use associated terms (e.g. radial density function descriptors58,59). Hence, a direct description of orbital–orbital overlap and orbital symmetry factors may also characterize electron affinity and improve ΔGr predictions.
4.3. Transition state stabilization: Δ(ΔG‡TS)
4.3.1. Proton-coupled electron transfer versus hydrogen atom transfer.
The LFERs for HA were developed using a thermochemical cycle (Fig. 3) based on the assumption that the relative proton affinities of the reactants are not influenced by ET, and vice versa. In the case of a concerted mechanism, e.g. proton-coupled electron transfer (PCET),60,61 ‘low’ ΔG‡ might be involved. Though at elevated temperature, abstraction of a ring and benzylic hydrogen dominate over addition, indicating larger ΔG‡ values.62 In such cases, sequential mechanisms might be involved. Indeed, Fig. 6B and 7 illustrate the involvement of Δ(ΔG‡TS) and the presence of mechanistically distinct pathways. In analogy, Pompe et al. predicted kr based on differentiation between C–H bond types.63,64
For H-donors with neighboring π-electrons (π in Fig. 5), μπ positively correlates with ΔG‡, eqn (8). This is because charge redistribution in the TS is involved. Abstraction of ‘π-type hydrogens’ normally occurs via proton transfer between the R–H and X˙ (σ-orbitals), but the electron transfers from the adjacent sp2-π orbital (only atomic orbitals of the same symmetry can combine65). This often involves relatively high ΔG‡. In analogy, captodative stabilization can influence ΔG‡.
The rate is faster in H2O if the reaction involves movement of only 1 electron, rather than 3 electrons, or when the proton and electron are transferred between the same sets of orbitals66 (e.g. PCET reactions). This fits with the data for e.g. ammonia and 2-methylalanine (Table T2). These are polar and zwitterionic compounds, respectively, but OH˙ abstracts both the electron and proton via the σ R–H bond (‘σ-type’ H-atom) since there are no neighboring π-electrons. Hence their ‘high’ reactivity as compared to molecules containing polar and zwitterionic ‘π-type’ H atoms (Fig. S8E, ESI†). The principles also apply to reactions involving pairs of electrons: in water, 2-electron reactions involving singlet oxygen (1O2) are faster than 4-electron reactions.49
4.3.2. Connecting mechanisms to thermodynamics.
Reactivity depends on the relative probability and location of the electron transferred during the reaction, i.e. the reaction coordinate. For example, while the proton is abstracted from the σ-orbital, the σ-electron might delocalize into a sp2-π system. In this case, the delocalization might be interpreted as an electronic coupling67 or a third (intermediate) vibrational state.18 Only before or after the delocalization, rearrangement of solvent/intermediates can occur (Franck–Condon approximation).
Theoretically, ΔG‡TS is composed of entropic (ΔS‡TS) and enthalpic (ΔH‡TS) terms. We found offsets of Δ(ΔGr) ≈ 100 kJ mol−1 (Δ(ΔG‡TS) ≈ 13 kJ mol−1) between LFERs (Fig. S8E, S11A and S12A, ESI,† involving HA by HO˙, H˙ and CH3˙, resp.) for non-polar (μπ 0–1 D) and zwitterionic compounds (μπ = 10–15 D). The difference between non-polar (μπ = 0–1 D) and polar (μπ = 5 ± 2 D) compounds is Δ(ΔGr) ≈ 30 kJ mol−1 (Δ(ΔGTS) ≈ 4 kJ mol−1, involving HA by HO˙). Solvent (H2O) rearrangement enthalpies depend on the polarity of the molecule and its effective solvation, but values in the same range have been reported68–70 (note also that 1 hydrogen bond in H2O is ∼18 kJ mol−1).
For similar mechanisms such as sequential HAT, ΔS‡TS may be a constant factor, in which case ΔG‡TS is only determined by ΔH‡TS.71 Thus, μπ is likely to characterize ΔH‡TS. We infer that σ and π could characterize ΔS‡TS, e.g. by distinguishing between concerted and stepwise mechanisms. Analogously, we might discern between outer- and inner-sphere ET via Marcus Theory72 (S2, ESI†). We may further investigate the components of ΔG‡TS by looking at ‘exotic’ radicals with lower Δ(ΔGr) (Fig. 1) e.g. ‘nucleophillic’ tert-butyl or HS˙. This may help extending eqn (8) to addition reactions.73,74
4.4. Outlook
Empirical prediction methods often use many parameters and ambiguous algorithms, which does not facilitate interpretation and can be error-prone. Therefore, one should not accept beforehand that complex methods are needed. Alternatively, TS and frontier molecular orbital theory are closer to chemical intuition than a purely mathematical fitting process. Indicators, or proxies, for the free energy of activation ΔG‡ are more easily retrievable than e.g. with ‘ab initio’ methods. The approach presented here reflects textbook knowledge of basic chemistry, implying that the parameters used have a physical basis. Despite the uncertainties involved, mathematical fitting yields surprisingly accurate results and may easily compete with machine learning methods with little computational effort. For the examples and controls shown here, it might even compete with computationally expensive quantum chemical (‘ab initio’) methods. The method presented here can effectively screen a large number of reactions, for multiple radicals.
5. Summary
In this study we used a thermodynamic/quantum-mechanical framework and kinetic data to parametrize the Eyring equation to predict the rate constants of one-electron oxidation reactions. Our method assumes a linear-free-energy relationship between activation and reaction energies. Via a thermodynamic cycle we combined frontier orbital interaction, |ESOMO − EHOMO| and charge (proton) transfer to adequately describe hydrogen abstraction reactions. On the basis of Hammett substituent constants, we inferred that all one-electron oxidation reactions entail similar rate limiting steps. Thus, offsets between different reaction pathways were approximately constant, which we attribute to specific transition state effects. These effects can be described by local polarity (dipole moment, μ) and atom type orbital delocalizability indices. Our method, parametrized to OH˙, successfully predicted rate constants for H˙ and CH3˙ and it is within expectation that rate constants for OOH˙ and O2˙− can also be evaluated. Predicted and experimental values for kr show appreciable correlations.
Conflicts of interest
The authors declare that they have no conflicts of interest.
Acknowledgements
Funding by the Swiss National Science Foundation (SNSF) is gratefully acknowledged (grant no. 175493). This work is part of the research programme TTW financing the Contaminants of Emerging Concern in the Water Cycle (CERCEC) project number 15759, which is financed by the Dutch Research Council (NWO). Discussions with Minju Lee (Glasgow University) and Jingwen Chen (Dalian University) were greatly appreciated.
References
-
F. D. Coms, The Chemistry of Fuel Cell Membrane Chemical Degradation, Proton Exchange Membrane Fuel Cells 8, Pts 1 and 2, 2008, vol. 16, issue 2, pp. 235–255.
- T. M. Nolte and A. M. J. Ragas, A review of quantitative structure-property relationships for the fate of ionizable organic chemicals in water matrices and identification of knowledge gaps, Environ. Sci.: Processes Impacts, 2017, 19(3), 221–246 RSC.
- B. Lipinski, Hydroxyl Radical and Its Scavengers in Health and Disease, Oxid. Med. Cell. Longevity, 2011, 809696 Search PubMed.
-
T. G. Truscott, Pulse radiolysis and flash photolysis, in Photobiology, ed. E. Riklis, Springer, Boston, MA, 1991 Search PubMed.
- A. J. Hendriks, How To Deal with 100,000 + Substances, Sites, and Species: Overarching Principles in Environmental Risk Assessment, Environ. Sci. Technol., 2013, 47(8), 3546–3547 CrossRef CAS.
- ECHA, ECHA's REACH 2018 Roadmap, Helsinki, Finland, 2015.
-
C. J. van Leeuwen and J. L. M. Hermens, Properties of Chemicals and Estimation Methodologies, Risk Assessment of Chemicals: An Introduction, Springer, 1995, pp. 261–262 Search PubMed.
- ECHA, Practical guide How to use and report (Q)SARs, 2016.
- Development, O.f.E.C.-o.a., Guidance document on the validation of (quantitative) structure–activity relationship (QSAR) models. 2017.
- D. Gadaleta,
et al., Applicability Domain for QSAR Models: Where Theory Meets Reality, Int. J. Quant. Struct.-Prop. Relat., 2016, 1(1), 45–63 Search PubMed.
- P. Carrio,
et al., Applicability Domain Analysis (ADAN): A Robust Method for Assessing the Reliability of Drug Property Predictions, J. Chem. Inf. Model., 2014, 54(5), 1500–1511 CrossRef CAS.
- F. Sahigara,
et al., Comparison of Different Approaches to Define the Applicability Domain of QSAR Models, Molecules, 2012, 17(5), 4791–4810 CrossRef CAS.
- H. Eyring, The Activated Complex in Chemical Reactions, J. Chem. Phys., 1935, 3(2), 107–115 CrossRef CAS.
-
K. Matzjasyewski and T. P. Davis, Theory of radical reactions, in Handbook of radcial polymerization, Wiley-Interscience, Hoboken, 2002 Search PubMed.
- T. Bucko, Ab initio calculations of free-energy reaction barriers, J. Phys.: Condens. Matter, 2008, 20(6), 064211 CrossRef CAS.
- L. P. Hammett, The effect of structure upon the reactions of organic compounds benzene derivatives, J. Am. Chem. Soc., 1937, 59, 96–103 CrossRef CAS.
- C. Hansch, A. Leo and R. W. Taft, A Survey of Hammett Substituent Constants and Resonance and Field Parameters, Chem. Rev., 1991, 91(2), 165–195 CrossRef CAS.
-
S. Braun-Sand, M. H. M. Olsson and A. Warshell, Proton transport in carbonic anhydrase as an example of the difference between microscopic and phenomenological LFERs, in Computer modelling of enzyme catalysis and its relationship to concepts in physical organic chemistry, in Advances in Physical Organic Chemistry, ed. J. P. Richard, Elsevier, Amsterdam, 2005, pp. 212–217 Search PubMed.
- E. Rorije,
et al., Modeling reductive dehalogenation with quantum chemically derived descriptors, SAR QSAR Environ. Res., 1995, 4(4), 237–252 CrossRef CAS.
- L. H. Jing, J. J. Nash and H. I. Kenttamaa, Correlation of hydrogen-atom abstraction reaction efficiencies for aryl radicals with their vertical electron affinities and the vertical ionization energies of the hydrogen-atom donors, J. Am. Chem. Soc., 2008, 130(52), 17697–17709 CrossRef CAS.
- T. M. Nolte and W. J. G. M. Peijnenburg, Use of quantum-chemical descriptors to analyse reaction rate constants between organic chemicals and superoxide/hydroperoxyl (O2•−/HO2•), Free Radical Res., 2018, 52(10), 1118–1131 CrossRef CAS.
- E. Rosta and A. Warshel, On the Origins of the Linear Free Energy Relationships: Exploring the Nature of the Off-Diagonal Coupling Elements in S(N)2 Reactions, J. Chem. Theory Comput., 2012, 8(10), 3574–3585 CrossRef CAS.
- R. W. Taft,
et al., Linear Solvation Energy Relations, J. Solution Chem., 1985, 14(3), 153–186 CrossRef CAS.
- S. S. Shaik, The Lego Way - Curve Crossing Diagrams as General-Models in Physical Organic-Chemistry, Pure Appl. Chem., 1991, 63(2), 195–204 CAS.
- G. Klopman, Chemical-reactivity and the concept of charge-controlled and frontier-controlled reactions, Curr. Cont. Eng. Technol. Appl. Sci., 1984,(20), 16 Search PubMed.
-
K. Fukui and H. Fujimoto, Frontier orbitals and reaction paths, World Scientific Publishing, Singapore, 1997 Search PubMed.
- R. G. Pearson, Hard and Soft Acids and Bases, J. Am. Chem. Soc., 1963, 85(22), 3533–3539 CrossRef CAS.
-
T. L. Ho, Hard and Soft Acids and Bases in Organic Chemistry, Academic Press, New York, 1977 Search PubMed.
- W. B. Jensen, Lewis Acid-Base Definitions - Status-Report, Chem. Rev., 1978, 78(1), 1–22 CrossRef CAS.
-
M. Jonsson, Redox Chemistry and Energetics of Radical Cations od Substituted Benzenes, in Radication Chemistry Present Status and Future Trends, ed. B. S. M. Rao and C. D. Jonah, Elsevier Science B.V., 2001 Search PubMed.
- A. M. D. Nicholas and D. R. Arnold, Thermochemical Parameters for Organic Radicals and Radical Ions 1. The Estimation of the pKa of Radical Cations Based on Thermochemical Calculations, Can. J. Chem., 1982, 60(17), 2165–2179 CrossRef CAS.
- T. Nauser,
et al., Why Selenocysteine Replaces Cysteine in Thioredoxin Reductase: A Radical Hypothesis, Biochemistry, 2014, 53(30), 5017–5022 CrossRef CAS.
- K. Fukui, H. Kato and T. Yonezawa, A New Quantum-Mechanical Reactivity Index for Saturated Compounds, Bull. Chem. Soc. Jpn., 1961, 34(8), 1111–1115 CrossRef CAS.
- P. F. Barbara, T. J. Meyer and M. A. Ratner, Contemporary issues in electron transfer research, J. Phys. Chem., 1996, 100(31), 13148–13168 CrossRef CAS.
- ChemAxon, Calculator Plugin for structure property prediction. MarVin Version 5.2.0.
- P. Gramatica and E. Papa, Screening and ranking of POPs for global half-life: QSAR approaches for prioritization based on molecular structure, Environ. Sci. Technol., 2007, 41(8), 2833–2839 CrossRef CAS.
- X. Luo,
et al., Development of a QSAR model for predicting aqueous reaction rate constants of organic chemicals with hydroxyl radicals, Environ. Sci.: Processes Impacts, 2017, 19(3), 350–356 RSC.
-
P. W. N. M. van Leeuwen, Section 1.5.3 - Linear Free Energy Relationships, in Homogeneous Catalysis: Understanding the Art, 2004 Search PubMed.
- S. Endo and K. Goss, Applications of Polyparameter Linear Free Energy Relationships in Environmental Chemistry, Environ. Sci. Technol., 2014, 48(21), 12477–12491 CrossRef CAS.
- M. T. Baumgartner,
et al., Theoretical study of the relative reactivity of chloroethenes with atmospheric oxidants (OH, NO3, O(P-3), Cl(P-2) and Br(P-2)), Phys. Chem. Chem. Phys., 2002, 4(6), 1028–1032 RSC.
- J. Tauber, D. Imbri and T. Opatz, Radical Addition to Iminium Ions and Cationic Heterocycles, Molecules, 2014, 19(10), 16190–16222 CrossRef.
- N. R. Greiner, Hydroxyl Radical Kinetics by Kinetic Spectroscopy 6. Reactions with Alkanes in Range 300–500 Degrees K, J. Chem. Phys., 1970, 53(3), 1070–1076 CrossRef CAS.
- E. S. C. Kwok and R. Atkinson, Estimation of Hydroxyl Radical Reaction-Rate Constants for Gas-Phase Organic-Compounds Using a Structure-Reactivity Relationship – an Update, Atmos. Environ., 1995, 29(14), 1685–1695 CrossRef CAS.
- J. Markelj and M. Pompe, Modeling of atmospheric OH reaction rates using newly developed variable distance weighted zero order connectivity index, Atmos. Environ., 2016, 131, 418–423 CrossRef CAS.
- G. V. Buxton, C. L. Greenstock, W. P. Helman and A. B. Ross, Critical Review of Rate Constants for Reactions of Hydrated Electrons, Hydrogen Atoms and Hydroxyl Radicals (.OH/.O-) in Aqueous Solution., J. Phys. Chem. Ref. Data, 1988, 17, 513 CrossRef CAS.
-
J. Kostal, Computational Chemistry in Predictive Toxicology: status quo et quo vadis?, Advances in Molecular Toxicology, 2017, vol. 10, pp. 139–186 Search PubMed.
- J. K. Thomas, Pulse Radiolysis of Aqueous Solutions of Methyl Iodide and Methyl Bromide. Reactions of Iodine Atoms and Methyl Radicals in Water, J. Phys. Chem., 1967, 71(6), 1919–1925 CrossRef CAS.
- Y. N. Wang,
et al., Estimation of Aqueous-Phase Reaction Rate Constants of Hydroxyl Radical with Phenols, Alkanes and Alcohols, QSAR Comb. Sci., 2009, 28(11–12), 1309–1316 CrossRef CAS.
- T. M. Nolte and W. J. G. M. Peijnenburg, Aqueous-phase photooxygenation of enes, amines, sulfides and polycyclic aromatics by singlet (a1Δg) oxygen: prediction of rate constants using orbital energies, substituent factors and quantitative structure–property relationships, Environ. Chem., 2018, 14(7), 442–450 CrossRef.
- X. Xu and X. G. Li, QSAR for Predicting Biodegradation Rates of Polycyclic Aromatic Hydrocarbons in Aqueous Systems, Chin. J. Struct. Chem., 2012, 31(8), 1212–1221 CAS.
- J. Gasteiger,
et al., Finding the 3D structure of a molecule in its IR spectrum, Fresenius' J. Anal. Chem., 1997, 359(1), 50–55 CrossRef CAS.
- J. H. Schuur, P. Selzer and J. Gasteiger, The coding of the three-dimensional structure of molecules by molecular transforms and its application to structure-spectra correlations and studies of biological activity, J. Chem. Inf. Comput. Sci., 1996, 36(2), 334–344 CrossRef CAS.
- P. M. Morse, Diatomic molecules according to the wave mechanics. II. Vibrational levels, Phys. Rev., 1929, 34(1), 57–64 CrossRef CAS.
- F. De Vleeschouwer,
et al., Electrophilicity and nucleophilicity index for radicals, Org. Lett., 2007, 9(14), 2721–2724 CrossRef CAS.
- E. N. Sharp, P. Rupper and T. A. Miller, The structure and spectra of organic peroxy radicals, Phys. Chem. Chem. Phys., 2008, 10(27), 3955–3981 RSC.
- N. Santschi and T. Nauser, An Experimental Radical Electrophilicity Index, ChemPhysChem, 2017, 18(21), 2973–2976 CrossRef CAS.
-
A. Bottoni, J. Fossey and D. Lefort, On the Role of the Orbital Interaction Concept in the Interpretation of Organic Free Radical Structures and Reactivities, in Molecules in Physics, Chemistry and Biology, ed. J. Maruani, Kluwer Academic Publishers, Dordrecht, 1989, pp. 173–219 Search PubMed.
-
A. Borgoo, M. R. Godefroid and P. Geerlings, Atomic Density Functions: Atomic Physics Calculations Analyzed with Methods from Quantum Chemistry, Advances in the Theory of Quantum Systems in Chemistry and Physics, 2012, vol. 22, pp. 139–171 Search PubMed.
- R. M. V. Abreu, I. C. F. R. Ferreira and M. J. R. P. Queiroz, QSAR model for predicting radical scavenging activity of di(hetero)arylamines derivatives of benzo[b]thiophenes, Eur. J. Med. Chem., 2009, 44(5), 1952–1958 CrossRef CAS.
- J. M. Mayer and I. J. Rhile, Thermodynamics and kinetics of proton-coupled electron transfer: stepwise vs. concerted pathways, Biochim. Biophys. Acta, Bioenerg., 2004, 1655(1–3), 51–58 CrossRef CAS.
- F. G. Bordwell,
et al., Bond-Dissociation Energies in Dmso Related to the Gas-Phase, J. Am. Chem. Soc., 1991, 113(26), 9790–9795 CrossRef CAS.
- F. P. Tully,
et al., Kinetics of the Reactions of Hydroxyl Radical with Benzene and Toluene, J. Phys. Chem., 1981, 85(15), 2262–2269 CrossRef CAS.
- M. Pompe,
et al., Using variable and fixed topological indices for the prediction of reaction rate constants of volatile unsaturated hydrocarbons with OH radicals, Molecules, 2004, 9(12), 1160–1176 CrossRef.
-
D. R. Lide, Handbook of Chemistry and Physics, CRC Press, Boca Raton, FL, 80th edn, 1999 Search PubMed.
-
N. J. Turro, Theoretical organic photochemistry, section 7.5, Modern Molecular Photochemistry, University Science Books, US, 1991, ch. 7, p. 207 Search PubMed.
- L. Munoz-Rugeles and J. R. Alvarez-Idaboy, A proton-electron sequential transfer mechanism: theoretical evidence about its biological relevance, Phys. Chem. Chem. Phys., 2015, 17(43), 28525–28528 RSC.
- L. Blancafort and A. A. Voityuk, Exciton delocalization, charge transfer, and electronic coupling for singlet excitation energy transfer between stacked nucleobases in DNA: An MS-CASPT2 study, J. Chem. Phys., 2014, 140(9), 095102 CrossRef.
- D. Sakic, H. Zipse and V. Vrcek, Base-catalyzed reactions of environmentally relevant N-chloro-piperidines. A quantum-chemical study, Org. Biomol. Chem., 2011, 9(11), 4336–4346 RSC.
- J. J. C. Teixeira-Dias,
et al., Transition states for H atom transfer reactions in the CH2CH2OH radical: The effect of a water molecule, Phys. Chem. Chem. Phys., 2003, 5(22), 5063–5069 RSC.
- V. Vaquero,
et al., Alanine Water Complexes, J. Phys. Chem. A, 2014, 118(14), 2584–2590 CrossRef CAS.
- I. H. Um, J. E. Park and Y. H. Shin, Combined dual substituent constant and activation parameter analysis assigns a concerted mechanism to alkaline ethanolysis at phosphorus of Y-substituted phenyl diphenylphosphinates, Org. Biomol. Chem., 2007, 5(21), 3539–3543 RSC.
-
R. G. Endres, Inner-sphere reorganization energies of outer-sphere self-exchange reactions utilizing density functional theory, in Trends in Chemical Physics Research, ed. A. N. Linke, Nova Science Publishers, Inc., New York, 2006, ch. 7, p. 140 Search PubMed.
- C. J. Chen and J. W. Bozzelli, Analysis of tertiary butyl radical plus O-2, isobutene plus HO2, isobutene plus OH, and isobutene-OH
adducts plus O-2: A detailed tertiary butyl oxidation mechanism, J. Phys. Chem. A, 1999, 103(48), 9731–9769 CrossRef CAS.
- N. J. Khalil, Factors Controlling the Addition of Tert-Butyl Radical to 1,2-disubstituted and 1,1,2-trisubstituted Alkenes, Tikrit J. Pure Sci., 2011, 16(2), 75–79 Search PubMed.
Footnote |
† Electronic supplementary information (ESI) available. See DOI: 10.1039/d0cp03750h |
|
This journal is © the Owner Societies 2020 |
Click here to see how this site uses Cookies. View our privacy policy here.