DOI:
10.1039/D0AN01274B
(Tutorial Review)
Analyst, 2020,
145, 7162-7185
From single cells to complex tissues in applications of surface-enhanced Raman scattering
Received
25th June 2020
, Accepted 21st September 2020
First published on 23rd September 2020
Abstract
As surface-enhanced Raman scattering (SERS) continues to grow in popularity, more work needs to be done to evaluate its compatibility with a wider scope of applications. With such a strong emphasis on SERS being used for biosensing, it is important to examine how SERS is used in bioanalytical nanoscience, and more importantly, look towards where SERS is heading. For many, the initial steps involve demonstrating in vivo sensing by SERS using cultures of live cells. To further and better demonstrate the capabilities of SERS as a technique in bioanalytical nanoscience, it is necessary to transition away from studies involving single cells or small quantities of cells. This means working with tissue, typically as an ex vivo slice or a spheroid, before moving onto in vivo animal models. Although working with tissue as opposed to single cells introduces new challenges, the types of approaches developed for single cell studies serve as the foundation for the more complex biomaterials. The aim of this tutorial review is to better facilitate the transition from single cells to complex tissues by demonstrating the similarities in the methodologies that have been used and how to overcome some of the challenges of working with tissue. Specifically, we explore how three of the most common methods of working with nanoparticles and cells have been adapted and incorporated for experiments involving different types of tissues. Overall, this review highlights a variety of methods that can be readily implemented for those wishing to perform SERS measurements with or in complex tissues.
1 Introduction
The ability to accurately differentiate between molecular species at low concentrations and chemical environments in relatively short periods of time and by non-destructive means are important criteria for techniques looking to be used in biosensing. Vibrational spectroscopies, such as Raman scattering, can differentiate analytes based on their distinct vibrational spectra in a non-invasive manner. However, Raman scattering is an inefficient process with approximately only 1 in 106–108 photons being inelastically scattered.1 As a result, some combinations of large quantities of material, long acquisition times, and high laser powers are often needed. These limitations can hinder the applicability of traditional Raman experiments with biosensing. The incorporation of conductive (typically metallic) particles with sub-wavelength geometries allows for an enhancement of the otherwise weak Raman signal for analytes adsorbed onto or very near the surface of the nanoparticle. Surface-enhanced Raman scattering (SERS) relies on enhancements related to the opto-geometric properties of the nanostructure (electromagnetic enhancement) and the properties of the analyte (chemical and resonance enhancements).2 The combination of these enhancement mechanisms allows for low quantities of analytes (down to single molecules) to be detected with rapid acquisition times and lower laser powers. As a result, SERS has emerged as a powerful tool throughout applications in analytical chemistry,3 including forensics,4 food safety,5,6 environmental science,7 and most notably biosensing.8–17
The versatility of SERS arises from the ability to use different sensing schemes. Intrinsically, SERS is a label-free technique as it allows for the direct acquisition of an analyte's SERS spectrum. Depending on the analyte, this can however be rather challenging. As a result, alternative methods based on the use of Raman reporter molecules can be used. A summary of different sensing schemes applicable to biosensing are shown in Scheme 1. The simplest approach is to collect the SERS spectra of the analyte(s) (Scheme 1i). In order to better understand the results obtained using this method, subsequent use of machine learning algorithms is advised as these can be used to group and classify the obtained spectra.18 Alternatively, the surface of the nanoparticle can be functionalized with a SERS reporter molecule (identified as a star) that is capable of undergoing a chemical transformation as a result of the chemical environment or exposure to a specific analyte (Scheme 1ii). By comparing the SERS spectra obtained before and after exposure, it is possible to extract information about specific biological processes. Molecular interactions between species bound to different surfaces can be used to create hybrid nanostructures. Importantly, this hybrid structure must have a nanoparticle with a SERS reporter molecule either on the surface or protected within a core–molecule–shell structure. The sensing schemes using this approach can be based on the loss of or the gain in the SERS response. In the first case, one of the surface bound species, such as an aptamer, is selective for a target analyte. When the analyte is present, the hybridization between the surface and functionalized nanoparticles is lost (Scheme 1iii), resulting in a loss in the signal of the SERS reporter on the nanoparticle. The reverse process can also occur (Scheme 1iv) where the SERS response is obtained only after exposure to the target analyte. In this case, a two-step process is required where the analyte first interacts with the species bound on one surface before interacting with the other surface-bound molecule. These sensing schemes will be referred to and discussed in greater detail throughout this review.
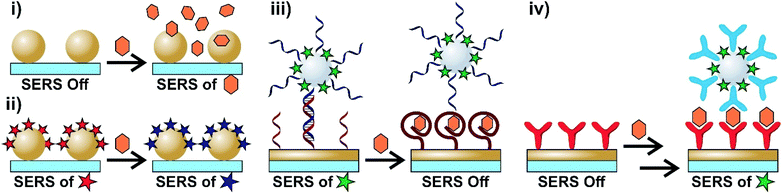 |
| Scheme 1 Common SERS biosensing sensing schemes. (i) Intrinsic SERS spectra of analytes are collected. (ii) Species present in the sample cause a chemical change in an adsorbed Raman reporter causing a chemical change in the reporter and a different SERS spectrum. (iii) A SERS nanoparticle with a Raman reporter is bound to a metallic surface (nanoparticle or film). When a specific analyte interacts with the adsorbed molecule (i.e. aptamer), that molecule undergoes a structural change and the reporter nanoparticle is released, causing a loss of the SERS signal. (iv) The target analyte binds to a molecule that is adsorbed onto the metal surface. Reporter functionalized nanoparticles are subsequently introduced that also interact with the analyte allowing for the SERS spectrum of the nanotag to be collected. | |
The end goal for many SERS-based biosensing studies is to eventually demonstrate that they can be used for in vivo studies. SERS measurements involving single cells are a common means of demonstrating biosensing, specifically in vivo sensing. It is however necessary to recognize that there are physiological processes that involve complex organizations of cells. Examples of these phenomena include intercellular transmission and the progression of tumor microenvironments. Although some insight into intercellular communication can be gained by working with cell cultures, such as detecting the secreted molecules and biomaterials,19–21 the overall effect of the cell–cell signalling is difficult to explore at the single cell level. Likewise, the biochemical composition of tumor microenvironments is derived from the large quantities and different types of cells that compose the tumor.22 Therefore, to explore these and other physiological phenomena, it is necessary to work with tissue samples. One alternative is to use tissue spheroids (three-dimensional cell cultures) as they possess their own microenvironment and act as a better model than monolayer cultures.23–26
Making the transition away from single cell studies can often be a significant challenge for many researchers as tissue samples bring their own unique set of difficulties. As the biochemical composition of the tissue can provide a large quantity of information, finding ways of extracting that knowledge is important. One way of gaining this insight is to extract the biochemical contents of the tissue, for example using mechanical methods (i.e. crushing, squeezing, poking, centrifugation). The subsequent addition of a colloidal nanoparticle solution and or plasmonic surface allows for the SERS spectra of the biochemical contents to be acquired. This can then be used to differentiate cancerous and normal tissue,27,28 and the grade of the cancer as was demonstrated for gliomas.29 Alternatively, enzymatic digestion can be used to take the tissue and revert it to single cells.30 The focus however of this tutorial review is to examine methods whereby the tissue remains intact during the experiment.
Given the depth of studies involving intact cells, we use how the nanoparticles are incorporated with or into the cell to provide the foundation needed to ease the transition to working with complex tissues. The three common approaches are shown in Scheme 2, where the nanoparticles are either on the outside of the cell, internalized within the cell, or adsorbed onto a nanofiber that is subsequently inserted into the cell. These three approaches can and have also be used for SERS measurements in tissue. Before demonstrating how this can be done, it is necessary to examine what role the tissue itself will play when performing these experiments.
 |
| Scheme 2 Three approaches for performing SERS experiments with cells, where the plasmonic nanoparticles can be located at the surface of the cell, internalized within the cell, or on a nanofiber that is then inserted into the cell. | |
2 Optical properties of tissue
Given the microscale height of cells, optical interference from the various biological components is considerably less than that of tissue. For situations where the nanoparticles are within the tissue, the ideal scenario is for excitation photons to penetrate the tissue, reach the nanoparticles, excite the LSPR, reach the molecule of interest, inelastically scatter, excite the LSPR again, travel back through the tissue and reach the collection optics. Optical interference from the tissue can occur in a variety of ways including interface reflection, absorption, scattering, and autofluorescence,31 with the latter three occurring within the tissue, and happening before the photons reach the nanoparticles and after the photons have been inelastically scattered and reach the detector. Here, we emphasize losses due to absorbance and scattering. Although the chemical compositions of tissues have considerable variability, there are key components that can be used to create theoretical absorption spectra.32 These components are as follows:
B, average blood volume fraction; S, hemoglobin oxygen saturation of mixed arterio-venous vasculature; W, water content; F, fat content; M, melanosome volume fraction or molar concentration of melanin monomers; Cbili, bilirubin concentration; CβC, β-carotene concentration; oxy, oxyhemoglobin; deoxy, deoxyhemoglobin; μa, absorption coefficient
|  | (1) |
| μa = BSμa.oxy + B(1 − S)μa.deoxy + Wμa.water + Fμa.fat | (2) |
Eqn (1) considers all these components, whereas eqn (2) uses a simplified version that only considers oxy- and deoxyhemoglobin, water, and fat as the contents for the other components appear in lower quantities. A series of theoretic absorption spectra based on eqn (2) are shown in Fig. 1A where the water and fat concentrations vary. The values for B, S, W, F, M will differ depending on the type of tissue. Here, we are only considering a generic tissue. In the visible region, there is almost no variation with changing water and fat content. This is because the absorbance in this region is from the oxy- and deoxyhemoglobin. Within this region, other components previously mentioned (melanin, bilirubin, and β-carotene) will also absorb photons. The absorption spectra for these components can be found in ref. 32. In the first near-infrared window (near-IR – I) the variation occurs as the relative amounts of water and fat change. Without any water present (purple spectrum of Fig. 1A), there is a distinct peak at 930 nm attributed to the lipids. As the water content increases and the fat content decreases, this peak becomes dampened in favour of a broad band from 920 to 1000 nm.
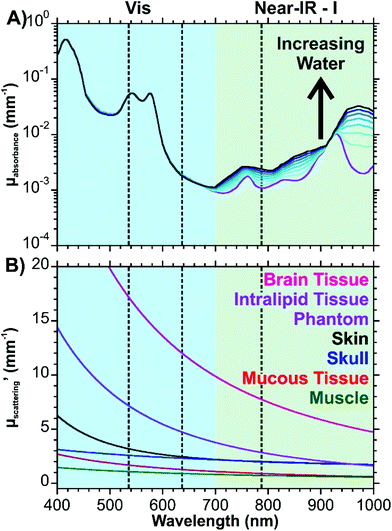 |
| Fig. 1 Optical properties of tissue. (A) Theoretical absorption spectrum of tissue based on eqn (2). Parameters used are as follows: B = 0.002, S = 0.75, F + W = 0.7 (0.1 increments). Values for μa.oxy and μa.deoxy are from ref. 41, μa.water from ref. 42, and μa.fat from ref. 43. A logarithmic scale is used to better show the variation. (B) Wavelength dependent reduced scattering coefficients for different types of tissues using eqn (3)–(8). The dashed lines indicate common excitation wavelengths (532, 633, and 785 nm) used in Raman measurements. | |
The equations for the reduced scattering coefficient (mm−1) for various tissues are provided in eqn (3)–(8):33–38
| μ′s(brain tissue) = 4.72(λ/μm)−2.07 | (3) |
| μ′s(1% Intralipid tissue phantom) = 1.6(λ/μm)−2.4 | (4) |
| μ′s(skin) = 0.11(λ/μm)−4 + 1.61(λ/μm)−0.22 | (5) |
| μ′s(skull) = 1.72(λ/μm)−0.65 | (6) |
| μ′s(mucous tissue) = 0.61(λ/μm)−1.62 | (7) |
| μ′s(muscle) = 0.56(λ/μm)−1.045 | (8) |
These equations are based off results obtained from human (skin, skull, mucous, muscle), mouse (brain), and tissue phantom (Intralipid) samples. All the equations have an exponential decay, resulting in decreasing reduced scattering coefficients (μ′s) with increasing wavelength (Fig. 1B). This figure shows that losses due to scattering will occur more frequently with brain tissue over the other tissues shown. Interestingly, the Intralipid tissue phantom has a spectrum that is closest to brain tissue. As a result, this type of sample may make for a decent mimic of brain tissue during the development of experimental protocols.
The results of Fig. 1 provide an insight into the “biological window” as it relates to the wavelength of the excitation photons and the Raman scattered photons. It is necessary to consider both types of photons as the optical interference from the tissue will occur as the photons reach the nanoparticles and after the photons are scattered. With regards to the absorption of photons, excitation wavelengths of 633 and 785 nm are comparable and are an order of magnitude less than that of 532 nm. Depending on the type of tissue, the scattering coefficients can be more similar between the excitation wavelengths, though as shown by brain and Intralipid tissue samples, longer excitation wavelengths are preferable due to the exponential decay. Biological chromophores, such as melanins, aromatic amino acid residues in proteins, NADH, heterocyclic flavins, and pigmented cellular structures will not only absorb photons, they will also contribute to the autofluorescence background.31,39 Taking into consideration that biological fluorophores and chromophores are most susceptible to visible excitations, it is preferable to work with an excitation wavelength in the near-IR to minimize the autofluorescence background of complex tissues.40 In circumstances where this is not possible, a laser with as long of a wavelength in the visible region as possible would need to be used.
3 Nanostructures on the outer surface
As was shown in Scheme 2, nanoparticles can be added to the surface of cells. Since the SERS enhancement only extends several nm's from the surface of the nanostructures, it is only sensitive to the membrane of the cell or the outermost layer of the tissue. However, as the SERS-active layer is nearer to the excitation source than the tissue, this method overcomes the optical properties of the tissue because the incident photons will not be absorbed or scattered by the tissue prior to reaching the nanostructures. This allows for compatibility with a wider range of excitation wavelengths.
3.1 Targeted delivery of nanotags
The more commonly used approach is to locally deliver nanoparticles to the membrane of the cell or domains of the tissue that express a specific biomolecule, such as a protein. This requires the preparation of bio-orthogonal nanoparticles, herein referred to as nanotags. In the field of bio-SERS, a considerable amount of work has been and is consistently being done in the development of nanotags and are therefore constantly reviewed in greater detail.2,44–46 Here, we simply provide insight into the general design of nanotags and the types of SERS measurements that are often performed using them.
3.1.1 General design of nanotags for localized SERS measurements.
A general approach to the preparation of SERS nanotags is shown in Scheme 3. In the initial step, metallic nanoparticles are synthesized. The shape and size of the nanoparticles are typically tuned to maximize the enhancement at the desired excitation wavelength. Once prepared, it is necessary to add a SERS-active molecule to the surface of the nanoparticle. The SERS spectrum of this molecule is what is used to identify the location of the SERS nanotags. Molecules used in this step typically exhibit one or more of the following spectral characteristics: (i) resonance enhancement with the excitation wavelength causing a surface-enhanced resonance Raman scattering (SERRS) effect; (ii) a strong SERS spectrum without resonance enhancement; (iii) SERS active vibrational modes that are positioned away from the vibrational modes of biomolecules. Generating SERRS enhancement is typically done by selecting a dye molecule that is in electronic resonance with the excitation wavelength. Continuing from the early development of SERS nanotags,47 dye molecules remain a popular choice.48 Aromatic thiols, notably derivatives of benzene thiol, exhibit strong SERS spectra due to the large Raman scattering cross-section of the aromatic ring.49 Alternatively, aryl diazonium salts can be used instead of a thiol.50 The ability to differentiate the SE(R)RS signal from the background signal is important, and as a result, evaluating multiple reporter molecules is a beneficial step.51,52 Simply relying on the strong signal is not always ideal, and therefore having a distinct peak in a region that is typically dormant (1800–2400 cm−1) is helpful in this regard. Alkynes, nitriles, and azides are ideal functional groups as their vibrational modes lie within this region.53 Transition metal carbonyls can also be incorporated into SERS nanotags as they can have vibrational modes at Raman shifts greater than 1800 cm−1.54,55 By using a dye or aromatic thiol with a nitrile group (Prussian Blue or 4-mercaptobenzonitrile respectively) it is possible to obtain both a strong signal and a peak that lies within the dormant domain.56,57 An outer shell is then added, with a chemical composition that is designed to minimize aggregation of the nanoparticles in the biofluid and non-specific interactions with the cells or tissue. Furthermore, the formation of a protein corona around the nanoparticle upon exposure to biological media will occur, and can therefore be minimized or prevented using shorter peptides as opposed to long chain polymers.58 It is possible to combine this layer with the reporter layer. For example, ethylene glycol-modified Raman reporter molecules can be used during the preparation of the nanotags.59 As well, a metallic shell can also be prepared to create a stronger enhancement in the nanoscale gap between the metallic core and shell.60 However, it is important to recognize that the use of a silver layer alone is often harmful to cells.61 The final step in the preparation of nanotags is to incorporate a means of targeting a specific biomarker, such as a protein.
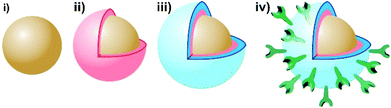 |
| Scheme 3 Preparation of nanotags using (i) a gold nanoparticle as the plasmonic core. (ii) A Raman-active molecule is added to the surface of the metallic nanoparticle. (iii) An outer shell is added that is subsequently functionalized with a biomolecule (i.e. antibody) that binds with a membrane-bound receptor. | |
3.1.2 Use of external SERS nanotags in cell and tissue studies.
The purpose of the nanotags is to use the outermost biomolecule to target a specific membrane structure, and then use the internalized Raman reporter to evaluate the distribution of the membrane structure across the surface of the cell and various cell types, most commonly cancer cells. Although the common approach is to use the add the nanotags to the cells, nanotags immobilized onto a planar surface can also be used to capture cells that express the desired target.62 As opposed to going into detail about the specific targets that nanotags have been developed for, we want to briefly discuss one of the greatest advantages that SERS nanotags provide, the capability of readily performing multiplexing measurements. To perform these experiments, it is necessary to develop nanotags with distinct “flavours”. This is done by preparing a series of nanotags with each nanotag using a different Raman reporter molecule for each target.53,63–66 It is therefore important that the Raman reporters have distinct SE(R)R spectra. Depending on the desired approach, this can be done by using distinct dyes, or variations on the same principal structure. Though the latter approach most often requires that each type of molecule be synthesized, it allows for the same functional group (i.e. alkyne) to act as the reference peak (Fig. 2A–C).66 The multiplexing experiments can be used to differentiate types of cells by looking at the expression of different proteins, using an approach resembling that of flow cytometry.67–69 Alternatively, on the same cell, the expression of the various target proteins can be mapped. As examples, folate receptors and luteinizing hormone-releasing hormone receptors are overexpressed in cancer cells. By creating SERS nanotags that are functionalized with the corresponding molecules, it is possible to image their distributions (Fig. 2D).66
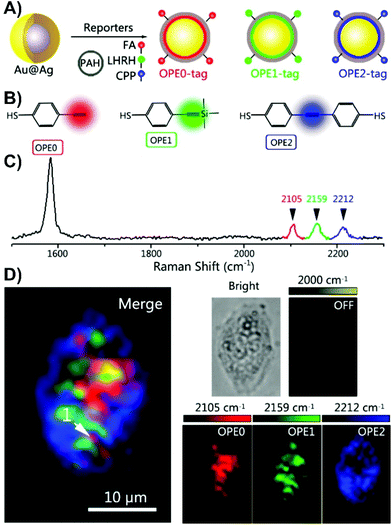 |
| Fig. 2 (A) The schematic diagram for the polyaromatic hydrocarbon (PAH) coated and peptide/small-molecule modified alkyne-based SERS nanotags. (FA = folate, LHRH = luteinizing hormone-releasing hormone, CPP = CALNNR8 (polypeptide)). (B) Structures of the 4-ethynylbenzenethiol-derivatives used as Raman reporters. (C) Normalized Raman spectra of the alkyne SERS nanotags. (D) Three-colour SERS imaging using the alkyne SERS nanotags in the same live HeLa cell. Adapted with permission from Anal. Chem., 2016, 88, 6115–6119 (ref. 66). Copyright (2016) American Chemical Society. | |
The use of SERS nanotags can be extended to complex tissues by working with tissue biopsies (thin tissue slices). Immunohistochemistry, a staining process, is typically used to evaluate the expression of various receptors within a biopsy. However, for each receptor, a different slice is needed, thereby increasing both the preparation and acquisition time. Since the nanotags are individualized to a specific type of receptor, they act as a pseudo-stain when the vibrational mode of interest is investigated. By assigning each vibrational mode, and therefore nanotag, a different colour, a false-colour map is generated showing the distribution of the receptors within the tissue sample. As the tissue slice can be exposed to all the nanotags simultaneously, only one staining step is needed, and so long as the spectral range of vibrational modes meets the requirements of the instrument, all the SERS spectra can be recorded in a single mapping experiment. The outcome of this process is shown in Fig. 3 for three biopsies taken from different breast cancer patients. In the first biopsy, only the HER2 and EGFR nanotags were present (Fig. 3B). For biopsies 2 and 3, ER and PR, and HER2 and ER receptors were observed. Importantly, these results matched what was found using classical immunohistochemistry methods (Fig. 3C), where brown colours indicate the presence of the receptor and purple corresponds to the nuclei of the cells within the tissue slice. This process of using classical techniques in correlation with SERS is important in validating the SERS results.
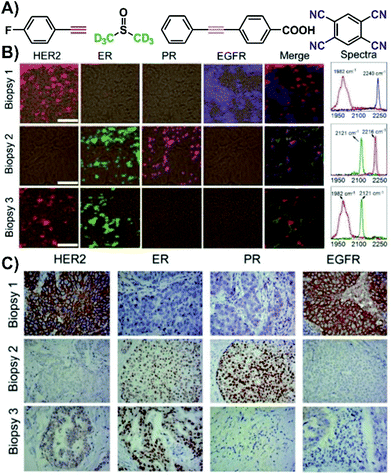 |
| Fig. 3 (A) Structures of the various Raman reporters. (B) Four-colour SERS mapping of three tissue biopsies exposed to various SERS nanotags with different Raman reporters for different targets (HER2 = human epidermal growth factor receptor 2, ER = estrogen receptor, PR = progesterone receptor, EGFR = epidermal growth factor receptor). SERS spectra from the maps indicating the presence of vibrational modes in the biologically silent window. Scale bar = 50 μm. (C) Expression of HER2, ER, PR, and EGFR in the biopsies measured by immunohistochemistry (brown = stained biomarker, purple = nucleus). Adapted from ref. 52 with permission from The Royal Society of Chemistry. | |
3.2 Non-targeted delivery of nanoparticles for tissue studies
Unlike cell studies where nanotags are predominantly used, complex tissue studies involving thin slices can also benefit from other approaches. As opposed to having to prepare the nanotags, simple colloidal nanoparticles can be drop-casted directly onto the tissue and allowed to dry. As the nanoparticles lack a means of targeting a specific membrane-bound biomarker, a SERS-active coating will form at the surface of the tissue (Fig. 4A).70 This non-specific approach is most adapted for differentiating between normal and diseased tissue, such as cancer. Tissue samples from liver,70,71 breast,72 thyroid,73 esophageal,74 nasopharyngeal,75 and brain76 cancers have been studied using this method. To account for sample-to-sample variability, it is necessary to have multiple tissue slices from both normal and diseased states. The difference spectrum obtained by subtracting the average SERS spectra of the diseased and normal tissue provides insight into how the composition of the tissue varies between the states (Fig. 4B).70 Based on tentative assignments of these peaks, the variations can be attributed to the influence of the metabolic rate changing the biochemical composition of the tissue. By incorporating multivariate analyses, it can become possible to predict if an acquired SERS spectrum comes from a normal or diseased sample.18 In addition to studying animal tissues, nanoparticles can be easily drop-cased onto plant-based tissues, such as leaves.77,78 Since some of the nanoparticles will also enter into the tissue, this approach allows for probing molecules (i.e. pesticides) both at the surface of and inside of leaves. As opposed to drop-casting the nanoparticles, it is also possible to immerse fixed tissue in a solution of the nanoparticles.79 This method is favourable for larger and complex tissue samples where having an electrostatic interaction-based distribution of nanoparticles is beneficial. This was demonstrated for fixed brain tissue slices that were immersed in a solution of black phosphorus–gold nanosheets (Fig. 4C–E). The SERS spectra of Fig. 4C collected from four encephalic regions (CTX, cerebral cortex; HYP, hypothalamus; TH, thalamus; Hi, hippocampus) showed that the cerebral cortex and thalamus had the strongest intensity, with the hippocampus and hypothalamus having weaker signals. This variation was attributed to difference in the density of cells within these regions and different affinities for the local biochemicals to the nanosheets. The different hemispheres showed some variation (Fig. 4D and E), but nowhere near as dramatic as the differences seen with normal and diseased tissue (Fig. 4B). These minor differences can be attributed to variation in the local biochemical environments.
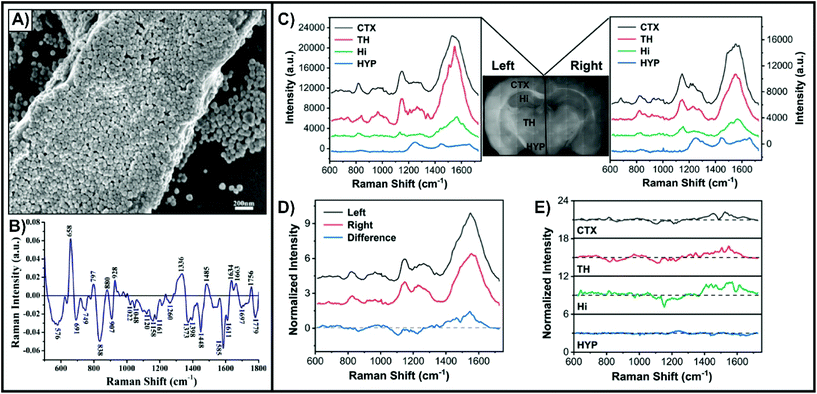 |
| Fig. 4 (A) SEM image of a liver tissue slice covered with silver nanoparticles. (B) Difference spectrum of the mean SERS spectrum of cancerous liver tissue (n = 56) and the mean SERS spectrum of normal liver tissue (n = 46). Reprinted from ref. 70 with permission from Elsevier. Mean SERS spectra extracted from four encephalic regions in the left and right hemisphere for a fixed tissue slice coated with black phosphorus–gold nanosheets. (D) Mean SERS spectra acquired in the left and right hemispheres and the corresponding difference spectrum (left–right). (E) SERS difference spectra of the four encephalic regions (left–right). Reprinted from ref. 79, Creative Commons License (https://creativecommons.org/licenses/by/4.0/legalcode). | |
Instead of coating the surface of the tissue with the nanoparticles, it is also possible to place the tissue onto a SERS-active surface. Such surfaces have two main requirements: (i) to be larger than the size of the tissue that is to be placed onto it, and (ii) exhibit as uniform enhancement as possible over the surface. Studies involving cells have used these types of surfaces, and have been prepared using Langmuir–Blodgett films of nanoparticles,80 and different lithographic techniques.81,82 Although these types of surfaces may be applicable to tissue, another fabrication method has been used. This is driven by the need for having coverage over a large surface area. Instead, glass substrates are initially coated with a thin layer of aluminum and boiled to form boehmite (Fig. 5A). Gold is then deposited onto the boehmite substrate to form structures described as gold nanocoral (Fig. 5B),83 or gold nanofève84 depending on the angle of the gold deposition. Mouse brain slices were taken from normal brains and ischemic brains, with ischemia caused by a unilateral middle cerebral artery occlusion. The occlusion allowed for one side of the brain to have the metabolism severely compromised with the other side being less affected. SERS spectra taken from the ischemic brain slices from the ischemic core and contralateral regions had variations in peaks at 518 and 736 cm−1 (Fig. 5C). By mapping the distributions of these peaks in both ischemic and normal brain slices (Fig. 5D), several key observations were made. In the normal tissue, only the peak at 518 cm−1 was observed. In the ischemic brain, the peak at 736 cm−1 was localized to the ischemic core and was more intense, while the 518 cm−1 peak was distributed throughout the remainder of the tissue. The origin of the peak 518 cm−1 was not ascertained, but it was known that adenine rings have a vibrational mode near 736 cm−1. As ischemia causes a breakdown of ATP to other purine degraded metabolites, SERS spectra of these metabolites were acquired to try and ascertain the origin of the 736 cm−1 (Fig. 5E). Of the tested metabolites, xanthine did not have a peak near 736 cm−1. As well, the sensitivity of the SERS substrate for the different metabolites varied (Fig. 5F). To better understand the distribution of the metabolites, quantitative imaging mass spectrometry was used (Fig. 5G). These images show that within the ischemic core, adenosine and inosine are present, and when coupled with the SERS measurements, shows that the detected xanthine in the ischemic core is hypoxanthine as it has a vibrational mode near 736 cm−1. Given the different sensitivities of these techniques, combining them provides a more complete understanding of metabolic processes for diseased tissue.
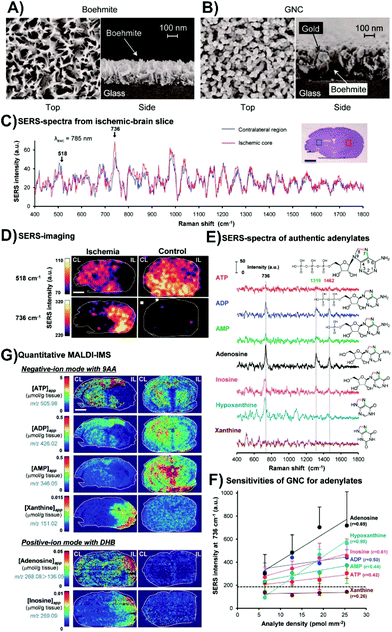 |
| Fig. 5 SEM images of (A) boehmite and (B) gold nanocoral (GNC). (C) Average SERS spectra from an ischemic core (red) and its contralateral region (blue) of an ischemic brain slice. (D) SERS maps of the bands at 518 and 736 cm−1 for ischemic and control brain slices. (E) SERS spectra of various adenylates. (F) Relative sensitivity of the GNC substrates to adenine related compounds. (G) Quantitative imaging mass spectrometry showing the spatial distribution of apparent tissue contents of various adenylates from tissue slices taken from the same animal as the SERS images of (D). The scale bars in the images is 2 mm. Adapted with permission from ACS Nano, 2014, 8, 5622–5632 (ref. 83). Copyright (2014) American Chemical Society. | |
Overall, coating the surface of a tissue biopsy with nanoparticles or adding the tissue to a plasmonic surface provides an excellent introduction to combining SERS with complex tissues. It demonstrates how tissue specific information can be obtained, without the optical interference from the background causing significant issues. However, it is limiting as it does not provide any chemical information about the inside of the tissue, and more importantly, is not applicable to animal studies as the nanoparticles or plasmonic surfaces are only added once the tissue has been excised.
4 Internalized nanotags and nanoprobes
Although the cellular membrane or tissue surface can provide interesting information that can be helpful in distinguishing the origin of a sample (i.e. cancerous vs. non-cancerous), as the SERS enhancement is limited to only a few nanometers above the metal surface, no information about the internal chemical environment is obtained. In order to evaluate the intracellular environment, the nanoparticles must be uptaken by the cells. This is easier in studies involving single or small group of cells, whereas tissue studies often require that the nanoparticles be injected into an animal and allowed to accumulate within the tissue. We have used the term nanotags to describe nanoparticles that are designed to image the distribution of specific receptors. On the other hand, nanoprobes provide insight into the local chemical environment or specific analytes within the biological system. Where a nanotag is designed to have a constant SERS response, a nanoprobe will have a varying SERS signal (gain, loss, or change) upon being internalized and exposed to the analyte or condition of interest.
4.1 Nanotags and nanoprobes internalized in cells
Even though this approach is the workhorse method, the mechanisms by which functionalized nanoparticles (i.e. nanotags and nanoprobes) enter the cell are still being evaluated.85 Nanoparticles are uptaken by cells via different mechanisms including phagocytosis, micropinocytosis, clathrin- and caveolae-dependent and clathrin- and caveolae-independent endocytosis.86 Geometric parameters including the shape87,88 and size89 of the nanoparticles will influence the internalization, as will the presence of targeted antibodies,90 and experimental parameters such as incubation time and nanoparticle concentration.91,92 Owing to the shape of the cells, 3D SERS measurements can provide greater information about the distribution of the nanoparticles within the cell.93
It is important to remember that even though nanoparticles functionalized with a self-assembled monolayer can enter cells,94 the formation of a protein corona around the nanoparticles will influence its functionality.95 Given the popularity of this approach, there is a wide range of studies that have been performed. Probing organelles, notably the nucleus96–98 and mitochondria,97,99–101 pH,99,102–105 and small molecules106–110 are common studies. Detecting other biomolecules,111 such as peptides,112 proteins,105,113–115 and miRNA116,117 is also popular. With such a wide range of applications, the sensing protocols (or variations of them) shown in Scheme 1 can be readily implemented.
As an example, spherical gold nanoparticles can be internalized by cells by endocytosis, and as a result be a non-specific nanoprobe. This approach was used to examine the effect of infecting live macrophage cells with Leishmania-mexicana.118 By performing SERS mapping measurements of the cells, representative SERS spectra could be collected (Fig. 6). Unsurprisingly, the spectra are complex and variable. Table 1 of ref. 118 provides tentative assignments for the various vibrational modes, with the assignments typically corresponding to either lipids or proteins. The use of a generalized approach is beneficial in its simplicity but challenging in the interpretation of the results. Given the variability in the spectra, careful analyses of the results are critical.
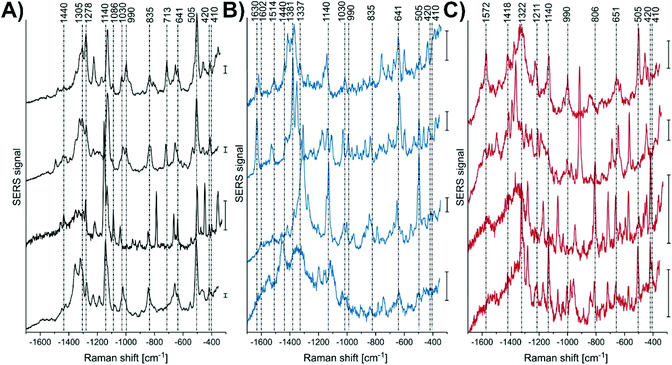 |
| Fig. 6 Representative SERS spectra, extracted from the mapping data sets of several L. mexicana-infected primary macrophage cells from the areas of (A) parasite, (B) the parasitophorous vacuole, and (C) endolysosomes located in the cytoplasmic regions. Scale bar = 50 cps. Adapted with permission from Anal. Chem., 2018, 90, 8154–8161 (ref. 118). Copyright (2018) American Chemical Society. | |
Alternatively, more nuanced nanoprobes can be prepared. Much like nanotags where the presence of a SERS signal indicates that the receptor is present, nanoprobes with a SERS “on” to SERS “off” or vice versa sensing scheme have a similar approach. The dramatic change in the observed spectra indicates the detection of the target analyte. However, verifying the complete preparation of the nanoprobe and validating the sensing scheme using a simple on–off approach may not be ideal. Instead, an on–off–on scheme has been developed and applied to the detection of a target viral RNA strand (Fig. 7A).119 In this method, gold nanostars were functionalized with thiolated PEG and a specific DNA strand that was chemically modified to have a cyanine-3 (Cy3) molecule to act as a SERS beacon. Under non-hybridized conditions, the Cy3 molecule was close enough to the surface of the nanostar so that a SERS spectrum could be recorded. Upon introducing the complementary strand of DNA, the conformation of the beacon DNA changed, and the Cy3 SERS spectrum was lost. Once a strand of RNA was introduced, one of two things happened. If the strand of RNA was the target strand, the previously added complementary strand hybridized with the RNA strand, resulting in the beacon DNA reverting to its hairpin geometry and a return of the Cy3 SERS spectrum. If a random RNA sequence was present, no SERS spectrum was observed. This approach was verified using HeLa cells transfected with a plasmid that would generate the desired target RNA strand as well as using various other controls (no transfection and transfected with different plasmid). Only with the HeLa cells transfected with the correct plasmid exhibited a return of the SERS spectrum (Fig. 7B).
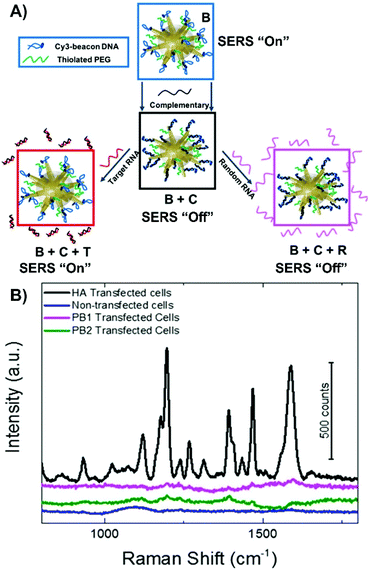 |
| Fig. 7 (A) Fabrication of SERS-active nanostar probes functionalized with cyanine3-beacon DNA (B) that upon introduction of the complementary strand (C) causes the cyanine-3 to be displaced from the surface resulting in a loss in the SERS signal. The signal can be gained by introducing the target viral RNA (T) or remained loss if random viral RNA (R) is present. (B) SERS signals measured from the nanoprobes incubated in HeLa cells transfected with hemagglutinin (HA) coding plasmid, transfected with two control plasmids (PB1 and PB2), and non-transfected HeLa cells. Adapted with permission from J. Phys. Chem. C, 2020, 124, 3211–3217 (ref. 119). Copyright (2020) American Chemical Society. | |
4.2 Nanotags and nanoprobes within tissue
Depending on the type of tissue of sample and the purpose of the experiment, nanoparticles can be incorporated into the tissue in the same way as cells can. As a result, there is a great deal of cross-over between cell and tissue experiments involving nanotags and nanoprobes within tissue. When working with excised tissue, incubating the tissue with the nanoparticle solution can be used to introduce the nanoparticles into the tissue. Depending on the nanoprobe, events such as hypoxia120 and hypoxia-induced acidification121 can be evaluated. It is also possible to synthesize the nanoparticles within the tissue and use the formation of an aggregate to collect SERS spectra of various analytes.122 Aggregating nanoparticles in solution can not only increase the electromagnetic enhancement as smaller junctions between adjacent nanoparticles are formed, target analytes can become trapped within the aggregation, leading to a stronger and more stable SERS response. The aggregation of amyloid beta (Aβ) in the brain has been related to the development of neurodegenerative diseases.123,124 In solution, upon the introduction of the monomer or the fibril to an aqueous solution containing the gold precursor followed by the introduction of ascorbic acid as a reducing agent,122 different nanostructures were observed. With Aβ monomer, nanospheres were formed whereas nanofibrils resulted in the formation of nanowalnuts. The acquired SERS spectra of the monomers and fibrils were distinct from each other (Fig. 8A–D), and more importantly, were different from other biomolecules tested that also formed aggregates (Fig. 8E). Specifically, distinct vibrational modes at 1268 and 1244 cm−1 were used to differentiate Aβ40 monomers from Aβ40 fibrils respectively. To induce the formation of Aβ aggregates in brain tissue, mice were fed different diets. Upon excising brain tissue slices and exposing them to the gold precursor and the reducing agent, nanoparticles formed within the hippocampus (Fig. 8F–J). In a zinc rich diet, the Aβ40 monomer was present in greater quantities than the Aβ40 fibrils as shown by the stronger peak at 1568 cm−1 in Fig. 8Jiii. Given that this method prepares label-free nanoprobes, this could be an interesting approach for detecting other types of biomolecules that are present within tissue and ascertaining their distribution within the sample.
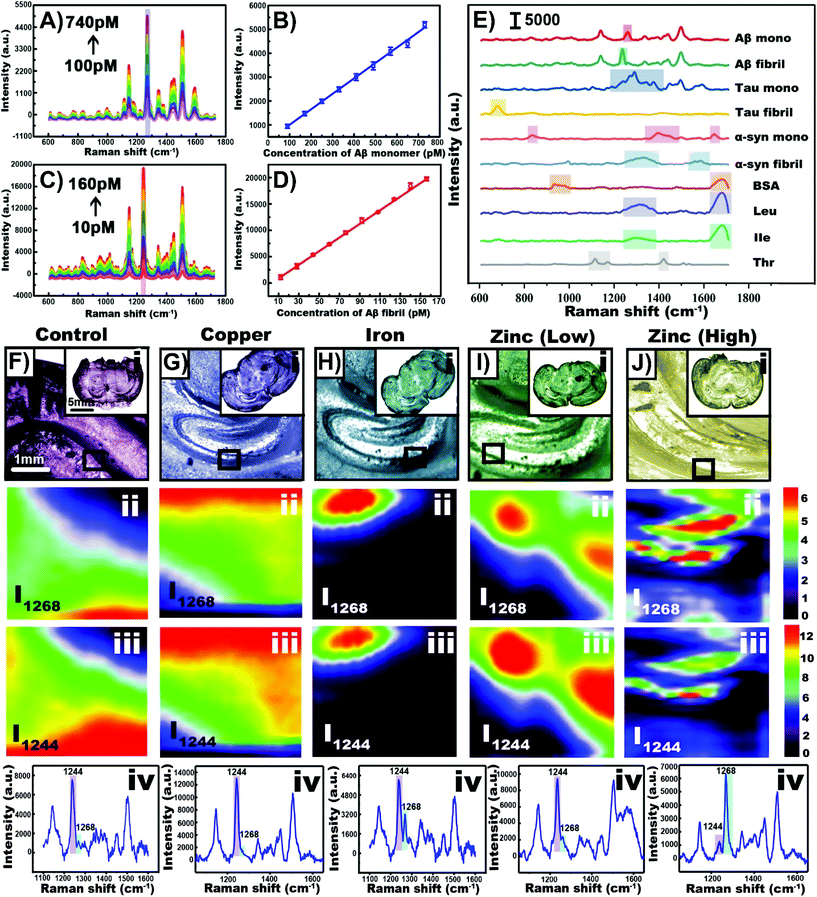 |
| Fig. 8 (A) SERS spectra of gold nanoparticles synthesized with Aβ40 monomer with concentrations ranging from 100 to 740 pM. (B) Plot of SERS intensity at 1268 cm−1 with varying monomer concentration. (C) SERS spectra of gold nanowalnuts synthesized with Aβ40 fibral with concentrations ranging from 10 to 160 pM. (D) Plot of SERS intensity at 1244 cm−1 with varying fibral concentration. (E) SERS spectra obtained using various biomolecules and the template method. (F–J) Results obtained for mice given different diets. (i) Bright-field images of tissue slices obtained from the treated transgenic mice. SERS imaging of Aβ40 in the hippocampus in the tissue slices at (ii) 1268 cm−1 and (iii) 1244 cm−1. (iv) SERS spectra of Aβ40 in the hippocampus. Adapted with permission from Anal. Chem., 2020, 92, 5910–5920 (ref. 122). Copyright (2020) American Chemical Society. | |
Alternatively, as opposed to incubating the tissue slice, nanoparticles can be injected directly into the tissue.125,126 This can be used as an early step before moving onto in vivo injections as was done to probe the metabolic composition of tumor microenvironments.126 The use of intravenous injections is the most diagnostically relevant approach, as once injected, SERS nanoparticles, and more commonly nanotags, will distribute within different types of tissue, such as tumors. This process has helped to demonstrate the potential of Raman spectroscopy as a tool during for intra-operative margin analysis used to determine the location of tumors.127 When performing these types of measurements, it is important to recognize how the nanoparticles will distribute throughout the animal. For Raman reporter functionalized nanoparticles coated with a silica shell, in the absence of any means of targeted delivery, the nanoparticles accumulated in the liver, spleen, and gallbladder of mice (Fig. 9A).128 The accumulation of the nanoparticles in these organs is related to the high phagocytic activity within the reticuloendothelial system. However, in cancerous tissue, the phagocytic activity is lower. This results in fewer nanoparticles being present (Fig. 9B). As a result, when livers containing a series of tumors were imaged (Fig. 9C–H), it was possible to differentiate between normal and cancerous tissue as the normal tissue exhibited a SERS response of the Raman reporter due to the accumulation of the nanoparticles. Alternatively, when nanotags are used, such that they are delivered to the tumors that contain cells expressing a specific membrane receptor, the presence of a SERS signal is used to identify the location of the tumor as opposed to a significantly decreased signal. As was discussed when mentioning the use of nanotags with cells, by using different molecules, different types of tumors can be targeted for intra-operative experiments. This has been demonstrated for prostate,129,130 bladder,129–131 and brain132 tumors. As opposed to targeting the tumor or relying on varying uptake between tissue types, pH sensitive nanoprobes that will enter both cancerous and normal tissue can be used to delineate a tumor margin.133 This is possible because the pH in tumor extracellular microenvironments is acidified.134 For brain tissue, it is necessary to overcome the blood brain barrier, and this was achieved using angiopep2 peptides adsorbed onto the nanoprobes.
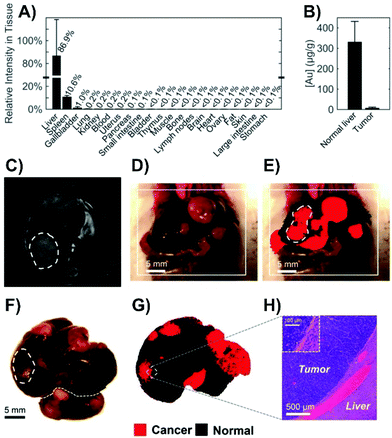 |
| Fig. 9 (A) SERS intensity of spectra collected from tissue homogenates of healthy mice (n = 2) 18 hours after intravenous administration of silica-coated, trans-1,2-bis(4-pyridyl)ethylene functionalized gold nanoparticles. (B) Neutron activation analyses of healthy liver and tumor tissue from Myc-driven HCC mice (n = 3) quantifying the gold content related to the core of the nanoparticles. (C) MR image through the liver showing hyperintense lesions corresponding to the tumors (one is outlined with a dashed line). (D) Photograph showing the liver with several tumors. (E) SERS image produced using direct classical least-squares overlaid on (D) showing areas of cancerous (red) and normal (black) tissue. The outlined tumor corresponds to the location outlined in (C). (F) Photograph and (G) corresponding SERS image of the excised liver showing multiple liver tumors. (H) Histology with hematoxylin and eosin staining confirming the precise delineation of the liver tumor margin obtained by SERS imaging. Adapted with permission from ACS Nano, 2016, 10, 5015–5026 (ref. 128). Copyright (2016) American Chemical Society. | |
The development of nanotags and nanoprobes for use in cells and tissue requires a long list of conditions to be considered. These range from minimizing aggregation (except when aggregation is the point) to more complex issues like biocompatibility. In the following section, we discuss the third approach of Scheme 1, the use of inserted nanosensors.
5 Inserted nanosensors
In the context of this review, nanosensors are the combination of a plasmonic structure on a substrate that is capable of being inserted into cells and/or tissue. Unlike the previous section where it was necessary to have the nanoprobes and nanotags uptaken by the cell/tissue for targeted delivery, nanosensors simply need to be inserted into specific domains of the cell or tissue. This is achieved using a micro-positioning system, such as a micromanipulator or a piezoelectric positioner typically used for AFM experiments. This method has started to have an increase in popularity and is therefore described in greater detail than the other sections.
5.1 Fabrication of nanosensors
Using methods developed for other techniques, such as electrophysiology, the most common approach involves the use of glass rods or capillaries with a laser puller.135–147 When the rod or capillary is heated and pulled, the resulting nanofibers have a pipette-like geometry, and by tuning the parameters, it is possible to vary the tip diameter. Other types of substrates that have been used include AFM tips,148 optical fibers,149,150 and acupuncture needles.137,151–157 Owing to the dimensions of these different substrates, it is necessary to point out that unlike the nanofibers, some of these substrates are better suited for either cells or tissue. For example, the small size of the AFM probe may make it difficult to probe depths in tissue, and the wide tip diameter of an acupuncture needle is less suitable for piercing cells than it is for tissue.
The means by which SERS compatibility is introduced is often influenced by the desired sensing scheme. All the sensing schemes shown in Scheme 1 can and have been applied using nanosensors inserted into cells and tissue. The simplest approach to preparing SERS compatible nanosensors is to create a roughened metallic surface or a surface composed of metallic nanoislands (Fig. 10A).144 Even though roughened silver surfaces were used in the earliest studies of SERS,158–160 these types of surfaces are still being prepared and evaluated for plasmonic activity.161–164 An alternative approach is to adsorb colloidal nanoparticles onto the surface via electrostatic interactions. Although this is commonly done using aminosilane chemistry, a different approach involving a block copolymer of polystyrene and poly(4-vinylpyridine) has been used to great success as well (Fig. 10B).145 By tuning the synthesis of the nanoparticles (shape, size, material, capping ligand) it is possible to tune the plasmonic response of the structure and facilitate the necessary electrostatic interactions. Other molecular interactions can also be used to anchor nanoparticles to a nanofiber, to create a nanosensor with a “sandwich” geometry. As an example, a nanofiber is first coated with a thin gold film. A DNA aptamer is then functionalized onto the metal film, and the complementary DNA sequence functionalized onto a metallic nanofiber. Through molecular interactions between the DNA sequences, the nanoparticle becomes tethered to the nanofiber (Fig. 10C).139 As a result of these interactions, the nanosensors can be described as “hybridized” nanosensors. A final methodology involves the use of nanofabrication to create grooves in the substrate. It has been demonstrated that nanoscale materials can become trapped within nanowells,165 where the relative size ratio of the structures is important for influencing the number of the nanoparticles within the well. By fabricating huge grooves into the substrate (10 μm × 10 μm × 1 μm) relative to the size of the nanoparticle (∼55 nm), it is possible to introduce well-defined SERS regions on the substrate with high densities of nanoparticles (Fig. 10D).156 Due to the size of the grooves, this approach requires fibers with larger diameters (10's of μm's), and is therefore best suited for acupuncture needles as opposed to pulled nanofibers. There are of course other approaches that can be used to generate highly localized sensing regions. For example, a carbon nanotube decorated with gold nanoparticles can be added to the end of a pulled glass capillary.166 As the diameter of the carbon nanotube is considerably smaller than that of the capillary, far less damage is done to cells when it is inserted.
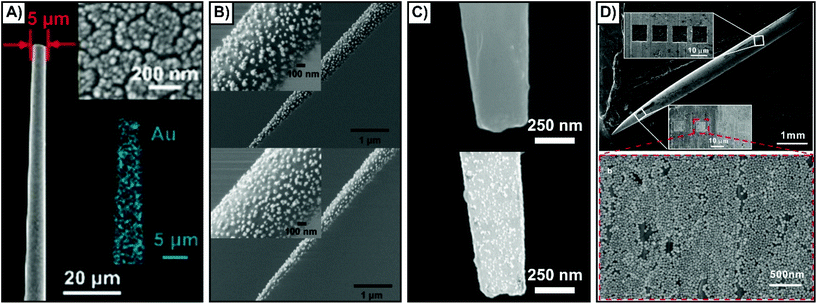 |
| Fig. 10 SEM images of nanosensors fabricated by different methods. (A) Magnetron sputtering of a thin gold film resulting in the formation of nanoislands. Reprinted with permission from ref. 144. Copyright 2019, Wiley-VCH. (B) Gold nanoraspberries (top) and gold nanostars (bottom) deposited onto a nanofiber coated with a poly(styrene-b-4-vinylpyridine) copolymer brush layer. Adapted with permission from ACS Sens., 2020, 5, 2155–2167 (ref. 145) Copyright (2020) American Chemical Society. (C) Aptamer functionalized gold-coated nanofiber (top) that is subsequently coated with silver nanoparticles functionalized with complementary DNA. Adapted with permission from Anal. Chem., 2017, 89, 9911–9917 (ref. 139) Copyright (2017) American Chemical Society. (D) Silver acupuncture needle with a series of 10 μm × 10 μm × 1 μm grooves milled into it using a focused ion beam (top). The nanoparticles then accumulated within those grooves (bottom). Adapted with permission from ACS Appl. Nano Mater., 2019, 2, 2752–2757 (ref. 156) Copyright (2019) American Chemical Society. | |
5.2 Use of SERS nanosensors with cells
One of the advantages of working with nanosensors and a micro-positioning system is the ability to perform measurements outside of the cell at defined positions and relative heights. This allows for the nanosensors to evaluate biological process such as secretion events,138,141,146 as well as to explore metabolite and pH gradients.142,145 These types of measurements can be used to evaluate the state of the cell both before and after inserting the nanosensor into the cell.
Interestingly, the SERS sensing scheme shown in Scheme 1i was used in one of the first examples of an inserted nanosensor in a cell.135 In this work, poly-L-lysine coated nanofibers were used to adsorb gold nanoparticles in the preparation of the SERS nanosensors. Aided with the use of a micromanipulator, the nanosensor was inserted into different domains of the cells, with subsequent SERS spectra collected with the nanosensor still inserted (Fig. 11A). The complex SERS spectra reflect the varying biochemical composition of the cytoplasm and the nucleus. Owing to the long acquisition time (10 to 20 seconds), the spectra represent an average response of molecules that have diffused towards and adsorbed onto the metal surface, as well as the orientations of these molecules. As expected, the cytoplasm lacked bands associated with DNA (660 and 722 cm−1). By increasing the concentration of KCl in the cell media to 55 mM, it was possible to depolarize the cell membrane, and therefore probe changes to the chemical composition of the cytoplasm (right side of Fig. 11A). By advancing the fabrication of the nanosensor so that a narrower tip was present, it was later possible to interrogate the cell in a less invasive manner.166 Using a nanosensor composed of a nanopipette with self-assembled nanoparticles at the tip, it was possible to measure intracellular glutathione as the thiol will form a Au–S bond.167 This required leaving the nanosensor in the cell for 10 minutes, with the SERS measurements taken after the nanosensor was removed from the cell.
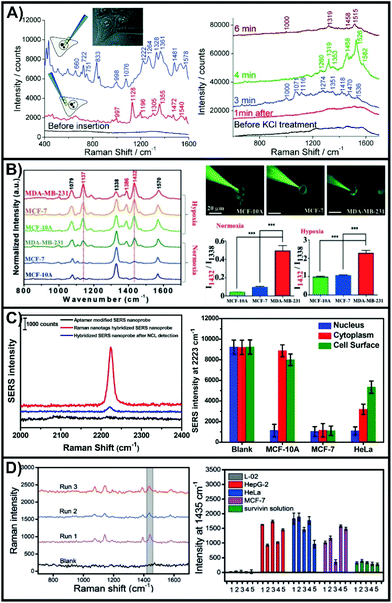 |
| Fig. 11 (A) SERS spectra obtained with a nanosensor inserted in the nucleus (upper left spectrum) and cytoplasm (lower left spectrum) of a HeLa cell. SERS spectra obtained of a nanosensor inserted in the cytoplasm after exposure to 55 mM KCl after different times. Adapted with permission from ACS Nano, 2009, 3, 3529–3536 (ref. 135). Copyright (2009) American Chemical Society. (B) SERS spectra of 4-NTP functionalized nanosensor inserted into three different cell lines under normoxia and hypoxia incubation conditions. Images of the nanosensors being inserted into the different types of cells and the quantitative analysis of the intensity ratio of I1432/I1338. Reprinted with permission from ref. 143. Copyright 2019, Wiley-VCH. (C) SERS spectra of a hybridized nanosensor before and after addition of nucleolin. SERS intensities at different 2223 cm−1 for specific domains of different cell lines. Adapted with permission from Anal. Chem., 2017, 89, 9911–9917 (ref. 139) Copyright (2017) American Chemical Society. (D) SERS spectra of hybridized nanosensors after having injected 100 fL of a 1.0 × 10−10 M survivin solution. Comparison of the SERS intensity 1435 cm−1 for hybridized nanosensors after having been inserted in different cell lines or exposed to a survivin solution (5 μL of 10 pg mL−1). Reprinted with permission from ref. 137. Copyright 2016, Wiley-VCH. | |
To better understand the local physiological conditions within the cells, the surface of the nanosensors can be functionalized with different SERS reporter molecules, as was the case with nanoprobes. In pH sensing, 4-mercaptobenzoic acid (4-MBA),144,145 and 4-mercaptopyridine150 are most commonly used, though DNA has also been used.168 Though not an aromatic thiol, 1-(4-aminophenyl)-2,2,2-trifluoroethanone will adsorb onto gold surfaces and can be used to detect carbonate.144 Due to interactions involving the cyanide group, 4-mercaptobenzonitrile (4-MBN) is sensitive to free Fe3+ and oxyhemoglobin.140 The conversion of adjacent 4-nitrothiophenol (4-NTP) molecules to 4,4-dimercaptoazobenzene (4,4-DMAB) can be driven under laser illumination, and is a quintessential example of a plasmon-mediated photocatalytic reaction.169–173 When performed in cells, this reaction is sensitive to the local reductive conditions.143 The rapid growth of cancer cells is known to produce hypoxic (low oxygen) environments in tumor microenvironments requiring the cancer cells to alter their metabolism. To simulate this effect, three cell lines (MCF-10A (non-cancerous); MCF-7 (weakly metastatic); MDA-MB-231 (aggressive)) were grown under normal and hypoxic conditions. The conversion of 4-NTP to 4,4-DMAB is characterized by the presence of new bands at 1137, 1386, and 1432 cm−1. The SERS spectra of Fig. 11B show that under normal oxygen conditions, only the MDA-MB-231 cells exhibited an internal reductive environment capable of aiding the conversion of 4-NTP on the inserted nanosensors. Under hypoxic conditions, all three cell lines exhibited increased conversion, with MDA-MB-231 once again having the greatest conversion. This demonstrates that using a SERS nanosensor to evaluate the local chemical environment within the cytosol can be used to differentiate general cell types.
There are two critical steps to the design of hybridized nanosensors. First and foremost is the selection of the target analyte as this will influence the choice of binding molecule, and therefore the sensing scheme. The second step is the same as to what was mentioned earlier in this review, the selection of an appropriate SERS reporter molecule. Here, we highlight two examples that detect two different molecules, use opposite sensing schemes, and different SERS reporter molecules. Nucleolin plays a role in cell division and is found throughout the cell,174,175 with cancer cells having altered sub-cellular expression.176 Due to its relevance, a DNA aptamer (AS1411) has been developed that is capable of binding to nucleolin. By working with a thiolated version of the aptamer, it will bind to the gold coated nanopipette.139 Silver nanoparticles were used to introduce SERS compatibility, with the surface of the nanoparticles functionalized with the complementary DNA strand and 4-MBN. The C–N stretching mode of 4-MBN at 2223 cm−1 was used to evaluate the detection of nucleolin. When the DNA strands interacted, a strong SERS response was observed (Fig. 11C), that was subsequently diminished when nucleolin was introduced. Three cell lines (MCF-10A, MCF-7, HeLa) and three domains within the cells were compared. The nanosensors were inserted into the cells, and left for 5 minutes before being removed, rinsed, dried, and having the SERS measurements taken. In all cases, nucleolin was observed in the nucleus as noted by the decrease in SERS intensity. Both the cytoplasm and cell surface of MCF-7 cells exhibited a comparable intensity to that of the nucleus, whereas only the cytoplasm did with the HeLa cells, though the intensity was still lower at the cell surface than in the blank (∼47%). As opposed to working with an aptamer, monoclonal antibodies or synthetic antibodies produced by molecular imprinting can be adsorbed onto a gold coated nanofiber and used to collect the target analyte. These methods were demonstrated for survivin and alkaline phosphatase respectively.137 Silver nanoparticles were prepared with a secondary antibody (survivin) or a boronic acid (alkaline phosphatase) as well as a SERS reporter (4-aminothiophenol and 4-mercaptophenylboronic acid respectively). The SERS results for survivin are shown in Fig. 11D. As these are found in cancer cells, but are low expression proteins, initial tests were performed by injecting minute quantities into normal hepatic cells (L-02). After ∼6 survivin molecules were injected, the antibody coated nanofiber was inserted into the cell and left for 3 minutes, before moving on to the subsequent steps. These steps including rinsing the nanofiber and adding the functionalized nanoparticles. The SERS spectra exhibited the spectra of 4-aminothiophenol. Using the intensity of the 1435 cm−1 peak, a comparison was made between normal L-02 cells (no injected survivin) and a series of cancerous cells. The SERS measurements for nanosensors inserted in the cancerous cells had the 1435 cm−1, but not the L-02 cell, indicating that survivin was present in the cancerous cells. The results also highlight an important aspect of working with biological samples. Within the same cell line, there was a considerable cell-to-cell variation due to cell microheterogeneity. This can be accounted for by performing measurements on large quantities of cells.
5.3 Use of SERS nanosensors in tissue
As was discussed in section 2, the optical interference from the tissue introduces new sets of challenges. Unlike inserted nanotags and nanoprobes where the nanoparticles must remain in the tissue during the SERS measurements, nanosensors can either be kept in or removed from the tissue for SERS measurements.
More so than in the previous sections, transitioning to tissue with an inserted nanosensor often will start with the use of a tissue mimic. When detecting analytes within a mimic, ensuring that the analyte is evenly distributed throughout the sample is important, otherwise the measurements will be based on gradients.151 It is very important to recognize that the optical properties of agar and agarose gel may not reflect those of the tissue. Therefore, if the goal is to keep the nanosensor in the tissue during the SERS measurement, how the tissue background influences the measurements is unlikely to be considered. By adding different absorbers and scatters into the sample, such as nigrosin and intralipid-20%, it is possible to better mimic tissue samples, like human skin.177 This mimic could be prepared with known concentrations of glucose (0–140 mM), and by inserting an array of microneedles coated with silver, it was shown to be possible to detect the intrinsic SERS spectrum of glucose. However, agar does provide a good means of evaluating a proposed experimental methodology and sensing scheme prior to moving on to tissue samples and animals. In one study that used this combined approach,152 a tailored nanosensor was inserted into agarose gels spiked with either glucose, fructose, sucrose, or a combination of them. An acupuncture needle was decorated with gold nanoshells functionalized with 4-MBA and subsequently given a microporous polystyrene coating and exposed to glucose oxidase. As opposed to directly detecting glucose, the change in pH that occurs when glucose oxidase converted the diffused glucose to gluconic acid was monitored using changes in the SERS spectrum of 4-MBA (Fig. 12A and B). To facilitate the oxidation reaction, the nanosensor had to be removed from the sample. Only after having evaluated this method did the authors move to an animal model. Here, two nanosensors were inserted into the vastus lateralis tendon and an ear vein of a rabbit. After injecting the rabbit with a glucose solution, two other sensors were inserted into the same locations and a blood sample collected. The ratio of the SERS intensities (Fig. 12C) changed upon the introduction of glucose and agreed with the results of a commercial blood glucose test kit that showed an increase in blood glucose concentration after injection.
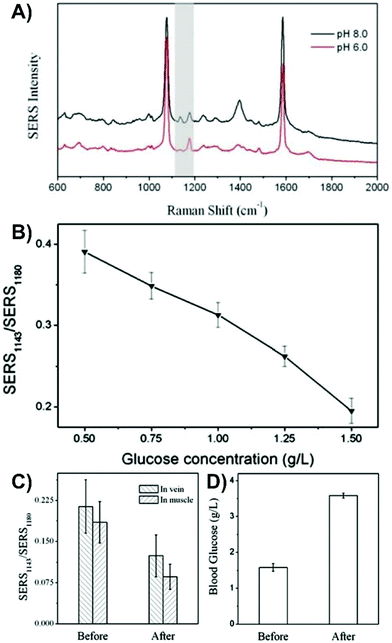 |
| Fig. 12 (A) SERS spectra of 4-MBA on a SERS nanosensor at pH 8.0 and 6.0. The grey highlighted region indicates the peaks (1143 and 1180 cm−1) used to evaluate the influence of pH. (B) Influence of glucose concentration on the ratio of the SERS intensities of the pH responsive vibrational modes. (C) Ratio for the intensity of SERS1143/SERS1180 before and after injection of glucose into a rabbit. (D) Blood glucose concentration of the rabbit before and after glucose injection using a commercial glucose test kit. Adapted from ref. 152 with the permission of the Royal Society of Chemistry. | |
If the desired approach is to insert the nanosensor into the tissue and then remove it for the SERS measurements, it is not always required to use a mimic, though it is helpful in verifying that the proposed method will work. It is also advisable that the analyte have a strong affinity for the nanosensor such that it is not removed after the nanosensor is taken out of the sample. In this regard, sandwich assays can be helpful as in order to introduce a SERS signal, the nanosensor must be removed anyway to add the nanoparticles. Many studies involving glucose and fructose have used boronic acids as they form cyclic boronate esters with 1,2- and 1,3-diols.178–181 By incorporating boronic acids into the nanosensor design, this method was used to detect glucose at different depths within an apple,153 with the highest concentration of the sugars being present in the pulp as opposed to the core or peel. Instead of using a capture-based method, it is also possible to rely on the affinity of the analyte with the metal surface. 6-mercaptopurine is used in the treatment of certain cancers and autoimmune diseases, and as it contains a thiol, will bind with gold nanoparticles. Although the authors had tested the nanosensors in agarose gel containing a dye to evaluate the compatibility of their approach, but not 6-mercaptopurine.151 Instead, anticoagulated blood was used. After injecting 6-mercaptopurine into a rabbit, a stronger SERS intensity was observed for a nanosensor inserted into a vein as opposed to a tendon. In addition to monitoring drug distribution with an animal, this approach can also be applied to monitoring contamination of wildlife. Malachite green, sometimes used as a biocide for fungal infections, is known to be carcinogenic and genotoxic.182 In the context of food safety, detection of malachite green within an animal, without causing harm to the animal is ideal.156 To investigate this, the fish were incubated in a 10−6 M solution of malachite green for 60 minutes and rinsed thoroughly to remove malachite green from the surface of the fish that could otherwise contaminate the nanosensor. A nanosensor was subsequently inserted into the fish (Fig. 13A) and left for 10 minutes before being removed, rinsed, and placed under a Raman microscope (Fig. 13B). Spectra were collected every 2 mm along the length of the nanosensor (Fig. 13C–E), where the spectra of malachite green were easily observed, with the highest intensity observed at 14 mm inside the fish (Fig. 13D).
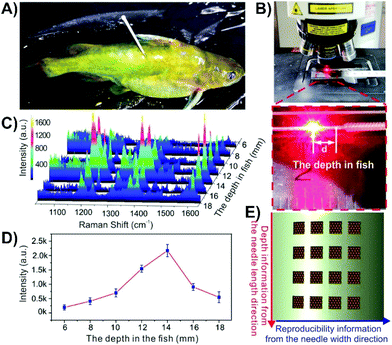 |
| Fig. 13 Photos of (A) a malachite green treated fish with a nanosensor composed of a grooved silver acupuncture needle with gold nanoparticles inserted into it, and (B) acquisition of the SERS signal from a nanosensor after having been inserted in the fish. (C) SERS spectra obtained at different depths in the fish. (D) Intensity distribution of the 1169 cm−1 band of malachite green at different depths in the fish. (E) Schematic representation of how the array of grooves is used to measure depth information and signal reproducibility. Adapted with permission from ACS Appl. Nano Mater., 2019, 2, 2752–2757 (ref. 156). Copyright (2019) American Chemical Society. | |
Another successful approach is to first evaluate a protocol with cell studies and then perform the same type of experiment with tissue. This step was taken for two of the highlighted studies involving cancer cells,137,143 with some measurements subsequently taken involving tumors on animals. To facilitate the transition, and to make it easier to insert the nanosensors, switching to an acupuncture needle as the substrate can be done.137 There is a strong desire to also understand the physiological environment of live, healthy tissue (as well as disease state tissue). Fundamentally, this requires that the nanosensor be within the tissue during the SERS measurements. As has been stated, this will mean that the tissue will cause optical interference. However, if this can be overcome, near-real-time information about physiological environments and processes can be obtained. This was demonstrated for middle cerebral artery occlusion in the cortex of the brains of live mice.144 Brain tissue is especially troublesome as it has strong optical scattering properties (Fig. 1B). The development of the nanosensor was done using cultured neurons. The nanosensor was functionalized with three molecules, 4-MBN, 1-(4-aminophenyl)-2,2,2-trifluoroethanone (AT), and 4-MBA. The intensity of the peak at 2227 cm−1 is from the 4-MBN and was used as an internal standard. The ring stretching mode of AT (1077 cm−1) decreases in relative intensity when carbonate binds to adjacent AT molecules through hydrogen bonding. As the pH increases, the COOH for 4-MBA is converted COO− and a band at 1395 cm−1 increases in relative intensity. This simple approach of functionalizing the metal surface of the nanosensor with three molecules provides multiplexing capabilities. The only difference in the nanosensor design from neurons to brain was increasing the tip diameter from 0.2 to 5 μm. For the brain experiments, 8 nanosensors were inserted into the cortex of a sedated mouse, with the mouse subsequently placed on the stage of the Raman microscope (Fig. 14A). SERS spectra from all 8 nanosensors could be recorded in less than one minute. To evaluate the near-real-time SERS response, a middle cerebral artery occlusion operation was performed just prior to inserting the nanosensors. Compared to the signals obtained with the control measurement (no operation), the relative intensity of I1077/I2227 increased and I1395/I2227 decreased (Fig. 14B and C), meaning a decrease in carbonate concentration and a decrease in pH. The change in pH was caused by a lack of O2 and ATP, leading to a build up of lactic acid. This caused a shift in the equilibrium towards bicarbonate instead of carbonate. The pH level and carbonate concentration reached equilibrium within 10 minutes (Fig. 14D). Upon reperfusion, the O2 and ATP levels were restored, yielding a return to the pH and carbonate concentrations of the control experiment.
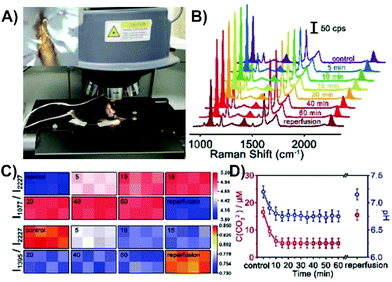 |
| Fig. 14 (A) Photo of a live mouse on a mobile platform under the SERS laser lens for detection with an inserted SERS probe. The inset is of a single probe inserted in the cortex under the SERS laser lens. (B) SERS spectra of a microscope in the cortex of a mouse brain upon middle cerebral artery occlusion and reperfusion. (C) Matrix diagrams of the SERS mapping of the ratiometric peak intensities of I1077/I2227 and I1395/I2227 obtained with a microarray consisting of eight microprobes in the cortex upon middle cerebral artery occlusion and reperfusion. (D) Variation of the CO32− concentration and pH value in the cortex upon middle cerebral artery occlusion and reperfusion (n = 5, S.D.). Reprinted with permission from ref. 144. Copyright 2019, Wiley-VCH. | |
It is not always feasible to work with a live animal under the Raman microscope, so instead, experiments can be performed using live tissue slices. Furthermore, none of the studies shown involve a scenario where the sensing scheme does not involve a surface-bound reporter molecule. This is a critical step in being able to study molecules secreted within the tissue. Due to our group's previous endeavours involving the SERS detection of neurotransmitters secreted from cultured neurons,141,146 we sought to make the transition to live brain tissue slices. Interestingly, the transition from cells to tissue appears to have skipped tissue slices and instead gone directly to tissue still within the animal. As such, we have developed a blueprint that provides various several methods for overcoming challenges associated with making this transition.147
The first issue that was addressed was how to position and insert the nanosensor into the tissue. As the Raman microscope is equipped with a piezoelectric positioner (normally used to control an AFM tip), this was initially evaluated (Fig. 15A). In the current configuration, there is some concern about the vertical insertion of the nanosensor causing excessive damage to the tissue. To overcome this, a home-made Raman stage was built with an attached micromanipulator (Fig. 15B). To have the best possible control over the position of the nanosensor relative to the tissue and the laser beam, both the fluidic cell and micromanipulator are mounted on the same stage. The greatest challenge is how to focus on the sensor once it is inserted at the target depth (Fig. 15C). It is difficult to visualize the narrow nanosensor (derived from a laser-pulled glass rod) at this depth, and therefore difficult to focus on the nanosensor. To ensure that the laser beam is focused on the nanosensor we explored some creative options. Functionalizing the surface of the nanosensors with a SERS active molecule was viewed to be impractical. With the goal being to continue studying neurotransmitter secretion, it was thought that being able to observe as much of the SERS spectrum of the neurotransmitters as possible without spectral interference from molecules functionalized at the surface, would be critical. Although surface-bound molecules would make focusing straightforward, there is also concern about how the any potential functional groups will alter the interactions of the secreted molecules with the metal surface.183 Given that core–molecule–shell nanoparticles are prevalent in SERS experiments in tissue, various types of these nanoparticles were also explored. The challenge once again being spectral overlap. This however can be minimized by working with a nanosensor that has different types of nanoparticles on the surface, with the core–molecule–shell nanoparticles only being present in low quantities. To achieve a SERS signal compatible with focusing on the nanosensor, longer acquisition times are required (Fig. 15D). Since shorter acquisition times are needed to evaluate secretion events, the spectral interference should be dramatically reduced. As well, the Rayleigh scattering was also thought to be a potential avenue for focusing on the sensor and was found to have some merit. The blueprint provides a high degree of flexibility, allowing for specific pathways to be taken, or even combining approaches, such as using both the Rayleigh scattering and an internalized molecule. The Rayleigh scattering can offer real-time information during the measurement as there may not always be a molecule at the surface of the nanosensor. Furthermore, the use of an extended platform at the stage allows for a means of triggering secretion to be installed. The adaptability of the stage design offers considerable versatility across different types of experiments and microscope designs.
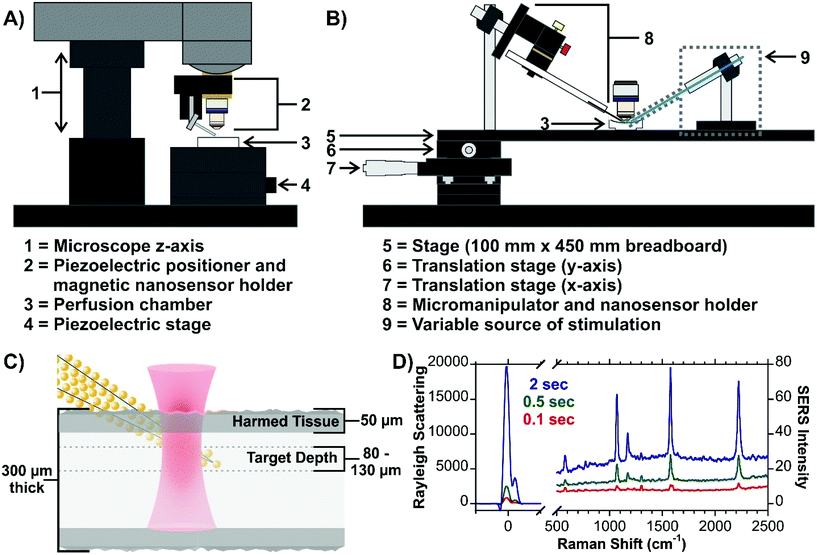 |
| Fig. 15 (A) Scheme for a micromanipulator-based set-up for inserting SERS nanosensors into live tissue. The design can be configured to include a means of stimulating secretion of molecules, such as neurotransmitters in brain tissue. Included is an optical image of a nanosensor at a depth of 70 μm. (B) Scheme for live tissue SERS measurements indicating the target depth of the measurements. (C) SERS spectra of a hybrid nanosensor composed of gold nanoraspberries and core–molecule–shell nanoparticles illustrating different ways of focusing on the nanosensor once it is inserted into the tissue. Reprinted from J. Chem. Phys., 2020, 153, 124702 (ref. 147), with the permission of AIP Publishing. | |
6 Overcoming the tissue barrier
Except for having the nanoparticles on the outer surface of the tissue, the other described methods suffer from the same issue, optical interference from the tissue. Simply removing the tissue and replacing it with an optical window184 is not often practical or possible. This is especially the case for ex vivo tissue samples. Alternatively, an optical clearing agent can be introduced as it reduces the scattering of turbid dermal tissue.185 With this in mind, we briefly present alternative approaches that have been or potentially could be used to greatly reduce the negative effects associated with working with tissue.
6.1 Longer excitation wavelength
As was discussed in section 2, in the visible region, the scattering and absorbance of the tissue is high. As a result, it is preferable to use an excitation wavelength outside of this region. Typically, an excitation wavelength near 785 nm is used for bio-SERS experiments. It has been shown that biomedical imaging can benefit from using near-infrared fluorophores,31 in particular fluorophores that lie within the second near-infrared window (1000–1700 nm). Since similar challenges exist between fluorescence imaging and SERS measurements in tissue, it is thought that SERS can also benefit from making a transition to longer wavelengths.186 Within this range, 1064 nm is commonly used.187–189 Longer excitation wavelengths, such as 1280 and ∼1550 nm have also been used in SE(R)RS studies,190–193 with one of the applications being their use with designed SERRS nanotags.190,193 In addition to tuning the plasmonic properties of the nanostructure, the use of a longer excitation wavelength requires that the Raman set-up be modified, most notably the detector. Instead of using a silicon detector, an InGaAs camera is needed. It is also important to recognize that although the scattering from the components of the tissue is lower in the second near-IR window, water has an absorbance from ∼1400–1600 nm from vibrational overtones that must also be considered (Fig. 16).31,194 Given the limited number of SERS studies involving these excitation wavelengths, there is considerable development that can be done to show the utility and applicability of these wavelengths.
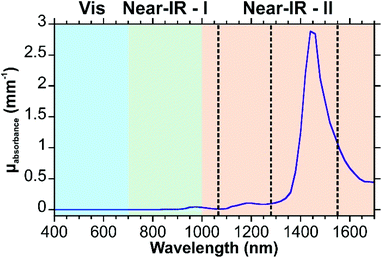 |
| Fig. 16 Absorption spectrum of water in the visible and near-IR. Excitation wavelengths of 1064, 1280, and 1550 nm are indicated with dashed lines. Spectrum reproduced using the data of ref. 42. | |
6.2 Guided delivery of the excitation light into the tissue
Regardless of whether a Raman microscope or handheld Raman spectrometer is used, in most experiments, the excitation photons travel through the tissue to reach the plasmonic nanostructures and then the Raman scattered photons must travel once again through the tissue to reach the collection optics. By delivering the light directly into the tissue using an optical fiber, it is possible to eliminate one or both steps involving the photons travelling through the tissue. This can be done by having the optical fiber deliver the photons and collect the scattered photons, or by having additional collection optics (i.e. microscope objective) collect the scattered photons. Nanoparticles that are coated onto the surface of the optical fiber allow for the types of experiments shown in Scheme 1i–iii to be performed, allowing for the plasmonic optical fiber to act as a “lab-on-a-fiber”.195–198 As mentioned in the section on SERS nanosensors, this approach has been used for studies involving cells.150 On a larger scale, this has been used in clinical settings with Raman endoscopes to probe nanoparticles199–201 or for local SERS sensing using functionalized plasmonic optical fibers.202 Although it may be possible to insert fibers of their original diameter into the tissue to detect analytes or local environments, to minimize the damage to ex vivo tissue slices, tapered plasmonic optical fibers should be used as a starting point.
6.3 Deep Raman measurements
By tuning the composition of a SERRS nanotag, it was demonstrated that SERRS spectra can be recorded at a depth of 10 mm into a tissue sample using a handheld Raman spectrometer with an excitation wavelength of 785 nm.203 There is however another Raman-based technique that is highly suited to measurements deep within tissue. Spatially offset Raman scattering (SORS) considers the background contributions (Raman peaks, fluorescence) to extract spectral information from a target depth.204–206 This is done by collecting at least two spectra. The first spectrum is recorded with no offset (classical backscattering configuration). A second spectrum is then collected at a position that is offset from the excitation position. By varying the offset positions, different depths can be probed. Using scaled subtraction, the zero offset and offset spectra are subtracted from one another allowing for the signals not at the target depth to be suppressed. Like Raman scattering, SORS can benefit from surface-enhancement, yielding the technique SESORS.207 Although commonly used for probing SE(R)RS nanotags at a variety of depths within tissue samples,208–211 SESORS can also be used to collect spectra of analytes within the tissue.212–215 In these instances, precise controlling of the nanoparticle depth or a homogeneous distribution of the nanoparticles throughout the tissue is beneficial. In addition to being able to probe deep within tissue, a major advantage of SESORS is that spectra can be acquired behind a physical barrier, such as bone.216,217 For example, being able to probe the brain without removing any or all of the skull is an important step in the further development of surface-enhanced Raman techniques.218 As an example, this approach has been used to detect neurotransmitters that were added to brain tissue placed beneath bone.214,215 Although these have been proof-of-concept studies, they have demonstrated that SESORS can be an interesting alternative to SERS when the optical properties of the tissue and other biomaterials are difficult to overcome using other methods.
7 Conclusions and outlook
The transition from working with single cells to tissues can be daunting. It is however important to recognize that the developments that have been made and the approaches used for SERS experiments involving cells can be used with studies involving complex tissues. The important step is to recognize the desired outcome, as this will influence the approach that is employed. This can range from simply coating fixed tissue slices with nanoparticle or designing nanotags for targeted delivery within the tissue or the fabrication of nanosensors that can be inserted directly into the tissue. All these schemes have merit and have the potential to see greater use as the applications of SERS continue to grow. An interesting area that will likely continue to grow is combining SERS with other techniques, such as fluorescence. This requires the preparation of dual-mode nanotags.219–221 Dual-mode nanoparticles, and therefore nanotags, can also be designed for compatibility with diagnostic imaging and tomography.56,222–224 Similarly, SERS nanosensors can be fabricated with an electrode,225 leading to the possibility of performing simultaneous electrophysiology and SERS measurements. Developing means of combining SERS with the conventional methods that are used in our desired applications is an important step in broadening the usage of SERS, especially as a bioanalytical technique.
Conflicts of interest
There are no conflicts to declare.
Acknowledgements
This work was funded by the Fonds de Recherche du Québec – Nature et Technologies (FRQ-NT; J.-F. M.), – Santé (FRQS; G. Q. W.), and the Natural Science and Engineering Research Council of Canada (NSERC; J.-F. M.).
References
-
E. Smith and G. Dent, Modern Raman Spectroscopy – A Practical Approach, John Wiley & Sons, Ltd., Chichester, United Kingdom, 2005 Search PubMed.
- J. Langer, D. Jimenez de Aberasturi, J. Aizpurua, R. A. Alvarez-Puebla, B. Auguié, J. J. Baumberg, G. C. Bazan, S. E. J. Bell, A. Boisen, A. G. Brolo, J. Choo, D. Cialla-May, V. Deckert, L. Fabris, K. Faulds, F. J. García de Abajo, R. Goodacre, D. Graham, A. J. Haes, C. L. Haynes, C. Huck, T. Itoh, M. Käll, J. Kneipp, N. A. Kotov, H. Kuang, E. C. Le Ru, H. K. Lee, J.-F. Li, X. Y. Ling, S. A. Maier, T. Mayerhöfer, M. Moskovits, K. Murakoshi, J.-M. Nam, S. Nie, Y. Ozaki, I. Pastoriza-Santos, J. Perez-Juste, J. Popp, A. Pucci, S. Reich, B. Ren, G. C. Schatz, T. Shegai, S. Schlücker, L.-L. Tay, K. G. Thomas, Z.-Q. Tian, R. P. Van Duyne, T. Vo-Dinh, Y. Wang, K. A. Willets, C. Xu, H. Xu, Y. Xu, Y. S. Yamamoto, B. Zhao and L. M. Liz-Marzán, ACS Nano, 2020, 14, 28–117 CrossRef CAS.
- M. Fan, G. F. S. Andrade and A. G. Brolo, Anal. Chim. Acta, 2020, 1097, 1–29 CrossRef CAS.
- M. A. Fikiet, S. R. Khandasammy, E. Mistek, Y. Ahmed, L. Halámková, J. Bueno and I. K. Lednev, Spectrochim. Acta, Part A, 2018, 197, 255–260 CrossRef CAS.
- C.-C. Huang, C.-Y. Cheng and Y.-S. Lai, Trends Food Sci. Technol., 2020, 100, 349–358 CrossRef CAS.
- M.-L. Xu, Y. Gao, X. X. Han and B. Zhao, J. Agric. Food Chem., 2017, 65, 6719–6726 CrossRef CAS.
- T. T. X. Ong, E. W. Blanch and O. A. H. Jones, Sci. Total Environ., 2020, 720, 137601 CrossRef CAS.
- T. J. Moore, A. S. Moody, T. D. Payne, G. M. Sarabia, A. R. Daniel and B. Sharma, Biosensors, 2018, 8, 46 CrossRef.
- A. Chakraborty, A. Ghosh and A. Barui, J. Raman Spectrosc., 2020, 51, 7–36 CrossRef CAS.
- A. Merdalimova, V. Chernyshev, D. Nozdriukhin, P. Rudakovskaya, D. Gorin and A. Yashchenok, Appl. Sci., 2019, 9, 1135 CrossRef CAS.
- G. Kuku, M. Altunbek and M. Culha, Anal. Chem., 2017, 89, 11160–11166 CrossRef CAS.
- A. H. Nguyen, E. A. Peters and Z. D. Schultz, Rev. Anal. Chem., 2017, 36, 20160037 CAS.
- X.-S. Zheng, I. J. Jahn, K. Weber, D. Cialla-May and J. Popp, Spectrochim. Acta, Part A, 2018, 197, 56–77 CrossRef CAS.
- S. Laing, L. E. Jamieson, K. Faulds and D. Graham, Nat. Rev. Chem., 2017, 1, 0060 CrossRef CAS.
- L. Cui, D. Zhang, K. Yang, X. Zhang and Y.-G. Zhu, Anal. Chem., 2019, 91, 15345–15354 CrossRef CAS.
- J. Wang, K. M. Koo, Y. Wang and M. Trau, Adv. Sci., 2019, 6, 1900730 CrossRef CAS.
- N. Akkilic, S. Geschwindner and F. Höök, Biosens. Bioelectron., 2020, 151, 111944 CrossRef CAS.
- F. Lussier, V. Thibault, B. Charron, G. Q. Wallace and J.-F. Masson, Trends Anal. Chem., 2020, 124, 115796 CrossRef CAS.
- L. Wu, Z. Wang, Y. Zhang, J. Fei, H. Chen, S. Zong and Y. Cui, Nano Res., 2017, 10, 584–594 CrossRef CAS.
- Y.-F. Tian, C.-F. Ning, F. He, B.-C. Yin and B.-C. Ye, Analyst, 2018, 143, 4915–4922 RSC.
- W. Zhang, L. Jiang, R. J. Diefenbach, D. H. Campbell, B. J. Walsh, N. H. Packer and Y. Wang, ACS Sens., 2020, 5, 764–771 CrossRef CAS.
- U. E. Martinez-Outschoorn, M. Peiris-Pagés, R. G. Pestell, F. Sotgia and M. P. Lisanti, Nat. Rev. Clin. Oncol., 2017, 14, 11–31 CrossRef CAS.
- V. L. Camus, G. Stewart, W. H. Nailon, D. B. McLaren and C. J. Campbell, Analyst, 2016, 141, 5056–5061 RSC.
- L. E. Jamieson, V. L. Camus, P. O. Bagnaninchi, K. M. Fisher, G. D. Stewart, W. H. Nailon, D. B. McLaren, D. J. Harrison and C. J. Campbell, Nanoscale, 2016, 8, 16710–16718 RSC.
- M. Altunbek, D. Çetin, Z. Suludere and M. Çulha, Talanta, 2019, 191, 390–399 CrossRef CAS.
- P. T. Sujai, M. M. Joseph, G. Saranya, J. B. Nair, V. P. Murali and K. K. Maiti, Nanoscale, 2020, 12, 6971–6975 RSC.
- Ö. Aydin, M. Kahraman, E. Kiliç and M. Çulha, Appl. Spectrosc., 2009, 63, 662–668 CrossRef.
- Ö. Aydin, M. Altaş, M. Kahraman, Ö. F. Bayrak and M. Çulha, Appl. Spectrosc., 2009, 63, 1095–1100 CrossRef.
- J. Li, C. Wang, Y. Yao, Y. Zhu, C. Yan, Q. Zhuge, L. Qu and C. Han, Talanta, 2020, 216, 120983 CrossRef CAS.
- L. Shen, Y. Du, N. Wei, Q. Li, S. Li, T. Sun, S. Xu, H. Wang, X. Man and B. Han, Spectrochim. Acta, Part A, 2020, 237, 118364 CrossRef CAS.
- G. Hong, A. L. Antaris and H. Dai, Nat. Biomed. Eng., 2017, 1, 0010 CrossRef CAS.
- S. L. Jacques, Phys. Med. Biol., 2013, 58, R37–R61 CrossRef.
- N. G. Horton, K. Wang, D. Kobat, C. G. Clark, F. W. Wise, C. B. Schaffer and C. Xu, Nat. Photonics, 2013, 7, 205–209 CrossRef CAS.
- A. N. Bashkatov, E. A. Genina, V. I. Kochubey and V. V. Tuchin, J. Phys. D: Appl. Phys., 2005, 38, 2543–2555 CrossRef CAS.
- A. N. BASHKATOV, E. A. GENINA and V. V. Tuchin, J. Innovative Opt. Health Sci., 2011, 04, 9–38 CrossRef.
- A. Bashkatov, E. Genina, V. Kochubey and V. Tuchin, Proc. SPIE, 2006, 6163, 616310 CrossRef.
- H. J. van Staveren, C. J. M. Moes, J. van Marie, S. A. Prahl and M. J. C. van Gemert, Appl. Opt., 1991, 30, 4507–4514 CrossRef CAS.
- G. Hong, S. Diao, A. L. Antaris and H. Dai, Chem. Rev., 2015, 115, 10816–10906 CrossRef CAS.
- M. S. Viegas, T. C. Martins, F. Seco and A. do Carmo, Eur. J. Histochem., 2009, 51, 59–66 Search PubMed.
- J. V. Frangioni, Curr. Opin. Chem. Biol., 2003, 7, 626–634 CrossRef CAS.
-
S. A. Prahl, A compendium of tissue optical properties, 1998 (http://omlc.ogi.edu/spectra/hemoglobin) Search PubMed.
- G. M. Hale and M. R. Querry, Appl. Opt., 1973, 12, 555–563 CrossRef CAS.
-
R. L. P. van Veen, H. J. C. M. Sterenborg, A. Pifferi, A. Torricelli and R. Cubeddu, OSA Annual BIOMED Topical Meeting, 2004, p. SF4 Search PubMed.
- E. Lenzi, D. Jimenez de Aberasturi and L. M. Liz-Marzán, ACS Sens., 2019, 4, 1126–1137 CrossRef CAS.
- Z. Wang, S. Zong, L. Wu, D. Zhu and Y. Cui, Chem. Rev., 2017, 117, 7910–7963 CrossRef CAS.
- J. Kneipp, ACS Nano, 2017, 11, 1136–1141 CrossRef CAS.
- W. E. Doering and S. Nie, Anal. Chem., 2003, 75, 6171–6176 CrossRef CAS.
- Q. Yu, Y. Wang, R. Mei, Y. Yin, J. You and L. Chen, Anal. Chem., 2019, 91, 5270–5277 CrossRef CAS.
- F. Sun, D. D. Galvan, P. Jain and Q. Yu, Chem. Commun., 2017, 53, 4550–4561 RSC.
- Y. Luo, Y. Xiao, D. Onidas, L. Iannazzo, M. Ethève-Quelquejeu, A. Lamouri, N. Félidj, S. Mahouche-Chergui, T. Brulé, N. Gagey-Eilstein, F. Gazeau and C. Mangeney, Chem. Commun., 2020, 56, 6822–6825 RSC.
- M. Sánchez-Purrà, B. Roig-Solvas, C. Rodriguez-Quijada, B. M. Leonardo and K. Hamad-Schifferli, ACS Omega, 2018, 3, 10733–10742 CrossRef.
- J. Li, H. Liu, P. Rong, W. Zhou, X. Gao and D. Liu, Nanoscale, 2018, 10, 8292–8297 RSC.
- J. Wang, D. Liang, J. Feng and X. Tang, Anal. Chem., 2019, 91, 11045–11054 CrossRef CAS.
- K. V. Kong, Z. Lam, W. D. Goh, W. K. Leong and M. Olivo, Angew. Chem., Int. Ed., 2012, 51, 9796–9799 CrossRef CAS.
- T. Gong, Z.-Y. Hong, C.-H. Chen, C.-Y. Tsai, L.-D. Liao and K. V. Kong, ACS Nano, 2017, 11, 3365–3375 CrossRef CAS.
- W. Zhu, M.-Y. Gao, Q. Zhu, B. Chi, L.-W. Zeng, J.-M. Hu and A.-G. Shen, Nanoscale, 2020, 12, 3292–3301 RSC.
- Y. Zhang, Y. Gu, J. He, B. D. Thackray and J. Ye, Nat. Commun., 2019, 10, 3905 CrossRef.
- S. Sloan-Dennison, M. R. Bevins, B. T. Scarpitti, V. K. Sauvé and Z. D. Schultz, Analyst, 2019, 144, 5538–5546 RSC.
- Y. Zhang, X.-P. Wang, S. Perner, A. Bankfalvi and S. Schlücker, Anal. Chem., 2018, 90, 760–768 CrossRef CAS.
- J. Li, Z. Zhu, B. Zhu, Y. Ma, B. Lin, R. Liu, Y. Song, H. Lin, S. Tu and C. Yang, Anal. Chem., 2016, 88, 7828–7836 CrossRef CAS.
- N. Choi, H. Dang, A. Das, M. S. Sim, I. Y. Chung and J. Choo, Biosens. Bioelectron., 2020, 164, 112326 CrossRef CAS.
- M. Bhamidipati, H.-Y. Cho, K.-B. Lee and L. Fabris, Bioconjugate Chem., 2018, 29, 2970–2981 CrossRef CAS.
- B. R. Lutz, C. E. Dentinger, L. N. Nguyen, L. Sun, J. Zhang, A. N. Allen, S. Chan and B. S. Knudsen, ACS Nano, 2008, 2, 2306–2314 CrossRef CAS.
- L. Sun, K.-B. Sung, C. Dentinger, B. Lutz, L. Nguyen, J. Zhang, H. Qin, M. Yamakawa, M. Cao, Y. Lu, A. J. Chmura, J. Zhu, X. Su, A. A. Berlin, S. Chan and B. Knudsen, Nano Lett., 2007, 7, 351–356 CrossRef CAS.
- D. Jimenez de Aberasturi, A. B. Serrano-Montes, J. Langer, M. Henriksen-Lacey, W. J. Parak and L. M. Liz-Marzán, Chem. Mater., 2016, 28, 6779–6790 CrossRef CAS.
- Y. Chen, J.-Q. Ren, X.-G. Zhang, D.-Y. Wu, A.-G. Shen and J.-M. Hu, Anal. Chem., 2016, 88, 6115–6119 CrossRef CAS.
- J. P. Nolan, E. Duggan, E. Liu, D. Condello, I. Dave and S. A. Stoner, Methods, 2012, 57, 272–279 CrossRef CAS.
- C. M. MacLaughlin, N. Mullaithilaga, G. Yang, S. Y. Ip, C. Wang and G. C. Walker, Langmuir, 2013, 29, 1908–1919 CrossRef CAS.
- A. Pallaoro, M. R. Hoonejani, G. B. Braun, C. D. Meinhart and M. Moskovits, ACS Nano, 2015, 9, 4328–4336 CrossRef CAS.
- K. Zhang, C. Hao, B. Man, C. Zhang, C. Yang, M. Liu, Q. Peng and C. Chen, Vib. Spectrosc., 2018, 98, 82–87 CrossRef CAS.
- K. Lin, J. Xu, L. Li, F. Liao, X. Dong and J. Lin, Laser Phys. Lett., 2018, 15, 125601 CrossRef CAS.
- B. Brozek-Pluska, M. Kopec and J. Surmacki, J. Spectrosc., 2018, 2018, 4893274 Search PubMed.
- Z. Li, C. Li, D. Lin, Z. Huang, J. Pan, G. Chen, J. Lin, N. Liu, Y. Yu, S. Feng and R. Chen, Laser Phys. Lett., 2014, 11, 045602 CrossRef.
- S. Feng, J. Lin, Z. Huang, G. Chen, W. Chen, Y. Wang, R. Chen and H. Zeng, Appl. Phys. Lett., 2013, 102, 043702 CrossRef.
- D. Lin, G. Chen, S. Feng, J. Pan, J. Lin, Z. Huang and R. Chen, Appl. Phys. Lett., 2015, 106, 013701 CrossRef.
- D. Bury, C. L. M. Morais, K. M. Ashton, T. P. Dawson and F. L. Martin, Biosensors, 2019, 9, 49 CrossRef CAS.
- T. Yang, Z. Zhang, B. Zhao, R. Hou, A. Kinchla, J. M. Clark and L. He, Anal. Chem., 2016, 88, 5243–5250 CrossRef CAS.
- T. Yang, J. Doherty, H. Guo, B. Zhao, J. M. Clark, B. Xing, R. Hou and L. He, Anal. Chem., 2019, 91, 2093–2099 CrossRef CAS.
- T. Guo, F. Ding, D. Li, W. Zhang, L. Cao and Z. Liu, Appl. Sci., 2019, 9, 398 CrossRef CAS.
- S. K. Das, K. Pal, T. S. Bhattacharya, P. Karmakar and J. Chowdhury, Sens. Actuators, B, 2019, 299, 126962 CrossRef CAS.
- M. Tabatabaei, G. Q. Wallace, F. A. Caetano, E. R. Gillies, S. S. G. Ferguson and F. Lagugné-Labarthet, Chem. Sci., 2016, 7, 575–582 RSC.
- V. Caprettini, J.-A. Huang, F. Moia, A. Jacassi, C. A. Gonano, N. Maccaferri, R. Capozza, M. Dipalo and F. De Angelis, Adv. Sci., 2018, 5, 1800560 CrossRef.
- S. Yamazoe, M. Naya, M. Shiota, T. Morikawa, A. Kubo, T. Tani, T. Hishiki, T. Horiuchi, M. Suematsu and M. Kajimura, ACS Nano, 2014, 8, 5622–5632 CrossRef CAS.
- M. Shiota, M. Naya, T. Yamamoto, T. Hishiki, T. Tani, H. Takahashi, A. Kubo, D. Koike, M. Itoh, M. Ohmura, Y. Kabe, Y. Sugiura, N. Hiraoka, T. Morikawa, K. Takubo, K. Suina, H. Nagashima, O. Sampetrean, O. Nagano, H. Saya, S. Yamazoe, H. Watanabe and M. Suematsu, Nat. Commun., 2018, 9, 1561 CrossRef.
- A. Kapara, V. Brunton, D. Graham and K. Faulds, Chem. Sci., 2020, 11, 5819–5829 RSC.
- B. Yameen, W. I. Choi, C. Vilos, A. Swami, J. Shi and O. C. Farokhzad, J. Controlled Release, 2014, 190, 485–499 CrossRef CAS.
- A. Sohrabi Kashani, S. Badilescu, A. Piekny and M. Packirisamy, ACS Appl. Nano Mater., 2020, 3, 4114–4126 CrossRef CAS.
- R. E. Darienzo, J. Wang, O. Chen, M. Sullivan, T. Mironava, H. Kim and R. Tannenbaum, ACS Appl. Nano Mater., 2019, 2, 6960–6970 CrossRef CAS.
- D. Y. Öztaş, M. Altunbek, D. Uzunoglu, H. Yılmaz, D. Çetin, Z. Suludere and M. Çulha, Langmuir, 2019, 35, 4020–4028 CrossRef.
- H. Gao, Z. Yang, S. Zhang, S. Cao, S. Shen, Z. Pang and X. Jiang, Sci. Rep., 2013, 3, 2534 CrossRef CAS.
- T. Mironava, M. Hadjiargyrou, M. Simon, V. Jurukovski and M. H. Rafailovich, Nanotoxicology, 2010, 4, 120–137 CrossRef CAS.
- A. Albanese, P. S. Tang and W. C. W. Chan, Annu. Rev. Biomed. Eng., 2012, 14, 1–16 CrossRef CAS.
- D. Jimenez de Aberasturi, M. Henriksen-Lacey, L. Litti, J. Langer and L. M. Liz-Marzán, Adv. Funct. Mater., 2020, 30, 1909655 CrossRef CAS.
- M. Pannico, A. Calarco, G. Peluso and P. Musto, Biosensors, 2018, 8, 87 CrossRef CAS.
- G. P. Szekeres, M. Montes-Bayon, J. Bettmer and J. Kneipp, Anal. Chem., 2020, 92, 8553–8560 CrossRef CAS.
- Y. Shen, L. Yang, L. Liang, Z. Li, J. Zhang, W. Shi, C. Liang, W. Xu and S. Xu, Anal. Bioanal. Chem., 2019, 411, 6021–6029 CrossRef CAS.
- G. Qi, Y. Zhang, S. Xu, C. Li, D. Wang, H. Li and Y. Jin, Anal. Chem., 2018, 90, 13356–13364 CrossRef CAS.
- J. Wang, G. Qi, X. Qu, X. Ling, Z. Zhang and Y. Jin, Anal. Chem., 2020, 92, 3735–3741 CrossRef CAS.
- J. Yue, Y. Shen, L. Liang, L. Cong, W. Xu, W. Shi, C. Liang and S. Xu, Anal. Chem., 2020, 92, 6081–6087 CrossRef CAS.
- J. Yue, Y. Shen, L. Liang, L. Cong, X. Guan, Z. Li, S. Xu, C. Liang, W. Shi and W. Xu, J. Raman Spectrosc., 2020, 51, 602–610 CrossRef CAS.
- G. Qi, B. Wang, Y. Zhang, H. Li, C. Li, W. Xu and Y. Jin, Anal. Chem., 2019, 91, 9571–9579 CrossRef CAS.
- X.-S. Zheng, C. Zong, X. Wang and B. Ren, Anal. Chem., 2019, 91, 8383–8389 CrossRef CAS.
- S.-S. Li, M. Zhang, J.-H. Wang, F. Yang, B. Kang, J.-J. Xu and H.-Y. Chen, Anal. Chem., 2019, 91, 8398–8405 CrossRef CAS.
- Z. Zhang, K. Bando, K. Mochizuki, A. Taguchi, K. Fujita and S. Kawata, Anal. Chem., 2019, 91, 3254–3262 CrossRef CAS.
- G. Qi, H. Li, Y. Zhang, C. Li, S. Xu, M. Wang and Y. Jin, Anal. Chem., 2019, 91, 1408–1415 CrossRef CAS.
- X. Qin, Y. Si, Z. Wu, K. Zhang, J. Li and Y. Yin, Anal. Chem., 2020, 92, 924–931 CrossRef CAS.
- H.-Y. Chen, E. Kouadio Fodjo, L. Jiang, S. Chang, J.-B. Li, D.-S. Zhan, H.-X. Gu and D.-W. Li, ACS Sens., 2019, 4, 3234–3239 CrossRef CAS.
- W.-S. Zhang, Y.-N. Wang and Z.-R. Xu, Anal. Chim. Acta, 2020, 1094, 106–112 CrossRef CAS.
- P. Wang, L. Lux, M. Jin, Y. Wan, W. Wang, C.-T. Hung, F. H. Albaqami, A. M. El-Toni, M. S. Alhoshan, X. Li and F. Zhang, ACS Appl. Bio Mater., 2019, 2, 417–423 CrossRef CAS.
- L. Bai, X. Wang, K. Zhang, X. Tan, Y. Zhang and W. Xie, Chem. Commun., 2019, 55, 12996–12999 RSC.
- A. P. Silwal and H. P. Lu, ACS Omega, 2018, 3, 14849–14857 CrossRef CAS.
- C. Wei, X. Liu, Y. Gao, Y. Wu, X. Guo, Y. Ying, Y. Wen and H. Yang, Anal. Chem., 2018, 90, 11333–11339 CrossRef CAS.
- G. Qi, D. Sun, Y. Tian, C. Xu, Y. Zhang, D. Wang, K. Ma, S. Xu and Y. Jin, Anal. Chem., 2020, 92, 7861–7868 CrossRef CAS.
- X. Qin, M. Lyu, Y. Si, J. Yang, Z. Wu and J. Li, Anal. Chim. Acta, 2018, 1043, 115–122 CrossRef CAS.
- J. Zhang, X. Ma and Z. Wang, Anal. Chem., 2019, 91, 6600–6607 CAS.
- N. Zhang, S. Ye, Z. Wang, R. Li and M. Wang, ACS Sens., 2019, 4, 924–930 CrossRef CAS.
- C. Liu, C. Chen, S. Li, H. Dong, W. Dai, T. Xu, Y. Liu, F. Yang and X. Zhang, Anal. Chem., 2018, 90, 10591–10599 CrossRef CAS.
- V. Živanović, G. Semini, M. Laue, D. Drescher, T. Aebischer and J. Kneipp, Anal. Chem., 2018, 90, 8154–8161 CrossRef.
- K. Dardir, H. Wang, B. E. Martin, M. Atzampou, C. B. Brooke and L. Fabris, J. Phys. Chem. C, 2020, 124, 3211–3217 CrossRef CAS.
- X. Qin, Y. Si, D. Wang, Z. Wu, J. Li and Y. Yin, Anal. Chem., 2019, 91, 4529–4536 CrossRef CAS.
- D. Ma, J. Zheng, P. Tang, W. Xu, Z. Qing, S. Yang, J. Li and R. Yang, Anal. Chem., 2016, 88, 11852–11859 CrossRef CAS.
- Y. Zhou, J. Liu, T. Zheng and Y. Tian, Anal. Chem., 2020, 92, 5910–5920 CrossRef CAS.
- W. Qiang, W.-M. Yau, J.-X. Lu, J. Collinge and R. Tycko, Nature, 2017, 541, 217–221 CrossRef CAS.
- M. G. Iadanza, M. P. Jackson, E. W. Hewitt, N. A. Ranson and S. E. Radford, Nat. Rev. Mol. Cell Biol., 2018, 19, 755–773 CrossRef CAS.
- J. K. Register, A. M. Fales, H.-N. Wang, S. J. Norton, E. H. Cho, A. Boico, S. Pradhan, J. Kim, T. Schroeder, N. A. Wisniewski, B. Klitzman and T. Vo-Dinh, Anal. Bioanal. Chem., 2015, 407, 8215–8224 CrossRef CAS.
- C. Zhang, X. Cui, J. Yang, X. Shao, Y. Zhang and D. Liu, Chem. Sci., 2020, 11, 6111–6120 RSC.
- T. J. E. Hubbard, A. Shore and N. Stone, Analyst, 2019, 144, 6479–6496 RSC.
- C. Andreou, V. Neuschmelting, D.-F. Tschaharganeh, C.-H. Huang, A. Oseledchyk, P. Iacono, H. Karabeber, R. R. Colen, L. Mannelli, S. W. Lowe and M. F. Kircher, ACS Nano, 2016, 10, 5015–5026 CrossRef CAS.
- Y. Zhang, Y. Qiu, L. Lin, H. Gu, Z. Xiao and J. Ye, ACS Appl. Mater. Interfaces, 2017, 9, 3995–4005 CrossRef CAS.
- Y. Qiu, Y. Zhang, M. Li, G. Chen, C. Fan, K. Cui, J.-B. Wan, A. Han, J. Ye and Z. Xiao, ACS Nano, 2018, 12, 7974–7985 CrossRef CAS.
- R. M. Davis, B. Kiss, D. R. Trivedi, T. J. Metzner, J. C. Liao and S. S. Gambhir, ACS Nano, 2018, 12, 9669–9679 CrossRef CAS.
- H. Arami, C. B. Patel, S. J. Madsen, P. J. Dickinson, R. M. Davis, Y. Zeng, B. K. Sturges, K. D. Woolard, F. G. Habte, D. Akin, R. Sinclair and S. S. Gambhir, ACS Nano, 2019, 13, 2858–2869 CrossRef CAS.
- W. Duan, Q. Yue, Y. Liu, Y. Zhang, Q. Guo, C. Wang, S. Yin, D. Fan, W. Xu, J. Zhuang, J. Gong, X. Li, R. Huang, L. Chen, S. Aime, Z. Wang, J. Feng, Y. Mao, X.-Y. Zhang and C. Li, Chem. Sci., 2020, 11, 4397–4402 RSC.
- R. J. Gillies, Z. Liu and Z. Bhujwalla, Am. J. Physiol., 1994, 267, C195–C203 CrossRef CAS.
- E. A. Vitol, Z. Orynbayeva, M. J. Bouchard, J. Azizkhan-Clifford, G. Friedman and Y. Gogotsi, ACS Nano, 2009, 3, 3529–3536 CrossRef CAS.
- J.-F. Masson, J. Breault-Turcot, R. Faid, H.-P. Poirier-Richard, H. Yockell-Lelièvre, F. Lussier and J. P. Spatz, Anal. Chem., 2014, 86, 8998–9005 CrossRef CAS.
- J. Liu, D. Yin, S. Wang, H.-Y. Chen and Z. Liu, Angew. Chem., Int. Ed., 2016, 55, 13215–13218 CrossRef CAS.
- F. Lussier, T. Brulé, M. Vishwakarma, T. Das, J. P. Spatz and J.-F. Masson, Nano Lett., 2016, 16, 3866–3871 CrossRef CAS.
- S. Hanif, H.-L. Liu, S. A. Ahmed, J.-M. Yang, Y. Zhou, J. Pang, L.-N. Ji, X.-H. Xia and K. Wang, Anal. Chem., 2017, 89, 9911–9917 CrossRef CAS.
- S. Hanif, H. Liu, M. Chen, P. Muhammad, Y. Zhou, J. Cao, S. A. Ahmed, J. Xu, X. Xia, H. Chen and K. Wang, Anal. Chem., 2017, 89, 2522–2530 CrossRef CAS.
- F. Lussier, T. Brulé, M.-J. Bourque, C. Ducrot, L.-É. Trudeau and J.-F. Masson, Faraday Discuss., 2017, 205, 387–407 RSC.
- F. Lussier, D. Missirlis, J. P. Spatz and J.-F. Masson, ACS Nano, 2019, 13, 1403–1411 CAS.
- T. D. Nguyen, M. S. Song, N. H. Ly, S. Y. Lee and S.-W. Joo, Angew. Chem., Int. Ed., 2019, 58, 2710–2714 CrossRef CAS.
- W. Wang, F. Zhao, M. Li, C. Zhang, Y. Shao and Y. Tian, Angew. Chem., Int. Ed., 2019, 58, 5256–5260 CrossRef CAS.
- X. Zhao, S. Campbell, G. Q. Wallace, A. Claing, C. G. Bazuin and J.-F. Masson, ACS Sens., 2020, 5, 2155–2167 CrossRef CAS.
- H. Zhu, F. Lussier, C. Ducrot, M.-J. Bourque, J. P. Spatz, W. Cui, L. Yu, W. Peng, L.-É. Trudeau, C. G. Bazuin and J.-F. Masson, ACS Appl. Mater. Interfaces, 2019, 11, 4373–4384 CrossRef CAS.
- G. Q. Wallace, B. Delignat-Lavaud, X. Zhao, L.-É. Trudeau and J.-F. Masson, J. Chem. Phys., 2020, 153, 124702 CrossRef.
- P.-H. Liao, C.-Y. Tseng, Z.-Y. Ke, C.-L. Hsieh and K. V. Kong, Chem. Commun., 2020, 56, 4852–4855 RSC.
- J. P. Scaffidi, M. K. Gregas, V. Seewaldt and T. Vo-Dinh, Anal. Bioanal. Chem., 2009, 393, 1135–1141 CrossRef CAS.
- J. Wang, Y. Geng, Y. Shen, W. Shi, W. Xu and S. Xu, Sens. Actuators, B, 2019, 290, 527–534 CrossRef CAS.
- J. Dong, Q. Chen, C. Rong, D. Li and Y. Rao, Anal. Chem., 2011, 83, 6191–6195 CrossRef CAS.
- J. Dong, Q. Tao, M. Guo, T. Yan and W. Qian, Anal. Methods, 2012, 4, 3879–3883 RSC.
- P. Muhammad, J. Liu, R. Xing, Y. Wen, Y. Wang and Z. Liu, Anal. Chim. Acta, 2017, 995, 34–42 CrossRef CAS.
- B. Zhou, M. Mao, X. Cao, M. Ge, X. Tang, S. Li, D. Lin, L. Yang and J. Liu, Anal. Chem., 2018, 90, 3826–3832 CrossRef CAS.
- S. Han, J. Sun, J. Wang, W. Qian and J. Dong, J. Raman Spectrosc., 2018, 49, 1747–1755 CrossRef CAS.
- B. Zhou, J. Shen, P. Li, M. Ge, D. Lin, Y. Y. Li, J. Lu and L. Yang, ACS Appl. Nano Mater., 2019, 2, 2752–2757 CrossRef CAS.
- P. Li, M. Ge, D. Lin and L. Yang, Anal. Bioanal. Chem., 2019, 411, 5669–5679 CrossRef CAS.
- M. Fleischmann, P. J. Hendra and A. J. McQuillan, Chem. Phys. Lett., 1974, 26, 163–166 CrossRef CAS.
- D. L. Jeanmaire and R. P. Van Duyne, J. Electroanal. Chem., 1977, 84, 1–20 CrossRef CAS.
- M. G. Albrecht and J. A. Creighton, J. Am. Chem. Soc., 1977, 99, 5215–5217 CrossRef CAS.
- R. M. Stöckle, V. Deckert, C. Fokas and R. Zenobi, Appl. Spectrosc., 2000, 54, 1577–1583 CrossRef.
- J. Huang, Z. He, X. He, Y. Liu, T. Wang, G. Chen, C. Tang, R. Jia, L. Liu, L. Zhang, J. Wang, X. Ai, S. Sun, X. Xu and K. Du, ACS Appl. Mater. Interfaces, 2017, 9, 28902–28910 CrossRef CAS.
- D. Gkogkou, T. Shaykhutdinov, C. Kratz, T. W. H. Oates, P. Hildebrandt, I. M. Weidinger, K. H. Ly, N. Esser and K. Hinrichs, Analyst, 2019, 144, 5271–5276 RSC.
- F. Zhou, M. Wang, L. Yuan, Z. Cheng, Z. Wu and H. Chen, Analyst, 2012, 137, 1779–1784 RSC.
- L. H. Kaufman, T. Cooper, G. Q. Wallace, D. Hawke, D. Betts, D. Hess and F. Lagugné-Labarthet, Proc. SPIE, 2019, 10894, 108940B Search PubMed.
- J. J. Niu, M. G. Schrlau, G. Friedman and Y. Gogotsi, Small, 2011, 7, 540–545 CrossRef CAS.
- H.-L. Liu, J. Cao, S. Hanif, C. Yuan, J. Pang, R. Levicky, X.-H. Xia and K. Wang, Anal. Chem., 2017, 89, 10407–10413 CrossRef CAS.
- H. Liu, Q. Jiang, J. Pang, Z. Jiang, J. Cao, L. Ji, X. Xia and K. Wang, Adv. Funct. Mater., 2018, 28, 1703847 CrossRef.
- D. A. Nelson and Z. D. Schultz, J. Phys. Chem. C, 2018, 122, 8581–8588 CrossRef CAS.
- Q. Zhang, Y. Zhou, X. Fu, E. Villarreal, L. Sun, S. Zou and H. Wang, J. Phys. Chem. C, 2019, 123, 26695–26704 CrossRef CAS.
- J.-J. Sun, H.-S. Su, H.-L. Yue, S.-C. Huang, T.-X. Huang, S. Hu, M. M. Sartin, J. Cheng and B. Ren, J. Phys. Chem. Lett., 2019, 10, 2306–2312 CrossRef CAS.
- R. M. Sarhan, W. Koopman, R. Schuetz, T. Schmid, F. Liebig, J. Koetz and M. Bargheer, Sci. Rep., 2019, 9, 3060 CrossRef.
- F. Liebig, R. M. Sarhan, M. Bargheer, C. N. Z. Schmitt, A. H. Poghosyan, A. A. Shahinyan and J. Koetz, RSC Adv., 2020, 10, 8152–8160 RSC.
- W. Qiu, F. Zhou, Q. Zhang, X. Sun, X. Shi, Y. Liang, X. Wang and L. Yue, APMIS, 2013, 121, 919–925 CrossRef CAS.
- M. Fähling, A. Steege, A. Perlewitz, B. Nafz, R. Mrowka, P. B. Persson and B. J. Thiele, Biochim. Biophys. Acta, Gene Struct. Expression, 2005, 1731, 32–40 CrossRef.
- F. Pichiorri, D. Palmieri, L. De Luca, J. Consiglio, J. You, A. Rocci, T. Talabere, C. Piovan, A. Lagana, L. Cascione, J. Guan, P. Gasparini, V. Balatti, G. Nuovo, V. Coppola, C. C. Hofmeister, G. Marcucci, J. C. Byrd, S. Volinia, C. L. Shapiro, M. A. Freitas and C. M. Croce, J. Exp. Med., 2013, 210, 951–968 CrossRef CAS.
- C. Yuen and Q. Liu, J. Biophotonics, 2014, 7, 683–689 CrossRef CAS.
- K. V. Kong, Z. Lam, W. K. O. Lau, W. K. Leong and M. Olivo, J. Am. Chem. Soc., 2013, 135, 18028–18031 CrossRef CAS.
- F. Sun, T. Bai, L. Zhang, J.-R. Ella-Menye, S. Liu, A. K. Nowinski, S. Jiang and Q. Yu, Anal. Chem., 2014, 86, 2387–2394 CrossRef CAS.
- G. Q. Wallace, M. Tabatabaei, M. S. Zuin, M. S. Workentin and F. Lagugné-Labarthet, Anal. Bioanal. Chem., 2016, 408, 609–618 CrossRef CAS.
- H. Yuan, W. Ji, S. Chu, S. Qian, F. Wang, J.-F. Masson, X. Han and W. Peng, Biosens. Bioelectron., 2018, 117, 637–643 CrossRef CAS.
- S. Srivastava, R. Sinha and D. Roy, Aquat. Toxicol., 2004, 66, 319–329 CrossRef CAS.
- N. Kim, M. R. Thomas, M. S. Bergholt, I. J. Pence, H. Seong, P. Charchar, N. Todorova, A. Nagelkerke, A. Belessiotis-Richards, D. J. Payne, A. Gelmi, I. Yarovsky and M. M. Stevens, Nat. Commun., 2020, 11, 207 CrossRef CAS.
- D. A. Stuart, J. M. Yuen, N. Shah, O. Lyandres, C. R. Yonzon, M. R. Glucksberg, J. T. Walsh and R. P. Van Duyne, Anal. Chem., 2006, 78, 7211–7215 CrossRef CAS.
- Y. Zhang, H. Liu, J. Tang, Z. Li, X. Zhou, R. Zhang, L. Chen, Y. Mao and C. Li, ACS Appl. Mater. Interfaces, 2017, 9, 17769–17776 CrossRef CAS.
- L. A. Lane, R. Xue and S. Nie, Curr. Opin. Chem. Biol., 2018, 45, 95–103 CrossRef CAS.
- H. Kearns, F. Ali, M. A. Bedics, N. C. Shand, K. Faulds, M. R. Detty and D. Graham, R. Soc. Open Sci., 2017, 4, 170422 CrossRef.
- M. Becucci, M. Bracciali, G. Ghini, C. Lofrumento, G. Pietraperzia, M. Ricci, L. Tognaccini, S. Trigari, C. Gellini and A. Feis, Nanoscale, 2018, 10, 9329–9337 RSC.
- A. Sánchez-Solís, F. Karim, M. S. Alam, Q. Zhan, T. López-Luke and C. Zhao, Opt. Lett., 2019, 44, 4997–5000 CrossRef.
- M. A. Bedics, H. Kearns, J. M. Cox, S. Mabbott, F. Ali, N. C. Shand, K. Faulds, J. B. Benedict, D. Graham and M. R. Detty, Chem. Sci., 2015, 6, 2302–2306 RSC.
- K. A. Lynn, G. McNay, D. A. Eustace, N. C. Shand and W. E. Smith, Analyst, 2010, 135, 1904–1905 RSC.
- H. Huang, C. Shende, A. Sengupta, F. Inscore, C. Brouillette, W. Smith and S. Farquharson, J. Raman Spectrosc., 2012, 43, 701–705 CrossRef CAS.
- H. Kearns, M. A. Bedics, N. C. Shand, K. Faulds, M. R. Detty and D. Graham, Analyst, 2016, 141, 5062–5065 RSC.
- J. A. Curcio and C. C. Petty, J. Opt. Soc. Am., 1951, 41, 302–304 CrossRef CAS.
- H. Fleming, S. McAughtrie, B. Mills, M. G. Tanner, A. Marks and C. J. Campbell, Analyst, 2018, 143, 5918–5925 RSC.
- Y. Ran, P. Strobbia, V. Cupil-Garcia and T. Vo-Dinh, Sens. Actuators, B, 2019, 287, 95–101 CrossRef CAS.
- J. Cao, D. Zhao and Y. Qin, Talanta, 2019, 194, 895–902 CrossRef CAS.
- Q. Wang and L. Wang, Nanoscale, 2020, 12, 7485–7499 RSC.
- S. Harmsen, S. Rogalla, R. Huang, M. Spaliviero, V. Neuschmelting, Y. Hayakawa, Y. Lee, Y. Tailor, R. Toledo-Crow, J. W. Kang, J. M. Samii, H. Karabeber, R. M. Davis, J. R. White, M. van de Rijn, S. S. Gambhir, C. H. Contag, T. C. Wang and M. F. Kircher, ACS Nano, 2019, 13, 1354–1364 CAS.
- S. E. Bohndiek, A. Wagadarikar, C. L. Zavaleta, D. Van de Sompel, E. Garai, J. V. Jokerst, S. Yazdanfar and S. S. Gambhir, Proc. Natl. Acad. Sci. U. S. A., 2013, 110, 12408–12413 CrossRef CAS.
- E. Garai, S. Sensarn, C. L. Zavaleta, N. O. Loewke, S. Rogalla, M. J. Mandella, S. A. Felt, S. Friedland, J. T. C. Liu, S. S. Gambhir and C. H. Contag, PLoS One, 2015, 10, e0123185 CrossRef.
- D. Choudhury, M. G. Tanner, S. McAughtrie, F. Yu, B. Mills, T. R. Choudhary, S. Seth, T. H. Craven, J. M. Stone, I. K. Mati, C. J. Campbell, M. Bradley, C. K. I. Williams, K. Dhaliwal, T. A. Birks and R. R. Thomson, Biomed. Opt. Express, 2017, 8, 243–259 CrossRef CAS.
- F. Nicolson, L. E. Jamieson, S. Mabbott, K. Plakas, N. C. Shand, M. R. Detty, D. Graham and K. Faulds, Analyst, 2018, 143, 5965–5973 RSC.
- P. Matousek, I. P. Clark, E. R. C. Draper, M. D. Morris, A. E. Goodship, N. Everall, M. Towrie, W. F. Finney and A. W. Parker, Appl. Spectrosc., 2005, 59, 393–400 CrossRef CAS.
- N. A. Macleod and P. Matousek, Appl. Spectrosc., 2008, 62, 291A–304A CrossRef CAS.
- P. Matousek and N. Stone, Chem. Soc. Rev., 2016, 45, 1794–1802 RSC.
- N. Stone, K. Faulds, D. Graham and P. Matousek, Anal. Chem., 2010, 82, 3969–3973 CrossRef CAS.
- P. Dey, W. Olds, I. Blakey, K. J. Thurecht, E. L. Izake and P. M. Fredericks, J. Raman Spectrosc., 2013, 44, 1659–1665 CrossRef CAS.
- F. Nicolson, L. E. Jamieson, S. Mabbott, K. Plakas, N. C. Shand, M. R. Detty, D. Graham and K. Faulds, Analyst, 2018, 143, 5358–5363 RSC.
- F. Nicolson, L. E. Jamieson, S. Mabbott, K. Plakas, N. C. Shand, M. R. Detty, D. Graham and K. Faulds, Chem. Commun., 2018, 54, 8530–8533 RSC.
- N. Stone, M. Kerssens, G. R. Lloyd, K. Faulds, D. Graham and P. Matousek, Chem. Sci., 2011, 2, 776–780 RSC.
- J. M. Yuen, N. C. Shah, J. T. Walsh, M. R. Glucksberg and R. P. Van Duyne, Anal. Chem., 2010, 82, 8382–8385 CrossRef CAS.
- K. Ma, J. M. Yuen, N. C. Shah, J. T. Walsh, M. R. Glucksberg and R. P. Van Duyne, Anal. Chem., 2011, 83, 9146–9152 CrossRef CAS.
- A. S. Moody, P. C. Baghernejad, K. R. Webb and B. Sharma, Anal. Chem., 2017, 89, 5688–5692 CrossRef CAS.
- A. S. Moody, T. D. Payne, B. A. Barth and B. Sharma, Analyst, 2020, 145, 1885–1893 RSC.
- B. Sharma, K. Ma, M. R. Glucksberg and R. P. Van Duyne, J. Am. Chem. Soc., 2013, 135, 17290–17293 CrossRef CAS.
- R. A. Odion, P. Strobbia, B. M. Crawford and T. Vo-Dinh, J. Raman Spectrosc., 2018, 49, 1452–1460 CrossRef CAS.
- F. Nicolson, B. Andreiuk, C. Andreou, H.-T. Hsu, S. Rudder and M. F. Kircher, Theranostics, 2019, 9, 5899–5913 CrossRef CAS.
- S. Lee, H. Chon, S.-Y. Yoon, E. K. Lee, S.-I. Chang, D. W. Lim and J. Choo, Nanoscale, 2012, 4, 124–129 RSC.
- L. Xiao, A. K. Parchur, T. A. Gilbertson and A. Zhou, Anal. Methods, 2018, 10, 22–29 RSC.
- S. Ye, Y. Wu, F. Wan and Y. Li, Chem. Commun., 2019, 55, 9967–9970 RSC.
- V. Amendola, S. Scaramuzza, L. Litti, M. Meneghetti, G. Zuccolotto, A. Rosato, E. Nicolato, P. Marzola, G. Fracasso, C. Anselmi, M. Pinto and M. Colombatti, Small, 2014, 10, 2476–2486 CrossRef CAS.
- J. Reguera, D. Jiménez de Aberasturi, M. Henriksen-Lacey, J. Langer, A. Espinosa, B. Szczupak, C. Wilhelm and L. M. Liz-Marzán, Nanoscale, 2017, 9, 9467–9480 RSC.
- C. D. Ahrberg, J. Wook Choi and B. Geun Chung, Sci. Rep., 2020, 10, 1737 CrossRef CAS.
- D. Yang, G. Liu, H. Li, A. Liu, J. Guo, Y. Shan, Z. Wang and J. He, Analyst, 2020, 145, 1047–1055 RSC.
|
This journal is © The Royal Society of Chemistry 2020 |