DOI:
10.1039/D0AN01423K
(Critical Review)
Analyst, 2021,
146, 33-47
Advances in fingermark age determination techniques
Received
17th July 2020
, Accepted 5th October 2020
First published on 6th October 2020
Abstract
Fingermarks have long been recognized as one of the most reliable and valuable evidence for personal identification. In practice, fingerprint analysis primarily concentrates on latent fingerprint visualization. However, fingerprint visualization techniques do not always enable individualization when fingermarks collected in crime scenes are fragmentary, ambiguous, or deformed. Age determination techniques based on physical and chemical composition changes in fingerprints over time have attracted researchers’ attention in recent years. Nevertheless, the components of fingerprints are liable to factors including donor features, deposition conditions, substrate properties, environmental conditions and revealing methods. All the influences mainly contribute to unreliable outcomes of age estimation. Recent developments in fingermark age determination have moved forward to more precise approaches. The advanced methods can be classified into two categories including techniques based on the modifications of physical characteristics and chemical composition characteristics. Herein, the review includes the five types of variables that influence the aging process. The methodologies are subsequently highlighted along with their advantages and disadvantages. Furthermore, photography, optical, microscopy and electrochemical methods, and vibrational spectroscopy and mass spectrometry (MS) techniques are summarized in detail, with an emphasis on their utilization.
1. Introduction
Fingermarks reflect the physical characteristics (ridge width, ridge patterns, minutiae, etc.), chemical compositions (water, inorganic salts, amino acids, fatty acids, urea, squalene, etc.), and even the biological characteristics of human fingerprints (age, gender, health conditions, diet, DNA information, etc.) of donors. They have long been considered to be one of the most valuable and solid evidence for forensic investigation and law enforcement.1,2 Therefore, it is essential to accurately establish recording and analysis methods of fingerprints.3
Generally, fingermarks in a crime scene are invisible to the naked eye and are called latent fingermarks (LFMs).4 In addition, the ridge pattern of a fingerprint is unique and remains unchanged throughout one's lifetime.5 Thus in practice, fingerprint analysis mainly focuses on the visualization of ridge patterns and details for acquiring donor profiles. Up to now, optical, powder dusting, cyanoacrylate fuming, iodine fuming and vacuum metal deposition methods have been routinely employed in LFM enhancement, which usually do harm to the human body.6,7 In recent years, considerable efforts have been devoted to exploring simpler, safer and cheaper treatments. A variety of novel techniques such as nanomaterial reagents, quantum dots reagents and antibody recognition reagents are nowadays available.7–10 Nonetheless, it is not always desirable to utilize these techniques to process LFMs, especially for low-quality patterns with fragmentary, ambiguous and deformed features. In that case, ridge details could not provide sufficient information for personal identification.
According to Locard's exchange principle, whenever a finger touches the substrate, the substances originating from the epidermis are transferred onto the substrate.11 Hence, fingerprint residues are composed of natural secretions from eccrine and sebaceous glands and contaminants in the environment.12 Remarkably, fingerprint residues are variable and likely to change over time, resulting in the physical and chemical changes of fingerprints. Accordingly, they are the key to investigate the physical and chemical changes over time for assessing the fingermark aging process. Generally, determining the fingermark age has superiority in estimating crime timelines. It can be further used for identifying suspects, assessing statements from witnesses, victims, and also screening crime-related evidence.13 Therefore, it is significant for the forensic community to strengthen the value of evidence.
Over the last 40 years, various treatments have been proposed for establishing fingermarks’ deposition timelines. However, fingerprint residues vary among individuals and are highly unstable and vulnerable to complex variables such as donor features, deposition conditions, substrate properties, environmental conditions and development methods.14,15 To date, it is still a difficult issue in the field of fingerprint analysis to accurately determine the age of fingermarks.16
Recent developments on age assessment have led to a better understanding of how to determine the age of fingermarks more precisely. Similarities among these developments suggest researchers focus on visual changes in the physical characteristics and degradation of the chemical composition of fingerprints.17
In this review, the current research interests are divided into two categories: (i) fingermark age determination techniques based on physical characteristics and (ii) fingermark age determination techniques based on chemical composition characteristics. We have addressed the issues associated with the interfering factors in the fingermark deposition and aging stages, and then summarized the recent progress in fingermark age determination over the past few years. Finally, the perspectives in the field of fingermark age estimation are also outlined.
2. Variables in fingermark age determination
The compositions of fingerprint residues are the material base to fingermark age determination. Ordinarily, fingerprint residues are composed of endogenous or semi-exogenous constituents of the skin and exogenous substances in the environment.18,19 The endogenous constituents mainly consist of sweat secretions and sebum secretions such as water (98%–99%), inorganic salts (e.g. chloridion, potassium and phosphate) and organic constituents (e.g. proteins, amino acids, urea, polypeptides, sugars, fatty acids, wax esters, squalene and sterol esters).18–20 On the other hand, exogenous substances contain a wide range of contaminants or contact components, such as blood, food, make-up, gunshot residues, explosives and drug poisons.18,19,21 Semi-exogenous substances are metabolites produced by exogenous molecules and excreted by sweat pores (e.g. metabolites of nicotine and drugs).
As shown in Fig. 1, there are a number of variables that affect fingerprint compositions in two stages namely the ‘deposition stage’ and the ‘aging stage’. The variables in the deposition stage such as donor features, deposition conditions and substrate properties lead to differences in the initial composition.15,18 Subsequently, the variables of environmental conditions (e.g. air circulation, dust, humidity, light exposure, precipitation, temperature, and ultraviolet and other radiation conditions), enhancement methods (e.g. physical, physico-chemical, and chemical methods) and substrate properties in the aging stage cause the aged composition differences.15,18 De Alcaraz-Fossoul et al. explored the 6-month degradation patterns of fingermarks when exposed to certain factors involving temperature, relative humidity, fingerprint compositions (eccrine and sebaceous), daylight (direct, penumbra, and darkness), and substrate types (glass and polystyrene).22 It was indicated that the factors combined differently resulted in different outcomes.22 For example, when remaining for longer periods, sebaceous fingermarks on the glass are identifiable compared to eccrine fingermarks on plastic.22 In other words, the factors have influences on the degradation of fingermarks and thus bring errors in fingermark dating.23
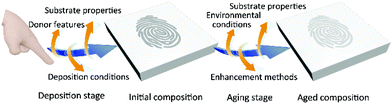 |
| Fig. 1 The variables that affect fingerprint compositions before and after deposition. | |
2.1 The fingerprint donor features
The donor characteristics include age, gender, races, physical conditions, diet, etc. The research results indicate that the degradation speed of children is faster than that of adults.24 It is also demonstrated that the chloride in fingerprint residues decreases with the fingermark aging.24 Furthermore, the fingerprint composition can differ significantly between different genders.25,26 For example, the fatty acid of male prints is higher than that of female prints whereas the amino acid of male prints is lower than that of female prints. Moreover, the diet may affect the components of fingerprints. It seems that alanine, glycine, serine, saturated C17, both saturated and unsaturated C18 fatty acids will increase.25
2.2 The deposition conditions
The deposition conditions include deposition pressure, contact time, the angle of the finger in contact with the substrate and whether the donors washed their hands or not. In fact, it is indicated that the more pressure applied, the greater number of residues were transferred onto the substrate.27
2.3 The substrate types
The substrate types are usually classified into three categories: non-porous, semi-porous and porous. Actually, the high porosity of the surface contributes to the adhesion between fingermarks and substrates. The specific absorption features of fingermarks in these three kinds of substrates are illustrated in Table 1.18,28,29
Table 1 The absorption characteristics of fingerprint residues on three types of substrates18,28,29
Substrate types |
Samples |
Eccrine |
Sebaceous |
Non-porous |
Glass, metal, plastic, etc. |
Stayed on the surface until aging occurs |
Semi-porous |
Varnished wood, glossy paper, etc. |
Absorbed slower than porous |
Absorbed slower than eccrine on semi porous |
Porous |
Paper, wood, etc. |
Rapidly absorbed |
Slowly absorbed |
2.4 The environmental conditions
Light exposure, temperature and humidity are the most common environmental conditions. When stored in the dark, fatty acids and squalene decay less rapidly than under light conditions.30 C18 fatty acid increases first and then decreases in the dark, while the changes cannot be detected under light conditions.14 The degradation speed of fingerprint residues is highly related to temperature. The higher the temperature is, the more rapid the aging process will be. Furthermore, there is no study to observe the influence of humidity on fingerprint compositions.
2.5 The enhancement techniques
We investigated whether utilizing the development approach will influence the fingerprint compositions and the morphological features of fingermarks in the aging stage. One research has explored the impact of three visualization approaches on squalene, cholesterol and myristyl myristate (Table 2).31
Table 2 The influence of the enhancement techniques on three lipids31
Visualization reagents |
Influence |
Aluminum powder |
Contaminate the samples, but not affect the analysis |
Indanedione |
Extract the lipids and thus influence the recovery rate of compounds |
Cyanoacrylate fuming |
Not significantly influence the recovery rate of the targeted lipids and no contaminants |
With this regard, as many variables as possible should be carefully taken into account and integrated into age determination models. Suppose a specific scenario in which not all the influential factors of a fingermark left on the crime scene would be known. More concretely, the substrate nature, enhancement methods, and recent environmental conditions would be the known factors while the deposition conditions would not be known. If the fingerprint donor is identified, the donor features will be also known. Therefore, (i) the impact of as many factors as possible should be considered by adopting statistical methods. For example, we can alter one or more conditions and obtain the data of aging parameters. Whether the factors are significant to the results can be judged by variance analysis. The insignificant factors can be excluded in the following study; (ii) the factors which dramatically affect the aging process can be integrated into different regression models such as multiple linear regression model or logarithm regression model; (iii) for known variables, their values are determined. After substituting the values into a formula, the specific age can be obtained; for unknown factors, only a candidate range of values can be used in the model. As a result, the predicated age falls within a range.
3. Fingermark age determination techniques based on physical characteristics
3.1 The application of morphological characteristics to fingermark age analysis
When fingermarks are deposited for a longer time, water is significantly reduced along with other components, accompanied by the morphological modifications of ridges. Morphometry can be a potential measurement to recognize the aging patterns of fingermarks.32 An early research group has used the powder dusting method to estimate the deposition timeline based on how well the powder adheres to the ridges, as well as the overall clarity of the visualized ridge details.33 However, the lack of quantification and ignoration of the possible variables, such as the initial composition differences between donors, environmental conditions and stickiness of fingermarks, in the experiment produced unreliable results.
The morphological characteristics have been usually monitored by photography, microscopy, or scanning techniques, and then quantified by image statistical processing, through which both morphological images and aging information of fingermarks can be captured simultaneously.34 In general, the morphological characteristics primarily include the two dimensional (2D) features such as ridge widths, ridge continuities, minutiae counts and sweat pore sizes of fingerprints and three dimensional (3D) characteristics (e.g. ridge heights). Specifically, visual changes take place over time, involving the narrowed width of ridges, the increased size of sweat pores, the decreased continuity, height and minutiae counts in ridges, as well as the loss of color contrast between ridges and furrows.35,36 All the changes can be chosen as parameters and applied to build aging models for dating fingermarks. It should be noted that only focusing on 2D morphological variations may lead to information (e.g. ridge thickness) omissions on the account that fingermarks are 3D impressions.
Herein, we mainly review the advances in fingermark age determination through various instruments, including using 2D and 3D morphological characteristics as aging markers.
General photography technique.
The visual parameters can be classified into subject-independent parameters (SIPs) and subject-non independent parameters (SNIPs). If the donor has not been known, any additional information will not be available except the conditions of substrate type, nature of sweat, and degree of light exposure. Hence, investigators could rely on the SIPs (e.g. color contrast and the number of ridge discontinuities).37,38 In another case, when the fingerprints were matched to a certain donor, SNIPs (e.g. ridge widths and the number of minutiae) could be utilized in that the initial conditions are known.39,40 De Alcaraz-Fossoul et al. conducted a systematic study of the degradation patterns of fingermarks over 6 months by using the two types of aging parameters.37–40 Before optical photographing and data processing, the fingerprint samples were pretreated with titanium dioxide powder (TiO2). In addition, they explored which and how variables would impact the fingermark degradation by using various statistical tests. It is suggested that the degradation was highly dependent on the interaction with environmental factors. To reduce the influence of TiO2 powder on ridges, every sample was standardized with the “reference” ink impression. Finally, the regression models of degradation patterns were established based on the variations in the four parameters over time, respectively. The data processing method provides a clear idea and detailed information (Table 3).
Table 3 Summary of data analysis that De Alcaraz-Fossoul's group adopted in the dating process37–40
Process of data analysis |
Ref. 37
|
Ref. 38
|
Ref. 39
|
Ref. 40
|
Aging parameters |
Minutiae ratio (the minutiae number of samples to the minutiae number of the inked impressions) |
Color contrast (MI: the average color intensity from all the array of colors in a given image, IA: the spread of color intensities) |
Discontinuity index (four ranks correspond to four ranges of the discontinuity number) |
Ridge width ratio (the ridge width of samples to the ridge width of the inked impressions) |
Reproducibility of the deposition method |
Standard deviation test |
Standard deviation test |
Standard deviation test |
Kruskal–Wallis test |
Efficiency of the powder-developing method |
Wilcoxon rank-sum test and the Bonferroni correction |
Not mentioned |
Not mentioned |
Not mentioned |
Differences in parameters per investigator |
Wilcoxon signed-rank test or bootstrapping |
Standard deviation test |
U-Mann–Whitney test |
Kruskal–Wallis test |
Analysis of single factor and combination factors |
Standard deviation test |
Standard deviation test |
U-Mann–Whitney test and Kruskal–Wallis test |
If Kolmogorov–Smirnov test and Levene's test passed, apply t-test or one-way ANOVA. If not, apply Mann–Whitney U-test and Kruskal–Wallis test |
Samples of regression models (plastic/greasy/light) |
Only visual degradation patterns (five types) |
MI: 120.02–0.304 day (linear, R2 = 0.361) |
20.942 + 0.285 day (R2 = 0.384) |
0.928–0.003 day (R2 = 0.388) |
IA: 42.32–11.95 log(d) (logarithmic, R2 = 0.569) |
Unfortunately, three limitations still exist in De Alcaraz-Fossoul's method: (i) for the visualization method: fingermarks were enhanced by treating with titanium dioxide powder (TiO2). In the development process, the brush could directly touch the ridges, which would damage the ridge details. Meanwhile, there was no guarantee that the position and quantity of powders distributed on the ridges were the same during the development process each time; (ii) for sample collection: the limited number of donors and fingerprint samples were not representative enough and thus could not be generalized to other conditions; and (iii) for data processing: the four parameters were used to constitute the regression models separately, which lead to a relatively low R2 value. Additionally, the parameter of ridge discontinuity was a semi-quantitative indicator. To address these limitations, three solutions should be adopted: (i) it is still urgent to find a development technique which can stably reflect the four aging parameters. For example, cellulose membranes are an excellent alternative to transfer fingerprint residues onto various substrates. The membranes enable high-resolution optical microscopy to capture level 2 and 3 details of water-developed sebaceous fingermarks. Thus, the membrane-aqueous method has great potential in reliably extracting aging markers; (ii) it can speed up the application process of this technique to include a larger number of fingerprint samples; and (iii) the multiple regression model can accommodate four or more aging indicators simultaneously.
Chromatic white light technique.
Contactless sensors can offer more detailed information about the targeted trace. For example, the detection, classification and feature extraction of fingerprints can be automatically realized. Non-invasive high-resolution optical chromatic white light sensors (CWL) based on the chromatic aberration of light provide great potential for solving the challenges of LFM age determination.41 It acquires high-resolution 2D-intensity and 3D topographic images of fingermarks with a spatial resolution of up to 1 μm and a longitudinal resolution of 20 nm.41,42 When it comes to fingermark dating, it extracts ‘binary pixel’ to construct aging parameters from high-resolution images.42 Furthermore, the ‘binary pixel’ can be available in two ways. In the 2D intensity mode, the reflective behaviors of residues are different from those of surfaces, thus resulting in a difference in contrast between fingermarks and backgrounds.42 With this regard, the contrast expressed by binary pixels can be chosen as aging parameters. In the 3D topography mode, focal points of different wavelengths on substrates will be reflected which deliver the height data.42
Merkel's group comprehensively proposed the short-term and long-term aging estimation method utilizing the CWL technique.43 In 2012, they firstly reported that this technique could obtain the aging behavior of 17 different features. The ages of 41
520 fingermarks were divided into [0, 5 h] and [5, 24 h], reaching up to 79.29% accuracy.43 Later, they further explored the CWL technique and compared it with confocal laser scanning microscopy (CLSM).44 The results showed the accuracies of separating fresh marks and those that remained for at least half a day were 89.2% (eccrine fingermarks) and 98.7% (sebaceous fingermarks), respectively.44 Subsequently, they used 19 features to differentiate between the age of short-term fingermarks (24 h) and long-term fingermarks (0.5 years and 3 years).45 Since the image scanning does not offer certain compositions, it is expected that the CWL technique could be combined with other capturing devices (e.g. spectroscopes) to estimate the age of fingermarks more accurately.43,45
Optical profiling technique.
Highly sensitive optical profiling technique (OP) can provide all topographical data of fingerprints based on white light interferometry, even for valuable 3D topographical images. Hence, the morphological changes in ridges of untreated fingermarks can be quantitatively measured. Very recently, De Alcaraz-Fossoul's group has reported the OP technique for the examination of the aging process.46 Two donors (female and male) were asked to deposit fingermarks (e.g. index, middle, and ring fingers) on glass and plastic substrates, respectively.38 They measured the thickness of ridges as an aging marker and obtained the 3D images of fingermarks.46Fig. 2 displays the donor's (a Southeast Asian female named ‘M’) fingermark images and the degradation patterns of changes in the ridge height over time.46 The ridge height modifications (Sa values in micrometers) over one year were then observed. The experimental results indicated that the loss in the ridge height was apparent within the first 60 days after deposition.46 Compared with the traditional powder dusting method, the OP technique is more sensitive and offers high-quality data in all dimensions. Unfortunately, the application is limited due to the restricted analysis area, slow data acquisition time, insensitivity to thin fingermarks and interference from substrate roughness. Theoretically, if the toughness of surfaces is higher than the ridge height, the ridge signals will be covered. Moreover, image processing techniques can be used to address some of the disadvantages to some extent.
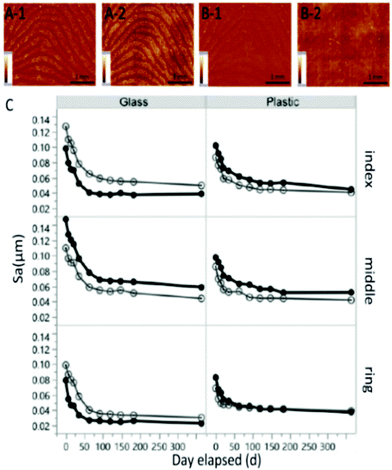 |
| Fig. 2 Images of fresh (A) and aged fingermarks (B) (that remained for one year) on glass (1) and plastic (2) and the average ridge height (C) of latent fingermarks of two replicates over time.46 | |
Atomic force microscopy technique.
Atomic force microscopy (AFM) has been widely applied to interfacial science and can be of value to highlight specific fingerprint ridge details.47 Ordinarily, the lowest resolution of fingermark imaging is 1 μm. Goddard et al. visualized fingermarks on polished brass substrates with an unprecedented resolution (<10 nm) of ridge details (in tapping or intermittent mode). The height information of ridges was then offered by line scanning across the ridges.48 Moreover, they noted that the roughness of substrates blocked the height measurement, although the roughness problem could be solved by polishing the brass surfaces.47–49 A recent report from Popov et al. have demonstrated the usefulness of AFM for fingermark age estimation.50 In their report, it was observed how the topographic variations at the micro/nano level varied with time after the deposition.50 Variations in the nature of the intermediate area including the coverage rate, limit and thickness are presented in Fig. 3A–C.50 The results demonstrated the expansion of the fingermarks over time occurred in an intermediate area around the main ridges.50 The intermediate area of ridges with a height of 4 nm expanded about 2 μm from the edge after 1 day. It spread to approximately 4 μm after 23 days (Fig. 3D).50 Meanwhile, the continuity was destroyed after 16 days. On day 61, the ridges were like a series of interconnected islands with a coverage rate of about 60% (Fig. 3D).50
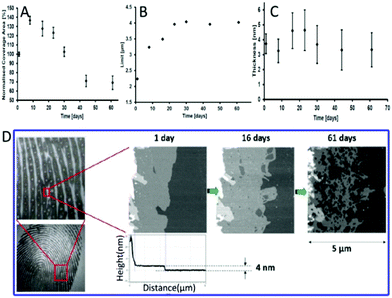 |
| Fig. 3 (A) The images of ridge expansions on polished silicon. The coverage area rate (B), limit (C) and thickness (D) of the intermediate area over time.50 | |
3.2 The application of electrical characteristics to fingermark age analysis
Electrical characteristics might undergo certain regularity, which are capable to be captured as alternatives to investigate the age of fingermarks. Conventional electrical methods such as electrostatic detection apparatus (ESDA) were previously proposed and deemed inconvenient because of the requirement of a large electric field.51 Here, three techniques are discussed as follows.
Electric potential sensor techniques.
An ultrahigh impedance electric potential sensor (EPS) is a non-contact raster scanning probe which can measure surface charge density with a spatial resolution of up to 5 μm.16 The sensor consists of a robust electronic amplifier. An ultra-high input resistance (1015 ohms) and input capacitance (10−16 farads) together contribute to non-invasive electric field measurements. An electrode combined with the sensor enable the measurement of electric field disturbances over a broad range (10–200 MHz). The surface potential and electrostatic charge on an insulator can be observed by integrating one or more sensor arrays. Prance et al. detected the electrical charge of fingermarks on insulated surfaces (e.g. PVC, PTFE, acetate and PVDF sheets).52 The electrostatic charge patterns of fingermarks over 14 days were subsequently visualized by an EPS. The EPS was applicable not only for the age estimation but also for the sequence detection of overlapped fingermarks.52 Notably, the spatial resolution of the surface potential image was over 500 dpi. However, this technique currently suffers from the limitation of long scanning time, small detection area, and the insulated substrate.52
Electrochemical impedance spectroscopy technique.
Generally, the physical modifications of fingerprints on conductive substrates will lead to an increase in the capacitance between fingermarks and substrates. Electrochemical impedance spectroscopy technique (EIS) has been firstly proposed to monitor the capacitance degradation over time by Rosa et al.34 Thus, the impedance spectra, along with the aging curve based on capacitance parameters were quantitatively obtained.34 In this work, a metal substrate was used as the working electrode, platinum foil as the counter electrode and Ag/AgCl as the reference electrode in an electrochemical cell. The evaporation/re-deposition of fingerprint residues and the roughness of the metal account for the change of ESI. Unfortunately, EIS was only suitable for conductive substrates and its applications were thus restricted. Further experiments need to be conducted considering various factors in the aging process.34
Peak force quantitative nano-mechanical mapping atomic force microscopy technique.
Peak force quantitative nano-mechanical mapping atomic force microscopy (PFQNM-AFM) is also a method for imaging the electrostatic properties of fingermarks. It has been proposed to observe the variations in surface adhesion and topography of fingermark droplets over time.53 The high-resolution topography images as well as adhesion, stiffness, elastic modulus and deformation of samples could be simultaneously captured.53Fig. 4 shows the surface adhesion force modifications in the middle of eccrine droplets over time. The adhesive forces of D2's fingermarks increased first and then dropped as time elapsed.
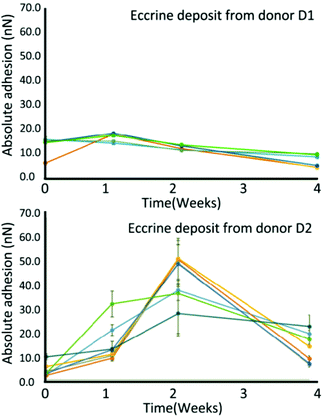 |
| Fig. 4 Variations in surface adhesion at the middle of eccrine droplets over time from donors D1 and D2. The error bars present the standard deviation of the mean adhesion value of individual droplets.53 | |
4. Fingermark age determination techniques based on chemical composition characteristics
Besides physical modifications, chemical composition characteristics are other ideal makers for fingermark age determination. The specific target compounds including endogenous, exogenous and semi-exogenous substances commonly undergo certain regularity and are often chosen to establish the aging patterns. Hence, to find suitable aging markers is of vital importance. The ratios of target components have been proved successful with regard to unknown initial components from unknown donors. The mixed factors in the aging process should also be considered to achieve more accurate outcomes. Below are several advanced methods that have been reported in recent years. Herein, we introduce those techniques by dividing them into two categories (spectroscopic and spectrometric methods) rather than from the aspect of fingerprint residue types (endogenous, exogenous and semi-exogenous substances) because most research studies have explored endogenous constituents as aging parameters.
4.1 Chromatography techniques for fingermark dating
Since the early 1980s, chromatography techniques including thin layer chromatography (TLC) and high performance liquid chromatography (HPLC) have been employed in fingermark dating.54,55 Although these research studies are systematic, there still exist huge limitations with many influential factors not being taken into account. Meanwhile, they are only used for qualitative testing. In recent years, a number of emerging methods combined with chromatography techniques have been introduced, accompanied by improving the limitations. Consequently, single chromatography techniques will not be included in this part. The other techniques (e.g. fluorescence spectroscopy and mass spectrometry) combined with chromatography techniques will be presented in sections 4.2 and 4.3.
4.2 Vibrational spectroscopy techniques for fingermark dating
Infrared spectroscopy technique.
Infrared spectroscopy (IR) is a rapidly developing technology that has been widely utilized in forensic chemistry.56 It is ideal for non-destructive and fast detection of fingerprint residues based on molecular absorptions with high sensitivity.21 Fourier transform infrared spectroscopy (FTIR) has been considered a rapid, non-destructive tool for analyzing specific chemical components. It can be used to detect the initial components in fingerprint residues and the aging variations.
Girod et al. investigated the initial components and the aging pattern of fingermarks by using Fourier transform infrared spectroscopy (FTIR).57 The attenuated total reflection mode (ATR) was chosen since it exhibits better-resolved spectra on both aluminum and glass surfaces than the reflection mode.57 This research is based on the framework that it is impossible to build an aging model suitable for all people. Hence, only one volunteer was asked to deposit samples with an approximate pressure of 500 ± 100 g for a contact time of 15 s.57 While exploring the aging behavior of the selected compounds over 34 days, this group took the substrate types (e.g. aluminum foils and glass slides) and preservation conditions (e.g. light and dark) into consideration. Principal component analysis (PCA), correlation calculations and partial least squares regression (PLSR) were adopted in the data processing.57 Satisfactory results indicated that the regions of 1000–1850 cm−1 and 2700–3600 cm−1 corresponded to the molecular vibrations of eccrine and sebaceous substances.57 The eccrine substances disappeared immediately while the sebaceous substances did not completely disappear over a period of up to 34 days.57 Furthermore, the older fingermarks (e.g. 24 and 34 days old) and fresh fingermarks (1, 3, 7 and 9 days old) could be precisely differentiated with an accuracy of ±3 days.57 Meanwhile, the accuracy rised to ±1 day after excluding the fingermarks aged for 20 days or 34 days.57 However, FTIR only works best on non-porous surfaces. Except the substrate nature and light conditions, the other variables should be included in future research.
In addition to endogenous residues, exogenous residues can also be potentially used for age dating. One study noted that the degradation of illicit contaminants in latent fingerprints could be chemically imaged by FTIR.58 Three exogenous substances including ammonium nitrate (AN), sodium chlorate (SC) and cocaine (C) were selected to contaminate fingermarks, respectively.58 The aging degradation by FTIR analysis and chemical map were then carried out in five-day intervals.58Table 4 displays the absorbance bands selected for FTIR analysis.58Fig. 5A provides the chemical map information of AN and C in which the ridge patterns can be recognized for individualization. Fig. 5B demonstrates the absorbance of AN, SC and C over a 30-day period.58 All the contaminants were detectable after 30 days. However, only AN suffered from a sharp reduction over the 30-day period, particularly in the first 10 days.58 The lower lattice enthalpy of AN than SC contributes to the degradation difference since AN is more likely to form ions in solutions.58 No change in the absorbance of cocaine has been detected due to its stable covalent bond. In addition to demonstrating the degradation of contaminated fingermarks, the uncontaminated fingermark aging model over a 30-day period was also described. The absorbance of the anti-symmetric and symmetric C–H stretching of CH2 groups (2920–2854 cm−1) was expected to dramatically decrease over 30 days.58 It is urgent to explore more kinds of illicit contaminants and to identify which factors would be of significance to degradation speed.
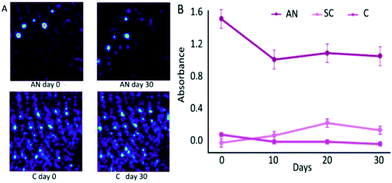 |
| Fig. 5 (A) Chemimap comparison of AN and C. (B) Changes in the specific peak absorbance over a 30-day period for AN, SC and C.58 | |
Table 4 Absorbance bands selected for analysis58
Target |
Band 1 (cm−1) |
Band 2 (cm−1) |
Band 3 (cm−1) |
Ammonium nitrate (AN) |
1628 |
1044 |
828 |
Sodium chlorate (SC) |
1887 |
1595 |
1415 |
Cocaine (C) |
2946 |
1735 |
1449 |
Fingerprint |
2925 |
2858 |
1738 |
Raman spectroscopy technique.
Raman spectroscopy (RS) technique is not only non-destructive for a wide range of materials (solids, liquids, and gases) but also does not need a pre-treatment process. It exhibits high selectivity and sensitivity so that samples with picogram quantities or femtoliter volumes can also be detected. It shows great performance in identifying exogenous substances that adhere to fingerprints such as explosives, gunshot residues, drugs, make-up, etc. Lately, many reports have primarily focused on the detection of components using the unique ‘chemical fingerprint’ without further exploring the component variations over time.59 In 2017, Andersson et al. established the RS technique by targeting the Raman band variation of carotenoids, squalene, unsaturated fatty acids and proteins.60 The obvious difference in the Raman spectra between fresh and one-month-old fingermarks can be observed. All the Raman bands in fresh and aged fingerprint residues are shown in Fig. 6A.60 In parallel, the light effects on Raman spectra were monitored by storing two duplicates in the dark (four weeks) and under ambient light conditions (three weeks), respectively.60 It was indicated that the light affected the degradation rates of carotenoids, squalene and unsaturated fatty acids, while proteins (e.g. aromatic side chains and amide I) remained more stable.60 Clearly, the Raman bands chosen as aging parameters showed remarkable decay regularity (Fig. 6B–D). Andersson et al. finally concluded that the degradation kinetics was in the order of carotenoids > squalene > unsaturated fatty acids > proteins.60
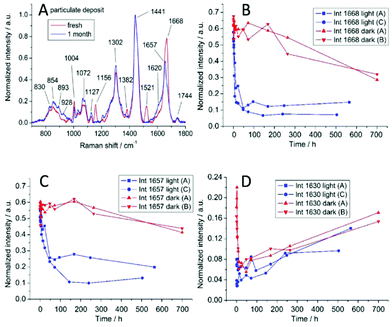 |
| Fig. 6 (A) Raman spectra from a freshly deposited fingermark and a one-month-old fingermark. The changes in certain Raman peaks: 1668 cm−1 (B), 1657 cm−1 (C), and 1630 cm−1 (D).60 | |
Fluorescence spectroscopy technique.
Fingerprint residues consist of proteins and lipids which emit auto-fluorescence. Notably, the tryptophan derivatives, including indoleacetic acid, beta-carbolines and xanthurenic acid are the main contributors to auto-fluorescence whose intensity decreases and color changes from green to yellow over time.61 Hence, the degree of red shift can be selected as an aging marker. Thin layer chromatography combined with fluorescence spectroscopy (TLC-FS) was used to identify the fluorescent aging products.61 Specifically, to obtain a stronger fluorescent signal, a certain number of overlapping fingermarks were deposited on an activated silica-coated TLC plate.61 Then, they were stored and aged for three weeks under natural or dark conditions.61 After running with chloroform/methanol (1
:
4), the fluorescence images, Rf-values and fluorescence spectra of separated spots were obtained and compared to those of the reference compounds.61 Finally, the plates were developed with Ehrlich's reagent which could react with tryptophan and indole-groups resulting in a specific color.61 Detailed information on the reference compounds is included in Table 5. The results demonstrated that tryptophan derivatives (e.g. b-3-indoleacetic acid, norharman, harman and xanthurenic acid) are important contributors to the autofluorescence of aged fingermarks.61 Light and bacterial breakdown will also affect the degradation of the fingermarks on TCL plates.61 Consequently, it gives new sights into monitoring tryptophan derivatives for establishing the aging model. However, it indicates that the fluorescent substances are used for preliminary investigation. Further identification needs to be performed by mass spectrometry or other techniques. A single fingermark rather than stacked fingermarks in a crime scenario would also obtain a weaker signal which may not be suitable for age estimation.
Table 5 Detailed information of reference compounds in aged fingermark61
Compounds |
Formation |
Rf-value and fluorescence color (fresh) |
Rf-value and fluorescence color (aged) |
Emission maxima (nm) |
3-Indole acetic acid |
Bacterial breakdown |
0.98, blue |
0.84–0.97, yellow |
400–500 |
Norharman |
Tryptophan degradation |
0.84, blue |
—, blue |
400–500 |
Harman |
Tryptophan degradation |
0.79, blue |
—, blue |
400–500 |
Xanthurenic acid |
Tryptophan degradation |
0.93, yellow |
— |
400–500 |
0.98, yellow |
— |
Targeting only one chemical component is not ideal for fingermark age determination in case the donors were unknown. The changes in many substances should be covered, especially the ratios of two substances which can avoid their dependence on the initial components. Research studies have indicated that the tryptophan-containing proteins (Tryp) are attributed to the auto-fluorescence of fingermarks. When exposed to air, the reactive oxidation products (LipOx) in fingermarks react with Tryp and thus form fluorescent oxidation products (FOX).62 Lambrechts's research group used fluorescence spectroscopy (FS) to detect the degradation and generation rate of proteins and oxidation products, respectively.62 They calculated the ratio of fluorescence peak integral area of Tryp to FOX (Trypfl/FOXfl ratio) to establish an estimation model (Fig. 7).62 The male fingermarks that remained for up to three weeks could be estimated with an accuracy of up to 55% and an average error of ±1.9 days.62 Due to lower excretion of epidermal components in female fingermarks, this method was only suitable for male fingermark age determination.62 Additionally, the residues were susceptible to be interfered with environmental factors (e.g. light and temperature conditions).62 In practice, this method can be optimized in the following two aspects: (i) reducing the analysis time and onsite monitoring by the optimization of the experimental set-up and (ii) reducing the number of measurements by using mass spectroscopy or other methods for calculating the ratios of components to un-oxidized proteins. Thereafter, this group successfully utilized the same technique to estimate the time of the deposition of semen traces.63
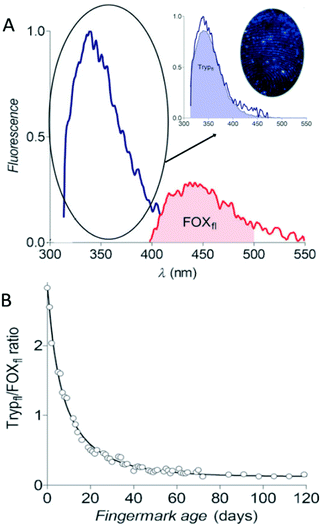 |
| Fig. 7 (A) The fluorescence emission spectra of Tryp and FOX. (B) The aging curve of the Trypfl/FOXfl ratio of fingermarks.62 | |
Other spectroscopy techniques.
Besides the techniques described above, other spectroscopy techniques have emerged as well. Hyperspectral imaging is used to capture images according to the electromagnetic spectrum wavelengths of targets. Merkel et al. reported the ultraviolet-visible hyper-spectral imaging (UV/vis-HSI) method to explore the degradation behaviors of fingermarks. This technique mainly extends the electromagnetic spectrum range to the UV range.64 To investigate whether ultraviolet radiation will alter the prints, one set of fingermarks is constantly radiated by UV spectrum while another set is not.64 No apparent degradation can be observed.64 The influences on the aging process grouped into five main classes were also studied.64 Notably, the different behaviors of eccrine and sebaceous fingermarks were observed. Sebaceous fingermarks rather than eccrine prints were reflective to the emitted radiation, especially in the ultraviolet band. It exhibited an aging behavior that is closest to the expected logarithmic trend.64 Unfortunately, the method cannot identify various kinds of chemical components in fingermarks. Although the sebaceous compositions lead to unpredictable short-term age, they can be utilized for exploring long-term aging patterns.
Except for illicit contaminants studied by Johnston,58 blood is another kind of exogenous contaminant for which the aging pattern has to be achieved. More recently, Cadd et al. have reported that the visible wavelength reflectance hyperspectral imaging technique (Vis-HSI) is suitable for the age determination of blood-stained fingermarks. It focused on the absorption spectrum of hemoglobin between 400 and 680 nm.65 The ratios of 525 nm peak to 550 nm trough in the absorption spectrum were utilized as aging parameters. The logarithmic aging curve is shown in Fig. 8A.65 Moreover, the 30-day and 24 h color scales were made to estimate the fingermark age more effectively and conveniently.65Fig. 8B exhibits the color changes that occurred in the absorption spectrum over a 24-hour period.65
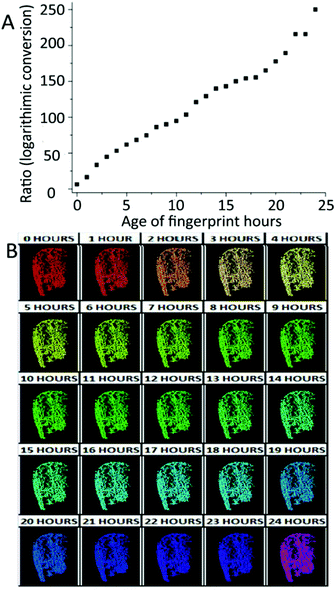 |
| Fig. 8 (A) The absorption ratios of 550 and 525 nm after logarithmic conversion over 24 h. (B) 24 h false color aging scale.65 | |
4.3 Mass spectrometry techniques for fingermark dating
Mass spectrometry has been proved to be a useful technology in recognizing and imaging the chemical components of fingerprint residues, including gunshot residues, drugs, explosives, wax esters, triacylglycerols, fatty acids, proteins, etc. Over the past few years, there have been many mass spectrometry techniques reported such as gas chromatography with mass spectrometry (GC-MS), liquid chromatography with mass spectrometry (LC-MS), time-of-flight secondary ion mass spectrometry (TOF-SIMS) and matrix-assisted laser desorption ionization mass spectrometry (MALSI-MS). We have summarized these techniques in detail below.
Gas chromatography with mass spectrometry technique.
Lipids (e.g. wax esters, squalene and sterol esters) are common aging markers in age determination because they are more durable than other components. The gas chromatography with mass spectrometry (GC-MS) technique has been comprehensively applied for targeting lipids in fingerprints over the years. In Girod's report, six kinds of lipids including isopropyl dodecanoate, cholesterol, squalene and their derivatives were selected from 13 kinds of lipids.66 Then the unknown effects (e.g. deposition moment, pressure, temperature and light conditions) were explored using statistical methods of PCA, univariate exponential regression and partial least squares regression (PLSR).66 The aging model was finally tested using eight unknown fingermarks. Significant results showed that five fingermarks out of eight were correctly estimated.66 In the same year, Frick et al. reported the GC-MS technique to track the aging pattern of 15 lipids in fingermarks over 28 days.67 Moreover, the variables that influence the aging process were explored.67 For data analysis, PCA was also employed to reduce the dimension of datasets.67 The chromatograms were normalized to the sum of all integrated peak areas.67 Consequently, the residue amount differences caused by the distinctions in fingermark size or deposition conditions were overcome.67 The degradation rate of fingermarks was significantly affected by preservation conditions. It was found that squalene exposed to light and air flow was significantly decreased, especially after a 7-day period, while other substances such as free fatty acids and wax esters were stable up to 28 days, regardless of light exposure.67
Gas chromatography with tandem mass spectrometry (GC-MS/MS) exhibits higher sensitivity and selectivity than GC-MS. Szabóová et al. proposed the age estimation method by calculating the ratio of squalene to pentadecanoic acid (C15:0) in fingermarks over time through GC-MS/MS.68 The results showed that aging degradation was highly vulnerable to light conditions and varied from person to person.68 It allowed the age determination of two samples from the same donor whereas those from different donors could not be estimated.68
Liquid chromatography with mass spectrometry technique.
Proteins originating from fingermarks change over time, which makes them also good biomarkers for fitting the aging curve. Oonk et al. developed the high performance liquid chromatography with quadrupole time-of-flight mass spectrometry (HPLC-QTOF-MS) technique for observing the degradation of proteins.2 More specifically, the protein map of fingermarks was drafted, from which the candidates (e.g. four keratin proteins and the anti-microbial peptide dermcidin) were then selected as aging parameters.2 The high-throughput approach worked well for 16-day-old fingermarks. To reduce the environmental contaminants, two methods can be employed: (i) searching MS record information in a contaminant database and (ii) adopting a procedural blank to evaluate the background proteome. Compared to previous studies, the proteomic approach offers new insight into fingerprint and donor profiling analysis. The high sensitivity allows many kinds of proteins to be included in the fingermark proteome database. Meanwhile, this approach speeds up the process of fingerprint examination.
Glyceride is also another aging marker in fingermarks. However, it is difficult to be identified and monitored for degradation due to its diverse structures and products. Ultra-performance liquid chromatography-ion mobility spectrometry-quadrupole time-of-flight mass spectrometry (UPLC-IMS-QTOF-MS) was applied to detect the changes in di- and triglycerides over a period of 28 days.4Table 6 lists the number of triglycerides detected in fingerprints from 5 donors in 1–28 days aging period. Additionally, the process of oxidation of diunsaturated triglycerides to mono- and diozonides was firstly detected. After being stored for 28 days, only diozonides could be identified regardless of whether the fingerprint samples were exposed to light.4 In the future, more research could be focused on the impact of environmental conditions on the degradation of glycerides.
Table 6 The number of triglycerides detected in fingerprints from 5 donors within a period of 28 days4
Triglycerides |
1 day |
7 days |
28 days (desk) |
28 days (drawer) |
Saturated TGs |
26 |
23 |
26 |
25 |
Monounsaturated TGs |
22 |
10 |
0 |
5 |
Diunsaturated TGs |
20 |
7 |
4 |
1 |
Polyunsaturated TGs |
15 |
0 |
0 |
1 |
Time-of-flight secondary ion mass spectrometry technique.
The time-of-flight secondary ion mass spectrometry (TOF-SIMS) technique is a very sensitive surface analytical technique and is well established for a wide range of forensic chemistry applications. The scientific officers in the National Institute of Standards and Technology (NIST) proposed a novel age determination technique by mapping and observing the diffusion of saturated fatty acid molecules in fingerprint residues (molecular weight ranging from 143 g mol−1 to 395 g mol−1).69 The age of fingermarks that remained for less than 96 h can be accurately detected based on the diffusion extent of palmitic acid.69 However, this technique has two limitations: (i) the diffusion property of molecules varied with the substrate types, roughness and contaminant rate69 and (ii) the equation (x = 0.02t1/2) cannot make a correct prediction because the molecules will be exhausted, degraded or oxidized and cannot diffuse indefinitely.69
Matrix-assisted laser desorption ionization mass spectrometry technique.
Since 2009, matrix-assisted laser desorption ionization mass spectrometry (MALDI-MS) has been used to offer the ridge details and chemical information of fingerprints.70 It can detect endogenous and exogenous compounds simultaneously. The mass to charge ratio (m/z) of each molecule over time is profiled due to desorption, ionization and vaporization of the residues.71 One latest study has reported targeting the ratio between triglyceride trihexadecanoyl glycerol (TG(48:1)) and its unsaturated derivatives (TG(48:0)), as well as the formation ratio between TG(48:0)-monoozonide and TG(48:0). The samples were stored under different storage conditions (e.g. lad bench and drawer) and were detected through high performance liquid chromatography coupled with mass spectrometry (HPLC-MS), as shown in Fig. 9A.72 MALDI-MS was additionally utilized to image the chemical components of fresh fingermarks and 26-day-old fingermarks (Fig. 9B).72 The relative signal intensity values can avoid the quantitative difference in the compositions of various fingerprints.72 As O'Neill et al. indicated, the compounds in fingermarks diffused from the ridges to the furrows over time.73 They investigated the diffusion of fatty acids and triacylglycerols by using MALDI-MS. It is found that the diffusion aging model was greatly influenced by surface interactions.73 Whether the component with higher molecular weight could be used to identify the older age is dependent on the substrate nature.73 Therefore, to achieve an accurate aging curve, both the surface properties and other variables should be taken into consideration.73
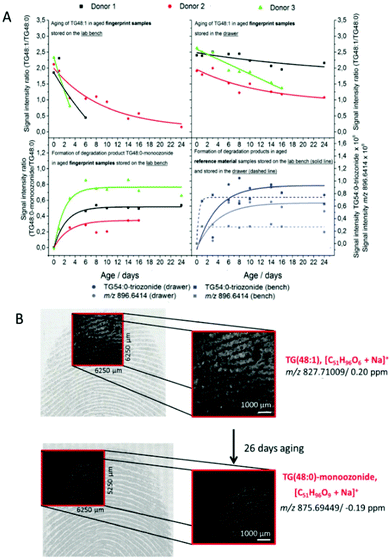 |
| Fig. 9 (A) The aging curve of TG(48:1) to TG(48:0) and the formation curve of TG(54:0) as well as TG(48:0)-monoozonide to TG(48:0). (B) The m/z images overlaid with the optical images of fresh fingermarks and 28-day-old fingermarks.73 | |
5. Conclusion and perspectives
Fingermarks carry the morphological information of fingerprint ridge patterns, and in addition provide the chemical information of fingerprint residues and the characteristics of donors. Over the past 50 years, advances in analytical instruments and new technologies have accelerated the development of forensic chemistry, especially in fingermark age determination. Fingermark age exploration provides a novel insight into the applications to forensic investigation.
This review is organized around recent efforts in fingermark dating by choosing physical and chemical composition characteristics as aging parameters. Specifically, variables that influence the fingermark aging process including donor features (e.g. gender, age, physical conditions and diet), deposition conditions (e.g. pressure, angle and contact time), substrate properties (e.g. porosity, curvature and surface texture), environmental factors (e.g. light, temperature and humidity conditions), and enhancement methods (physical, physico-chemical or chemical) are introduced. Then, fingermark age determination techniques based on physical characteristics including general photography technique, CWL, OP, AFM, EPS, EIS and PFQNM-AFM are discussed in detail. Among them, photography technique, CWL, OP and AFM are primarily based on the morphological changes while EPS, EIS and PFQNM-AFM are mainly based on the electrical changes. The vibrational spectroscopy (e.g. IR, RS, TLC-FS, FS, UV/vis-HIS and Vis-HSI) and mass spectroscopic techniques (e.g. GC-MS, LC-MS, TOF-SIMS and MALDI-MS) are subsequently reviewed. As might be expected, these approaches, along with both advantages and disadvantages, have shown great potential in scientific and accurate age estimation, as presented in Table 7.
Table 7 Summary of fingermark age determination techniques
Techniques |
Ref. |
Aging parameters |
Aging periods investigated |
Advantages |
Disadvantages |
Morphological characteristics |
Photography |
29
|
- Number of minutiae |
- 6 months |
- Take many variables in aging process into account |
- Damage to fingerprint ridges using powder dusting |
30
|
- SIPs including color contrast |
- 6 months |
- High resolution and sensitivity |
- Subjective assessment of ridge discontinuities |
31
|
- Number of ridge discontinuities |
- 6 months |
|
- Limited number of donor and fingerprint samples |
32
|
- Ridge widths |
- 6 months |
- Non-destructive and contactless |
- Used four parameters separately |
CWL |
34
|
- Contrast between fingermarks and backgrounds (2D intensity mode) |
- [0.8 h, 15 h] |
- Deliver high-resolution 2D-intensity as well as 3D topographic images |
- Only suitable for smooth substrates |
35
|
- Ridge heights (3D topography mode) |
- [0, 5 h] and [5, 24 h], |
- Provide all topographical data |
- Cannot be precise to a certain time |
36
|
- Fresh and half a day |
- Take many variables into consideration |
37
|
- [0, 24 h] and [0.5Y, 3Y] |
|
OP |
38
|
- Ridge heights |
- 1 year |
- Deliver high-resolution 2D and 3D images |
- Restricted analysis area |
- Provide all topographical data |
- Slow data acquisition time |
- Insensitivity to very thin fingermark |
- Interference from rough substrates |
AFM |
40
|
- Ridge heights |
- 11 days |
- Deliver 2D and 3D images at nano/micro scale |
- Interference from rough substrates |
42
|
- Coverage area rates, limit and thickness of the intermediate area |
- 61 days |
- Provide all topographical data |
|
Electrical characteristics |
EPS |
44
|
- Electrostatic charges |
- 14 days |
- Contactless |
- Long-scanning time |
- High spatial resolution over 500 dpi |
- Restricted analysis area |
- Only suitable for insulated substrates |
EIS |
26
|
- Capacitance between fingermarks and substrates |
- 45 days |
- Good response to physical modifications in fingermarks |
- Only suitable for conductive substrates |
- High sensitivity and low noise |
- Variables in aging process are not taken into account |
PFQNM-AFM |
45
|
- Surface adhesion |
- 4 weeks |
- Deliver high-resolution images, as well as adhesion, stiffness, elastic modulus and deformation information |
- Interference from rough substrates |
|
Spectroscopy |
IR |
49
|
- Sebaceous: C–H and C-H2, C O, C–H3, C–C–O and O–C–C |
- 34 days |
- Non-destructive |
- Cannot be used for every substrate type |
49
|
- Eccrine: N–H and C–N |
- 1 month |
- Rapid analysis |
50
|
- Exogenous substances including AN, SC and C |
- Take substrate types and preservation conditions into consideration |
- Work well on non-porous substrates |
RS |
52
|
- Carotenoids |
- 700 hours |
- Non-destructive |
- Limited the number and variability of donors |
- Squalene |
- Suitable for a wide range of materials |
- Unsaturated fatty acids |
- Needs no pre-treatment process |
- Proteins |
- Strong identification ability of exogenous substances |
- Take light conditions and substrate types into consideration |
TLC-FS |
53
|
- Tryptophan derivatives |
- 3 weeks |
- Stronger fluorescence on older marks |
- Destructive |
- Take preservation conditions into consideration |
- Only a 3-week aging period |
- No quantification, only gave an indication about the fluorescent substances |
- Single fingermarks in crime scenario obtain weaker signal |
FS |
54
|
- The fluorescence peak integral area of Tryp to FOX |
- 120 days |
- Non-destructive |
- Interference due to environmental influences (e.g. light and temperature) |
- Ratios between target compounds were investigated, which avoid their dependence on initial components |
UV/vis-HIS or Vis-HSI |
56
|
- Sebaceous and eccrine fingermarks: reflectance |
- 3 days |
- Take five variables in aging process into consideration |
- Long capturing time |
57
|
- Hemoglobin in blood-stained fingermarks: the ratios of 525 nm peak to 550 nm trough |
- 30 days |
- Make 30-day color scale to estimate effectively and conveniently |
|
Mass spectrometry |
GC-MS |
58
|
- Lipids including wax esters, squalene and sterol esters |
- 28 days |
- Sensitive and reliable |
- Destructive |
59
|
- The ratio of squalene to pentadecanoic acid (C15:0) |
- 9 days |
- Take many variables in aging process into consideration |
- The sample from different donors could not be estimated |
- Sensitive and reliable |
- Take light conditions and donor features of aging process into consideration |
LC-MS |
2
|
- Proteins including four keratin proteins and the anti-microbial peptide dermcidin |
- 16 days |
- High-throughput |
- No consideration for influential factors in fingermark aging process |
4
|
- Di- and triglycerides as well as their products |
- 28 days |
- Highly sensitive |
- Draft a first fingerprint protein map |
- Continuing oxidation over time can be seen |
TOF-SIMS |
61
|
- The diffusion of saturated fatty acid molecules |
- 96 hours |
- High sensitivity and resolution |
- Only suitable for fingermarks that remained for less than 96 hours |
- Detect multiple chemical components simultaneously |
- Only suitable for limited substrates |
MALDI-MS |
64
|
- The ratio between TG(48:1) and its unsaturated derivatives |
- 1 week |
- Provide great ridge details and chemical information simultaneously |
- No consideration for influential factors in fingermark aging process |
65
|
- The diffusion of fatty acids and triacylglycerols |
- 26 days |
- High sensitivity and resolution |
Although considerable state-of-the-art achievements have been attained in fingerprint analysis, age determination is still at its early stage in that fingerprint residues are highly unstable to various variables in the aging process. Commonly, the acquisition of aging markers and exploration of the impact of various variables on fingermark degradation need to be achieved for fingermark age estimation in forensic investigations. Specifically, (i) for aging parameters: the aging indicators can be obtained in three routes. Firstly, new techniques of forensic science (e.g. non-invasive optical microscopy, non-destructive spectroscopy, electrochemical microscopy, spectroscopic methods, etc.) can be employed to obtain the existing aging markers. For example, high-resolution optical microscopy can be employed to capture level 2 and 3 details of water-developed sebaceous fingermarks on cellulose membranes; secondly, it is expected to explore new targets in fingermarks for monitoring the physical or chemical changes; thirdly, reliable markers can be also achieved by using data analysis and processing of existing aging parameters; and (ii) for variables: investigating the primary and secondary factors in fingermark deposition and aging stages is necessary. Without doubt, the main factors should be integrated into the degradation model of fingermarks.
Conflicts of interest
There are no conflicts to declare.
Acknowledgements
The authors acknowledge the financial support from the National Natural Science Foundation of China (No. 21775011 and 21727815), National Engineering Laboratory for Forensic Science (No. 2019NELKFKT10) and the National Key Research and Development Program of China (No. 2018YFC0807205).
Notes and references
- Y. Wang, J. Wang, Q. Ma, Z. Li and Q. Yuan, Nano Res., 2018, 11, 5499–5518 CrossRef CAS.
- S. Oonk, T. Schuurmans, M. Pabst, L. de Smet and M. de Puit, Sci. Rep., 2018, 8, 16425 CrossRef.
- M. Zhang and H. H. Girault, Analyst, 2009, 134, 25–30 RSC.
- A. A. Frick, N. Kummer, A. Moraleda and C. Wayermann, Analyst, 2020, 145, 4212–4223 RSC.
- N. Lauzon and P. Chaurand, Analyst, 2018, 143, 3586–3594 RSC.
- H. Chen and L. Liu, Bull. Chem. Soc. Jpn., 2018, 91, 1319–1324 CrossRef CAS.
- Q. Wei, X. Li, X. Du, X. Zhang and M. Zhang, Sci. China: Chem., 2017, 60, 1250–1257 CrossRef CAS.
- S. Moret, A. Bécue and C. Champod, Forensic Sci. Int., 2016, 259, 10–18 CrossRef CAS.
- Y. B. Zhao, Y. J. Ma, D. Song, Y. Liu, Y. Luo, S. Lin and C. Y. Liu, Anal. Methods, 2017, 9, 4770–4775 RSC.
- L. R. Xu, Z. Y. Cao, R. l. Ma, Z. Z. Wang, Q. Qin, E. Y. Liu and B. Su, Anal. Chem., 2019, 91, 12859–12865 CrossRef CAS.
- E. Locard, Am. J. Police Sci., 1930, 1, 401–418 CrossRef.
-
R. Byard and J. Payne-James, Encyclopedia of forensic and legal medicine, Academic Press, Salt Lake, 2015 Search PubMed.
-
A. Girod, C. Roux and C. Weyermann, Revue Internationale de Criminologie et de Police Technique et Scientifique, 2014, vol. 67, pp. 226–249 Search PubMed.
- N. E. Archer, Y. Charles, J. A. Elliott and S. Jickells, Forensic Sci. Int., 2005, 154, 224–239 CrossRef CAS.
- S. Cadd, M. Islam, P. Manson and S. Bleay, Sci. Justice, 2015, 55, 219–238 CrossRef.
- P. Watson, R. J. Prance, S. T. Beardsmore-Rust and H. Prance, Forensic Sci. Int., 2011, 209, e41–e45 CrossRef.
- A. O'Hagan and S. Green, Forensic Res. Criminol. Int. J., 2018, 6, 491–503 Search PubMed.
- A. Girod, R. Ramotowski and C. Weyermann, Forensic Sci. Int., 2012, 223, 10–24 CrossRef CAS.
-
C. Champod, C. Lennard, P. Margot and M. Stoilovic, Fingerprints and Other Ridge Skin Impressions, CRC Press, Boca Raton, 2017 Search PubMed.
- L. Xu, C. Zhang, Y. He and B. Su, Sci. China: Chem., 2015, 58, 1090–1096 CrossRef CAS.
- Q. Wei, M. Zhang, B. Ogorevc and X. Zhang, Analyst, 2016, 141, 6172–6189 RSC.
- J. De Alcaraz-Fossoul, C. M. Patris, A. B. Muntaner, C. B. Feixat and M. G. Badia, Int. J. Leg. Med., 2013, 127, 857–870 CrossRef.
- B. N. Dorakumbura, R. E. Boseley, T. Becker, D. E. Martin, A. Richter, M. J. Tobin, W. van Bronswjik, J. Vongsvivut, M. J. Hackett and S. W. Lewis, Analyst, 2018, 143, 4027–4039 RSC.
-
F. Cuthbertson, The chemistry of fingerprints, HM Stationery Office, 1969 Search PubMed.
- R. S. Croxton, M. G. Baron, D. Butler, T. Kent and V. G. Sears, Forensic Sci. Int., 2010, 199, 93–102 CrossRef CAS.
- D. J. Penn, E. Oberzaucher, K. Grammer, G. Fischer, H. A. Soini, D. Wiesler, M. V. Novotny, S. J. Dixon, Y. Xu and R. G. Brereton, J. R. Soc., Interface, 2007, 4, 331–340 CrossRef.
- O. P. Jasuja, M. A. Toofany, G. Singh and G. S. Sodhi, Sci. Justice, 2009, 49, 8–11 CrossRef.
- K. Bobev, J. Forensic Sci., 1995, 45, 176–183 Search PubMed.
- J. Almog, M. Azoury, Y. Elmaliah, L. Berenstein and A. Zaban, J. Forensic Sci., 2004, 49, 981–985 Search PubMed.
- N. Jones, D. Mansour, M. Stoilovic, C. Lennard and C. Roux, Forensic Sci. Int., 2001, 124, 167–177 CrossRef CAS.
- A. Koenig, A. Girod and C. Weyermann, J. Forensic Identif., 2011, 61, 652–676 Search PubMed.
- R. M. Barros, B. E. Faria and S. A. Kuckelhaus, Sci. Justice, 2013, 53, 402–408 CrossRef.
- K. Wertheim, J. Forensic Identif., 2003, 53, 42 Search PubMed.
- R. Rosa, R. Giovanardi, A. Bozza, P. Veronesi and C. Leonelli, Forensic Sci. Int., 2017, 273, 144–152 CrossRef CAS.
- A. Girod, R. Ramotowski, S. Lambrechts, P. Misrielal, M. Aalders and C. Weyermann, Forensic Sci. Int., 2016, 262, 212–226 CrossRef CAS.
-
S. M. Bleay, R. S. Croxton and M. De Puit, Fingerprint Development Techniques, John Wiley Sons Ltd. Publication, Manhattan, 2018 Search PubMed.
- J. De Alcaraz-Fossoul, C. B. Feixat, J. Tasker, L. McGarr, K. Stow, C. Carreras-Marin, J. T. Oset and M. G. Badia, J. Forensic Sci., 2016, 61, 947–958 CrossRef CAS.
- J. De Alcaraz-Fossoul, C. B. Feixat, C. Carreras-Marin, J. Tasker, S. C. Zapico and M. G. Badia, J. Forensic Sci., 2017, 62, 1180–1187 CrossRef CAS.
- J. De Alcaraz-Fossoul, C. B. Feixat, S. C. Zapico, L. McGarr, C. Carreras-Marin, J. Tasker and M. G. Badia, J. Forensic Sci., 2019, 64, 1057–1066 CrossRef CAS.
- J. De Alcaraz-Fossoul, C. M. Patris, C. B. Feixat, L. McGarr, D. Brandelli, K. Stow and M. G. Badia, J. Forensic Sci., 2016, 61, 322–333 CrossRef CAS.
-
S. Kiltz, M. Hildebrandt, J. Dittmann and C. Vielhauer, presented in part at the 2012 Proceedings of the 20th European Signal Processing Conference (EUSIPCO), Bucharest, Romania, 8, 2012.
-
R. Merkel, S. Gruhn, J. Dittmann, C. Vielhauer and A. Bräutigam, presented in part at the Three-Dimensional Image Processing (3DIP) and Applications II, Burlingame, California, United States, 1, 2012.
- R. Merkel, S. Gruhn, J. Dittmann, C. Vielhauer and A. Bräutigam, Forensic Sci. Int., 2012, 222, 52–70 CrossRef.
-
R. Merkel, J. Dittmann and C. Vielhauer, presented in part at the 2016 Sixth International Conference
on Image Processing Theory, Tools and Applications (IPTA), Oulu, Finland, 12, 2016.
-
R. Merkel, J. Dittmann and C. Vielhauer, presented in part at the IEEE Transactions on Information Forensics and Security, 5, 2017.
- J. De Alcaraz-Fossoul, M. Mancenido, E. Soignard and N. Silverman, J. Forensic Sci., 2019, 64, 570–576 CrossRef.
-
T. Smijs and F. Galli, in Atomic-force Microscopy and Its Applications, IntechOpen, New York, 2018 Search PubMed.
- A. J. Goddard, A. R. Hillman and J. W. Bond, J. Forensic Sci., 2010, 55, 58–65 CrossRef.
- T. Smijs, F. Galli and A. van Asten, Forensic Chem., 2016, 2, 93–104 CrossRef CAS.
- K. T. Popov, V. Sears and B. J. Jones, Forensic Sci. Int., 2017, 275, 44–56 CrossRef CAS.
- S. J. Fieldhouse, N. Kalantzis and A. W. Platt, Forensic Sci. Int., 2011, 206, 155–160 CrossRef.
- P. Watson, R. Prance, H. Prance and S. T. Beardsmore-Rust, Opt. Photonics Count. Crime Fighting VI & Opt. Mater. Defence Syst. Tech. VII, 2010, 7838, 783803 Search PubMed.
- B. N. Dorakumbura, T. Becker and S. W. Lewis, Forensic Sci. Int., 2016, 267, 16–24 CrossRef.
- Y. Dikshitulu, L. Prasad, J. Pal and C. Rao, Forensic Sci. Int., 1986, 31, 261–266 CrossRef CAS.
- J. M. Duff and E. R. Menzel, J. Forensic Sci., 1978, 23, 129–134 CAS.
- S. Zha, X. Wei, R. Fang, Q. Wang, H. Lin, K. Zhang, H. Zhang, R. Liu, Z. Li and P. Huang, Forensic Sci. Res., 2019, 1–7 Search PubMed.
- A. Girod, L. Xiao, B. Reedy, C. Roux and C. Weyermann, Forensic Sci. Int., 2015, 254, 185–196 CrossRef CAS.
- A. Johnston, J. Forensic Res., 2018, 9, 418–423 Search PubMed.
- K. C. Doty and I. K. Lednev, TrAC, Trends Anal. Chem., 2018, 103, 215–222 CrossRef CAS.
- P. O. Andersson, C. Lejon, T. Mikaelsson and L. Landström, ChemistryOpen, 2017, 6, 706–709 CrossRef CAS.
- A. van Dam, M. C. Aalders, T. Todorovski, T. G. van Leeuwen and S. A. Lambrechts, Forensic Sci. Int., 2016, 258, 19–25 CrossRef CAS.
- A. van Dam, J. C. Schwarz, J. de Vos, M. Siebes, T. Sijen, T. G. van Leeuwen, M. C. Aalders and S. A. Lambrechts, Angew. Chem., Int. Ed., 2014, 53, 6272–6275 CrossRef CAS.
- N. Achetib, L. S. Wilk, J. C. Schwarz, S. A. Lambrechts, T. G. van Leeuwen, M. C. Aalders and A. van Dam, Anal. Chem., 2019, 91, 3204–3208 CrossRef CAS.
-
R. Merkel, presented in part at the 2015 Ninth International Conference on IT Security Incident Management & IT Forensics, Magdeburg, Germany, 5, 2015.
- S. Cadd, B. Li, P. Beveridge, W. O'Hare and M. Islam, J. Imaging, 2018, 4, 141 CrossRef.
- A. Girod, A. Spyratou, D. Holmes and C. Weyermann, Sci. Justice, 2016, 56, 165–180 CrossRef.
- A. Frick, G. Chidlow, J. Goodpaster, S. Lewis and W. Van Bronswijk, Forensic Chem., 2016, 2, 29–36 CrossRef CAS.
- Ž. Szabóová, P. Galbavá, A. H. Szabó, M. Cigáň, Ľ. Nižnanský, R. Kubinec and J. Blaško, Monatsh. Chem., 2017, 148, 1673–1678 CrossRef.
- S. Muramoto and E. Sisco, Anal. Chem., 2015, 87, 8035–8038 CrossRef CAS.
- R. Wolstenholme, R. Bradshaw, M. Clench and S. Francese, Rapid Commun. Mass Spectrom., 2009, 23, 3031–3039 CrossRef CAS.
- M. Gorka, M. Augsburger, A. Thomas and A. Bécue, Forensic Chem., 2019, 12, 99–106 CrossRef CAS.
- S. Pleik, B. Spengler, D. R. Bhandari, S. Luhn, T. Schafer, D. Urbach and D. Kirsch, Analyst, 2018, 143, 1197–1209 RSC.
- K. C. O'Neill and Y. J. Lee, J. Forensic Sci., 2018, 63, 708–713 CrossRef.
|
This journal is © The Royal Society of Chemistry 2021 |